DOI:
10.1039/C7RA09522H
(Paper)
RSC Adv., 2017,
7, 51069-51078
Discovery and verification of the potential targets from bioactive molecules by network pharmacology-based target prediction combined with high-throughput metabolomics†
Received
28th August 2017
, Accepted 13th October 2017
First published on 2nd November 2017
Abstract
Natural products are an invaluable source for drug candidates. Currently, plasma metabolome has suggested that compounds present in herbs may exert bioactivity. The present investigation employed global metabolome analysis technology to explore the key target and action mechanism of scoparone, a representative ingredient of Yinchenhao (Artemisia capillaris Thunb.). First, we applied different databases for target prediction and focused on the potential targets of scoparone by network pharmacology, which also theoretically characterizes the effectiveness of scoparone on molecular docking. Among them, we selected the top predictions as the potential and crucial target. Then, non-targeted metabolomics technology based on an advanced UPLC-MS instrument coupled with a robust data processing platform was employed to characterize the metabolic profiling of alcoholic liver disease (ALD) rats. Furthermore, the ingenuity pathway analysis platform was used for metabolic network analysis, which mainly involved multiple-pathways, including tyrosine metabolism, glutathione metabolism, and primary bile acid biosynthesis. Interestingly, as a core biomarker, dopaquinone is directly related with target prediction of tyrosinase and finally resulted in a series of disturbances. Moreover, the prediction also validated the target on a metabolic level. The present investigation demonstrated that global metabolome analysis could provide a novel strategy for deciphering the potential drug targets of natural products.
Introduction
Natural products are indispensable candidates in current drug discovery programs, which have inspired chemists and physicians for millennia.1,2 However, specific action mechanisms and target locations appear to be the bottleneck for further development of natural products. Therefore, novel strategies or combined methodologies are required to decipher complex systems. With the revolutionary development of analytical instruments and the emergence of omics technology, much more biological information can be excavated, which can greatly accelerate exploration of the life sciences and can provide an expansive horizon for the interpretation of complex systems. Among them, metabolomics technology was proposed as a new strategy followed by genomics, transcriptomics, and proteomics. It focuses on small molecule metabolites generated with the expression of exclusive enzymatic protein. With advanced and comprehensive data collection technology, integrity and system phenotypic characterization could be precisely obtained.3–10 Moreover, a robust analysis platform provides unparalleled technical support. With high throughput and resolution, SYNAPT™-G2Si-HDMS possesses the latest technology in ion mobility spectrometry performance, which combined with the proprietary technology of T-wave™ and Quantof can greatly improve the resolution and sensitivity into 40
000 and 10
000 dpi, respectively. Then, we applied Progenesis QI software (Nonlinear Dynamics, 2014, version 1.0) for alignment processing, peak picking, normalization, deconvolution, identification and multivariate statistical analysis.
Increasing improvements in bioinformatics have provided multiple opportunities to locate action targets and to determine drug effects from rich databases and algorithmic resources.11–13 Yinchenhao possess an irreplaceable effect for the treatment of liver disease or jaundice in the application of TCM. Therefore, it is necessary to investigate the active ingredient and to define the mechanism of this medication. Based on the theory of plasma pharmacochemistry and pharmacology, we focused on scoparone as the representative ingredient of Yinchenhao (Artemisia capillaris Thunb.). Herein, we first applied a network pharmacology approach based on two independent machine learning systems for the target prediction of scoparone. A constructed platform, including UPLC-high definition mass spectrometry metabolomics, a Progenesis QI and an IPA network data processing system (Qiagen, Redwood City, http://www.qiagen.com/ingenuity), were used for the comprehensive verification and exploration of the metabolic network interaction (Fig. 1).
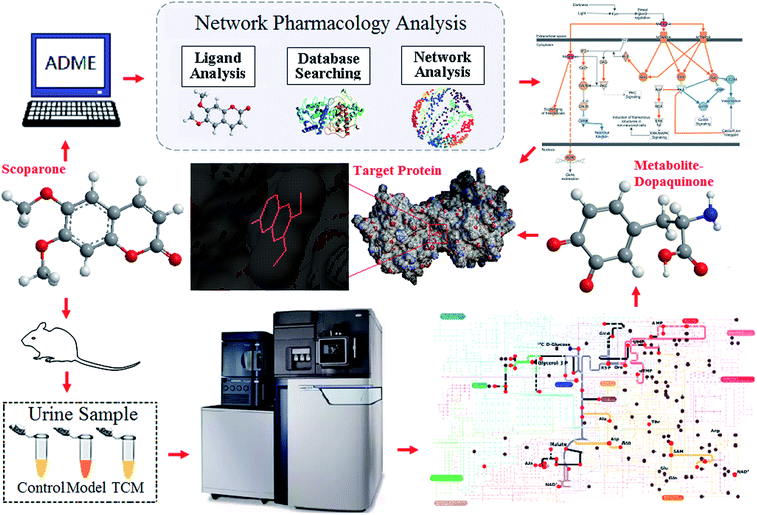 |
| Fig. 1 The comprehensive workflow of the investigation for target prediction and verification by the combination of metabolomics and network pharmacology technology. | |
Materials and methods
Chemicals and reagents
Acetonitrile and methanol were obtained from Merck (Darmstadt, Germany); water was obtained from a Milli-Q Ultra-pure water system (Millipore, Billerica, USA); formic acid was obtained from Honeywell Company (Morristown, New Jersey, USA); leucine enkephalin was purchased from Sigma-Aldrich (St. Louis, MO, USA); alcohol was purchased from Beijing Reagent Company (Beijing, China). Scoparone (purity 99%) was purchased from Sichuan Provincial Institute for Food and Drug Control (Sichuan, P. R. China). The ELISA kit for tyrosinase was purchased from Shanghai Caiyou Co., Ltd, China.
