DOI:
10.1039/C7RA04788F
(Paper)
RSC Adv., 2017,
7, 44820-44826
Discriminatory analysis based molecular docking study for in silico identification of epigallocatechin-3-gallate (EGCG) derivatives as B-RafV600E inhibitors†
Received
28th April 2017
, Accepted 4th September 2017
First published on 19th September 2017
Abstract
Virtual screening and biological testing were utilized to identify novel B-RafV600E inhibitors. The employed LigandFit program was evaluated by examining the accuracy of the binding conformation prediction and binding affinity estimation (scoring function) via discriminative analysis training. Ten novel compound hits from the database screening were selected and subjected to in vitro biological tests. The natural product EGCG (A8) was discovered to have promising B-RafV600E inhibitory, and then we evaluated six structurally similar compounds (B1, B2, B3, C1, C2, and C3) for their B-RafV600E inhibitory activities in order to establish a structure–activity relationship. One of these compounds, B2, demonstrated a promising B-RafV600E inhibitory activity with an IC50 value of 9.1 μM, providing a theoretical basis for the development of novel agents as B-RafV600E inhibitors.
Introduction
B-Raf is one of the most commonly mutating proto-oncogenes with high clinical impact and plays a significant role in the development of various cancer species.1 Moreover, B-Raf represents an attractive target for small molecules in anticancer drug development.2 B-Raf belongs to the serine/threonine Raf kinase family, which consists of three isoforms, A-Raf, B-Raf and Raf-1 (also coined C-Raf).3 It is also an integral member in the Ras–Raf–MEK–ERK signal transduction pathway4 (also known as the MAPK signaling cascade) participating in cell proliferation and cell survival.5 In the MAPK pathway, active Ras may induce conformational changes in B-Raf upon binding,4 followed by the phosphorylation status triggering kinase activity. Then, phosphorylation of B-Raf activates the MEK protein, which in turn activates the phosphorylation of several other substrates.6 B-Raf kinase is found to frequently mutate in melanomas, with the Val600 → Glu600 (V600E) transition representing the most common mutation, accounting for over 90% in all of the B-Raf oncogenic mutants reported to date.7 Furthermore, this mutation type causes B-Raf to signal independently from upstream regulation.4 In an effort to selectively target the B-RafV600E mutant, numerous clinically used small molecule inhibitors have been published, including LGX-818,8 PLX-8394,9 CEP-2496 (https://clinicaltrials.gov/), and BGB-283 (ref. 10) (Fig. 1).
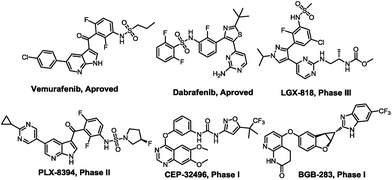 |
| Fig. 1 Known potent B-RafV600E inhibitors. | |
The clinical approval of Vemurafenib11 and Dabrafenib12 for the treatment of metastatic melanoma bearing the B-RafV600E mutation has further confirmed that B-RafV600E may be an attractive target for anticancer therapy.2,13 Moreover, B-RafV600E inhibitors have been found to represent crucial drugs used in patients suffering from inoperable metastatic melanomas. However, some cutaneous adverse side effects often occur during clinical use, e.g. dermatologic reactions.14 In particular, the rate of cutaneous adverse effects associated with the use of Vemurafenib was reported to be 92% to 95% in patients participating in B-Raf inhibitor melanoma (BRIM) studies.15 To combat this major disadvantage, we designed an efficient virtual screening method to obtain novel structures of B-Raf inhibitors with potentially reduced side effects compared to Vemurafenib.
