Latent constructs of the students' assessment of their learning gains instrument following instruction in stereochemistry†
Received
25th November 2015
, Accepted 16th January 2016
First published on 18th January 2016
Abstract
Pedagogical practitioners who emphasise active learning in undergraduate chemistry courses widely use the Student Assessment of Learning Gains (SALG) instrument to measure students' perceptions of their gains in knowledge and skills in chemistry. Although numerous studies have reported SALG results in support of successful pedagogical interventions, a comprehensive construct-verified version measuring students' perceptions of their chemistry learning is lacking. This paper aims to identify latent constructs of the SALG instrument that was administered in Process Oriented Guided Inquiry Learning (POGIL) classes by using exploratory and confirmatory factor analyses. When the SALG was administered on two separate occasions with two different groups of students following four weeks of instruction on topics in stereochemistry, the results revealed a four-factor structure consisting of 32 items that included Active Learning, Concept Learning, Resources, and Process Skills. These findings demonstrate an approach to collect evidence to support the match between intended constructs and measured variables in light of a targeted pedagogical intervention.
Introduction
There has been an increasing interest in research-based learner-centred teaching approaches aimed at improving students' chemistry learning outcomes. Abundant research literature is available acknowledging the outcomes of the implementation of such practices via student evaluations of faculty and courses (Fairweather, 2008; Weaver et al., 2008; Smith et al., 2009; Danielle and Janice, 2012; Mataka and Kowalske, 2015). These student evaluations are considered helpful to the academic community for a wide variety of purposes such as identifying components of effective teaching and areas of instruction in need of improvement, and also recognising excellence in teaching (Wachtel, 1998). Researchers studying the effectiveness of teaching innovations focus increasingly on the measurement and evaluation of student perceptions of their learning (Arjoon et al., 2013) in conjunction with their cognitive achievement in the disciplinary area (Anaya, 1999; Bowman, 2013). The affective dimensions of pedagogical inventions are generally assessed either through questionnaires or interviews. In questionnaire/interview situations, students are presented with items asking about how each component of the instruction helped their learning and they judge each item using a pre-determined numerical scale or respond to it verbally (Schunk, 1992). However, students vary in their ability to accurately identify the extent to which various learning experiences from a pedagogical intervention positively influenced their learning (Bowman, 2011). Moreover, the nature and quality of learning experiences or learning gains expressed by the students are sensitive to the items of the instruments and their factorial structure (American Psychological Association, 1999). Therefore, it is of prime importance to continue to conduct research on instruments meant for students' self-evaluations mainly to provide some evidence of reliability, validity, and utility. In the case of adapted survey instruments where the researchers or practitioners make changes to its constituent items, the need for the demonstration of evidence of reliability and validity (Malhotra and Grover, 1998) is helpful to maximise the usefulness of the instrument in any study.
The objective of this research was to provide evidence of validity and reliability of the Students' Assessment of Learning Gains (SALG) – an instrument used for students to assess their perceptions of learning in chemistry classes – in the context of teaching and learning stereochemistry. Despite its wide usage, and availability of several versions, only the version of SENCER–SALG (Weston et al., 2006) had been construct validated to reveal its factorial structure. In their report as part of science education for new civic engagement and responsibilities (SENCER) project, Weston et al. hinted on the validation of SALG data although no information was provided on the implied statistical procedures. Furthermore, convergent validity has not been previously determined. Of note is that Moody and Sindre (2003) revealed that SALG was withdrawn from their research study because it lacked evidence for its theoretical construct. The need for the establishment of a statistically-evident factorial structure was of a particular concern in this research because an instrument with evidence of validity provides a more coherent set of measures. With a factorial structure obtained through a systematic statistical technique, the SALG instrument can provide assurance to the administrators that the psychometric inferences from the data of students' self-measures of learning gains will be reliable and valid for the context within which it has been used. Furthermore, the administrators or instructors can use these factor scores as units of analysis in various statistical tests in order to make judgements on the impact of their teaching.
The primary research questions that guided this research were:
(1) Within the context of teaching and learning stereochemistry, using Exploratory Factor Analysis (EFA), what are the latent constructs of the SALG instrument?
(2) Within the context of teaching and learning stereochemistry, does Confirmatory Factor Analysis (CFA) of the SALG instrument identify latent constructs consistent with the exploratory model?
The above research questions were carefully planned as they helped the researchers explore data obtained from a pedagogical intervention known as process oriented guided inquiry learning – POGIL (Moog et al., 2009) in order to provide answers in support of SALG's factor composition and their further verification. An overarching question that is often posed to any administrator of SALG is, “does SALG measure what it is supposed to measure”. Therefore, this question sets an expectation to report factor analysis results. In short, “Factor analysis is intimately involved with questions of validity…. Factor analysis is at the heart of the measurement of psychological constructs” (Nunnally, 1978, pp. 112–113). Consequently, four latent constructs namely active learning, concept learning, resources, and process skills were found to underlie the version of SALG used in this study as shown in Appendix 1 (ESI†).
Background
Developed by Seymour et al. (2000), the SALG instrument garners information related to the content, pedagogical approach, learning activities, grading and assessment procedures, resources, and student engagement in terms of workload and pace of learning. The SALG instrument is usually used at the end of the semester to measure the students' self-perception of their learning gains and their progress towards course learning gains. Instructors also may use the instrument halfway through the course to enable them make informed course corrections. Additionally, instructors also use a baseline or introductory survey to sense the position of the students with respect to the desired learning goals.
Furthermore, the SALG data collected during pre-course and post-course evaluations are considered helpful in providing a snapshot (Middlecamp et al., 2006) of students' skills and attitudes before and after an intervention. The SALG instrument and various other traditional end-of-course questionnaires differ from each other in that the former primarily focuses on students' self-reporting of their learning gains in the specific activities or course elements, whereas the latter provides students with the opportunity to rate the instructors' teaching competencies, practices and resources.
