DOI:
10.1039/C7RA00698E
(Review Article)
RSC Adv., 2017,
7, 17217-17226
Metabolomic applications in hepatocellular carcinoma: toward the exploration of therapeutics and diagnosis through small molecules
Received
17th January 2017
, Accepted 24th February 2017
First published on 20th March 2017
Abstract
Hepatocellular carcinoma (HCC), a complex public health issue that is the most common primary hepatic malignancy, remains the highest incidence in developing countries and is showing sustained growth across the developed world. HCC is asymptomatic in nature; in the clinic, the low sensitivity of tumor detection is insufficient to characterize its category, risk prediction, or morbidity progression and to direct reasonable preventive measures. Therefore, HCC is usually diagnosed at a terminal cancer stage, and interventions are not effective in time to help patients. Thus, early diagnosis before onset and better comprehension of underlying mechanisms in HCC for molecular targets are urgently needed. Currently, the emerging field of metabolomics, involving high-throughput identification and measurement of metabolites in biological fluids, is a powerful method for appraising disease mechanisms and for biomarker discovery by elucidating the framework of the general dissection of HCC. In this article, we first provided the latest understanding of HCC on risk factors, diagnosis and treatment, and then highlighted a summary of metabolomics research, which is a vital field in HCC. We also discuss the current state of HCC biomarkers and future challenges in the digital and information era of medicine.
Introduction
Liver cancer is one of the leading causes of cancer death worldwide, after lung and stomach cancer; it is also the fifth leading reason in cancer diagnosis among men and the seventh among women.1,2 The incidence of liver cancer was as high as 782
000 new cases in 2012. The mortality of primary liver cancer is abnormally increasing at a surprisingly high rate due to the incomplete and unfavorable prognosis of primary liver malignancy.3 According to records, HCC is one of the most common types of primary liver cancers, representing up to 90% of all primary hepatic malignancies.4 Its incidence tends to be maximal at an age of about 70 years and is comparatively infrequent before 40 years of age. HCC is a crucial livelihood issue in high risk areas such as East Asia, Sub-Saharan Africa and Melanesia and even in the United States and Europe, primarily due to high rates of infection with HCV virus, different environmental pathogens, obesity epidemics, fat hepatitis, and other risk factors.5–7 The high morbidity rate of this cancer related to the late diagnosis and ineffective treatment decisions is primarily relying on the physiological histology of the liver, limited serological markers, and incomplete clinical presentation. It is essential to detect HCC in early stages to enable successful treatment and management of patients. Currently, in clinical applications, insufficiently sensitive or specific measurements are used to screen early disease, detect disease progression, and evaluate treatment response. Hence, more specific and reliable biomarkers for the early diagnosis and prognosis of HCC are urgently needed.
The emerging field of metabolomics has increased the hope of discovering novel biomarkers of HCC to ameliorate asymptomatic screening and track cancer recurrence and response to treatment. Metabolomics provides a comprehensive chemical fingerprint of cell metabolism, which clarifies the physiological and pathological states of an organism. Therefore, metabolomics provides a powerful platform to obtain insight into the molecular mechanisms of HCC. Not only are metabolites gene or protein end expression products, but they are also a consequence of the mutual relationship between the genome and the internal environment.8,9 It is necessary to move from small, research-oriented studies to validation studies involving a large number of disease sufferers.10 The recent development of metabolomics to identify biomarkers of HCC will be greatly advantageous to patients and health care systems. Profound comprehension of the biological metabolic pathways of the overall disorder and useful biomarkers by a metabolomics approach could offer valuable insight into the mechanism of HCC.11 In the current review, we present reasons for the growing need of biomarkers of HCC and for new understanding of HCC; we then pay particular attention to novel techniques used in metabolomics. Importantly, we provide an overview of the studies that have used metabolomics to reveal the pathological mechanisms of HCC. Finally, we probe the future directions and challenges of this research.
Risk factors, diagnosis and treatment
According to previous studies, various risk factors have been related to HCC morbidity, including alcoholism, hepatitis B virus (HBV), hepatitis C virus (HCV), non-alcoholic fatty liver disease, obesity, diabetes mellitus, cirrhosis, aflatoxin, Wilson's disease and other blood diseases. The discrepancy of the geographical distribution of HCC is due to different degrees of hepatitis virus transmission. In Africa and East Asia, chronic HBV infection is the main risk factor, while in Western countries, the most familiar risk factor related to HCC is HCV infection. HBV may combine with the host genome, resulting in genetic abnormalities, transforming of HCC-related gene expression and the expression of active proteins which may stimulate the expression of HCC-related proteins; HBV is associated with specific proteins that sensitize signaling pathways and lead to epigenetic modifications.12 Patients with <500 copies per mL of HBV DNA are in danger of postoperative HBV reactivation, which is regarded as a risk factor for HCC recurrence.13 A recent study found that accumulated risk of HCC was higher in people with high titers of HCV RNA than in subjects with low titers, which indicates that HCV RNA titer can