DOI:
10.1039/C8RA07342B
(Paper)
RSC Adv., 2018,
8, 36534-36541
A targeted metabolomic protocol for quantitative analysis of volatile organic compounds in urine of children with celiac disease
Received
3rd September 2018
, Accepted 21st October 2018
First published on 29th October 2018
Abstract
Volatile organic compounds (VOCs) in biological samples have gained popularity for disease monitoring and diagnosis. Celiac disease (CD) is one of the many prevalent health conditions which are challenging to diagnose. The aim of this study was to optimize a solid phase microextraction followed by gas chromatography-mass spectrometry, for quantitative analysis of a wide range of VOCs in the urine of patients with CD. Multivariate design of experiment was used to optimize the extraction conditions for the analysis of 15 urinary VOCs. Based on the performed experiments, extraction using 2.98 g of sodium chloride and 21 μL of 6 M hydrochloric acid for 15 min at 30 °C, using a CAR/PDMS fiber in headspace mode was found to be the most effective procedure for the analysis of the selected biomarkers. It was also demonstrated that the proposed method could distinguish between children with CD and healthy children based on the profile of VOCs. It is believed that quantitative analysis of these biomarkers will extend our understanding of CD and could be used for monitoring in patients under treatment.
1. Introduction
Celiac disease (CD) is an autoimmune, gluten-induced enteropathy, observed in genetically predisposed individuals, and affects approximately 1% of the global population.1 CD often remains undiagnosed due to its atypical and silent manifestations.2 The only available treatment of CD is to follow a gluten-free diet, however, many patients continue to experience symptoms of the disease even after years of an appropriate dietary compliance. It is believed that more knowledge is required for better understanding, screening and monitoring of CD, consequently improving the gluten-free diet, alleviating the symptoms of the disease.
Analysis of volatile organic compounds (VOCs) in urine samples holds promise for non-invasive detection of the medical conditions.3 VOCs are a diverse group of carbon-based compounds that are released by the human body as by-products of metabolism, hormonal changes, microbiota activity and presence of environmental pollutants.4 They can be detected in all biological specimens including breath, blood, saliva, urine, feces and sweat.5 There is an increasing number of studies analysing urine for detection of various health conditions due to the ease of sampling without compromising the comfort of patients even with repeated sampling.6 Analysis of VOCs in urine can provide information about changes in metabolism caused by the presence of the disease. Urinary biomarkers, including VOCs, were reported for the detection of cancer,7,8 oxidative stress,9 inflammatory bowel disease,10 urological11 and infectious diseases.12 Fewer studies have reported changes in the profile of VOCs in urine and fecal samples in patients with CD, compared to healthy individuals.13,14 Moreover, the analysis of VOC in urine has been used to distinguish people with CD from those with irritable bowel syndrome.15 Although, interest in the analysis of urinary VOCs is growing, scarce information is available on the quantitative analyses of individual compounds or selected classes of metabolites of urinary biomarkers,6,9,16,17 highlighted by Mochalski and Unterkofler.6 Since VOCs serve as markers of bodily biological mechanisms, a change in their relative concentrations may provide a deeper understanding of different stages and propagation of a disease. Therefore, it is imperative to develop simple and robust analytical methods for the quantitative analysis of a wide range of biomarkers from urine for patients with CD.
A number of analytical methods have been used to study the composition of urine, including field asymmetric ion mobility spectrometry,15 sensor arrays (e.g. e-nose),18 nuclear magnetic resolution spectroscopy,19 ion flow tube mass spectrometry20 and most commonly gas chromatography-mass spectrometry (GCMS) with various extraction techniques.6,8,9,21 Urine contains a wide range of metabolites including ketones, alcohols, pyrroles and sulfur-containing compounds.5 The diversity of VOCs in terms of chemical properties, functional groups and polarities presents challenges that need to be addressed by more sensitive analytical methods. In the present study, solid phase microextraction (SPME) was optimized and validated followed by GCMS, for quantitative analysis of a wide range of urinary biomarkers in patients with CD. SPME is a robust sample treatment technique which offers flexibility to be fine-tuned for quantitative analysis of a wide range of organic compounds22 at trace levels. The extraction method was optimized by multivariate design of experiment (DoE) for 15 urinary biomarkers representing various classes of organic compounds. The biomarkers were chosen on the basis of previously published research on the most dominant differences between the metabolic profiles of patients with CD and healthy individuals.13 The VOCs studied here comprised of 2,3-butanedione; 2-butanone; thiophene; dimethyl disulfide; hexanal; 4-heptanone; 2-heptanone; 2-pentylfuran; dimethyl trisulfide; 6-methyl-5-hepten-2-one; benzaldehyde; octanal; trans-3-octen-2-one; p-cresol and 1,3-di-tert-butylbenzene (Table 1). It is believed that quantitative analysis of these biomarkers will extend our understanding not only towards the propagation of CD but also be useful to monitor the condition of patients under treatment. Moreover, in this study, the use of the optimized method for the analysis of real samples from healthy and CD children was demonstrated. To our knowledge, this is the first ever study dedicated to the quantitative analysis of urinary volatile biomarkers for CD.
