DOI:
10.1039/C6AN02109C
(Paper)
Analyst, 2017,
142, 177-185
Importance of specimen pretreatment for the low-level detection of mycobacterial lipoarabinomannan in human serum†
Received
20th September 2016
, Accepted 26th November 2016
First published on 28th November 2016
Abstract
Patient care and prevention of disease outbreaks rely heavily on the performance of diagnostic tests. These tests are typically carried out in serum, urine, and other complex sample matrices, but are often plagued by a number of matrix effects such as nonspecific adsorption and complexation with circulating proteins. This paper demonstrates the importance of sample pretreatment to overcome matrix effects, enabling the low-level detection of a disease marker for tuberculosis (TB). The impact of pretreatment is illustrated by detecting a cell wall component unique to mycobacteria, lipoarabinomannan (LAM). LAM is a major virulence factor in the infectious pathology of Mycobacterium tuberculosis (Mtb) and has been successfully detected in the body fluids of TB-infected individuals; however, its clinical sensitivity – identifying patients with active infection – remains problematic. This and the companion paper show that the detection of LAM in an immunoassay is plagued by its complexation with proteins and other components in serum. Herein, we present the procedures and results from an investigation of several different pretreatment schemes designed to disrupt complexation and thereby improve detection. These sample pretreatment studies, aimed at determining the optimal conditions for complex disruption, were carried out by using a LAM simulant derived from the nonpathogenic M. smegmatis, a mycobacterium often used as a model for Mtb. We have found that a perchloric acid-based pretreatment step improves the ability to detect this simulant by ∼1500× with respect to that in untreated serum. This paper describes the approach to pretreatment, how pretreatment improves the detection of the LAM simulant in human serum, and the results from a preliminary investigation to identify possible contributors to complexation by fractionating serum according to molecular weight. The companion paper applies this pretreatment approach to assays of TB patient samples.
1. Introduction
Advances in diagnostic tests often require improvements in limits of detection (LoD), quantitative accuracy, and multiplexing capabilities.1–6 The importance of these developments in tuberculosis (TB) diagnostics, the focus of this paper, is but one example where breakthroughs can have a major impact on global health care.7–9 As shown herein, a key obstacle faced in TB tests is the inability of immunoassays to detect a potential marker of this disease due to the matrix effects caused by constituents in serum and other body fluids. This situation necessitates the development of a sample pretreatment approach that can counter these effects and enhance detection.6,10 Sample pretreatment is commonly used in chemical analysis to mitigate matrix effects by removing or masking interferences.11 In immunoassays, sample pretreatment is also used as a means to combat cross reactions, nonspecific adsorption, immunocomplexation, and other matrix effects.6,10 This paper and the accompanying companion paper12 detail the ability to detect an antigenic serum marker for TB in a heterogeneous immunoassay by means of sample pretreatment.
In beginning this work, we carried out a set of experiments designed to assess the feasibility of detecting a lipoglycan component of the cell wall of Mycobacterium tuberculosis (Mtb), mannose-capped lipoarabinomannan (ManLAM).13–16 ManLAM is a major virulence factor in the infectious pathology of Mtb found in the serum, urine, and other fluids of TB-infected individuals.13,14,17–19 Our preliminary findings, however, showed a ∼250× degradation in LoD when comparing immunoassays for ManLAM spiked in the idealized matrix of a buffer to that directly from human serum. Drawing on literature evidence20,21 and past experience, we hypothesized that (1) the drop in performance was due to the complexation of ManLAM with antibodies and other proteins in serum, and (2) this complexation was responsible for the poor clinical sensitivity reported for immunoassays that tested the potential of ManLAM as a TB marker.18,20–24 While the term immunocomplexation refers to the binding of an antigen to an immune system-generated antibody,6,10,25 we will refer to the complexation of an antigen with an antibody and any of the other constituents in serum simply as complexation.
The most common approaches to managing matrix effects are to match the matrix used to prepare the calibrant solutions with that of the sample or to employ the method of standard addition.11,26 Neither of these approaches, however, address the measurement complications associated with complexation,6,27 which can sterically interfere with the selective capture and/or labeling of the antigen in an immunoassay. Indeed, the difficulties raised by complexation apply not only to ManLAM detection,20–22,24,28–30 but to other markers of importance to human health as well (e.g., measurements of insulin, which circulates in the body both in free and complexed forms).31 There are a number of pretreatment methods that can be used to combat complexation. These range from the simple dilution of the sample with buffer to more involved strategies that selectively denature proteins by means of heat, organic solvents, detergents, salts, acids, and enzymes.6,10,32,33 In assessing these strategies, we must nonetheless recognize both the potential susceptibility of the marker to degradation and the compatibility of the resulting matrix with the antibodies and other components used in an immunoassay.
In examining these problems, we evaluated the utility of several different pretreatment approaches to counter complexation. These assessments were carried out by means of the sandwich-styled immunoassay platform shown in Fig. 1, which uses surface-enhanced Raman scattering (SERS) for readout.5,34–36 This platform embodies many of the functional elements found in enzyme-linked immunosorbent assays (ELISA) and other types of bioassays.10 The main differences rest with the capture substrate, which is an antibody (Ab)-coated gold film, and the label, which is a gold nanoparticle modified with a monolayer of Raman reporter molecules (RRMs) and a layer of Abs. The modified gold nanoparticles are referred to as extrinsic Raman labels (ERLs) and are designed to take advantage of the strong SERS signal from the RRMs enhanced by the plasmonic coupling between the ERLs and the underlying gold used for the capture substrate.37,38 The spectral features of the RRMs are used in indirect analyte quantification.