The SALG instrument has been used in a number of studies related to the student learning of university level chemistry that primarily focused on active learning and student-centred pedagogies. Seymour (2002) administered paper–pencil and online SALG instruments in a multi-institutional study both at the end and mid-points of the semester to measure the students' perception of learning. Middlecamp et al. (2006) developed and used online SENCER–SALG as a tool for assessing how SENCER courses were successfully influencing student learning. Hopkins and Samide (2013) used SALG in their inquiry-based laboratory curriculum to teach general chemistry. The students reported their scores on their pre-class knowledge and subsequent gains in knowledge after the inquiry-based laboratory instruction. SALG was also used as a post-course survey of the inquiry-based instruction by Prescott (2013) in a general chemistry course for non-majors. Similarly, Walker and Sampson (2013) used the SALG survey to determine how students viewed the argument-driven inquiry instruction in the chemistry laboratory.
The SALG instrument is used to gauge students' perceptions of skills, understanding, and attitudes towards teaching or laboratory courses (Seymour, 2002; van Rooij, 2009; Yadav et al., 2011; Herreid and Schiller, 2013). Carroll (2010) inferred that a combination of SALG and student achievement tests could offer curriculum practitioners a powerful triangulation on measures and causes of student learning. Straumanis and Simons (2008) used SALG as an indicator of growth of students' process skills in non-didactic organic chemistry classes and reported that non-didactic responses were higher than those in the control didactic group. According to Seymour et al. (2000), SALG provides average scores and standard deviations for responses to each statement and requests that students include written explanations for their responses to each main question.
Descriptive statistical analysis and response frequencies of SALG are widely used to interpret students' responses (Keeves, 1995; Heady, 2002; Keeney-Kennicutt et al., 2008; Douglas and Chiu, 2009; van Rooij, 2009; Johnson et al., 2011) to each or a set of the Likert scale questions in an effort to provide a glimpse of students' perception of course implementation. Heady (2002) administered the SALG survey successively to two student cohorts over two years in introductory biology classes to find out what helps students to learn. The study compared the mean values for all of the student responses to the items of SALG. Johnson et al. (2011) had used an on-line SALG survey containing 9 measurement domains to explore the virtual learning environment in nursing education. Their SALG instrument contained 4-point Likert type items organised as domains but information in support of domain composition was not available. In another study on the effectiveness of project management methodology in a psychology class, van Rooij (2009) administered a 20-item SALG survey and presented comparative mean scores of students' SALG responses in project management methodology and traditional project scaffolding. Keeney-Kennicutt et al. (2008) used a web-based SALG instrument to investigate general chemistry students' perceptions of an educational web-based tool called, calibrated peer review. The results of the trend analysis included the percentage values of students' responses to the 5-item SALG survey. A review of the above studies revealed researchers' attempts to establish the validity and reliability of SALG data by comparing: (i) student's SALG responses with their interview data; (ii) mean values and other measures of learning like achievement. Comparing mean values from SALG with other measures of learning may not provide greater correspondence because students' perceptions and achievement may not be the same (Poe, 1969).
Theoretical background on the SALG survey
The original SALG had no theoretical evidence in support of its design and also subsequent users were less vigilant about the important aspect of theory that guided the design of the instrument. Based on the nature of the items used, it appears that the instrument may have been informed by the sociological theory of Merton (1968). Merton inferred that empirical uniformities can be derived from logically interconnected propositions and attributed manifest and latent functions to social processes. Accordingly, Seymour et al. (1997) identified two characteristic features from effective teachers Angelo and Cross (1993) that were found to be relevant for the SALG instrument: (1) regular evaluation of teaching practice in the form of assessment and feedback to understand whether such practices are beneficial to students' learning, and (2) familiarity of students' academic preparation, knowledge, and abilities and fine-tuning teaching strategies to enhance students' learning gains. The SALG instrument also reflects the characteristics recommended by Kuh (2001) for student self-assessment surveys. These characteristics include students' awareness of the information they are asked for, precision and clarity of the questions, and question items focusing on meaningful activities that could evoke thoughtful responses from the students. According to Kuh, the information, ideas and the language presented in the instruments are relevant to the learning context of the students. According to Seymour et al. (2000), the flexibility of adapting SALG in between different disciplines of science is dependent on the extent of cohesiveness of various course elements such as goals of class or laboratory activities, curriculum, resources used and tested.
Construct validity
At present, there is no evidence in support of construct validity for any of the adapted versions of SALG used in active learning pedagogical implementations. Construct validity indicates whether or not the instrument actually measures the construct under investigation (Coll et al., 2002) and it is inclined to the nature of items in the modified SALG. Cronbach and Meehl (1955) warranted that construct validation is to be identified by the orientation of the investigator rather than by a specific investigatory approach. The construct validation procedures are theoretically based and include establishing the convergent validity and discriminant validity of the measure (Agarwal, 2013). Factorial analysis is often used to assess construct validity and is often achieved by including information from the items (observed variables) of the instrument in as few derived factors as possible to keep the solution understandable (Gorsuch, 1983). Convergent validity is established when the variables that tap the same construct are correlated with each other, whereas discriminant validity is established when variables that tap different constructs are not correlated with each other (Hsiao et al., 2014).
Design and procedures
The research reported in this article was part of a major research project approved by the Human Research Ethics Committee (HREC) of the investigators' university that focused students' perceptions of learning chemistry in a student-centred intervention like POGIL. A short description of POGIL philosophy is included in the following section. The research design for the major study consisted of a post-positivist paradigm using a quasi-experimental design (Creswell, 2003) with quantitative data collected using SALG. Post-positivist research is commonly aligned with quantitative methods of data collection and analysis. Post-positivist paradigm emphasises well-defined concepts and variables, controlled conditions, precise instrumentation and empirical testing (Weaver and Olson, 2006). Post-positivism was considered appropriate for this study as it offered the researchers an impersonal position to make context-dependent generalisations (Cooper, 1997) using methods that minimise the susceptibility of participants. Furthermore, the scope of this article restricted the authors to avail only quantitative data.
Pedagogical context
The POGIL teaching–learning method has shown to be effective in chemistry major courses at several institutions in the United States. More recently, in Australia, Active Learning in University Science (ALIUS), a collaborative project of six Australian universities, uses POGIL as a model of teaching innovation to engage students in large first year chemistry classes (Bedgood Jr. et al., 2009). Consequently, the research study was undertaken at a large tertiary institution in Australia where POGIL has been actively practiced in selected first year chemistry courses.
