DOI:
10.1039/C7RA06146C
(Paper)
RSC Adv., 2017,
7, 38784-38797
Transcriptomic analysis and driver mutant prioritization for differentially expressed genes from a Saccharomyces cerevisiae strain with high glucose tolerance generated by UV irradiation†
Received
2nd June 2017
, Accepted 24th July 2017
First published on 8th August 2017
Abstract
The Saccharomyces cerevisiae strain UV02_HG, which has faster growth and a higher ethanol yield in 40% (w/v) glucose culture medium than the wild type, is a mutant generated from the MF02 wild-type strain by UV irradiation. Transcriptome sequencing was performed to analyze the differential gene expression between the two strains. 1203 genes had significantly different expression levels in response to high sugar stress. Based on KEGG enrichment analysis, the identified genes were involved in pathways such as the ribosome, oxidative phosphorylation, protein processing, protein export, carbon metabolism, and the MAPK pathway. Real-time quantitative PCR (qPCR) was used to validate the reliability of the results obtained by RNA-Seq. The network-based gene expression Quantitative Trait Locus (eQTL) tool was used to interpret the driver mutations that elicited the adaptive phenotype. According to this network, we speculated that mutations in STE12 and TUP1 are the main drivers of the adaptive phenotype. Sixteen poorly understood genes whose expression was downregulated by STE12 were annotated by gene ontology, all of which are highly associated with the cell membrane.
Introduction
Saccharomyces cerevisiae is an organism predominantly used for industrial bioethanol production, owing to its high fermentation rate and high tolerance for industrial processing conditions. Sugarcane manufacturing produces the by-product molasses, containing 24–36% sucrose and 12–24% other sugars. Molasses can be used as a raw material for ethanol production, which may eventually be a renewable and environmentally friendly replacement for gasoline. The primary issue to overcome is hyperosmotic stress for cells growing in sugarcane molasses. S. cerevisiae strains with better hyperosmotic resistance, higher ethanol yield and faster growth are needed to meet the demand for sugarcane molasses fermentation. Glucose participates in ethanol metabolism directly and is the preferred hexose sugar for S. cerevisiae cells. Therefore, we used a hyperosmotic glucose solution as the carbon source to screen for strains with hyperosmotic resistance and fast growth.
Cell metabolism is a complicated and dynamic network of interactions. Usually the ideal mutation is a systemic evolution decided by many genes, including structural genes, regulator genes and auxiliary genes. It is difficult to obtain these mutants by adjusting a single gene; however, this disadvantage can be mitigated by using UV irradiation, which can generate a potential mutant library by producing large numbers of random mutations.1 Here, we generated a mutant library from the wild-type S. cerevisiae strain MF02 by UV irradiation. Next, we screened for a mutant strain that grows faster and yields more ethanol in medium containing 40% glucose and found UV02_HG. To determine the probable elements that lead to the difference between the wild type and mutant strains, transcriptome sequencing was used to explore differences in gene expression levels. Transcriptome sequencing identifies differentially expressed genes that can be used to understand metabolic pathways, discover new genes, improve genome annotation, and identify splice variants and single nucleotide polymorphisms.2,3
Cells use a holistic synergism process to resist hyperosmotic stress, involving ion and glycerol transport, metabolism, protein translation, the cell cycle, regulation of gene expression, etc. These responses are frequently controlled by the high-osmolarity glycerol (HOG) pathway, which encompasses membrane osmosensors, signal pathways, and proteins driving effector functions in the cytoplasm and nucleus.4 The HOG pathway mechanism is well studied in S. cerevisiae. However, most research on the hypertonic response of S. cerevisiae conducted to date has used salt or sorbitol to create a hypertonic environment. Responses to high-sugar environments have not been investigated sufficiently to understand the high-sugar tolerance mechanism, although some studies have been conducted in this respect using differentially expressed genes identified by microarrays or transcriptome sequencing. In high sugar stress, important transcriptomic changes occur in stress response pathways, such as altered respiration and implication of the HOG, protein kinase A (PKA) and target of rapamycin (TOR) pathways.5 Using high-density DNA microarrays, Daniel J. Erasmus et al. found that most genes involved in the glycolytic and pentose phosphate pathways are up-regulated when S. cerevisiae is grown in grape juice containing 40% (w/v) sugars (equimolar amounts of glucose and fructose).6
In this research, we compared differentially expressed genes between wild-type and UV mutant S. cerevisiae strains in medium containing 40% glucose by transcriptome sequencing. The results show that most of the differentially expressed genes involved in the ribosome, oxidative phosphorylation and protein export were down-regulated and most genes involved in the HOG pathway, carbon metabolism, protein processing in the endoplasmic reticulum (ER), and phagosome were up-regulated. The network-based eQTL data was used to interpret the driver mutations that elicited the adaptive phenotype.7 Only transcription factor–DNA interactions were found in the network. Thus, we hypothesized that two transcription factors, STE12 and TUP1, play crucial roles in the evolution of the adaptive phenotype.
Experimental
Strains and culture conditions
Two strains were used in this study, S. cerevisiae MF02 and S. cerevisiae UV02_HG. Strain MF02, used for ethanol fermentation of sugar cane molasses, was isolated in our laboratory from year-old sugar mill waste in Nanning, China. Strain UV02_HG, which grew faster in high glucose medium, was an MF02 mutant induced by UV irradiation with 20 W of light power and a vertical dimension of 15.8 cm. The irradiated cells were cultivated in YPD medium (20 g L−1 tryptone, 10 g L−1 yeast extract and 20 g L−1 glucose) at 30 °C without light for 2 days to obtain the mutant library, then the cells were spread on a plate with solid YP40 medium (20 g L−1 tryptone, 10 g L−1 yeast extract, 400 g L−1 glucose and 20 g L−1 agarose) at 30 °C. 2 days later, the big colonies were transferred into liquid YP40 medium and screened for the best mutant by testing the growth speed using a spectrophotometer (OD600).
The MF02 and UV02_HG cells were cultivated in YPD at 30 °C (200 rpm) for 12 h and counted under a microscope (Nikon 80i, Japan), then comparative amounts (about 1.5 × 108 cells per mL) of fresh cells were inoculated with 50 mL YP40 medium in 250 mL Erlenmeyer flasks. The flasks were covered with cotton and shaken at 30 °C (200 rpm). Samples were taken to count the number of cells every four hours, and to quantitate the yields of ethanol on a gas chromatograph (Agilent 6890, USA) every eight hours. Cells in the mid-exponential growth phase were rapidly collected, washed, immediately frozen in liquid nitrogen and stored at −80 °C for RNA extraction.
RNA extraction and examination
The total RNA was extracted by grinding the cells in TRIzol reagent (TaKaRa, Japan) in liquid nitrogen, isolated with chloroform, and precipitated with isopropanol. The sediment was washed with 75% alcohol and dissolved in RNA-free distilled water. RNA degradation was detected on 1% agarose gels. The RNA purity was checked using a NanoPhotometer spectrophotometer (IMPLEN, CA, USA). The precise quantification of RNA was measured using an RNA Assay Kit in Qubit (Life Technologies, CA, USA). The RNA integrity was assessed using an RNA Nano 6000 Assay Kit with the Bioanalyzer 2100 system (Agilent Technologies, CA, USA).
Library preparation and transcriptome sequencing (HiSeq PE 150)
Three micrograms of RNA per sample was used for sample preparation. Sequencing libraries were generated using the NEBNext Ultra™ RNA Library Prep Kit from Illumina (NEB, USA). First, the mRNA was separated from the total RNA using poly-T oligo-attached magnetic beads and fragmented using divalent cations under elevated temperature in NEBNext First Strand Synthesis Reaction Buffer (5×). Second, the mRNA was reverse transcribed to synthesize double-stranded cDNA using random hexamer primers. Third, the base A and an adaptor with a hairpin loop structure were added to the 3′ ends of the cDNA to prepare for hybridization. The library fragments were purified with the AMPure XP system (Beckman Coulter, Beverly, USA) to select fragments 150–200 bp in length. Then, PCR was performed with universal PCR primers and an Index (X) Primer. Finally, the PCR products were purified (AMPure XP system) and the library quality was assessed using the Agilent Bioanalyzer 2100 system. The library was loaded into a flow cell and the fragments hybridized to the flow cell surface. Each bound fragment was amplified into a clonal cluster through bridge amplification. Sequencing reagents including fluorescently labelled nucleotides were added and the first base was incorporated. The flow cell was imaged and the emission from each cluster was recorded. The emission wavelength and intensity were used to identify the base. This cycle was repeated 150 times to create a read length of 150 bases on an Illumina HiSeq platform.
Data analysis
The original images from Illumina HiSeq were transformed into sequenced reads (raw data) by base calling, using the CASAVA package. The sequencing error rate and GC content of the data were calculated. Meanwhile, the raw data were refined by filtering the reads that contained the adapter, which had a ratio of “N” greater than 10%, and the low quality reads to obtain clean data for downstream analysis.