As one method used in modern structural-based drug design, molecular docking is used routinely to predict the binding strength of ligands in the target binding pocket. Recently, Li et al. reported the identification of organohalogen drugs as B-RafV600E inhibitors by using molecular docking and bioassay.16 Recently, there is a growing interest in applying various methods to improve the accuracy of molecular docking, ultimately elevating the discriminatory ability of molecular docking to be more efficient.17–19 Here, the discriminatory ability to each scoring function by recognizing overlapping regions is generally affected by the receiver operating characteristic (ROC) curve. As such, the efficacy of molecular docking may be significantly enhanced through discriminatory analysis for the selection of docking scores. We have applied this method to discover and develop a novel series of JAK2 inhibitors by using the distributions and ROC curve of LigandFit docking scores to distinguish JAK2 inhibitors from inactive compounds.20 As part of our continued interest in rational drug design,21–26 in the present study, the LigandFit program was evaluated by examining the accuracy of the binding conformation prediction and binding affinity estimation (i.e. scoring function). Virtual screening involves the performance of docking simulations for a large number of inactive diverse compounds and known B-RafV600E inhibitors in order to obtain a rational score function according to the distribution of two series of compound scores. The optimal function was applied to screen the SPECS library and a natural product library as well as the activity of the compounds obtained by screening was evaluated. Fortunately, we found that epigallocatechin-3-gallate (EGCG, A8), a compound as the main chemical constituents of green tea, exhibits moderate inhibitory activity against B-RafV600E. Indeed, other natural products featuring large-scale structural diversity have been the major sources of bioactive agents in the fields of medicine and life sciences. Six other compounds featuring similar structural characteristics as EGCG (Fig. 5B) were biologically evaluated for their B-RafV600E inhibitory activities. Most of these compounds exhibited moderate inhibitory activity of B-RafV600E with IC50 values ranging between 9.1 and 43.6 μM. Among these compounds, compound B2 demonstrated a promising inhibition of B-RafV600E with an IC50 value of 9.1 μM. Interestingly, the screened natural products feature vastly different molecular scaffolds and are structurally distinct from current B-RafV600E inhibitors. This further demonstrates the feasible identification of novel hit compounds using our discriminatory ability training-based molecular docking study. Finally, molecular dynamics calculations were implemented to explore the potential binding mode of the promising compounds with the B-RafV600E protein, providing important information for further structural modification considerations and structure–activity relationship studies.
Methods
Docking-based virtual screening by LigandFit
Operating environment: Discovery Studio 2.5. Preparation of the target binding pocket: (a) the crystal structure of B-RafV600E (pdb: 4EHG) was employed as the template for molecular docking with the LigandFit protocol;27 (b) all crystallographic water molecules were removed; (c) all hydrogen atoms were added by CHARMm force field of “Prepare Protein module”; (d) the “Find Sites as Volume of Selected Ligand” tool was applied to define the docking site of the resulting protein structure. Preparation of the ligand library: (a) variable numbers of M onte Carlo simulations were implemented for different conformer generations of ligands; (b) all atoms were added by CHARMm force field; (c) all conformers were treated with the “ligand minimization” of the receptor–ligand interaction module.LigandFit protocol: (a) the ligand–receptor interaction energies were calculated based on the Piecewise Linear Potential 1 (PLP1) force field; (b) a short rigid body minimization was performed and the top one pose for each ligand was saved; (c) scoring was performed with a set of scoring functions employed in LigandFit module, including LigScore1_Dreiding, LigScore2_Dreiding, -PLP1, -PLP2, Jain, -PMF, Dock_Score.
In vitro biological assays for BRafV600E (or BRaf) inhibitory activity
The inhibitory activities of the compounds against the B-RafV600E enzyme (Invitrogen, USA) were evaluated according to a published procedure.2 Specifically, 2.5 μL of a reaction solution in assay buffer (50 mM HEPES, pH 7.5, 10 mM MgCl2, 1 mM EGTA, 0.01% BRIJ-35) was placed in a 384-well plate containing 0.6 nM B-RafV600E (or 2 nM B-Raf (Invitrogen, USA) for BRaf assay), 0.2 μM Fluorescein-MAP2K1, and 1.5 μM ATP (or 0.1 μM ATP for BRaf assay). The reaction was then incubated at room temperature for 1 h and subsequently quenched with 10 μL of a detection solution containing 2 nM antibody (Cat. no. PV3574, Invitrogen, USA) and 10 mM EDTA. After incubation for 30 min, the reaction mixture was analyzed on a Caliper LabChip 3000 (Caliper LifeSciences, Hopinkton, MA, USA) by electrophoretic separation of the fluorescent substrate and the phosphorylated product. All compounds were first evaluated at a concentration of 10 μM and then further tested at the concentration prepared from 3-fold serial dilutions exhibiting a preliminary activity of more than 40%. The IC50 values were determined by the analytical software Prism 5.0 (GraphPad Software Pte Ltd.).
