DOI:
10.1039/C7RA11956A
(Paper)
RSC Adv., 2018,
8, 1875-1883
Environmental factors, but not abundance and diversity of nitrifying microorganisms, explain sediment nitrification rates in Yangtze lakes†
Received
30th October 2017
, Accepted 28th December 2017
First published on 9th January 2018
Abstract
Sediment nitrification plays a vital role in nitrogen (N) biogeochemical cycling and ecological function of an aquatic ecosystem. The relative importance of environmental factors and nitrifying microbial communities in regulating sediment nitrification process has received less attention, especially in aquatic habitats where high N loads are frequently detected. Here, we report the potential nitrification rates of 35 sediment samples from 10 shallow lakes in the Yangtze River basin. The diversity and abundance of ammonia-oxidizing archaea (AOA) and bacteria (AOB) were quantified using archaeal and bacterial amoA genes. The results showed that there was no significant difference in sediment nitrification rates among sites of different trophic state. The nitrification rates were positively related to water chlorophyll-a, sediment N and carbon levels, but not significantly associated with diversity and abundance of ammonia-oxidizing microorganisms and submerged plants. Interestingly, the abundance and diversity of sediment AOB but not AOA communities were significantly influenced by trophic state. In addition, AOB communities were more sensitive to changes in local environments and catchment land uses than the AOA communities. Using path analysis, we found that 55–60% of the indirect effect of catchment land uses on nitrification rates was mediated via sediment N content. Our findings suggest that, although nitrification is a microbial process, variation in sediment nitrification rates in Yangtze lakes is mainly explained by abiotic factors but not by microbial abundance and diversity.
1. Introduction
Eutrophication has become one of the most serious environmental problems in aquatic ecosystems throughout the world, especially in China.1,2 Nitrogen (N) is essential for synthesizing nucleic acids and proteins and can be the limiting or co-limiting nutrient for phytoplankton production in lakes and other bodies of water.3,4 However, N pollution has increased considerably in recent decades due to increased fertilizer use, wastewater discharge, atmospheric deposition, and N fixation by agricultural crops in terrestrial ecosystems.5 High N loading in shallow lakes is generally linked to water eutrophication and several attendant ecological problems, including water quality deterioration, toxic algal blooms, and decline of freshwater biodiversity.6
The ability and mechanism of shallow lakes to cope with excess N input have attracted much attention in recent decades.7–9 The coupled nitrification–denitrification processes convert ammonium (NH4+) to nitrite (NO2−) and nitrate (NO3−) via nitrification, which can then be lost to the atmosphere as N2 gas through denitrification.10,11 In addition, the anammox process can use NH4+ as electron donor and NO2− as electron acceptor to accomplish N removal.12 The first key step of coupled nitrification–denitrification processes, the oxidation of NH4+ to NO2−, is catalyzed by ammonia monooxygenase enzyme. Archaeal and bacterial amoA genes have been frequently used as molecular markers to examine the community structure of ammonia-oxidizing archaea (AOA) and bacteria (AOB) in sediments and soils.13–16
Microbially mediated nitrification generally occurs in the presence of oxygen in lake sediments and varies greatly across spatial and temporal scales.17 Sediment nitrification rates are strongly influenced by local water quality and sediment properties, including NH4+ availability and organic matter.9,18,19 In addition, several studies have reported that the presence of vegetation can influence nitrification in both terrestrial and aquatic environments.20,21 Rooted submerged macrophytes can impact sediment nitrification by altering the organic carbon (C) inputs from root excretion, by competing with nitrification for NH4+ and by regulating the oxygen and redox status in the rhizosphere.22 Recently, many studies have examined the relationships between nitrifying microbial communities and sediment nitrification rates, but their results are not consistent.14,23–26 Hou et al. (2013) reported that sediment potential nitrification rates were positively related to AOB abundance in two large eutrophic lakes in China.25 Wankel et al. (2011) found no significant correlation between sediment nitrification rates and the abundance of AOA and AOB in an estuary, U.S.A.14
Catchment land uses can reflect the degree of anthropogenic activities, such as agricultural expansion and urban development.27 It has been shown that catchment land uses may significantly affect both local environmental factors and biological communities in aquatic habitats,28 which in turn can impact sediment nitrification processes. Arango and Tank29 have reported that sediment nitrification rates in Michigan headwater streams increase with increasing agriculture in catchment. Such a positive association is possible due to the fact that agricultural streams generally have high sediment C content, which is positively related to nitrification rates.29 Although several works have examined the correlations between land use and sediment nitrification,30 none have revealed the mechanisms by which catchment land uses indirectly influence the nitrification process of aquatic ecosystems.
In the Yangtze River basin there are 648 natural lakes with an area larger than 1 km2.31 Most of Yangtze lakes are shallow (mean depth < 5 m) and mostly located in the middle and lower Yangtze River basin. These shallow lakes play a vital role in providing fresh water for various purposes (human consumption, agricultural and industrial use) and developing aquaculture and tourism. However, due to rapid economic development and uncontrolled discharge of pollutants in catchments, most of the lakes in the Yangtze River basin have faced many environmental problems, including water eutrophication, harmful algal blooms, heavy metal pollution and degradation of submerged vegetation.31 It has been reported that the percent of eutrophic and hypereutrophic lakes has increased from approximately 41% in 1980s to nearly 85% in 2005 in China.2
In this study, a total of 35 sediment samples were collected from 10 shallow lakes in the Yangtze River basin. We measured the sediment potential nitrification rates and quantified the diversity and abundance of nitrifying microbes. The objectives of our study were (1) to compare sediment potential nitrification rates and nitrifying microorganisms among site types; (2) to examine the relationships between nitrification rates and abiotic factors (water quality and sediment properties) and biotic factors (nitrifying microorganisms and submerged vegetation); and (3) to explore the possible indirect effect of catchment land uses on sediment nitrification rates. Two hypotheses were tested: (1) both local abiotic and biotic factors would significantly impact sediment nitrification; and (2) catchment agriculture and urban would alter the sediment nitrification in lakes mainly through their effects on sediment properties.