For mapping the reads, the reference genome FA file and the gene model annotation GTF file were downloaded from the Ensemble Database, URLs: ftp://ftp.ensembl.org/pub/release-81/fasta/saccharomyces_cerevisiae/dna/ and ftp://ftp.ensembl.org/pub/release-81/gtf/saccharomyces_cerevisiae/. The index of the reference genome was built using Bowtie v2.2.3, and paired-end reads were mapped to the reference genome using TopHat v2.0.12. The read numbers mapped to each gene were counted using HTSeq v0.6.1 and the fragments per kilobase per exon per million fragments mapped (FPKM)8 for each gene were calculated to estimate the gene expression level.
Different gene expression levels between the two groups were analyzed using statistical routines from the DESeq R package (1.18.0). In order to control the false discovery rate, the resulting P-values were corrected using Benjamini and Hochberg’s approach.9 Genes with a corrected P-value < 0.05 were considered differentially expressed. KEGG enrichment analysis for the differentially expressed genes was performed using the KOBAS 2.0 package. Gene Ontology (GO) enrichment of differentially expressed genes was carried out with online tools at http://geneontology.org/. Single nucleotide polymorphisms (SNPs) are genetic markers in the genome that form from variations in single nucleotides. After excluding duplicated reads and reordering the bam alignment results of each sample, the GATK2 (V3.2) software was used to perform SNP calling.
Real-time quantitative PCR (qPCR)
10 up-regulated and 10 down-regulated genes involved in different metabolism pathways were selected randomly to detect their expression in the two strains by qPCR. Reverse transcription was done using the TaKaRa PrimeScript™ RT-PCR (TaKaRa Japan) Kit and qPCR was done using the SYBR® Premix Ex Taq™ II kit (TaKaRa, Japan) in AnalytikJena qTOWERE2.2, each with three repeats. The 2−ΔΔCt method, with the expression of strain MF02 as the control and the expression of the ALG9 gene as the internal standard,10 was used to detect the relative expression levels. Primers for qPCR are listed in Table 1.
Table 1 Quantitative PCR primers of selected genes
Gene name |
Primer sequence |
ALG9-qF |
GTCACGGATAGTGGCTTTGGTG |
ALG9-qR |
TCTGGCAGCAGGAAAGAACTTG |
PDC5-qF |
TGATTCACGGTCCTCATGCC |
PDC5-qR |
TGTGGAGCATCAAAGACTGGC |
SSA3-qF |
AGCAGTTGGTATTGATTTGGGAAC |
SSA3-qR |
GACCAATTAACCGCTTTGCATC |
ABP140-qF |
TGATGATACAACCGGCGACAC |
ABP140-qR |
TTCTGATCGCCTGTGTTATCACC |
GRE3-qF |
AAGGAAGTTGGTGAAGGTATCAGG |
GRE3-qR |
TTGAAGGCGATTGGGAAGTG |
HXT1-qF |
AGTGAAAGTCAAGTGCAACCCG |
HXT1-qR |
ATGACTACCGTCGTGGTGCTTC |
IRE1-qF |
AAGGCGGCAGATAGTGGAAG |
IRE1-qR |
GTGGTTCTTGGAGCAGGAATG |
THI11-qF |
TGACCTCTGTTGCCTCTT |
THI11-qR |
TTCATACCGTAGTGCTTGG |
PGI1-qF |
ACGCTAACACTGCCAAGAAC |
PGI1-qR |
AGCCGACCAGACAGAGTAAC |
PFK27-qF |
CGATTCAGACTCTCACGATGG |
PFK27-qR |
AACCTTGGGACCTCTGTAGAC |
CNE1-qF |
AAGGTCCAGGCGAACTCAAT |
CNE1-qR |
GAGGATCACACCACTGATAACG |
ATP17-qF |
TATAGGTTCGGCACCAAATGC |
ATP17-qR |
GCCAAAGGCAATTATACCTAGAGC |
COX6-qF |
GTGCAAAGAGTGCTCAACAACTG |
COX6-qR |
GGGAACGCCCAATTCTTGTC |
COX7-qF |
AATCTTCCAATCTTCCACTAAACCTC |
COX7-qR |
CTATGCCTTCTTGGCTTTGATACC |
QCR10-qF |
TGGCGTACACTTCTCATCTGTCTTC |
QCR10-qR |
TTCCAATGTAGGTCCTAACAACGG |
OLI1-qF |
GGAGCAGGTATCTCAACAATTGG |
OLI1-qR |
AAACCTGTAGCTTCTGATAAGGCG |
RPS31-qF |
TTGGTCTTGAGATTGAGAGGTGG |
RPS31-qR |
AACACCAGCACCACAAGTTGG |
SKP1-qF |
CTGACGAAGATGACGACGATT |
SKP1-qR |
AGGGCTTGATGTTGAGGTAGT |
RKI1-qF |
GGCTCCATTGAACAGTATCCTC |
RKI1-qR |
ATAGACAAGCACCACCACCTT |
FBP1-qF |
CCAAGCAGACAACAACAACAAG |
FBP1-qR |
GCCTCATAAAGCAACCTCAGT |
AIF1-qF |
TGGTGTGTCTGTGGCAAATCAC |
AIF1-qR |
ATCAAAGCTGGCAGCCGTATC |
Driver mutant prioritization for differentially expressed genes
PheNetic is a network-based eQTL tool that traces differentially expressed genes to their driver mutants by multistep probabilistic network trimming with the Binary Determination algorithm. A background interaction network for S. cerevisiae containing 6592 genes and 135
266 interactions was built by integrating known protein–protein interactions, DNA–protein interactions, and metabolite coupling interactions with specific weights. In this study, we adopted the interaction network collected and curated by Dries De Maeyer et al.7 A differentially expressed network was built by linking all the differentially expressed genes through the background interaction network. A bundle of 2250 mutated genes, represented by sense mutations derived from the SNP and insertion/deletion calling results, was used to provide candidates for the driver mutants. A final network was achieved by eliminating the lower-weighted edges with a specific cost systematically until S(D), the surviving network score, was at its largest. For details, the parameters applied in this study were the 20 best paths to keep a maximum path length of 4 and a search tree cutoff of 0.05. The final network was visualized by an online SVG graphing API of D3JS (http://d3js.org) in the PheNetic package. The regulation spectra of high-ranking driver mutants were also submitted to perform functional analysis such as GO term enrichment and KEGG identification. GO term enrichment was carried out with online tools at http://geneontology.org/. KEGG identification was determined using a comprehensive report from the standalone package of KOBAS 2.0.
Results
Strain screening and glucose tolerance
The growth curves and ethanol yield curves of the control and mutant strains in YP40 medium are shown in Fig. 1. Both the growth rate and the ethanol yield of the mutant strain were higher than those of the control strain. The growth rate of strain UV02_HG was faster after 8 h incubation, and the mean maximum number of UV02_HG cells rose to 8.767 × 108 cells per mL, which was 27.86% higher than that of MF02. The mean maximum ethanol yield of strain UV02_HG rose to 15.304% (v/v), which was 8.88% higher than that for MF02.
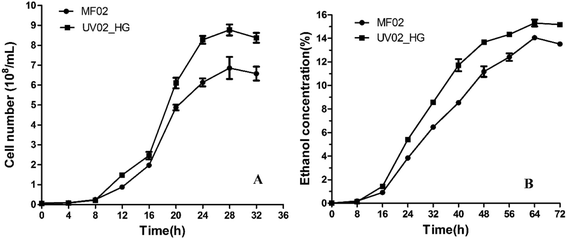 |
| Fig. 1 The curves of growth and ethanol yield for the mutant strain UV02_HG and control strain MF02 in YP40 medium. (A) Growth curves of the two strains; (B) ethanol yield curves of the two strains. | |
Reads quality evaluation
Two biological replicates were sequenced for each strain and the total raw data was deposited in the NCBI’s Gene Expression Omnibus public archive database (accession number GSE86448). The overall data quality is shown in Table 2. The Pearson correlation coefficient of determination, R2, was calculated to measure the quality of the biological replicates. In the experiment, we required the coefficient of determination R2 to be greater than 0.8 between biological replicates.11 As shown in Fig. 2, the coefficients of determination R2 between the two biological replicates were 0.965 and 0.892, indicating that the data were suitable for use in the subsequent analysis.
