DOI:
10.1039/C8RA04213F
(Paper)
RSC Adv., 2018,
8, 31396-31405
Metabolomic profiling of matured coconut water during post-harvest storage revealed discrimination and distinct changes in metabolites
Received
17th May 2018
, Accepted 28th August 2018
First published on 6th September 2018
Abstract
The metabolites of coconut water stored at room temperature were analyzed using UPLC-MS/MS and multivariate statistical analysis to identify the differential biomarkers and metabolic pathways during post-harvest storage. Principal component analysis (PCA), partial least squares-discriminate analysis (PLS-DA), and orthogonal projections to latent structures discriminant analysis (OPLS-DA) were employed to analyze the UPLC-MS/MS data set of 34 matured coconut water samples collected after 0, 1, 2, 3, 4, and 5 months of storage (MOS); moreover, the p-value and fold change were chosen to identify the differential biomarkers; furthermore, a KEGG pathway was applied to analyze the metabolic pathways. All samples were discriminated well in the OPLS-DA model and were divided into two clusters: groups A (0 MOS, and so on), B, C, and D were in one cluster, and groups E and F were in another. A total of 18 biomarkers were identified among all groups and 12 biomarkers between groups A and E, from which we concluded that the post-harvest storage life of matured coconut water shall not exceed 3 months and the pathways of the TCA cycle, protein hydrolysis from coconut meat, and interconversion among amino acids were mainly enriched during the post-harvest storage.
1. Introduction
Since the 1940s, researchers have conducted extensive research on coconut water, which is a natural health drink and is widely consumed due to its health benefits.1–3 For example, coconut water can be used as an alternative to oral rehydration medicine, and even used as intravenous rehydration for patients in remote areas.2 Moreover, coconut water has been found to help prevent myocardial infarction.3 In addition, micronutrients, such as inorganic ions and vitamins, in coconut water are helpful in bolstering the human body's antioxidant system, which can catalyze the removal of oxidizing substances by supplying radicals or being a part of metallo-enzymes, thereby reducing oxidative damage from polyunsaturated fatty acids in the cell membrane or nucleic acids in the nucleus.4 Furthermore, coconut water is widely used in plant tissue culture, which dates back to more than half a century ago when van Overbeek et al.5 first used coconut water as a novel component in callus culture medium. In addition to its nutritional effects, coconut water appears to have growth-regulating properties, such as cytokinin-type activity.6
Metabolomics is a metabolic network established to illustrate the state of an organism within a certain moment or environment. The main research objects of metabolomics are some small molecules which are the end products of gene expression and the biochemical phenotype of a cell or tissue, such as carbohydrates, lipids, amino acids, and nucleotides.7 The main aim of metabolomics is to quantify the metabolic changes in a biological system under certain stimuli (internal stimulation such as genes changes, external one such as temperature changes) and construct information models by means of modern scientific detection and processing methods based on the investigation of the organism community and analysis of the community's overall index.8 The first problem in metabolomics is dynamically detecting the changes in metabolites within the organism and describing the rules of these changes by using a mathematical formula, in order to establish the relationship between the organism and the changing rules of metabolites.9 Therefore, metabolomic analysis possesses indispensable research value in view of fully deriving biological information and improving the use of this information for humans.
Metabolomics has been applied in various fields, such as disease diagnosis,10 drug toxicity and mechanism research,11 gene function elucidation,12 plant metabolomics,13 nutrition science, microbiological research,14 and emerging ecological metabolomics.15,16
Plant metabolomics mostly focuses on areas involving metabolic profiling or metabolite fingerprinting, including (1) metabolomic studies of certain plant species, wherein a plant organ or tissue are selected to analyze the metabolites therein qualitatively and quantitatively; (2) metabolomic phenotype studies of different genotype plants, wherein two or more plants of the same species are selected and metabolomics is applied to compare and identify their different genotypes; (3) metabolomic studies of certain ecological plants, wherein the same plant species in different ecological environments are selected to study the effects of the growth environments on plant metabolites; (4) studies on the autoimmune responses of plants after external stimulation.
Metabolomics has attained breakthroughs in the post-harvest research of fruits and vegetables in recent years, and has become an important research method by revealing the metabolic and quality changes in the growth or post-harvest storage of fruits and vegetables. Rudell et al.17,18 applied various multivariate data analysis to analyze the metabolomic dataset of apple skin and confirmed that superficial scalding is strongly influenced by light treatments and secondary metabolites, such as isoprene and phenylpropanoid. Moreover, dramatic changes in metabolites accelerate the progress of superficial scalding and UV radiation, and can reduce superficial scald incidence in Granny Smith Apple. Sun et al.19 used GC-MS to explore organic acid regulatory mechanisms in post-harvest Hirado Buntan Pummelo, and the results showed that the concentration of organic acids is significantly related to the physiological changes (such as hydrogen peroxide content, superoxide dismutase, and peroxidase activities) during senescence processes, thus indicating that organic acids are an important index for estimating citrus fruit post-harvest senescence processes.
The tender coconut water can be directly consumed or processed into various beverages, whereas matured coconut water is often wasted due to its unpleasant taste; thus, more studies are focused on tender coconut water than on matured coconut water, except for a small part that is used as the culture medium of nata de coco. A series of physiological and biochemical changes occur in coconut water during harvesting; however, how these changes influence the metabolism of matured coconut water during post-harvest storage has not yet been reported. Therefore, this study applied a metabolomic tool (multivariate data analysis based on UPLC-MS/MS) to reveal the rules in the changes and accumulation models of metabolites in matured coconut water during post-harvest storage to provide a theoretical basis for the analysis of deterioration process and metabolic regulation during storage.
2. Materials and methods
2.1 Reagents and chemicals
Double-distilled water (ddH2O) was produced by purifying demineralized water in a purification system (arium® mini, Sartorius, Göttingen, Germany). LC-MS-grade methanol, acetonitrile, and formic acid were obtained from Merck (Darmstadt, Germany).