Chemical ingredient database building
Based on numerous studies reported earlier, the present exploration focused on the representative ingredient of Yinchenhao (Artemisia capillaris Thunb.), which is an authoritative herbal medicine used for all types of liver diseases. After oral administration, scoparone was detected as the major compound of Artemisia capillaris Thunb in plasma.14–24 Therefore, we concentrated on the pharmacological effects and target organs of the potential ingredient by network pharmacology.25–27 The chemical structure was obtained from Chemspider (http://www.chemspider.com/) and saved as a mol2 file for target prediction.
Target prediction and ingredient-target docking with network construction analysis
TCMID (http://www.megabionet.org/tcmid/) and TCMSP (http://lsp.nwsuaf.edu.cn/index.php) were employed for target prediction and we selected the consistent results by the distinct machine learning systems. The structure view of the focused proteins was downloaded from the PDB database (http://www.rcsb.org/pdb/home/home.do). Then, we conducted molecular docking with the visual tool.
Sample collection and preparation
Male Wistar rats were maintained within the Department of Center for Drug Safety Evaluation and Research of Heilongjiang University of Chinese Medicine. The study was approved by the Ethical Committee of Heilongjiang University of Chinese Medicine and was conducted according to the principles expressed in the Declaration of Helsinki. Before treatment, animals were acclimatized in metabolic cages for 1 week. They had free access to food and water and were housed under comfortable conditions of temperature (22 ± 1 °C), humidity (50 ± 5%) and a 12 h light/dark cycle. Then, 18 healthy rats were selected randomly and divided into 3 groups: a control group, an ALD group and a scoparone group. The ALD group and scoparone group rats were orally administrated a dose of 0.8 mL/100 g mixture with 6% alcohol liquor and a high-fat diet (basal feed
:
cholesterol
:
lard = 79%
:
1%
:
20%) for 12 consecutive weeks. The control group received orally administered water at the same dose once daily. In the 13th week, the scoparone group was treated with 7 mg kg−1 for seven days. The control and ALD groups received orally administered 0.9% saline at the same dose once daily. The experimental procedures were approved by the Animal Care and Ethics Committee at Heilongjiang University of Chinese Medicine and all experiments were performed in accordance with the declaration of Helsinki.
Urine samples were collected daily from the metabolic cages at ambient temperature throughout the entire procedure. They were centrifuged at 13
000 rpm at 4 °C for 15 min to remove any solid debris; the supernatants were stored frozen at −80 °C for UPLC-Q/TOF-G2Si-HDMS analysis. To optimize and ensure the stability and reproducibility of the UPLC-Q/TOF-G2Si-HDMS system, we employed a quality control (QC) specimen from each group, which contained information of all urine samples during the entire process.
Urine metabolic profiling analysis
Chromatography. Urine sample separation was performed on a Waters ACQUITY UPLC system with chromatographic analysis MassLynx™ software (V4.1SCN901, Waters Corporation, Milford, USA). The separation was carried out on an ACQUITY UPLC HSS T3 column, with a set column temperature of 45 °C (100 mm × 2.1 mm, 1.8 μm). The separation was carried out with the optimal mobile phase, which consisted of a linear gradient condition: (A) acetonitrile with 0.1% formic acid and (B) water with 0.1% formic acid, and the flow rate was set at 0.4 mL min−1. The gradient elution condition was: 1 to 10% A, 0–3min; 10 to 20% A, 3–5min; 20%–40% A, 5–8.5 min; 40 to 99% A, 8.5–9.5 min; maintaining 99% A at 9.5–11.5 min; linearly decreasing from 99% to 1% A, 11.5–12 min,; held at 1% A for 3 min for equilibration of the column. The sample injection volume was 3 μL.
Mass spectrometry. Advanced technologies SYNAPT™-G2Si-High-definition mass spectrometry (Waters SYNAPT™, Waters Corp, Manchester, England) was performed with an electrospray ion source to run all urine samples. The optimal parameters were as follows: capillary voltage was set at 3 kV and cone voltage at 25 V. The desolvation gas flow rate was maintained at 600 L h−1 and the cone gas flow was maintained at 60 L h−1. The desolvation temperature was set at 300 °C and source temperature at 110 °C. The scan time and inter-scan delay were set to 0.3 s and 0.1 s, respectively. Full scan mode was used for MS collection from m/z 50–1000. Leucine enkephalin was used as the vital reference compound with a concentration of 0.2 ng mL−1 under a flow rate of 100 μL min−1 ([M + H]+ = 556.2771, [M
−
H]− = 554.2615).