Table 2 Overall quality of the sequencing data
Sample namea |
Raw reads |
Clean reads |
Clean bases |
Error rate (%) |
Q20b (%) |
Q30b (%) |
GC content (%) |
“-1” and “-2” are biological replicates. Q20 and Q30 indicate that the correct rates of base recognition were 99% and 99.9%, correspondingly. |
MF02-1 |
33 642 972 |
32 455 452 |
4.87G |
0.02 |
96.48 |
91.25 |
40.76 |
MF02-2 |
35 445 516 |
34 114 618 |
5.12G |
0.02 |
96.47 |
91.30 |
40.50 |
UV02_HG-1 |
34 465 566 |
33 431 086 |
5.01G |
0.02 |
96.71 |
91.68 |
42.80 |
UV02_HG-2 |
38 395 836 |
37 512 216 |
5.63G |
0.02 |
96.78 |
91.78 |
41.63 |
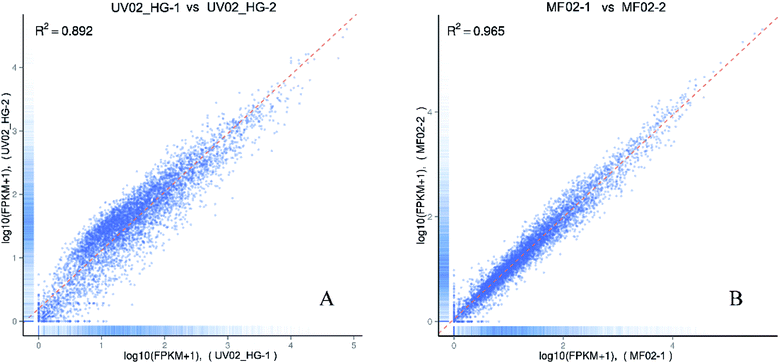 |
| Fig. 2 The Pearson correlation coefficients of determination, R2, between two biological replicates. (A) Biological replicates of strain UV02_HG; (B) biological replicates of strain MF02. | |
Analysis of differentially expressed genes and metabolic pathways
Based on the corrected P-value < 0.05, 1203 genes were considered to have significantly different expression in response to high sugar stress. As shown in Fig. 3, among these 1203 genes, 363 were up-regulated and 840 were down-regulated. The KEGG enrichment analysis results showed that the differentially expressed genes were found mainly in pathways such as the ribosome, oxidative phosphorylation, protein processing in the ER, thiamine metabolism, the phagosome, protein export, endocytosis, carbon metabolism, the MAPK pathway, and fatty acid and amino acid metabolism. All the genes involved in the ribosome and protein export were down-regulated and most of the genes involved in oxidative phosphorylation were also down-regulated. Genes involved in carbon metabolism (glycolysis, pyruvate metabolism, pentose phosphate pathway, citrate cycle, fructose and mannose metabolism, starch and sucrose metabolism, thiamine metabolism, and vitamin B6 metabolism), cell wall stress, high osmolarity via the MAPK pathway, fatty acid metabolism, and amino acid metabolism were up-regulated. Details are shown in Table 3. The most obvious enrichment was found for the ribosome, which had 72 enriched genes down-regulated. In view of the large gene number, we have not included them in Table 3. All of these genes were ribosomal proteins except RPL14A (SH3-like translation protein), RPL40A(B) (ubiquitin subgroup), RPS31 (ubiquitin subgroup), RPS10B (winged helix-turn-helix transcription repressor DNA-binding), RPS28A (nucleic acid-binding), and RPS23A(B) (nucleic acid-binding).
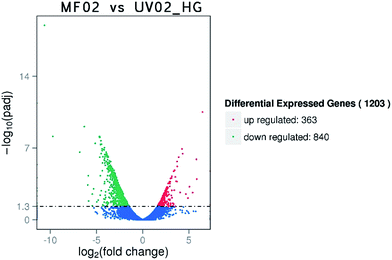 |
| Fig. 3 Overview of the differentially expressed genes between strains MF02 and UV02_HG under 40% (w/v) glucose stress. | |
Table 3 The significantly enriched KEGG pathways of differentially expressed genes between strains MF02 and UV02_HG
Gene name |
Description |
Corrected P-valuea |
log 2-fold changeb |
A hypergeometric test was used for statistical analysis, and P-values have been corrected for multiple testing by the Benjamini and Hochberg adjustment method. A corrected P value of <0.05 is considered statistically significant. log 2-fold change of differential expression; “+” means up-regulated genes, “−” means down-regulated genes. |
Oxidative phosphorylation |
COX12 |
Cytochrome c oxidase, subunit VI b |
1.54 × 10−4 |
−2.9 |
ATP17 |
ATPase, F0 complex, subunit F, mitochondria, fungi |
7.11 × 10−6 |
−3.4 |
VMA13 |
Vacuolar (H+)-ATPase H subunit |
1.81 × 10−2 |
+1.9 |
VMA10 |
Vacuolar (H+)-ATPase G subunit |
3.63 × 10−3 |
−1.7 |
QCR 10 |
Cytochrome b-c1 complex, subunit 10 |
6.35 × 10−5 |
−3.0 |
COX6 |
Cytochrome c oxidase subunit VIa |
1.56 × 10−2 |
−1.8 |
COX9 |
Cytochrome c oxidase, subunit VIIa |
6.14 × 10−3 |
−2.1 |
VMA1 |
ATPase, F1 complex beta subunit/V1 complex, C-terminal |
3.11 × 10−2 |
+1.8 |
QCR9 |
Ubiquinol-cytochrome-c reductase subunit 9 |
3.57 × 10−5 |
−3.1 |
QCR7 |
Cytochrome d ubiquinol oxidase, 14 kDa subunit |
1.58 × 10−2 |
−1.8 |
ATP19 |
ATPase, F0 complex, subunit K, mitochondrial |
3.08 × 10−3 |
−2.2 |
ATP20 |
ATPase, F0 complex, subunit G, mitochondrial |
2.89 × 10−3 |
−2.3 |
COX15 |
Heme A synthase |
3.22 × 10−2 |
+1.8 |
COX13 |
Cytochrome c oxidase subunit Via |
3.78 × 10−3 |
−2.2 |
TIM11 |
ATPase, F0 complex, subunit E, mitochondrial |
9.54 × 10−5 |
−2.9 |
COX8 |
Cytochrome c oxidase subunit VIIc |
1.84 × 10−3 |
−2.3 |
COX7 |
Cytochrome c oxidase subunit VII |
7.54 × 10−7 |
−3.8 |
ATP15 |
ATPase, F1 complex, epsilon subunit, mitochondrial |
5.87 × 10−3 |
−2.3 |
VMA2 |
ATPase, alpha/beta subunit, nucleotide-binding domain, active site |
2.52 × 10−2 |
+1.8 |
COX4 |
Cytochrome c oxidase, subunit Vb, zinc binding site |
4.16 × 10−3 |
−2.1 |
OLI1 |
ATPase, F0 complex, subunit C, DCCD-binding site |
4.04 × 10−3 |
−4.0 |
![[thin space (1/6-em)]](https://www.rsc.org/images/entities/char_2009.gif) |
Protein processing in the endoplasmic reticulum |
SSA1 |
Heat shock protein 70, conserved site |
2.55 × 10−2 |
+1.8 |
CNE1 |
Calreticulin/calnexin, conserved site |
4.44 × 10−2 |
+1.8 |
SSA3 |
Heat shock protein 70, conserved site |
1.87 × 10−4 |
+3.7 |
HSP26 |
HSP20-like chaperone |
1.95 × 10−4 |
+2.9 |
DER1 |
Der1p, ER membrane protein |
3.30 × 10−2 |
−1.8 |
PDI1 |
Protein disulfide isomerase, conserved site |
1.48 × 10−2 |
+2.0 |
YET3 |
B-cell receptor-associated 31-like |
3.67 × 10−2 |
−1.6 |
SEC31 |
Protein transport protein SEC31 |
3.08 × 10−2 |
+1.7 |
OST4 |
Oligosaccaryl transferase |
7.23 × 10−3 |
−2.0 |
SSS1 |
Protein translocase SecE domain |
1.86 × 10−2 |
−2.3 |
HSP42 |
HSP20-like chaperone |
3.02 × 10−3 |
+2.3 |
SKP1 |
E3 ubiquitin ligase, SCF complex, Skp subunit |
1.39 × 10−4 |
−2.7 |
SBH2 |
Preprotein translocase Sec, Sec61-beta subunit, eukarya |
2.56 × 10−4 |
−2.7 |
SSA4 |
Heat shock protein 70, conserved site |
2.25 × 10−2 |
+2.3 |
OST5 |
Oligosaccharyl transferase complex, subunit OST5 |
7.92 × 10−3 |
−2.3 |
IRE1 |
Pyrrolo-quinoline quinone beta-propeller repeat |
1.87 × 10−3 |
+2.5 |
SEC24 |
Sec23/Sec24 beta-sandwich |
2.02 × 10−2 |
+1.9 |
UBC7 |
Ubiquitin-conjugating enzyme, active site |
1.32 × 10−2 |
−1.9 |
SCJ1 |
Heat shock protein DnaJ, conserved site |
2.11 × 10−3 |
+2.4 |
SSB2 |
Heat shock protein 70, conserved site |
2.26 × 10−2 |
+1.8 |
![[thin space (1/6-em)]](https://www.rsc.org/images/entities/char_2009.gif) |
Thiamine metabolism |
THI21 |
Phosphomethylpyrimidine kinase |
4.45 × 10−3 |
+2.7 |
THI22 |
Phosphomethylpyrimidine kinase |
3.12 × 10−3 |