be seen as an independent risk factor for the incidence of HCC.14 Compared with other HCV genotypes, subjects infected with HCV genotype 3 are at high risk for HCC, end-stage liver disease and liver-related death.15 Diabetes and cirrhosis have been associated with incremental risk of HCC development after treatment of sustained virological response. Patients without cirrhosis have a low chance of developing HCC after sustained virological response compared to patients who have diabetes and cirrhosis.16 As an independent risk factor for liver fibrosis/cirrhosis and HCC development, obesity induces elevated tissue inflammation together with increased hepatic stellate cell-derived tenascin-C and toll-like receptor 4 expression.17 It has been reported that alcohol is the second leading cause of liver disease; in 4% to 5% of cases, fatty liver hepatitis evolves into HCC.18 Due to fungal contamination in hot and humid environments, high exposure to aflatoxins existing naturally in maize, corn and rice leads to chronic toxicity and causes high incidences of HCC by obstructing the DNA of hepatic cells and causing mutation of tumor suppressor genes.19 Infection with the species Schistosoma mansoni, which is associated with risk of developing HCC, is already regarded as a possible human carcinogen in patients infected with HBV and HCV.20 In addition to occupational exposure and growth factors,21,22 genetic factors such as HLA-DRB1 allele polymorphisms, tumor necrosis factor-alpha-308G/A beta 1 polymorphisms, mRNA expression and other genetic factors are attracting increasing attention from researchers in modern medical environments.23–26
Serum tests, diagnostic imaging and histology can be beneficial to the clinical diagnosis of HCC. Currently, liver biopsy is unnecessary in some clinical cases where the tumour diameter exceeds 1 cm and the characteristics of typical imaging, such as dynamic computed tomography scans and magnetic resonance, demonstrate relatively strong adherence to the latest consensus conferences and practice guidelines. In addition, identifying tumours which increase more strongly in the arterial stage than the adjacent liver tissue and present the opposite effect in the venous phase has been advocated as a method to accurately diagnose HCC. However, the abovementioned diagnosis rules are not suitable for all patients, except for patients identified as having cirrhosis or HBV chronic hepatitis. Liver biopsy is still required for the diagnosis of patients who also present with cirrhosis and atypical histopathology or people without typical symptoms of liver disease. Previously, identifying liver cancer in high risk patients depended on the detection of serum α-fetoprotein levels;27 this is being used less often because the sensitivity is so limited that the high blood levels of α-fetoprotein observed in many other relevant liver diseases are similar to those seen in HCC.28,29 To decrease the variability of liver injury diagnoses and normalize the study of typical imaging information, the Liver Imaging-Reporting and Data System (LI-RADS) has been proposed by the American College of Radiology for periodic classification; it classifies nodules in five categories as definitively benign, probably benign, intermediate probability of being HCC, probably HCC, and definitively HCC by radiologic characteristics, diseased region diameters or other abnormal behavior.30 It has been found that LI-RADS category 4 and category 5 possess high sensitivity and specificity for the diagnosis of HCC; the LR-5 criteria show especially good positive predictive value in diagnosing HCC by gadoxetate disodium-enhanced magnetic resonance, but use of the LR-4 criteria is restricted to a certain extent.31,32 Further reduction of the complexity and management of imaging features in high-risk HCC patients by different organizations may be indispensable.33
Tumor staging plays an important role in directing and deciding on further treatment. Unfortunately, most patients who present with advanced HCC symptoms at diagnosis are not candidates for therapy. Currently, the Barcelona Clinic Liver Cancer (BCLC) classification is the most valid and most frequently used system applied to clinical HCC patients.34 Utilizing the tumor stage, liver function reserve, and performance status, the BCLC treatment strategy is commonly accepted as a guideline for appropriate treatment of HCC. Based on the different stages of cancer, there are several main therapeutic options, including surgical resection, percutaneous ethanol injection (PEI), radiofrequency ablation (RFA), transarterial chemoembolization (TACE), radioactive embolism, and systemic targeted agents.35 In early stage HCC, therapeutic options such as surgical resection and liver transplantation are the predominant treatments. Under the conditions of single nodules, no cirrhosis and normal liver function, surgical resection is the appropriate treatment.36 In addition, ablation is deemed a good option at early HCC stages. As alternative therapies in the clinic, percutaneous ethanol injection and radioactive embolism are adopted for some patients who cannot undergo safe resection due to the combined state of liver lesions and severe tumors. Therapy with PEI and RFA is an available choice for patients who are identified to have small tumours and who cannot undergo an operation or liver transplant.37 Ideal treatment for patients in the intermediate stage of HCC is TACE, which is also pivotal to palliative treatment and is a standard treatment option for the asymptomatic multinodular disease group.38 For terminal cancer sufferers, adoption of combined treatment with sorafenib or the best available chemotherapy care should be considered.39 Due to previous research progress in HCC, the short-term survival rate of HCC has increased; however, the disease still represents a pivotal problem, as the pathological mechanisms of HCC are an inexplicable and intricate process that is closely related to early detection and treatment for patients (Fig. 1).