Table 1 Metabolites investigated in the present study with their CAS number, retention time (RT) and calculated retention index (RI)
Compound |
CAS number |
RT (min) |
RI |
2,3-Butanedione |
431-03-8 |
11.45 |
646 |
2-Butanone |
78-93-3 |
11.74 |
653 |
Thiophene |
110-02-1 |
14.09 |
707 |
Dimethyl disulfide |
624-92-0 |
17.44 |
790 |
Hexanal |
66-25-1 |
19.80 |
850 |
4-Methylthiazole |
693-95-8 |
20.59 |
869 |
4-Heptanone |
123-19-3 |
22.61 |
921 |
2-Heptanone |
110-43-0 |
23.48 |
945 |
2-Pentylfuran |
3777-69-3 |
26.16 |
1017 |
Dimethyl trisulfide |
3658-80-8 |
26.88 |
1038 |
6-Methyl-5-hepten-2-one |
110-93-0 |
27.11 |
1045 |
Benzaldehyde |
100-52-7 |
27.24 |
1048 |
Octanal |
124-13-0 |
27.52 |
1057 |
trans-3-Octen-2-one |
18402-82-9 |
29.29 |
1107 |
p-Cresol |
106-44-5 |
32.46 |
1206 |
1,3-Di-tert-butylbenzene |
1014-60-4 |
34.99 |
1290 |
Hypothesis: children with CD can be distinguished from healthy children based on the profile of urinary VOCs.
2. Experimental
A. Standards and materials
Standards of all analyzed compounds: 2,3-butanedione, 2-butanone, thiophene, dimethyl disulfide, hexanal, 4-heptanone, 2-heptanone, 2-pentylfuran, dimethyl trisulfide, 6-methyl-5-hepten-2-one, benzaldehyde, octanal, trans-3-octen-2-one, p-cresol and 1,3-di-tert-butylbenzene, internal standard: 4-methyl-2-phenol and saturated alkanes (C7–C40) were supplied by Sigma-Aldrich (Saint Louis, USA). Retention indexes for selected compounds were calculated according to Zellner and co-authors.23 MilliQ water (Millipore, Bedford, USA) was used for the preparation of standards. Sodium chloride (NaCl, ≥99.5%) was purchased from Sigma-Aldrich (Saint Louis, USA), sodium hydroxide (NaOH, 99%) from POCH (Gliwice, Poland) and hydrochloric acid (HCl, 37%) was purchased from Chempur (Piekary Śląskie, Poland). The SPME fibers used in the experiments were: 50/30 μm divinylbenzene/carboxen/polydimethylsiloxane (DVB/CAR/PDMS) (stable flex), 65 μm polydimethylsiloxane/divinylbenzene (PDMS/DVB) (stable flex), 7 μm polydimethylsiloxane (PDMS7), 100 μm polydimethylsiloxane (PDMS100), 75 μm carboxen/polydimethylsiloxane (CAR/PDMS) (stable flex), 85 μm polyacrylate (PA) (stable flex) and 60 μm carbowax-polyethylene glycol (PEG), purchased from Supelco (Bellefonte, PA, USA).
B. Sampling
Urine samples were collected in the morning (before breakfast) from healthy children (5–13 years old; 50% girls) as well as children with CD (4–14 years old; 78% girls), 9 samples from each group. Approximately 30 mL of morning urine (second spontaneous urine) was collected. The samples were immediately divided into aliquots of 4 mL and stored at −80 °C until further analysis. All procedures involving human participants were in accordance with the Bioethics Committee of the Faculty of Medical Sciences of the University of Warmia and Mazury in Olsztyn (agreement no. 23/2015 of 16 June 2015) and with the 1964 Helsinki declaration and its later amendments or comparable ethical standards. Informed consent was obtained from all individual participants included in the study.
C. Preparation of calibration solutions and SPME fibers
Standard stock solutions were prepared by dissolving 1 μL of each reference standard (3 μL for 2,3-butanedione; 2-butanone) in MilliQ water in a 50 mL measuring flask. The standard working solutions were obtained by diluting the stock solutions. 4-Methyl-2-pentanol was used as an internal standard (196.24 nmol L−1). All fibers were conditioned prior to analysis as per the manufacturer's recommendations.