2. Material and methods
2.1 Site description and field sampling
In July 2013, 10 lakes located in the middle and lower Yangtze River basin were chosen randomly (Fig. 1). All selected lakes are shallow and have a mean water depth less than 4 m. The smallest lake is Lake Cihu with an area of 8.15 km2 and the largest lake is Lake Taihu with an area of 2338 km2. Three to four sampling sites were selected along one transect at regular intervals from the littoral areas to lake centre in each lake. Littoral sites were in general located at water depth less than 2 m, approximately 100–200 m away from the lake shore.6 At each site, replicate surface sediments were randomly collected within an area about 20 m2 using a home-made grab sampler and the top 2 cm of sediment was mixed and homogenized to form a composite sample. If submerged vegetation was present in a sampling site, rhizosphere sediments were collected by shaking off sediments that were loosely adhering to the plant roots.
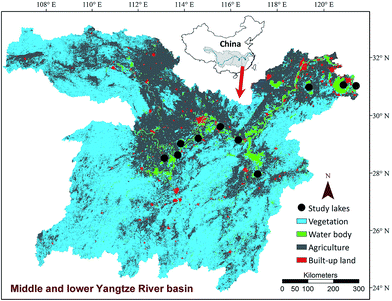 |
| Fig. 1 Location of 10 study lakes in the Yangtze River basin, China. | |
Approximately 500 g sediments from each site were put into plastic bags and stored in anoxic conditions at 5 °C in a portable refrigerator. Moreover, about 10 g sediments were collected in a centrifuge tube and immediately frozen in liquid N2. Before sediment sampling, a 500 mL bottom water sample was collected at about 1 m above the sediments at each sampling site. In addition, submerged plant communities were investigated using a plant grab (25 × 35 cm) with three replicates. Species richness, reflecting the α-diversity of submerged vegetation, was defined as the species number recorded in each sampling site. The fresh biomass of submerged vegetation per square meter was calculated after rinsing the plants with lake water.
2.2 Measurements of potential nitrification rates
The potential nitrification rates of lake sediments were measured in triplicate for each site by the shaken slurry method as modified by Hou et al.25 This method provided an upper-bound estimate of in situ nitrification under the conditions of aeration, unlimited NH4+ and phosphorus. Approximately 10 g of fresh sediments from each sampling site were weighed into 250 mL brown glass bottles and mixed with 100 mL of phosphate buffer (1 mM, pH 7.4) and 0.5 mL of (NH4)2SO4 (0.25 M). All bottles were then incubated on a horizontal shaker (200 rpm) at room temperature for 24 h. At five time intervals (1, 4, 10, 16 and 24 hours), 5 mL samples were collected from the slurry, centrifuged for 5 min at 2800 rpm and filtered through 0.45 μm membrane filters. Concentrations of NH4+ and NO3− in the filtrate were measured using an automatic nutrient analyser (EasyChem plus, Systea, Italy). The potential nitrification rates were calculated from the change in NO3− concentration during incubation using linear regression, and data were reported in ng NO3−–N g−1 h−1.
2.3 Measurements of nitrifying community diversity and abundance
DNA was extracted from 0.5 g sediment using the PowerSoil DNA Isolation Kit (MoBio Laboratories, CA, USA). The primer sets Arch-amoAF/Arch-amoAR and amoA-1F/amoA-2R were used for the amplification of the archaeal and bacterial amoA genes, respectively.32,33 Each reaction was performed in a 25 μL volume consisting of 1 μL of DNA template (10–100 ng μL−1), 0.5 μL of each primer (10 mM), 0.2 μL of rTaq polymerase (5 U μL−1), 0.5 μL of deoxynucleotide triphosphates (10 mM) and 2.5 μL of 10× buffer. The primers and thermal cycling profiles were adapted from Li et al.13 The amplified PCR products were gel purified (Axygen Biosciences, Union City, USA) and cloned into the pMD18-T vector (TaKaRa, DaLian, China), which was subsequently transferred into Trans-5α competent cells (Transgen Biotech, Beijing, China). Sixty randomly selected clones per sample for each gene were sequenced using an ABI-3730XL (Applied BioSystems, USA). The sequences with more than 95% similarity were classified into operational taxonomic units (OTUs) using the Mothur software by the furthest neighbor algorithm. The diversity indices (i.e., Chao1, Shannon and Simpson) were calculated for each sediment sample using the software Mothur. The archaeal and bacterial amoA sequences have been deposited in the GenBank with the following accession numbers: KY244149–KY244154 and KY244243–KY244299 for archaeal amoA and KY244155–KY244242 for bacterial amoA.
Quantitative PCR (qPCR) was used to determine the abundance (i.e., copy number) of archaeal and bacterial amoA genes in sediments. The qPCR was performed on an ABI Prism 7900 Sequence Detection System (Applied Biosystems, CA, USA) with the SYBR green Master Mix (Takara, Dalian, China). Primer sets of Arch-amoAF/Arch-amoAR and amoA-1F/amoA-2R were applied for the archaeal and bacterial amoA genes, respectively. The 25 μL qPCR mixture contained 10 μL of SYBR green qPCR Master Mix, 1 μL of each primer (10 μM) and 2 μL of DNA template. Standard curves were constructed with serial plasmid dilutions of a known amount of plasmid DNA involving the archaeal and bacterial amoA genes. The AOA and AOB abundance was estimated based on the constructed standard curve, and converted into copies per gram of sediment.