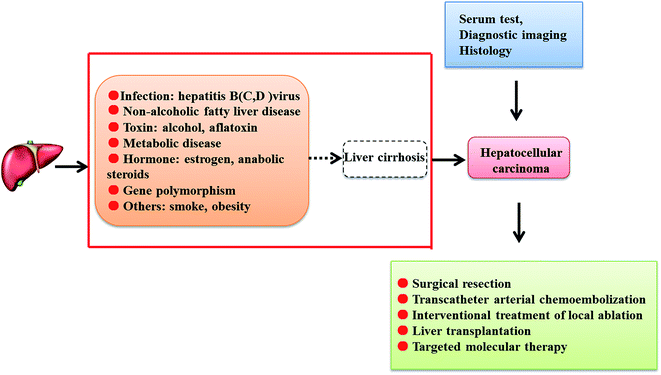 |
| Fig. 1 Common risk factors, diagnostic methods and treatment of hepatocellular carcinoma. The dashed line “……” indicates risk factors that can directly lead to cirrhosis, such as viral hepatitis, fatty liver disease, metabolic diseases and alcoholic liver. | |
Metabolomics technologies
Metabolomics refers to the comprehensive profiling of all low molecular weight compounds in a biological system. Metabolic profiling, as a generic term containing “metabolomics”, involves research of the integral metabolism of physiological responses, drugs and disease stimuli;40 “metabolomics” attempts to identify and quantify all the small molecules in biological fluid samples to build a novel bridge between genotype and phenotype.41 Currently, nuclear magnetic resonance spectroscopy (NMR), liquid chromatography-mass spectrometry (LC-MS) and gas chromatography-mass spectrometry (GC-MS) metabolic techniques are widely used analysis methods.42 NMR spectroscopy was applied in many early metabolomics studies. Currently, chromatographic techniques, including GC and LC combined with MS, are also frequently used;43 capillary electrophoresis-MS and Fourier transform spectrometry are also emerging as major spectroscopic techniques utilized in metabolomics analysis44,45 (Fig. 2). NMR shows lower sensitivity while samples are in the intact state; NMR spectral profiles have been systematically classified so that metabolites can be more directly identified.46–48 Compared with NMR-based metabolomics, MS-based metabolomics possesses higher sensitivity; thus, generally, large numbers of small molecules can be surveyed. However, this method has a high chance of generating technical artifacts along with high uncertainty of the measurements. GC-MS, a highly sensitive, repeatable and cost-efficient MS analysis method, involves time-consuming sample preparation, resulting in degradation during the operation and metabolite identification.49,50 The challenge of LC-MS is that requires smaller amounts of sample than GC-MS in the overall preparation process, including extraction, drying, and concentration. In general, the different metabolomics methods have both merits and drawbacks; MS requires more complicated biological sample extraction methods, and NMR involves the application of larger sample volumes.51,52 However, both methods can enable identification of metabolites to deepen the understanding of a phenotype or disease. A combination of different metabolomics technique approaches could enable acquisition of comprehensive and sufficient metabolite information from intricate biological samples.53
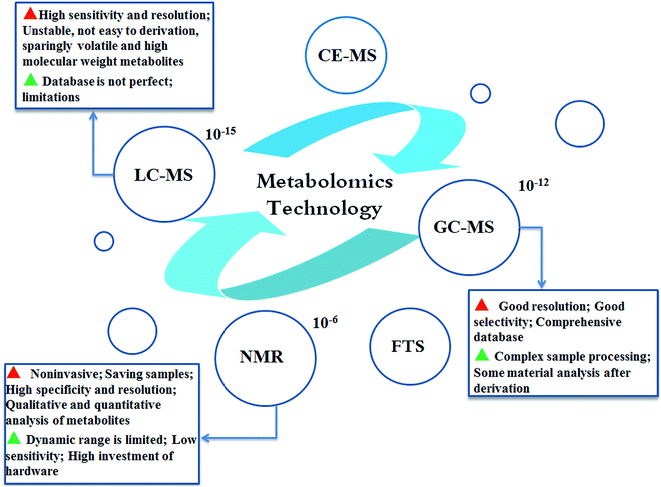 |
| Fig. 2 General technologies used in metabolomics analysis and the advantages and disadvantages of commonly used techniques such as NMR, LC-MS and GC. CE-MS indicates capillary electrophoresis-MS and FTS indicates Fourier transform spectrometry. “ ” = advantages, “ ” = disadvantages, 10−X = sensitivity. | |
Introduction of metabolomics to hepatocellular carcinoma
Small changes caused by external stimuli and internal metabolic responses in living systems can give rise to large changes in metabolite levels; the metabolome can be deemed the magnifying glass of the fluctuation of a biological system.54 Compared with other “omics”, the advantages of metabolomics are its high level of sensitivity and its capacity to analyse relatively fewer metabolites for research, in comparison to large numbers of complicated corresponding genes or mRNA molecules. In clinical practice, screening metabolite changes in disease populations has become a significant method to identify critical indicators.55,56 A small amount of biological fluid may contain countless metabolites, which enables functional characterization of the metabolic situation. Based on the accumulation of knowledge found in databases, distinct metabolic disturbances in HCC patients with biological interpretation can be fully demonstrated by metabolite profiles. Latent characteristics of metabolomics in HCC clinical practice include biomarker discovery and validation, disease-related target molecule discovery, treatment decisions, and patient monitoring.57,58 By offering biochemical profiling to provide insight into the biological characteristics of HCC, the novel and rapidly developing omics technology is already promising for researching HCC biomarkers in metabolites, which contributes to superior clinical trial design and distinguishes high risk groups at earlier stages of disease for personalized medicine exploration59 (Fig. 3). The advent of the “HCC-OMICS” era is a welcome development in the effort to reliably identify biomarkers for fully understanding pathological mechanisms and early molecular diagnosis so that patients can receive appropriate treatment.
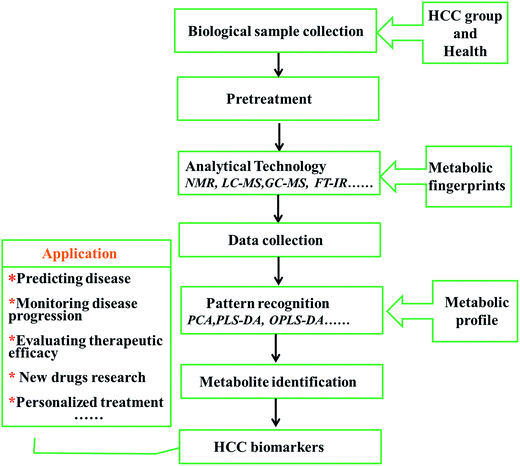 |
| Fig. 3 A procedural chart of metabolomics analysis for HCC biomarker discovery and major applications of HCC biomarkers. PCA, principal component analysis; PLS-DA, partial least squares-discriminate analysis; OPLS-DA, orthogonal partial least squares-discriminate analysis. | |
Metabolomics for the study of hepatocellular carcinoma
Biomarkers have distinct characteristics that can be used to objectively illustrate and clarify normal or abnormal physiological states, pathogenic processes and responses to therapeutic drug intervention by dissecting biomolecules such as DNA, RNA, proteins, peptides, and other modified molecules.60 In clinical practice, numerous metabolic-based biomarkers have been reported for the diagnosis and prognosis of HCC.61 Tumor occurrence and development is associated with metabolic implications. Currently, increasing evidence reveals that metabolism change is not only a cause but is also a result of carcinogenesis in tumor cells, which require large amounts of energy and substrates for nucleotide and lipid synthesis and rapid protein transport.62 It has been reported that glycolysis in tumor cells, including HCC, is a source of substrates in the pentose phosphate pathway for nucleotide synthesis instead of providing energy in the form of ATP.63,64 Essentially, tumor formation is characterized by maximal proliferation of cell components at the cost of energy generation (Fig. 4). A study was conducted to examine the metabolic functions of hypoxia-inducible factors, which play a key role in oxygen-deprived environments, even in HCC tumour models. Metabolic profiling showed that 4-hydroxyproline appeared to be a regulating target in low oxygen survival of wild type cells, while fructose was a regulating target in HIF deficient cells.65
