A computational study of self-assembled hexapeptide inhibitors against amyloid-β (Aβ) aggregation†
Received
27th October 2016
, Accepted 22nd November 2016
First published on 23rd November 2016
Abstract
The fibrillation and deposition of amyloid-β (Aβ) peptides in human brains are pathologically linked to Alzheimer's disease (AD). Development of different inhibitors (peptides, organic molecules, and nanoparticles) to prevent Aβ aggregation becomes a promising therapeutic strategy for AD treatment. We recently propose a “like-interacts-like” design principle to computationally design/screen and experimentally validate a new set of hexapeptide inhibitors with completely different sequences from the Aβ sequence. These hexapeptide inhibitors inhibit Aβ aggregation and reduce Aβ-induced cytotoxicity. However, inhibitory mechanisms of these hexapeptides and the underlying interactions between hexapeptides and Aβ remain unclear. Herein we apply multi-scale computational methods (quantum-chemical calculations, molecular docking and explicit-solvent molecular dynamic simulation) to explore the structure, dynamics, and interaction between 3 identified hexapeptides (CTLWWG, GTVWWG, and CTIYWG) and different Aβ-derived fragments and an Aβ17–42 pentamer. When interacting with 6 Aβ-derived fragments, 3 hexapeptide inhibitors show stronger interactions with two lysine-included fragments (16KLVFFA21 and 27NKGAII33) than other fragments, indicating different sequence-specific interactions with Aβ. When interacting with the Aβ17–42 pentamer, the 3 peptides show similar binding modes and interaction mechanisms by preferentially binding to the edge of the Aβ17–42 pentamer to potentially block the Aβ elongation pathway. This work provides structural-based binding information on further modification and optimization of these peptide inhibitors to experimentally enhance their inhibitory abilities against Aβ aggregation.
Introduction
Alzheimer's disease (AD), associated with loss of memory, decline of cognition, and disability of behavior, ultimately results in dementia and death.1–3 The generation of “senile plaques” involving extracellular deposits of the amyloid-β peptide (Aβ), which is the main histopathological criterion for AD,4–7 includes amyloid precursor protein cleaving into Aβ, and soluble Aβ assembling into amyloid oligomers and fibrils. In recent years, various inhibitors based on different strategies such as blocking APP expression, inhibiting APP cleavage, or decreasing Aβ aggregates have been developed,8–10 but there is so far no drug which can be used to thoroughly cure AD. In the human body of AD patients, conformation of Aβ changes from random coil or alpha helix to β-sheet in the process of Aβ monomers assembling into aggregates with neurotoxicity,11–13 so the interference to the formation of β-sheet structures is a feasible therapeutic strategy.14 To date, many inhibitors, including small molecules,15 surfactants,16 chaperone proteins,17 peptides18 and nanoparticles,19 have been proposed to inhibit Aβ aggregation. Because of the particular effects on the anti-aggregation of Aβ, as well as the benign biocompatibility and biomimetic natures, peptide-based inhibitors are promising potential drugs for AD therapy.20–25
Most peptide-based inhibitors are derived from the hydrophobic fragments of Aβ, and exhibit favorable inhibitory effects,26–28 however, the sequence source of those Aβ-derived peptides is very limited. Extensively, existences of cross-sequence interactions between different amyloid peptides29–38 indicate that amyloid peptides, regardless of the homologous or nonhomologous sequence, could interact with each other as long as amyloid peptides contain similar β-sheet conformation, which is a process controlled by a conformational selection mechanism.39 This suggests that the sequence search of peptide-based inhibitors against Aβ aggregation should not be restricted to the hydrophobic fragments of Aβ.40–42 In our previous work, we proposed a “like-interacts-like” method which was applied to design peptide-based Aβ inhibitors. The “like-interacts-like” method means that the self-assembled polypeptides could interact with the Aβ-derived fragments with similar characteristics and competitively disturb the interaction between Aβ monomers to inhibit the self-assembly of Aβ monomers or cause dis-aggregation of Aβ oligomers. In our previous work, we combined various computational and experimental methods, including quantitative structure–activity relationship (QSAR) modeling, molecular dynamics (MD) simulation, Thioflavin T (ThT) fluorescence assay, circular dichroism (CD), atomic force microscopy (AFM), surface plasmon resonance (SPR) and an MTT SHSY5Y cell assay, to design and identify potential exogenous hexapeptides as Aβ inhibitors.42 Finally, our results indicate that 11 self-assembled hexapeptides could inhibit Aβ aggregation, and in particular the 3 hexapeptides (CTIYWG, CTLWWG and GTVWWG) had superior inhibitory effects. However, the mechanisms of action of these designed hexapeptides and the underlying interactions between hexapeptides and Aβ remain unclear.
Motivated by our previous work,42 here we computationally studied the binding modes (e.g. binding sequences, affinities, and conformations) between the 3 peptide inhibitors (CTIYWG, CTLWWG and GTVWWG) and 6 different Aβ-derived fragmental dimers (16KLVFFA21, 27NKGAII32, 29GAIIGL34, 30AIIGLM35, 35MVGGVV40 and 37GGVVIA42) and the Aβ17–42 pentamer using a combination of quantum-chemical calculations, molecular docking and all-atom MD simulations. The computational results indicate that while all peptide inhibitors can interact with Aβ17–42 fragments and the pentamer, they exhibited similar binding modes with Aβ. These three peptides exhibited the same interaction preferences with the side of the Aβ17–42 pentamer, targeting on two common Aβ regions of 16KLVFFA21 or 27NKGAII33. Our computational results also interpreted how our previously identified inhibitors interacted with Aβ17–42, thereby performing the favorable inhibition effect on Aβ aggregation. This work provides atomic-level structural information on the design of effective peptide-based inhibitors against Aβ aggregation.
Materials and methods
Construction and optimization of hexapeptide-based dimers
Given that a dimer is a basic building block for any large self-assembled aggregate, we performed quantum-chemical calculations to examine the structural stability of dimers of 3 hexapeptides (CTIYWG, CTLWWG and GTVWWG) and 6 Aβ-derived fragments (16KLVFFA21, 27NKGAII32, 29GAIIGL34, 30AIIGLM35, 35MVGGVV40 and 37GGVVIA42), with two typical peptide organizations (parallel and antiparallel orientation relative to each other). A dimer was constructed by packing two hexapeptides, each adopting a β-chain conformation, on the top of each other in parallel or antiparallel manners, with an initial peptide–peptide separation distance of ∼4.7 Å. Such a conformation corresponds to the characteristic X-ray scattering diffraction signal of the interchain distance in β-sheets of amyloid fibrils.40
Three hexapeptides bound with 6 Aβ-derived fragments in parallel and antiparallel ways, thus a total of 36 dimer-based models, were considered. In addition, 18 dimers in parallel and antiparallel self-assembly, including 12 self-aggregates of 6 Aβ-derived fragments, and 6 self-aggregates of 3 hexapeptides, were designed to compare with the corresponding dimers formed by 3 hexapeptides with 6 Aβ-derived fragments. In all the dimer models, the 6 Aβ-derived fragments (16KLVFFA21 (PDB entry 2Y2A), 27NKGAII32 (PDB entry 3Q2X), 29GAIIGL34 (PDB entry 3PZZ), 30AIIGLM35 (PDB entry 2Y3J), 35MVGGVV40 (PDB entry 2ONA) and 37GGVVIA42 (PDB entry 2ONV) downloaded from the RCSB Protein Data Bank (http://www.rcsb.org/pdb/home/home.do) retained their crystal structures, and the initial configuration of the hexapeptides maintained their β-chain secondary structures.