2.4 Measurements of local abiotic factors and catchment land use
Water depth and secchi depth (SD) were measured in field at each sampling site. Concentrations of total organic C (TOC) and total N (TN) in water samples were determined using an elemental analyzer (Vario TOC cube, Elementar, Germany). Total phosphorus (TP) concentration was analyzed using the colorimetric method on a spectrophotometer (PerkinElmer, Inc., Waltham, USA). Chlorophyll-a (Chl-a) content was determined by filtration through 0.45 μm membrane filters followed by extraction with 90% acetone and fluorometry. The trophic state index (TSI) was calculated based on the water SD, TP and Chl-a concentrations.34 TSI ranges <30, 30–50, 50–70 and >70 respectively indicated oligotrophic, mesotrophic, eutrophic and hypereutrophic state.2 Therefore, the 35 sampling sites could be classified into mesotrophic sites (N = 6), eutrophic sites (N = 21) and hypertrophic sites (N = 8). Sediment moisture was determined by oven drying 50 g sediments at 105 °C for 48 h, while sediment density was analyzed by weighing 50 cm3 sediments after drying overnight at 105 °C. Sediment total C (STC) and total N (STN) contents of air-dried sediments were measured using an elemental analyzer (Vario TOC cube, Elementar, Germany). Concentrations of sediment NH4+ and NO3− were measured by extracting 10 g of fresh sediments with 100 mL of 2 M KCl for 1 hour and using an automatic nutrient analyser (EasyChem plus, Systea, Italy).
To determine the land use characteristics of lake catchments, we first extracted the catchment boundaries of the 10 study lakes by a 1 km resolution digital elevation model (DEM). We obtained the land use/land cover map (GlobeLand30) of the Yangtze River basin from the National Geomatics Center of China.35 This map has a 30 m resolution and describes the spatial distribution of 10 land use types based on Landsat TM and HJ-1-CCD images in 2010. The original land use types were grouped into four main classes: (1) agriculture; (2) built-up land, including urban, rural settlements and industrial areas; (3) vegetation, including forest, grassland and shrubland; and (4) water body, including lakes and other aquatic habitats. The area and percentage of four land use classes in a lake catchment were calculated in software ArcGIS 10.0 using the overlay function on the reclassified land use map and the catchment boundaries.
2.5 Statistical analyses
We checked the data for normal distribution using the Shapiro–Wilk test before statistical analyses. Non-normally distributed data were square root (Sqrt) and natural log (Ln) transformed to reach a normal distribution when possible. One-way ANOVA followed by Tukey post-hoc tests was used to determine the differences in nitrification rates, nitrifying communities, water quality, sediment properties and submerged vegetation among sites of different trophic status. The relationships among nitrification rates, nitrifying communities, local factors and catchment land uses were assessed by the Pearson correlation and simple regression analyses. All above statistical analyses were conducted by the software PASW 19.0 (IBM SPSS Inc., Chicago, USA).
The path analysis was employed to determine the indirect effect of catchment land uses on sediment nitrification rates. Firstly, we constructed a conceptual model linking catchment land use and local factors to nitrification rates based on the existing literature and ecological principles (Fig. S1†). Secondly, according to the results of Pearson correlation and regression analyses, promising explanatory variables were chosen to include in path analysis. Finally, the path coefficients, R2, direct and indirect effects, and model fit parameters were determined using software AMOS 20.0 (Amos Development Corporation, Chicago, USA) with the maximum likelihood estimation method. The chi-square (χ2) test and comparative fit index (CFI) were used to evaluate the overall fit of the final path models. An insignificant χ2 statistic (P > 0.05) and CFI value > 0.9 indicated that the final path models provide a reasonable fit.6
3. Results
3.1 Water quality, sediment properties and submerged vegetation
The TOC content in water varied between 3.67 and 10.93 mg L−1, with a mean value of 6.64 ± 1.48 mg L−1 (Table S1†). The highest concentrations of water TN (2.13 mg L−1) and TP (0.51 mg L−1) were found in Lake Dianshanhu and Lake Donghu, respectively (Table S1†). The Chl-a concentration ranged from a minimum value of 1.38 to a maximum value of 78.77 mg m−3. The averaged STC and STN contents were 28.22 ± 22.65 and 2.18 ± 1.35 mg g−1, respectively. Submerged plants were detected in 20 sampling sites, with the greatest species richness of 7 and largest fresh biomass of 2640 g m−2 (Table S1†).
The water column TP, Chl-a concentrations and TSI in hypertrophic sites were significantly greater than those in mesotrophic and eutrophic lake sites, while the water SD was considerably lower in hypertrophic sites compared to other sites (Table 1). The maximum species richness and biomass of submerged plants were found in mesotrophic sites (Table 1). There are no submerged macrophytes in hypertrophic sites. Catchment agriculture showed a positive correlation with water TOC and TN concentrations (Table S2†). Catchment built-up land was positively related to water TN contents but negatively associated with STC and STN.