2.2 Sample collection
Matured coconuts (high-native species) were harvested from a coconut planting base in Wenchang City (Hainan, China). All coconuts had reached the age of 12 months, had uniform color, no damage, and no pest infections as observed from the surface, and were stored indoors at room temperature. Samples (coconut water) were taken out from coconuts collected at 0, 1, 2, 3, 4, and 5 months during storage and quickly stored in a refrigerator (Haier, Qingdao, China) at −80 °C after freezing in liquid nitrogen.
The sampling details and appearance changes of coconuts during storage are shown in Fig. 1.
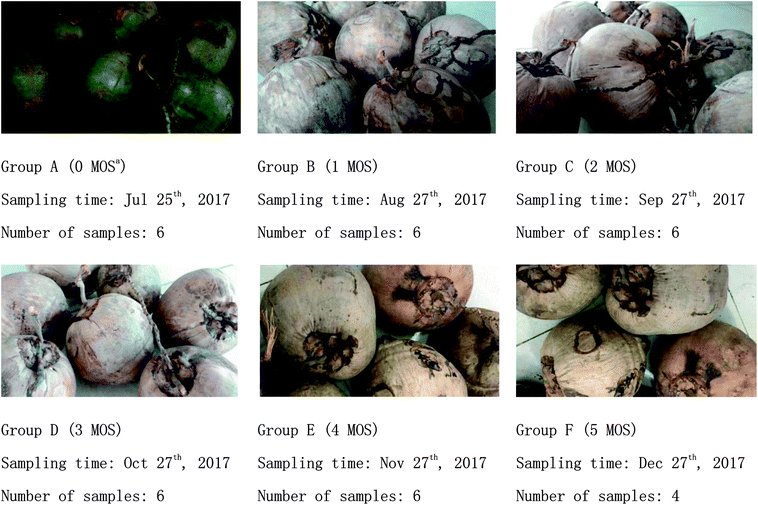 |
| Fig. 1 Appearance changes of coconut water during storage. a MOS: months of storage. | |
2.3 Determination of conductivity, °Brix, pH, and sugar/acid ratio
The conductivity of matured coconut water was measured using a METTLER TOLEDO FE30 conductivity meter; °Brix was measured using an ATAGO PAL-1 refractometer; and pH was measured using a METTLER TOLEDO FE20 pH meter; moreover, we used the formula: sugar/acid ratio = °Brix value/pH value.
2.4 Sample preparation for UPLC-MS/MS analyses
The sample preparation was conducted according to previous studies20,21 with some modifications. After thawing at 4 °C, 100 μL of each sample was transferred into 1.5 mL centrifuge tubes, and each centrifuge tube was added with 200 μL methanol (pre-cooled at −20 °C) and vortexed for 60 s. Afterward, all tubes were centrifuged for 10 min at 12
000 rpm 4 °C and each supernatant was transferred into another 1.5 mL centrifuge tube for blow-drying by vacuum concentration. All dried samples were dissolved with 300 μL methanol aqueous solution (4
:
1, 4 °C) and filtered using a 0.22 μm PTFE membrane (Jinteng, Tianjin, China) to obtain the prepared sample extracts for LC-MS. Finally, 20 μL from each prepared sample extract was taken and mixed as the quality control (QC) sample (QC sample was used to monitor deviations of the analytical results from the pool mixtures and to analyze the errors caused by the instrument itself), and the remaining sample extracts were used for LC-MS detection.
2.5 Metabolomic analysis based on UPLC-MS/MS
The sample extracts were separated using HPLC with a Waters ACQUITY UPLC system equipped with an ACQUITY UPLC® BEH C18 (2.1 mm × 100 mm × 1.7 μm) analysis column maintained at 40 °C. The temperature of the autosampler was 4 °C. Gradient elution of extracts was carried out with 0.1% formic acid in water (A) and 0.1% formic acid in acetonitrile (B) at a flow rate of 0.25 mL min−1. Moreover, 4 μL injections of each extract was done after equilibration. An increasing linear gradient of solvent B (v/v) was used as follows: 0–1 min, 2% B; 1–9.5 min, 2–50% B; 9.5–14 min, 50–98% B; 14–15 min, 98% B; 15–15.5 min, 98–2% B; 15.5–17 min, 2%.
The ESI-MS experiments were executed on the Thermo LTQ Orbitrap XL mass spectrometer with spray voltage values of 4.8 and −4.5 kV in positive and negative modes, respectively. Moreover, sheath gas and auxiliary gas were set at 45 and 15 arbitrary units, respectively. The capillary temperature was 325 °C. The voltages of the capillary and tube were 35 and 50 V, and −15 and −50 V in positive and negative modes, respectively. The Orbitrap analyzer scanned over a mass range of m/z 89–1000 for full scan at a mass resolution of 60
000. Data-dependent acquisition (DDA) MS/MS experiments were performed with CID scan. The normalized collision energy was 30 eV. Dynamic exclusion was implemented with a repeat count of 2, and an exclusion duration of 15 s.22
2.6 Data processing and multivariate data analysis
The raw data obtained was converted by using Proteowizard (v3.0.8789) into mzXML format (xcms input file format) and imported into the XCMS package of R software (v3.3.2) to derive the peak identification, filtration, and alignment with main parameters of bw = 5, ppm = 15, peak width = c (10, 120), mzwid = 0.015, mzdiff = 0.01, and method = “centWave”. Thus, some data matrices, including mass-to-charge ratio (m/z), retention time, and peak area were obtained, including 1405 precursor molecules in positive ion mode and 1275 in negative ion mode. Moreover, non-normalized values for peak areas were exported to Excel (Microsoft Corp., Redmond, WA, USA) for batch normalization and calculation. Pareto scaling and visualization of data were accomplished by plotting principal component scores, wherein each coordinate represented a single biological sample. Principal component analysis (PCA) and partial least squares discriminant analysis (PLS-DA) were employed to classify and discriminate the samples according to the multidimensional data generated from the analytical techniques. The predictive ability of the models estimated by cross validation was analyzed using three predictive components (R2X, R2Y, and Q(cum)2). Afterward, orthogonal projection to latent structure discriminant analysis (OPLS-DA) model was applied to extract the interpretable information from the multidimensional data and isolate the metabolites responsible for the differences among each group. Features with high loadings in the corresponding loading plots that exerted high influence for PCA, PLS-DA, or OPLS-DA calculation were picked for further identification. First, one-way ANOVA with Tukey's test was used to analyze the significant difference of each metabolite in the group discrimination, and fold change ≥1.5 or ≤0.667 and significance at p < 0.05 were used to identify differential biomarkers for grouping. Additionally, differential biomarkers were ranked using support vector machine (SVM) according to their influence or importance. Furthermore, pathway analysis was conducted using MetaboAnalyst 3.0, which is an open-source bioinformatics Web site mainly based on KEGG pathway for metabolite data interpretation.
