DOI:
10.1039/C9RA03141C
(Paper)
RSC Adv., 2019,
9, 24722-24732
Application of a definitive screening design for the synthesis of a charge-transfer complex of sparfloxacin with tetracyanoethylene: spectroscopic, thermodynamic, kinetics, and DFT computational studies
Received
27th April 2019
, Accepted 19th July 2019
First published on 8th August 2019
Abstract
Herein, a spectrochemical approach was adopted to study the charge-transfer (CT) complexation of sparfloxacin (SFX) with tetracyanoethylene (TCNE). In this study, a three-level design of experiments (DOE) involving a definitive screening design (DSD) was implemented. This is the first effort to operate this new category of design to determine a pharmaceutical compound in its pure form and in formulations. The proposed design allowed the establishment of a regression model that described the relation between the factorial input and the response surface. Moreover, two charge-transfer states (CTSs) were observed at 390 and 464 nm. The DFT calculations conducted using B3LYP/6-31+G showed that SFX had several donation sites (donor, D), whereas TCNE had two acceptor (A) sites. The two states were influenced differently by the experimental conditions as per the findings of the DSD analysis. In general, the diluting solvent had the largest impact. Probability plots, histograms, individual value plots, residual plots as well as analysis of variance (ANOVA) were delineated at the 95.0% confidence interval (CI). A Job's plot showed that a 1
:
1 complex was formed. The results were further confirmed using Benesi–Hildebrand plots. The proposed approach was proved to be linear in the range of 10–90 μg mL−1 SFX when the absorbance was measured at 464 nm. Different set-ups were adopted for studying the reaction kinetics. Analytical method performance was assessed following the ICH guiding principles, and the results obtained were found to be satisfactory. Complex formation was found to be an exothermic reaction.
1 Introduction
Sparfloxacin (SFX), chemically known as [5-amino-1-cyclo-propyl-7-(cis-3,5-dimethyl-piperazin-1-yl)-6,8-di-fluoro-1,4-dihydro-4-oxoquinoline-3-carboxylicacid], is an antibiotic that belongs to the quinolone family with the chemical formula C19H22F2N4O3 (Scheme 1). The spectrum of the antibacterial activity of SFX includes salmonella, staphylococcus and chlamydia. SFX is therefore used to treat different sorts of bacterial diseases such as community-acquired lower respiratory tract infections. The effect on susceptible bacterial strains would be attributed to the capability of SFX to inhibit DNA gyrase, the enzyme controlling the DNA topology and replication.1–4
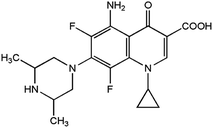 |
| Scheme 1 Chemical structure of sparfloxacin (SFX). | |
Having a questionable safety profile, the usage of SFX has been prohibited in many countries. Controlled clinical trials have shown that SFX may be responsible for elongated cardiac QTc interval, especially in elderly patients administered with SFX and having pre-existing rhythm ailments. Other side effects include phototoxicity (face and hand erythema), insomnia and other sleep disorders.5,6 Having a protracted half-life and hence good bioavailability, SFX is an effective antibiotic that is marketed as an OTC drug, especially in the Middle East region.
Various techniques for the determination of SFX concentration as such and in formulations exist in the literature. Some reported methods include high-performance liquid chromatography (HPLC),7,8 capillary electrophoresis (CE),9,10 polarography,11 enzyme-linked immunosorbent assay (ELISA),12 fluorescent molecularly imprinted ordered mesoporous microspheres (quantum dots, QDs)13 and spectrophotometry.14–16 However, it is important to point out that many of the published techniques for the determination of SFX, especially those applying chromatography as an approach, require a proficient operator to perform them, in addition to an expensive instrumental set-up and long time consumption. Moreover, to the best of our knowledge, many of the reported methods, if not all, are univariate based (UVA), an issue that adds to the cons of these techniques in terms of the time, resource and effort consumption.