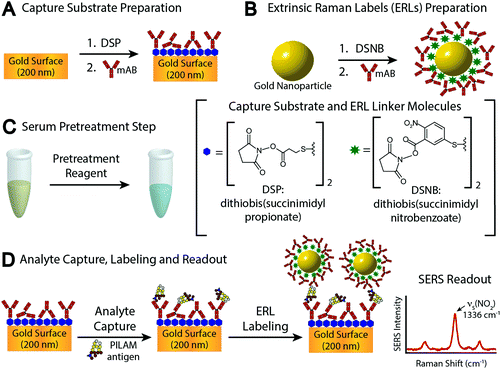 |
| Fig. 1 Schematic representation of the SERS-based immunoassay for the detection of PILAM: (A) gold capture substrate preparation; (B) extrinsic Raman label preparation; (C) serum pretreatment step to release PILAM from complexation; and (D) pretreated PILAM capture, labeling, and SERS readout. | |
For this work, we used phosphoinositol-capped LAM (PILAM) as a ManLAM simulant.39,40 PILAM is derived from M. smegmatis, an often used nonpathogenic model organism for Mtb. M. smegmatis shares >2000 homologous genes with Mtb and both have similar cell wall structures.13,16,41–48 Moreover, M. smegmatis is a fast grower and does not require biosafety level 3 protocols for growth and purification.
This paper reports that the pretreatment of serum is effective and necessary when attempting to detect PILAM at low levels in human serum by means of an immunoassay. We show this by investigating the effectiveness of several protein denaturing methods (i.e., heat, organic solvents, and acids) in triggering the release of PILAM and thereby improving its capture and/or labeling. Although PILAM is acid labile,15,49,50 we found that pretreatment with perchloric acid proved to be an effective means for releasing PILAM from complexation, enabling a 1500× improvement in LoD for this analyte when compared to untreated serum. We also conducted experiments aimed at gaining a preliminary mechanistic perspective behind perchloric acid-driven pretreatment and at identifying potential serum constituents involved in complexation. Collectively, these results serve as the foundation for the procedures used in the companion paper,12 which examines the importance of sample pretreatment when coupled with a sandwich immunoassay using SERS readout in detecting ManLAM in TB patient specimens.
2. Materials and methods
Details on reagents and materials, monoclonal antibody (mAb) selection, and capture substrate and label preparation are included in the ESI.†
2.1 Serum pretreatment
This section pertains to Step C in Fig. 1C: the pretreatment for human sera, which uses HClO4 and was adopted from a classic procedure for breaking antibody and antigen complexes.51 Details for Steps A and B are given in sections 2 and 3 in the ESI.† Pretreatment starts by vortexing a 100 μL serum sample spiked with PILAM at room temperature (21 °C) with 2.0 μL of HClO4 in a centrifuge tube to bring the pH to ∼1. The resulting mixture appeared “milky” due to protein denaturation. Denatured proteins were removed by centrifuging at 13
000g for 5 min, and 75 μL of the resulting clear supernatant was then transferred to a new centrifuge tube. Next, the solutions were neutralized to pH ∼8 by the addition of 6.0 μL of an aqueous solution of 2.0 M K2CO3. This step forms a KClO4 precipitate and an easily removed supernatant containing PILAM. The precipitation rate was increased by cooling the samples to 4 °C for 30 min. The solution was brought back to room temperature (21 °C) prior to analysis.
Two other pretreatments were tested and compared to the HClO4 process for effectiveness: heat and methanol. Heat pretreatment entailed heating a 100 μL aliquot of PILAM spiked in human serum (1.0 ng mL−1) at 95 °C for 5 min, followed by centrifugation at 13
000g for 5 min. The supernatant was then assayed for PILAM. Methanol pretreatment involved mixing 200 μL of serum (1.0 ng mL−1 PILAM) with 200 μL of methanol. The solution was centrifuged at 13
000g for 5 min and the supernatant was removed and heated gently to remove methanol. The resulting solution was assayed for PILAM.
To assess the effectiveness of acid pretreatment in releasing PILAM, 100 μL serum samples spiked with PILAM (1.0 ng mL−1) at room temperature (21 °C) were pretreated with either concentrated HClO4, CF3CO2H, HNO3, HCl, or H2SO4 added until each mixture showed signs of denaturation by a milky appearance. The volumes of acid used were: 4 μL HClO4, 4 μL CF3CO2H, 10 μL HNO3, 10 μL HCl, or 10 μL H2SO4. Denatured proteins and other insoluble materials were pelleted for removal by centrifugation at 13
000g for 5 min. The clear supernatants were adjusted to pH ∼8 with 2.0 M K2CO3.
2.2 Assay and data analysis procedures
The assay steps preceding PILAM capture are detailed in the ESI.† The subsequent steps begin by exposing the capture surface to 20.0 μL of a PILAM-containing sample (Fig. 1D) for 1 h. The substrates were then rinsed three times with 2.0 mM borate buffer containing 0.1% Tween 20 and 150 mM NaCl and exposed to 20.0 μL of the ERL suspension for 16 h. Finally, the substrates were rinsed with 2.0 mM borate buffer containing 0.1% Tween 20 and 10 mM NaCl and dried under ambient conditions for ∼1 h prior to SERS interrogation.
A modified NanoRaman I instrument (Concurrent Analytical) was used in the analysis of the Raman samples.52 The instrument includes a 632.8 nm HeNe laser coupled to a f/2.0 Czerny Turner imaging spectrometer (6–8 cm−1 resolution) by means of a fiber optic probe. The detector is a Kodak 0401E charged coupled device thermoelectrically cooled to 0 °C. The samples were irradiated with a laser power of 3.0 ± 0.1 mW and a 1 s integration time. Using a laser spot size of 20 μm, each sample was interrogated at 10 different substrate locations. The calibrant samples were prepared in duplicate, and, as a consequence, the calibrant responses are the average and standard deviation of 20 separate spectra. The PILAM responses were reported as the baseline-corrected height (6th order polynomial fit) of the symmetric nitro stretch, νs(NO2), at 1336 cm−1 of the RRM 5,5′-dithiobis(succinimidyl-2-nitrobenzoate) (DSNB). The LoD is defined as the average blank signal plus three times its standard deviation.