Table 1 Water quality, sediment properties and submerged vegetation characteristics (mean ± standard deviation) among site types in lakesa
|
Abbreviation |
Mesotrophic sites (N = 6) |
Eutrophic sites (N = 21) |
Hypertrophic sites (N = 8) |
Mean ± standard deviation followed by different lowercase letters indicates significant difference (P < 0.05) among site types. |
Water quality |
Depth (m) |
|
2.02 ± 0.87 |
2.26 ± 0.64 |
2.24 ± 0.77 |
Secchi depth (m) |
SD |
1.22 ± 0.19a |
0.95 ± 0.36a |
0.35 ± 0.20b |
Total organic carbon (mg L−1) |
TOC |
6.57 ± 1.32 |
6.33 ± 1.42 |
7.52 ± 1.55 |
Total nitrogen (mg L−1) |
TN |
0.93 ± 0.22 |
0.96 ± 0.45 |
1.13 ± 0.41 |
Total phosphorus (mg L−1) |
TP |
0.01 ± 0.00b |
0.06 ± 0.06b |
0.23 ± 0.15a |
Chlorophyll-a (mg m−3) |
Chl-a |
12.00 ± 9.21b |
16.15 ± 9.25b |
58.00 ± 15.78a |
Trophic state index |
TSI |
43.34 ± 3.98c |
58.67 ± 5.05b |
75.95 ± 3.58a |
![[thin space (1/6-em)]](https://www.rsc.org/images/entities/char_2009.gif) |
Sediment properties |
Moisture (%) |
|
69.69 ± 5.17a |
66.29 ± 7.84a |
56.69 ± 6.95b |
Density (g cm−3) |
|
1.12 ± 0.05b |
1.24 ± 0.14b |
1.40 ± 0.09a |
Sediment total carbon (mg g−1) |
STC |
30.40 ± 20.86 |
27.97 ± 26.31 |
27.25 ± 14.10 |
Sediment total nitrogen (mg g−1) |
STN |
2.36 ± 1.34 |
2.15 ± 1.56 |
2.02 ± 0.69 |
Sediment ammonia (mg kg−1) |
NH4+ |
1.03 ± 0.44 |
0.90 ± 0.38 |
0.76 ± 0.21 |
Sediment nitrate (mg kg−1) |
NO3− |
0.16 ± 0.12 |
0.59 ± 0.65 |
0.51 ± 0.55 |
![[thin space (1/6-em)]](https://www.rsc.org/images/entities/char_2009.gif) |
Vegetation characteristics |
Richness |
|
4.17 ± 1.17a |
2.43 ± 2.23a |
0.00 ± 0.00b |
Biomass (g m−2) |
|
1688 ± 761a |
508 ± 328b |
0.00 ± 0.00b |
3.2 Diversity and abundance of nitrifying community
The Shannon index of AOA ranged between 0.20 and 2.58, while the Shannon index of AOB varied from 0.41 to 2.66 (Table S3†). The abundance of AOA in lake sediments ranged from 0.02 × 104 to 333.34 × 104 gene copies per g sediment, while the abundance of the AOB varied between 0.17 × 104 and 10.37 × 104 gene copies per g sediment (Table S3†). The abundance and diversity of sediment AOB but not AOA were significantly different among sites of different trophic status (Table 2). Interestingly, the lowest AOB diversity and abundance were generally found in eutrophic sites.
Table 2 Diversity and abundance (mean ± standard deviation) of sediment nitrifying communities among site types in lakesa
|
Mesotrophic sites (N = 6) |
Eutrophic sites (N = 21) |
Hypertrophic sites (N = 8) |
Mean ± standard deviation followed by different lowercase letters indicates significant difference (P < 0.05) among site types. |
Chao1 index |
AOA |
11.40 ± 8.53 |
10.94 ± 9.29 |
5.63 ± 2.49 |
AOB |
27.33 ± 12.89a |
14.82 ± 9.72b |
27.38 ± 18.76a |
![[thin space (1/6-em)]](https://www.rsc.org/images/entities/char_2009.gif) |
Shannon index |
AOA |
1.14 ± 1.00 |
1.36 ± 0.53 |
0.87 ± 0.50 |
AOB |
2.02 ± 0.67a |
1.10 ± 0.50b |
1.98 ± 0.56a |
![[thin space (1/6-em)]](https://www.rsc.org/images/entities/char_2009.gif) |
Simpson index |
AOA |
0.53 ± 0.37 |
0.35 ± 0.19 |
0.56 ± 0.26 |
AOB |
0.25 ± 0.22b |
0.51 ± 0.19a |
0.21 ± 0.15b |
![[thin space (1/6-em)]](https://www.rsc.org/images/entities/char_2009.gif) |
Abundance |
AOA |
1.73 ± 1.33 |
1.33 ± 1.20 |
53.96 ± 115.68 |
AOB |
1.33 ± 1.10b |
0.56 ± 0.28b |
3.50 ± 3.30a |
![[thin space (1/6-em)]](https://www.rsc.org/images/entities/char_2009.gif) |
Abundance ratio |
AOA/AOB |
2.12 ± 1.75 |
2.81 ± 3.49 |
7.64 ± 11.07 |
Compared with AOA communities, AOB communities were more sensitive to changes in local environments (Table 3). Among the diversity indices and abundance of AOA, only abundance had a significant relationship with local water quality and sediment properties (Table 3). In contrast, the Shannon and Simpson indices of AOB showed a significant correlation with TOC and Chl-a concentration. In addition, the abundance of AOB was positively associated with Chl-a and TSI, but negatively correlated with and SD (Table 3).