Data preprocessing and multivariate data analyses
The high resolution and high sensitivity performance of the latest UPLC-G2Si-HDMS technology provides much more signals for unknown icons. However, a series of intractability emerged with the tremendous dataset. Therefore, metabolomics brings in chemometrics technology to handle this problem. Before the multivariate data analysis, all the raw files were imported into Progenesis QI software (Nonlinear Dynamics, 2014, version 1.0) for data preprocessing, which contained noise reduction, normalization and peak picking. These necessary operations were performed with standardized procedures for data optimization. Then, we employed multivariate analysis, which included principal component analysis (PCA) and S-VIP plot of orthogonal partial least squares discriminant analysis (OPLS-DA) for the metabolomics study. High VIP value and furthest distance from the origin ions were selected as the valuable metabolites. In addition, we used SPSS software (Version 18.0 for Windows, IBM, Chicago, IL) for the Student's t test between control and ALD groups. The content of the ions between the two groups was compared to filter the difference. Combining VIP lists of OPLS-DA and the p value of the t test, a series of biomarkers were selected to be differences in metabolites.
Identification of biomarkers
The high throughput metabolomics study presents a comprehensive and non-targeted profiling investigation of low molecular mass metabolites. With the high performance of Ultra Performance Liquid Chromatography, complex biological samples get maximum separation within a few minutes. However, there still existed large sets of the same molecule with different ionization behaviors, including nine and ten types of adduct ion forms in negative and positive ion mode, respectively. Therefore, we used Progenesis QI software to remove adducts from the compound by robust deconvolution performance. Then, we employed tutorial data using the Human Metabolome Database (HMDB) for identification within a few minutes. As a result, we selected 24 ions as ALD biomarkers through the above procedure.
Results
Target prediction using network pharmacology
In order to predict the target proteins of scoparone for the treatment of liver disease, the present investigation employed two different machine learning systems for the screening. We selected the common prediction as the potential and vital target for further exploration with the TCMID and TCMSP databases. As a result, 331 targets were matched with target prediction, all of which possess a high degree of compatibility for each comprehensive database search. Then, we imported the network visualization tool for the intuitive analysis involved in all the targets (Fig. 2). In total, there were 331 nodes for the interaction between scoparone and targets. Among them, tyrosinase was found to be the common prediction and had the highest degree values from the scores listed in the prediction.
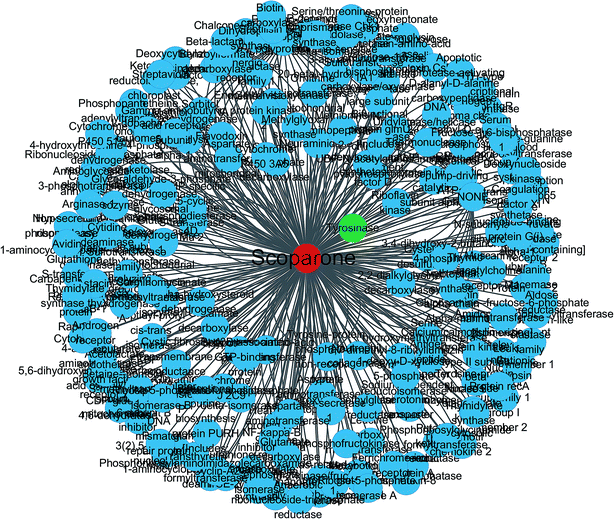 |
| Fig. 2 Different independent machine learning systems for target prediction of scoparone based on a network pharmacology approach. (●) The supplied ligand for the different target prediction systems. (●) The optimal receptor from different molecule-docking methods. (●) All the targets from the different machine learning systems. | |
Pattern recognition analysis of metabolome
Massive metabolic profiling data were collected from the high throughput technology of UPLC-ESI-G2Si-HDMS (Fig. 3A). Then, they were preprocessed by the advanced and authoritative platform of Progenesis QI, which possesses a robust full-scale deconvolution for removing adducts from the compounds; finally, a comprehensive multidimensional matrix was generated (Fig. 3B and C), including RT, m/z, and peak height intensity for pattern recognition analysis by EZinfo plug-ins. Combined with chemometrics and statistical analysis, a series of interesting ions were filtered by OPLS-DA and t test. From the location of PCA analysis, a clear separation was represented between the normal group and ALD group, which suggests that significant changes occurred on the metabolic level (Fig. 4A and B). The loading plot and S-VIP plot were employed for the visualization and screening of different metabolites (Fig. 5C–F). These small molecule metabolites were vital elements for the contribution of groups.