Definitive screening design (DSD), a novel 3-level multivariate analysis approach (MVA), was used for screening the investigated variables. Contrary to the traditional UVA approach, the MVA studies the influence of the detached factors while reducing the effort and resources required by executing the lowest possible number of experimentations. In general, DSD is used when the number of factors (k) is four or more and when assuming the lowest possible number of categorical variables. By and large, the usual sequence for instigating MVA through a DOE set-up requires two subsequent steps: screening and optimization. Yet, a DSD combines both phases in a single step, and is therefore more effective compared to the standard screening designs, such as fractional factorial (Fr-FD) or Plackett–Burman (PBD) designs, for detecting non-linear interactions. The number of runs for DSD is only N = 2k + 1 or 2k + 3, for both an even and odd number of factors, respectively.17–19
Charge-transfer complexes (CTCs) are complexes formed between two or more molecules, where the electronic charge is transferred between the two species. The source of the transferred charge is an electron donor (D) and the received charge goes accordingly to an electron acceptor (A). Therefore, new charge-transfer (CT) electronic states are developed due to this charge migration. These states can be detected spectroscopically, and even visually in some cases. CTCs have become the target of considerable studies due to their various biological and physical properties.20–23 Based on our previous research and preliminary studies,22,24 several factors, both numerical and categorical, are known to influence the CT reaction, including the reaction time (RT), reaction temperature (temp), reagent volume (RV) and the diluting solvent (DS).
The aim of the current approach was to determine SFX in its pure form and in formulations via a facile, sensitive, rugged and trustworthy approach that could be used for the routine analysis of SFX in quality control laboratories. A smart approach involving a DSD-CTC-based spectrochemical method could be executed to achieve such an objective. Optimum conditions that could maximize the response (absorbance) were obtained through the composite desirability function (D). To the best of our knowledge, this is the first effort to use DSD for pharmaceuticals. Moreover, the interaction of SFX with TCNE and the electron-density distribution for the donor (SFX) and the acceptor (TCNE) were determined via high level quantum mechanical calculations using the density functional theory (DFT) at the B3LYP level of calculation and the 6-31+G basis set. The rate and order of the reaction were determined, and a thermodynamic study was performed by conducting the reaction at different temperatures and by applying Benesi–Hildebrand as a maneuver. The developed method was validated following the ICH guidelines.25
2 Experimental
2.1. Instrumentation
A UV-Vis spectrophotometer (Agilent 8453, USA) with 10 mm matched quartz cells was used to measure the absorbance of the samples. A thermostatically controlled water bath was used to heat the samples to the temperature needed.
2.2. Materials
All the chemicals used were of analytical grade. The candidate donor sparfloxacin (SFX, 98.0%, HPLC, Batch# BCBN3519V), acceptor tetracyanoethylene (TCNE, 98%, Batch# S84306), SiO2 as silica gel (99%, Batch# 11615CH) and TiO2 (99.9%, Batch# 1317-70-0) were purchased from Sigma-Aldrich, USA. Cellulose (Batch# 33820) was purchased from Riedel-de Haen, USA. Acetonitrile (98%, GLC, BDH, 2 Batch# 9220, USA) and methanol (99.8%, Merck, Batch# I838218633, USA) were used as received. All the materials used were with a stated purity of >98% so were used without further purification.
2.3. Reagents and standards
Working stock solutions of SFX (0.1%, 2.58 mM, and 2.50 mM) and TCNE (0.1%, 2.50 mM and 0.50 mM) were prepared by weighing the requisite amounts of SFX and TCNE powders followed by dilution to the mark with acetonitrile. Both the drug and reagent stock solutions were freshly prepared daily.
2.4. General procedure
2.4.1. Spectroscopic studies.
2.4.1.1 Authentic samples: design of experiments (DOE). Minitab®18 software was used to compose the DSD matrix. The software was purchased from Minitab® (Minitab Inc., State College, Pennsylvania, USA). The list of investigated variables as well as their levels is shown in Table 1. Fourteen runs (with 2 added central points) were performed. Aliquots of the 0.1% drug stock solution containing 300 μg mL−1 were transferred into a series of 10 mL volumetric flasks, followed by the appropriate volume of 0.1% TCNE. The volume was then made up to the mark with an appropriate amount of diluting solvent. The reagent volume in each of the 14 volumetric flasks, type of diluting solvent and temp at which reaction will occur were varied based on the detailed experimental set-up shown in the design table, Table 2. All the measurements were made against a reagent blank similarly prepared. The absorbance of the resulting yellow coloured solution was measured at the 2 CT states (CTSs: λmax = 390 nm and 464 nm). To construct the calibration curve, different volumes of stock solutions of the SFX were used in the range of 10–90 μg mL−1. The optimum conditions obtained following the analysis of data were applied and the obtained solutions were measured at a λmax of 464 nm.