2.3 Residual protein and pH measurements of pretreated serum
To determine the pH and protein level in serum after pretreatment, a dilution series of each acid was prepared. Next, 10 μL of each acid at increasing concentrations were vortexed for 10 s with 100 μL of serum. These mixtures were centrifuged at 13
000g for 5 min and the supernatant was transferred to a fresh centrifuge tube. The pH of this liquid was analyzed using an OrionStar™ pH meter (Thermo Scientific) and Orion 9810BN micro pH electrode (Thermo Scientific). Due to the well-known acid error, pH values <1 are not reported.
The same solutions were analyzed for protein content by measuring the absorbance at 280 nm using a NanoDrop® 1000 spectrophotometer (Thermo Scientific). This measurement was carried out by placing a 2.0 μL drop between two fiber optic probes separated by 0.2 mm. The resulting absorbance was converted to a protein concentration (mg mL−1) using a protein extinction coefficient of 10 mL mg−1 cm−1.53–55
2.4 Molecular weight fractionation of serum
Two centrifugal molecular weight cutoff (MWCO) filters, 30 and 100 kDa, were used to fractionate human serum. Serum was diluted 4× with phosphate-buffered saline (PBS) to mitigate filter blockage; 500 μL of the resulting solution was added to either a 30 or 100 kDa MWCO filter device and centrifuged at 14
000g for 30 min to separate the serum into two fractions. The filtrates and concentrates were diluted to a final volume of 500 μL with PBS. The fractions selected were the 30 kDa filtrate (containing proteins <30 kDa), the 100 kDa filtrate (containing proteins <100 kDa), and the 100 kDa concentrate (containing proteins >100 kDa). These fractions were subsequently spiked with PILAM to final concentrations of 0, 0.1, 1, 10, 100 and 1000 ng mL−1 and analyzed by SERS.
3. Results and discussion
3.1 PILAM detection in spiked buffer
Experiments were first carried out to establish a measurement benchmark for the assay detailed in Fig. 1 by spiking PILAM into phosphate-buffered saline containing 0.1% Tween 20 (PBST), an arguably innocuous matrix, in concentrations up to 10 ng mL−1. The dose–response plot, shown in Fig. 2A, was constructed by plotting the average of the peak height of the strongest feature (1336 cm−1) in the SERS spectra that was obtained in duplicate runs (see Fig. S1A† for example spectra and band assignment description).
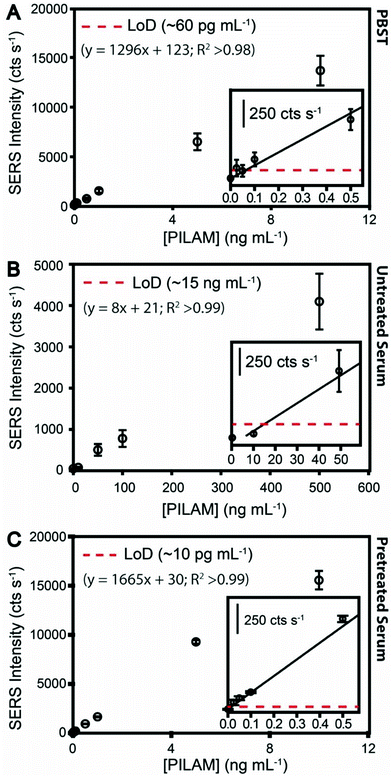 |
| Fig. 2 Dose–response plots established from SERS results for sandwich immunoassays of PILAM-spiked samples: (A) PBST (0–10 ng mL−1) with a linear fit applied and shown in the inset (y = 1296x + 123; R2 >0.98). The LoD was estimated to be ∼60 pg mL−1 (∼3.5 pM). (B) Untreated serum (0–500 ng mL−1) with a linear fit applied and shown in the inset (y = 8x + 21; R2 >0.99). The LoD was estimated to be ∼15 ng mL−1 (∼0.87 nM). (C) HClO4-pretreated serum containing PILAM (0–10 ng mL−1) with a linear fit applied and shown in the inset (y = 1665x + 30; R2 >0.99). The LoD was estimated to be ∼10 pg mL−1 (∼0.57 pM). Data points represent the average and standard deviation of the peak height of the νs(NO2) resulting from the measurement of 10 locations on each of the duplicate substrates. | |
The response at low PILAM levels follows a linear dependence up to ∼10 ng mL−1. The plot plateaus at ∼100 ng mL−1 due to the saturation of mAb binding sites (data not shown). The LoD estimated from these data is ∼60 pg mL−1. The next section compares these results for PILAM in PBST to those for the detection of PILAM directly from human serum.
3.2 PILAM detection in untreated human serum
Samples for these assays were prepared by spiking PILAM into human serum. Fig. 2B shows the dose–response plot from these measurements (SERS spectra are given in Fig. S1B†). As evident from the differences in signal strength, the responses for PILAM in human serum are much weaker than those in PBST. For example, the response for PILAM at 10 ng mL−1 in PBST is ∼13
000 cts s−1; the same level of PILAM in human serum is not measurable. Thus, the estimated LoD for PILAM in PBST (0.06 ng mL−1) is ∼250× lower than that directly from serum (15 ng mL−1). There are two likely factors, acting alone or in concert, for the increase in LoD in serum: (1) the complexation of PILAM, which would sterically hinder its capture and/or labeling; and (2) passivation of the sensor surface, which would arise from the nonspecific adsorption of serum constituents. While we believe that surface passivation plays a role in the loss of performance, the remainder of this paper focuses on examining the possible contribution of complexation to the signal degradation.