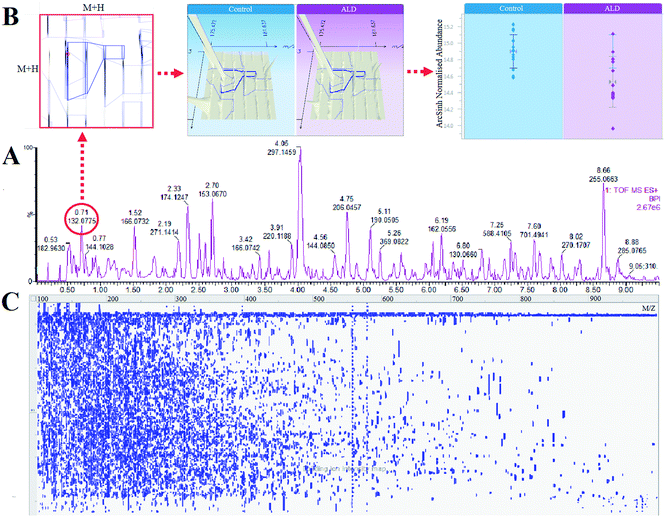 |
| Fig. 3 The robust data processing and visualization procedure by the Progenesis QI data-processing platform. The high throughput metabolic profiling analysis of the urine sample from the ALD group (A); the full-scale deconvolution for removing adducts from the compounds (B); the overall data program from the multidimensional metabolic matrix among the control, ALD and scoparone groups (C). | |
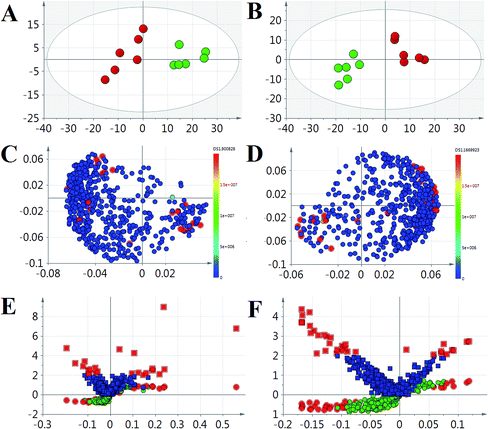 |
| Fig. 4 Pattern recognition analysis of the full-scan collection using UPLC-Q/TOF-G2Si-HDMS. Note: (●) control group. (●) ALD group. (●) QC. The score plot of PCA between control group and ALD group in positive mode (A) and negative mode (B); loading plot of the control group and ALD group in positive mode (C) and negative model (D); S-VIP plot for potential biomarker screening of ALD in positive mode (E) and negative mode (F). | |
Metabolic pathways and function analysis
The metabolomics investigation identified 24 ions as the biomarkers of ALD, which combined UPLC-ESI-G2Si-HDMS with Progenesis QI technology (ESI Table 1†). For the macroscopical metabolic network analysis, we employed the IPA data processing platform (Qiagen, Redwood City, http:\\www.qiagen.com/ingenuity) and MetaboAnalyst 3.0 (http://www.metaboanalyst.ca/MetaboAnalyst/faces/home.xhtml) for comprehensive analysis of the present metabolimics data, which primarily involved taurine and hypotaurine metabolism, catecholamine biosynthesis, cysteine and methionine metabolism, tryptophan metabolism, glutathione metabolism, vitamin B6 metabolism, pantothenate and coA biosynthesis, phenylalanine and tyrosine metabolism, folate and pterine biosynthesis, protein biosynthesis, glycine, serine and threonine metabolism, and bile acid biosynthesis (Fig. 5B). Besides, IPA analysis provided the canonical pathways, diseases and biofunctions, which integrated the expression as a heat map or histogram visually (Fig. 5A and C).