POGIL is a student-centred instructional approach where students work in small groups with the instructor acting as a facilitator. In a POGIL classroom, students work in learning teams using specially designed activities that promote mastery of the discipline content and the development of skills necessary for scientific inquiry. POGIL practitioners have used SALG and published their results of student engagement, their perceptions of the value of small group learning and the perceived growth in process skills. The major characteristic features of the POGIL model are concept learning, development of process skills, active engagement and use of resources or activities. Henceforth, it is desirable to identify the comprehensive measurement scales for the SALG instrument in order to make it more reflective of the characteristics of the pedagogical approach for which it is used.
Disciplinary context
Stereochemistry is an important aspect of organic chemistry that primarily includes the study of relative spatial arrangement of atoms within molecules and the study of stereochemical requirements and outcomes of chemical reactions. Topics in Stereochemistry, taught as part of a chemistry course to first year students, included: chirality, stereocenter, stereoisomers, molecular orientation at stereo-carbons, and identifying chiral molecules on the basis of the plane of symmetry, non-superimposable mirror image formation, and the ability to estimate the possible number of stereoisomers from a stereocenter of the molecule, distinguishing isomers, SN1 and SN2 reactions, curved arrow processes, and nucleophilic substitution reactions. A modified POGIL approach in the form of embedded mini-lectures, small group POGIL discussions, followed by clicker questions was utilised.
Sample
The sample comprised a cohort of first year chemistry students enrolled during 2011 and 2012, referred to as Group 1 (n = 114) and Group 2 (n = 154), respectively. Most of the students were in Engineering, Science, and Pharmacy programmes opting to study chemistry during first and second semesters. The majority of the students (domestic and international) were school leavers, however, non-traditional students included mature age learners and students with vocational qualifications comprised a minority of the population. Students' participation in the study was voluntary and only self-selected students were invited to complete the paper–pencil SALG during chemistry workshops/tutorial sessions. The topic being studied, over a period of four weeks, was stereochemistry.
The SALG instrument
The paper–pencil SALG instrument was administered to Groups 1 and 2 in 2011 and in 2012 to obtain data for the exploratory and confirmatory analyses, respectively. The SALG instrument, comprising 62, 5-point Likert scale items, was administered during the end of the second semester 2011 for exploratory factor analysis (n = 114). Based on the results, the instrument was refined and the 44 item 5-point Likert scale SALG instrument was administered to Group 2 students during the end of the second semester of 2012 for confirmatory factor analysis (n = 154). The outline of the development and validation of the SALG instrument is shown in Fig. 1. In addition to the Likert scale items, SALG also included items that were aimed at seeking students' written responses on various aspects of the POGIL class (but not reported in this article).
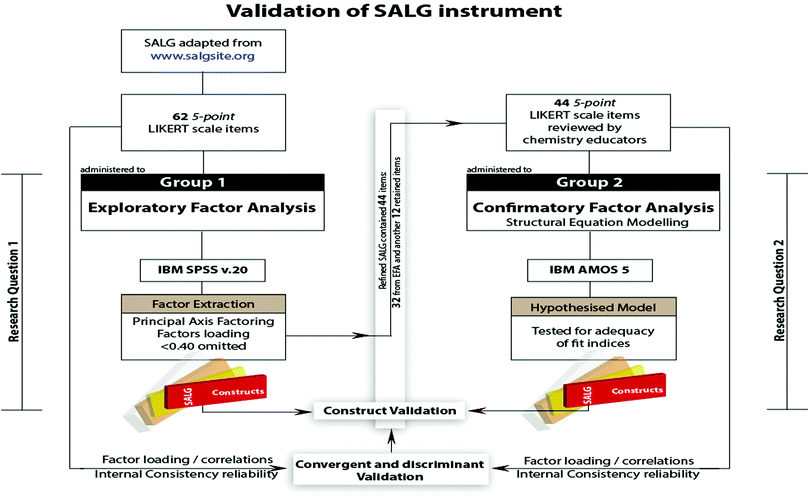 |
| Fig. 1 The process for collecting evidence for determining the SALG Instrument validity from administration in this study. | |
For establishing the convergent validity of SALG, the factor loadings and internal consistency reliability measures were computed for the 2011 data. Brown (2006) suggested a strong interrelation of different measures of theoretically similar or overlapping constructs for convergent validity. The explored model was then fitted to the 2012 data and fit statistics examined with reference to established criteria (Hu and Bentler, 1999).
Analysis and findings
The most commonly used procedure for psychometric evaluation of questionnaires is factor analysis which can be performed in two ways: exploratory factor analysis (EFA) and confirmatory factor analysis (CFA). In EFA, researchers follow data reduction procedures (reducing a large set of variables to a manageable number) and further explore the data for the appropriate number of common factors that can reasonably serve as indicators of a set of measured variables; in CFA, a pre-specified factor solution is evaluated (Brown, 2006).
Construct validity: exploratory factor analysis (EFA)
Exploratory factor analysis (EFA) is generally employed in the process of scale development and construct validation (Brown, 2006) and is a data-driven approach to see the relevant common factors emerging from it (Johnson and Stevens, 2001) and also investigate the relationship between manifest variables and factors (Everitt and Hothorn, 2011).
The purpose of EFA in this study involved the first research question to investigate the latent constructs encompassing the SALG instrument. Subsequently, the EFA, performed on all 62 items of SALG, used a principal axis factoring analysis with the varimax rotation procedure using SPSS version 20 to extract four sets of factors from a total of 32 items. Appendix 2 (ESI†) summarizes the results of EFA carried out in this study. Varimax rotation was chosen due to its easiness to interpret the factors as it maximises variance between factors (Foster et al., 2006). The feasibility of factor analysis was determined by examining the Kaiser–Meyer–Olkin (KMO) measure of sampling and Bartlett's test of sphericity. The KMO measure of sampling adequacy was 0.785, indicating that the data were appropriate for exploratory factor analysis (Tabachnick and Fidel, 1989). The acceptable limit of the KMO measure is 0.50 (Kaiser, 1974). Bartlett's test of sphericity indicated that χ2 = 2196.521 which was statistically significant (p < 0.001) indicating that the correlation matrix is significantly different from an identity matrix. the identity matrix is usually obtained in situations where there is no existence of correlation between any of the variables. Items loading on more than one factor with a loading score of equal to or less than 0.40 on each factor were eliminated from the analysis to indicate sufficient loading (Hinkin, 1998). Table 1 shows the results of the varimax rotation and the factors obtained after EFA.