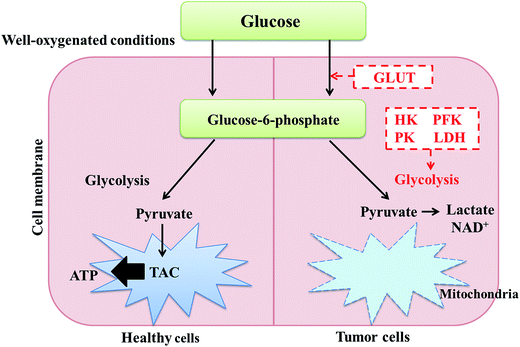 |
| Fig. 4 Comparison of glucose metabolisms of tumor cells and normal cells. In the case of sufficient oxygen, the tumor cells supply the required energy by the glycolysis pathway. Red text represents increased activity. GLUT, glucose transporter; HK, hexokinase; PFK, phosphofructokinase; PK, pyruvate kinase; LDH, lactic dehydrogenase; TAC, tricarboxylic acid cycle. | |
Emerging biomarker discovery in HCC is still a thorny issue, mainly due to the inhomogeneity of the clinical symptoms of cancer and the pathological changes resulting from potential pathogenic factors, such as cirrhosis, chronic inflammation and fatty liver disease. HCC is caused by hepatocytes consisting of liver parenchyma; it arises from liver cirrhosis in 80% of clinical cases.66 As a major cause of HCC, cirrhosis is the result of chronic liver injury, which leads to hepatocyte regeneration and results in abnormal structural nodules with peripheral fibrosis.67 A GC-TOFMS-based approach has been put into practice together with Random Forest (RF) analysis to discriminate metabolic characteristics in the progression of hepatocellular carcinogenesis. Compared with healthy controls (NC), hepatitis B virus (HBV) and liver cirrhosis (LC) serum samples, metabolic disorders in hepatocellular carcinogenesis are related to energy metabolism, macromolecular synthesis, and protection of tumor cells from oxidative stress.68 In the precancerous period of HCC, there is a transformation from carbohydrate metabolism to lipid and amino acid metabolism, while the severity of inflammation in cirrhosis increases by further metabolic pathways; these different metabolites have been studied. The most significant alterations in the levels of D-glucose and D-mannitol were observed in the HCC development stage, which elucidates the relationship between chronic inflammation and the pathogenesis of HCC.69 Metabolic profiling of high-risk individuals and HCC was applied to detect new biomarkers in serum with high sensitivity and specificity for the early diagnosis of HCC using multi-analysis of serum metabolites.70 A study identified useful biomarkers that indicate branched-chain amino acid (BCAA) metabolism imbalances associated with HCC onset. Compared with LC patients, HCC patients had significant fluctuations of glutamic acid, citric acid, lactic acid, valine, isoleucine, leucine, alpha tocopherol, cholesterol, and sorbose levels.71 Inspecting metabolic changes with multiple metabolomics platforms in the assessment of HCC may enable the determination of its pathogenesis mechanism. GC/MS and UPLC/MS-MS analysis of all serum metabolites of healthy controls and HCV patients showed that increased levels of 12-hydroxyeicosatetraenoic acid, (12-HETE), 15-HETE, sphingosine, xanthine, the amino acids serine, glycine and aspartate, acylcarnitines, and some metabolites related to γ-glutamyl oxidative stress indicate abnormal amino acid biosynthesis, cell regulation, and neutralization reactions as well as eicosanoid pathways occurring in the metabolism of HCC patients.72 In addition, 32 potential biomarkers have been identified in the serum of HCC patients, LC patients and healthy volunteers by NMR and LC/MS-based methods combined with randomized forest (RF) analysis; HCC can also be fully detected in patients whose AFP values are lower than 20 ng mL−1. Metabolic disturbances induced by HCC were found to be involved in ketone biosynthesis, the citric acid cycle, phospholipid metabolism, sphingolipid metabolism, fatty acid oxidation, amino acid catabolism and bile acid metabolism in HCC patients.73 Abnormal lipid metabolism regulation may forecast progression of the disease pathology to HCC in metabolic syndrome patients.74 Using a steroid hormone metabolomics method based on LC-MS combined with logistic regression analysis, epitestosterone and allotetrahydrocortisol were found to be altered very early in this disease; this method displayed excellent diagnostic capability, with the most highly represented alterations being severe turbulence of the steroid hormone network and greatly decreased urinary steroid hormone levels.75 It has been found that specific AAs, such as phenylalanine, tyrosine, glutamate, and tryptophan, as well as kynurenine and biogenic amines, may indicate HCC development.76 Aspartate metabolism is a particularly important and differentiable metabolic pathway of HCC in comparison with non-tumor livers, according to NMR and MS studies based on the metabolomics of human tissue extracts.77 Regulating the Wnt signaling pathway and disrupting several metabolites, including multiple acyl-lysophosphatidylcholine metabolites related to HCC, is necessary to clarify the pathophysiological process from nonalcoholic fatty liver disease to HCC.78 The development of metabolomics methods based on liquid chromatography-hybrid triple quadrupole linear ion trap mass spectrometry (LC-QTRAP/MS) and capillary electrophoresis-time of flight mass spectrometry (CE-TOF/MS) as well as modified k-TSP algorithms and a “serum biomarker model” including tryptophan, glutamine, and 2-hydroxybutyric acid is beneficial for the differential discovery of metabolites to understand the biological mechanisms of HCC79–82 (Table 1).