Table 3 Pearson correlation coefficients between nitrifying communities and local factors and catchment land uses (N = 35)
|
AOA |
AOB |
AOA/AOB |
Chao1 |
Shannon |
Simpson |
Abundance |
Chao1 |
Shannon |
Simpson |
Abundance |
Abundance ratio |
P < 0.05. P < 0.01; see Table 1 for the abbreviations. |
Depth |
0.14 |
0.26 |
−0.28 |
−0.04 |
−0.08 |
−0.12 |
0.13 |
−0.02 |
−0.13 |
SD |
−0.02 |
−0.01 |
0.01 |
−0.34a |
−0.15 |
−0.27 |
0.25 |
−0.44b |
−0.31 |
TOC |
−0.34 |
−0.29 |
0.28 |
0.14 |
0.46b |
0.46b |
−0.49b |
0.29 |
−0.04 |
TN |
−0.07 |
−0.09 |
0.11 |
−0.08 |
0.17 |
0.17 |
−0.25 |
0.02 |
−0.10 |
TP |
−0.31 |
−0.27 |
0.24 |
0.07 |
−0.01 |
0.11 |
−0.20 |
0.19 |
0.00 |
Chl-a |
−0.08 |
−0.02 |
0.03 |
0.43a |
0.28 |
0.39a |
−0.37a |
0.60b |
0.35a |
TSI |
−0.14 |
−0.11 |
0.07 |
0.32 |
0.05 |
0.12 |
−0.16 |
0.39a |
0.27 |
Moisture |
0.03 |
0.22 |
−0.26 |
−0.19 |
−0.11 |
−0.18 |
0.16 |
−0.23 |
−0.11 |
Density |
−0.05 |
−0.07 |
0.08 |
0.11 |
−0.09 |
−0.02 |
0.02 |
0.15 |
0.09 |
STC |
−0.29 |
−0.29 |
0.28 |
−0.01 |
0.10 |
0.05 |
−0.08 |
0.09 |
−0.11 |
STN |
−0.12 |
−0.14 |
0.12 |
−0.07 |
0.00 |
−0.09 |
0.10 |
−0.08 |
−0.11 |
NH4+ |
−0.04 |
−0.07 |
0.05 |
−0.17 |
−0.05 |
−0.06 |
0.05 |
−0.20 |
−0.18 |
NO3− |
0.22 |
0.23 |
−0.23 |
0.04 |
−0.30 |
−0.24 |
0.20 |
0.02 |
0.18 |
Richness |
0.10 |
0.14 |
−0.13 |
−0.21 |
0.00 |
−0.03 |
0.01 |
−0.26 |
−0.12 |
Biomass |
0.09 |
−0.02 |
0.06 |
−0.17 |
0.19 |
0.14 |
−0.11 |
−0.20 |
−0.13 |
Catchment agriculture |
−0.23 |
−0.35 |
0.38a |
−0.18 |
0.39a |
0.44b |
−0.49b |
0.09 |
−0.33 |
Catchment built-up land |
0.01 |
−0.02 |
0.01 |
0.10 |
0.02 |
0.03 |
−0.01 |
−0.04 |
0.24 |
Catchment vegetation |
0.22 |
0.36a |
−0.36a |
−0.08 |
−0.33a |
−0.37a |
0.33a |
−0.17 |
−0.01 |
3.3 Sediment potential nitrification rates
Sediment potential nitrification rates ranged between 15.7 and 876.4 ng NO3−–N g−1 h−1, with a mean value of 192.7 ng NO3−–N g−1 h−1 (Table S3†). Sediment potential nitrification rates in hypertrophic sites (239.8 ± 75.9 ng NO3−–N g−1 h−1) were slightly but not significantly higher than those in eutrophic sites (185.4 ± 201.1 ng NO3−–N g−1 h−1) and mesotrophic sites (155.5 ± 215.4 ng NO3−–N g−1 h−1; Fig. 2). The potential nitrification rate showed positive relationships with Chl-a (R2 = 0.15, P = 0.026), STC (R2 = 0.17, P = 0.015) and STN (R2 = 0.33, P < 0.001; Fig. 3). However, the nitrification rate had no significant relationship with submerged vegetation characteristics. Furthermore, there was no significant correlation between potential nitrification rates and nitrifying community structure (Fig. S2†).
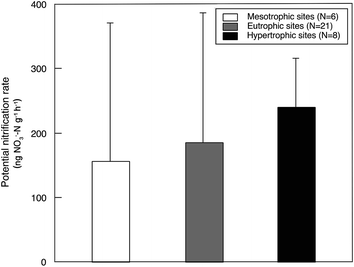 |
| Fig. 2 Sediment potential nitrification rate (mean ± standard deviation) among site types in lakes. | |
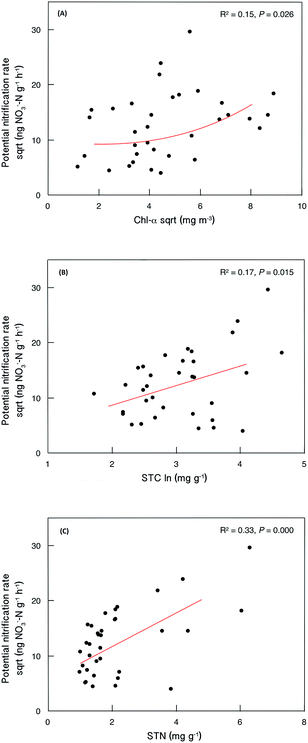 |
| Fig. 3 Relationships between sediment potential nitrification rate and selected local abiotic factors (N = 35). | |
The CFI values for the three path models in Fig. 4 were 0.970, 0.918 and 0.932, respectively, indicating that these path models were acceptable. As we hypothesized, catchment land uses indirectly affected the sediment potential nitrification in lakes mainly through their effects on sediment properties (Fig. 4). Over half of the indirect effect (58.3%) of catchment agriculture on potential nitrification was mediated via STN (Table 4). Similarly, approximately 55.0% and 41.8% of the indirect effects of catchment built-up land on potential nitrification were mediated via STN and STC, especially (Table 4).