+4.7 |
THI11 |
Thiamine precursor HMP synthase |
3.14 × 10−11 |
+6.5 |
THI5 |
Thiamine precursor HMP synthase |
5.98 × 10−4 |
+5.1 |
THI13 |
Thiamine precursor HMP synthase |
1.09 × 10−4 |
+5.8 |
THI4 |
Thiamine thiazole synthase |
1.30 × 10−3 |
+3.4 |
THI12 |
Thiamine precursor HMP synthase |
1.27 × 10−6 |
+5.8 |
![[thin space (1/6-em)]](https://www.rsc.org/images/entities/char_2009.gif) |
Phagosome |
YPT7 |
Small GTP-binding protein domain |
1.54 × 10−2 |
−1.9 |
VMA10 |
Vacuolar (H+)-ATPase G subunit |
3.63 × 10−3 |
−1.7 |
VMA13 |
Vacuolar (H+)-ATPase H subunit |
1.81 × 10−2 |
+1.9 |
VMA1 |
ATPase, F1 complex beta subunit/V1 complex, C-terminal |
3.11 × 10−2 |
+1.8 |
ACT1 |
Actin/actin-like conserved site |
9.93 × 10−3 |
+2.1 |
TUB2 |
Tubulin, C-terminal |
1.72 × 10−2 |
+1.9 |
VPS27 |
VHS subgroup |
1.41 × 10−2 |
+2.0 |
![[thin space (1/6-em)]](https://www.rsc.org/images/entities/char_2009.gif) |
Protein export |
IMP1 |
Mitochondrial inner membrane protease subunit 1 |
7.92 × 10−4 |
−2.6 |
IMP2 |
Mitochondrial inner membrane protease subunit 2 |
1.41 × 10−2 |
−1.9 |
SRP14 |
Signal recognition particle, SRP9/SRP14 subunit |
9.19 × 10−3 |
−2.1 |
SPC1 |
Signal peptidase subunit 1 |
1.01 × 10−2 |
−2.0 |
SPC2 |
Signal peptidase complex subunit 2 |
1.54 × 10−3 |
−2.5 |
![[thin space (1/6-em)]](https://www.rsc.org/images/entities/char_2009.gif) |
Central carbon metabolism |
PGI1 |
Phosphoglucose isomerase, C-terminal |
4.98 × 10−2 |
+1.6 |
GND2 |
NAD(P)-binding domain |
7.83 × 10−3 |
+2.2 |
PKI1 |
Ribose 5-phosphate isomerase, type A |
2.37 × 10−2 |
−1.9 |
FBP1 |
Fructose-1,6-bisphosphatase, active site |
3.20 × 10−2 |
−1.6 |
PFK27 |
6-Phosphofructo-2-kinase, PFK27 type |
4.22 × 10−3 |
+3.1 |
TAL1 |
Transaldolase, active site |
4.68 × 10−2 |
+1.7 |
PMI40 |
Phosphomannose isomerase, type I, conserved site |
1.03 × 10−2 |
+2.0 |
GRE2 |
NAD(P)-binding domain |
3.22 × 10−2 |
+1.7 |
CDC19 |
Pyruvate kinase, active site |
1.37 × 10−3 |
+2.5 |
PDB1 |
Transketolase-like, C-terminal |
2.64 × 10−2 |
+1.8 |
PYC1 |
PreATP-grasp-like fold |
2.03 × 10−2 |
+1.9 |
MDH2 |
Lactate/malate dehydrogenase, C-terminal |
7.56 × 10−3 |
+2.2 |
DAL7 |
Malate synthase, conserved site |
2.49 × 10−3 |
+2.5 |
TDH1 |
Glyceraldehyde/erythrose phosphate dehydrogenase family |
8.27 × 10−4 |
+2.7 |
PDC6 |
Pyruvate decarboxylase/indolepyruvate decarboxylase |
1.01 × 10−2 |
+2.1 |
PDC5 |
Pyruvate decarboxylase/indolepyruvate decarboxylase |
1.37 × 10−3 |
+3.1 |
![[thin space (1/6-em)]](https://www.rsc.org/images/entities/char_2009.gif) |
VB6 metabolism |
SNZ2 |
Pyridoxine biosynthesis protein |
1.23 × 10−7 |
+4.3 |
SNZ3 |
Pyridoxine biosynthesis protein |
2.09 × 10−6 |
+3.7 |
THR4 |
Threonine synthase |
4.80 × 10−2 |
+1.6 |
![[thin space (1/6-em)]](https://www.rsc.org/images/entities/char_2009.gif) |
MAPK pathway |
YCK1 |
Protein kinase, ATP binding site |
3.16 × 10−2 |
+1.8 |
GPA1 |
G protein alpha subunit, helical insertion |
1.05 × 10−2 |
−2.2 |
FUS3 |
Protein kinase, ATP binding site |
2.15 × 10−3 |
−2.3 |
MCM1 |
Transcription factor, MADS-box |
1.63 × 10−2 |
+2.6 |
MTL1 |
Mid2-like cell wall stress sensor |
8.43 × 10−5 |
+3.1 |
SLT2 |
Protein kinase, ATP binding site |
1.28 × 10−3 |
+2.5 |
GSC2 |
1,3-Beta-glucan synthase subunit FKS1-like |
1.04 × 10−2 |
+2.8 |
MSB2 |
Msb2p |
4.28 × 10−2 |
+1.9 |
OPY2 |
Membrane anchor Opy2, N-terminal |
4.82 × 10−2 |
+1.7 |
SMP1 |
Transcription factor, MADS-box |
1.30 × 10−2 |
+2.1 |
TUP1 |
Chromatin-silencing transcriptional regulator |
6.89 × 10−3 |
+2.1 |
GRE2 |
NAD(P)-binding domain |
3.22 × 10−2 |
+1.7 |
CLB5 |
Cyclin A/B/D/E |
3.67 × 10−2 |
−1.7 |
GO enrichment analysis of the differentially expressed genes
GO (Gene Ontology) is a framework for biological modelling. GO defines concepts used to describe gene function and the relationships between these concepts. Based on sequence homology, the identified genes are grouped according to three aspects: molecular function, cellular component, and biological process. As shown in Fig. 4, in 40% (w/v) glucose conditions, the differentially expressed genes between the wild-type and mutant strains were categorized into three groups. In order to show the results concretely, we conducted GO enrichment for the up-regulated and down-regulated genes. The enriched GO terms for the down-regulated genes included 17 subcategories in the biological process group. The cellular process category included 330 genes significantly altered, the maximum number found in one category, and the organonitrogen compound metabolic process included 132 genes. The cellular component category included 11 subcategories, seven of which involved expression changes in more than 300 genes. The altered genes related to the ribosome, intracellular organelles, and associated organelles were each significantly different. The molecular function group only had two subcategories: the structural constituent of the ribosome and structural molecular activity. The structural constituent of the ribosome was significantly involved with 77 genes. In the enriched GO terms for the up-regulated genes, the biological process group included 15 subcategories, including the small molecule metabolic process involving 60 genes. The cellular component group had 12 subcategories, including the top-ranked cell periphery involving 43 genes. The actin cortical patch and endocytic patch were also significantly enriched. The molecular function group only included three subcategories and involved few genes.
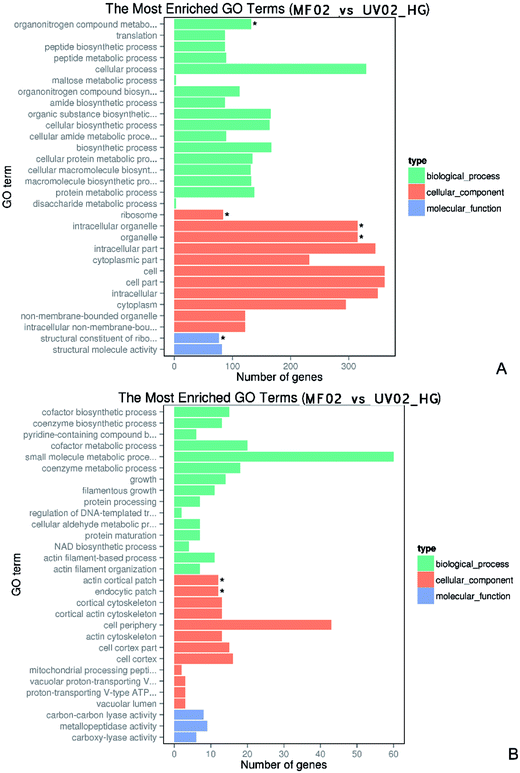 |
| Fig. 4 GO enrichment analysis of the differentially expressed genes between strains MF02 and UV02_HG under 40% (w/v) glucose stress. (A) The enriched GO terms of down-regulated genes; (B) the enriched GO terms of up-regulated genes. | |
qPCR
We randomly selected 10 up-regulated and 10 down-regulated genes involved in different metabolism pathways to confirm their expression in the two strains by qPCR. The values shown in Fig. 5 are the mean values of three parallel experiments. As shown in Fig. 5, the results of qPCR were consistent with those of RNA-Seq, except for the GRE3 gene. Therefore, the RNA-Seq accuracy rate was approximately 95%, indicating that the RNA-Seq results were credible. The relative expression levels of the AIF1 gene were −9.7 (RNA-Seq) and −3097 (qPCR). This result is not shown in Fig. 5 because of the large number.