Table 1 Metabolomics applications in hepatocellular carcinoma
Biological specimens |
Technological platform used |
Main changing small molecules |
Main relevant metabolism |
Serum68 |
GC-TOFMS |
Phenylalanine, malic acid, 5-methoxytryptamine, palmitic acid, asparagine and β-glutamate |
Energy metabolism, macromolecular synthesis, oxidative stress |
Cells69 |
GC-MS |
D-Glucose and D-mannitol |
Lipid and amino acid metabolism |
Serum70 |
HPLC-MS |
Xanthine, uric acid, cholyglycine, D-leucic acid, 3-hydroxycapric acid, arachidonyl lysolecithin, dioleoylphosphatidylcholine |
Purine catabolism lipid metabolism |
Plasma71 |
GC-MS |
Glutamic acid, citric acid, lactic acid, valine, isoleucine, leucine, alpha tocopherol, cholesterol, and sorbose |
Branched-chain amino acid metabolism |
Serum72 |
GC/MS UPLC/MS-MS |
12-Hydroxyeicosatetraenoic acid, (12-HETE), 15-HETE, sphingosine, xanthine, amino acids serine, glycine, aspartate, acylcarnitines |
Amino acid biosynthesis, cell regulation, neutralization reaction, eicosanoid pathways |
Serum73 |
NMR RPLC-MS RPLC-MS |
Formate, tyrosine, ascorbate, oxaloacetate, carnitine, phenylalanine, lysophosphatidylcholines, phosphatidylcholines, C16 sphinganine |
Ketone biosynthesis, citric acid cycle, phospholipid metabolism, sphingolipid metabolism, fatty acid oxidation, amino acid catabolism and bile acid metabolism |
Serum74 |
LC-MS |
Triglycerides, cholesterol, and fatty acids |
Lipid metabolism |
Plasma75 |
LC-MS |
Epitestosterone, allotetrahydrocortisol |
Steroid hormone network, steroid hormone pattern |
Serum76 |
LC-MS |
Phenylalanine, tyrosine, glutamate, kynurenine, tryptophan and biogenic amines |
Amino acid and biogenic amine metabolism |
Human tissue extracts77 |
NMR LC-MS/MS |
Alanine, succinate, lactate, glycerophosphoethanolamine, inorganic phosphate, leucine, isoleucine, and valine |
Aspartate metabolism, tricarboxylic acid metabolism |
Plasma78 |
LC/MS |
Lysine, phenylalanine, citrulline, creatine, creatinine, glycodeoxycholic acid, inosine, alpha-ketoglutarate, multiple acyl-lyso-phosphatidylcholine |
Krebs cycle, urea cycle, amino acid and purine metabolism |
Urine79 |
LC-QTRAP MS |
Nucleosides, bile acids, citric acid, several amino acids, cyclic adenosine monophosphate, glutamine, and short- and medium-chain acylcarnitines |
Purine metabolism, energy metabolism, and amino acid metabolism |
Serum80 |
LC/MS |
Acylcarnitines, fatty acids, phosphatidyl ethanolamine |
Fatty acid, β-oxidation, phosphatidylcholine, phosphatidyl ethanolamine metabolism |
Serum81 |
CE-TOF/MS |
Tryptophan, glutamine, and 2-hydroxybutyric acid |
Amino acid metabolism |
Serum82 |
CE-TOF/MS |
Creatine/betaine, betaine, creatine, kynurenine, pipecolic acid |
Central carbon metabolism, glycerolipid metabolism, methylation reactions, oxidative stress |
Conclusion and future outlook
Metabolomics, a non-targeted research method to study small molecules in biological samples from organisms, is currently considered to be an accessible approach for exploring diagnostic biomarkers. From the above description, we declare metabolomics to be a crucial integral field in biomarker discovery; we offer an overview of recent published research on HCC and summarize future development opportunities in the domain. We consider several aspects to be future directions which should rapidly be addressed by metabolomics approaches. Prospective studies may present valuable insights into the pathogenesis and progression of HCC. To use metabolomic assessments for identifying metabolic signatures as predictive biomarkers indicating disease stage or related clinical complications, exploration must begin before the disease occurs, and changes in metabolism must be compared in the development process of the disease. Metabolic profile changes and perturbation patterns may show a response to therapeutic measures and associated fluctuations of body function in metabolism. Mechanistic markers derived from metabolite signatures can be used for screening of drugs or treatment responses. Currently, the use of metabolomics for HCC prevention is still in an early stage; although it has many advantages, it has been in the technical optimization stage for a long time and has not achieved clinical use as a mature technology to differentiate high-risk patients with a 100% detection rate. In addition, there are few reports or studies on mortality predictions using HCC biomarkers. The development of combined markers is a general direction that is attracting the research interest of increasing numbers of scholars. Recently, metabolomics has undergone internal integration, including detection technique integration, multiple sample integration and data processing method integration, as well as external integration, such as integrated analysis of metabolomics and other methods. Integration in the development of metabolomics has enhanced scientific innovation and demonstrated value for various applications, encouraging continued progress in this direction. Taking into account the importance of early diagnosis of HCC, effective treatment and better patient life quality, we must intensify prospective studies to determine biomarkers of HCC based on metabolite profiles; we must also provide in-depth analysis of dynamic metabolomics associated with progression of symptoms, monitoring of side effects and establishment of targets for new drug studies. To realize this goal, we must develop international cooperation; progress in this area will eventually lead to personalized precision treatment of HCC patients.
Conflict of interest
The authors declare no competing financial interests.
Acknowledgements
The authors thank all members of the laboratory for insightful discussions. This work was supported by grants from the Key Program of Natural Science Foundation of State (Grant No. 81430093, 81373930, 81302905, 81673586), National Key Subject of Drug Innovation (Grant No. 2015ZX09101043-005, 2015ZX09101043-011), Natural Science Foundation of Heilongjiang Province of China (H2015038), University Nursing Program for Young Scholars with Creative Talents in Heilongjiang Province (UNPYSCT-2015118).
References
- A. Jemal, F. Bray and M. M. Center, et al. Global cancer statistics, Ca-Cancer J. Clin., 2011, 61, 69–90 CrossRef PubMed.
- H. B. El-Serag, Hepatocellular Carcinoma, N. Engl. J. Med., 2011, 365, 1118–1127 CrossRef CAS PubMed.
- J. Ferlay, I. Soerjomataram and R. Dikshit, et al. Cancer incidence and mortality worldwide: sources, methods and major patterns in GLOBOCAN 2012, Int. J. Cancer, 2015, 136, E359–E386 CrossRef CAS PubMed.
- K. J. Lafaro, A. N. Demirjian and T. M. Pawlik, Epidemiology of hepatocellular carcinoma, Surg. Oncol. Clin. N. Am., 2015, 24, 1–17 CrossRef PubMed.
- H. B. El-Serag and K. L. Rudolph, Hepatocellular carcinoma: epidemiology and molecular carcinogenesis, Gastroenterology, 2007, 132, 2557–2576 CrossRef CAS PubMed.
- D. M. Parkin, F. Bray, J. Ferlay and P. Pisani, Global cancer statistics, 2002, Ca-Cancer J. Clin., 2005, 55, 74–108 CrossRef PubMed.
- International Agency for Research on Cancer. Available from: URL: http://www-dep.iarc.fr/.
- K. Cho, N. G. Mahieu and S. L. Johnson, et al. After the feature presentation: technologies bridging untargeted metabolomics and biology, Curr. Opin. Biotechnol., 2014, 28, 143–148 CrossRef CAS PubMed.
- D. Gauguier, Application of quantitative metabolomics in systems genetics in rodent models of complex phenotypes, Arch. Biochem. Biophys., 2016, 589, 158–167 CrossRef CAS PubMed.
- M. S. Klein and J. Shearer, Metabolomics and Type 2 Diabetes: Translating Basic Research into Clinical Application, J. Diabetes Res., 2016, 2016, 3898502 Search PubMed.