Molecular dynamics (MD) simulations
The docked structures of an inhibitor bound into B-RafV600E were used as the initial structures for MD calculations. A CHARMm force field was applied to the complex and the resulting system was then immersed into an “Explicit Periodic Boundary” water box with a sodium cation used for complex neutralization. Afterwards, the solvated system was subjected to double-fold minimization (10
000 cycles of steepest descent minimization and 100
000 cycles of conjugate gradient minimization). The system was gradually heated from 50 K to 300 K over a period of 100 ps and subsequently equilibrated for 500 ps. Starting from the last frame of the equilibration, a production simulation was performed for 500 ps using the NPT ensemble under a constant temperature of 300 K and pressure of 1 atm. Other parameters of MD simulation were maintained at the default Discovery Studio configuration.
Results and discussion
Construction of drug-like library
Retrieved from the chemical SPECS database (http://www.specs.net), a total of 217
190 compounds were prepared by the Prepare Ligands protocol of Accelrys Discovery Studio 2.5 program including the following steps: (1) the two-dimensional (2D) structure was converted into a three-dimensional (3D) structure, (2) charges were calculated, (3) H-atoms were added, and (4) the “Lipinski's rule of five” filter was applied to the resulting compounds to simplify the compound library. After the above preparation, a drug-like library containing 153
691 compounds was obtained. Another 623 compounds from the natural product library (NPD from Zinc database), were prepared as well.
Evaluation of scoring functions in LigandFit
Accurate scoring functions play a key role in identifying active B-RafV600E inhibitors through scoring. The scoring functions are expected to be able to efficiently distinguish between the active compounds and the inactive compounds. In order to accomplish this goal, 731 B-RafV600E inhibitors were acquired from the Binding DB database,28 and 10
000 non-active compounds were randomly retrieved from the Maybridge database using the Find Diverse Molecules module of DS 2.5. The two groups of compounds gained a maximum diversity through 2D similarity studies based on the FCFP_6 fingerprints.29 Subsequently, all molecules were treated with a Prepare Ligands protocol, docked into the active site of B-RafV600E and evaluated by the different scoring functions of the LigandFit module, respectively. The docking scores (LigScore1_Dreiding, LigScore2_Dreiding, -PLP1, -PLP2, Jain, -PMF, Dock_Score) and distributions of the two series (Fig. 2) were analyzed. From the data analysis of Dock_Score in Fig. 2, the differentiating capacity of Dock_Score was found to be the most promising, with the minimal overlapping area of the active B-RafV600E inhibitor and the inactive compound.
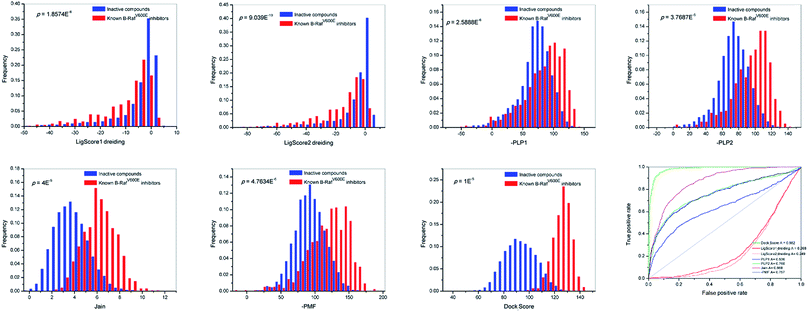 |
| Fig. 2 Distributions and ROC curve of different docking scores (LigScore1_Dreiding, LigScore2_Dreiding, -PLP1, -PLP2, Jain, -PMF, Dock_Score). | |
We also examined the discriminatory ability of these scores by receiver operating characteristic (ROC) curve studies.29 Consistent with previous results, Dock_Score exhibited a preferred corresponding AUC (area under the ROC curve) value of 0.982 when compared to the other scores. From the analysis of distributions and ROC curves, it can be concluded that the Dock_Score of the LigandFit module represents an objective scoring function for the present study and most of the scores of Dock_Scores were found between 115 and 135.
Virtual library screening using molecular docking
All of the structures in the two drug databases were docked in the active binding pocket by DS 2.5. In general, Dock_Score identifies B-RafV600E inhibitors more accurately than other scoring functions and was therefore used in virtual screening to obtain the optimal compound. Finally, further biological testing of the docking and structural diversity of the ten target compounds (Fig. 3) was carried out.