3.3 PILAM detection after pretreatment in spiked human serum
As noted, evidence for the presence of complexed forms of TB markers in human serum continues to grow,18,20–22 with recent findings describing the strong association of ManLAM with high density lipoproteins.29 To assess whether the measurement of PILAM directly from human serum is confounded by its complexation with serum proteins, three different pretreatments (i.e., heat, methanol, and acid) that trigger protein denaturation were screened for effectiveness as well as for the ease in which the PILAM-containing product of pretreatment could be manipulated for compatibility with the antibodies and other components in an immunoassay. We opted to examine the use of acid pretreatments as a means to lower sample pH and induce protein denaturing, even though lipoglycans (i.e., PILAM and ManLAM) are acid- and base-labile.15,49,50
The first experiment tested a heat-based pretreatment for human serum used for ManLAM56,57 and other markers.58 This process heated serum spiked with PILAM (1.0 ng mL−1) at ∼95 °C for 5 min, followed by centrifugation and supernatant collection. This process, however, proved to be only marginally useful, resulting in ∼24 ± 2% PILAM signal recovery in serum when compared to the response in PBST. We also investigated the utility of other deproteinizing methods,59 including an extraction with methanol and acidification with HClO4. The use of HClO4 to precipitate proteins dates back 60 years and has been successfully applied to the separation of glycoproteins, endotoxins, and carbohydrates from protein-rich body fluids.51,60–64 Acidification necessitated that a neutralization step be incorporated into the pretreatment process in order to avoid a pH-induced degradation of the monoclonal Ab layer on the capture substrate.
As discussed in the next section, acidification with HClO4 to pH <1 and neutralization with K2CO3 proved to be by far the most effective treatment at ∼1600 cts s−1 and a 115 ± 11% recovery when compared to ∼1400 cts s−1 for PILAM in PBST (1 ng mL−1). PILAM spiked at 1 ng mL−1 in human serum without pretreatment gave a signal indistinguishable from blank serum, whereas heat and methanol pretreatments resulted in 344 and 410 cts s−1, respectively. In other words, the HClO4 pretreatment gave a signal that was 4× larger than the other pretreatment approaches.
The results from an immunoassay using HClO4 pretreatment for PILAM spiked into human serum (0–1.0 ng mL−1) are shown by the dose–response plot in Fig. 2C (see Fig. S1C† for the spectral data). The significance of pretreatment is immediately evident: the strengths of the SERS responses are much stronger than those measured for PILAM directly from untreated serum and, for reasons not yet understood, even slightly above those for PBST in Fig. 2A. Furthermore, the responses for the HClO4-pretreated serum blanks are slightly lower than the PBST blanks, which we suspect is due to the low residual protein content in HClO4-pretreated serum and, therefore, reduced nonspecific ERL adsorption. The response from the nonspecific ERL adsorption approaches instrument noise levels (39 ± 7 cts s−1). The overall trend of the dose–response plot for HClO4-pretreated serum is similar to that for PBST (Fig. 2A), with the response at low PILAM levels up to ∼10 ng mL−1 following a linear dependence corresponding to a dynamic range of ∼3 orders of magnitude and then plateauing at ∼100 ng mL−1 (data not shown).
Importantly, the estimated LoD for PILAM is ∼10 pg mL−1 (∼0.57 pM), which is roughly 100× lower than that reported for ELISA in the analysis of ManLAM in human sera23 and in other matrices common to TB diagnostics (i.e., urine, cerebral spinal fluid, and sputum).65–67 We believe that this improvement in LoD has the potential to advance TB diagnostics detecting ManLAM in body fluids. To this end, the companion paper12 reports on the results from assays of a small sample set of TB patient specimens that were pretreated and analyzed for ManLAM with the same SERS immunoassay platform used herein. Those results show that this new approach yields high levels of clinical sensitivity and specificity, 87.5% and 100%, respectively, pointing to the potential importance of this new development.
3.4 Effect of acid composition on liberating PILAM
To this point, we have evaluated the effectiveness of the pretreatment approaches solely on the ability to measure PILAM. This section expands this assessment by testing several other acids: the strong mineral acids HCl, HNO3, and H2SO4 and CF3CO2H, a popular reagent used in proteomics.68 In these tests, PILAM was spiked in serum at 1 ng mL−1 and then analyzed after completing the acidification/neutralization process used for HClO4.
The resulting SERS responses, referenced to the corresponding signal in PBST, are summarized, along with heat and methanol pretreatment, in Fig. 3. Each pretreatment denatured the serum samples and resulted in a visible precipitate. The data, however, indicate that there are marked differences in PILAM detection. The three mineral acids did not perform nearly as well as HClO4 in improving the detection of PILAM. On the other hand, the signal after CF3CO2H pretreatment was on par with that for HClO4. It therefore appears that HClO4 and CF3CO2H have the potential to enable the detection of PILAM in serum at the same level found for PILAM in PBST.
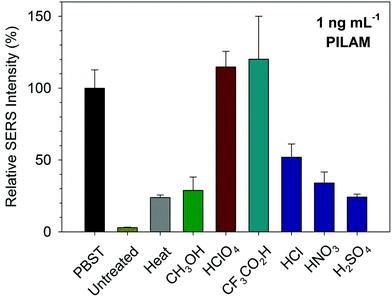 |
| Fig. 3 SERS responses from a sandwich immunoassay for 1 ng mL−1 of PILAM, relative to PBST, for a variety of different pretreatments in human serum: untreated, heat (95 °C), methanol, HClO4, CF3CO2H, HCl, HNO3, and H2SO4. The error bars represent the standard deviations from the SERS measurements of each substrate at 10 different locations. | |
These results were at first puzzling, since all of the pretreatments used strong acids. In an attempt to gain more insight into the origin of these differences, we measured the pH after pretreatment with each acid. Several studies have examined the dependence of protein stability on pH based on the acid dissociation constants (pKa) of the constituent amino acids.69–71 These works found that the pKa for the acidic side chains of most proteins is between ∼1 and 9, a distribution that reflects contributions from a wide range of factors (e.g., electrostatics, hydrophobicity, and hydrogen bonding).70 In general, these studies indicate that once the pH of the environment surrounding the ionizable groups is below the pKa of the most acidic groups, further decreases in pH should have little, if any, impact on protein stability.72 Based on these findings, we set this threshold at pH 1,70,72,73 the idea being that once the solution has reached this pH, the ensuing protein denaturation should liberate all of the complexed PILAM. Not surprisingly, we found that all of the acids tested reduced the serum pH to <1 (data not shown) which, based on the different performances evident in Fig. 3, indicates that pH is not the only factor important to this process.