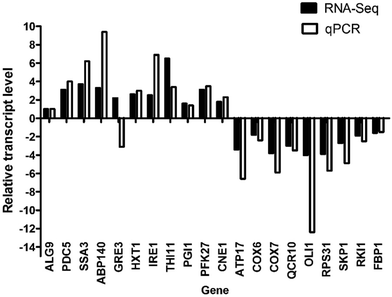 |
| Fig. 5 Real-time PCR analysis of the selected genes. | |
Unveiling the adaptive driver genes underlying high glucose tolerance
Interactions in the network could include protein–protein interactions, sigma factor–DNA interactions, transcription factor–DNA interactions, metabolic interactions, sRNA–protein interactions, and phosphorylation. However, we only found transcription factor–DNA interactions in this network. As Fig. 6 shows, STE12 and TUP1 are the suspected dominating driver mutants, with a resulting network of 75 genes connected by 77 interactions. For 1203 differentially expressed genes, 26 were selected in the resulting network by PheNetic. Each driver mutant was given a ranking score, indicating its capability of surviving the trimming algorithm. The lowest ranking score indicates the most important driver mutant. STE12 was ranked the number 1 driver mutant, affecting 16 genes, all of which were down-regulated. TUP1, ranked number 2, affected 9 genes. In addition to these two transcription regulators, there were 24 rated driver mutants retained by the resulting network. The names and descriptions of these genes are shown in order in Table 4. To reveal how the driver mutants affected expression levels, 75 genes were submitted for online GO term enrichment. For the biological process aspect, the regulation of transcription by RNA polymerase II promoters (25 genes), cellular processes (23 genes), and localization (2 genes) were the most enriched terms. For the molecular function aspect, transcriptional activator activity, RNA polymerase II core promoter proximal region sequence-specific binding (12 genes), RNA polymerase II core promoter proximal region sequence-specific DNA binding (7 genes), and catalytic activity (4 genes) were the most enriched terms. For the cellular component aspect, the intracellular membrane-bounded organelle (24 genes), cytoplasmic part (12 genes), and intracellular organelle part (11 genes) were the most enriched. KEGG term enrichment was also carried out with a standalone version of KOBAS 2.0. The MAPK signaling pathway (4 genes), cell cycle (4 genes), meiosis (3 genes), and the ubiquitin proteasome pathway (1 gene) were the most enriched terms.
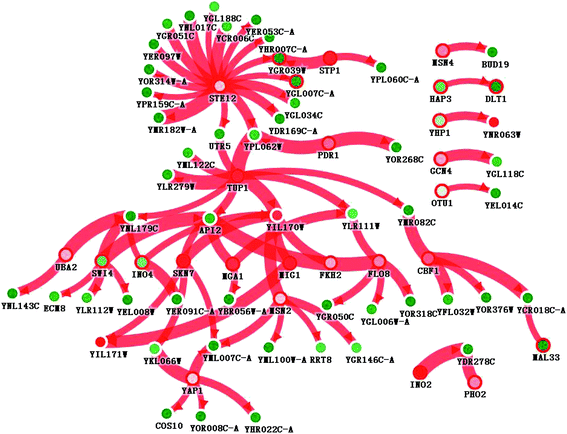 |
| Fig. 6 Visualization of the subnetwork inferred from the data of SNP calling and differentially expressed genes between strains MF02 and UV02_HG. There was only transcription factor–DNA interaction in the subnetwork; the breadth of the red line represents the chance that an interaction was involved. The inner color of the circle represents differential expression: red represents overexpression, green represents underexpression, and white represents no differential expression. The deepness of the color represents the degree of differential expression. A red color around the edge represents a mutated gene; a white color around the edge represents no mutation. | |
Table 4 In addition to STE12 and TUP1, 24 other genes were selected as drivers into the resulting network by PheNetic
Gene name |
Description |
FLO8 |
Transcription factor; required for flocculation, diploid filamentous growth, and haploid invasive growth |
INO2 |
Transcription factor; required for derepression of phospholipid biosynthetic genes in response to inositol depletion, involved in diauxic shift |
SWI4 |
DNA binding component of the SBF complex (Swi4p-Swi6p), a transcriptional activator that in concert with MBF regulates late G1-specific transcription of targets |
PDR1 |
Transcription factor that regulates the pleiotropic drug response |
UBA2 |
Subunit of heterodimeric nuclear SUMO activating enzyme E1 with Aos1p |
YAP1 |
Basic leucine zipper (bZIP) transcription factor; required for oxidative stress tolerance |
CBF1 |
Basic helix-loop-helix (bHLH) protein; associates with other transcription factors such as Met4p and Lsw1p to mediate transcriptional activation or repression |
MIG1 |
Transcription factor involved in glucose repression |
MSN2 |
Stress-responsive transcriptional activator |
YGR039W |
Dubious open reading frame |
STP1 |
Transcription factor; contains a N-terminal regulatory motif (RI) that acts as a cytoplasmic retention determinant and as an Asi dependent degron in the nucleus |
PHO2 |
Homeobox transcription factor; regulatory targets include genes involved in phosphate metabolism |
INO4 |
Transcription factor involved in phospholipid synthesis |
GCN4 |
BZIP transcriptional activator of amino acid biosynthesis |
SKN7 |
Nuclear response regulator and transcription factor; physically interacts with the Tup1-Cyc8 complex and recruits Tup1p to its targets |
MGA1 |
Protein similar to heat shock transcription factor |
FKH2 |
Forkhead family transcription factor; plays a major role in the expression of G2/M phase genes |
YHP1 |
Homeobox transcriptional repressor; binds Mcm1p and early cell cycle box (ECB) elements of cell cycle regulated genes, thereby restricting ECB-mediated transcription to the M/G1 interval |
MSN4 |
Stress-responsive transcriptional activator |
MAL33 |
MAL-activator protein |
YGL007C-A |
Putative protein of unknown function |
OTU1 |
Deubiquitylation enzyme that binds to the chaperone-ATPase; may contribute to regulation of protein degradation by deubiquitylating substrates that have been ubiquitylated by Ufd2p |
HAP3 |
Subunit of the Hap2p/3p/4p/5p CCAAT-binding complex; complex is heme-activated and glucose-repressed; complex is a transcriptional activator and global regulator of respiratory gene expression |
DLT1 |
Protein of unknown function |
The genes repressed by STE12 and TUP1 are poorly understood. As shown by the PheNetic analysis results, STE12 is a general transcription repressor for at least 16 genes and is highly associated with the S. cerevisiae osmosis stress response. We further investigated the STE12 regulation spectrum. The expression of YGL188C, YEL035C (UTR5), YGR039W, YER097W, YNL017C, YDR169C-A, YPL062W, YCR006C, YPR159C-A, YMR182W-A, YGL007C-A, YER053C-A, YGL034C, YGR051C, YHR007C-A, and YOR314W-A was repressed by STE12. Seven of those genes encode short peptides with less than 60 amino acids. None of them encode peptides longer than 180 amino acids. Although annotated by gene ontology, they were putative proteins or proteins with unknown functions and were thus poorly understood. Nevertheless, all of them are highly associated with the cell membrane when subjected to environmental osmosis stress. They interact with either the ER or cytoplasmic membrane through their hydrophobic transmembrane peptide regions.
The second most important driver mutant is TUP1. Unlike STE12, TUP1 represses a batch of genes through 3-layer or 4-layer cascades. Almost all of the terminal genes affected by TUP1 were down-regulated. These genes, like the STE12 subjects, are also poorly studied. GO term enrichment yields no significant result, indicating the wide and scattered regulation spectrum of TUP1. Among these genes, YKL066W, YCR018C-A, YER091C-A, YLR112W, YFL032W, and YOR318C could not be attributed to any gene function by GO analysis, but they may be osmotic stress response-related genes. YIL171W, YML100W, and YBR056W participate in sugar transport, synthesis, and degradation, especially for trehalose. Marked as dubious proteins, YEL008W, YGL006W-A, YGR050C, YGR146C-A, YHR022C-A, YML007C-A, YNL143C, and YOR376W may also be osmotic stress response related genes.
Discussion
High sugar stress is a challenge for S. cerevisiae primarily encountered during the fermentation of molasses in industrial production. The original strain, MF02, which was isolated in our laboratory from year-old sugar mill waste, could effectively ferment sugar cane molasses to produce ethanol. When fermenting 40 Bx cane molasses, the largest number of cells was 3.58 × 108 at 32 h, and the highest yield of ethanol was 11.07% (v/v) at 48 h. This strain had potential for use in industrial production, so we chose it as a primeval strain to obtain mutants with better characteristics. Strain UV02_HG was the mutant we obtained from MF02 by UV irradiation, which grew better and produced more ethanol in culture medium containing 40% (w/v) glucose. We tried to explore the driving factors of new characteristics using transcriptomic data. Differential expression between the two strains was mainly reflected in the ribosome, oxidative phosphorylation, protein processing in the endoplasmic reticulum (ER), thiamine metabolism, the phagosome, protein export, endocytosis, carbon metabolism and the MAPK pathway. Most of the differentially expressed genes involved in the ribosome, oxidative phosphorylation and protein export were down-regulated and most of the genes involved in the HOG pathway, carbon metabolism, protein processing in the ER, and the phagosome were up-regulated. Differential expression between the two strains was likely to show us that the mutant strain partially curtailed the functions of protein translation, respiration and protein export, while it enhanced the abilities of the stress response, protein processing and sugar use under high sugar stress. PheNetic analysis found 26 genes as driving factors for the characteristics of the mutant strain under high sugar stress. STE12 and TUP1 were ranked highly and were thought to be significant drivers.