D. SPME optimization
Qualitative variables such as the type of SPME fiber and mode of extraction including headspace (HS) and direct immersion (DI) were investigated. Seven fibers comprising PA, PDMS100, PDMS7, CAR/PDMS, PDMS/DVB, DVB/CAR/PDMS and PEG were tested for the selected biomarkers. Prior to the extractions, samples were incubated for 20 min at 50 °C with a shaking speed of 500 rpm using MultiTherm shaker (Benchmark Scientific, Edison, USA). The extractions were then performed manually by inserting the fiber into the sample vials and exposing it for 20 min. HS and DI experiments were performed using 4 mL and 9 mL of sample respectively, in 20 mL vials sealed with metal caps. 4 mL of sample was used in HS mode as reported by Mills et al.24 In DI mode, 9 mL of sample was used to overcome practical limitations of submerging fiber into smaller samples. The values were normalized for comparison. After extractions, the fibers were introduced into the inlet of a gas chromatograph and the biomarkers were thermally desorbed for 10 min at 240 °C in a splitless mode.25 The time of desorption was selected based on preliminary results obtained to avoid carryover. Screening experiments were performed in duplicates. The most suitable fiber coating and extraction mode were selected to optimize peak areas of individual compounds. Factors such as the amount of sample and headspace volume (4 mL of sample in 20 mL vial) were kept constant as described elsewhere.25,26
A DoE using MODDE 10.1.1 software (Umetrics, Umeå, Sweden) was employed to study interactions between different quantitative variable i.e. pH, ionic strength, extraction time and temperature. A quadratic central composite faced (CCF) design including 27 experiments with 3 center points was used to optimize the variables. The lower and upper ranges of pH were maintained by the addition of 100 μL of 6 M hydrochloric acid and 100 μL of 6 M sodium hydroxide into 4 mL of the sample, respectively. The experimental ranges of ionic strength were between 0–4 g of sodium chloride added to the sample, extraction time between 15–60 min and temperature between 30–60 °C. In CCF, as a standard procedure, the variables were optimized using the lower limits, the middle points and the upper limits of the experimental ranges.
E. Gas chromatography-mass spectrometry
Urinary biomarkers were analyzed using a HP 5972 mass selective detector coupled with a HP 5890 gas chromatograph (Agilent Technologies, Santa Clara, USA). The compounds were separated using a Zebron ZB-624 capillary column, 60 m × 0.25 mm × 1.40 μm, (Phenomenex, Torrance, United States of America). A 0.75 mm ID splitless glass liner (Supelco, Bellefonte, PA) was used with an inlet temperature of 240 °C. The oven temperature program was 40 °C for 2 min then it was increased to 220 °C at a rate of 5 °C min and maintained for 5 min with a total run time of 42 min.25 The carrier gas was helium at a constant flow rate of 1 mL min−1. A solvent delay was set for 5 min. Mass spectra were obtained by electron ionization (EI) in the range of 40–550 m/z. Transfer line temperature, ion source temperature and quadrupole temperature were 240 °C, 230 °C, and 180 °C respectively. Total ion chromatograms were analyzed with the MSD ChemStation E.02.02.1431 software (Agilent Technologies, Santa Clara, USA). Identification of compounds was performed by comparison of the retention times and m/z. Quantification of compounds was done by external standard calibration.
F. Statistical analyses
Real sample analysis was performed in duplicates. The normality of the quantitative variables was tested by the Shapiro–Wilk test. As the data showed non-normal distribution, quantitative variables are expressed as a median value (P25–P75). Differences in the level of individual VOCs between healthy children and children with CD were tested with the non-parametric Mann–Whitney U test. Results were considered statistically significant at the 5% critical level (p < 0.05). The analyses were performed using the Statistica 10.0 software (StatSoft, Tulsa, USA).
Partial least squares-discriminant analysis (PLS-DA) was performed to evaluate separation between healthy and CD children, considering the urinary VOC data. The analysis was performed using the XLSTAT statistical software for Microsoft Excel.
