DOI:
10.1039/C8RA02805B
(Paper)
RSC Adv., 2018,
8, 20250-20258
Retracted Article: High performance liquid chromatography coupled with high resolution mass spectrometry-based characterization of lipidomic responses from rats with kidney injuries †
Received
1st April 2018
, Accepted 22nd May 2018
First published on 4th June 2018
Abstract
Metabolism of lipids is essential for the regulation of a variety of key cellular functions. Recent advances in high performance liquid chromatography coupled with high resolution mass spectrometry have expanded our knowledge of lipid metabolism in diseases. Currently, sepsis is one of the most important public health problems all over the world, which is a serious systemic inflammatory syndrome leading to infection by various agents or trauma and subsequently to a multiple organ dysfunction response. However, little is known about the lipids affected by sepsis and their roles in kidney injuries. In this study, we present targeted and non-targeted lipidomics strategies to discover the lipid metabolism variation in serum in rats with sepsis-induced kidney injuries. Liquid chromatography (LC) coupled with mass spectrometry (MS) and multivariate data analysis were used to obtain the global lipid metabolic profiles. In addition, biochemical parameters and histopathological examination results for the kidney were analyzed to support the pathological changes during sepsis-induced kidney injury. The identification of ten proposed lipids and five relevant pathways will promote a better understanding of lipid profile alterations in kidney injury. The results suggested that lipid metabolism in sepsis-induced kidney injury had changed significantly and contribute by offering potential targets for clinical diagnosis and therapy in the future, which would be worth further studies to broaden the applications of high performance liquid chromatography coupled with high resolution mass spectrometry in the study of lipid metabolism.
1. Introduction
Sepsis, one of the leading causes of death in critically ill patients, is a characteristic set of serious systemic inflammation reactions to infection or trauma. The incidence of sepsis is increasing which usually results in inflammation and immunological failure causing multiple organ injury such as lung, kidney, liver as well as central system.1,2 The kidney shows extreme metabolic activity, and becomes the pivotal potential target for inflammatory alteration and nearly 50% of all episodes of acute renal injury are ascribed to sepsis.3,4 During sepsis, acute kidney damage arises from a combination of the effects of local ischemia, inflammation, and additional factors.5 Some studies have reported that sepsis-related kidney injury is related to the pathogenesis of MODS attributed to poor prognosis. However, pathological mechanisms for sepsis-induced kidney injury are largely unknown leading to delayed early detection and treatment in the clinic. Therefore it is imperative to resolve these mechanisms to widen our knowledge of the pathophysiology and offer possible biomarker candidates to identify sepsis-related kidney injury early on in its development to optimize the therapeutic options.6,7
Lipids are an important part of biological membranes and are characterized by their molecular diversity and variety of biological functions including providing a suitable environment for protein interactions, storing energy, operating as messenger molecules, and others.8,9 Lipids are closely involved in apoptosis, signal transduction, disease infection, immune function, and fetal metabolic defects. In addition, the metabolism of lipid compounds is also strongly related to diabetes, liver cancer and breast cancer.10 In 2003, the concept of lipidomics was put forward by scholars, which is a comprehensive description of the species and biological function of lipid molecules.11,12 As the emerging and most important branch of metabolomics related to human health, lipidomic identification and quantification in living organisms have provided a new chance to better understand the functional role of various lipids in disease development. The combination of LC and MS characterized by a larger analytical range has been applied to directly identify and accurately quantify hundreds of lipids in a relatively short analysis time.13
The use of LC-MS based lipidomics to discover serum lipid metabolism variations from sepsis-induced kidney injury has been evaluated in several studies. In this article, a non-targeted lipidomics approach based on LC/MS is applied to investigate the lipid metabolism profiling in rats with sepsis-induced kidney injury caused by lipopolysaccharide (LPS), to assess the value of lipidomics for early detection and prediction of kidney function in sepsis patients, and provide a new platform for drug discovery and medical development.
2. Materials and methods
2.1 Chemicals and reagents
HPLC grade methanol, acetonitrile and formic acid were purchased from Thermo Fisher Scientific (Pittsburgh, PA, USA); distilled water was acquired from Wahaha purified water company (Hangzhou, China). Lipid standards were purchased from Sigma-Aldrich (St. Louis, MO, USA), for which the purity was more than 99%. LPS (E. coli, serotype O127:B8) was supplied by Biosharp Biotech Company (Hefei, China), and was diluted in 0.9% saline. Isofluorane and physiological saline solution were purchased from Kermel Chemical Reagent Co., Ltd. (Tianjin, China). The assay kits for serum creatinine (SCr), blood urea nitrogen (BUN) and cystatin C (Cys C) were purchased from Thermo Fisher Scientific (Waltham, MA, USA). Tumor necrosis factor-α (TNF-α), interleukin-1β (IL-1β), interleukin-6 (IL-6) and interleukin-10 (IL-10) were acquired from Sigma-Aldrich (Munich, Germany). Maleicdialdehyde (MDA) and superoxide dismutase (SOD) were purchased from Zhongsheng Beikong Biological Technology Limited Corporation (Beijing, China). The other reagents and chemicals were of analytical grade.