MAPK pathway
S. cerevisiae cell responses to different stresses utilize different pathways to adapt to new situations. However, these responses may overlap. For example, the well-studied mitogen-activated protein kinase (MAPK) pathway could be triggered through pheromones, hyperosmotic pressure, cell wall stress or starvation. MAPK pathways are dynamic signaling modules for S. cerevisiae to respond to stressful environments. The MAPK-HOG pathway is a sensitive inducer for S. cerevisiae to perceive and quickly respond to osmolarity variations.12 The HOG pathway first senses hyperosmotic shock; then, an increased glycerol concentration activates the PKC pathway sensors; finally, Slt2p phosphorylation is triggered,13 connecting this pathway to the cell wall stress pathway. In this study, the up-regulation of Mtl1 (+3.1), Slt2 (+2.5), and Gsc2/Fks2 (+2.8) in the UV02_HG strain indicated more highly dynamic cell wall remodeling in the mutant strain under hyperosmotic stress. Differentially expressed genes involved in cell mating were all down-regulated in the mutant strain, including the a-factor receptor gene STE3 (YKL177W −4.2), signal transduction of the mating factor gene GPA1 (−2.2), and mitogen-activated protein kinase FUS3 (−2.3). These results are consistent with the fact that the mutant strain strongly prefers budding to mating when under hyperosmotic pressure.
The up-regulated genes in the HOG pathway included two up-stream genes (Msb2 (+1.9) and Opy2 (+1.7)) and four tail-end genes (Smp1 (+2.1), Mcm1 (+2.6), Tup1 (+2.1) and Gre2 (+1.7)). The up-regulation of the four tail-end genes affected a series of genes involved in glycerol accumulation, the cell wall, cell cycle and cell growth to help S. cerevisiae cells to respond to external hypertonic stress.14–16 The Tup1-Ssn6 complex is an important transcriptional repressor for S. cerevisiae cells that is used to adapt to nonstandard growth. More than 300 genes were significantly de-repressed when the Tup1 gene was deleted.17 In our results, Tup1 was up-regulated 2.1-fold in the mutant strain. However, the genes repressed by Tup1, as observed by Sarah R. Green et al., were not all down-regulated in our results. The genes involved in carbon metabolism, including THI11 (+6.5), SNZ2 (+4.3), SNZ3 (+3.7), THI22 (+4.7), AAD6 (+2.0), and TDH1 (+2.7), were up-regulated. Therefore, we considered that the changed gene expressions found in the deletion study were not all induced directly by the deletion of one gene, but may have been induced by metabolic variations triggered by the deleted gene.
Genes involved in membrane and cytoplasmic proteins
Under high sugar stress, except for the differentially expressed genes involved in protein export, most of the differentially expressed genes involved in the membrane and cytoplasmic proteins of the UV02_HG strain were up-regulated, such as protein processing in the ER, the phagosome (Table 3) and endocytosis (SSA3 (+3.7), VPS27 (+2.0), HSE1 (+1.9), SSA4 (+2.3), SSA1 (+1.8) and SSB2 (+1.8)). This result illustrated that mutant cells enhanced their inner protein disposal, while protein export was decreased when the cells were exposed to a high osmotic environment. Molecular chaperones (SSA1 (+1.8), SSA3 (+3.7), SSA4 (+2.3), SSB2 (+1.8), SCJ1 (+2.4), HSP26 (+2.9), HSP42 (+2.3), CNE1 (+1.8) and PDI (+2.0)) played an important role in this process. Protein folding and degradation in the ER are disturbed when the cells experience environmental stresses. Unfolded proteins accumulate in the ER, activate the unfolded protein response (UPR), and increase the expression of genes encoding ER chaperones to equilibrate cellular proteins.18 Heat shock proteins in the cytoplasm or in the ER regulate protein folding and secretion, as molecular chaperones are important in the cellular stress response and are activated by various metabolic stresses.19 Calnexin is an ER membrane protein that acts as a molecular chaperone and is associated with new glycoprotein synthesis and protein folding intermediates.20,21 Under heat stress conditions, calnexin may interact with protein disulfide isomerase (PDI), which is also a molecular chaperone in the ER, and may play a major role in the protein-folding process.22 Ire1 can be activated by unfolded proteins as an ER stress sensor.18 Ethanol stress reduces the viability of ire1Δ cells more than that of IRE1+ cells.23 Vascular H+-ATPases (V-ATPases) are ATP-driven proton pumps in the endomembrane system of all eukaryotic cells. V-ATPase assembly is regulated by glucose, pH and osmotic stress in S. cerevisiae and is closely associated with glycolysis and protein kinase A (PKA).24,25
Genes involved in the main glucose metabolism pathway
Sugars are the preferred food for S. cerevisiae. Glucose is a monosaccharide that directly participates in sugar metabolism to generate energy and the by-products are used in the synthesis of other molecules. Expression analysis between the two strains revealed that most of the genes involved in glycolysis/gluconeogenesis, the pentose phosphate pathway (PPP), pyruvate metabolism, and the citrate cycle of the mutant strain were up-regulated (PGI (+1.6), PFK27 (+3.0), TDH1 (+2.7), CDC19 (+2.5), PDC5 (+3.1), PDC6 (+2.1), PDB1 (+1.8), PYC1 (+1.9), MDH2 (+2.2), DAL7 (+2.5), GND2 (+2.2) and TAL1 (+1.7)). However, two genes from these pathways were down-regulated: FBP1 (−1.6) and RKI1 (−1.9). The functions of these genes in metabolism pathways are shown in Fig. 7. The FBP1 gene is responsible for the conversion of fructose-1,6-bisphosphate to fructose-6-phosphate. PFK27 encodes 6-phosphofructo-2-kinase and phosphorylates fructose-6-phosphate into fructose 2,6-bisphosphate.26 Fructose 2,6-bisphosphate can stimulate phosphofructokinase, increase the glycolysis rate, and inhibit gluconeogenesis.27,28 The synergy between PFK27 and FBP1 should contribute to the advantages of the mutant strain in high sugar conditions. The RKI1 gene was down-regulated by sugar stress and might decrease the non-oxidative part of the pentose phosphate pathway, which is consistent with the results of Daniel J. Erasmus et al.6 The TDH1 gene encodes glyceraldehyde-3-phosphate dehydrogenase (GAPDH), which is responsible for the conversion of glyceraldehyde-3-phosphate to 1,3 bisphosphoglycerate. CDC19 encodes pyruvate kinase, which catalyzes the conversion of phosphoenolpyruvate to pyruvate and produces two ATP molecules. Many molecular chaperones depend on the energy from ATP hydrolysis.29 Reduced pyruvate kinase expression causes a decrease in glucose consumption, the glycolysis rate, and the specific growth rate.30 Increasing pyruvate kinase activity is required for high-temperature tolerance in S. cerevisiae,31 and for high-glucose tolerance in this study.
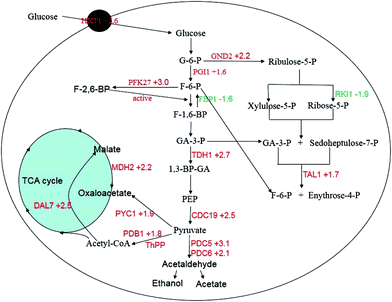 |
| Fig. 7 Genes with differential expression involved in the central carbon metabolism. The up-regulated genes are marked in red and the down-regulated genes are marked in green. | |
Pyruvate is a major junction between assimilation and dissimilation, which branches into three metabolism pathways in S. cerevisiae: (i) conversion to acetyl-CoA by the pyruvate dehydrogenase complex, (ii) conversion to acetaldehyde by pyruvate decarboxylase, and (iii) conversion to oxaloacetate by pyruvate carboxylase.32 Acetyl-CoA and oxaloacetate condense into citrate and enter the citrate cycle. The citrate cycle is the last metabolism pathway utilized by sugars, lipids and amino acids and provides free energy and precursor substances for cells. Excess acetyl-CoA is an activator of pyruvate carboxylase, catalyzing the conversion of pyruvate to oxaloacetate.33
After the conversion of pyruvate to acetyl-CoA by PDB1 (+1.8), malate synthesis is catalyzed by Dal7p (+2.5), which is an Mls1p isoenzyme.34 Next, malate is converted to oxaloacetate by malate dehydrogenase, encoded by MDH2 (+2.2).35 These genes act cooperatively to propel the TCA cycle and provide energy to cells.
Pyruvate decarboxylase is a key enzyme in S. cerevisiae, catalyzing the conversion of pyruvate to acetaldehyde. Acetaldehyde branches directly to ethanol or indirectly to acetyl-CoA through acetate. Three structure genes, PDC1, PDC5 and PDC6, were characterized. The PDC− S. cerevisiae strain was unable to grow in basic medium with glucose as the sole carbon source. However, these three genes contribute differently to the activity of pyruvate decarboxylase. PDC1 encodes the main PDC activity, PDC5 compensates for PDC1 under certain conditions, and PDC6 is used for nonfermentable carbon sources.36,37 Daniel J. Erasmus et al.6 found that PDC6 is up-regulated 26-fold in grape juice containing 40% (w/v) sugar (equimolar amounts of glucose and fructose) compared to in 22% (w/v) sugar, whereas PDC5 was unexpectedly down-regulated. In our study, both PDC5 and PDC6 were up-regulated in the mutant strain under 40% (w/v) glucose stress.