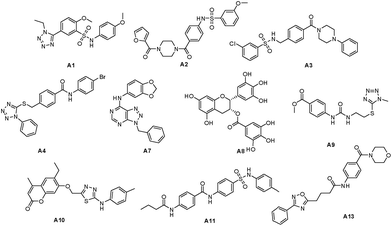 |
| Fig. 3 Target compounds from SPECS database and natural library. | |
In vitro B-RafV600E inhibitory activities assay
The ten target compounds were purchased from SPECS and evaluated for their B-RafV600E inhibitory activities. Interestingly, compound A8 showed significant B-RafV600E inhibitory activity with an IC50 value of 11.1 μM and an inhibition rate of 44.1% at a concentration of 10 μM (Fig. 4). Compound A8, also known as epigallocatechin-3-gallate (EGCG), represents a compound extracted from green tea and has been shown to exhibit biophysical effects as described in a number of reports found in the literature.30–32 However, some properties have never been studied before and a variety of other factors need to be considered when using EGCG. In the field of antitumor activity, EGCG has been found to reduce the proliferation of cancer cells and induce apoptosis,33,34 more specify, mutant BRAF melanoma cell lines were found to be sensitive to EGCG.35,36 However, the metabolic reason for this anticancer activity has never been described. Here, we further explored the inhibitory activity of EGCG and its derivatives on B-RafV600E. Furthermore, preliminary structure–activity relationships were evaluated through a docking study.
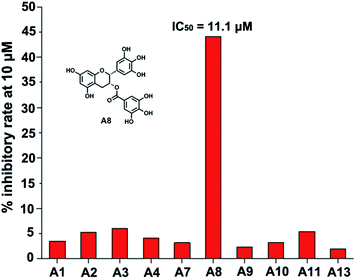 |
| Fig. 4 B-RafV600E inhibitory activities of target compounds A1–A13. | |
EGCG was used as a starting point for further studies. The binding pattern of EGCG within the proposed B-RafV600E active site is shown in Fig. 5A. In order to improve the structural analysis of EGCG, the molecular structure was separated into three individual sections, A fragment, B fragment and linker. Of these, we hypothesize that the A fragment is important for the B-RafV600E activity. This notion may be due to the fact that among the previous 10 compounds, only the A8 structure was found to feature the A fragment, presumably resulting in inhibitory activity. Thus, in the following study, the A fragment as the primary functional group was retained while the other two fragments were optimized. We believe that the number of hydroxyl groups may be adjusted to increase the combinatory ability of the inhibitor. On the other hand, we also considered modifying the extension connectors to better match the pockets. According to the docking evaluation, the structural design of the derivatives was carried out focusing on two parts: the hydrolysis of EGCG esters and the substitution of different substrates. Finally, we evaluated the activities of six compound species, B(1–3) and C(1–3), which were synthesized as reported previously (Fig. 5B).21
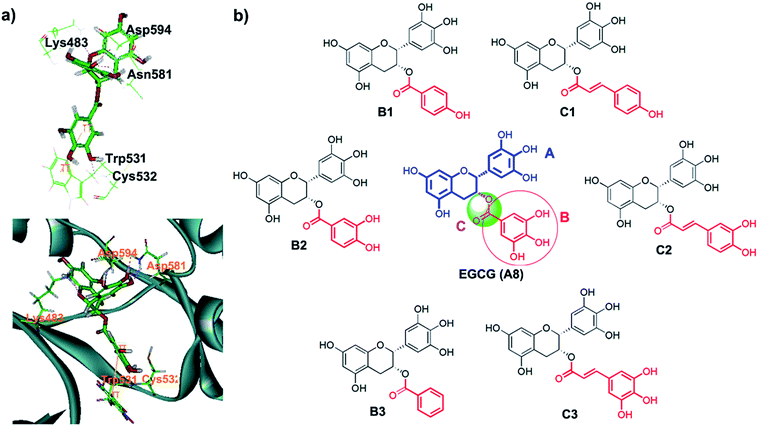 |
| Fig. 5 (a) Binding mode of EGCG (A8) in the active site of B-RafV600E (relevant amino acid residues in the binding site are shown in line form. Green, orange, pink lines represent conventional hydrogen bonds, π–π hydrophobic interactions, respectively), (b) the structures of EGCG derivatives. | |
The B-RafV600E inhibitory activities of these compounds were evaluated and GW5074 was used as positive control. The results obtained represent the mean of three experiments and are expressed as IC50 values (Table 1). Compound B2 demonstrated an improved inhibitory activity against B-RafV600E, with an IC50 value of 9.1 μM. Other compounds exhibited somewhat lower B-RafV600E inhibitory activities. The data showed that two hydroxyl groups are indeed necessary for inhibitory activity and the studied inhibitors may therefore provide a reasonable basis for the future development of advanced agents to treat metastatic melanoma.