For all the dimers, all geometries were completely optimized in all internal degrees of freedom using density functional theory (DFT) at the M062X43,44/6-31G(d) level of theory. For all the optimized models, frequency calculations were subsequently performed, with no imaginary frequency results, indicating that all optimal geometries were situated in true minimum points of the potential energy surfaces. All the calculations above were performed using the Gaussian09 program.45
Interaction energy of hexapeptide-based dimers
The interaction energy between two monomers of the dimer was calculated using the following equation: | Eint = Edim − (Em1 + Em2) | (1) |
where Eint, Edim, Em1 and Em2 are the interaction energy between two monomers in the optimized dimer, the energy of the optimized dimer, and the energy of each monomer in the optimized dimer, respectively. All the energies were calculated at the M062X/6-311G+(d,p) level of theory, and the counterpoise correction for the basis set superposition error was applied in all the calculations. All the calculations were performed using the Gaussian09 program.45
Noncovalent interaction of hexapeptide-based dimers
The noncovalent interaction (NCI) was calculated using 3 quantities including electron density (ρ), reduced density gradient (s(r)) and sign of the second eigenvalue of electron-density hessian matrix (sign(λ2)).46,47 The function of s(r) was performed as follows: | 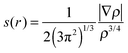 | (2) |
The ρ value about the spike is an indicator of the strength of the corresponding interaction, the value in the electron density ranging in 0.01 < ρ < 0.05 (a.u.) represents hydrogen bonds or strong steric effects, and the value in the low-density range in ρ < 0.01 (a.u.) represents weaker interactions such as VDW effects.48
To understand the binding in more detail, the Laplacian is often decomposed into a sum of contributions along 3 principal axes of maximal variation. These components are 3 eigenvalues λi (i = 1, 2 and 3) of the electron-density Hessian (second derivative) matrix as follows:
| ∇2ρ = λ1 + λ2 + λ3, (λ1 ≤ λ2 ≤ λ3) | (3) |
The Laplacian in the interatomic region always displays a positive contribution no matter whether it is bonding or nonbonding, thus it is not used to distinguish the weak and noncovalent interactions.
49 Therefore, the sign of the second eigenvalue of the electron-density hessian matrix often is used to distinguish the interaction between attraction (
λ2 < 0) and repulsion (
λ2 > 0).
The MULTIWFN program50 was used to calculate the distribution of ρ, function s(r) and sign(λ2) where the lattice spacing was 0.1 a.u. on the 3 directions of X, Y and Z. The 3D NCI was displayed using VMD software.51 The interior forces in every monomer were ignored.
Model of the Aβ pentamer
The Aβ pentamer model was utilized to probe the binding modes and simulate orientations with 3 hexapeptides. The Aβ pentamer for computational simulations was constructed based on the solid-state NMR structure (PDB entry 2BEG from the RCSB Protein Data Bank (http://www.rcsb.org/pdb/home/home.do)) determined by Luhrs et al.52 The β-hairpin Aβ pentamer (17–42) consists of two β-sheets (residues L17–S26 and I31–A42) connected by a U-bend (residues N27–A30).
Docking method
Selections of the optimal docking sites for 3 hexapeptides on the Aβ pentamer were done using the ‘Lamarckian’ genetic algorithm using AUTODOCK.53 The grid box size was set to 120_90_90 points with a grid spacing of 0.375, which was big enough to cover the whole structure of the receptor (Aβ pentamer), for determining the region of preferred docking on the Aβ pentamer. The Aβ pentamer was kept rigid and 1000 conformations of the flexible hexapeptides were searched and docked. The optimal binding conformation was taken as the initial structure of the complex for the following MD simulations.
MD simulation protocol
The NAMD package54 combined with the CHARMM27 force field and the TIP3P solvent model was employed to accomplish all the MD simulations. The complexes consisting of hexapeptides and the Aβ pentamer were solvated in a water box with a minimal margin of 15 Å from any edge of the water box to any peptide atom. Then, each system was neutralized by adding Cl− and Na+ ions to mimic an ionic strength of 200 mM. The resulting systems were first subject to 5000 steps of the steepest descent minimization with position constraints on the Aβ pentamer and hexapeptides, followed by an additional 5000 steps of conjugate gradient minimization without any position constraints. Short 1 ns MD simulations were performed to heat the solvated and neutralized systems from 0 to 310 K by constraining the backbones of the complexes. MD simulations for 100 ns were performed using the NPT ensemble (T = 310 K and P = 1 atm) under periodic boundary conditions. The RATTLE algorithm was applied to constrain all covalent bonds involving hydrogen atoms. Short-range VDW interactions were computed by a switch function with a 12 and 14 Å cutoff, whereas long-range electrostatic interactions were calculated using the force-shifted method with a 14 Å cutoff. MD trajectories were saved every 4 ps for subsequent analyses which were performed using tools within CHARMM, VMD, and codes developed in-house.
Generalized born molecular volume
The last 40 ns trajectories were extracted from the explicit MD simulation excluding water molecules. The solvation energies of all systems were calculated using the generalized Born molecular volume (GBMV).55,56 In this method, the dielectric constant of water was set to 80.0. The hydrophobic solvent-accessible surface area term factor was set to 0.00592 kcal mol−1.57 Each variant was minimized for 1000 cycles, in order to eliminate the simulation fluctuations.57 The binding energies (dG) between hexapeptides and the Aβ pentamer were calculated using the molecular mechanics (MM)–GBMV method. | dG = GAβ+hexapeptide − GAβ − Ghexapeptide | (4) |
where GAβ+hexapeptide, GAβ and Ghexapeptide denote the GBMV energies for the Aβ–hexapeptide complex, Aβ and hexapeptide, respectively.
Results and discussion
Eisenberg's group has reported crystal structures of several Aβ-derived aggregation-forming fragments, such as 16KLVFFA21, 27NKGAII32, 29GAIIGL34, 30AIIGLM35, 35MVGGVV40 and 37GGVVIA42.58,59 It has been illustrated that these fragments play an important role in Aβ aggregation.27,60–62 Recently, many inhibitors targeting these fragments have exhibited satisfactory inhibitory effects.14,25,60,63,64 According to the “like-interacts-like” principle,42 we supposed that our designed ectogenic hexapeptides can interact with these key Aβ-derived fragments, thereby to disturb Aβ aggregation.
Herein we computationally determined with which Aβ-derived fragment the 3 hexapeptides interacted by using quantum-chemical calculations. We took these fragments instead of the whole Aβ sequence as the explored object in the process of building dimer models. This not only makes the hexapeptides rationally orientate to the determinative fragments of Aβ, but also simplify the simulated model while ensuring the efficiency of the calculations. The Aβ17–42 pentamer is a very abundant soluble oligomer and has very high toxicity,65,66 therefore, we chose the Aβ17–42 pentamer as a receptor of the hexapeptides to explore the potential mechanism of action between the 3 hexapeptides and Aβ. First, the most possible binding positions of the hexapeptides with the Aβ17–42 pentamer were determined by molecular docking. The explicit-solvent MD simulations were then used to investigate the orientation, conformation, and binding modes of the hexapeptides with the Aβ17–42 pentamer.
Search of Aβ-derived fragments bound by 3 hexapeptides
We optimized the conformation of dimers in a vacuum at the M062X/6-31G(d) level, then calculated the interaction energies between two monomers in each dimer at the M062X/6-311+G(d,p) level. For the optimized dimers, the smaller the interaction energy is, the more closely the monomers bind, and the higher the possibility for the formed dimer is. Accordingly, we chose the models with smaller interaction energies in parallel and antiparallel ways as the final binding models, and finally acquired 27 dimers (Fig. 1) from all the optimized dimer models.