Table 1 Factor loading, eigenvalue and percentage of variance for the SALG (Group 1, 2011) (n = 114)
Item number |
Factor loadings |
Active learning |
Concept learning |
Resources |
Process skills |
Extraction method: principal axis factoring. Rotation method: varimax with Kaiser normalization. |
1 |
0.45 |
|
|
|
2 |
0.55 |
|
|
|
3 |
0.60 |
|
|
|
4 |
0.42 |
|
|
|
5 |
0.48 |
|
|
|
6 |
0.52 |
|
|
|
7 |
0.64 |
|
|
|
8 |
0.61 |
|
|
|
9 |
0.64 |
|
|
|
10 |
0.49 |
|
|
|
11 |
0.62 |
|
|
|
12 |
0.71 |
|
|
|
13 |
0.41 |
|
|
|
14 |
0.52 |
|
|
|
15 |
0.55 |
|
|
|
16 |
0.61 |
|
|
|
17 |
0.56 |
|
|
|
18 |
0.61 |
|
|
|
19 |
|
0.55 |
|
|
20 |
|
0.52 |
|
|
21 |
|
0.77 |
|
|
22 |
|
0.77 |
|
|
23 |
|
0.72 |
|
|
24 |
|
0.58 |
|
|
25 |
|
0.56 |
|
|
26 |
|
|
0.80 |
|
27 |
|
|
0.87 |
|
28 |
|
|
0.89 |
|
29 |
|
|
0.46 |
|
30 |
|
|
|
0.89 |
31 |
|
|
|
0.68 |
32 |
|
|
|
0.80 |
|
% Variance |
18.12 |
10.69 |
9.33 |
7.65 |
Eigenvalue |
9.46 |
2.86 |
2.64 |
1.90 |
Cumulative % |
18.12 |
28.82 |
38.15 |
45.79 |
Variance |
|
|
|
|
Following the exploratory factor analysis, factor loadings indicate how strongly each item was related to a particular factor. Eigenvalues showed the relative importance of each factor, and the cumulative variance was used to check whether a sufficient number of factors have been retained. The eigenvalue for each factor was greater than 1, as per Kaiser criterion (Kaiser, 1960) and the cumulative variance for all the four factors was 45.79%. This showed that four factors can explain over 45 per cent of the total variability in the 32 items. After consideration of the intent of the items clustered on each factor, the derived four factors were labelled as Active Learning (18 items), Concept Learning (7 items), Resources (4 items) and Process Skills (3 items). Representative items of each factor are listed in Table 2. The Cronbach alpha reliability coefficients, shown in Table 4, are highly satisfactory (Arjoon et al., 2013) being greater than 0.7.
Table 2 A sample of representative items in each latent factor
Factor |
Item: as a result of your work in this class, what gains did you make in the following |
Active learning |
Participating in group work |
Listening to discussions |
Concept learning |
Understanding and classifying chiral–achiral molecules |
Understanding and distinguishing isomers |
Resources |
Mini-lectures helped my learning |
Clicker questions helped my learning |
Process skills |
Skill of argumental use of evidence |
Skill of identifying data pattern |
Construct validity: confirmatory factor analysis (CFA)
Confirmatory factor analysis (CFA) was used to determine the construct validity, namely, whether the factor structure resulting from the exploratory factor analysis (EFA) could be consistent with the data obtained from another similar group – in this case the Group 2 cohort during semester 2 in 2012 when the refined SALG was used. The refined SALG contained 44 items, of which 32 were from the four-factored EFA and another 12 that were retained because of their relevancy to the intervention was completed by 154 students. An outline on the development and administration of the SALG instrument is presented in the right-hand side of Fig. 1.
Subsequently, CFA was conducted using a Structural Equation Modelling (SEM) approach to test the four factor model derived from the EFA (Fig. 2). Unlike other statistical procedures meant for establishing construct validity of factors, SEM offers the researcher the ability to use multiple measures to represent constructs and test their relationship with other constructs addressing the issue of specific errors of measurement (Weston and Gore, 2006). All SEM measurement models are tested against a host of fit indices to evaluate their representation of the relationship among constructs and observed variables. For this study, the four factor model of EFA was applied to Group 2 data (n = 154) in an effort to answer the second research question. From the proposed four factor model, using AMOS v20 software, a χ2 = 619.406, df = 385, and p < 0.001 were obtained indicating that the model can be estimated and tested. The proposed four factor measurement model was identified fulfilling the two recommended general requirements (Hu and Bentler, 1999); first, the number of pieces of information in the model shall be at least as large as the number of parameters to be estimated. The four factor model contained 80 distinct parameters to be estimated and 465 distinct pieces of information, thereby meeting the first requirement. The second requirement: every latent variable including the residual terms must be assigned a scale; all the latent variables in the model and the errors terms have a scale assigned to each one of them. Though the χ2 statistics obtained from the four factor model appeared significant, finding an exact fit to the data is rare (Weston and Gore, 2006). Owing to the limitations of χ2 statistics, this statistics is not used as the sole index of overall model fit (Brown, 2006). Therefore, other fit indices were explored to determine whether the model fit is acceptable. Furthermore the fit indices χ2/df = 1.60, CFI = 0.92, RMSEA = 0.06, TLI = 0.91, and SRMR = 0.07 appeared to have met the adequacy criteria of goodness-of-fit. Appendix 3 (ESI†) summarizes the results of CFA carried out in this study. Hu and Bentler (1999) suggested the following cut-off limits for achieving a reasonably good fit between the target model and the observed data: (1) CFI values greater than 0.90; (2) RMSEA values close to 0.06 or lower; (3) TLI values close to 0.95 or lower; and (4) SRMR values close to 0.80 or lower.