Table 1 Inhibitory activity for synthesized compounds against B-RafV600E
Compounds |
IC50 (μM) |
Compounds |
IC50 (μM) |
EGCG (A8) |
11.1 |
GW5074 |
0.0015 |
B1 |
43.6 |
C1 |
22.1 |
B2 |
9.1 |
C2 |
12.7 |
B3 |
25.1 |
C3 |
30.1 |
Besides, we tested the wild-type Braf inhibitory activity of representative compounds EGCG and C2, both of which show weaker activity against wild-type Braf (IC50 values of EGCG and C2 are 57.86 μM and > 200 μM, respectively). Thus, the compound C2 exhibited even more selective to B-RafV600E than that of EGCG, with selective index more than 15.7 folds.
MD simulations and mechanistic analysis of interactions
In order to investigate the interaction mode between the most promising compound, B2, and the B-RafV600E protein, molecular dynamics (MD) simulations and an interaction decomposition analysis were carried out to explore the quantitative energy contributions per residue to the binding affinity of the most promising inhibitor species B2. The RMSD value of B-Raf was found to reach equilibrium and remained an average value after a simulation time of 100 ps. The RMSD value of the protein backbone was calculated from a 200 to 500 ps trajectory and the data points were found to fluctuate 0.574 ± 0.06 nm. After 500 ps production simulation, the distance between the inhibitor B2 and the key amino acids Ser465, Thr529 Asn580 and Asp594 in B-Raf tends to converge, indicating that the system reached equilibrium conditions (Fig. 6c and Table S1, ESI†). Based on the stable conformation obtained from MD simulation, the interactions involved in the protein/ligand complex were analyzed. As shown in Fig. 5B, the scaffold was found to establish H-bonds in inhibitor B2 with NH and the carbonyl group in the B-Raf hinge residue ASN580 establishing an L-shaped geometry. Furthermore, the hydroxyl group on the scaffold formed H-bonds with Ser465. In accordance with the interaction energy decomposition, the contribution of ASN580 and Ser465 in B2 was determined to be −10.3 kcal mol−1 and −6.4 kcal mol−1, respectively (Table S2, ESI†). The trihydroxylphenyl substituent in inhibitor B2 was found to be located in the hydrophobic pocket adjacent to the Thr529 group. Besides, the m-dihydroxylphenyl group in B2 also forms H-bonds with Asp594. Therefore, according to the binding pattern and chemical structure of B2, we speculate that the aromatic moiety, linked by the catechins scaffold containing hydrogen-donors and receptors, may represent an important pharmacophore target of the studied B-RafV600E inhibitor.