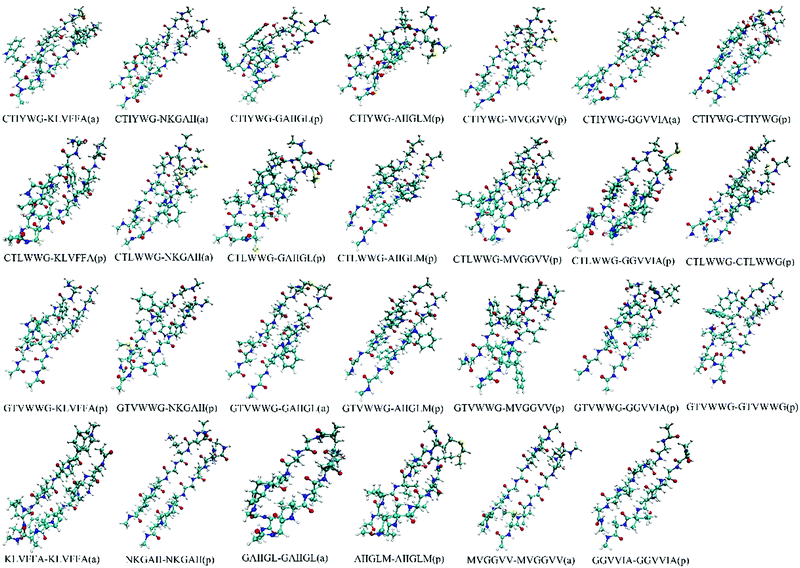 |
| Fig. 1 Conformations of the optimized dimers. The “a” and “p” in the bracket represent the conformation in an antiparallel and parallel manner, respectively. | |
Table 1 shows that the interaction energies of CTIYWG, CTLWWG and GTVWWG with 6 Aβ-derived fragments are sorted by 16KLVFFA21 < 27NKGAII32 < 37GGVVIA42 < 29GAIIGL34 < 30AIIGLM35 < 35MVGGVV40, 16KLVFFA21 < 27NKGAII32 < 37GGVVIA42 < 30AIIGLM35 < 29GAIIGL34 < 35MVGGVV40, and 27NKGAII32 < 16KLVFFA21 < 30AIIGLM35 < 35MVGGVV40 < 29GAIIGL34 < 37GGVVIA42, respectively. Three hexapeptides have higher interaction energies with 16KLVFFA21 and 27NKGAII32 than the other Aβ-derived fragments (37GGVVIA42, 29GAIIGL34, 30AIIGLM35 and 35MVGGVV40), indicating that two fragments, 16KLVFFA21 and 27NKGAII32, are the most potential regions with which 3 hexapeptides interact. Particularly, both CTIYWG and CTLWWG have the highest interaction with the highly hydrophobic fragment, 16KLVFFA21, which has been considered as the crucial region in Aβ aggregation,62 and also as the target region of Aβ inhibitors.25,64
Table 1 Interaction energy of hexapeptide-based dimers (kcal mol−1) calculated at the M062X/6-31G(d) level of theory in a vacuum
Hexapeptide |
KLVFFA |
NKGAII |
GAIIGL |
AIIGLM |
MVGGVV |
GGVVIA |
Self-aggregation |
a: antiparallel manner; p: parallel manner.
|
CTIYWG |
−121.2(a)a |
−100.3(a) |
−70.5(p) |
−70.4(p) |
−69.8(p) |
−76.5(a) |
−81.7(p) |
CTLWWG |
−121.7(p) |
−101.2(a) |
−75.0(p) |
−76.3(p) |
−69.7(p) |
−86.7(p) |
−75.7(p) |
GTVWWG |
−96.2(p) |
−109.3(p) |
−71.4(a) |
−85.8(p) |
−76.3(p) |
−71.1(p) |
−87.5(p) |
Self-aggregation |
−66.1(a) |
−47.2(p) |
−71.3(a) |
−72.9(p) |
−60.0(a) |
−68.8(p) |
— |
The interaction energy of CTIYWG–16KLVFFA21 and CTIYWG–27NKGAII32 was both less than that of CTIYWG–CTIYWG, 16KLVFFA21–16KLVFFA21 and 27NKGAII32–27NKGAII32, respectively, indicating there was a strong binding force between CTIYWG and the two fragments (16KLVFFA21 and 27NKGAII32) relative to their self-aggregation. The interaction energy of CTLWWG–16KLVFFA21, CTLWWG–27NKGAII32, CTLWWG–30AIIGLM35 and CTLWWG–37GGVVIA42) was lower than that of the self-aggregates of each monomer (CTLWWG–CTLWWG, 16KLVFFA21–16KLVFFA21, 27NKGAII32–27NKGAII32, 30AIIGLM35–30AIIGLM35 and 37GGVVIA42–37GGVVIA42), respectively, thus there was a higher binding force in the dimers of CTLWWG with four Aβ-derived fragments (16KLVFFA21, 27NKGAII32, 30AIIGLM35 and 37GGVVIA42) than in the dimers formed by each self-monomer (CTLWWG, 16KLVFFA21, 27NKGAII32, 30AIIGLM35 and 37GGVVIA42). Both GTVWWG–16KLVFFA21 and GTVWWG–27NKGAII32 had lower interaction energies than their respective self-aggregates (GTVWWG–GTVWWG, 16KLVFFA21–16KLVFFA21 and 27NKGAII32–27NKGAII32), demonstrating that GTVWWG preferred to bind the key Aβ-derived sequences (16KLVFFA21 and 27NKGAII32) rather than to form self-aggregates.
We explored the influence of different solvents on the interaction energy of all dimers. In our previous study,67 the aggregation characteristics and the activities of the designed hexapeptides were assayed in the intermixture of water and DMSO, thus we calculated the interaction energies of all dimers at the M062X/6-311+G(d,p) level in water and DMSO solvent, respectively. Obviously, compared with interaction energies in a vacuum (Table 1), the trend of interaction energies in water and DMSO solvent (Table S1 of the ESI†) generally remained unchanged. Meanwhile, the 3 studied hexapeptides still had higher interaction energies with KLVFFA or NKGAII than with other fragments of Aβ.
Driving forces between hexapeptides and Aβ-derived fragments
Electron density (ED) in quantum chemistry is the capital quantity in DFT, thus all chemical properties could be obtained from calculations of ED in principle.68 The parallel CTLWWG–16KLVFFA21 dimer had the highest interaction energy in all the dimers, thus we took this dimer as a template to explore the dominating forces maintaining the conformation of dimers by NCI analysis.
The formed hydrogen bonds of the CTLWWG–16KLVFFA21 dimer are exhibited in Fig. 2. Generally, the bigger the absolute value of critical density is, the stronger the corresponding hydrogen bond is. The hydrogen bond weakened subsequently according to the critical densities denoted by letters from a to s, including 3 kinds of hydrogen bonds, i.e., strong (a–f, ρ > 0.020), moderate (g–l, 0.015 < ρ < 0.020), and weak (m–s, 0.010 < ρ < 0.015). According to the distribution and strength of the hydrogen bonds, we concluded that the strong hydrogen bonds are always formed with the participation of the –NH donor on the lysine side chain, or by the NH group and the O atom between two backbones. The moderate hydrogen bonds mainly consist of NH⋯OC and CH⋯OC between two backbones. The weak hydrogen bonds generally take the –CH group as a donor on both the backbones and side chains, and the O atom as an acceptor on the backbones; moreover, compared with those of the side chain, the –CH groups on the backbone generally have a closer distance and a larger angle with the O atom, which is beneficial to the formation of hydrogen bonds. In addition, hydrogen bonds between the two backbones are always stronger than those formed with the participation of the side chain group. Fig. 3 displays the VDW interaction of CTLWWG with 16KLVFFA21. It was shown that the isosurfaces, representing the VDW interaction, appeared between atoms and/or groups with a close distance. Compared with the hydrogen bond forces, the VDW forces were weak, but they had a very large action scope, thus the VDW interactions were an indispensable force on the maintenance of the dimers.