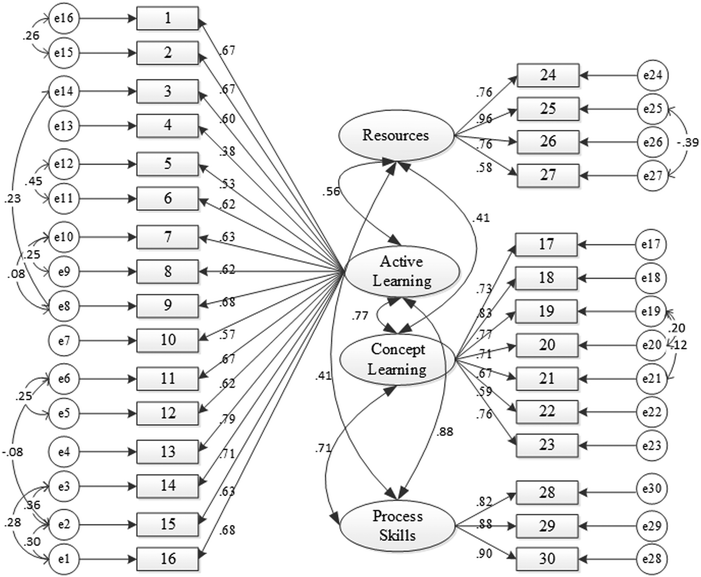 |
| Fig. 2 The four factor measurement model of SALG. | |
For improving the model fit, the model modification indices were used. Two of the active learning items (15 and 18), ‘grading system what I need to work’ and ‘Willingness to seek help from others (instructor, peers, tutor) when working on academic problems’ did not fit the CFA model. Items 15 and 18 are yet useful (if retained) to the researchers/instructors in order to capture students' perceptions of their learning in POGIL because these items inquire the usefulness of seeking help from instructors/tutors/peers and their understanding of the grading system used in workshops. Similarly, the items numbered 24, 25 and 29 also were not included in the CFA measurement model due to the fact that, the planning of analysis is driven principally by theoretical relationships of observed (items) and unobserved variables (latent constructs) (Schreiber et al., 2006). Furthermore the use of correlated measurement errors is allowed when such practices are theoretically or methodologically justifiable (Shah and Goldstein, 2006). As an example of a substantive evidence for modifications in the model, items 14 and 16 convey nearly the same and serve as mutual controls on the consistency of answer, hence they were correlated. A similar example existed for items 3 and 9. The items of the four factor measurement model – for active learning, resources and process skills are relevant to all the teachings of chemistry by a student-centred approach – are presented in Table 3. The concept learning in stereochemistry is specific to the study. When using a different topic, the concept learning outcomes need to be changed accordingly.
Table 3 Items of the four factor measurement model
Item number CFA |
Item number EFA |
Item |
Active learning |
1 |
2 |
Attending class |
2 |
1 |
Pace of class |
3 |
3 |
Working with peers |
4 |
4 |
Working with peers outside the class |
5 |
5 |
Explanation of the instructor for involving small groups |
6 |
6 |
Explanation of focus on topics presented |
7 |
10 |
Participating in class discussions |
8 |
11 |
Listening to discussions |
9 |
12 |
Participating in group work |
10 |
13 |
Class activities help learning |
11 |
14 |
Number and spacing of tests |
12 |
16 |
Feedback on my work received during and after tutorials |
13 |
17 |
Connecting key ideas to other knowledge |
14 |
7 |
Confidence that you understand the material |
15 |
8 |
Confidence in the ability to do POGIL activities |
16 |
9 |
Comfort level involving complex ideas |
|
Concept learning |
17 |
20 |
SN1 and SN2 reaction mechanisms |
18 |
21 |
Distinguishing different types of isomers |
19 |
22 |
Classifying chiral–achiral molecules |
20 |
23 |
Identifying stereocentres in molecules |
21 |
19 |
Attractive forces between molecules and their effect on physical properties |
22 |
— |
Applying curved arrow conventions to describe bond forming and bond breaking processes |
23 |
— |
The reactions of alkyl halides, nucleophilic substitution reactions |
|
Resources |
24 |
26 |
Mini lectures |
25 |
27 |
Posted pencasts |
26 |
28 |
Pencasts solutions of homework problems |
27 |
— |
Clickers |
|
Process skills |
28 |
31 |
Identifying patterns in data |
29 |
30 |
Recognising a sound argument and the appropriate use of evidence |
30 |
32 |
Develop logical argument |
Convergent validity
Convergent validity was assessed by examining the inter-correlations of the constructs of SALG. As shown in Table 7, the correlations among the four constructs were found to be statistically significant.
Internal consistency reliability was established by calculating the Cronbach's alpha coefficient for each factor. The guidelines (Nunnally, 1978; Cohen et al., 2000) indicate that an alpha coefficient of 0.70 is adequate for an instrument in the early stage of development; a coefficient of at least 0.80 is adequate for a more developed instrument. The results portrayed in Table 4 show that the Cronbach's alpha coefficient for each factor was above 0.80, affirming the reliability of the scales of SALG. Based on the analysis of the data, the factor loadings and internal consistency measure confirmed the convergent validity of the SALG questionnaire used in this study.
Table 4 Internal consistency reliability (Cronbach's alpha) for the SALG scales
Factor |
Number of items |
Cronbach's alpha |
Active learning |
18 |
0.90 |
Concept learning |
7 |
0.84 |
Resources |
4 |
0.81 |
Process skills |
3 |
0.89 |
The Cronbach alpha internal consistency reliability of the items of SALG after CFA was calculated and the values are presented in Table 5. These values are highly satisfactory with similar values to those presented in Table 4.
Table 5 Internal consistency reliability of SALG scales after CFA using EFA scales
Factor |
Number of items |
Cronbach's alpha |
Active learning |
16 |
0.92 |
Concept learning |
7 |
0.89 |
Resources |
4 |
0.82 |
Process skills |
3 |
0.90 |
This four factor CFA – SEM analysis would appear to demonstrate Brown's (2006) criteria for convergent validity with strong interrelation of different measures of theoretically similar or overlapping latent constructs (see Table 6).