3. Results and discussion
3.1 Changes in conductivity, °Brix, pH, and sugar/acid ratio of matured coconut water during storage
The influence of storage time on conductivity, °Brix, pH, and sugar/acid ratio is shown in Fig. 2. No significant change in the conductivity of matured coconut water was found during 0–1 MOS; however, a significant increase was observed until the end of storage (5 MOS). The °Brix of matured coconut water declined rapidly at 1 MOS from 4.17 to 3.14, but increased continuously to 6.67 until the end, thus indicating that the °Brix of matured coconut water experienced dramatic fluctuations during storage. The rapid decrease in °Brix at 1 MOS might have been caused by high metabolic activity, and the following increase may be due to the weakening metabolism and the rapid decrease in coconut water volume. The pH of matured coconut water rose rapidly from 5.49 to 5.78 at 1 MOS, then gradually decreased, reaching a peak value of 5.20 at 4 MOS. After this time, it rose again, but with no significant changes. The increase in pH at 1 MOS may be caused by the consumptions of organ acids due to the oxidative metabolism via the Krebs cycle, gluconeogenesis, fermentation, anthocyanin synthesis, amino acids interconversion, etc.23
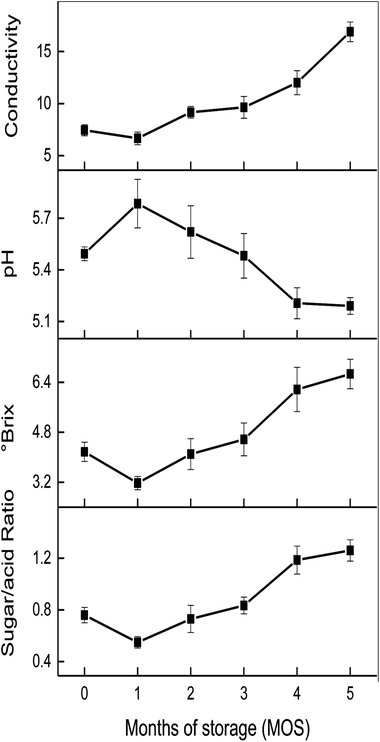 |
| Fig. 2 Changes in conductivity, °Brix, pH and sugar/acid ratio of matured coconut water during storage. | |
The sugar/acid ratio, a quantitative indicator for taste and consumer preferences,19 showed a global upward trend (Fig. 2), reaching a peak of 1.25 at 5 MOS. However, the sugar/acid ratio dropped sharply at 1 MOS from 0.78 to 0.54, and the sharp decline corresponded to the dramatic changes in °Brix and pH values during this period. After 1 MOS, the sugar/acid ratio rose steadily until the end of storage, mainly due to the increase in °Brix and decrease in pH value. Therefore, the °Brix, pH, and sugar/acid ratio of matured coconut water at 3 MOS were similar to that of the fresh sample; however, the sugar/acid ratio increased dramatically, leading to flavor fading and commercial decrease. Therefore, the 1, 3, and 4 MOS during post-harvest storage were the key time points for changes in coconut water flavor and quality (including °Brix, pH, and sugar/acid ratio).
3.2 Metabolic profiling analysis of matured coconut water during storage
The differentially expressed (DE) metabolites are shown in Fig. 3a and e. For each phenotypic change, the metabolites that are upregulated or downregulated from the standard in cells or between the experimental and control groups are known as DE metabolites. The method used to identify these DE metabolites in the present study was Student's-test (for two groups).24 A total of 1405 precursor molecules were obtained from nontargeted UPLC-MS/MS analysis in the positive ion mode and 1275 precursor molecules were obtained in the negative ion mode (hereafter referred to as NT-pos and NT-neg). Fig. 3a and e show that in NT-pos, the number of upregulated DE metabolites show a continually increasing trend and reached a peak of 200 at 5 MOS (group F), and the downregulated DE metabolites initially increased to 148 and then decreased; whereas in NT-neg, both upregulated and downregulated DE metabolites reached the maximum values in group E. In addition, multivariate statistical analysis was employed to discriminate samples based on the dataset obtained from NT-pos and NT-neg. With regard to the data from NT-pos, the resulting PCA score plot (Fig. 3b) showed, that except for F1 and F3, the samples were mostly located in the 95% confidence interval (hotelling T2 ellipse). The separation of MOS was insufficient with strong overlapping of samples from groups A, B, C, and D. However, PLS-DA (Fig. 3c) showed no improvement over PCA with overlapping samples from groups A and B, as well as groups C and D; although being a supervised method and having better separation of sample groups by maximizing the covariance between the explanatory variables (metabolites) and the response (groups).25 OPLS-DA model was further used to analyze the metabolites, and its score plot (Fig. 3d) showed that all samples were discriminated well. Meanwhile, the same conclusion could be drawn from data of NT-neg. The PCA score plot (Fig. 3f) showed that A3 was out of the 95% hotelling T2 ellipse, and separation of MOS was insufficient with strong overlapping of samples from all groups. However, PLS-DA (Fig. 3g) showed better discrimination over PCA with less overlapping of samples. Furthermore, OPLS-DA score plot (Fig. 3h) showed that all samples were grouped well. Therefore, compared with PLS-DA and PCA, OPLS-DA led to better discrimination of the sample groups in both NT-pos and NT-neg.