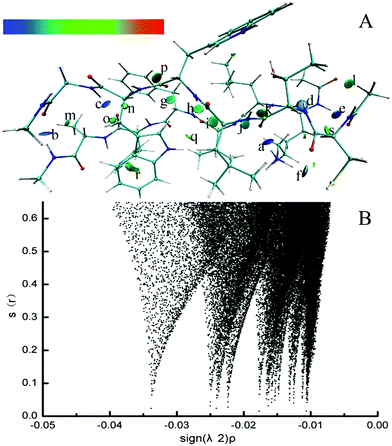 |
| Fig. 2 3D (A) and 2D (B) noncovalent interaction of hydrogen bonds in the parallel CTLWWG–KLVFFA dimer. The deeper the blue is, the stronger the hydrogen bond is. The “a” denotes the hydrogen bond between the –NH group on the lysine side chain and the O atom on the C-terminal of threonine. The “b” denotes the hydrogen bond between the –NH group on the C-terminal of KLVFFA and the O atom on the C-terminal of glycine. The “c” denotes the hydrogen bond between the –NH group on the N-terminal of glycine and the O atom on the C-terminal of phenylalanine (PHE20). The “d” denotes the hydrogen bond between the –NH group on the N-terminal of threonine and the O atom on the C-terminal of lysine. The “e” denotes the hydrogen bond between the –NH group on the N-terminal of lysine and the O atom on the N-terminal of CTLWWG. The “f” denotes the hydrogen bond between the –NH group on the lysine side chain and the S atom on the cysteine side chain. The “g” denotes the hydrogen bond between the –CαH group of phenylalanine (PHE19) and the O atom on the C-terminal of tryptophan. The “h” denotes the hydrogen bond between the –NH group on the N-terminal of tryptophan and the O atom on the C-terminal of valine. The “i” denotes the hydrogen bond between the –CαH group of leucine and the O atom on the C-terminal of valine. The “j” denotes the hydrogen bond between the –NH group on the N-terminal of valine and the O atom on the C-terminal of threonine. The “k” denotes the hydrogen bond between the –CαH group of leucine and the O atom on the C-terminal of threonine. The “l” denotes the hydrogen bond between the –CH group on the N-terminal of CTLWWG and the O atom on the N-terminal of the monomer KLVFFA. The “m” denotes the hydrogen bond between the –CαH group of alanine and the O atom on the C-terminal of glycine. The “n” denotes the hydrogen bond between the –CαH group of tryptophan and the O atom on the C-terminal of phenylalanine (PHE20). The “o” denotes the hydrogen bond between the –CαH group of the tryptophan side chain and the O atom on the C-terminal of phenylalanine (PHE20). The “p” denotes the hydrogen bond between the ortho position –CH on the phenylalanine (PHE19) benzene ring and the O atom on the C-terminal of tryptophan. The “q” denotes the hydrogen bond between the –NH group on the tryptophan side chain and the O atom on the C-terminal of valine. The “r” denotes the hydrogen bond between the –CαH group of the phenylalanine (PHE20) side chain and the C atom on the aromatic ring of tryptophan. The “s” denotes the hydrogen bond between the –CαH group of the lysine side chain and the O atom on the N-terminal of CTLWWG. | |
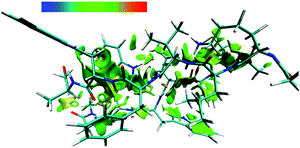 |
| Fig. 3 3D noncovalent interaction of the VDW interaction (green and yellowish green isosurface) in the parallel CTLWWG–KLVFFA dimer. | |
The 3D visible NCI of other dimers (Fig. S1 of the ESI†) shows that the dimers mainly depended on both hydrogen bonds and VDW forces to maintain their β-sheet architectures. Extensive existence of hydrogen bond networks in all dimers indicated the indispensable role of hydrogen bond interactions between two backbones in the aggregated architecture of the polypeptide dimers.69 If we put hydrogen bonds as the “bole” for propping the aggregated β-sheet structure, VDW were branches filling in the surrounding of the “bole”, these two kinds of forces complemented each other and cooperatively stabilized the dimers.
Docking poses of hexapeptides to the Aβ pentamer
We utilized molecular docking to explore the potential interaction poses of 3 hexapeptides with the Aβ pentamer. A total of top 1000 conformations were obtained for each hexapeptides (ligand) with the Aβ pentamer (acceptor). The binding conformations with the lowest binding energies (Fig. 4) indicate that all 3 hexapeptides bound to the side of the Aβ pentamer, particularly, targeting the region of L17–A21. This was obviously consistent with the above conclusion that the K16–A21 fragment of Aβ was an important binding region for the 3 hexapeptides by our quantum-chemical calculations, which was also supported by previous studies.62,64,70
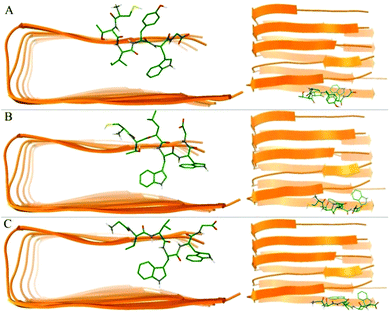 |
| Fig. 4 The best conformations of the 3 hexapeptides docked to the Aβ17–42 pentamer. (A)–(C) Indicate the best conformation of CTIYWG, CTLWWG and GTVWWG with the Aβ17–42 pentamer, respectively. | |
Binding energy of 3 hexapeptides with the Aβ pentamer
The binding energies of 3 hexapeptides with the Aβ pentamer reveal that 3 hexapeptides could spontaneously bind with the Aβ pentamer; particularly, GTVWWG exhibited the strongest binding ability with the Aβ pentamer (Table 2). A residue-based interaction energy map of 3 hexapeptides with the Aβ pentamer (Fig. 5) suggests that the 3 hexapeptides had significant interaction forces with the regions of L17–D23 and I32–V39, especially, all including the residues of F19, A21, E22, D23 and V36, which are potential target sites or regions attacked by Aβ inhibitors.
Table 2 Binding energy between 3 hexapeptides and the Aβ17–42 pentamer in the last 40 ns (kcal mol−1)
Hexapeptide |
Binding energy (kcal mol−1) |
CTIYWG |
−19.2 ± 8.3 |
CTLWWG |
−25.1 ± 15.5 |
GTVWWG |
−30.4 ± 4.2 |
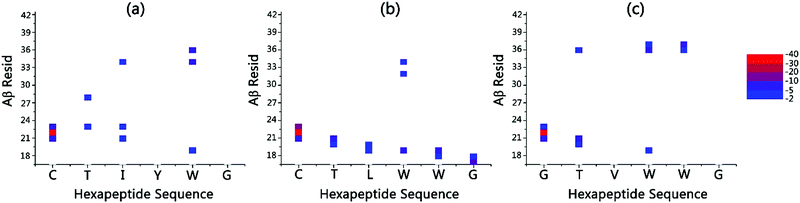 |
| Fig. 5 Residue-based interaction energy map of the 3 hexapeptides with the Aβ17–42 pentamer. | |
In particular, we noticed that the first amino acid of the hexapeptides exhibited the greatest affinity with the E22 residue of Aβ. This implied that the E22 residue was a potential key binding site by the hexapeptides, thus we could replace or modify the amino acid residue of the first site of the hexapeptides to obtain novel inhibitors with a higher affinity against Aβ aggregation.