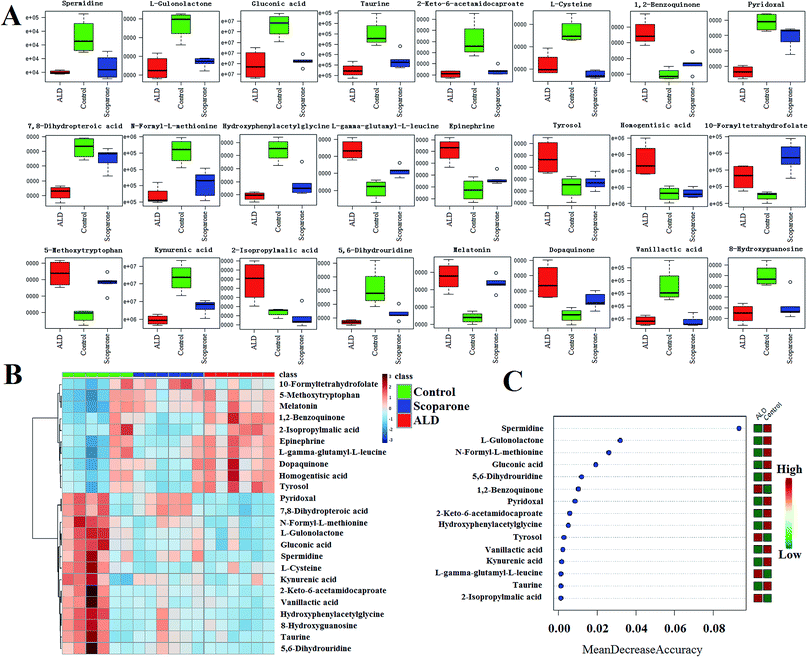 |
| Fig. 5 The heat map and histogram visualization for the treatment of scoparone on ALD. Bar chart of IPA canonical pathways visualization of the phenotypic characterization of ALD (A); metabolic enrichment analysis of the ALD group using the metaboAnalyst 3.0 online database (B). The comprehensive summary of the published canonical literature by IPA omics-platform (C). | |
Effects of scoparone against ALD
From the box plot and heat map analysis of the three groups, a remarkable inverse trend emerged from the ALD group to the control group after intervention by scoparone (Fig. 6A and B). Further, with the deep exploration of metabolic pathway analysis, we focused on the potential biomarkers associated with ALD, all of which possess a high VIP value among the groups (Fig. 6C). Content monitoring and upstream analysis support the unknown and vital information about ALD. Notably, scoparone possesses an evident hepatoprotective effect through adjusting the disturbed metabolism pathways such as taurine and hypotaurine metabolism, catecholamine biosynthesis, cysteine and methionine metabolism, tryptophan metabolism, glutathione metabolism, vitamin B6 metabolism, pantothenate and CoA biosynthesis, tyrosine metabolism, and phenylalanine and tyrosine metabolism.
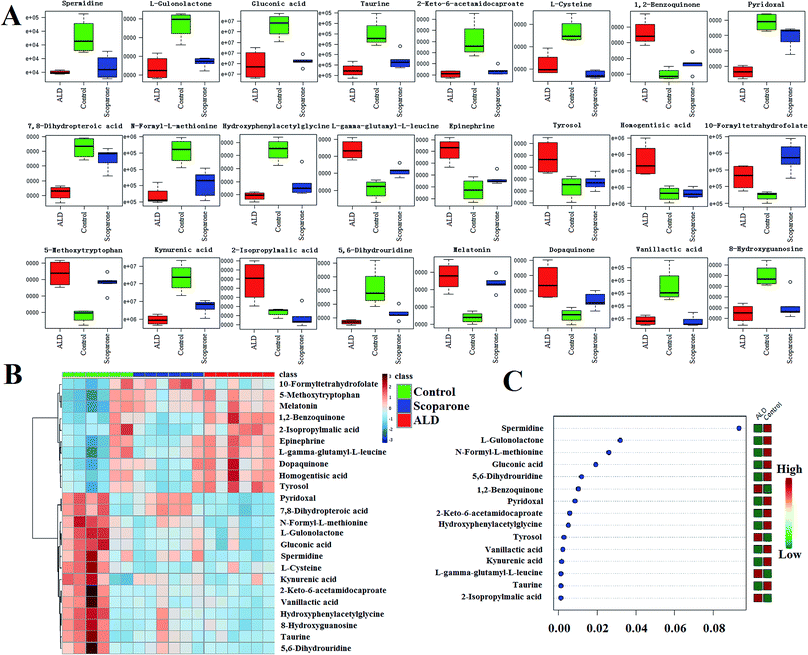 |
| Fig. 6 The content variation of the top 24 significant features from the metabolic markers among different groups. The box plot of the content of 24 ALD biomarkers among control, ALD and scoparone groups (A); the heat map analysis for the visual presentation of the obvious metabolic phenotype (B); the VIP contribution of the ALD biomarkers for the differential expression among groups (C). | |
Integration passway analysis
After the non-targeted metabolomic investigation, we identified 24 potential biomarkers associated with ALD. Then, an ingenuity pathway analysis omics-platform was used for the exploration of up and downstream pathways. Among them, we focused on a core biomarker, which was directly associated with the prediction of network pharmacology. Briefly, as the common and most matched target of different machine learning systems, tyrosinase possesses optimal molecular docking for scoparone. The former proved a potential relationship with liver disease and scoparone could have to restrain the activity of tyrosinase [EC1.14.18.1] to impede the anabolism from tyrosine to dopaquinone by metabolomic and network pharmacology analysis. Besides, the former report about “the treatment of scoparone on Yanghuang syndrome” has a similar conclusion, which focused on the core metabolite of phenylpyruvic acid.25 From “phenylalanine, tyrosine and tryptophan biosynthesis” pathway analysis, we found that the present investigation has two enzymes directly associated with phenylpyruvic acid and tyrosine. They both have vital functions in the diagnosis of liver disease, such as tyrosine aminotransferase and aspartate aminotransferase. Finally, we employed visualization tools and molecular docking software to simulate and represent the combination of receptor and ligand (Fig. 7).