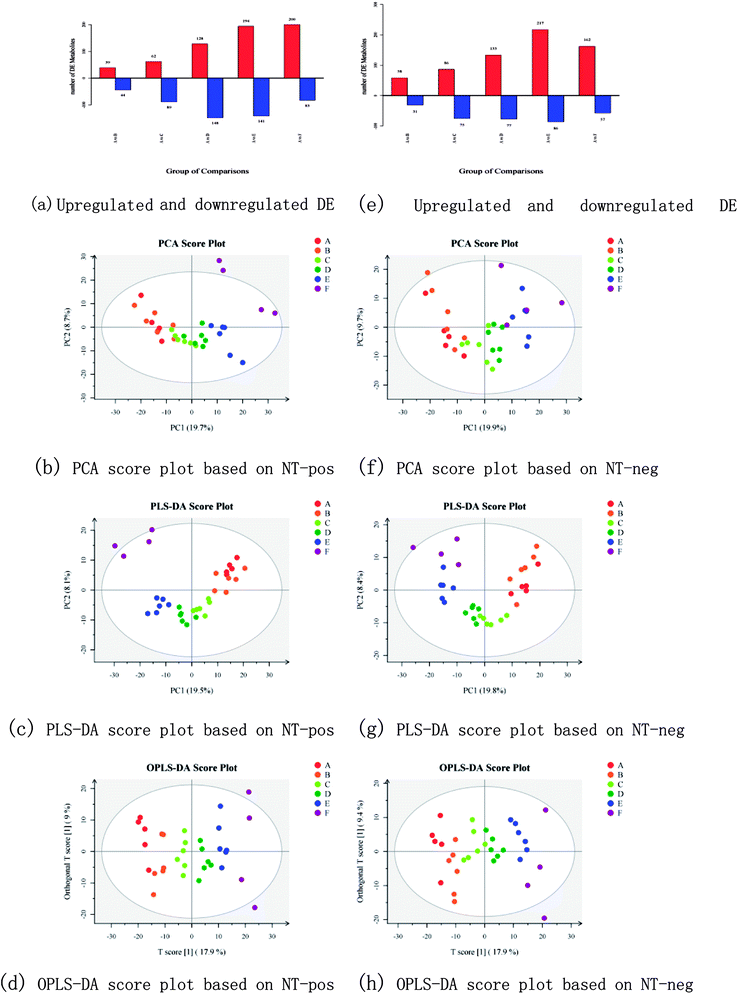 |
| Fig. 3 Upregulated and downregulated DE metabolites, PCA score plots, PLS-DA score plots, and OPLS-DA score plots based on NT-pos ((a)–(d)) and NT-neg ((e)–(h)). | |
3.3 Metabolomic analyses of matured coconut water among all groups
The criteria of one-way ANOVA p-value < 0.05 and false discovery rate (FDR) multiple test were used to compare the DE metabolites obtained from NT-pos and NT-neg. A total of 14 differential biomarkers, namely, L-valine, L-threonine, L-isoleucine, L-leucine, L-proline, 5-oxo-D-proline, gamma-aminobutyric acid zwitterion, taurine, dibutyl phthalate, deethylatrazine, 4-hydroxyphenylacetic acid, (R)-pantothenic acid, acetoacetate, and sphinganine at m/z [M + H]+ ions; and three biomarkers, namely, keto-D-fructose, malic acid, and fenbufen at m/z [M − H]− ions, as well as (S)-malate at m/z [M + Na]+ ion (Fig. 4a), were determined and identified in all samples. Afterward, these differential biomarkers in all groups were used for hierarchical Cluster Analysis, and the samples were grouped into two clusters: Cluster A had higher concentrations of taurine, sphinganine, malic acid, fenbufen, 5-oxo-D-proline, L-isoleucine, L-valine, L-threonine, deethylatrazine, L-leucine, (R)-pantothenic acid, (S)-malate, di-4-hydroxyphenylacetic acid, and butyl phthalate; and Cluster B contained higher gamma-aminobutyric acid zwitterion, L-proline, acetoacetate, and keto-D-fructose concentrations (Fig. 4a). Furthermore, in observing the distribution patterns of the samples in the two clusters, the samples of groups E and F were mainly concentrated on Cluster A, samples of groups A, B, C, and D were mainly concentrated on Cluster B, which meant that high metabolomic differences existed between groups A and E. Moreover, the metabolomic analyses between groups A and E are further discussed in Section 3.4.