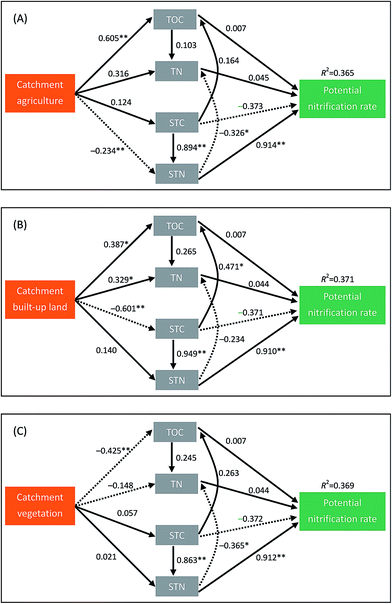 |
| Fig. 4 Structural equation models depicting the indirect effects of catchment agriculture (A), built-up land (B) and vegetation (C) on the sediment potential nitrification rate. Chl-a is not included in the final path models, because no evidence exists for a direct influence of Chl-a on sediment nitrification. Solid and dashed lines indicate positive and negative effects, respectively. Numbers adjacent to the lines are standardized path coefficients. *indicates P < 0.05, **indicates P < 0.01. The R2 values above the nitrification rate boxes represent the total variance explained by the models. | |
Table 4 Indirect effects of catchment land uses on the sediment potential nitrification mediated by local environmental factorsa
Environmental factors |
Catchment agriculture |
Catchment built-up land |
Catchment vegetation |
Indirect effects |
Contribution (%) |
Indirect effects |
Contribution (%) |
Indirect effects |
Contribution (%) |
See Table 1 for the abbreviations. Indirect effects refer to the mathematical product of all of the possible paths from catchment land uses to potential nitrification via environmental factors (see Fig. 4). Contribution (%) was calculated using the absolute values of indirect effects. |
TOC |
0.007 |
3.61 |
0.002 |
0.28 |
−0.007 |
6.67 |
TN |
0.019 |
9.92 |
0.020 |
2.84 |
−0.012 |
11.43 |
STC |
0.054 |
28.19 |
−0.295 |
41.84 |
0.023 |
21.90 |
STN |
−0.111 |
58.28 |
−0.388 |
55.04 |
0.063 |
60.00 |
Total indirect effects |
−0.031 |
100 |
−0.661 |
100 |
0.066 |
100 |
4. Discussion
4.1 Influences of multi-scale abiotic factors on sediment nitrification
Contrary to our first hypothesis, we found that the spatial variation of sediment nitrification rates in Yangtze lakes could be mainly due to changes in water quality and sediment properties rather than submerged vegetation and microbial abundances (Fig. 3). The effects of local environmental factors on sediment potential nitrification have been frequently investigated in previous studies.9,15,18,19 In the present study, we found that STN, STC and Chl-a were the main factors affecting the sediment nitrification rates in Yangtze lakes. Although nitrification rates have been positively correlated with NH4+ availability in a variety of habitats,18,36 such a positive relationship was not detected in our study. Instead, we found that STN was positively associated with sediment potential nitrification. This relationship has been frequently reported and may suggest that sediment nitrification is closely tied to rate of N mineralization from sediment organic matter.37,38 Many studies have examined the effect of sediment C availability on nitrification rate, but their results are not always consistent.11,18 Strauss et al. indicated that experimental addition of organic C might reduce the nitrification rates, because increased C availability would decrease the ability of nitrifying microorganisms to compete for NH4+.18 In contrast, consistent with the findings of Dodds and Jones,39 we observed that nitrification potential was positively correlated with the sediment C level. This positive relationship may be explained by the fact that high STC is generally associated with high STN, which is positively correlated with sediment potential nitrification. In the path analyses, STC had a negative path coefficient (−0.37) to potential nitrification rate (Fig. 4), which provided an evidence to support our explanation.
Although many studies have determined the nitrification rates in lake sediments worldwide,9,11,25 to our knowledge, no study has investigated how catchment land uses regulate sediment nitrification in lake ecosystems. As we hypothesized, the indirect effects of catchment land use on sediment potential nitrification in Yangtze lakes were more likely driven via changes in sediment properties than through changes in water quality. Arango and Tank found that mean nitrification rate was positively correlated with catchment agriculture in Michigan headwater streams.29 They speculated that this positive association was due to the fact that agricultural streams generally had high sediment C content, which was positively related to nitrification rates.29 In the present study, using the path analyses, we revealed that indirect influences of catchment land uses on sediment nitrification rates were mainly mediated by the STN (Table 4). In the Yangtze River basin, human activities especially urban areas have expanded dramatically in the past decades, accompanied by strong increases in nutrients (C, N and P) input to shallow lakes from catchments. Our findings highlight that anthropogenic activities in the catchments can have profound effects, not only on the local environments of lakes but also on the rates of N-cycling processes.
4.2 Nitrifying microorganisms and their role in regulating sediment nitrification
We found that the mean abundance of AOA (13.42 × 104 copies per g sediment) was approximately 10 times greater than that of AOB (1.36 × 104 copies per g sediment) in sediments of the Yangtze lakes. Similarly, an overwhelming dominance of AOA over AOB in nitrifying communities has been frequently observed in a wide range of aquatic and terrestrial ecosystems.23,40–42 In contrast, the Chao1 richness (9.65 ± 8.12) and Shannon diversity (1.20 ± 0.63) of AOA communities were both significantly lower than those of AOB communities (19.84 ± 13.83 and 2.73 ± 0.76) in lake sediments, which were consistent with some previous works.15,43 Compared with AOA communities, AOB communities in sediments of the Yangtze lakes were more sensitive to changes in local environmental factors especially trophic states (Tables 2 and 3). The AOB diversity and abundance were found to be significantly lower in eutrophic sites than in mesotrophic and hypertrophic sites. However, Herrmann et al.44 found an increasing trend in the OTUs number of AOA and AOB from oligotrophic to mesotrophic lakes in Denmark. Moreover, Hou et al.25 indicated that AOA abundance decreased sharply from mesotrophic sites to eutrophic sites, while AOB abundance was greater in eutrophic sites than in mesotrophic sites in Lake Taihu and Lake Chaohu, China. These inconsistent results may suggest that the effects of water eutrophication on sediment ammonia-oxidizing communities are complex and site specific.