To gauge the impact of acidification on denaturation, serum was acidified to different levels and centrifuged, and the absorbance of the supernatant at 280 nm was measured. These results, given in Fig. 4, show clear differences in levels of protein precipitation. The treatments with HClO4 and CF3CO2H have the lowest levels of residual protein (∼4%), followed by HNO3 (15%), H2SO4 (52%), and HCl (65%). While HClO4, CF3CO2H, and HNO3 reach limits in inducing precipitation, H2SO4 and HCl do not appear to have reached a limit in the pH range tested. Interestingly, HNO3 is an effective denaturant (∼85% removal), but performs as poorly as HCl and H2SO4 in the release of PILAM.
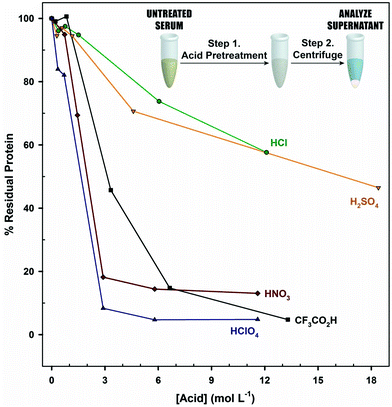 |
| Fig. 4 Effect of each acid pretreatment on the protein level of a 100 μL human serum aliquot. A volume of 10 μL of either HCl, H2SO4, HNO3, CF3CO2H, or HClO4 was added at different concentrations to the serum aliquot, followed by centrifugation to remove the precipitated protein. The supernatant is then subjected to a UV-Vis spectrophotometric measurement (280 nm) to determine protein content. The residual protein levels are plotted as a function of the acid concentration of the 10 μL aliquot. At acid concentrations above 2 mol L−1, the supernatant pH is below 1. | |
If we base the performance of pretreatment only on the extent of protein removal, it appears that ∼96% of the protein content in serum must be removed for the optimal release of PILAM and that the level of release is dependent on the identity of the acid. In accordance with the Hofmeister series, anions differ in their ability to disrupt hydrogen bonding,74–76 which alters the exposure of hydrophobic regions of the protein to solvent and, thereby, the propensity for precipitation.77 The Hofmeister series orders the ability of anions to disrupt this network as: ClO4− > NO3− > Cl− > SO42−. This trend, in part, explains the differences observed in Fig. 4. Studies are underway to fully elucidate the underpinnings of acidification and the role of the anion.
In closing this section, we add that in moving to the analysis of TB-infected patient samples presented in the companion paper, we chose HClO4 pretreatment.12 This decision was based on two factors: (1) the neutralization reaction of HClO4 with K2CO3 forms a precipitate that returns the ionic strength of the supernatant close to that prior to pretreatment; and (2) the reproducibility of the PILAM measurements after HClO4-based pretreatment was superior to those using the other approaches (see Fig. 3).
3.5 Serum fractionation by molecular weight
The results from a preliminary study to size classify the potential culprits in the complexation of PILAM are shown in the two sets of data in Fig. 5. The first set of experiments (1) fractionated human serum by passage through either a 30 or 100 kDa cutoff filter; (2) spiked the <30 and <100 kDa filtrates and the >100 kDa concentrate with PILAM to a final concentration of 1.0 ng mL−1; and (3) assayed PILAM levels. These results are presented in Fig. 5A, along with those for a PBST buffer sample containing 1.0 ng mL−1 of PILAM and a serum blank. The results show clear differences in how the molecular weight (MW) fractions of serum affect the measurable PILAM signal. The low MW fraction (<30 kDa) shows a small loss in signal (∼13%) relative to the PBST sample. A larger signal loss (∼39%) is observed for the <100 kDa fraction. These data indicate that the components in serum between 30 and 100 kDa increase the signal loss by ∼26% over those for the <30 kDa fraction. The response for the highest MW fraction (>100 kDa) is markedly different. Virtually all the signal relative to the PBST sample is lost, with the resulting response being on par with that of the serum blank. This corresponds to ∼61% of the signal being lost due to serum constituents >100 kDa. Collectively, these results indicate that there are components in all three of the serum fractions (0–30 kDa, 30–100 kDa, and >100 kDa) that interfere with PILAM detection, and that the largest loss in signal originates from those with a molecular weight >100 kDa.
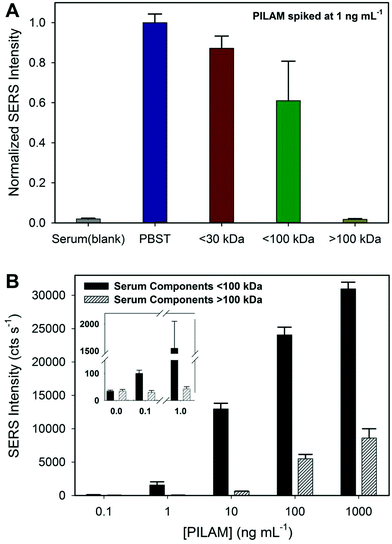 |
| Fig. 5 Results from an investigation of the ability of different molecular weight fractions of human serum to complex PILAM and negatively affect its detection. Human serum was fractionated by weight using molecular weight cutoff (MWCO) filters. (A) The normalized SERS response for 1 ng mL−1 of PILAM spiked in the various weight fractions (0–30 kDa, 0–100 kDa, and >100 kDa) was compared to PILAM spiked in PBST. (B) A PILAM concentration study (0–1000 ng mL−1) investigating the PILAM concentration necessary to overcome complexation in the two human serum weight fractions (0–100 kDa and >100 kDa). | |
Experiments were also carried out to determine the lowest amount of PILAM required to overcome complexation by means of a titration-styled experiment. This study used two MW fractions of serum, <100 and >100 kDa, and changed the final levels of spiked PILAM in these fractions from 0.1 to 1000 ng mL−1 in factors of 10. These results, summarized in Fig. 5B, reinforce the interpretations of the data in Fig. 5A, but in a way that provides insight into how the two fractions affect the LoD. First, the signal from the <100 kDa fraction is detectable at ∼0.1 ng mL−1 (∼6 pM). This implies that only a small amount of PILAM is complexed in the <100 kDa fraction and that 0.1 ng mL−1 of PILAM has more than saturated the binding equilibrium between PILAM and the complexing agents in this fraction. On the other hand, the data indicate that components in the >100 kDa serum fraction are largely responsible for the loss in signal, requiring between 1 and 10 ng mL−1 of PILAM to overcome complexation and enable detection.