Pyruvate carboxylase plays an important anaplerotic role in oxaloacetate supplementation. Two isozymes, PYC1 and PYC2, were identified.38 PYC2 was activated only in the early growth stage, whereas PYC1 played an important role not only in fermentative growth, but also in gluconeogenic growth.39
Cofactors play an essential role in many biochemical reactions and metabolic pathways. Vitamin B1 forms the enzyme cofactor for thiamin diphosphate (ThPP), which is essential for sugar metabolism. Pyruvate decarboxylase (PDC1p, PDC5p and PDC6p), pyruvate dehydrogenase (PDA1p) and transketolase (TKl1p and TKL2p) are ThPP-dependent enzymes.40 Vitamin B6 (pyridoxal, pyridoxine or pyridoxamine) is not only an important cofactor for amino acid metabolism, but is also the precursor for thiamin (vitamin B1). Thiamin includes two ring structures, 4-methyl-5-(L-hydroxymethyl) thiazole (HET) and 2-methyl-4-amino-5-hydroxy-methylpyrimidine (HMP). Pyridoxine and histidine are the precursors to the HMP unit of thiamin.41 The gene THI4 regulates HET biosynthesis, and the THI5 gene family (THI5, THI11, THI12 and THI13) regulates HMP biosynthesis.42,43 The gene families SNO1–3 and SNZ1–3 are responsible for the biosynthesis of vitamin B6. SNO2/SNZ2 and SNO3/SNZ3 are used in the biosynthesis of vitamin B1 during the exponential growth phase.44
Relative to the reference laboratory strain S. cerevisiae S288C, the SNO and SNZ genes involved in the biosynthesis of vitamin B1 and vitamin B6 were significantly amplified in five industrial S. cerevisiae strains responsible for fuel ethanol production from sugarcane in Brazil.45 In this study, SNZ2 (+4.3), SNZ3 (+3.7), THR4 (+1.6), THI4 (+3.4), THI5 (+5.0), THI11 (+6.5), THI12 (+5.8), THI13 (+5.8), THI21 (+2.7) and THI22 (+4.7) were up-regulated in the UV02_HG strain in the 40% (w/v) glucose condition. From vitamin B6 to vitamin B1, to ThPP-dependent enzymes, a continuous line of coadjutants shunts the intermediate products of glycolysis and coordinates sugar metabolism.
Genes involved in mitochondrial functions
Most of the differentially expressed genes involved in mitochondrial functions were down-regulated, including mitochondrial inner and outer membrane protease and translocase: IMP1 (−2.6), IMP2 (−1.9), TOM6 (−2.4), TOM7 (−2.4), TIM12 (−2.3), TIM9 (−2.5), TIM10 (−2.9), SFC1 (−2.1), DIC1 (−1.7) and AAC1 (−1.5); oxidative phosphorylation: COX4 (−2.1), COX6 (−1.8), COX7 (−3.8), COX8 (−2.3), COX9 (−2.1), COX12 (−2.8), COX13 (−2.2), COX15 (+1.8), QCR7 (−1.8), QCR9 (−3.1), QCR10 (−3.0), ATP15 (−2.3), ATP17 (−3.4), ATP19 (−2.2), ATP20 (−2.3), VMA1 (+1.8), VMA2 (+1.8), VMA10 (−1.7), VMA13 (+1.9), OLI1 (−4.0) and TIM11 (−2.9); and riboflavin: FMN1 (−2.3), LIP1 (−2.1) and RIB5 (−2.8). Mitochondria are essential organelles for eukaryotes that provide energy to cells by oxidative phosphorylation.46 Respiratory chains shuttle elements in the mitochondrial inner membrane, supplying optimized energy to cells depending on cell requirements and external factors.47 S. cerevisiae cells easily lose their respiratory function to increase their resistance to stress by deletions in mitochondrial DNA.48 The TCA cycle is affected in cells with dysfunctional mitochondria and respiratory deficiency. Acetyl-CoA and oxaloacetate (OAA) must be complemented by other pathways.49 In the UV02_HG strain, the expression of genes involved in the supplement of acetyl-CoA and OAA (PDB1, PYC1, MDH2, FAA4 and BAT1) was elevated. These results are consistent with those of Charles B. Epstein et al.46 Therefore, down-regulation of the genes involved in mitochondrial functions is a rational phenomenon for S. cerevisiae cells to resist high glucose stress and obtain a higher ethanol yield. Meanwhile, subdued respiration didn’t repress the growth of UV02_HG, which may be due to energy remediation by glycolysis and the TCA cycle.
The driver mutation interaction network
According to the PheNetic analysis, 26 genes with SNP calling were identified as hits for driving factors. 9 of them were the differently expressed genes TUP1 (+2.1), INO2 (+3.0), MIG1 (+2.2), STP1 (+2.1), YGR039W (−3.3), MGA1 (+2.0), MAL33 (−2.6), YGL007C-A (−2.4) and DLT1 (−2.9). The 5 up-regulated genes were all transcription factors; TUP1 was a chromatin-silencing transcription regulator, which repressed the transcription of more than 100 genes. INO2 was a component of the heteromeric Ino2p/Ino4p basic helix-loop-helix transcription activator that binds inositol/choline-responsive elements (ICREs), which are required for the de-repression of phospholipid biosynthetic genes in response to inositol depletion. MIG1 was a transcription factor involved in glucose repression, which regulates filamentous growth in response to glucose depletion. STP1 was a transcription factor involved in pre-tRNA processing and SpS amino acid sensing. MGA1 was a protein similar to a heat shock transcription factor. 3 of the 4 down-regulated genes had dubious open reading frames, except MAL33. MAL33 was a MAL-activator protein. In 26 drivers, STE12 and TUP1 ranked highly as suspected dominating driver mutants. The genes affected by STE12 and TUP1 in the resulting network were involved mainly in RNA transcription, cellular processes, and membrane proteins. Most of these genes are putative proteins or have dubious open reading frames. Sixteen poorly understood genes repressed by STE12 were annotated by gene otology and all are highly associated with the cell membrane. We inferred that these genes are either transport facilitators or proteins vital for the stress response. This study illuminated a new STE12 function. It may play a core role in the osmotic stress response by affecting the cell membrane. Whether this function is related to protein processing and protein export requires more verification.
Tup1 is recognized as a global repressor that interacts with histone proteins to modulate the chromatin response to changing environmental conditions.50 In the resulting network, some transcription factors, such as MSN2, SKN7, MIG1 and YAP1, were related to TUP1 in different regulation layers. MSN2 is a main stress response regulator. S. cerevisiae cells sense environmental stress from various signaling pathways and MSN2 integrates these signals and promptly activates a nuclear response.51 YAP1 activates and cooperates with SKN7 in response to redox stress signals.52 TUP1 cooperates with MIG1 and regulates glycolysis and gluconeogenesis.53 This network may help to identify the interactions between TUP1 and other transcription factors during high osmotic stress. Both TUP1 and MSN2 are located downstream of the MAPK pathway, which may facilitate the identification of the MAPK pathway downstream metabolism response to high sugar stress by the two genes. On the other hand, the genes regulated by TUP1 are largely poorly understood, highlighting the necessity of further studies to better understand the roles of those genes in the stress response.
In this study, we speculated a mechanism of the adaptive phenotype of the mutant strain. Mutations or differential expression of the 26 driver genes triggered the differential expression of genes involved in the metabolic pathways (ribosome, oxidative phosphorylation, protein processing in the ER, phagosome, protein export, endocytosis, carbon metabolism and MAPK pathway). Adjusting the metabolism endowed the mutant strain with better characteristics, a higher tolerance to glucose and fast growth in high sugar medium. Further work is needed to confirm the respective effects of 26 driver genes on characteristic evolution.
Conclusions
The high sugar condition is a common stressor to S. cerevisiae cells used in brewing and fermentation. We generated a mutant strain with improved glucose tolerance by UV irradiation. Transcriptome sequencing was used to identify differences between the wild-type strain and the mutant strain in gene expression levels to understand the changed response. Expression analysis of the sequencing results found that the stress responses were embodied mainly in the MAPK pathway, protein translation, processing and transport, mitochondrial dysfunction, and carbon metabolism. Network-based analysis identified the priority driver mutations. Based on the network, we speculated that the transcription factors STE12 and TUP1 are two main drivers used to elicit the S. cerevisiae adaptive phenotype. Most of the genes regulated by STE12 and TUP1 had decreased expression and were putative proteins or had dubious open reading frames. Sixteen poorly understood genes repressed by STE12 were annotated by gene otology and were highly associated with the cell membrane. More experimental work is needed to verify the functions of STE12 and TUP1 and the poorly understood genes that they regulate.
Acknowledgements
This study was funded by the General Program of the Natural Science Foundation of Guangxi, China (2014GXNSFAA118103), Natural Science Foundation for Young Scholars of Guangxi, China (2015GXNSFBA139044), and Scientific Research and Technological Development Foundation of Guangxi, China (15104001-8; 14123001-6; 14122004-1; 14122004-3; 1517-07).
References
- S. R. Hughes, W. R. Gibbons, S. S. Bang, R. Pinkelman, K. M. BischoV, P. J. Slininger, N. Qureshi, C. P. Kurtzman, S. Liu, B. C. Saha, J. S. Jackson, M. A. Cotta, J. O. Rich and J. E. Javers, J. Ind. Microbiol. Biotechnol., 2012, 39, 163–173 CrossRef CAS PubMed.
- S. Rudd, Trends Plant Sci., 2003, 8, 321–329 CrossRef CAS PubMed.