2.2 Animal treatments and sepsis model
Male Wistar rats weighing 200 ± 20 g were purchased from the Vital River Laboratory Animal Technology Co., Ltd. (Beijing, China). All animals were allowed 7 days of acclimatization. The room temperature was regulated at 22–26 °C. 12 h diurnal cycles and access to standard rodent pellets and water were provided in pathogen-free cages. After environmental adaptation, 16 male Wistar rats were randomized into control (8 rats) or model (8 rats) groups. The sepsis-induced kidney injury model was reproduced by injection with lipopolysaccharide (LPS) at 4 mg kg−1 diluted to 1 mL by isotonic NaCl solution into the tail vein.14,15 The control group of rats was treated with the same dose of saline in the same way. All animal procedures were performed in accordance with the Guidelines for Care and Use of Laboratory Animals of Heilongjiang University of Chinese Medicine and approved by the Animal Ethics Committee of Heilongjiang University of Chinese Medicine.
2.3 Biological sample collection and preparation
After 24 h of model replication, all the rats were injected with 10% chloral hydrate (5 mL kg−1) in their abdominal cavity for anesthesia. Blood samples of approximately 6 mL from the ventral vena cava were placed in Eppendorf tubes. After centrifugation at 3000 rpm and 4 °C for 15 min, serum samples were obtained and then divided into two groups: those for kit detection and those for lipid extraction for lipidomic analysis. The serum samples for biochemical investigation were stored at −80 °C until kit detection. 50 μL serum samples for lipidomics analysis in 2 mL microcentrifuge tubes had 350 μL methanol solution containing a lipid internal standard on ice added and then 1 mL methyl tert-butyl ether was added after vortexing for 30 s. 400 μL of supernatant lyophilizate was dissolved again in 250 μL water, then vortexed for 30 s and centrifuged at 12
000 rpm for 10 min at 4°C. 30 μL of dichloromethane–methanol (2
:
1, v/v) was added followed by 120 μL of acetonitrile–isopropanol–water (65
:
30
:
5, v/v) containing 5 mmol L−1 ammonium acetate and vortexed for 30 s. 30 μL of dichloromethane–methanol (2
:
1, v/v) was added followed by 120 μL of acetonitrile–isopropanol–water (65
:
30
:
5, v/v) containing 5 mmol L−1 ammonium acetate. The supernatant was transferred into a glass vial for injection after centrifugation. The right-hand side renal tissue was removed straight away after blood collection and soaked in 10% neutral solution of paraformaldehyde for paraffin section preparation and then hematoxylin and eosin (H&E) staining analysis was performed.
2.4 Biochemical and histopathological studies
The clinical biochemical parameters closely related to renal function (SCr, BUN and Cys C), inflammatory response (TNF-α, IL-1β, IL-4 and IL-6) and oxidation–reduction reactions (MDA and SOD) were measured using standard method requirements in kits. The kidney tissue fixed by 10% neutral solution of paraformaldehyde was first embedded using paraffin. Then, approximately 5 μm tissue sections of the kidney from the control and model groups of rats set apart from the paraffin blocks were observed using Image-Pro plus 5.0 software (Media Cybernetics, Bethesda, MD, USA) after staining with hematoxylin and eosin dye.
2.5 LC/MS analysis
A combination of LC and MS (Thermo Fisher Scientific) equipped with a pump, autosampler and an ACQUITY BEH C 18 chromatography column with a 0.17 μm stationary phase was applied to separate the serum samples with an injection volume of 3 μL. The column temperature was maintained at 30 °C with a flow rate of 0.3 mL min−1. A quality control (QC) sample is an important sample containing all the constituents of the samples to be analyzed, and is used to investigate the basic parameters of the column. After comprehensive optimization of the chromatography parameters, the mobile phase was acetonitrile containing 0.1% formic acid (A) and water with 0.1% formic acid (B) and the elution gradient program was performed as follows: 0–1 min, 1–20% A; 1–5 min, 20–80% A; 5–7 min, 80–100% A; 8–9 min, 100–1% A; 10–11 min, 1% A.
Mass spectra of the lipids were obtained by a mass spectrometer equipped with an electrospray ionization source including positive ionization mode (ESI+) and negative ionization mode (ESI−) operating at 100–2000 m/z in full scan mode. The final analysis conditions were chosen as follows: a capillary voltage of 3500 V in positive ion mode and 2000 V in negative ion mode, a cone voltage of 25 V, a source temperature of 110 °C, a desolvation temperature of 360 °C, a desolvation gas flow of 600 L h−1, and a cone gas flow of 40 L h−1 in both ion modes. 0.1 ng mL−1 leucine enkephalin was used as a reference to check the mass accuracy under a flow rate of 110 μL min−1. The scan time was set to 0.2 s and the inter-scan delay was set to 0.1 s. Then, MS/MS fragments were obtained for the detection and characterization of the lipids in the serum samples under 10–25 eV low energy, then 25–50 eV high-energy and then 10–25 eV low energy. Nitrogen was used for drying and collisions.