This study, while beginning to size classify the serum constituents involved in complexation, still leaves a significant number of molecules as potential culprits. Based on studies of the formation of circulating immune complexes (CICs) in TB-infected patients and the clearance of ManLAM from the circulatory system,29,78–83 we have identified several types of molecules that may complex ManLAM in human serum: IgG, IgA, IgM, HDL, and mannose-binding lectin. These groupings, for the most part, have MWs >100 kDa, which is in line with our findings for PILAM. We also have preliminary evidence that ManLAM can weakly bind to human serum albumin, which may explain the loss in signal in <100 kDa fractions. These and related experiments to identify the molecules responsible for ManLAM complexation are underway, which could ultimately lead to a more targeted pretreatment approach in the analysis of ManLAM in human serum.
4. Conclusions
We believe this work shows that the integration of sample pretreatment approaches with immunoassays will become increasingly important as diagnostic tests seek to surpass existing levels of performance. This especially applies to disease markers that are prone to immunocomplexation in body fluids. Here, we have demonstrated the effectiveness of applying a relatively straightforward and rapid HClO4-based sample pretreatment to the detection of PILAM in human serum. Compared to PILAM detection in untreated serum, HClO4 pretreatment results in a 1500× improvement in LoD (i.e., 10 pg mL−1). The importance of this finding is twofold: (1) it demonstrates the necessity to selectively pretreat when dealing with immunocomplexation, and (2) it shows the possibility of detecting PILAM at extremely low levels. The potential implications of these two findings are vital to ManLAM detection in TB diagnostic tests. Our companion paper evaluates this new pretreatment scheme for ManLAM detection in TB-infected patient specimens.12
Acknowledgements
This work was supported by the Critical Path Initiative of the U.S. Food and Drug Administration (contract U18 FD004034), the Bill and Melinda Gates Foundation (OOP10396210), and the Kerith Foundation.
References
- F. Martinelli, R. Scalenghe, S. Davino, S. Panno, G. Scuderi, P. Ruisi, P. Villa, D. Stroppiana, M. Boschetti, L. R. Goulart, C. E. Davis and A. M. Dandekar, Agron. Sustainable Dev., 2015, 35, 1–25 CrossRef.
- A.-C. Gourinat, O. O'Connor, E. Calvez, C. Goarant and M. Dupont-Rouzeyrol, Emerging Infect. Dis., 2015, 21, 84–86 CrossRef PubMed.
- M. Lin, H. Pei, F. Yang, C. Fan and X. Zuo, Adv. Mater., 2013, 25, 3490–3496 CrossRef CAS PubMed.
- L. B. Laurentius, N. A. Owens, J. Park, A. C. Crawford and M. D. Porter, Expert Rev. Mol. Diagn., 2016, 16, 883–895 CrossRef CAS PubMed.
- J. H. Granger, N. E. Schlotter, A. C. Crawford and M. D. Porter, Chem. Soc. Rev., 2016, 45, 3865–3882 RSC.
- M. L. Chiu, W. Lawi, S. T. Snyder, P. K. Wong, J. C. Liao and V. Gau, J. Lab. Autom., 2010, 15, 233–242 CrossRef CAS.
- L. Phillips, Nature, 2013, 493, 14 CrossRef PubMed.
-
World Health Organization, 2015 Global Tuberculosis Report, 2015 Search PubMed.
- A. Zumla, M. Raviglione, R. Hafner and C. F. von Reyn, N. Engl. J. Med., 2013, 368, 745–755 CrossRef CAS PubMed.
-
D. Wild, The Immunoassay Handbook, Elsevier Ltd., Oxford, 2005 Search PubMed.
-
D. C. Harris, Quantitative Chemical Analysis, W.H. Freeman and Company, New York, 2007 Search PubMed.
- A. C. Crawford, L. B. Laurentius, T. S. Mulvihill, J. H. Granger, J. S. Spencer, D. Chatterjee, K. E. Hanson and M. D. Porter, Analyst, 2016 10.1039/c6an02110g.
- D. Chatterjee and K.-H. Khoo, Glycobiology, 1998, 8, 113–120 CrossRef CAS PubMed.
- I. Vergne, M. Gilleron and J. Nigou, Front. Cell. Infect. Microbiol., 2015, 4, 1–9 Search PubMed.
- D. Chatterjee, C. M. Bozic, M. McNeil and P. J. Brennan, J. Biol. Chem., 1991, 266, 9652–9660 CAS.
- C. J. Petzold, L. H. Stanton and J. A. Leary, J. Am. Soc. Mass Spectrom., 2005, 16, 1109–1116 CrossRef CAS PubMed.
- T. A. Tessema, B. Hamasur, G. Bjune, S. Svenson and B. Bjorvatn, Scand. J. Infect. Dis., 2001, 33, 279–284 CrossRef CAS PubMed.
- P. Sarkar, D. Biswas, G. Sindhwani, J. Rawat, A. Kotwal and B. Kakati, Postgrad. Med. J., 2014, 90, 155–163 CrossRef CAS PubMed.