- S. Kalra, B. L. Puniya, D. Kulshreshtha, S. Kumar, J. Kaur, S. Ramachandran and K. Singh, PLoS One, 2013, 8, e83336 Search PubMed.
- H. Saito and F. Posas, Genetics, 2012, 192, 289–318 CrossRef CAS PubMed.
- E. J. Martí, A. Zuzuarregui, M. G. Alba, D. Gutiérrez, C. Gil and M. D. Olmo, Int. J. Food Microbiol., 2011, 145, 211–220 CrossRef PubMed.
- D. J. Erasmus, G. K. V. D. Merwe and H. J. J. V. Vuuren, FEMS Yeast Res., 2003, 3, 375–399 CrossRef CAS PubMed.
- D. D. Maeyer, B. Weytjens, L. D. Raedt and K. Marchal, Genome Biol. Evol., 2016, 8, 481–494 CrossRef PubMed.
- C. Trapnell, B. A. Williams, G. Pertea, A. Mortazavi, G. Kwan, M. J. V. Baren, S. L. Salzberg, B. J. Wold and L. Pachter, Nat. Biotechnol., 2010, 28, 511–518 CrossRef CAS PubMed.
- Y. Benjamini and Y. Hochberg, J. Roy. Stat. Soc. B, 1995, 57, 289–300 Search PubMed.
- M. A. Teste, M. Duquenne, J. M. Francois and J. L. Parrou, BMC Mol. Biol., 2009, 10, 1–15 CrossRef PubMed.
- Y. Ding, X. Zhang, K. W. Tham and P. Z. Qin, Nucleic Acids Res., 2014, 42, e140 CrossRef PubMed.
- A. P. Capaldi, T. Kaplan, Y. Liu, N. Habib, A. Regev, N. Friedman and E. K. O’Shea, Nat. Genet., 2008, 40, 1300–1306 CrossRef CAS PubMed.
- L. J. G. A. Rodríguez, R. Valle, A. n. Durán and C. s. Roncero, FEBS Lett., 2005, 579, 6186–6190 CrossRef PubMed.
- E. l. d. Nadal, L. C. Casadome and F. Posas, Mol. Cell. Biol., 2003, 23, 229–237 CrossRef PubMed.
- F. Lim, A. Hayes, A. G. West, A. P. Taylor, Z. Darieva, B. A. Morgan, S. G. Oliver and A. D. Sharrocks, Mol. Cell. Biol., 2003, 23, 450–461 CrossRef CAS PubMed.
- J. Warringer and A. Blomberg, Yeast, 2006, 23, 389–398 CrossRef CAS PubMed.
- S. R. Green and A. D. Johnson, Mol. Biol. Cell, 2004, 15, 4191–4202 CrossRef CAS PubMed.
- D. Pincus, M. W. Chevalier, T. Aragona, E. V. Anken, S. E. Vidal, H. El-Samad and P. Walter, PLoS Biol., 2010, 8, 1–14 Search PubMed.
- A. F. Jarnuczak, C. E. Eyers, J.-M. Schwartz, C. M. Grant and S. J. Hubbard, Proteomics, 2015, 3126–3139 CrossRef CAS PubMed.
- W. Ou, P. Cameron, D. Thomas and J. Bergeron, Nature, 1993, 364, 771–776 CrossRef CAS PubMed.
- F. E. Ware, A. Vassilakos, P. A. Peterson, M. R. Jackson, M. A. Lehrman and D. B. Williams, J. Biol. Chem., 1995, 270, 4697–4704 CrossRef CAS PubMed.
- H. Zhang, J. He, Y. Ji, A. Kato and Y. Song, Cell. Mol. Biol. Lett., 2008, 13, 38–48 CAS.
- K. I. Miyagawa, Y. I. Kimata, K. Kohno and Y. Kimata, Biosci., Biotechnol., Biochem., 2014, 78, 1389–1391 CrossRef CAS PubMed.
- K. J. Parra, C. Chan and J. Chen, Eukaryotic Cell, 2014, 13, 706–714 CrossRef PubMed.
- S. Bond and M. Forgac, J. Biol. Chem., 2008, 283, 36513–36522 CrossRef CAS PubMed.
- E. Boles, H. W. H. Gohlmann and F. K. Zimmermann, Mol. Microbiol., 1996, 20, 65–76 CrossRef CAS PubMed.
- E. V. Schaftingen, L. Hue and H. G. Hers, Biochem. J., 1980, 192, 887–895 CrossRef PubMed.
- J. A. Benanti, S. K. Cheung, M. C. Brady and D. P. Toczyski, Nat. Cell Biol., 2007, 9, 1184–1191 CrossRef CAS PubMed.
- S. Lindquist, Curr. Opin. Genet. Dev., 1992, 2, 748–755 CrossRef CAS PubMed.
- A. K. Pearce, K. Crimmins, E. Groussac, M. J. E. Hewlins, J. R. Dickinson, J. Francois, I. R. Booth and A. J. P. Brown, Microbiology, 2001, 147, 391–401 CrossRef CAS PubMed.
- S. Benjaphokee, P. Koedrith, C. Auesukaree, T. Asvarak, M. Sugiyama, Y. Kaneko, C. Boonchird and S. Harashima, New Biotechnol., 2012, 29, 166–176 CrossRef CAS PubMed.
- J. T. Pronk, H. Y. Steensma and J. P. V. Dijken, Yeast, 1996, 12, 1607–1633 CrossRef CAS PubMed.
- M. F. Utter and D. B. Keech, J. Biol. Chem., 1960, 235, 17–18 Search PubMed.
- E. Fernandez, M. Fernandez and R. Rodicio, FEBS Lett., 1993, 320, 271–275 CrossRef CAS PubMed.
- K. I. Minard and L. M. Henn, Mol. Cell. Biol., 1991, 11, 370–380 CrossRef CAS PubMed.
- S. Hohmann, J. Bacteriol., 1991, 173, 7963–7969 CrossRef CAS PubMed.
- M. T. Flikweert, L. V. D. Zanden, W. M. T. M. Janssen, H. Y. Steensma, J. P. V. Dijken and J. T. Pronk, Yeast, 1996, 12, 247–257 CrossRef CAS PubMed.
- M. E. Walker, D. L. Val, M. Rohde, R. J. Devenish and J. C. Wallace, Biochem. Biophys. Res. Commun., 1991, 176, 1210–1217 CrossRef CAS PubMed.
- N. K. Brewster, D. L. Val, M. E. Walker and J. C. Wallace, Arch. Biochem. Biophys., 1994, 311, 62–71 CrossRef CAS PubMed.
- S. Hohmann and P. A. Meacock, Biochim. Biophys. Acta, 1998, 1385, 201–219 CrossRef CAS.
- K. Nosaka, Appl. Microbiol. Biotechnol., 2006, 72, 30–40 CrossRef CAS PubMed.
- U. M. Praekelt, K. L. Byrne and P. A. Meacock, Yeast, 1994, 10, 481–490 CrossRef CAS PubMed.
- R. Wightman and P. A. Meacock, Microbiology, 2003, 149, 1447–1460 CrossRef CAS PubMed.
- S. R. Navarro, B. Llorente, M. T. R. Manzaneque, A. Ramne, G. Uber, D. Marchesan, B. Dujon, E. Herrero, P. Sunnerhagen and J. E. P. Ortin, Yeast, 2002, 19, 1261–1276 CrossRef PubMed.
- B. U. Stambuk, B. Dunn, S. L. Alves, E. H. Duval and G. Sherlock, Genome Res., 2009, 19, 2271–2278 CrossRef CAS PubMed.
- C. B. Epstein, J. A. Waddle, W. Hale, V. Dave, J. Thornton, T. L. Macatee, H. R. Garner and R. A. Butow, Mol. Biol. Cell, 2001, 12, 297–308 CrossRef CAS PubMed.
- C. R. Lundin, C. V. Ballmoos, M. Ott, P. Ädelroth and P. Brzezinski, Proc. Natl. Acad. Sci. U. S. A., 2016, E4476–E4485 CrossRef CAS PubMed.
- A. V. Litvinchuk, S. S. Sokolov, A. G. Rogov, O. V. Markova, D. A. Knorre and F. F. Severin, Eur. J. Cell Biol., 2013, 50720, 50721–50726 Search PubMed.
- Z. Liu and R. A. Butow, Annu. Rev. Genet., 2006, 40, 159–185 CrossRef CAS PubMed.
- J. M. Rizzo, P. A. Mieczkowski and M. J. Buck, Nucleic Acids Res., 2011, 39, 8803–8819 CrossRef CAS PubMed.
- N. Petrenko, R. V. Chereji, M. N. McClean, A. V. Morozovb and J. R. Broach, Mol. Biol. Cell, 2013, 24, 2045–2057 CrossRef CAS PubMed.
- A. Delaunay, A. D. Lsnard and M. B. Toledano, EMBO J., 2000, 19, 5157–5166 CrossRef CAS PubMed.
- B. K. Han and S. D. Emr, J. Biol. Chem., 2013, 288, 20633–20645 CrossRef CAS PubMed.
Footnote |
† Electronic supplementary information (ESI) available. See DOI: 10.1039/c7ra06146c |
|
This journal is © The Royal Society of Chemistry 2017 |
Click here to see how this site uses Cookies. View our privacy policy here.