2.6 Data analysis
The Micromass MarkerLynx applications manager was utilized to convert all the raw LC-MS data for multivariate data analysis in view of the analytical performance including the mass accuracy, precision, and retention time reproducibility during detection. Firstly, the retention time and m/z data pair of each peak were monitored using the software for each sample. Then, the ion intensities of each detected peak were normalized to the sum of the peak intensities, which were subsequently enlarged 10
000 times. Finally, a table including the mass, retention time and all the relevant relative intensities of the detected peaks was obtained from the procedure. EZinfo 2.0 software (Waters Corporation, Manchester, UK) was used to perform multivariate analyses such as unsupervised principal component analysis (PCA), supervised partial latent structure-discriminant analysis (PLS-DA) and orthogonal projection to latent structure-discriminant analysis (OPLS-DA) with the normalized data preprocessed by MarkerLynx. In light of their contribution to the variation and correlation, the ions with a VIP value of more than 1 were screened from a VIP-plot formed by OPLS analysis, and were regarded as providing a significant contribution to the differences between the results from control and those from the model group. These potential ions were filtered through data analysis with a p value of less than 0.05. Structural characterization was carried out using Mass Fragment software with the MS/MS data and exact molecular mass. The selected lipid potential biomarkers were identified based on databases such as HMDB (http://www.hmdb.ca/), MetaboAnalyst (http://www.metaboanalyst.ca/), and LIPID MAPS (http://www.Lipidmaps.org/tools/index.html). Pathway analysis and the internal relationship of the lipid biomarkers in this study were carried out using the MetaboAnalyst 3.0 database. After using SPSS Statistics 19.0 (SPSS, Chicago, IL, USA) for statistical analysis of the different groups, all the quantitative data were expressed as the mean ± SD. The p values of less than 0.05 and the p values of less than 0.01, respectively, stand for significant and extremely meaningful in the comparison with the control and model group.
3. Results
3.1 Biochemical parameters and H&E staining analysis
After LPS injection, almost all the rats in the model group showed heightened abnormal symptoms such as slowness of movement, poor balance and drowsiness, as well as anorexia. The serum biochemical parameters SCr, BUN, Cys C, TNF-α, IL-1β, IL-6, IL-10, MDA and SOD are widely used in diagnostic tools in the clinic and their quantitative measurement was conducted to demonstrate the success of the sepsis-induced kidney injury animal models. In the control group, the serum concentration of the biochemical parameters was within the normal range when compared to the apparent disturbance of that for the model group. As shown in Fig. 1, the rats with sepsis-induced kidney injury had significantly higher SCr, BUN, Cys C, TNF-α, IL-1β, IL-6, IL-10 and MDA relative to the control rats. However, the reverse can also occur: the level of SOD was evidently decreased in the model group of rats. The biochemical results implied that the model group of rats was in a pathological state connected to renal damage, immune reactions, lipid peroxidation and others. Representative photomicrographs of H&E stained rat kidney tissues are shown in Fig. 2, which were collected for further verification of the disease severity. Kidney tissues from the rats with sepsis-induced kidney injury showed an abnormal structure, tubular dilatation, severe tubular atrophy, lipid droplets, swollen cells, severe inflammatory cell infiltration and kidney congestion. The histopathological examination indicated that sepsis-induced kidney injury had been successfully reproduced.
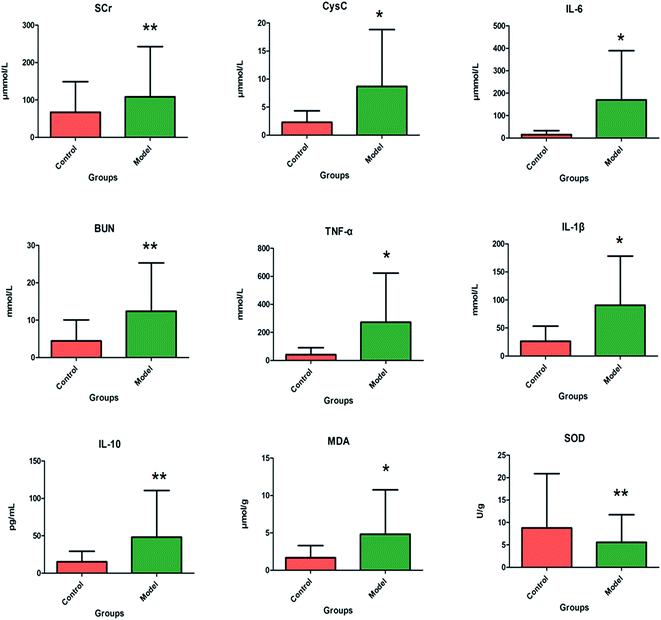 |
| Fig. 1 The pathological changes during sepsis-induced kidney injury were displayed in the clinical biochemistry analysis. In the model group, the serum levels of SCr (p < 0.01), BUN (p < 0.01), Cys C (p < 0.05), TNF-α (p < 0.05), IL-1β (p < 0.05), IL-6 (p < 0.05), IL-10 (p < 0.01) and MDA (p < 0.05) were significantly increased and the serum level of SOD was the exact reverse of this (p < 0.01). | |
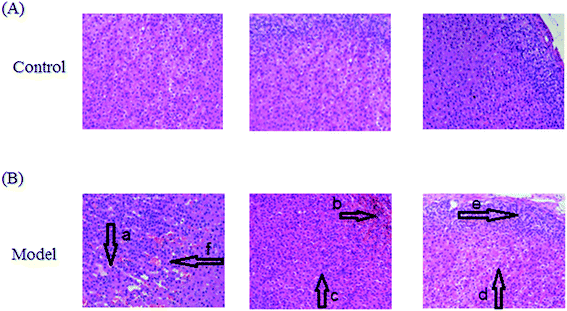 |