- M. Shah, E. Variava, C. B. Holmes, A. Coppin, J. E. Golub, J. McCallum, M. Wong, B. Luke, D. J. Martin, R. E. Chaisson, S. E. Dorman and N. A. Martinson, J. Acquired Immune Defic. Syndr., 2009, 52, 145–151 CrossRef PubMed.
- R. Wood, K. Racow, L.-G. Bekker, K. Middelkoop, M. Vogt, B. N. Kreiswirth and S. D. Lawn, BMC Infect. Dis., 2012, 12, 47 CrossRef PubMed.
- S. D. Lawn and A. Gupta-Wright, Trans. R. Soc. Trop. Med. Hyg., 2016, 110, 180–185 CrossRef PubMed.
- J. A. Cox, R. L. Lukande, S. Kalungi, E. Van Marck, K. Van de Vijver, A. Kambugu, A. M. Nelson, R. Colebunders and Y. C. Manabe, PLoS One, 2015, 10, e0123323 Search PubMed.
- S. Sarkar, X. Tang, D. Das, J. Spencer, T. Lowary and M. Suresh, PLoS One, 2012, 7, e32340 CAS.
- C. E. Chan, S. Götze, G. T. Seah, P. H. Seeberger, N. Tukvadze, M. R. Wenk, B. J. Hanson and P. A. MacAry, Sci. Rep., 2015, 5, 10281 CrossRef CAS PubMed.
- J. Tate and G. Ward, Clin. Biochem. Rev., 2004, 25, 105–120 Search PubMed.
- M. Thompson and S. L. Ellison, Accredit. Qual. Assur., 2005, 10, 82–97 CrossRef CAS.
-
S. L. Prabu and T. N. K. Suriyaprakash, in Applied Biological Engineering - Principles and Practice, ed. G. R. Naik, InTech, Rijeka, 2012, ch. 21, pp. 479–506 Search PubMed.
- E. Sada, D. Aguilar, M. Torres and T. Herrera, J. Clin. Microbiol., 1992, 30, 2415–2418 CAS.
- R. Sakamuri, D. Price, M. Lee, S. Cho, C. Barry, L. Via, B. Swanson and H. Mukundan, Tuberculosis, 2013, 93, 301–307 CrossRef CAS PubMed.
- T. Tessema, G. Bjune, B. Hamasur, S. Svenson, H. Syre and B. Bjorvatn, Scand. J. Infect. Dis., 2002, 34, 97–103 CrossRef PubMed.
- R. Sapin, Clin. Chem. Lab. Med., 2002, 40, 705–708 CrossRef CAS PubMed.
-
D. Wells, in Progress in Pharmaceutical and Biomedical Analysis, Elsevier, Amsterdam, 2003, vol. 5, pp. 199–254 Search PubMed.
- D. Petsch and F. B. Anspach, J. Biotechnol., 2000, 76, 97–119 CrossRef CAS PubMed.
- D. S. Grubisha, R. J. Lipert, H.-Y. Park, J. Driskell and M. D. Porter, Anal. Chem., 2003, 75, 5936–5943 CrossRef CAS PubMed.
- J. D. Driskell, R. J. Lipert and M. D. Porter, J. Phys. Chem. B, 2006, 110, 17444–17451 CrossRef CAS PubMed.
- M. D. Porter, R. J. Lipert, L. M. Siperko, G. Wang and R. Narayanan, Chem. Soc. Rev., 2008, 37, 1001–1011 RSC.
- J. D. Driskell, C. G. Larrick and C. Trunell, Langmuir, 2014, 30, 6309–6313 CrossRef CAS PubMed.
- A. Lopez, F. Lovato, S. H. Oh, Y. H. Lai, S. Filbrun, E. A. Driskell and J. D. Driskell, Talanta, 2016, 146, 388–393 CrossRef CAS PubMed.
- Z. He and J. De Buck, BMC Microbiol., 2010, 10, 121 CrossRef PubMed.
- G. Etienne, F. Laval, C. Villeneuve, P. Dinadayala, A. Abouwarda, D. Zerbib, A. Galamba and M. Daffé, Microbiology, 2005, 151, 2075–2086 CrossRef CAS PubMed.
- A. Venisse, J. M. Berjeaud, P. Chaurand, M. Gilleron and G. Puzo, J. Biol. Chem., 1993, 268, 12401–12411 CAS.
- K. H. Khoo, E. Douglas, P. Azadi, J. M. Inamine, G. S. Besra, K. Mikušová, P. J. Brennan and D. Chatterjee, J. Biol. Chem., 1996, 271, 28682–28690 CrossRef CAS PubMed.
- A. K. Singh and J. M. Reyrat, Curr. Protoc.
Microbiol., 2009, 14:C:10C.1, 10C.1.1–10C.1.12 Search PubMed.
- L. T. N. Thi, R. B. Maura, S. Férnandez, G. Reyes, J. L. Perez, F. t. Reyes, M. a. d. l. A. García, M. Fariñas, J. F. Infante, Y. Tirado, A. Puig, G. Sierra, N. Álvarez, J. C. Ramírez, M. a. E. Sarmiento, M.-N. Norazmi and A. Acosta, Vaccimonitor, 2010, 19, 20–26 Search PubMed.
- J. M. Reyrat and D. Kahn, Trends Microbiol., 2001, 9, 472–474 CrossRef CAS PubMed.
- R. O. Akinola, G. K. Mazandu and N. J. Mulder, J. Bacteriol. Parasitol., 2013, 4, 1–11 Search PubMed.
- A. Waagmeester, J. Thompson and J.-M. Reyrat, Trends Microbiol., 2005, 13, 505–509 CrossRef CAS PubMed.
- A. Mohan, J. Padiadpu, P. Baloni and N. Chandra, Genome Announc., 2015, 3, e0152014 CrossRef PubMed.
- P. Ludwiczak, T. Brando, B. Monsarrat and G. Puzo, Anal. Chem., 2001, 73, 2323–2330 CrossRef CAS PubMed.