- A. Zhang, H. Sun and G. Yan, et al. Mass spectrometry-based metabolomics: applications to biomarker and metabolic pathway research, Biomed. Chromatogr., 2016, 30(1), 7–12 CrossRef PubMed.
- Y. Zhang and J. Guo, Pathogenesis of hepatitis B virus-related hepatocellular carcinoma, Zhonghua Ganzangbing Zazhi, 2016, 24(2), 152–156 Search PubMed.
- Z. B. Xie, X. B. Wang and D. L. Fu, et al. Postoperative hepatitis B virus reactivation in hepatitis B virus-related hepatocellular carcinoma patients with hepatitis B virus DNA levels <500 copies/mL, OncoTargets Ther., 2016, 9, 4593–4603 CrossRef PubMed.
- R. Noh, D. H. Lee and B. W. Kwon, et al. Clinical Impact of Viral Load on the Development of Hepatocellular Carcinoma and Liver-Related Mortality in Patients with Hepatitis C Virus Infection, Gastroenterol. Res. Pract., 2016, 2016, 7476231 Search PubMed.
- B. J. McMahon, D. Bruden and L. Townshend-Bulson, et al. Infection With Hepatitis C Virus Genotype 3 is an Independent Risk Factor for End-stage Liver Disease, Hepatocellular Carcinoma, and Liver-related Death, Clin. Gastroenterol. Hepatol., 2017, 15(3), 431–437 CrossRef PubMed.
- M. Hedenstierna, A. Nangarhari and O. Weiland, et al. Diabetes and Cirrhosis Are Risk Factors for Hepatocellular Carcinoma After Successful Treatment of Chronic Hepatitis C, Clin. Infect. Dis., 2016, 63(6), 723–729 CrossRef PubMed.
- J. H. Benbow, K. J. Thompson and H. L. Cope, et al. Diet-Induced Obesity Enhances Progression of Hepatocellular Carcinoma through Tenascin-C/Toll-Like Receptor 4 Signaling, Am. J. Pathol., 2016, 186(1), 145–158 CrossRef CAS PubMed.
- G. Testino, S. Leone and P. Borro, Treatment of alcohol use disorder patients affected by liver cirrhosis and/or hepatocellular carcinoma awaiting liver transplantation, Minerva Med., 2016, 107(4), 223–238 Search PubMed.
- B. Kucukcakan and Z. Hayrulai-Musliu, Challenging Role of Dietary Aflatoxin B1 Exposure and Hepatitis B Infection on Risk of Hepatocellular Carcinoma, Open Access Maced. J. Med. Sci., 2015, 3(2), 363–369 CrossRef PubMed.
- K. S. Toda, L. Kikuchi and A. L. Chagas, et al. Hepatocellular Carcinoma Related to Schistosoma mansoni Infection: Case Series and Literature Review, J. Clin. Transl. Hepatol., 2015, 3, 260–264 CrossRef PubMed.
- V. Rapisarda, C. Loreto and M. Malaguarnera, et al. Hepatocellular carcinoma and the risk of occupational exposure, World J. Hepatol., 2016, 8(13), 573–590 CrossRef PubMed.
- W. Q. Lu, J. L. Qiu and Z. L. Huang, et al. Enhanced circulating transforming growth factor beta 1 is causally associated with an increased risk of hepatocellular carcinoma: a mendelian randomization meta-analysis, Oncotarget, 2016, 7(51), 84695–84704 Search PubMed.
- S. Ma, J. Wu and J. Wu, et al. Relationship between HLA-DRB1 allele polymorphisms and familial aggregations of hepatocellular carcinoma, Curr. Oncol., 2016, 23(1), e1–e7 CrossRef CAS PubMed.
- M. Ono and T. Saibara, Are genetic variations the most important risk factors for development of hepatocellular carcinoma?, J. Gastroenterol., 2016, 51(4), 404–405 CrossRef PubMed.
- S. Tavakolpour and S. Sali, Tumor Necrosis Factor-alpha-308 G/A Polymorphisms and Risk of Hepatocellular Carcinoma: A Meta-Analysis, Hepatitis Mon., 2016, 16(4), e33537 Search PubMed.
- S. Teama, A. Fawzy and S. Teama, et al. Increased Serum Endoglin and Transforming Growth Factor beta 1 mRNA Expression and Risk of Hepatocellular Carcinoma in Cirrhotic Egyptian Patients, Asian Pac. J. Cancer Prev., 2016, 17(5), 2429–2434 Search PubMed.
- H. Kim, K. Kim and J. Jin, et al. Measurement of glycosylated alpha-fetoprotein improves diagnostic power over the native form in hepatocellular carcinoma, PLoS One, 2014, 9(10), e110366 Search PubMed.
- P. Gopal, A. C. Yopp and A. K. Waljee, et al. Factors that affect accuracy of alpha-fetoprotein test in detection of hepatocellular carcinoma in patients with cirrhosis, Clin. Gastroenterol. Hepatol., 2014, 12(5), 870–877 CrossRef CAS PubMed.
- S. Zhang, M. Yue and R. Shu, et al. Recent advances in the management of hepatocellular carcinoma, J. BUON, 2016, 21(2), 307–311 Search PubMed.
- R. C. Jha, D. G. Mitchell and J. C. Weinreb, et al. LI-RADS categorization of benign and likely benign findings in patients at risk of hepatocellular carcinoma: a pictorial atlas, AJR, Am. J. Roentgenol., 2014, 203, 48–69 CrossRef PubMed.
- S. H. Choi, J. H. Byun and S. Y. Kim, et al. Liver Imaging Reporting and Data System v2014 With Gadoxetate Disodium- Enhanced Magnetic Resonance Imaging: Validation of LI-RADS Category 4 and 5 Criteria, Invest. Radiol., 2016, 51(8), 483–490 CrossRef CAS PubMed.
- A. Darnell, A. Forner and J. Rimola, et al. Liver Imaging Reporting and Data System with MR Imaging: Evaluation in Nodules 20 mm or Smaller Detected in Cirrhosis at Screening US, Radiology, 2015, 275, 698–707 CrossRef PubMed.
- B. K. Barth, O. F. Donati and M. A. Fischer, et al. Reliability, Validity, and Reader Acceptance of LI-RADS-An In-depth Analysis, Acad. Radiol., 2016, 23(9), 1145–1153 CrossRef PubMed.