3. Results and discussion
A. Screening experiments
Screening experiments showed that the CAR/PDMS fiber was the most suitable in both HS and DI extraction mode. The fiber yielded the highest response for all candidate biomarkers than other fiber types (Fig. 1). The findings confirm the ability for CAR/PDMS fibers in extracting VOCs such as alcohols, sulfur-containing compounds, carbonyl group-containing organic compounds and other volatile compounds from challenging matrices such as urine. There are reports using CAR/PDMS fiber for the analysis of VOCs with small molecular masses27 in urine samples for the profiling of potential urinary cancer biomarkers,7 VOCs in the urine of elderly men28 and the human metabolome.19
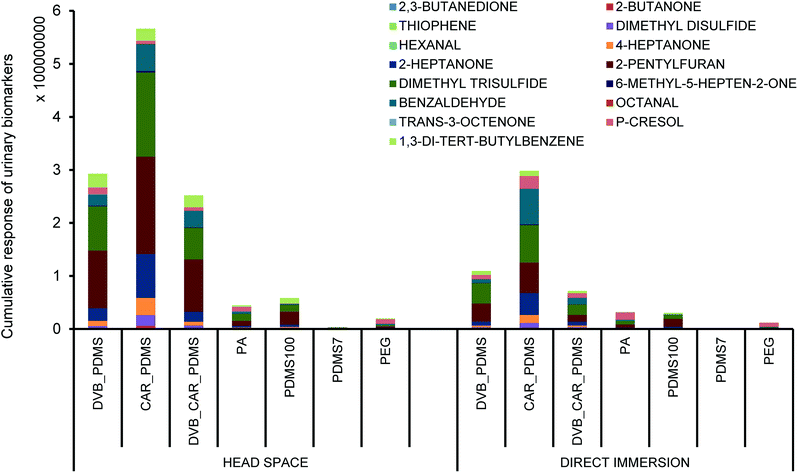 |
| Fig. 1 Comparison of extraction performance of different SPME fibers for selected urinary biomarkers. | |
A higher response to selected analytes was obtained by HS mode compared to DI for the majority of the fibers (Fig. 1). HS mode is commonly used for complex liquid and solid matrixes to overcome complications caused by dirty samples and to reduce sampling time.29 On the other hand, DI mode is preferred for the extraction of less volatile and more polar compounds.22 Another disadvantage of DI mode is that a significantly higher sample volume is required for complete immersion of the fiber in the sample. Hence, CAR/PDMS fiber in HS mode was selected for further optimization of the extraction parameters.
B. Method optimization
Central Composite Faced (CCF) design was used to optimize four quantitative variables i.e. extraction time, temperature, ionic strength and pH. The range of factors was selected based on the literature.26,30 A total explained variance of 90% [R2(Y) = 0.9] and a cross-validated predictability of 48% [Q2(Y) = 0.48] was observed in the optimized CCF model. The normalized influence of variables including extraction time, temperature, amount of salt and pH is shown in Fig. 2.
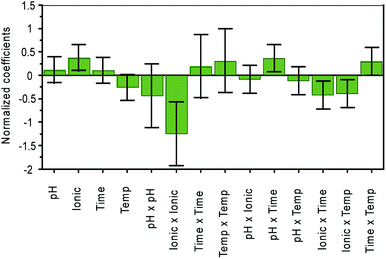 |
| Fig. 2 Normalized influence of variables on the extraction of the urinary biomarkers. Ionic = ionic strength; temp = temperature. | |
The ionic strength was found to be the most influential variable on extraction efficiency. Depending on the nature of the compound, the addition of salt can influence the extraction efficiency both positively and negatively as presented by Risticevic et al.22 The addition of salt facilitates the transfer of the molecules from the sample into the HS and ultimately to the fiber surface, increasing the overall sensitivity. However, too high ionic strength may result in electrostatic interactions of the compound with the salt molecules, reducing the ability of the metabolite to pass into the HS.27 In the present study, the type of salt was not investigated, and sodium chloride was used since it is the most commonly used salt in earlier studies on urine.7,25,26,30 In our study, 2.98 g of salt per 4 mL of urine sample was found to provide the optimum ionic strength.
It was found that pH had no significant effect on extraction efficiency (Fig. 2). The compounds analyzed by SPME can only adsorb onto the fiber surface from HS if they are present in a neutral form; in the selected matrix not all compounds are present in the neutral form. To achieve the conversion of molecules into the neutral form, the pH was adjusted.22 The extraction of acidic compounds is improved at a lower pH, whilst a high pH improves the extractability of basic compounds. Based on the results obtained by CCF, the pH was adjusted by adding 21 μL of 6 M hydrochloric acid.
Surprisingly, extraction time and temperature were found to be less significant factors than ionic strength, contrary to what was expected from the literature.16 Fig. 2 shows a normalized coefficient plot with the relative influence of different variables on extraction efficiency. A temperature of 30 °C was found to be the most suitable for the selected VOCs. The most suitable extraction time was 15 minutes, which could be explained by the volatile nature of the biomarkers.
Finally, the optimal conditions for the extraction and quantification of selected urinary biomarkers of CD were a temperature of 30 °C, and an extraction time of 15 min with the addition of 2.98 g of sodium chloride and 21 μL of 6 M hydrochloric acid in 4 mL of sample.
C. Analytical performance
The method was evaluated for inter and intraday precision, limits of detection (LOD), limits of quantification (LOQ) and linearity of the method for all 15 biomarkers. Furthermore, all the analyses were performed in triplicate and the peak areas of standards were normalized relative to the peak area of the internal standard. The parameters of method performance for each selected VOC using the developed method are presented in Table 2.