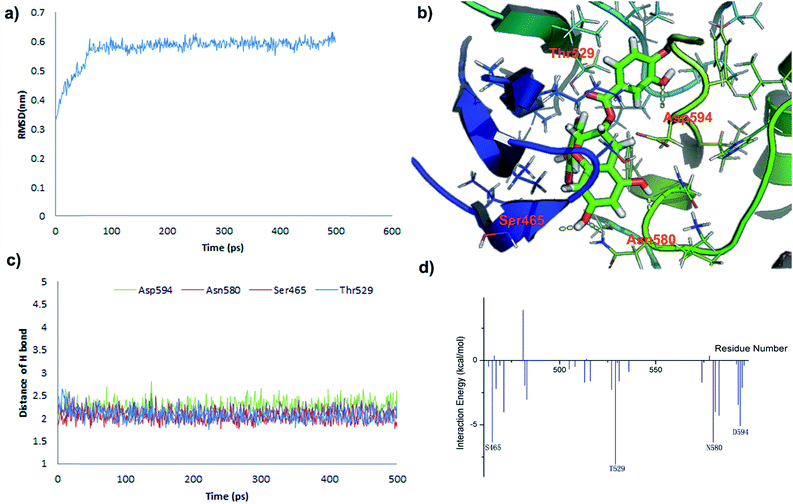 |
| Fig. 6 MD simulations and interaction mechanism analysis for the most potent inhibitor B2: (a) RMSD of B-RafV600E backbone during the 500 ps simulation time. (b) Plot the binding pattern of B2. (c) Plot the distances of H-bond between Ser465, Thr529 Asn580 and Asp594 and B2 during 500 ps of MD simulation; (d) plot the inhibitor – amino acid residue interaction spectrum for B2. | |
Conclusion
In this work, we established a reliable molecular docking scoring function evaluation method and used this method for the screening of a compound library to obtain a potent small molecule inhibitor, A8 (also known as EGCG), with potent B-RafV600E inhibitory activity (IC50 value of 11.1 μM). On this basis, we screened six structurally similar compounds (B1, B2, B3, C1, C2, and C3) for their B-RafV600E inhibitory activities based on a rational structure design. One of these compounds, B2, exhibited a promising B-RafV600E inhibitory activity with an IC50 value of 9.1 μM. Further studies are currently carried out in our laboratory to evaluate the pharmacological potency of this compound and a follow-up report will be published shortly.
Conflicts of interest
There are no conflicts to declare.
Acknowledgements
This study was financially supported by National Natural Science Foundation of China (81673294 and 81502926) and Zhejiang Province Public Welfare Projects (2016C33067) and China Postdoctoral Science Foundation (2017M612017).
Notes and references
- M. A. Rahman, A. Salajegheh, R. A. Smith and A. K. Y. Lam, Crit. Rev. Oncol. Hematol., 2014, 90, 220–232 CrossRef CAS PubMed.
- M. M. Vasbinder, B. Aquila, M. Augustin, H. Chen, T. Cheung, D. Cook, L. Drew, B. P. Fauber, S. Glossop, M. Grondine, E. Hennessy, J. Johannes, S. Lee, P. Lyne, M. Mortl, C. Omer, S. Palakurthi, T. Pontz, J. Read, L. Sha, M. Shen, S. Steinbacher, H. Wang, A. Wu and M. Ye, J. Med. Chem., 2013, 56, 1996–2015 CrossRef CAS PubMed.
- H. F. Li, Y. Chen, S. S. Rao, X. M. Chen, H. C. Liu, J. H. Qin, W. F. Tang, W. Yue, X. Zhou and T. Lu, Curr. Med. Chem., 2010, 17, 1618–1634 CrossRef CAS PubMed.
- R. Arora, M. Di Michele, E. Stes, E. Vandermarliere, L. Martens, K. Gevaert, E. Van Heerde, J. T. M. Linders, D. Brehmer, E. Jacoby and P. Bonnet, J. Med. Chem., 2015, 58, 1818–1831 CrossRef CAS PubMed.
- M. J. Robinson and M. H. Cobb, Curr. Opin. Cell Biol., 1997, 9, 180–186 CrossRef CAS PubMed.
- W. Kolch, Biochem. J., 2000, 351, 289–305 CrossRef CAS PubMed.
- H. Cheng, Y. Chang, L. Zhang, J. Luo, Z. Tu, X. Lu, Q. Zhang, J. Lu, X. Ren and K. Ding, J. Med. Chem., 2014, 57, 2692–2703 CrossRef CAS PubMed.
- Z. Li, K. Jiang, X. F. Zhu, G. B. Lin, F. Song, Y. F. Zhao, Y. J. Piao, J. W. Liu, W. Cheng, X. L. Bi, P. Gong, Z. Q. Song and S. S. Meng, Cancer Lett., 2016, 370, 332–344 CrossRef CAS PubMed.
- C. Zhang, W. Spevak, Y. Zhang, E. A. Burton, Y. Ma, G. Habets, J. Z. Zhang, J. Lin, T. Ewing, B. Matusow, G. Tsang, A. Marimuthu, H. Cho, G. X. Wu, W. R. Wang, D. Fong, H. Nguyen, S. Y. Shi, P. Womack, M. Nespi, R. Shellooe, H. Carias, B. Powell, E. Light, L. Sanftner, J. Walters, J. Tsai, B. L. West, G. Visor, H. Rezaei, P. S. Lin, K. Nolop, P. N. Ibrahim, P. Hirth and G. Bollag, Nature, 2015, 526, 583–586 CrossRef CAS PubMed.