Structural stability of Aβ pentamers
As can be seen from the final conformation (Fig. 6), although some minor twists occurred, three peptide-bound Aβ assemblies were able to well maintain their overall U-shaped β-sheet structures. The other distinct feature is that no β-sheet structure was formed for chain A of the pure Aβ pentamer, otherwise, after binding with three peptide inhibitors, these A chains of the Aβ pentamers all formed obvious β-sheet structures. Therefore, the overall stability of these Aβ pentamers binding with three peptides improved.
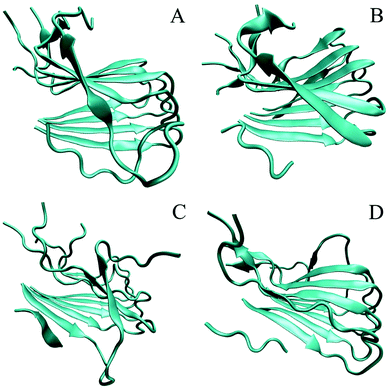 |
| Fig. 6 The conformation of MD simulations at the last frame. (A)–(D) Represent the conformation of the single Aβ17–42 pentamer, and the Aβ17–42 pentamer with CTIYWG, CTLWWG, and GTVWWG, respectively. | |
To further probe the structural characteristics of these Aβ pentamers bound by the peptide inhibitors, the time series of the two-dimensional backbone root-mean-square deviation (2D-RMSD) of the Aβ pentamer with the absence and presence of 3 hexapeptides are presented in Fig. 7. It can be seen that the RMSD of all the Aβ pentamers experienced a stable tendency in the last 40 ns. Moreover, all three hexapeptides promote the stability of the Aβ pentamers. As shown in Fig. 8, as the residue-based root-mean-square fluctuations (RMSF) in the 5 chains of the Aβ pentamer, relative to the pure Aβ pentamer, all the Aβ pentamers binding with hexapeptides generally experienced a RMSF decline on the whole.
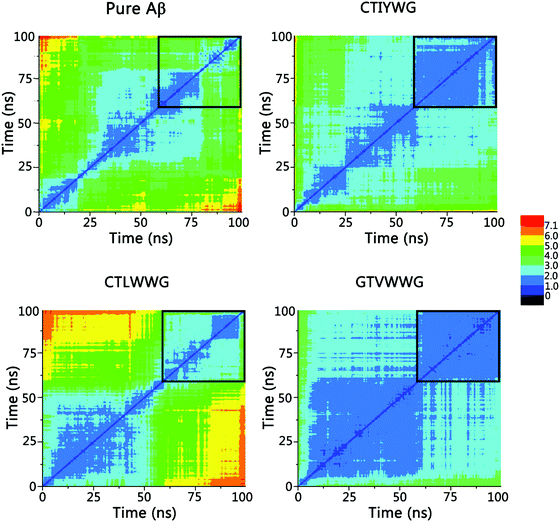 |
| Fig. 7 2D-RMSD of the backbone atoms of the Aβ17–42 pentamer. | |
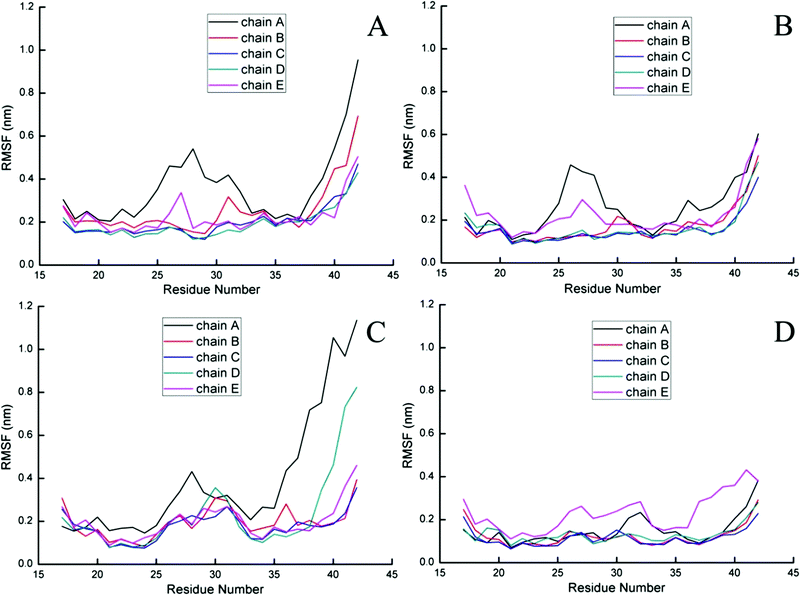 |
| Fig. 8 Residue-based RMSF of five chains of the Aβ pentamer. (A) Represents the single Aβ17–42 pentamer, and (B, C and D) represent the Aβ17–42 pentamer binding with CTIYWG, CTLWWG and GTVWWG, respectively. | |
We explored the distance and the hydrogen bonding (Table 3) between neighboring chains of the Aβ pentamer. The distance between neighboring chains of the Aβ pentamer was represented by the distance of mass centers of all Cα in the corresponding chains. The average distance in the last 40 ns (Table 3) reveals that the corresponding distances between neighboring chains of the single Aβ pentamer were almost all larger than that of all the Aβ pentamers binding with CTIYWG, CTLWWG and GTVWWG. In particular, the distance between chains A and B was distinctly reduced in the presence of the 3 inhibitors, indicating that the 3 inhibitors made the binding of chains A and B stronger. In parallel, we noticed that the H-bond number was in line with that of the tendency of the distance, i.e., the distance was larger, the H-bond number was small and vice versa. This further explained why chain A formed an obvious β-sheet, thereby making the Aβ pentamers more stable after binding with the three hexapeptides.
Table 3 Average distance and H-bond number between neighboring chains in the last 40 ns (nm)
Structure |
Parameter |
Chains A and B |
Chains B and C |
Chains C and D |
Chains D and E |
Pure Aβ17–42 pentamer |
Distance |
0.68 |
0.47 |
0.47 |
0.46 |
H-bond number |
13.6 |
24.8 |
20.1 |
22.4 |
|
Aβ17–42 pentamer with CTIYWG |
Distance |
0.38 |
0.45 |
0.43 |
0.38 |
H-bond number |
24.9 |
24.3 |
27.3 |
26.4 |
|
Aβ17–42 pentamer with CTLWWG |
Distance |
0.34 |
0.50 |
0.46 |
0.45 |
H-bond number |
14.9 |
26.7 |
19.8 |
22.2 |
|
Aβ17–42 pentamer with GTVWWG |
Distance |
0.50 |
0.46 |
0.45 |
0.47 |
H-bond number |
22.5 |
24.8 |
20.1 |
24.2 |
The extended conformation was closely related to the β-sheet secondary structure, thus we calculated the statistical percentage and probability of the extended conformation of every residue in the last 100 frames. In the Aβ pentamer, the larger percentage of the extended conformation was, the more the β-sheet structure had, the more stable Aβ pentamer became. Table 4 indicates that all 3 hexapeptides enhanced a percentage of the extended conformation in chain A of the Aβ pentamer, otherwise, there was no obvious change for the extended conformation within other chains. The probability of every residue (Fig. 9) reveals that the inhibitors enhanced the percentage of the extended conformation in fragment V18–D23 of chain A, promoting it to form the β-sheet structure with fragment V18–D23 in chain B, and finally, chain A more stably interacts with chain B. Therefore, it can be concluded that the alternation tendency of the secondary structure was consistent with that of the distance and H-bond number analyzed above, which further verified the accuracy of our results.