Table 2 Validation parameters calculated from a mixture of commercial standards. Compounds are ordered with respect to their increasing retention times
Compound |
R2 |
Linear range [nmol L−1] |
LODa [nmol L−1] |
LOQb [nmol L−1] |
Intraday precision [RSD%] |
Interday precision [RSD%] |
Limit of detection. Limit of quantification. |
2,3-Butanedione |
0.993 |
2671.6–170927.4 |
5.2 |
17.4 |
3.8 |
6.1 |
2-Butanone |
0.995 |
3494.7–223270.0 |
0.21 |
0.71 |
3.3 |
6.8 |
Thiophene |
0.973 |
487.3–31233.7 |
0.03 |
0.10 |
2.3 |
5.3 |
Dimethyl disulfide |
0.992 |
435.3–27763.0 |
0.03 |
0.09 |
1.3 |
4.5 |
Hexanal |
0.985 |
639.0–40684.9 |
0.04 |
0.13 |
2.0 |
3.5 |
4-Heptanone |
0.970 |
280.2–17891.5 |
0.02 |
0.06 |
2.1 |
2.7 |
2-Heptanone |
0.971 |
280.2–17952.9 |
0.02 |
0.06 |
1.9 |
2.4 |
2-Pentylfuran |
0.964 |
2004.2–32052.7 |
15.7 |
52.17 |
4.6 |
4.7 |
Dimethyl trisulfide |
0.950 |
744.6–11905.0 |
46.5 |
155.0 |
4.5 |
2.3 |
6-Methyl-5-hepten-2-one |
0.979 |
7.92–340.73 |
0.17 |
0.55 |
2.0 |
4.6 |
Benzaldehyde |
0.955 |
188.5–2949.4 |
0.09 |
0.30 |
3.8 |
2.4 |
Octanal |
0.999 |
241.8–967.1 |
0.0005 |
0.0015 |
3.3 |
2.6 |
trans-3-Octen-2-one |
0.952 |
23.8–0.340.7 |
2.7 |
8.8 |
2.9 |
1.9 |
p-Cresol |
0.961 |
1239.1–19835.4 |
155.0 |
516.5 |
6.0 |
6.1 |
1,3-Di-tert-butylbenzene |
0.979 |
0.28–2.3 |
0.14 |
0.47 |
8.0 |
16.0 |
Method linearity was determined by evaluating the regression curves of standard solutions and expressed by the squared determination coefficient R2. The method was found to have a linear response (R2 in a range of 0.950–0.999) for all the selected VOCs, their ranges are given in Table 2.
Interday precision was calculated by analyzing a batch of six standard mixtures. Intraday precision was determined by analyzing another batch of six standard mixtures the following day. Precision was expressed as relative standard deviation (RSD). In general, except for 1,3-di-tert-butylbenzene, the intraday precision was below 5% for the rest of the analytes, while interday precision ranged from 1.9 to 6.8% RSD, which was found as satisfactory.
LOD and LOQ were calculated by analyzing the signal-to-noise ratio (S/N). The LOD values were found to be <0.3 nmol L−1 for all the biomarkers except 2,3-butanedione, 2-pentylfuran, dimethyl trisulfide, trans-3-octen-2-one and p-cresol. Upon comparison with available methods, the optimized SPME method was found to be more sensitive for some of the candidate biomarkers analyzed by already available methods. The LOD for hexanal analyzed by HS combined with GCMS was 0.60 nmol L−1 (ref. 9) (in our study 0.04 nmol L−1). In another study using SPME with an additional derivatization step, the LOD for hexanal was 2.17 nmol L−1.16 In one more quantitative study optimizing SPME with focus on hexanal and heptanal, the LOD for hexanal was 1.10 nmol L−1.8 Hollow-fiber liquid-phase microextraction followed by capillary electrophoresis,31 and magnetic solid-phase extraction followed by high performance liquid chromatography32 were used for quantitative analysis of hexanal and heptanal, and the obtained LOD was 2.7 nmol L−1 and 1.87 nmol L−1, respectively, proving that among all the presenting methods, the one presented in this study was the most sensitive. Similarly, in our study LOD for octanal, benzaldehyde, 2-butanone and 4-heptanone were much lower than that presented by Calejo et al.16 Compared to LOD obtained by Mochalski et al.,6 a slightly lower LOD was obtained in our study for 2-butanone, 4-heptanone and 2-heptanone. The lowest sensitivity in our study was obtained for p-cresol. However, this is still a satisfactory result compared to high-performance liquid chromatography methods, electrochemical microneedles and fluorescence spectroscopy, as summarized by Usha & Gupta.33 The same authors reported a sensor with a layer of a nanocomposite of zinc oxide and molybdenum sulfide, which obtained LOD of 28 nmol L−1 for p-cresol.33
D. Method application
To prove the applicability of the method to the real matrix of human urine, the developed protocol was applied for the determination and quantification of target analytes in urine samples of healthy children and children with CD. The results revealed that the optimized method was applicable to the quantification of biomarkers in urine samples from both healthy children and children with CD. To prove the concept that the urinary profile of VOCs can be used to discriminate between CD and healthy children, a partial least squares-discriminant analysis (PLS-DA) was applied. A score scatter plot was obtained for CD (N = 9) and healthy children (N = 9) (Fig. 3a). Even though a limited number of samples were analyzed, the PLS-DA score scatter plot showed a distinction between healthy children (red triangles) and children with CD (blue dots). Samples from CD children were present mainly in 1 quadrant, while samples from healthy children showed a spread in quadrants 2, 3 and 4 (Fig. 3a). The distribution of healthy children can be explained by a more diverse diet compared to a gluten-free diet followed by children with CD. Variable Importance in the Projection (VIP) scores were measured to summarize the contribution each variable makes to the model (Fig. 3b). The higher value of the VIP score, the greater the contribution to the group separation. Based on VIP scores, 1,3-di-tert-butylbenzene, 2,3-butanedione, 2-heptanone, dimethyl disulfide and octanal were found to be discriminative compounds for CD vs. healthy children (VIP score above 1). Also, 4-heptanone, 2-butanone and hexanal have important contributions in the separation (VIP score above 0.83).