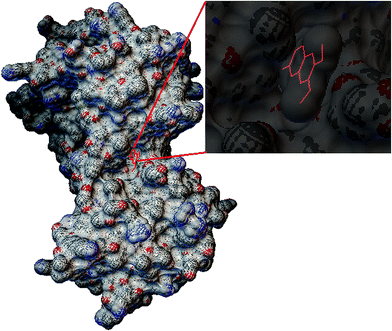 |
| Fig. 7 Tyrosinase as the potential anti-ALD target from the molecule docking of scoparone using visual tools. | |
The target verification using ELISA kit
For deep exploration and target verification of the prediction by network pharmacology and associated metabolites analysis, we employed an ELISA kit for the focused investigation of tyrosinase from serum. An evident callback trend was represented in the activity assay of tyrosinase (ESI Fig. 1†), which suggested that scoparone possesses a favorable inhibitory effect on the regulation of tyrosinase.
Discussion
Natural products are indispensable candidates in current drug discovery programs, which have inspired chemists and physicians for millennia. There are a number of successful approved drugs such as artemisinin, morphine and paclitaxel, which have widely influenced human health.28 However, despite these past triumphs, the progression from natural product research to academic lab chemosynthesis is inefficient due to blind screenings of large collections. Recent years have witnessed considerable advances in the exploration of natural compounds due to improvements in isolation, characterization and mechanism investigation. Besides, the reasonable and efficient approach is necessary for the evolvement of natural products. Scoparone, isolated from Yinchenhao (Artemisia capillaris Thunb.), possesses remarkable effects on anti-inflammatory analgesic, hypolipidemic, anticoagulant, and asthma. Based on the previous studies, we have focused on the target investigation from the established integration analysis combined with metabolomics and network pharmacology technology.15–27 A series of latest technologies, including a UPLC-Q/TOF-G2Si-HDMS instrument coupled with a robust Progenesis QI data-processing system and IPA omics-platform, were employed to handle the program. Then, we verified the target expression with an ELISA kit.
Dopaquinone, also known as o-dopaquinone, is a metabolite of L-DOPA and a precursor of melanin, which is also directly derived from tyrosine by tyrosinase [EC1.14.18.1]. From the comprehensive analysis of tyrosine metabolism (Fig. 8A), we focused on tyrosine aminotransferase [EC2.6.1.5] and aspartate transaminase [EC2.6.1.1]. Both were proven to be necessary factors in the metabolism of hepatocyte. In particular, aspartate transaminase has a special purpose for clinical examination. The abnormal tyrosine aminotransferase activity could also cause tyrosinemia, which is also a serious condition. With accurate detection, we found that the content of dopaquinone was significantly increased in the ALD group and this could reflect the metabolic disturbance of the above enzymes. Moreover, the rise of dopamine could also cause the synthesis of melanin (Fig. 8B), and then lead to liver dysfunction. After the treatment with scoparone, dopaquinone recovered to a normal level, demonstrating that scoparone has a specific function in the regulation of dopaquinone. Above all, as the common and optimal receptor of different machine learning systems by network pharmacology, tyrosinase possesses the optimal molecular docking for scoparone and this vital physiological process was verified by the high throughput metabolomics study.
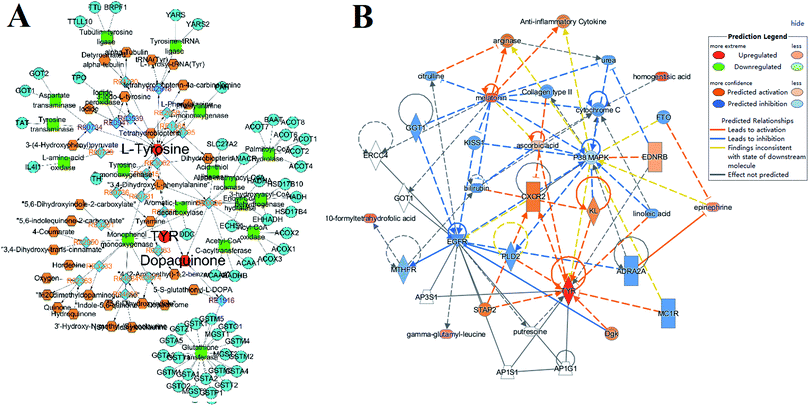 |
| Fig. 8 The comprehensive metabolic network from the target focused analysis of ALD biomarkers. Scoparone regulates the marker metabolite of putative effects and the relationship from L-tyrosine to dopaquinone by TYR (Tyrosinase) based on KEGG database analysis (A); the relationship between melanin and dopaquinone from the IPA omics-platform (B). | |
Taurine is the important constituent of bile, which participates in primary bile acid biosynthesis and is the precursor compound of taurocholate. The latter possesses a vital element for lipid absorption and the present processes also afford the main physiological performance of liver function. In this study, we found that the level of taurine was significantly lower compared with the normal group, which suggested that the metabolism of the above metabolites may be inordinate. Following treatment with scoparone, taurine recovered to a normal level, indicating that bile metabolism was partially relieved.