Table 4 Percentage (expressed as fractions) of the extended conformation in the last 100 frames
Structure |
Chain A |
Chain B |
Chain C |
Chain D |
Chain E |
Average value |
Pure Aβ pentamer |
0.212 |
0.493 |
0.587 |
0.569 |
0.448 |
0.462 |
Aβ17–42 pentamer with CTIYWG |
0.469 |
0.485 |
0.563 |
0.601 |
0.415 |
0.507 |
Aβ17–42 pentamer with CTLWWG |
0.398 |
0.499 |
0.362 |
0.378 |
0.347 |
0.397 |
Aβ17–42 pentamer with GTVWWG |
0.364 |
0.538 |
0.584 |
0.528 |
0.313 |
0.465 |
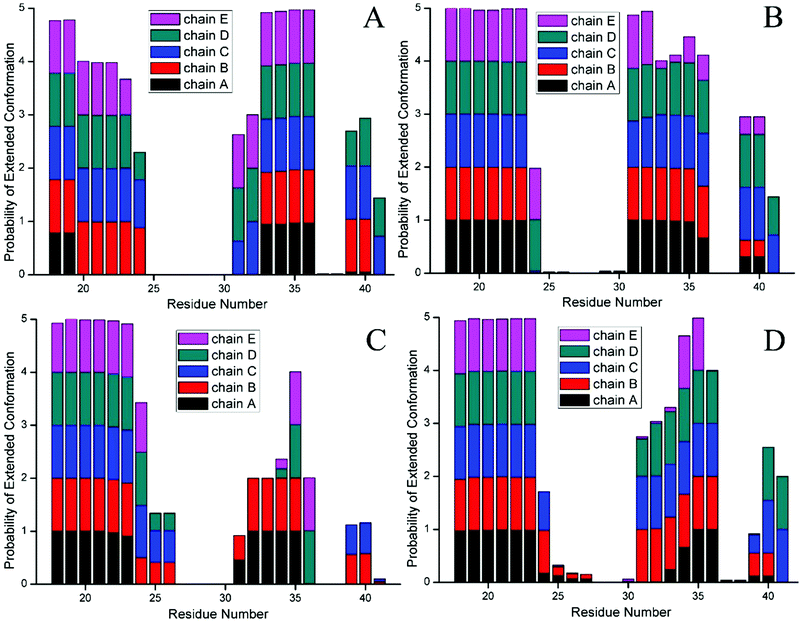 |
| Fig. 9 Percentages (expressed as fractions) of the extended conformation of every residue in the last 100 frames. (A) Represents the single Aβ17–42 pentamer, and (B)–(D) represent Aβ17–42 pentamer binding with CTIYWG, CTLWWG, and with GTVWWG, respectively. | |
The strong binding affinities of the 3 hexapeptides with the Aβ17–42 pentamer showed that the hexapeptides could spontaneously bind with the Aβ17–42 pentamer. The stable structures of the hexapeptides and the Aβ pentamer revealed that the 3 hexapeptides could competitively bind with the edge of the Aβ17–42 pentamer. Therefore, the binding energies along with the structural stability between the hexapeptides and the Aβ17–42 pentamer cooperatively demonstrated that the 3 hexapeptides could stably bind and occupy the key position or region which was indispensable for the Aβ17–42 pentamer to attract the Aβ monomer and thus to inhibit further aggregation of the Aβ17–42 pentamer.
Conclusions
In this work, we computationally studied the binding mechanism between 3 peptide inhibitors (CTLWWG, GTVWWG, and CTIYWG) and Aβ (6 Aβ-derived fragments and an Aβ17–42 pentamer) using a combination of quantum-chemical calculations, molecular docking and MD simulations. The computational results show that all 3 peptide inhibitors tend to bind strongly to the two Aβ fragments of 16KLVFFA21 and 27NKGAII33, suggesting sequence-specific binding modes (i.e. affinities, forces, and residues). All 3 peptide inhibitors strongly interact with the edge of the Aβ17–42 pentamer via electrostatic and VDW interactions. Since Aβ oligomers/fibrils grow via monomer addition to its edges, these peptides binding to the edges of the oligomers apparently block the interactive sites for fibril elongation. The peptides with a stronger binding affinity for Aβ have the better inhibition effect, consistent with our previous experimental data.42 Furthermore, we identify some important regions and residues of Aβ (e.g.16KLVFFA21, 27NKGAII33, E22, D23, L34, and V36), which can serve as potential targets for the structure-based design as Aβ inhibitors.
Undoubtedly, herein the used ssNMR structure of the Aβ17–42 pentamer by Luhrs et al.52 does not have any information on the N-termini, but we should not neglect the information on the N-termini of Aβ as many reports have done.57,71–73 Additionally, given those inhibitor sequences, further modifications by substitution or introduction of some unnatural residues in the inhibitor sequences could also improve the resistance against enzymatic degradation. This work can provide some atomic-level insights into the molecular design of effective peptide-based inhibitors against Aβ aggregation.
Acknowledgements
G. L. acknowledges financial support from the National Natural Science Foundation of China (No. 31571782 and 10901169), the Fundamental Research Funds for the Central Universities (No. CQDXWL-2014-Z009 and 106112016CDJZR235517), and the Natural Science Foundation Project of Chongqing CSTC (No. cstc2012gg-gjhz10003). J. Z. acknowledges financial support from the National Science Foundation (CAREER CBET-0952624, CBET-1510099, partial DMR-1607475), the Alzheimer Association New Investigator Research Grant (2015-NIRG-341372), and the National Natural Science Foundation of China (NSFC – 21528601).
References
- K. Blennow, M. J. de Leon and H. Zetterberg, Lancet, 2006, 368, 387–403 CrossRef CAS.
- M. P. Mattson, Nature, 2004, 430, 631–639 CrossRef CAS PubMed.
- G. M. McKhann, D. S. Knopman, H. Chertkow, B. T. Hyman, C. R. Jack, C. H. Kawas, W. E. Klunk, W. J. Koroshetz, J. J. Manly, R. Mayeux, R. C. Mohs, J. C. Morris, M. N. Rossor, P. Scheltens, M. C. Carrillo, B. Thies, S. Weintraub and C. H. Phelps, Alzheimer's Dementia, 2011, 7, 263–269 CrossRef PubMed.
- K. P. Kepp, Chem. Rev., 2012, 112, 5193–5239 CrossRef CAS PubMed.
- J. Hardy and D. Allsop, Trends Pharmacol. Sci., 1991, 12, 383–388 CrossRef CAS PubMed.
- F. G. De Felice, D. Wu, M. P. Lambert, S. J. Fernandez, P. T. Velasco, P. N. Lacor, E. H. Bigio, J. Jerecic, P. J. Acton, P. J. Shughrue, E. Chen-Dodson, G. G. Kinney and W. L. Klein, Neurobiol. Aging, 2008, 29, 1334–1347 CrossRef CAS PubMed.
- M. Jin, N. Shepardson, T. Yang, G. Chen, D. Walsh and D. J. Selkoe, Proc. Natl. Acad. Sci. U. S. A., 2011, 108, 5819–5824 CrossRef CAS PubMed.
- P. K. Dash, A. N. Moore and S. A. Orsi, Biochem. Biophys. Res. Commun., 2005, 338, 777–782 CrossRef CAS PubMed.
- M. Asai, C. Hattori, N. Iwata, T. C. Saido, N. Sasagawa, B. Szabo, Y. Hashimoto, K. Maruyama, S. Tanuma, Y. Kiso and S. Ishiura, J. Neurochem., 2006, 96, 533–540 CrossRef CAS PubMed.