- Z. Tang, X. Yuan, R. Du, S. H. Cheung, G. Zhang, J. Wei, Y. Zhao, Y. Feng, H. Peng, Y. Zhang, Y. Du, X. Hu, W. Gong, Y. Liu, Y. Gao, Y. Liu, R. Hao, S. Li, S. Wang, J. Ji, L. Zhang, S. Li, D. Sutton, M. Wei, C. Zhou, L. Wang and L. Luo, Mol. Cancer Ther., 2015, 14, 2187–2197 CrossRef CAS PubMed.
- G. Bollag, P. Hirth, J. Tsai, J. Z. Zhang, P. N. Ibrahim, H. N. Cho, W. Spevak, C. Zhang, Y. Zhang, G. Habets, E. Burton, B. Wong, G. Tsang, B. L. West, B. Powell, R. Shellooe, A. Marimuthu, H. Nguyen, K. Y. J. Zhang, D. R. Artis, J. Schlessinger, F. Su, B. Higgins, R. Iyer, K. D'Andrea, A. Koehler, M. Stumm, P. S. Lin, R. J. Lee, J. Grippo, I. Puzanov, K. B. Kim, A. Ribas, G. A. McArthur, J. A. Sosman, P. B. Chapman, K. T. Flaherty, X. W. Xu, K. L. Nathanson and K. Nolop, Nature, 2010, 467, 596–599 CrossRef CAS PubMed.
- N. Yaktapour, F. Meiss, J. Mastroianni, T. Zenz, N. Andriova, N. R. Mathew, R. Claus, B. Hutter, S. Frohling, B. Brors, D. Pfeifer, M. Pantic, I. Bartsch, T. S. Spehl, P. T. Meyer, J. Duyster, K. Zirlik, T. Brummer and R. Zeiser, J. Clin. Invest., 2014, 124, 5074–5084 CAS.
- L. Ren, K. A. Ahrendt, J. Grina, E. R. Laird, A. J. Buckmelter, J. D. Hansen, B. Newhouse, D. Moreno, S. Wenglowsky, V. Dinkel, S. L. Gloor, G. Hastings, S. Rana, K. Rasor, T. Risom, H. L. Sturgis, W. C. Voegtli and S. Mathieu, Bioorg. Med. Chem. Lett., 2012, 22, 3387–3391 CrossRef CAS PubMed.
- B. Gencler and M. Gonul, Dermatol. Res. Pract., 2016, 2016, 1–6 CrossRef PubMed.
- M. E. Lacouture, M. Duvic, A. Hauschild, V. G. Prieto, C. Robert, D. Schadendorf, C. C. Kim, C. J. McCormack, P. L. Myskowski, O. Spleiss, K. Trunzer, F. Su, B. Nelson, K. B. Nolop, J. F. Grippo, R. J. Lee, M. J. Klimek, J. L. Troy and A. K. Joe, Oncologist, 2013, 18, 314–322 CrossRef CAS PubMed.
- Y. Li, B. Guo, Z. Xu, B. Li, T. Cai, X. Zhang, Y. Yu, H. Wang, J. Shi and W. Zhu, Sci. Rep., 2016, 6, 31074 CrossRef CAS PubMed.
- G. Chemi, S. Gemma, G. Campiani, S. Brogi, S. Butini and M. Brindisi, Front. Chem., 2017, 5, 7 Search PubMed.
- R. K. Pandey, B. V. Kumbhar, S. Sundar, A. Kunwar and V. K. Prajapati, J. Recept. Signal Transduction, 2017, 37, 60–70 CrossRef CAS PubMed.
- J. C. Pereira, E. R. Caffarena and C. N. dos Santos, J. Chem. Inf. Model., 2016, 56, 2495–2506 CrossRef CAS PubMed.
- T. T. Yao, J. F. Xie, X. G. Liu, J. L. Cheng, C. Y. Zhu, J. H. Zhao and X. W. Dong, RSC Adv., 2017, 7, 10353–10360 RSC.
- S. Wang, R. Jin, R. Wang, Y. Hu, X. Dong and A. E. Xu, RSC Adv., 2016, 6, 106308–106315 RSC.