Table 6 Pearson correlation coefficient values of four factors of the SALG instrument
|
Active learning |
Concept learning |
Resources |
Process skills |
p < 0.01 level (2-tailed). |
Active learning |
|
0.69 |
0.54 |
0.77 |
Concept learning |
|
|
0.42 |
0.66 |
Resources |
|
|
|
0.41 |
Process skills |
|
|
|
|
Discriminant validity
Discriminant validity according to Brown (2006) is expressed by results showing that indicators of theoretically distinct constructs are not highly inter-correlated. He further argued that factor correlations above 0.80 imply the overlap of items and point towards poor discrimination validity. The discriminant validity of the items of the instrument was assessed by comparing the construct correlations with the square root of the average variance extracted (AVE). Fornell and Larcker (1981) specify that discriminant validity is achieved when the square root of the AVE of a construct is larger than its correlation with other constructs. The square roots of the AVE were calculated and are represented in bold on the main diagonal of Table 7. The off diagonal elements represent the correlations among the latent variables. The results reported in Table 7 confirm that the discrimination validity was achieved by all scales. As shown in Table 7, the correlations ranged from 0.17 to 0.51, providing further evidence in support of the discriminant validity.
Table 7 Inter construct correlations and square roots of average variance extracted for the SALG scales
|
Active learning |
Concept learning |
Resources |
Process skills |
p < 0.01 level (2-tailed). Note: square root of average variance extracted (AVE) is shown on the diagonal of the matrix. |
Active learning |
0.78
|
|
|
|
Concept learning |
0.45 |
0.82
|
|
|
Resources |
0.31 |
0.17 |
0.89
|
|
Process skills |
0.51 |
0.41 |
0.35 |
0.94
|
Discussion and conclusion
Based on the responses following instruction in stereochemistry, four factors containing 32 items were extracted from the SALG instrument during EFA. Appendix 1 (ESI†) provides a complete list of factors and their corresponding items. The factor analysis of the data obtained from 114 students from Group 1 resulted in a four factorial structure of the SALG instrument; active learning, concept learning, resources, and process skills. Since the study had occurred at only one institution, it was always difficult to acquire the desired sample size. However, considering the criteria of the variable to sample ratio (Bentler and Chou, 1987; Conway and Huffcutt, 2003), the sample for EFA (n = 114) had a ratio less than 5
:
1 and the sample for CFA (n = 154) had a ratio greater than 5
:
1. The internal consistency reliability was highly satisfactory where each factor had a Cronbach's alpha coefficient value greater than 0.80. For CFA, the explored four factor model was fitted to the data obtained from Group 2, 2012 (n = 154) using a measurement model of structural equation modelling (SEM); the fit statistics met the criteria of a good fit. The Cronbach's alpha internal consistency reliability values of the SALG constructs after CFA were highly satisfactory (>0.80). These findings give support to Hong et al. (2011) suggestion that, for adapted instruments, the CFA is used to test the fit of the factor structure from a sample different to the EFA.
The findings from the sophisticated use of EFA and CFA indicate that the SALG questionnaire has high convergent and discriminant validity when used with these first year chemistry classes learning stereochemistry. Therefore, data collected using this survey are likely to be valid and reliable in this study context. Although the results of this study need to be replicated with large samples across a range of chemistry units (by substituting items under subscale concept learning), and in different institutions, the four-factor model may provide POGIL practitioners with a useful approach to predict students' acceptance of the intervention when implemented in different cultural contexts. The causal relationships between the four subscales of SALG, when explored further, may provide opportunities for meaningful evaluation of students' perceptions of their learning gains in research-based student-centred pedagogies.
The data utilised in this study identified a fit between latent constructs and observed variables that are relevant to POGIL instruction. For example, the active learning construct obtained after EFA contained 18 items (see Appendix 1, ESI†) that are distinctly relevant to various elements of pedagogical intervention followed in this study. Similarly, the 8 items under concept learning were appropriate and broadly covered the disciplinary context.
According to Brown (2006), there are two categories of factors – overdetermined, and underdetermined, based on the number of strongly related indicators (items) in them. Conspicuously in this study, Active Learning, an overdetermined factor (factor with several indicators), and Process Skills, an underdetermined factor (factor with two or three indicators), have emerged. Though they have theoretical relevance to the pedagogical intervention that the study had followed, it becomes more practical for the purpose of interpretability if no such skewed breakdown of factors occurred. A large sample replication study is recommended to overcome to further ensure the recoverability of the proposed model.
Limitations
The SALG instrument has shown both good reliability and validity for measuring students' perceptions of their learning in active learning classrooms; in this case in teaching and learning the topic of stereochemistry using a modified POGIL approach. However, despite its rigor and depth to the interpretation of results, the research based on self-report data has potential for continuous errors in self-assessments to confound the results (Dunning et al., 2004; Beghetto, 2007). Despite its flexibility for adoption or adaptation, the various versions of SALG need to be consistently verified for underlying constructs in order to enhance their interpretability of the generated data. The data sets used for EFA and CFA emerged from a relatively smaller sample size which may lead to unstable solutions. Smaller samples increase the likelihood of obtaining underdetermined factors. The original SALG developed by Seymour et al. (2000) had 5 Likert scale points. However, chemistry educators who have successively used SALG contained 6 Likert scale points. Considering the variation in the number of Likert scale points used, the internal consistency reliability of the SALG instrument may (Chang, 1994) or may not (Cummins and Gullone, 2000) vary. The instrument could be enriched by reducing the number of items on the first factor active learning or alternatively creating a new scale comfort and confidence using items 12 to 16 to avoid overemphasis on modification indices while attempting to improve the model fit. The findings from this study may not be generalizable to other contexts due to the fact that SALG is flexible in terms of its constituent items thus making this outcome more context-dependent. Depending on the conceptual area taught, the items will change as required. This study specifically deals with the teaching and learning of stereochemistry. The scope of the article restricted the authors to the measurement model only, hence the fit of structural models to the observed data is not discussed. Above all, a considerable theoretical and statistical sophistication is required for the pedagogical practitioners intending to evaluate the impact of their implementations.
Research ethics
This research has been reviewed and given approval by the institution's Human Research Ethics Committee (Approval number SMEC-45-10).
Acknowledgements
We thank the students for their participation in this study and the Department of Chemistry for allowing the researchers to collect the data.
References
- Agarwal V., (2013), Investigating the convergent validity of organizational trust, J. Commun. Manag., 17(1), 24–39.