Citric acid is an important intermediate product of the TCA cycle (citrate cycle), which is directly associated with 20 key pathways, including alanine, aspartate and glutamate metabolism, glyoxylate and dicarboxylate metabolism, and carbon fixation pathways in prokaryotes. A metabolism disorder of TCA cycle would directly influence the conversion of basic nutrients. It is well known that ALD patients often suffer from energy dysmetabolism and have to be given aminosol for energy supplement. The present investigation found that the content of citric acid in ALD group is significantly lower compared to control group, which is favorably consistent with its clinical features and could provide a better understanding of ALD.
Conclusion
Natural products possess a dominant status in of drug discovery. It also supplies abundant candidate compounds for the strategies of new drug research and development. However, the targets and mechanisms of numerous effective natural products or compounds remain poorly understood. Herein, we presented a novel program for the discovery of potential drug targets. Based on the systematic literature study, scoparone was chosen as the representative ingredient of Yinchenhao (Artemisia capillaris Thunb.), which is a famous herbal medicine for the treatment of liver disease. The present investigation combined metabolomic and network pharmacology technology to focus on the target exploration for scoparone. After non-targeted metabolic pathway analysis and different molecule-docking systems, an optimal target of scoparone was identified based on theoretical data and metabolic datasets. Interestingly, the biggest highlight of the present investigation is that one of the core biomarkers of the metabolomic study, dopaquinone, was directly related with target prediction of network pharmacology with tyrosinase and finally resulted in a series of disturbances associated with liver injury. Moreover, the prediction also validated the target on a metabolic level. Besides, we prepared a series of robust platforms for metabolic pathway analysis, including Progenesis QI and IPA tools. Above all, the present study provides a novel strategy for the exploration of natural products and we believe that this optimal combination and multi-technological approach will greatly speed up discovery and characterization of potential drug targets.
Conflicts of interest
The authors declare no competing financial interests.
Acknowledgements
This work was supported by grants from the Key Program of Natural Science Foundation of State (Grant No. 81430093, 81373930, 81673586, 81302905), National Key Subject of Drug Innovation (Grant No. 2015ZX09101043-005, 2015ZX09101043-011), Special Fund of TCM State Administration for the Public Interest (2015468004), Specialized Research Fund for the Doctoral Program of Higher Education (20132327130001, 20122327120006), University Nursing Program for Young Scholars with Creative Talents in Heilongjiang Province (UNPYSCT-2015118).
References
- M. E. Welsch, S. A. Snyder and B. R. Stockwell, Privileged scaffolds for library design and drug discovery, Curr. Opin. Chem. Biol., 2010, 14(3), 347–361 CrossRef CAS PubMed.
- D. L. Ma, D. S. Chan and G. Wei, et al., Virtual screening and optimization of Type II inhibitors of JAK2 from a natural product library, Chem. Commun., 2014, 50(90), 13885–13888 RSC.
- X. Wang, A. Zhang and X. Zhou, et al., An integrated chinmedomics strategy for discovery of effective constituents from traditional herbal medicine, Sci. Rep., 2016, 6, 18997 CrossRef CAS PubMed.
- H. Dong, G. L. Yan and Y. Han, et al., UPLC-Q-TOF/MS-based metabolomic studies on the toxicity mechanisms of traditional Chinese medicine Chuanwu and the detoxification mechanisms of Gancao, Baishao, and Ganjiang, Chin. J. Nat. Med., 2015, 13(9), 687–698 CAS.
- X. Wang, A. Zhang and H. Sun, et al., Discovery and development of innovative drug from traditional medicine by integrated chinmedomics strategies in the post-genomic era, TrAC, Trends Anal. Chem., 2016, 76, 86–94 CrossRef CAS.
- A. Zhang, G. Yan and X. Zhou, et al., High resolution metabolomics technology reveals widespread pathway changes of alcoholic liver disease, Mol. BioSyst., 2015, 12(1), 262–273 RSC.
- H. Cao, A. Zhang and S. Hui, et al., Metabolomics-proteomics profiles delineate metabolic changes in kidney fibrosis disease, Proteomics, 2015, 15(21), 3699–3710 CrossRef CAS PubMed.
- A. Zhang, Q. Liu and H. Zhao, et al., Phenotypic characterization of nanshi oral liquid alters metabolic signatures during disease prevention, Sci. Rep., 2016, 6, 19333 CrossRef CAS PubMed.
- Y. Nan, X. Zhou and Q. Liu, et al., Serum metabolomics strategy for understanding pharmacological effects of ShenQi pill acting on kidney yang deficiency syndrome, J. Chromatogr. B: Anal. Technol. Biomed. Life Sci., 2015, 1026, 217–226 CrossRef PubMed.
- A. Zhang, G. Yan and H. Sun, et al., Deciphering the biological effects of acupuncture treatment modulating multiple metabolism pathways, Sci. Rep., 2015, 6 Search PubMed.
- A. L. Hopkins, Network pharmacology: the next paradigm in, drug discovery, Nat. Chem. Biol., 2008, 4(11), 682–690 CrossRef CAS PubMed.