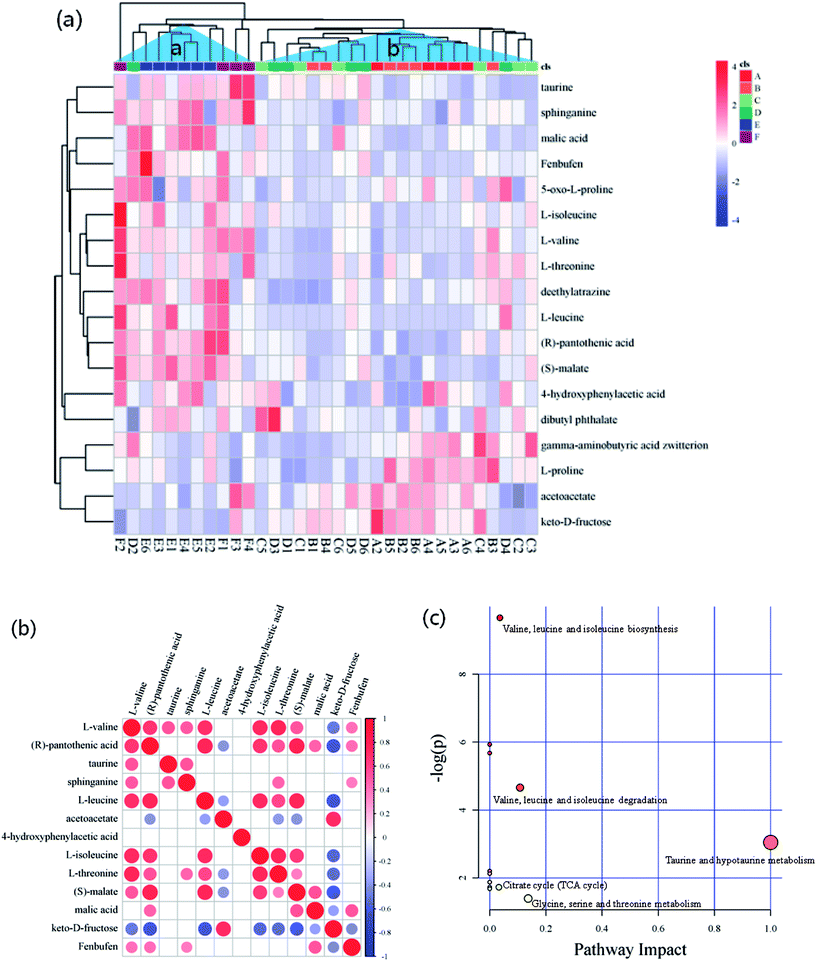 |
| Fig. 4 (a) Heatmap for the differential biomarkers combined with hierarchical cluster analysis, (b) correlation matrices of biomarkers, and (c) pathway analysis based on biomarkers of matured coconut water during storage. | |
Pairwise correlation analysis is helpful to identify biosynthetically related and coordinately regulated metabolites;26–28 it was performed to study the relationships between the 13 main differential biomarkers of all sample groups. The correlation matrix is shown in Fig. 4b, wherein the correlation of two biomarkers was considered high if the corresponding coefficient value ≥ 0.7. The results gave a total of 38 pairs of correlation coefficient values, thus confirming 12 highly positively correlated pairs and 3 highly negatively correlated pairs of differential biomarkers. Among these highly positively correlations, 5 pairs of amino acids were present (e.g., L-leucine and L-valine, L-isoleucine and L-valine, L-threonine and L-valine, L-isoleucine and L-leucine, and L-threonine and L-isoleucine). Except for the differential biomarkers of 4 amino acids with highly positively correlation to each other, a non-protein amino acid (taurine) was detected and identified as a biomarker. Fig. 4b shows that taurine is not correlated to any amino acid. There have been explanations for the high correlation between amino acids, which were also observed by Roessner et al.,29 who considered that the highly positive correlation between leucine and isoleucine is due to their common utilization of the same terminal enzyme activity (branched chain amino acid transaminase) and the same cofactor (glutamate) in their biosynthesis. The same authors reported a nonlinear curve for the correlation of lysine and methionine, which is a metabolite but not a differential biomarker in the present study; and this correlation mainly seemed to be in accordance with a feedback regulation model based on high flux.30 Moreover, Dobson et al.26 demonstrated a linear relationship of isoleucine and valine and a nonlinear relationship of lysine and serine. On the other hand, the correlation between fructose and pantothenic acid, fructose and leucine, and fructose and malate were highly negative, and all had coefficient values of ≤−0.7. These negative correlations were induced by the reduction of fructose to pyruvate, where malate was generated via TCA cycle, leucine was derived from alanine by transamination,31 and pantothenic acid, which is present as coenzyme A in animal tissues, as well as in microorganisms and plants, was involved in pyruvate utilization.32,33
Generally, according to the analysis of metabolic pathways (Fig. 4c) using KEGG pathway and MetPA databases, the differential biomarkers of matured coconut water during post-harvest storage mainly enriched the following metabolic pathways: (1) hydrolysis of proteins from coconut meat increased amino acids; (2) taurine and hypotaurine metabolism induce increase in glutamate and reduction in cysteine, wherein hypotaurine is an intermediate of taurine biosynthesis from cysteine,34 and glutamate production is modulated by taurine;35 (3) glycine, serine, and threonine metabolism reduces 3-phospho-D-glycerate, which is an intermediate of glycolysis and gives rise to serine and glycine, and also reduces aspartate, which gives rise to threonine in plants and bacteria; (4) pantothenate and CoA biosynthesis give rise to 4′-phosphopantetheine, promotes TCA cycle, β-oxidation, and fatty acid and polyketide biosynthesis pathways as essential cofactors;36 (6) valine, leucine, and isoleucine biosynthesis lead to 3-phospho-D-glycerate and pyruvate consumption through transamination;31 (7) activation of anaerobic metabolism leads to adenosine triphosphate depletion, sugar consumption, organic acid production, and pH decrease;37 (8) pH decrease hinders anaerobic metabolism.