- American Psychological Association., (1999), Standards for Educational and Psychological Testing, Washington, DC: American Psychological Association.
- Anaya G., (1999), College impact on student learning: Comparing the use of self-reported gains, standardized test scores, and college grades, Res. High. Educ., 40(5), 499–526.
- Angelo T. A. and Cross K. P., (1993), Classroom assessment techniques: a handbook for college teachers, San Francisco, CA: Jossey-Bass.
- Arjoon J. A., Xu X. and Lewis J. E., (2013), Understanding the state of the art for measurement in chemistry education research: examining the psychometric evidence, J. Chem. Educ., 90(5), 536–545.
- Bedgood Jr D. R., Yates B., Buntine M., Pyke S., Lim K., Mocerino M., Zadnik M., Southam D., Bridgeman A., Gardiner M. and Morris, G., (2009), ALIUS: active learning in university science: leading change in Australian science teaching, Chem. Aust., 77(5), 18–19.
- Beghetto R. A., (2007), Factors associated with middle and secondary students' perceived science competence, J. Res. Sci. Teach., 44(6), 800–814.
- Bentler P. M. and Chou C.-P., (1987), Practical issues in structural modeling, Sociol. Methods Res., 16(1), 78–117.
- Bowman N. A., (2011), Can college students accurately assess what affects their learning and development? J. Coll. Stud. Dev., 52(3), 270–290.
- Bowman N. A., (2013), Understanding and addressing the challenges of assessing college student growth in student affairs, Res. Pract. Assess., 8, 1–14.
- Brown T. A., (2006), Confrimatory factor analysis for applied research, New York: The Guildford Press.
- Carroll S. B., (2010), in Sheardy R. D. (ed.), Science education and civic engagement: the SENCER approach, Oxford: Oxford University Press, ch. 10, vol. 1037, pp. 149–198.
- Chang L., (1994), A psychometric evaluation of 4-point and 6-point Likert-type scales in relation to reliability and validity, Appl. Psychol. Meas., 18(3), 205–215, DOI: 10.1177/014662169401800302.
- Cohen L., Mannion L. and Morrison K., (2000), Research methods in education. New York: RoutledgeFalmer.
- Coll R., Dalgety J. and Salter D., (2002), The development of the chemistry attitudes and experiences questionnaire (CAEQ), Chem. Educ. Res. Pract., 3(1), 19–32, DOI: 10.1039/B1RP90038B.
- Conway J. M. and Huffcutt A. I., (2003), A review and evaluation of exploratory factor analysis practices in organizational research, Organ. Res. Methods, 6(2), 147–168.
- Cooper M. M., (1997), Distinguishing critical and post-positivist research, Coll. Compos. Commun., 48(4), 556–561.
- Creswell J. W., (2003), Research design, qualitative, quantitative, and mixed methods approaches, Thousand Oaks, CA: Sage Publications, Inc.
- Cronbach L. J. and Meehl P. E., (1955), Construct validity in psychological tests, Psychol. Bull., 52(4), 281–302.
- Cummins R. A. and Gullone E., (2000), Why we should not use 5-point Likert scales: the case for subjective quality of life measurement, Proceedings, Second International Conference on Quality of Life in Cities, Singapore: National University of Singapore, pp. 74–93.
- Danielle K. T. and Janice B., (2012), STEM practice and assessment: SENCER's influence on educators, in Sheardy R. D. and Burns W. D. (ed.), Science Education and Civic Engagement: The Next Level, Washington, DC: American Chemical Society, vol. 1121, pp. 163–178.
- Douglas E. P. and Chiu C. C., (2009), Use of guided inquiry as an active learning technique in engineering, Palm Cove, Qld, Australia.
- Dunning D., Heath C. and Suls J. M., (2004), Flawed self-assessment: implications for health, education, and the workplace, Psychol. Sci. Public Interest, 5(3), 69–106.
- Everitt B. and Hothorn T., (2011), An Introduction to Applied Multivariate Analysis with R, New York: Springer, ch. 5, pp. 135–161.
- Fairweather J., (2008), Linking evidence and promising practices in science, technology, engineering, and mathematics (STEM) undergraduate education, Washington, DC: Board of Science Education, National Research Council, The National Academies.
- Fornell C. and Larcker D. F., (1981), Evaluating structural equation models with unobservable variables and measurement error, J. Mark. Res., 18(1), 39–50.
- Foster J. J., Barkus E. and Yavorsky C., (2006), Understanding and using advanced statistics, Thousand Oaks, CA: Sage Publications.
- Gorsuch R. L., (1983), Factor analysis, 2nd edn, Hillsdale, NJ: Lawrance Erlbaum Associates, Inc.
- Heady J. E., (2002), Innovative Techniques for Large Group Instruction, Arlington, VA: National Science Teachers Association Press, pp. 57–62.
- Herreid C. F. and Schiller N. A., (2013), Case studies and the flipped classroom, J. Coll. Sci. Teach., 42(5), 62–66.
- Hinkin T., (1998), A brief tutorial on the development of measures for use in survey questionnaires, Org. Res. Methods, 1(1), 104–121.
- Hong T., Purzer, S. and Cardella M. E., (2011), A psychometric re-evaluation of the design, engineering and technology (DET) survey, J. Eng. Educ., 100(4), 800–818.
- Hopkins T. A. and Samide M., (2013), Using a thematic laboratory-centered curriculum to teach general chemistry, J. Chem. Educ., 90(9), 1162–1166.
- Hsiao Y.-Y., Wu, C.-H. and Yao, G., (2014), Convergent and discriminant validity of the WHOQOL-BREF using a multitrait-multimethod approach, Soc. Indic. Res., 116(3), 971–988, DOI: 10.1007/s11205-013-0313-z.
- Hu L. and Bentler M., (1999), Cutoff criteria for fit indexes in covariance structure analysis: Conventional criteria versus new alternatives, Struct. Equation Model. Multidiscip. J., 6(1), 1–55.
- Johnson B. and Stevens J. J., (2001), Exploratory and confirmatory factor analysis of the school level environment questionnaire (SLEQ), Learn. Environ. Res., 4, 325–344.