- A. L. Hopkins, Network pharmacology, Nat. Biotechnol., 2007, 25(10), 1110–1111 CrossRef CAS PubMed.
- S. Calvert, R. Tacutu and S. Sharifi, et al., A network pharmacology approach reveals new candidate caloric restriction mimetics in C. elegans, Aging Cell, 2016, 68(2), 495–497 Search PubMed.
- X. Wang, H. Lv and H. Sun, et al., Development of a rapid and validated method for investigating the metabolism of scoparone in rat using ultra-performance liquid chromatography/electrospray ionization quadruple time-of-flight mass spectrometry, Rapid Commun. Mass Spectrom., 2007, 21(23), 3883–3890 CrossRef CAS PubMed.
- A. Zhang, H. Sun and S. Dou, et al., Metabolomics study on the hepatoprotective effect of scoparone using ultra-performance liquid chromatography/electrospray ionization quadruple time-of-flight mass spectrometry, Analyst, 2013, 138(1), 353–361 RSC.
- Q. Yin, H. Sun and A. Zhang, et al., Pharmacokinetics and tissue distribution study of scoparone in rats by ultraperformance liquid-chromatography with tandem high-definition mass spectrometry, Fitoterapia, 2012, 83(4), 795–800 CrossRef CAS PubMed.
- A. Zhang, H. Sun and G. Wu, et al., Proteomics Analysis of Hepatoprotective Effects for Scoparone Using MALDI-TOF/TOF Mass Spectrometry with Bioinformatics, OMICS: J. Integr. Biol., 2013, 17(4), 224–229 CrossRef CAS PubMed.
- A. Zhang, H. Sun and X. Wang, Urinary metabolic profiling of rat models revealed protective function of scoparone against alcohol induced hepatotoxicity, Sci. Rep., 2014, 4, 6768 CrossRef PubMed.
- X. Wang, W. Sun and H. Sun, et al., Analysis of the constituents in the rat plasma after oral administration of Yin Chen Hao Tang by UPLC/Q-TOF-MS/MS, J. Pharm. Biomed. Anal., 2008, 46(3), 477–490 CrossRef CAS PubMed.
- X. Wang, H. Lv and H. Sun, et al., Metabolic urinary profiling of alcohol hepatotoxicity and intervention effects of Yin Chen Hao Tang in rats using ultra-performance liquid chromatography/electrospray ionization quadruple time-of-flight
mass spectrometry, J. Pharm. Biomed. Anal., 2008, 48(4), 1161–1168 CrossRef CAS PubMed.
- X. Wang, H. Sun and A. Zhang, et al., Pharmacokinetics screening for multi-components absorbed in the rat plasma after oral administration traditional Chinese medicine formula Yin-Chen-Hao-Tang by ultra performance liquid chromatography-electrospray ionization/quadrupole-time-of-flight mass sp, Analyst, 2011, 136(23), 5068–5076 RSC.
- H. Lv, H. Sun and X. Wang, et al., Simultaneous determination by UPLC-ESI-MS of scoparone, capillarisin, rhein, and emodin in rat urine after oral administration of Yin Chen Hao Tang preparation, J. Sep. Sci., 2008, 31(4), 659–666 CrossRef PubMed.
- H. Sun, A. H. Zhang and D. X. Zou, et al., Metabolomics coupled with pattern recognition and pathway analysis on potential biomarkers in liver injury and hepatoprotective effects of yinchenhao, Appl. Biochem. Biotechnol., 2014, 173(4), 857–869 CrossRef CAS PubMed.
- H. Sun, A. Zhang and G. Yan, et al., Proteomics study on the hepatoprotective effects of traditional Chinese medicine formulae Yin-Chen-Hao-Tang by a combination of two-dimensional polyacrylamide gel electrophoresis and matrix-assisted laser desorption/ionization-time of flight mass spectrome, J. Pharm. Biomed. Anal., 2013, 75(5), 173–179 CrossRef CAS PubMed.
- H. Fang, A. Zhang and Y. Jingbo, et al., Insight into the metabolic mechanism of scoparone on biomarkers for inhibiting Yanghuang syndrome, Sci. Rep., 2016, 6, 37519 CrossRef CAS PubMed.
- X. Liu and X. Zhao, Scoparone attenuates hepatic stellate cell activation through inhibiting TGF-β/Smad signaling pathway, Biomed. Pharmacother., 2017, 93, 57 CrossRef CAS PubMed.
- A. Zhang, Q. Shi and S. Hui, et al., Scoparone affects lipid metabolism in primary hepatocytes using lipidomics, Sci. Rep., 2016, 6, 28031 CrossRef CAS PubMed.
- J. Clardy and C. Walsh, Lessons from natural molecules, Nature, 2005, 432(7019), 829–837 CrossRef PubMed.
Footnotes |
† Electronic supplementary information (ESI) available. See DOI: 10.1039/c7ra09522h |
‡ These authors contributed equally to this work. |
|
This journal is © The Royal Society of Chemistry 2017 |
Click here to see how this site uses Cookies. View our privacy policy here.