| Fig. 2 After LPS injection, abnormal structure, tubular dilatation (a), severe tubular atrophy (b), lipid droplets (c), swollen cells (d), severe inflammatory cell infiltration (e) and kidney congestion (f) could be seen in the kidney tissue of the model group of rats compared to that of the control group. The histopathological examination indicated that sepsis-induced kidney injury had been successfully reproduced. | |
3.2 Lipid metabolic profile and multivariate analysis
Lipid metabolic profiles of the serum samples from the control and model rats were acquired using LC-MS in ESI+ and ESI− modes combined with the above optimum analytical conditions and the Masslynx analysis platform. Owing to the spectra complexities under visual inspection, the LC/MS spectra present less distinct and obvious differences between the different groups. The clustering expression of all the datasets from each group was explored using multivariate data analysis for the purpose of further uncovering any variables contributing to the diversity between the control rats and the rats with sepsis-induced kidney injury. With Progenesis QI and EZinfo software, precise molecular masses and retention times of the lipids were provided for the multivariate data analysis. After monitoring and analyzing the original data from the LC-MS results using the single acquisition method in MarkerLynx, the worked data were imported into the EZinfo 2.0 software. A PCA score plot clearly shows the original data average weights, and then OPLS-DA score plots for further detection and a more sophisticated analysis of the clear differences by accurate calculation of the discrepancies between the control and model rats were applied to gain clear and comprehensive metabolic profiling of sepsis-induced kidney injury. With both positive ion mode (Fig. 3A and B) and negative ion mode (Fig. 4A and B), the OPLS-DA score plots and their 3D score plots of the control and model groups were well separated, which indicates that the rats show lipid metabolism alteration in the serum under LPS injection. The VIP score derived from the OPLS/DA data showing the contribution of the variables to clustering and discrimination was used to optimize the potential markers in positive and negative ion mode (Fig. 3C and 4C). The significant and differentially expressed lipids were selected in line with their having a threshold VIP value of more than 1 and a p value of less than 0.05 after statistical analysis.
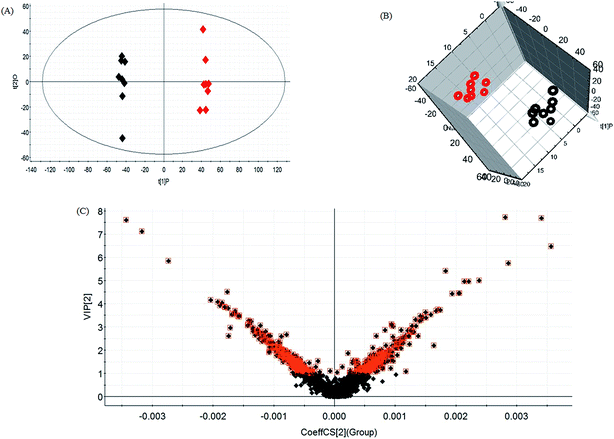 |
| Fig. 3 Multivariate analyses of the metabolites from sepsis-induced kidney injury in positive mode. OPLS-DA score plot (A), 3D OPLS-DA score plot (B) and VIP score plots of the control and model groups in positive ion mode from multivariate statistical analysis of the LC/MS data (C). In (A) and (B), the black icons represent the control group and the red icons represent the model group. | |
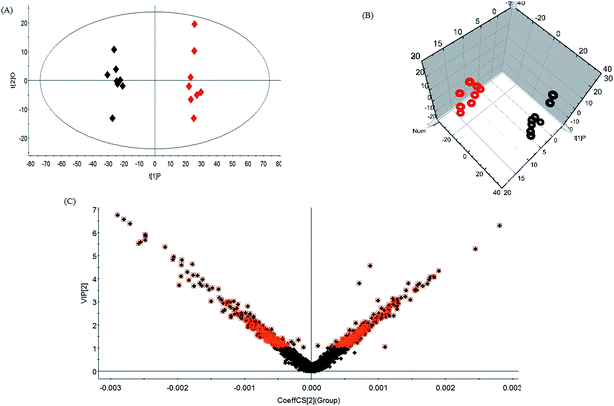 |
| Fig. 4 Multivariate analyses of the metabolites in sepsis-induced kidney injury in negative mode. OPLS-DA score plot (A), 3D OPLS-DA score plot (B) and VIP score plots of the control and model groups in negative ion mode from multivariate statistical analysis of the LC/MS data (C). In (A) and (B), the black icons represent the control group and the red icons represent the model group. | |
By obtaining MS/MS fragmentation data, the potential elemental composition of ions that were less than 5 ppm was screened. As shown in Table 1, 10 different lipids in serum samples from rats with sepsis-induced kidney injury were characterized after searching and comparing the LIPID MAPS and HMDB databases. The variation in each serum lipid in the rats with sepsis-induced kidney injury is shown in Fig. 5 compared to those of the control group rats. PCA score plots (Fig. 5A) and 3D PCA score plots (Fig. 5B) of the control and model groups were also well separated, which suggest that the rats show lipid metabolism variation in the serum under LPS injection. From the lipids hierarchical clustering heat map (Fig. 5C), PC(17:1(9Z)/0:0), PC(18:3(6Z,9Z,12Z)/20:2(11Z,14Z)), PG(18:1(9Z)/22:4(7Z,10Z,13Z,16Z)), PE(19:0/0:0) and SM(d18:1/22:0) showed an upward trend in the serum of the model rats, and PC(17:1/0:0), PG(18:2(9Z,12Z)/18:3(6Z,9Z,12Z)), LysoPE(18:0/0:0), PS(16:1(9Z)/20:3(8Z,11Z,14Z)) and Cer(d18:0/18:0) showed a downward trend. These variational lipids are associated with glycerophospholipid metabolism, alpha-linolenic acid metabolism, sphingolipid metabolism, linoleic acid metabolism and arachidonic acid metabolism (Fig. 6).