- A. Vercellone, J. Nigou and G. Puzo, Front. Biosci., 1998, 3, e149–e163 CrossRef CAS.
- A. J. Anderson, Nature, 1965, 208, 491–492 CrossRef CAS PubMed.
-
H.-Y. Park, J. D. Driskell, K. M. Kwarta, R. J. Lipert, M. D. Porter, C. Schoen, J. D. Neill and J. F. Ridpath, in Surface-Enhanced Raman Scattering - Physics and Applications, ed. K. Kneipp, M. Moskovits and H. Kneipp, Springer-Verlag Berlin, Heidelberg, 2006, vol. 103, pp. 427–446 Search PubMed.
-
J. E. Noble and M. J. A. Bailey, in Methods Enzymol, ed. R. R. Burgess and M. P. Deutscher, Academic Press, New York, 2009, vol. 463, ch. 8, pp. 73–95 Search PubMed.
- C. N. Pace, F. Vajdos, L. Fee, G. Grimsley and T. Gray, Protein Sci., 1995, 4, 2411–2423 CrossRef CAS PubMed.
-
G. D. Fasman, Practical Handbook of Biochemistry and Molecular Biology, CRC Press, Boca Raton, 1992 Search PubMed.
- L. S. Schlesinger, S. R. Hull and T. M. Kaufman, J. Immunol., 1994, 152, 4070–4079 CAS.
- S. D. Lawn, BMC Infect. Dis., 2012, 12, 103 CrossRef PubMed.
- D. Stynen, A. Goris, J. Sarfati and J. Latgé, J. Clin. Microbiol., 1995, 33, 497–500 CAS.
-
R. K. Scopes, Protein Purification: Principles and Practice, Springer, New York, 1994 Search PubMed.
- R. J. Winzler, A. W. Devor, J. W. Mehl and I. M. Smyth, J. Clin. Invest., 1948, 27, 609–616 CrossRef CAS PubMed.
- N. De Jonge, Y. E. Fillié and A. M. Deelder, J. Immunol. Methods, 1987, 99, 195–197 CrossRef CAS PubMed.
- T. Obayashi, J. Lab. Clin. Med., 1984, 104, 321–330 CAS.
- K. Inada, S. Endo, K. Takahashi, M. Suzuki, T. Narita, T. Yoshida, H. Suda, T. Komuro and M. Yoshida, Microbiol. Immunol., 1991, 35, 303–314 CrossRef CAS PubMed.
- T. Hirai, H. Ohyama and R. Kido, Anal. Biochem., 1987, 163, 339–342 CrossRef CAS PubMed.
- L. M. P. Arias-Bouda, L. N. Nguyen, L. M. Ho, S. Kuijper, H. M. Jansen and A. H. Kolk, J. Clin. Microbiol., 2000, 38, 2278–2283 CAS.
- B. Hamasur, J. Bruchfeld, M. Haile, A. Pawlowski, B. Bjorvatn, G. Källenius and S. Svenson, J. Microbiol. Methods, 2001, 45, 41–52 CrossRef CAS PubMed.
- V. B. Patel, R. Singh, C. Connolly, V. Kasprowicz, A. Zumla, T. Ndungu and K. Dheda, PLoS One, 2010, 5, e15664 CAS.
-
J. von Hagen, Proteomics sample preparation, WILEY-VCH, Weinheim, 2008 Search PubMed.
- R. L. Thurlkill, G. R. Grimsley, J. M. Scholtz and C. N. Pace, Protein Sci., 2006, 15, 1214–1218 CrossRef CAS PubMed.
- C. N. Pace, G. R. Grimsley and J. M. Scholtz, J. Biol. Chem., 2009, 284, 13285–13289 CrossRef CAS PubMed.
- G. R. Grimsley, J. M. Scholtz and C. N. Pace, Protein Sci., 2009, 18, 247–251 CAS.
- D. E. Anderson, W. J. Becktel and F. W. Dahlquist, Biochemistry, 1990, 29, 2403–2408 CrossRef CAS PubMed.
- W. Kauzmann, Adv. Protein Chem., 1959, 14, 1–63 CrossRef CAS PubMed.
- R. L. Baldwin, Biophys. J., 1996, 71, 2056–2063 CrossRef CAS PubMed.
- Y. Zhang and P. S. Cremer, Curr. Opin. Chem. Biol., 2006, 10, 658–663 CrossRef CAS PubMed.
- W. H. Sawyer and J. Puckridge, J. Biol. Chem., 1973, 248, 8429–8433 CAS.
- D. Rajalingam, C. Loftis, J. J. Xu and T. K. Kumar, Protein Sci., 2009, 18, 980–993 CrossRef CAS PubMed.
- A. Bhattacharya, S. N. Ranadive, M. Kale and S. Bhattacharya, Am. Rev. Respir. Dis., 1986, 134, 205–209 CAS.
- A. Raja, P. R. Narayanan, R. Mathew and R. Prabhakar, J. Lab. Clin. Med., 1995, 125, 581–587 CAS.
- A. Glatman-Freedman, A. J. Mednick, N. Lendvai and A. Casadevall, Infect. Immun., 2000, 68, 335–341 CrossRef CAS PubMed.
- A. Raja, K. R. Uma Devi, B. Ramalingam and P. J. Brennan, Clin. Diagn. Lab. Immunol., 2002, 9, 308–312 CAS.
- W. K. Ip, K. Takahashi, R. A. Ezekowitz and L. M. Stuart, Immunol. Rev., 2009, 230, 9–21 CrossRef PubMed.
- A. M. Samuel, M. D. Ashtekar and R. D. Ganatra, Clin. Exp. Immunol., 1984, 58, 317–324 CAS.
Footnote |
† Electronic supplementary information (ESI) available. See DOI: 10.1039/c6an02109c |
|
This journal is © The Royal Society of Chemistry 2017 |
Click here to see how this site uses Cookies. View our privacy policy here.