A number of studies have investigated the contributions of AOA and AOB communities to nitrification processes in different habitats, but their results are inconsistent.14,25,45–47 The relationships between sediment nitrification rates and nitrifier diversity and abundance can be positive, negative, or nonexistent.26 Sims et al. revealed that potential nitrification rate was positively related to both bacterial and archaeal amoA gene abundances.45 In addition, Hou et al. indicated that sediment potential nitrification rate was positively related to the AOB diversity and abundance, but negatively correlated with AOA abundance.25 In the Yangtze lakes, sediment potential nitrification rates were not significantly related to community structure of both AOA and AOB (Fig. S2†). This result is not surprising and can be due to two main reasons. First, the presence of nitrifying genes in sediments does not necessarily mean that such functional genes will be expressed or that the protein products of genes will function equivalently.26 Second, functional gene pools only partly contribute to the activity of nitrifying enzymes in the sediments at a given time.15 Graham et al. proposed that studies whose primary purposes were to examine or predict the rates of N cycling processes might not need to determine functional gene abundance.48 Nonetheless, our results may suggest that local environmental factors rather than abundance and diversity of nitrifying microorganisms play a vital role in determining the sediment potential nitrification in Yangtze lakes.
5. Conclusions
In the Yangtze River basin, sediment potential nitrification rate in hypertrophic lake sites was slightly but not significantly higher than that in eutrophic and mesotrophic lake sites. However, abundance and diversity of AOB but not AOA were significantly influenced by lake trophic state. The potential nitrification rates were positively related to Chl-a, STC and STN contents, but not significantly correlated with nitrifying microorganisms and submerged plants. Path analyses demonstrated that the indirect effect of catchment land uses on lake sediment nitrification was largely mediated through STN. The diversity and abundance of AOA and AOB were mainly controlled by water quality and catchment land uses rather than sediment properties and submerged vegetation characteristics. Our results suggest that the responses of sediment nitrification potentials and nitrifying microorganisms to water eutrophication in Yangtze lakes are inconsistent. In addition, our findings highlight the importance of multi-scale abiotic factors, especially sediment properties, in regulating the nitrification process of lake sediments.
Conflicts of interest
The authors declare no conflict of interest.
Acknowledgements
Many thanks to Xiaoliang Jiang, Yujing Wu, Ziqian Xiong, Bei Lu, Guomin Li and Nanhua Liu for their help in the field and laboratory. This work has been funded by the National Natural Science Foundation of China (31570535 and 31400327).
References
- V. H. Smith, Environ. Sci. Pollut. Res., 2003, 10, 126–139 CrossRef CAS PubMed
. - W. Z. Liu, Q. F. Zhang and G. H. Liu, Hydrobiologia, 2010, 644, 289–299 CrossRef CAS
. - P. M. Vitousek, J. D. Aber, R. W. Howarth, G. E. Likens, P. A. Matson, D. W. Schindler, W. H. Schlesinger and D. G. Tilman, Ecol. Appl., 1997, 7, 737–750 Search PubMed
. - J. M. Abell, D. Özkundakci and D. P. Hamilton, Ecosystems, 2010, 13, 966–977 CrossRef CAS
. - N. Gruber and J. N. Galloway, Nature, 2008, 451, 293–296 CrossRef CAS PubMed
. - W. Z. Liu, L. Yao, Z. X. Wang, Z. Q. Xiong and G. H. Liu, Biogeosciences, 2015, 12, 6059–6070 Search PubMed
. - S. P. Seitzinger, Limnol. Oceanogr., 1988, 33, 702–724 CAS
. - R. M. Kreiling, W. B. Richardson, J. C. Cavanaugh and L. A. Bartsch, Biogeochemistry, 2011, 104, 309–324 CrossRef CAS
. - B. J. Bellinger, T. M. Jicha, L. P. Lehto, L. R. Seifert-Monson, D. W. Bolgrien, M. A. Starry, T. R. Angradi, M. S. Pearson, C. Elonen and B. H. Hill, J. Great Lakes Res., 2014, 40, 392–403 CrossRef CAS
. - S. Leininger, T. Urich, M. Schloter, L. Schwark, J. Qi, G. W. Nicol, J. I. Prosser, S. C. Schuster and C. Schleper, Nature, 2006, 442, 806–809 CrossRef CAS PubMed
. - D. A. Bruesewitz, J. L. Tank and S. K. Hamilton, J. Geophys. Res.: Biogeosci., 2012, 117, 1–12 Search PubMed
. - L. Hou, Y. Zheng, M. Liu, J. Gong, X. Zhang, G. Yin and L. You, J. Geophys. Res.: Atmos., 2013, 118, 1237–1246 CAS
. - M. Li, H. L. Cao, Y. G. Hong and J. D. Gu, Appl. Microbiol. Biotechnol., 2011, 89, 1243–1254 CrossRef CAS PubMed