Table 1 The lipid biomarker candidates of differentially expressed metabolites in rats with sepsis-induced kidney injury using the lipidomics strategy
No. |
Rt (min) |
Ion form |
m/z |
Proposed compound |
Formula |
Trend in model |
1 |
7.91 |
M − H |
506.32 |
PC(17:1(9Z)/0:0) |
C25H50NO7P |
↑ |
2 |
7.27 |
M + H |
808.58 |
PC(18:3(6Z,9Z,12Z)/20:2(11Z,14Z)) |
C46H82NO8P |
↑ |
3 |
6.09 |
M + H |
507.33 |
PC(17:1/0:0) |
C25H50NO7P |
↓ |
4 |
5.37 |
M − H |
767.49 |
PG(18:2(9Z,12Z)/18:3(6Z,9Z,12Z)) |
C42H73O10P |
↓ |
5 |
1.76 |
M − H |
823.55 |
PG(18:1(9Z)/22:4(7Z,10Z,13Z,16Z)) |
C46H75O10P |
↑ |
6 |
5.74 |
M + H |
495.33![[thin space (1/6-em)]](https://www.rsc.org/images/entities/char_2009.gif) |
PE(19:0/0:0) |
C24H50NO7P |
↑ |
7 |
10.68 |
M − H |
480.31 |
LysoPE(18:0/0:0) |
C23H48NO7P |
↓ |
8 |
1.62 |
M + H |
784.51 |
PS(16:1(9Z)/20:3(8Z,11Z,14Z)) |
C42H74NO10P |
↓ |
9 |
5.10 |
M − H |
566.55 |
Cer(d18:0/18:0) |
C36H73NO3 |
↓ |
10 |
2.78 |
M − H |
785.65 |
SM(d18:1/22:0) |
C45H91N2O6P |
↑ |
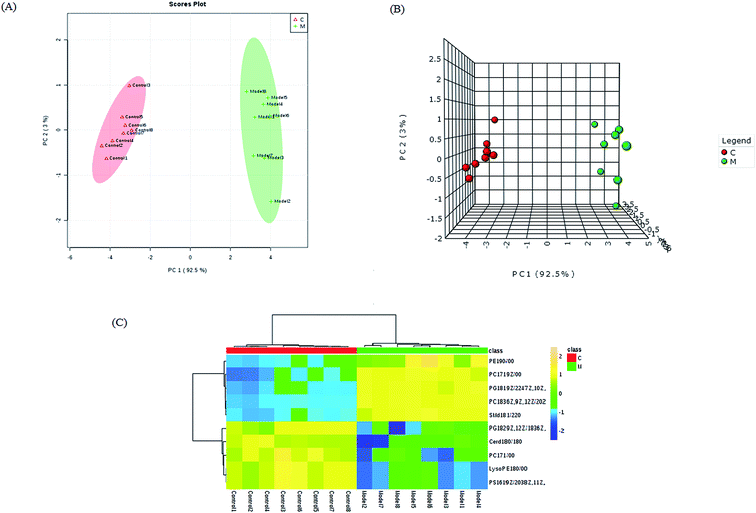 |
| Fig. 5 Significant changes in the metabolite biomarker candidates between the control and model groups. PCA score plot (A) and 3D PCA score plot (B); hierarchical clustering heat map of the 10 different lipids with the degree of change marked by the color, including up-regulation (yellow) and down-regulation (blue) (C). | |
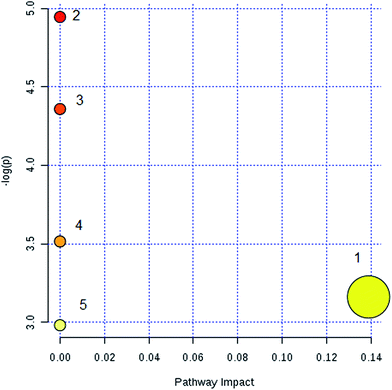 |
| Fig. 6 Pathway analysis of the sepsis-induced kidney injury using serum lipidomics. (1) Glycerophospholipid metabolism; (2) linoleic acid metabolism; (3) alpha-linolenic acid metabolism; (4), sphingolipid metabolism; (5) arachidonic acid metabolism. | |
4. Discussion
Many studies have found that the results of various treatments in different animal models are different, which indicates that the animal model is a key factor in experimental research. In order to establish a standardized and clinically relevant animal model of sepsis for exploring the pathophysiology of sepsis, controlled measurement is the primary concern. At present, animal models of sepsis include the following three types: LPS-induced inflammation models, cecal ligation and perforation (CLP) of polymicrobial sepsis and bacterial infusion or instillation models. The CLP model imitates a relatively natural state, which the disease has evolved into: a gradual process characterized by the high mortality of animals. The source of the infection can lead to an uncontrollable inflammatory cascade; due to continuity differences, its effects are different to human pathogenesis.16–18 In addition, due to different operating intensities it is difficult to standardize for comparison among diverse groups, and so this experiment did not use the CLP model. Although the bacterial infusion or instillation model is easy to operate, the model is greatly affected by bacterial species, inoculum size, susceptible sites and infection routes leading to poor stability. The bacterial infusion or instillation model is not the ideal animal model for this experiment.19 Animal models of sepsis induced by LPS injection are widely utilized in the study of sepsis. LPS injection induces a series of signal transduction mechanisms; a variety of inflammatory cells and effector cells cause the body to release inflammatory