3.4 Metabolomic analyses of matured coconut water between groups A and E
From the hierarchical clustering analysis (Fig. 4a) of differential biomarkers during post-harvest storage, samples of groups E and F were mainly restricted to Cluster A, and samples of groups A, B, C, and D were mainly restricted to Cluster B. Further metabolomic analysis was therefore performed to analyze the difference between groups A and E, and this clustering was supported by the results of °Brix, pH, and sugar/acid ratio, as shown in Fig. 2, wherein dramatic changes are shown between groups A, B, C, and D, and groups E and F. The criteria of one-way ANOVA p-value < 0.05 and fold change (FC) ≥ 1.5 or ≤−0.667 multiple test were used to compare these DE metabolites obtained from both NT-pos and NT-neg. A total of 9 differential biomarkers, namely, dibutyl phthalate, L-leucine, (S)-malate, L-valine, deethylatrazine, L-threonine, (R)-pantothenic acid, taurine, and malic acid, were upregulated significantly in groups A to E; whereas 4 biomarkers, including gamma-aminobutyric acid zwitterion, acetoacetate, and keto-D-fructose, were downregulated, as shown in Table 1. Afterward, we ranked the 12 differential biomarkers using SVM technique based on their importance, and the ranked upregulated and downregulated biomarkers are shown in Fig. 5a, where red bars denote upregulated biomarkers and blue bars denote downregulated biomarkers. Taurine was thus identified as the most influential upregulated biomarker and keto-D-fructose was the most influential downregulated biomarker between groups A and E. In addition, we constructed a correlation network using the 12 upregulated and downregulated differential biomarkers data set, as shown in Fig. 5b. The results gave a total of 40 pairs of correlation coefficient values, confirming the 13 highly positively correlated pairs of biomarkers with values of ≥0.7; 12 positive correlated pairs with values of 0.6–0.7; 12 highly negatively correlated pairs with values of ≤−0.7; and 3 negative correlated pairs with values of −0.6–−0.7. From Fig. 5c, (S)-malate showed the most connected nodes and the most connections to other biomarkers. Furthermore, L-valine, L-threonine, and pantothenic acid showed the same correlation characteristics, showed the second most connected nodes, and displayed no correlations with dibutyl phthalate and gamma-aminobutyric acid zwitterion, as well as the same negative correlations with acetoacetate and keto-D-fructose; whereas gamma-aminobutyric acid zwitterion showed the least connected nodes (Fig. 5b).
Table 1 A list of 12 ions as the identified biomarkers in LC-MS-based analysis between group A and E of matured coconut water
No. |
Metabolites |
mz |
RT |
Exact mass |
Precursor_type |
Formula |
Fold change |
p-value |
FDR |
1 |
Dibutyl phthalate |
279.16 |
48.6329 |
278.3435 |
[M + H]+ |
C16H22O4 |
1.5642 |
0.015450 |
0.061998 |
2 |
L-Leucine |
132.10 |
562.92 |
131.1730 |
[M + H]+ |
C6H13NO2 |
3.2962 |
0.001121 |
0.013333 |
3 |
(S)-Malate |
133.01 |
331.87 |
134.0215 |
[M + H]+ |
C4H6O5 |
1.5003 |
0.013515 |
0.074707 |
4 |
L-Valine |
118.09 |
118.09 |
119.1192 |
[M + H]+ |
C5H11NO2 |
6.3049 |
0.008480 |
0.043862 |
5 |
Deethylatrazine |
188.07 |
254.66 |
187.0625 |
[M + H]+ |
C6H10ClN5 |
1.6827 |
0.015390 |
0.06199 |
6 |
L-Threonine |
120.07 |
80.9026 |
119.1192 |
[M + H]+ |
C4H9NO3 |
2.6115 |
0.002244 |
0.020482 |
7 |
(R)-Pantothenic acid |
220.12 |
222.67 |
219.2350 |
[M + H]+ |
C9H17NO5 |
2.928 |
0.002717 |
0.021808 |
8 |
Taurine |
125.99 |
299.08 |
131.1730 |
[M + H]+ |
C2H7NO3S |
1.9281 |
0.001045 |
0.012768 |
9 |
Malic acid |
133.01 |
105.06 |
134.08744 |
[M − H]− |
C4H6O5 |
2.3213 |
0.001322 |
0.014273 |
10 |
Gamma-aminobutyric acid zwitterion |
104.07 |
74.72 |
103.1198 |
[M + H]+ |
C4H9NO2 |
0.46612 |
0.021746 |
0.076232 |
11 |
Acetoacetate |
103.04 |
93.00 |
102.09 |
[M + H]+ |
C4H6O3 |
0.61798 |
0.001131 |
0.013333 |
12 |
Keto-D-fructose |
179.06 |
95.69 |
180.1559 |
[M − H]− |
C6H12O6 |
0.33386 |
0.007132 |
0.037234 |
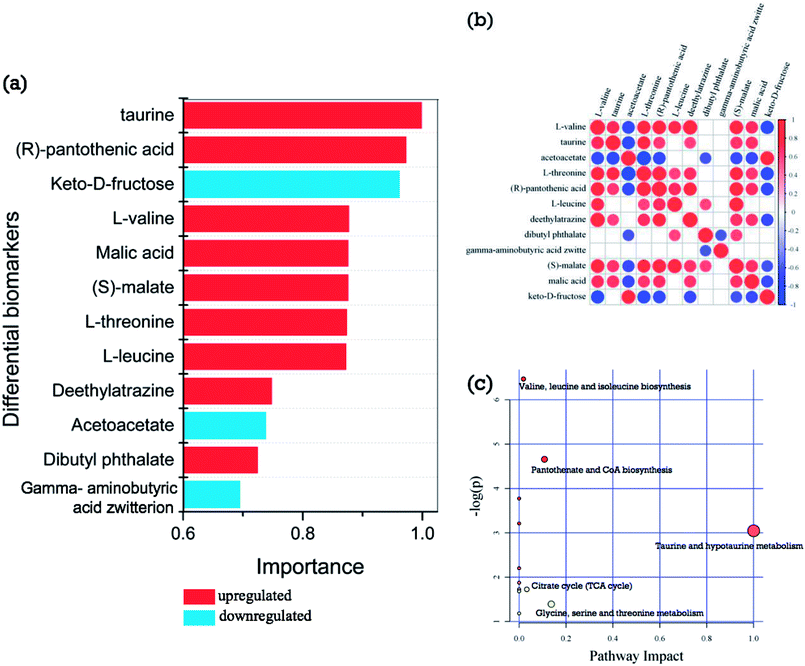 |
| Fig. 5 (a) Ranking the 12 biomarkers according to their importance; (b) correlation matrices of biomarkers, and (c) pathway analysis based on biomarkers of matured coconut water between group A and E. | |
According to the analysis of metabolic pathways (Fig. 5c) using KEGG pathway and MetPA databases, the differential biomarkers of matured coconut water between groups A and E mainly enriched the following metabolomic pathways: (1) hydrolysis of proteins from coconut meat increases amino acids; (2) taurine and hypotaurine metabolism induce glutamate increase and cysteine reduction; (3) glycine, serine, and threonine metabolism reduce 3-phospho-D-glycerate aspartate; (4) pantothenate and CoA biosynthesis give rise to 4′-phosphopantetheine, and promotes TCA cycle, β-oxidation, and fatty acid and polyketide biosynthesis pathways as essential cofactors; (6) valine, leucine, and isoleucine biosynthesis lead to 3-phospho-D-glycerate and pyruvate consumption; (7) activation of anaerobic metabolism leads to adenosine triphosphate depletion, sugar consumption, organic acid production, and pH decrease.