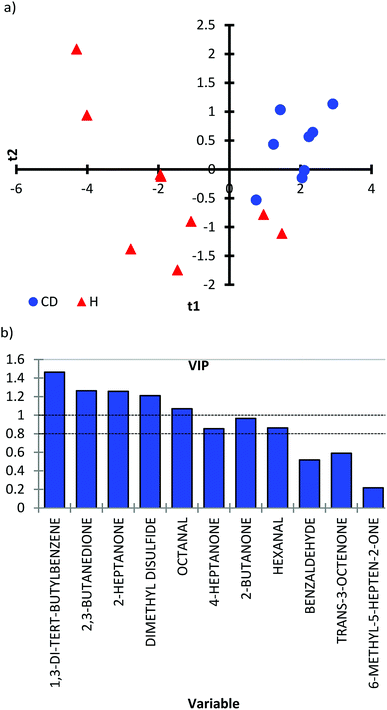 |
| Fig. 3 (a) PLS-DA scores plot (LV2, R2X = 0.491; R2Y = 0.609; Q2 = 0.395) obtained for target analysis between healthy (red triangles) and CD children (blue circles). (b) Variable importance in projection (VIP) plots for partial least squares analysis. A VIP value larger than 0.83 indicates the contribution of VOC in separating the groups (above the line). | |
The concentration of the analyzed VOCs and the results from the non-parametric Mann–Whitney test are presented in Table 3. Thiophene was detected in only one child with CD, whilst the concentrations of 2-pentylfuran, dimethyl trisulfide and p-cresol were below the LOQ. 1,3-Di-tert-butylbenzene was only found in children with CD, while trans-3-octen-2-one was observed in quantitative amounts only in healthy children. The concentrations of VOCs with high VIP scores were significantly different (p < 0.05) between healthy and CD children. The concentration of 2,3-butanedione, 2-butanone, dimethyl disulfide, hexanal, 4-heptanone, 2-heptanone and octanal was much higher in healthy children compared to CD children. It can be explained by the gut microbiota dysbiosis observed in CD, as many VOCs are produced during colonic fermentation.15
Table 3 Concentration (nmol L−1) of selected VOCs in urine of healthy and CD children to distinguish between the two groups
Compound |
Healthy children |
Celiac disease children |
p-Value |
Median |
P25 |
P75 |
Median |
P25 |
P75 |
ND – not detected. NQ – not quantified, below LOQ. |
2.3-Butanedione |
5253.6 |
2131.2 |
7760.9 |
251.9 |
191.3 |
361.0 |
0.0108 |
2-Butanone |
5539.2 |
3449.2 |
11270.7 |
801.7 |
663.8 |
2079.1 |
0.0141 |
Thiophene |
NDa |
|
|
ND |
|
|
|
Dimethyl disulfide |
386.5 |
232.1 |
530.7 |
96.0 |
73.3 |
154.0 |
0.0108 |
Hexanal |
1425.8 |
785.2 |
2131.0 |
179.1 |
167.6 |
229.2 |
0.0184 |
4-Heptanone |
712.7 |
338.8 |
1600.2 |
155.1 |
99.8 |
286.9 |
0.0237 |
2-Heptanone |
67.5 |
39.5 |
99.0 |
7.9 |
4.4 |
9.5 |
0.0108 |
2-Pentylfuran |
NQb |
|
|
NQ |
|
|
|
Dimethyl trisulfide |
NQ |
|
|
NQ |
|
|
|
6-Methyl-5-hepten-2-one |
9.1 |
7.3 |
16.2 |
15.4 |
15.0 |
17.6 |
0.1629 |
Benzaldehyde |
546.0 |
440.1 |
645.5 |
145.8 |
99.0 |
332.9 |
0.0485 |
Octanal |
163.9 |
91.8 |
221.7 |
15.4 |
12.7 |
20.5 |
0.0024 |
trans-3-Octen-2-one |
12.2 |
0.000 |
24.5 |
NQ |
|
|
0.1358 |
p-Cresol |
NQ |
|
|
NQ |
|
|
|
1,3-Di-tert-butylbenzene |
ND |
|
|
0.54 |
NQ |
0.87 |
0.0029 |
Based on the performed analysis, it is suggested that 1,3-di-tert-butylbenzene, 2,3-butanedione, 2-butanone, dimethyl disulfide, hexanal, 4-heptanone, 2-heptanone and octanal can be among the most significant biomarkers of CD.