mediators such as TNF-α, IL-1β and other proinflammatory mediators, which simulate the early stage symptoms of systemic inflammatory response syndrome.20,21 The main role of injection injury is the release of a large number of inflammatory mediators resulting in sepsis-induced kidney injury characterized by a decreased glomerular filtration rate and an up-regulated trend of Cr and BUN in the serum, as well as increased renal neutrophil infiltration. The advantage of this model is its simple operation and reproducible, hemodynamic and metabolic changes similar to those in sepsis patients.22 In this experiment, rats were injected with a certain concentration of LPS into the tail vein to establish an animal model of sepsis that is conducive to exploring the pathogenesis of the disease and observing the bodily response, to provide a stable and reliable experimental model method for our sepsis-induced kidney injury research using lipidomics.
As a special vascular organ, the kidney is one of the most vulnerable organs to sepsis. Kidney damage causes disorders in the body’s environment, especially acid–base and electrolyte imbalance, which induces and aggravates damage to other organs. Research shows that renal ischemia may not be the main pathogenesis of sepsis, and possible pathogeneses of sepsis include inflammatory factor storms, coagulation disorders, intestinal flora and microcirculation and mitochondrial dysfunction. When sepsis occurs, a large number of proinflammatory cytokines (TNF-α, IL-1β, Il-6) are generated that greatly exceed the level of anti-inflammatory cytokines (IL-10) that cause a systemic inflammatory response eventually leading to corresponding organ and tissue failure.23 In this experiment, the level of TNF-α, IL-1β, IL-6 increased after the injection of LPS which implies that the early proinflammatory cytokines are out of control causing tissue damage. As a potent anti-inflammatory cytokine, IL-10 began to rise after a period of injection of LPS indicating that anti-inflammatory mediators in the body began to increase, which could help to inhibit the production of proinflammatory cytokines and play a protective role. Oxidative stress generated reactive oxygen species are the main cause of mitochondrial damage. Compared to that of the control group, the serum MDA content was increased and the SOD content was decreased indicating that the accumulation of free radicals with the endotoxin role in the body exceeds the antioxidant and antioxidant enzyme scavenging capacity, which results in the depletion of antioxidants and antioxidant enzyme damage.24 The serum level of SCr, BUN, Cys C increased which indicated that abnormal renal function had appeared in the model rats.25 However, hyperthyroidism and acromegaly can also cause SCr to rise. Therefore, the application of lipidomics to the study of the mechanism of serum lipid metabolism variation in vivo can better explain the dynamic changes in organisms. In this study, sepsis-induced kidney injury is closely linked with glycerophospholipid metabolism which takes part in the recognition and signal transduction of proteins in cell membranes. Abnormal metabolism of glycerol phospholipids means that kidneys can not work properly, which can also be seen from the pathological section.