4. Conclusion
A series of physiological and biochemical changes and quality deterioration will occur in matured coconut water during the post-harvest storage, thus affecting flavor and industrial value. The metabolomic technique based on UPLC-MS/MS employed in the present study was efficient for exploring physiological and biochemical changes in coconut water during storage. Furthermore, compared with the other two multivariate statistics analyses, namely PCA and PLS-DA, OPLS-DA led to better discrimination of the sample groups in NT-pos and NT-neg. A total of 18 metabolites were identified as differential biomarkers in all groups, which were separated using hierarchal Cluster Analysis based on these biomarkers into two clusters: groups A, B, C, and D in one, and groups E and F in another. Therefore, by using metabolomic analysis, coconut water should be within a storage period of no more than 4 months, and this is the most suitable storage life.
The present study also has its limitations. For instance, during post-harvest, the metabolism of coconut meat plays an important influence on the metabolism of coconut water; thus, studying how the metabolomics, proteomics, and transcriptomics of coconut meat influence coconut water during post-harvest should be interesting.
Conflicts of interest
The authors report no conflicts of interest. The authors alone are responsible for the content and writing of the paper.
Acknowledgements
This research was supported by the Natural Science Foundation of Hainan Province of China (ID: 317002), the National Natural Science Foundation of China (ID: 31640061; 31801494), and the Hainan University Start-up Scientific Research Projects of China (ID: kyqd1551; kyqd1630).
References
- The encyclopedia of fruit and nuts, ed. J. Janick, and R. E. Paull, CABI, Wallingford, United Kingdom, 2008, p. 112 Search PubMed.
- D. Campbell-Falck, T. Thomas, T. M. Falck, N. Tutuo and K. Clem, The intravenous use of coconut water, Am. J. Emerg. Med., 2000, 18, 108–111 CrossRef PubMed.
- P. Anurag and T. Rajamohan, Cardioprotective effect of tender coconut water in experimental myocardial infarction, Plant Foods Hum. Nutr., 2003, 58, 1–12 CrossRef.
- A. Shenkin, The key role of micronutrients, Clin. Nutr., 2006, 25, 1–13 CrossRef PubMed.
- J. van Overbeek, M. E. Conklin and A. F. Blakeslee, Factors in coconut milk essential for growth and development of very young Datura embryos, Science, 1941, 94, 350–351 CrossRef PubMed.
- E. F. George, and P. D. Sherrington, Plant propagation by tissue culture-Handbook and directory of commercial laboratories, Exegetics Ltd, Eversley, Basingstoke, 1984 Search PubMed.
- M. D. Mailman, M. Feolo, Y. Jin, M. Kimura, K. Tryka and R. Bagoutdinov, et al., The NCBI dbGaP database of genotypes and phenotypes, Nat. Genet., 2007, 39, 1181–1186 CrossRef PubMed.
- H. U. Zhengqinga, X. Lin and M. Guo, Advances in research techniques of metabonomics, Chin. J. Mod. Appl. Pharm., 2010, 27, 485–490 Search PubMed.
- H. Tang and Y. Wang, Metabonomics: a revolution in progress, Prog. Biochem. Biophys., 2006, 33, 401–417 Search PubMed.
- J. Nielsen and S. Oliver, The next wave in metabolome analysis, Trends Biotechnol., 2005, 23, 544–546 CrossRef PubMed.
- T. M. Ebbels, H. C. Keun, O. P. Beckonert, M. E. Bollard, J. C. Lindon and E. Holmes, et al., Prediction and classification of drug toxicity using probabilistic modeling of temporal metabolic data: the consortium on metabonomic toxicology screening approach, J. Proteome Res., 2007, 6, 4407–4422 CrossRef PubMed.
- C. Denkert, J. Budczies, T. Kind, W. Weichert, P. Tablack and J. Sehouli, et al., Mass spectrometry-based metabolic profiling reveals different metabolite patterns in invasive ovarian carcinomas and ovarian borderline tumors, Cancer Res., 2006, 66, 10795–10804 CrossRef PubMed.
- O. Fiehn, Metabolic networks of cucurbita maxima phloem, Phytochemistry, 2003, 62, 875–886 CrossRef PubMed.
- L. W. Sumner, P. Mendes and R. A. Dixon, Plant metabolomics: large-scale phytochemistry in the functional genomics era, Phytochemistry, 2003, 62, 817–836 CrossRef PubMed.
- J. Sardans, J. Peñuelas and A. Rivas-Ubach, Ecological metabolomics: overview of current developments and future challenges, Chemoecology, 2011, 21, 191–225 CrossRef.
- J. P. J. Sardans, Ecological metabolomics, Chem. Ecol., 2009, 25(4), 305–309 CrossRef.
- D. R. Rudell, J. P. Mattheis and E. A. Curry, Prestorage ultraviolet-white light irradiation alters apple peel metabolome, J. Agric. Food Chem., 2008, 56, 1138–1147 CrossRef PubMed.