- B. J. Backskai, S. T. Kajdasz, R. H. Christie, C. Carter, D. Games, P. Seubert, D. Schenk and B. T. Hyman, Nat. Med., 2001, 7, 369–372 CrossRef.
- D. J. Selkoe, Physiol. Rev., 2001, 81, 741–766 CAS.
- S. Kumar and J. B. Udgaonkar, Curr. Sci., 2010, 98, 639–656 CAS.
- T. Hard and C. Lendel, J. Mol. Biol., 2012, 421, 441–465 CrossRef PubMed.
- J. Liu, W. Wang, Q. Zhang, S. H. Zhang and Z. Yuan, Biomacromolecules, 2014, 15, 931–939 CrossRef CAS PubMed.
- H. Amijee, J. Madine, D. A. Middleton and A. J. Doig, Biochem. Soc. Trans., 2009, 37, 692–696 CrossRef CAS PubMed.
- C. Q. He, Y. C. Han, L. Y. Zhu, M. L. Deng and Y. L. Wang, J. Phys. Chem. B, 2013, 117, 10475–10483 CrossRef CAS PubMed.
- T. Takahashi, K. Ohta and H. Mihara, ChemBioChem, 2007, 8, 985–988 CrossRef CAS PubMed.
- T. Takahashi and H. Mihara, Acc. Chem. Res., 2008, 41, 1309–1318 CrossRef CAS PubMed.
- C. Cabaleiro-Lago, F. Quinlan-Pluck, I. Lynch, S. Lindman, A. M. Minogue, E. Thulin, D. M. Walsh, K. A. Dawson and S. Linse, J. Am. Chem. Soc., 2008, 130, 15437–15443 CrossRef CAS PubMed.
- C. Hilbich, B. Kisterswoike, J. Reed, C. L. Masters and K. Beyreuther, J. Mol. Biol., 1992, 228, 460–473 CrossRef CAS PubMed.
- J. P. Cleary, D. M. Walsh, J. J. Hofmeister, G. M. Shankar, M. A. Kuskowski, D. J. Selkoe and K. H. Ashe, Nat. Neurosci., 2005, 8, 79–84 CrossRef CAS PubMed.
- M. A. Findeis, Biochim. Biophys. Acta, Mol. Basis Dis., 2000, 1502, 76–84 CrossRef CAS.
- C. Soto, E. M. Sigurdsson, L. Morelli, R. A. Kumar, E. M. Castano and B. Frangione, Nat. Med., 1998, 4, 822–826 CrossRef CAS.
- G. B. Zhang, M. J. Leibowitz, P. J. Sinko and S. Stein, Bioconjugate Chem., 2003, 14, 86–92 CrossRef CAS PubMed.
- L. O. Tjernberg, J. Naslund, F. Lindqvist, J. Johansson, A. R. Karlstrom, J. Thyberg, L. Terenius and C. Nordstedt, J. Biol. Chem., 1996, 271, 8545–8548 CrossRef CAS PubMed.
- T. Doi, S. Kamioka, S. Shimazu and T. Takahashi, Org. Lett., 2008, 10, 817–819 CrossRef CAS PubMed.
- E. A. Fradinger, B. H. Monien, B. Urbanc, A. Lomakin, M. Tan, H. Li, S. M. Spring, M. M. Condron, L. Cruz, C. W. Xie, G. B. Benedek and G. Bitan, Proc. Natl. Acad. Sci. U. S. A., 2008, 105, 14175–14180 CrossRef CAS PubMed.
- H. Y. Li, B. H. Monien, E. A. Fradinger, B. Urbanc and G. Bitan, Biochemistry, 2010, 49, 1259–1267 CrossRef CAS PubMed.
- J. P. Guo, T. Arai, J. Miklossy and P. L. McGeer, Proc. Natl. Acad. Sci. U. S. A., 2006, 103, 1953–1958 CrossRef CAS PubMed.
- E. Andreetto, L. M. Yan, M. Tatarek-Nossol, A. Velkova, R. Frank and A. Kapurniotu, Angew. Chem., Int. Ed., 2010, 49, 3081–3085 CrossRef CAS.
- N. Rezaei-Ghaleh, E. Andreetto, L. M. Yan, A. Kapurniotu and M. Zweckstetter, PLoS One, 2011, 6, e20289 CAS.
- J. N. Buxbaum, Z. Ye, N. Reixach, L. Friske, C. Levy, P. Das, T. Golde, E. Masliah, A. R. Roberts and T. Bartfai, Proc. Natl. Acad. Sci. U. S. A., 2008, 105, 2681–2686 CrossRef CAS PubMed.
- B. I. Giasson, M. S. Forman, M. Higuchi, L. I. Golbe, C. L. Graves, P. T. Kotzbauer, J. Q. Trojanowski and V. M. Y. Lee, Science, 2003, 300, 636–640 CrossRef CAS PubMed.
- X. Yu, Y. Luo, P. Dinkel, J. Zheng, G. H. Wei, M. Margittai, R. Nussinov and B. Y. Ma, J. Biol. Chem., 2012, 287, 14950–14959 CrossRef CAS PubMed.
- C. T. Middleton, P. Marek, P. Cao, C. C. Chiu, S. Singh, A. M. Woys, J. J. de Pablo, D. P. Raleigh and M. T. Zanni, Nat. Chem., 2012, 4, 355–360 CrossRef CAS PubMed.
- K. Hartman, J. R. Brender, K. Monde, A. Ono, M. L. Evans, N. Popovych, M. R. Chapman and A. Ramamoorthy, PeerJ, 2013, 1, e5 Search PubMed.
- J. M. Souza, B. I. Giasson, V. M. Y. Lee and H. Ischiropoulos, FEBS Lett., 2000, 474, 116–119 CrossRef CAS PubMed.
- P. Westermark, Z. C. Li, G. T. Westermark, A. Leckstrom and D. F. Steiner, FEBS Lett., 1996, 379, 203–206 CrossRef CAS PubMed.
- B. Y. Ma and R. Nussinov, J. Mol. Biol., 2012, 421, 172–184 CrossRef CAS PubMed.
- R. Nelson, M. R. Sawaya, M. Balbirnie, A. O. Madsen, C. Riekel, R. Grothe and D. Eisenberg, Nature, 2005, 435, 773–778 CrossRef CAS PubMed.
- E. Gazit, FEBS J., 2005, 272, 5971–5978 CrossRef CAS PubMed.
- Q. M. Wang, G. Z. Liang, M. Z. Zhang, J. Zhao, K. Patel, X. Yu, C. Zhao, B. R. Ding, G. Zhang, F. M. Zhou and J. Zheng, ACS Chem. Neurosci., 2014, 5, 972–981 CrossRef CAS PubMed.
- Y. Zhao and D. G. Truhlar, Theor. Chem. Acc., 2008, 120, 215–241 CrossRef CAS.
- Y. Zhao and D. G. Truhlar, Acc. Chem. Res., 2008, 41, 157–167 CrossRef CAS PubMed.