. - S. D. Wankel, A. C. Mosier, C. M. Hansel, A. Paytan and C. A. Francis, Appl. Environ. Microbiol., 2011, 77, 269–280 CrossRef CAS PubMed
. - Y. Zheng, L. Hou, M. Liu, M. Lu, H. Zhao, G. Yin and J. Zhou, Appl. Microbiol. Biotechnol., 2013, 97, 8351–8363 CrossRef CAS PubMed
. - Y. Yang, N. Li, Q. Zhao, M. Yang, Z. Wu, S. Xie and Y. Liu, Environ. Sci. Pollut. Res., 2016, 23, 15358–15369 CrossRef CAS PubMed
. - P. M. Vitousek, S. Hättenschwiler, L. Olander and S. Allison, AMBIO A J. Hum. Environ., 2002, 31, 97–101 CrossRef
. - E. A. Strauss, N. L. Mitchell and G. A. Lamberti, Can. J. Fish. Aquat. Sci., 2002, 59, 554–563 CrossRef CAS
. - Z. Luo, Z. Qiu, Q. Wei, G. Du Laing, Y. Zhao and C. Yan, J. Environ. Earth Sci., 2014, 72, 2225–2237 CrossRef CAS
. - K. J. Forshay and S. I. Dodson, Hydrobiologia, 2011, 668, 21–34 CrossRef CAS
. - M. Tsiknia, V. A. Tzanakakis and N. V. Paranychianakis, Appl. Soil Ecol., 2013, 64, 104–111 CrossRef
. - E. Soana and M. Bartoli, Aquat. Ecol., 2014, 48, 11–21 CrossRef CAS
. - A. E. Bernhard, Z. C. Landry, A. Blevins, J. R. de la Torre, A. E. Giblin and D. A. Stahl, Appl. Environ. Microbiol., 2010, 76, 1285–1289 CrossRef CAS PubMed
. - H. Yao, Y. Gao, G. W. Nicol, C. D. Campbell, J. I. Prosser, L. Zhang, W. Han and B. K. Singh, Appl. Environ. Microbiol., 2011, 77, 4618–4625 CrossRef CAS PubMed
. - J. Hou, C. Song, X. Cao and Y. Zhou, Water Res., 2013, 47, 2285–2296 CrossRef CAS PubMed
. - J. D. Rocca, E. K. Hall, J. T. Lennon, S. E. Evans, M. P. Waldrop, J. B. Cotner, D. R. Nemergut, E. B. Graham and M. D. Wallenstein, ISME J., 2015, 9, 1693–1699 CrossRef PubMed
. - J. A. Foley, R. DeFries, G. P. Asner, C. Barford, G. Bonan, S. R. Carpenter, F. S. Chapin, M. T. Coe, G. C. Daily, H. K. Gibbs, J. H. Helkowski, T. Holloway, E. A. Howard, C. J. Kucharik, C. Monfreda, J. A. Patz, I. C. Prentice, N. Ramankutty and P. K. Snyder, Science, 2005, 309, 570–574 CrossRef CAS PubMed
. - L. L. Sass, M. A. Bozek, J. A. Hauxwell, K. Wagner and S. Knight, Aquat. Bot., 2010, 93, 1–8 CrossRef
. - C. P. Arango and J. L. Tank, J. North Am. Benthol. Soc., 2008, 27, 90–107 CrossRef
. - M. D. Harrison, P. M. Groffman, P. M. Mayer and S. S. Kaushal, Ecol. Eng., 2012, 38, 1–10 CrossRef
. - W. Liu, Q. Zhang and G. Liu, Clean. - Soil, Air, Water, 2011, 39, 35–42 CrossRef CAS
. - J. H. Rotthauwe, K. P. Witzel and W. Liesack, Appl. Environ. Microbiol., 1997, 63, 4704–4712 CAS
. - C. A. Francis, K. J. Roberts, J. M. Beman, A. E. Santoro and B. B. Oakley, Proc. Natl. Acad. Sci. U. S. A., 2005, 102, 14683–14688 CrossRef CAS PubMed
. - R. E. Carlson, Limnol. Oceanogr., 1977, 22, 361–369 CrossRef CAS
. - J. Chen, J. Chen, A. Liao, X. Cao, L. Chen, X. Chen, C. He, G. Han, S. Peng, M. Lu and W. Zhang, ISPRS J. Photogrammetry Remote Sens., 2015, 103, 7–27 CrossRef
. - E. A. Strauss, W. B. Richardson, L. A. Bartsch, J. C. Cavanaugh, D. A. Bruesewitz, H. Imker, J. A. Heinz and D. M. Soballe, J. North Am. Benthol. Soc., 2004, 23, 1–14 CrossRef
. - M. S. Booth, J. M. Stark and E. Rastetter, Ecol. Monogr., 2005, 75, 139–157 CrossRef
. - C. A. O'Sullivan, S. A. Wakelin, I. R. P. Fillery and M. M. Roper, Soil Res., 2013, 51, 240–252 CrossRef
. - W. K. Dodds and R. D. Jones, Microb. Ecol., 1987, 14, 91–100 CrossRef CAS PubMed
. - D. Bru, A. Ramette, N. Saby, S. Dequiedt, L. Ranjard, C. Jolivet, D. Arrouays and L. Philippot, ISME J., 2011, 5, 532–542 CrossRef CAS PubMed
. - J. P. Shen, Z. Xu and J. Z. He, J. Soils Sediments, 2014, 14, 1023–1029 CrossRef CAS
. - X. Sun, A. Wang, L. Yang, L. Guo, Q. Chen, Z. Hu, L. Jiang and L. Xiao, J. Limnol., 2014, 73, 312–324 Search PubMed
. - D. Zhao, J. Zeng, W. Wan, H. Liang, R. Huang and Q. L. Wu, Curr. Microbiol., 2013, 67, 327–332 CrossRef CAS PubMed
. - M. Herrmann, A. M. Saunders and A. Schramm, Appl. Environ. Microbiol., 2009, 75, 3127–3136 CrossRef CAS PubMed
. - A. Sims, J. Horton, S. Gajaraj, S. McIntosh, R. J. Miles, R. Mueller, R. Reed and Z. Hu, Water Res., 2012, 46, 4121–4129 CrossRef CAS PubMed
. - H. Urakawa, W. Martens-Habbena, C. Huguet, J. R. de la Torre, A. E. Ingalls, A. H. Devol and D. A. Stahl, Limnol. Oceanogr., 2014, 59, 1321–1335 CrossRef CAS
. - J. M. Smith, A. C. Mosier and C. A. Francis, Microb. Ecol., 2015, 69, 13–24 CrossRef CAS PubMed
. - E. B. Graham, W. R. Wieder, J. W. Leff, S. R. Weintraub, A. R. Townsend, C. C. Cleveland, L. Philippot and D. R. Nemergut, Soil Biol. Biochem., 2014, 68, 279–282 CrossRef CAS
.
Footnote |
† Electronic supplementary information (ESI) available. See DOI: 10.1039/c7ra11956a |
|
This journal is © The Royal Society of Chemistry 2018 |
Click here to see how this site uses Cookies. View our privacy policy here.