- D. R. Rudell, J. P. Mattheis and M. L. A. T. M. Hertog, Metabolomic change precedes apple superficial scald symptoms, J. Agric. Food Chem., 2009, 57, 8459 CrossRef PubMed.
- X. Sun, A. Zhu, S. Liu, L. Sheng, Q. Ma, L. Zhang and Y. Cheng, et al., Integration of Metabolomics and Subcellular Organelle Expression Microarray to Increase Understanding the Organic Acid Changes in Post-harvest Citrus Fruit, J. Integr. Plant Biol., 2013, 55, 1038–1053 CrossRef PubMed.
- W. B. Dunn, D. Broadhurst, P. Begley, E. Zelena, S. Francismcintyre and N. Anderson, et al., Procedures for large-scale metabolic profiling of serum and plasma using gas chromatography and liquid chromatography coupled to mass spectrometry, Nat. Protoc., 2011, 6, 1060–1083 CrossRef PubMed.
- E. Zelena, W. B. Dunn, D. Broadhurst, S. Francismcintyre, K. M. Carroll and P. Begley, et al., Development of a robust and repeatable uplc−ms method for the long-term metabolomic study of human serum, Anal. Chem., 2009, 81, 1357–1364 CrossRef PubMed.
- E. J. Want, P. Masson, F. Michopoulos, I. D. Wilson, G. Theodoridis and R. S. Plumb, et al., Global metabolic profiling of animal and human tissues via uplc-ms, Nat. Protoc., 2013, 8, 17–32 CrossRef PubMed.
- F. Famiani, N. G. M. Cultrera, A. Battistelli, V. Casulli, P. Proietti and A. Standardi, et al., Phosphoenolpyruvate carboxykinase and its potential role in the catabolism of organic acids in the flesh of soft fruit during ripening, J. Exp. Bot., 2005, 56, 2959–2969 CrossRef PubMed.
- N. Kumar, M. Hoque, M. Shahjaman, S. M. Islam, M. Mollah and N. Haque, Metabolomic Biomarker Identification in Presence of Outliers and Missing Values, BioMed Res. Int., 2017, 11, 2437608 Search PubMed.
- K. H. Liland, Multivariate methods in metabolomics–from pre-processing to dimension reduction and statistical analysis, TrAC, Trends Anal. Chem., 2011, 30, 827–841 CrossRef.
- G. Dobson, T. Shepherd, S. R. Verrall, S. Conner, J. W. McNicol, G. Ramsay and D. Stewart, Phytochemical diversity in tubers of potato cultivars and landraces using a GC-MS metabolomics approach, J. Agric. Food Chem., 2008, 56, 10280–10291 CrossRef PubMed.
- G. Rao, J. Sui and J. Zhang, Metabolomics reveals significant variations in metabolites and correlations regarding the maturation of walnuts (Juglans regia L.), Biol. Open, 2016, 5, 829–836 CrossRef PubMed.
- Y. S. Shin, K. H. Bang, D. S. In, J. S. Sung, S. Y. Kim, B. C. Ku, S. W. Kim, D. Lee and H. K. Choi, Fingerprinting differentiation of Astragalus membranaceus roots according to ages using 1H-NMR spectroscopy and multivariate statistical analysis, Biomol. Ther., 2009, 17, 133–137 CrossRef.
- U. Roessner, A. Luedemann, D. Brust, O. Fiehn, T. Linke and L. Willmitzer, et al., Metabolic profiling allows comprehensive phenotyping of genetically or environmentally modified plant systems, Plant Cell, 2001, 13, 11–29 CrossRef.
- D. Bartlem, I. Lambein, T. Okamoto, A. Itaya, Y. Uda and F. Kijima, et al., Mutation in the threonine synthase gene results in an over-accumulation of soluble methionine in arabidopsis, Plant Physiol., 2000, 123, 101–110 CrossRef PubMed.
- P. Felig and J. Wahren, Amino acid metabolism in exercising man, J. Clin. Invest., 1971, 50, 2703–2714 CrossRef PubMed.
- F. Lipmann, N. O. Kaplan, G. D. Novelli, L. C. Tuttle and B. M. Guirard, Coenzyme for acetylation, a pantothenic acid derivative, J. Biol. Chem., 1947, 167, 869–870 Search PubMed.
- R. E. Olson and N. O. Kaplan, The effect of pantothenic acid deficiency upon the coenzyme A content and pyruvate utilization of rat and duck tissues, J. Biol. Chem., 1948, 175, 515–529 Search PubMed.
- A. Brand, D. Leibfritz, B. Hamprecht and R. Dringen, Metabolism of cysteine in astroglial cells: synthesis of hypotaurine and taurine, J. Neurochem., 2010, 71, 827–832 CrossRef.
- A. E. Idrissi, C. Harris, and E. Trenkner, Taurine Modulates Glutamate- and Growth Factors-Mediated Signaling Mechanisms, Taurine 3, Springer, US, 1998, pp. 385–396 Search PubMed.
- Y. Yokooji, H. Tomita, H. Atomi and T. Imanaka, Pantoate kinase and phosphopantothenate synthetase, two novel enzymes necessary for CoA biosynthesis in the Archaea, J. Biol. Chem., 2009, 284, 28137–28145 CrossRef PubMed.
- M. Sugimoto, S. Obiya, M. Kaneko, A. Enomoto, M. Honma, M. Wakayama and M. Tomita, Metabolomic profiling as a possible reverse engineering tool for estimating processing conditions of dry-cured hams, J. Agric. Food
Chem., 2017, 65, 402–410 CrossRef PubMed.
Footnote |
† These authors contributed to the work equally and should be regarded as co-first author. |
|
This journal is © The Royal Society of Chemistry 2018 |
Click here to see how this site uses Cookies. View our privacy policy here.