-
M. J. Frisch, G. W. Trucks, H. B. Schlegel, G. E. Scuseria, M. A. Robb, J. R. Cheeseman, G. Scalmani, V. Barone, B. Mennucci, G. A. Petersson, H. Nakatsuji, M. Caricato, X. Li, H. P. Hratchian, A. F. Izmaylov, J. Bloino, G. Zheng, J. L. Sonnenberg, M. Hada, M. Ehara, K. Toyota, R. Fukuda, J. Hasegawa, M. Ishida, T. Nakajima, Y. Honda, O. Kitao, H. Nakai, T. Vreven, J. A. Montgomery, Jr., J. E. Peralta, F. Ogliaro, M. Bearpark, J. J. Heyd, E. Brothers, K. N. Kudin, V. N. Staroverov, R. Kobayashi, J. Normand, K. Raghavachari, A. Rendell, J. C. Burant, S. S. Iyengar, J. Tomasi, M. Cossi, N. Rega, N. J. Millam, M. Klene, J. E. Knox, J. B. Cross, V. Bakken, C. Adamo, J. Jaramillo, R. Gomperts, R. E. Stratmann, O. Yazyev, A. J. Austin, R. Cammi, C. Pomelli, J. W. Ochterski, R. L. Martin, K. Morokuma, V. G. Zakrzewski, G. A. Voth, P. Salvador, J. J. Dannenberg, S. Dapprich, A. D. Daniels, Ö. Farkas, J. B. Foresman, J. V. Ortiz, J. Cioslowski and D. J. Fox, Gaussian 09, Gaussian, Inc., Wallingford CT, 2009 Search PubMed.
- E. R. Johnson, S. Keinan, P. Mori-Sanchez, J. Contreras-Garcia, A. J. Cohen and W. T. Yang, J. Am. Chem. Soc., 2010, 132, 6498–6506 CrossRef CAS PubMed.
- J. Contreras-Garcia, E. R. Johnson, S. Keinan, R. Chaudret, J. P. Piquemal, D. N. Beratan and W. T. Yang, J. Chem. Theory Comput., 2011, 7, 625–632 CrossRef CAS PubMed.
- R. Chaudret, B. de Courcy, J. Contreras-Garcia, E. Gloaguen, A. Zehnacker-Rentien, M. Mons and J. P. Piquemal, Phys. Chem. Chem. Phys., 2014, 16, 9876–9891 RSC.
- R. F. W. Bader and H. Essen, J. Chem. Phys., 1984, 80, 1943–1960 CrossRef CAS.
- T. Lu and F. W. Chen, J. Comput. Chem., 2012, 33, 580–592 CrossRef CAS PubMed.
- W. Humphrey, A. Dalke and K. Schulten, J. Mol. Graphics, 1996, 14, 33–38 CrossRef CAS PubMed.
- T. Luhrs, C. Ritter, M. Adrian, D. Riek-Loher, B. Bohrmann, H. Doeli, D. Schubert and R. Riek, Proc. Natl. Acad. Sci. U. S. A., 2005, 102, 17342–17347 CrossRef CAS PubMed.
- G. M. Morris, R. Huey, W. Lindstrom, M. F. Sanner, R. K. Belew, D. S. Goodsell and A. J. Olson, J. Comput. Chem., 2009, 30, 2785–2791 CrossRef CAS PubMed.
- L. Kale, R. Skeel, M. Bhandarkar, R. Brunner, A. Gursoy, N. Krawetz, J. Phillips, A. Shinozaki, K. Varadarajan and K. Schulten, J. Comput. Phys., 1999, 151, 283–312 CrossRef CAS.
- M. S. Lee, M. Feig, F. R. Salsbury, Jr. and C. L. Brooks, 3rd, J. Comput. Chem., 2003, 24, 1348–1356 CrossRef CAS.
- T. Hou, J. Wang, Y. Li and W. Wang, J. Chem. Inf. Model., 2011, 51, 69–82 CrossRef CAS PubMed.
- M. Baram, Y. Atsmon-Raz, B. Ma, R. Nussinov and Y. Miller, Phys. Chem. Chem. Phys., 2016, 18, 2330–2338 RSC.
- J. P. Colletier, A. Laganowsky, M. Landau, M. L. Zhao, A. B. Soriaga, L. Goldschmidt, D. Flot, D. Cascio, M. R. Sawaya and D. Eisenberg, Proc. Natl. Acad. Sci. U. S. A., 2011, 108, 16938–16943 CrossRef CAS PubMed.
- M. R. Sawaya, S. Sambashivan, R. Nelson, M. I. Ivanova, S. A. Sievers, M. I. Apostol, M. J. Thompson, M. Balbirnie, J. J. W. Wiltzius, H. T. McFarlane, A. O. Madsen, C. Riekel and D. Eisenberg, Nature, 2007, 447, 453–457 CrossRef CAS PubMed.
- P. P. Bose, U. Chatterjee, C. Nerelius, T. Govender, T. Norstrom, A. Gogoll, A. Sandegren, E. Gothelid, J. Johansson and P. I. Arvidsson, J. Med. Chem., 2009, 52, 8002–8009 CrossRef CAS PubMed.
- J. T. Jarrett, E. P. Berger and P. T. Lansbury, Biochemistry, 1993, 32, 4693–4697 CrossRef CAS PubMed.
- J. J. Balbach, Y. Ishii, O. N. Antzutkin, R. D. Leapman, N. W. Rizzo, F. Dyda, J. Reed and R. Tycko, Biochemistry, 2000, 39, 13748–13759 CrossRef CAS PubMed.
- T. S. Burkoth, T. L. S. Benzinger, D. N. M. Jones, K. Hallenga, S. C. Meredith and D. G. Lynn, J. Am. Chem. Soc., 1998, 120, 7655–7656 CrossRef CAS.
- P. N. Cheng, R. Spencer, R. J. Woods, C. G. Glabe and J. S. Nowick, J. Am. Chem. Soc., 2012, 134, 14179–14184 CrossRef CAS PubMed.
- M. Ahmed, J. Davis, D. Aucoin, T. Sato, S. Ahuja, S. Aimoto, J. I. Elliott, W. E. Van Nostrand and S. O. Smith, Nat. Struct. Mol. Biol., 2010, 17, 561–567 CAS.
- G. Bitan, M. D. Kirkitadze, A. Lomakin, S. S. Vollers, G. B. Benedek and D. B. Teplow, Proc. Natl. Acad. Sci. U. S. A., 2003, 100, 330–335 CrossRef CAS PubMed.
- Q. Wang, G. Liang, M. Zhang, J. Zhao, K. Patel, X. Yu, C. Zhao, B. Ding, G. Zhang, F. Zhou and J. Zheng, ACS Chem. Neurosci., 2014, 5, 972–981 CrossRef CAS PubMed.
- P. Hohenberg and W. Kohn, Phys. Rev. [Sect.] B, 1964, 136, B864–B871 CrossRef.
- T. P. Knowles, A. W. Fitzpatrick, S. Meehan, H. R. Mott, M. Vendruscolo, C. M. Dobson and M. E. Welland, Science, 2007, 318, 1900–1903 CrossRef CAS PubMed.
- L. O. Tjernberg, D. J. E. Callaway, A. Tjernberg, S. Hahne, C. Lilliehook, L. Terenius, J. Thyberg and C. Nordstedt, J. Biol. Chem., 1999, 274, 12619–12625 CrossRef CAS PubMed.
- Y. Atsmon-Raz and Y. Miller, ACS Chem. Neurosci., 2016, 7, 46–55 CrossRef CAS PubMed.
- Y. Miller, B. Ma and R. Nussinov, J. Am. Chem. Soc., 2011, 133, 2742–2748 CrossRef CAS PubMed.
- Y. Miller, B. Ma, C. J. Tsai and R. Nussinov, Proc. Natl. Acad. Sci. U. S. A., 2010, 107, 14128–14133 CrossRef CAS.
Footnotes |
† Electronic supplementary information (ESI) available. See DOI: 10.1039/c6cp07341g |
‡ These authors contributed equally to this work. |
|
This journal is © the Owner Societies 2017 |
Click here to see how this site uses Cookies. View our privacy policy here.