- L. Jiang, L. Yan and T. Wen, et al. Comparison of Outcomes of Hepatic Resection and Radiofrequency Ablation for Hepatocellular Carcinoma Patients with Multifocal Tumors Meeting the Barcelona-Clinic Liver Cancer Stage A Classification, J. Am. Coll. Surg., 2015, 221(5), 951–961 CrossRef PubMed.
- S. Zhang, M. Yue and R. Shu, et al. Recent advances in the management of hepatocellular carcinoma, J. BUON, 2016, 21(2), 307–311 Search PubMed.
- A. Raza and G. K. Sood, Hepatocellular carcinoma review: Current treatment, and evidence-based medicine, World J. Gastroenterol., 2014, 20(15), 4115–4127 CrossRef PubMed.
- M. Dhir, A. A. Melin and J. Douaiher, et al. A Review and Update of Treatment Options and Controversies in the Management of Hepatocellular Carcinoma, Ann. Surg., 2016, 263(6), 1112–1125 CrossRef PubMed.
- P. Wang, L. Sheng, G. Mang, H. Wang, X. Huang, X. Yan, X. Yang and R. Pei, Association of transarterial chemoembolization with survival in patients with unresectable hepatocellular carcinoma, Mol. Clin. Oncol., 2014, 2(2), 203–206 Search PubMed.
- J. Tejeda-Maldonado, I. Garcia-Juarez and J. Aguirre-Valadez, et al. Diagnosis and treatment of hepatocellular carcinoma: an update, World J. Hepatol., 2015, 7(3), 362–376 CrossRef PubMed.
- J. K. Nicholson and J. C. Lindon, Systems biology: metabonomics, Nature, 2008, 455, 1054–1056 CrossRef CAS PubMed.
- O. Fiehn, Combining genomics, metabolome analysis, and biochemical modelling to understand metabolic networks, Comp. Funct. Genomics, 2001, 2, 155–168 CrossRef CAS PubMed.
- N. J. Serkova and M. S. Brown, Quantitative analysis in magnetic resonance spectroscopy: from metabolic profiling to in vivo biomarkers, Bioanalysis, 2012, 4, 321–341 CrossRef CAS PubMed.
- C. Hughes, M. Brown and G. Clemens, et al. Assessing the challenges of Fourier transform infrared spectroscopic analysis of blood serum, J. Biophotonics, 2014, 7, 180–188 CrossRef CAS PubMed.
- A. A. Heemskerk, A. M. Deelder and O. A. Mayboroda, CE-ESI-MS for bottom-up proteomics: advances in separation, interfacing and applications, Mass Spectrom. Rev., 2016, 35(2), 259–271 CrossRef CAS PubMed.
- V. R. Robledo and W. F. Smyth, Review of the CE-MS platform as a powerful alternative to conventional couplings in bio-omics and target-based applications, Electrophoresis, 2014, 35, 2292–2308 CrossRef CAS PubMed.
- T. Yamashita and X. W. Wang, Cancer stem cells in the development of liver cancer, J. Clin. Invest., 2013, 123, 1911–1918 CrossRef CAS PubMed.
- A. S. Lok, R. K. Sterling and J. E. Everhart, et al. Des-gamma-carboxy prothrombin and alpha-fetoprotein as biomarkers for the early detection of hepatocellular carcinoma, Gastroenterology, 2010, 138, 493–502 CrossRef CAS PubMed.
- J. Bruix and M. Sherman, Management of hepatocellular carcinoma: an update, Hepatology, 2011, 53, 1020–1022 CrossRef PubMed.
- E. J. Want, B. F. Cravatt and G. Siuzdak, The expanding role of mass spectrometry in metabolite profiling and characterization, ChemBioChem, 2005, 6, 1941–1951 CrossRef CAS PubMed.
- E. J. Want, A. Nordström and H. Morita, et al. From exogenous to endogenous: the inevitable imprint of mass spectrometry in metabolomics, J. Proteome Res., 2007, 6, 459–468 CrossRef CAS PubMed.
- A. H. Emwas, R. Roy and R. T. McKay, et al. Recommendations and Standardization of Biomarker Quantification Using NMR-Based Metabolomics with Particular Focus on Urinary Analysis, J. Proteome Res., 2016, 15(2), 360–373 CrossRef CAS PubMed.
- A. Zhang, H. Sun and G. Yan, et al. Mass spectrometry-based metabolomics: applications to biomarker and metabolic pathway research, Biomed. Chromatogr., 2016, 30(1), 7–12 CrossRef PubMed.
- A. Berisha, S. Dold and S. Guenther, A comprehensive high-resolution mass spectrometry approach for characterization of metabolites by combination of ambient ionization, chromatography and imaging methods, Rapid Commun. Mass Spectrom., 2014, 28(16), 1779–1791 CrossRef CAS PubMed.
- J. Xu, C. Liu and S. Cai, et al. Metabolomic profilings of urine and serum from high fat-fed rats via 1H NMR spectroscopy and pattern recognition, Appl. Biochem. Biotechnol., 2013, 169(4), 1250–1261 CrossRef CAS PubMed.
- A. Zhang, H. Sun and G. Yan, et al. Metabolomics for Biomarker Discovery: Moving to the Clinic, BioMed Res. Int., 2015, 2015 Search PubMed.
- J. S. Tian, X. T. Xia and Y. F. Wu, et al. Discovery, screening and evaluation of a plasma biomarker panel for subjects with psychological suboptimal health state using 1H-NMR-based metabolomics profiles, Sci. Rep., 2016, 6, 33820 CrossRef CAS PubMed.
- M. R. NezamiRanjbar, Y. Luo and D. Poto, et al. GC-MS Based Plasma Metabolomics for Identification of Candidate Biomarkers for Hepatocellular Carcinoma in Egyptian Cohort, PLoS One, 2015, 10(6), e0127299 Search PubMed.
- A. Safaei, A. ArefiOskouie and S. R. Mohebbi, et al. Metabolomic analysis of human cirrhosis, hepatocellular carcinoma, non-alcoholic fatty liver disease and non-alcoholic steatohepatitis diseases, Gastroenterol. Hepatol. Bed Bench, 2016, 9(3), 158–173 Search PubMed.
- H. Gao, Q. Lu and X. Liu, et al. Application of 1H NMR-based metabonomics in the study of metabolic profiling of human hepatocellular carcinoma and liver cirrhosis, Cancer Sci., 2009, 100, 782–785 CrossRef CAS PubMed.