There are still many challenges in the research of lipidomics.26 First of all, although there have been many studies on lipids, there are no uniform and reliable standards for the method for extracting total lipids or various lipids from biological samples. Using different lipid preparation methods, the results of different research groups differ greatly which will result in differences in interpretation. Secondly, the process of homogenization is always involved in the isolation of specific lipid regions in subcellular organelles and cells resulting in the aggregation of lipid molecules or the restructuring of membrane structures. Although LC-MS and shotgun lipidomics have been shown to be fast, high-throughput and high-precision techniques, the software for the qualitative and quantitative analysis of lipids is relatively weak, especially the automation analysis software and the lipid database.27–36 Thirdly, the bioinformatics technology systems for data integration, metabolic pathways and network mapping in lipid metabolism and network research are still in the preliminary stage and need to be further developed and perfected. Fourthly, although lipidomics has made great progress, people do not pay enough attention to lipidomics. In the future, we should work out novel methods of lipid preparation for different samples in lipidomics research. It is necessary to improve the rapid, high-throughput, high-precision identification and quantitative technology systems in lipid analysis. Lipid metabolism pathways and in-depth network research systems still remain to be established and improved.37
5. Conclusion
In this study, the serum lipid profiles of rats with sepsis-induced kidney injury were determined using a nontargeted and comprehensive lipidomics strategy based on an LC-MS analysis platform coupled with multivariate data analysis and multi-database searching. In total, 10 sepsis-induced kidney injury biomarkers were detected, which primarily related to glycerophospholipid metabolism, glycerophospholipid metabolism, alpha-linolenic acid metabolism, sphingolipid metabolism and arachidonic acid metabolism. The results suggest that serum lipid profiles of rats with sepsis-induced kidney injury have great variation and the potential lipids have great diagnostic value for clinical applications.
Conflicts of interest
There are no conflicts to declare.
References
- Q. Liang, H. Liu, H. Xing and Y. Jiang, et al., UPLC-QTOF/MS based metabolomics reveals metabolic alterations associated with severe sepsis, RSC Adv., 2016, 6, 43293–43298 RSC.
- Q. Liang, H. Liu and T. Zhang, et al., High-throughput metabolic profiling for discovering metabolic biomarkers of sepsis-induced acute lung injury, RSC Adv., 2016, 6, 11008–11013 RSC.
- Q. Liang, H. Liu and T. Zhang, et al., Potential urine biomarkers from a high throughput metabolomics study of severe sepsis in a large Asian cohort, RSC Adv., 2015, 5, 102204–102209 RSC.
- Q. Liang, H. Liu and L. Xie, et al., High-throughput metabolomics enables biomarker discovery in prostate cancer, RSC Adv., 2017, 7, 2587–2593 RSC.
- Q. Liang, Y. Zhu and H. Liu, et al., High-throughput lipidomics enables lipids discovery in huaxian capsule impacting metabolism of sepsis, RSC Advances, RSC Adv., 2017, 7, 44990–44996 RSC.
- J. Cohen, J.-L. Vincent and N. K. J. Adhikari, et al., Sepsis: a road map for future research, Lancet. Infect. Dis., 2015, 15(5), 581–614 CrossRef PubMed.
- S. M. Opal, R. P. Dellinger and J.-L. Vincent, et al., The next generation of sepsis clinical trial designs: what is next after the demise of recombinant human activated protein C, Crit. Care Med., 2014, 42(7), 1714–1721 CrossRef PubMed.
- Q. Liang, T. Zhang and Y. Jiang, et al., Untargeted lipidomics study of coronary artery disease by FUPLC-Q-TOF-MS, Anal. Methods, 2016, 8, 1229–1334 RSC.
- S. B. Nayak, S. B. Mohammed and A. S. Nayak, Controlling Lipids AIDS in the Prevention of Type 2 Diabetes, Hypertension, and Cardiovascular Diseases, Int. J. Prev. Med., 2017, 8, 39 CrossRef PubMed.
- Z. Wu, J. Cheol Shon and K.-H. Liu, Mass Spectrometry-based Lipidomics and Its Application to Biomedical Research, Journal of Lifestyle Medicine, 2014, 4(1), 17–33 CrossRef PubMed.
- M. Lagarde, A. Géloën and M. Record, et al., Lipidomics is emerging, Acta Biochim. Biophys. Sin., 2003, 1634(3), 61 CrossRef.
- S. H. Lee, M. V. Williams and R. N. DuBois, et al., Targeted lipidomics using electron capture atmospheric pressure chemical ionization mass spectrometry, Rapid Commun. Mass Spectrom., 2003, 17(19), 2168–2176 CrossRef PubMed.
- J. Zhang, X. Zhang and L. Wang, et al., High Performance Liquid Chromatography-Mass Spectrometry (LC-MS) Based Quantitative Lipidomics Study of Ganglioside-NANA-3 Plasma to Establish Its Association with Parkinson’s Disease Patients, Med. Sci. Monit., 2017, 23, 5345–5353 CrossRef PubMed.
- D. Xu, M. Chen and X. Ren, et al., Leonurine ameliorates LPS-induced acute kidney injury via suppressing ROS-mediated NF-κB signaling pathway, Fitoterapia, 2014, 97, 148–155 CrossRef PubMed.
- J. W. Lee, J. H. Kwon and M. S. Lim, et al., 3,4,5-Trihydroxycinnamic acid increases heme-oxygenase-1 (HO-1) and decreases macrophage infiltration in LPS-induced septic kidney, Mol. Cell. Biochem., 2014, 397(1–2), 109–116 CrossRef PubMed.
- H. Freise, U. B. Bruckner and H. U. Spiegel, Animal models of sepsis, J. Invest. Surg, 2001, 14, 195–212 CrossRef PubMed.
- M. C. Gruda, K. G. Ruggeberg and P. O’Sullivan, et al., Broad adsorption of sepsis-related PAMP and DAMP molecules, mycotoxins, and cytokines from whole blood using CytoSorb® sorbent porous polymer beads, PLoS ONE, 2018, 13(1), 1–12 Search PubMed.