- Johnson C. M., Corazzini, K. N. and Shaw R., (2011), Assessing the feasibility of using virtual environments in distance education, Knowl. Manag. E-Learn. Int. J., 3(1), 5–16.
- Kaiser H. F., (1960), The application of electronic computers to factor analysis, Educ. Psychol. Meas., 20(1), 141–151.
- Kaiser H. F., (1974), An index of factorial simplicity, Psychometrika, 39(1), 31–36.
- Keeney-Kennicutt W. L., Gunersel, A. B. and Simpson N. J., (2008), Overcoming student resistance to a teaching innovation, Int. J. Scholarship. Teach. Learn., 2(1), 1–26.
- Keeves J. P., (1995), The contribution of IEA research to Australian Education, in Bos W., Lehmann R. H., (ed.), Reflections on Educational Achievement, Waxmann: New York.
- Kuh G. D., (2001), The national survey of student engagement: Conceptual framework for overview of psychometric properties, http://nsse.indiana.edu/2004_annual_report/pdf/2004_Conceptual_Framework.pdf, accessed July 2014.
- Malhotra M. K. and Grover, V., (1998), An assessment of survey research in POM: from constructs to theory, J. Oper. Manag., 16(4), 407–425.
- Mataka L. M. and Kowalske M. G., (2015), The influence of PBL on students' self-efficacy beliefs in chemistry, Chem. Educ. Res. Pract., 16(4), 929–938, DOI: 10.1039/C5RP00099H.
- Merton R. K., (1968), Social theory and social structure, New York: Free Press.
- Middlecamp C. H., Jordan T., Shachter A. M., Kashmanian O. K. and Lottridge S., (2006), Chemistry, society, and civic engagement (Part 1): the SENCER project, J. Chem. Educ., 83(9), 1301.
- Moody D. L. and Sindre G., (2003), Evaluating the effectiveness of learning interventions: an information systems case study. Paper presented at the European Conference on Information Systems, Naples, Italy.
- Moog R. S., Creegan J. F., Hanson M. D., Spencer N. J., Straumanis A. and Bunce M. D., (2009), POGIL: Process-oriented guided-inquiry learning, in Pienta N., Cooper M. M. and Greenbowe T. J. (ed.), Chemists' Guide To Effective Teaching, Upper Saddle River, NJ: Prentice Hall, vol. 2, pp. 90–101.
- Nunnally J. C., (1978), Psychometric Theory, 2nd edn, New York: McGraw-Hill.
- Poe C. A., (1969), Convergent and discriminant validation of measures of personal needs, J. Educ. Meas., 6(2), 103–107, DOI: 10.2307/1434286.
- Prescott S., (2013), Green goggles: designing and teaching a general chemistry course to nonmajors using a green chemistry approach, J. Chem. Educ., 90(4), 423–428.
- Schreiber J. B., Nora A., Stage F. K., Barlow E. A. and King, J., (2006), Reporting structural equation modeling and confirmatory factor analysis results: a review, J. Educ. Res., 99(6), 323–338.
- Schunk D. H., (1992), Theory and research on student perceptions in the classroom, in Schunk D. H. and Meece J. L. (ed.), Student perceptions in the classroom, Mahwah, NJ: Lawrence Erlbaum Associates, Inc, pp. 3–24.
- Seymour E., (2002), Tracking the processes of change in US undergraduate education in science, mathematics, engineering, and technology, Sci. Ed., 86(1), 79–105.
- Seymour E., Wiese D., Hunter A. and Daffinrud S., (1997), Student assessment of learning gains (SALG) CAT, http://www.flaguide.org/extra/download/cat/salg/salg.pdf.
- Seymour E., Wiese D., Hunter A. and Daffinrud S. M., (2000), presented in part at the National Meeting of the American Chemical Society, San Francisco, CA.
- Shah R. and Goldstein S. M., (2006), Use of structural equation modeling in operations management research: Looking back and forward, J. Oper. Manag., 24, 148–169.
- Smith K. A. Douglas T. C. and Cox M. F., (2009), Supportive teaching and learning strategies in STEM education, New Dir. Teach. Learn., 117, 19–32.
- Straumanis A. and Simons E. A., (2008), A multi-institutional assessment of the use of POGIL in organic chemistry, in Moog R. S. and Spencer J. N. (ed.), ACS Symposium Series 994: Process-Oriented Guided Inquiry Learning, Washington, DC: American Chemical Society, pp. 226–239.
- Tabachnick B. and Fidel F., (1989), Using multivariate statistics, New York: Harper Collins Publishers.
- van Rooij S. W., (2009), Scaffolding project-based learning with the project management body of knowledge (PMBOK), Comput. Educ., 52(1), 210–219.
- Wachtel H. K., (1998), Student evaluation of college teaching effectiveness: a brief review, Assess. Eval. High. Educ., 23(2), 191–212.
- Walker J. P. and Sampson V., (2013), Argument-driven inquiry: Using the laboratory to improve undergraduates' science writing skills through meaningful science writing, peer-review, and revision, J. Chem. Educ., 90(10), 1269–1274.
- Weaver K. and Olson J. K., (2006), Understanding paradigms used for nursing research, J. Adv. Nurs., 53(4), 459–469.
- Weaver G. C., Russell, C. B. and Wink, D. J., (2008), Inquiry-based and research-based laboratory pedagogies in undergraduate science, Nat. Chem. Biol., 4(10), 577–580.
- Weston R. and Gore P. A., (2006), A brief guide to structural equation modelling, Couns. Psychol., 34(5), 719–751.
- Weston T., Seymour E. and Thiry H., (2006), Evaluation of Science Education for New Civic Engagements and Responsibilities (SENCER) Project, Washington, DC: The National Center for Science and Civic Engagement.
- Yadav A., Subedi D., Lundeberg M. A. and Bunting, C. F., (2011), Problem-based learning: Influence on students' learning in an electrical engineering course, J. Eng. Educ., 100(2), 253–280.
Footnote |
† Electronic supplementary information (ESI) available: Appendix 1: the SALG instrument. Appendix 2: details of EFA results. Appendix 3: details of CFA results. See DOI: 10.1039/c5rp00214a |
|
This journal is © The Royal Society of Chemistry 2016 |
Click here to see how this site uses Cookies. View our privacy policy here.