- B. Zhang and R. S. Finn, Personalized Clinical Trials in Hepatocellular Carcinoma Based on Biomarker Selection, Liver Cancer, 2016, 5(3), 221–232 CrossRef CAS PubMed.
- P. P. Song, J. F. Xia and Y. Inagaki, Controversies regarding and perspectives on clinical utility of biomarkers in hepatocellular carcinoma, World J. Gastroenterol., 2016, 22(1), 262–274 CrossRef CAS PubMed.
- D. R. Minton, L. Fu and Q. Chen, et al. Analyses of the transcriptome and metabolome demonstrate that HIF1α mediates altered tumor metabolism in clear cell renal cell carcinoma, PLoS One, 2015, 10(4), e0120649 Search PubMed.
- L. H. Jin and C. Wei, Role of microRNAs in the Warburg effect and mitochondrial metabolism in cancer, Asian Pac. J. Cancer Prev., 2014, 15(17), 7015–7019 CrossRef PubMed.
- X. S. Chen, L. Y. Li and Y. D. Guan, et al. Anticancer strategies based on the metabolic profile of tumor cells: therapeutic targeting of the Warburg effect, Acta Pharmacol. Sin., 2016, 37(8), 1013–1019 CrossRef CAS PubMed.
- E. G. Armitage, H. L. Kotze and J. W. Allwood, et al. Metabolic profiling reveals potential metabolic markers associated with hypoxia inducible factor-mediated signalling in hypoxic cancer cells, Sci. Rep., 2015, 5, 15649 CrossRef CAS PubMed.
- A. Duseja, Staging of hepatocellular carcinoma, J. Clin. Exp. Hepatol., 2014, 4(3), S74–S79 CrossRef PubMed.
- H. Nakayama and T. Takayama, Management before hepatectomy for hepatocellular carcinoma with cirrhosis, World J. Hepatol., 2015, 7(20), 2292–2302 CrossRef PubMed.
- R. Gao, J. Cheng and C. Fan, et al. Serum Metabolomics to Identify the Liver Disease-Specific Biomarkers for the Progression of Hepatitis to Hepatocellular Carcinoma, Sci. Rep., 2015, 5, 18175 CrossRef CAS PubMed.
- B. Peng, F. Liu and R. Han, et al. Dynamic metabolic change is indicative of inflammation-induced transformation of hepatic cells, Int. J. Biochem. Cell Biol., 2015, 66, 45–58 CrossRef CAS PubMed.
- J. Bowers, E. Hughes and N. Skill, et al. Detection of hepatocellular carcinoma in hepatitis C patients: biomarker discovery by LC-MS, J. Chromatogr. B: Anal. Technol. Biomed. Life Sci., 2014, 966, 154–162 CrossRef CAS PubMed.
- M. R. NezamiRanjbar, Y. Luo and C. Di Poto, et al. GC-MS Based Plasma Metabolomics for Identification of Candidate Biomarkers for Hepatocellular Carcinoma in Egyptian Cohort, PLoS One, 2015, 10(6), e0127299 Search PubMed.
- A. I. Fitian, D. R. Nelson and C. Liu, et al. Integrated metabolomic profiling of hepatocellular carcinoma in hepatitis C cirrhosis through GC/MS and UPLC/MS-MS, Liver Int., 2014, 34(9), 1428–1444 CrossRef CAS PubMed.
- Y. Liu, Z. Hong and G. Tan, et al. NMR and LC/MS-based global metabolomics to identify serum biomarkers differentiating hepatocellular carcinoma from liver cirrhosis, Int. J. Cancer, 2014, 135(3), 658–668 CrossRef CAS PubMed.
- C. F. Teng, W. C. Hsieh and C. W. Yang, et al. A biphasic response pattern of lipid metabolomics in the stage progression of hepatitis B virus X tumorigenesis, Mol. Carcinog., 2016, 55(1), 105–114 CrossRef CAS PubMed.
- W. Dai, P. Yin and P. Chen, et al. Study of urinary steroid hormone disorders: difference between hepatocellular carcinoma in early stage and cirrhosis, Anal. Bioanal. Chem., 2014, 406(18), 4325–4335 CrossRef CAS PubMed.
- M. Stepien, T. Duarte-Salles and V. Fedirko, et al. Alteration of amino acid and biogenic amine metabolism in hepatobiliary cancers: findings from a prospective cohort study, Int. J. Cancer, 2016, 138(2), 348–360 CrossRef CAS PubMed.
- M. M. Darpolor, S. S. Basu and A. Worth, et al. The aspartate metabolism pathway is differentiable in human hepatocellular carcinoma: transcriptomics and 13C-isotope based metabolomics, NMR Biomed., 2014, 27(4), 381–389 CrossRef CAS PubMed.
- J. D. Clarke, P. Novak and A. D. Lake, et al. Characterization of hepatocellular carcinoma related genes and metabolites in human nonalcoholic fatty liver disease, Dig. Dis. Sci., 2014, 59(2), 365–374 CrossRef CAS PubMed.
- Y. Shao, B. Zhu and R. Zheng, et al. Development of urinary pseudotargeted LC-MS-based metabolomics method and its application in hepatocellular carcinoma biomarker discovery, J. Proteome Res., 2015, 14(2), 906–916 CrossRef CAS PubMed.
- X. Lin, J. Gao and L. Zhou, et al. A modified k-TSP algorithm and its application in LC-MS-based metabolomics study of hepatocellular carcinoma and chronic liver diseases, J. Chromatogr. B: Anal. Technol. Biomed. Life Sci., 2014, 966, 100–108 CrossRef CAS PubMed.
- J. Zeng, P. Yin and Y. Tan, et al. Metabolomics study of hepatocellular carcinoma: discovery and validation of serum potential biomarkers by using capillary electrophoresis-mass
spectrometry, J. Proteome Res., 2014, 13(7), 3420–3431 CrossRef CAS PubMed.
- J. Zeng, X. Huang and L. Zhou, et al. Metabolomics Identifies Biomarker Pattern for Early Diagnosis of Hepatocellular Carcinoma: from Diethylnitrosamine Treated Rats to Patients, Sci. Rep., 2015, 5, 16101 CrossRef CAS PubMed.
|
This journal is © The Royal Society of Chemistry 2017 |
Click here to see how this site uses Cookies. View our privacy policy here.