- Y. Xu, Y. Jiang and W. Gai, et al., Protective role of tenuigenin on sepsis-induced acute kidney injury in mice, Exp. Ther. Med., 2017, 14(5), 5051–5056 Search PubMed.
- J. T. Muenzer, C. G. Davis and B. S. Dunne, et al., Pneumonia after cecal ligation and puncture: a clinically relevant “two-hit” model of sepsis, Shock, 2006, 26(6), 565–570 CrossRef PubMed.
- J. Wang, H. Wang and R. Zhu, et al., Anti-inflammatory activity of curcumin-loaded solid lipid nanoparticles in IL-1β transgenic mice subjected
to the lipopolysaccharide-induced sepsis, Biomaterials, 2015, 53475–53483 Search PubMed.
- A. Marton, C. Kolozsi and E. Kusz, et al., Propylene-glycol aggravates LPS-induced sepsis through production of TNF-α and IL-6, Iran. J. Immunol., 2014, 11(2), 113–122 Search PubMed.
- M. M. Tiwari, R. W. Brock and J. K. Megyesi, et al., Disruption of renal peritubular blood flow in lipopolysaccharide-induced renal failure: role of nitric oxide and caspases, Am. J. Physiol., 2005, 289, 1324–1332 CrossRef PubMed.
- X. Chen, Y. Wang and H. Luo, et al., Ulinastatin reduces urinary sepsis-related inflammation by upregulating IL-10 and downregulating TNF-α levels, Mol. Med. Rep., 2013, 8(1), 29–34 CrossRef PubMed.
- H. Ma, J. Kou and D. Zhu, et al., Liu-Shen-Wan, a traditional Chinese medicine, improves survival in sepsis induced by cecal ligation and puncture via reducing TNF-alpha levels, MDA content and enhancing macrophage phagocytosis, Int. Immunopharmacol., 2006, 6(8), 1355–1362 CrossRef PubMed.
- T. Johannes, E. G. Mik and C. Ince, Nonresuscitated endotoxemia induces microcirculatory hypoxic areas in the renal cortex in the rat, Shock, 2009, 31, 97–103 CrossRef PubMed.
- T. A. Lydic and Y. H. Goo, Lipidomics unveils the complexity of the lipidome in metabolic diseases, Clin. Transl. Med., 2018, 7(1), 4 CrossRef PubMed.
- L. F. Eggers and D. Schwudke, Shotgun Lipidomics Approach for Clinical Samples, Methods Mol. Biol., 2018, 1730, 163–174 Search PubMed.
- Y. Zhang, P. Liu and Y. Li, et al., Exploration of metabolite signatures using high-throughput mass spectrometry coupled with multivariate data analysis, RSC Adv., 2017, 7(11), 6780–6787 RSC.
- Q. Liang, H. Liu and Y. Jiang, et al., Discovering lipid phenotypic changes of sepsis-induced lung injury using high-throughput lipidomic analysis, RSC Adv., 2016, 6, 38233–38237 RSC.
- X. Wang, J. Li and A. H. Zhang, Urine metabolic phenotypes analysis of extrahepatic cholangiocarcinoma disease using ultra-high performance liquid chromatography-mass spectrometry, RSC Adv., 2016, 6(67), 63049–63057 RSC.
- Q. Liang, C. Wang and B. Li, et al., Lipidomics Analysis Based on Liquid Chromatography Mass Spectrometry for Hepatocellular Carcinoma and Intrahepatic Cholangiocarcinoma, RSC Adv., 2015, 5, 63711–63718 RSC.
- Y. Li, S. Qiu and A. Zhang, High-throughput metabolomics to identify metabolites serve as diagnostic biomarkers of prostate cancer, Anal. Methods, 2016, 8, 3284–3290 RSC.
- Q. Liang, H. Liu and T. Zhang, et al., Discovery of serum metabolites for diagnosis of mild cognitive impairment to Alzheimer’s disease progression using an optimized metabolomics method, RSC Adv., 2016, 6, 3586–3591 RSC.
- Q. Liang, T. Zhang and Y. Jiang, et al., Metabolomics-Based Screening of Salivary Biomarkers for Early Diagnosis of Alzheimer’s Disease, RSC Adv., 2015, 5, 96074–96079 RSC.
- Q. Liang, H. Liu and X. Li, et al., High-throughput metabolomics analysis discovers salivary biomarkers for predicting mild cognitive impairment and Alzheimer’s disease, RSC Adv., 2016, 6, 75499–75504 RSC.
- Q. Liang, H. Liu and H. Xing, et al., Urinary UPLC-MS metabolomics dissecting the underlying mechanisms of huaxian capsule protects against sepsis, RSC Adv., 2016, 6, 40436–40441 RSC.
- T. Hu and J. L. Zhang, Mass-spectrometry-based lipidomics, J. Sep. Sci., 2018, 41(1), 351–372 CrossRef PubMed.
Footnote |
† Electronic supplementary information (ESI) available. See DOI: 10.1039/c8ra02805b |
|
This journal is © The Royal Society of Chemistry 2018 |
Click here to see how this site uses Cookies. View our privacy policy here.