4. Conclusions
In the presented work, optimization of a protocol for the quantitative analysis of 15 selected urinary biomarkers of CD, representing different chemical classes, was performed. This methodology proved to be simple, rapid and convenient to be included in the routine analysis of urine samples. The statistical experimental design was successfully applied to optimize the extraction parameters and study the most significant factors and interactions between them. When the method was applied to urine samples from healthy children and children with CD, it was demonstrated that the proposed method could distinguish the two groups of children based on the profile of urinary biomarkers. The potential of the method could be further explored by studying a larger group of participants to establish diagnostic protocols for CD. Moreover, the optimized method will be used to evaluate the effect of gluten-free diet modification on the profile of VOCs in urine of children with CD.
Authors contributions
ND conceived and designed the research. ND secured funding and administrated the project. HAA performed the statistical experimental design step. ND conducted all analytical experiments. UKK provided urine samples from children with CD. ND collected samples from healthy volunteers. ND and HAA analyzed data and interpreted results. ND wrote the draft of the manuscript. HAA contributed to the further writing of the manuscript. All authors read and approved the final version of the manuscript.
Conflicts of interest
There are no conflicts to declare.
Acknowledgements
The research was supported by the Polish National Science Centre through project PRELUDIUM 11 (project number: 2016/21/N/NZ9/01510).
References
- M. M. Leonard, A. Sapone, C. Catassi and A. Fasano, J. Am. Med. Assoc., 2017, 318, 647–656 CrossRef PubMed.
- F. Benkebil, C. Combescure, S. I. Anghel, C. Besson Duvanel and M. G. Schäppi, World J. Gastroenterol., 2013, 19, 5111–5117 CrossRef PubMed.
- T. Majchrzak, W. Wojnowski, G. Piotrowicz, J. Gębicki and J. Namieśnik, TrAC, Trends Anal. Chem., 2018, 108, 38–49 CrossRef.
- C. J. S. Probert, I. Ahmed, T. Khalid, E. Johnson, S. Smith and N. Ratcliffe, J. Gastrointestin. Liver Dis., 2009, 18, 337–343 Search PubMed.
- F. Buljubasic and G. Buchbauer, Flavour Fragrance J., 2015, 30, 5–25 CrossRef.
- P. Mochalski and K. Unterkofler, Analyst, 2016, 141, 4796–4803 RSC.
- C. L. Silva, M. Passos and J. S. Cmara, Br. J. Cancer, 2011, 105, 1894–1904 CrossRef PubMed.
- R. Guadagni, N. Miraglia, A. Simonelli, A. Silvestre, M. Lamberti, D. Feola, A. Acampora and N. Sannolo, Anal. Chim. Acta, 2011, 701, 29–36 CrossRef PubMed.
- A. P. Antón, A. M. C. Ferreira, C. G. Pinto, B. M. Cordero and J. L. P. Pavón, J. Chromatogr. A, 2014, 1367, 9–15 CrossRef PubMed.
- R. P. Arasaradnam, N. Ouaret, M. G. Thomas, N. Quraishi, E. Heatherington, C. U. Nwokolo, K. D. Bardhan and J. A. Covington, Inflammatory Bowel Dis., 2013, 19, 999–1003 CrossRef PubMed.
- R. B. M. Aggio, B. De Lacy Costello, P. White, T. Khalid, N. M. Ratcliffe, R. Persad and C. S. J. Probert, J. Breath Res., 2016, 10, 017106 CrossRef PubMed.
- N. D. McGuire, R. J. Ewen, B. De Lacy Costello, C. E. Garner, C. S. J. Probert, K. Vaughan and N. M. Ratcliffe, Meas. Sci. Technol., 2014, 25, 065108 CrossRef PubMed.