- T. T. Yao, J. L. Cheng, B. R. Xu, M. Z. Zhang, Y. Z. Hu, J. H. Zhao and X. W. Dong, RSC Adv., 2015, 5, 49195–49203 RSC.
- W. H. Zhan, D. Q. Li, J. X. Che, L. R. Zhang, B. Yang, Y. Z. Hu, T. Liu and X. W. Dong, Eur. J. Med. Chem., 2014, 75, 11–20 CrossRef CAS PubMed.
- X. W. Dong, Y. M. Zhao, X. Q. Huang, K. N. Lin, J. Z. Chen, E. Q. Wei, T. Liu and Y. Z. Hu, Eur. J. Med. Chem., 2013, 62, 754–763 CrossRef CAS PubMed.
- X. W. Dong, X. L. Zhou, H. Jing, J. Z. Chen, T. Liu, B. Yang, Q. J. He and Y. Z. Hu, Eur. J. Med. Chem., 2011, 46, 5949–5958 CrossRef CAS PubMed.
- X. W. Dong, C. Y. Jiang, H. Y. Hu, J. Y. Yan, J. Chen and Y. Z. Hu, Eur. J. Med. Chem., 2009, 44, 4090–4097 CrossRef CAS PubMed.
- S. Mathieu, S. N. Gradl, L. Ren, Z. Y. Wen, I. Aliagas, J. Gunzner-Toste, W. Lee, R. Pulk, G. L. Zhao, B. Alicke, J. W. Boggs, A. J. Buckmelter, E. F. Choo, V. Dinkel, S. L. Gloor, S. E. Gould, J. D. Hansen, G. Hastings, G. Hatzivassiliou, E. R. Laird, D. Moreno, Y. Q. Ran, W. C. Voegtli, S. Wenglowsky, J. Grina and J. Rudolph, J. Med. Chem., 2012, 55, 2869–2881 CrossRef CAS PubMed.
- T. Q. Liu, Y. M. Lin, X. Wen, R. N. Jorissen and M. K. Gilson, Nucleic Acids Res., 2007, 35, D198–D201 CrossRef CAS PubMed.
- S. R. Langdon, J. Mulgrew, G. V. Paolini and W. P. van Hoorn, J. Cheminf., 2010, 2, 11–29 CAS.
- L. Ortiz-Lopez, B. Marquez-Valadez, A. Gomez-Sanchez, M. D. Silva-Lucero, M. Torres-Perez, R. I. Tellez-Ballesteros, M. Ichwan, M. A. Meraz-Rios, G. Kempermann and G. B. Ramirez-Rodriguez, Neuroscience, 2016, 322, 208–220 CrossRef CAS PubMed.
- L. C. Tsai, H. Y. Hsieh, K. Y. Lu, S. Y. Wang and F. L. Mi, Nanomedicine, 2016, 11, 9–30 CrossRef CAS PubMed.
- D. Wu, J. Invest. Med., 2016, 64, 1213–1219 CrossRef PubMed.
- K. W. Luo, C. Wei, W. Y. Lung, X. Y. Wei, B. H. Cheng, Z. M. Cai and W. R. Huang, J. Nutr. Biochem., 2016, 41, 56–64 CrossRef PubMed.
- C. Yang, W. Du and D. Yang, Int. J. Food Sci. Nutr., 2016, 67, 818–827 CrossRef CAS PubMed.
- S. Tsukamoto, Y. Huang, D. Umeda, S. Yamada, S. Yamashita, M. Kumazoe, Y. Kim, M. Murata, K. Yamada and H. Tachibana, J. Biol. Chem., 2014, 289, 32671–32681 CrossRef CAS PubMed.
- C. G. Langdon, M. A. Held, J. T. Platt, K. Meeth, P. Iyidogan, R. Mamillapalli, A. B. Koo, M. Klein, Z. Liu, M. W. Bosenberg and D. F. Stern, Pigm. Cell Melanoma Res., 2015, 28, 417–430 CrossRef CAS PubMed.
Footnote |
† Electronic supplementary information (ESI) available: The original data of MD simulation (Tables S1 and S2). See DOI: 10.1039/c7ra04788f |
|
This journal is © The Royal Society of Chemistry 2017 |
Click here to see how this site uses Cookies. View our privacy policy here.