- R. Di Cagno, M. De Angelis, I. De Pasquale, M. Ndagijimana, P. Vernocchi, P. Ricciuti, F. Gagliardi, L. Laghi, C. Crecchio, M. Guerzoni, M. Gobbetti and R. Francavilla, BMC Microbiol., 2011, 11, 219 CrossRef PubMed.
- R. Di Cagno, C. G. Rizzello, F. Gagliardi, P. Ricciuti, M. Ndagijimana, R. Francavilla, M. E. Guerzoni, C. Crecchio, M. Gobbetti and M. De Angelis, Appl. Environ. Microbiol., 2009, 75, 3963–3971 CrossRef PubMed.
- R. P. Arasaradnam, E. Westenbrink, M. J. McFarlane, R. Harbord, S. Chambers, N. O'Connell, C. Bailey, C. U. Nwokolo, K. D. Bardhan, R. Savage and J. A. Covington, PLoS One, 2014, 9(10), e107312 CrossRef PubMed.
- I. Calejo, N. Moreira, A. M. Araújo, M. Carvalho, M. De Lourdes Bastos and P. G. De Pinho, Talanta, 2016, 148, 486–493 CrossRef PubMed.
- M. Kusano, E. Mendez and K. G. Furton, Anal. Bioanal. Chem., 2011, 400, 1817–1826 CrossRef PubMed.
- C. M. Weber, M. Cauchi, M. Patel, C. Bessant, C. Turner, L. E. Britton and C. M. Willis, Analyst, 2011, 136, 359–364 RSC.
- S. Bouatra, F. Aziat, R. Mandal, A. C. Guo, M. R. Wilson, C. Knox, T. C. Bjorndahl, R. Krishnamurthy, F. Saleem, P. Liu, Z. T. Dame, J. Poelzer, J. Huynh, F. S. Yallou, N. Psychogios, E. Dong, R. Bogumil, C. Roehring and D. S. Wishart, PLoS One, 2013, 8, e73076 CrossRef PubMed.
- A. Pysanenko, T. Wang, P. Španěl and D. Smith, Rapid Commun. Mass Spectrom., 2009, 23, 1097–1104 CrossRef PubMed.
- A. Naccarato, E. Gionfriddo, R. Elliani, G. Sindona and A. Tagarelli, J. Chromatogr. A, 2014, 1372, 253–259 CrossRef PubMed.
- S. Risticevic, H. Lord, T. Górecki, C. L. Arthur and J. Pawliszyn, Nat. Protoc., 2010, 5, 122–139 CrossRef PubMed.
- B. d'Acampora Zellner, C. Bicchi, P. Dugo, P. Rubiolo, G. Dugo and L. Mondello, Flavour Fragrance J., 2008, 23, 297–314 CrossRef.
- G. A. Mills and V. Walker, J. Chromatogr. B: Biomed. Sci. Appl., 2001, 753, 259–268 CrossRef.
- R. B. M. Aggio, A. Mayor, S. Coyle, S. Reade, T. Khalid, N. M. Ratcliffe and C. S. J. Probert, Chem. Cent. J., 2016, 10, 9 CrossRef PubMed.
- R. Cozzolino, L. De Magistris, P. Saggese, M. Stocchero, A. Martignetti, M. Di Stasio, A. Malorni, R. Marotta, F. Boscaino and L. Malorni, Anal. Bioanal. Chem., 2014, 406, 4649–4662 CrossRef CAS PubMed.
- K. Schmidt and I. Podmore, J. Mol. Biomarkers Diagn., 2015, 6, 253 Search PubMed.
- S. Smith, H. Burden, R. Persad, K. Whittington, B. De Lacy Costello, N. M. Ratcliffe and C. S. Probert, J. Breath Res., 2008, 2, 037022 CrossRef CAS PubMed.
- R. Shirley, SPME/GC Analyses of Sulfur Gases and VOCs, Using a New Carboxen/PDMS Fiber, 1999, vol. 16 Search PubMed.
- M. Monteiro, M. Carvalho, R. Henrique, C. Jerónimo, N. Moreira, M. De Lourdes Bastos and P. G. De Pinho, Eur. J. Cancer, 2014, 50, 1993–2002 CrossRef CAS PubMed.
- F. Chen, C. Wang, M. Zhang, X. Zhang, Y. Liu, J. Ye and Q. Chu, Talanta, 2014, 119, 83–89 CrossRef CAS PubMed.
- D. Song, Y. Gu, L. Liang, Z. Ai, L. Zhang and H. Xu, Anal. Methods, 2011, 3, 1418–1423 RSC.
- S. P. Usha and B. D. Gupta, Biosens. Bioelectron., 2018, 101, 135–145 CrossRef CAS PubMed.
|
This journal is © The Royal Society of Chemistry 2018 |
Click here to see how this site uses Cookies. View our privacy policy here.