Table 1 Screened numerical and categorical variables using DSD for the proposed CT reaction
Screened factors |
Symbol |
Minimum (−1) |
Medium (0) |
Maximum (+) |
Numerical variables |
Temperature (temp, °C) |
A |
25 |
42.5 |
60 |
Reagent volume (RV, mL) |
B |
1 |
3 |
5 |
Reaction time (RT, min) |
C |
0 |
20 |
40 |
![[thin space (1/6-em)]](https://www.rsc.org/images/entities/char_2009.gif) |
Categorical variables |
Diluting solvent (DS) |
D |
Methanol |
Acetonitrile |
![[thin space (1/6-em)]](https://www.rsc.org/images/entities/char_2009.gif) |
Dependent variables |
Absorbance at 390 nm |
YCTS1 |
Absorbance at 464 nm |
YCTS2 |
Table 2 Screened factors (coded and uncoded) and the obtained responses
Run order |
Pattern of coded variables |
Uncoded independent variables |
Dependent variables |
Aa (°C) |
Bb (mL) |
Cc (min) |
Dd |
YCTS1 Obs.e |
YCTS1 Pred.f |
YCTS2 Obs.e |
YCTS2 Pred.f |
A is the temperature (temp, °C). B is the reagent volume (RV, mL). C is the reaction time (RT, min). D is the diluting solvent (DS). Obs.: experimental values. Pred.: predicted values after response transformation. |
01 |
+−−+ |
60 |
1.0 |
0 |
Acetonitrile |
0.792 |
0.790 |
0.779 |
0.792 |
02 |
−−++ |
25 |
1.0 |
40 |
Acetonitrile |
0.223 |
0.224 |
0.788 |
0.782 |
03 |
++0+ |
60 |
5.0 |
20 |
Acetonitrile |
0.934 |
0.938 |
0.225 |
0.356 |
04 |
−++− |
25 |
5.0 |
40 |
Methanol |
1.170 |
1.173 |
0.604 |
0.641 |
05 |
+0+− |
60 |
3.0 |
40 |
Methanol |
1.119 |
1.120 |
0.322 |
0.541 |
06 |
+−+− |
60 |
1.0 |
40 |
Methanol |
1.000 |
0.999 |
0.399 |
0.375 |
07 |
0+++ |
42.5 |
5.0 |
40 |
Acetonitrile |
0.656 |
0.649 |
0.373 |
0.356 |
08 |
−−0− |
25 |
1.0 |
20 |
Methanol |
1.040 |
1.036 |
0.463 |
0.375 |
09 |
++−− |
60 |
5.0 |
0 |
Methanol |
1.430 |
1.428 |
0.700 |
0.641 |
10 |
−0−+ |
25 |
3.0 |
0 |
Acetonitrile |
0.525 |
0.526 |
0.736 |
0.647 |
11 |
0−−− |
42.5 |
1.0 |
0 |
Methanol |
1.156 |
1.158 |
0.321 |
0.375 |
12 |
000+ |
42.5 |
3.0 |
20 |
Acetonitrile |
0.620 |
0.624 |
0.589 |
0.647 |
13 |
−+−+ |
25 |
5.0 |
0 |
Acetonitrile |
0.642 |
0.641 |
0.345 |
0.356 |
14 |
000− |
42.5 |
3.0 |
20 |
Methanol |
1.182 |
1.182 |
0.591 |
0.541 |
2.4.1.2 Procedure for the formulations. A lab-made formulation was synthesized using the formulation composition of Zagam® (200 mg SFX) tablets, which was marketed as white 200 mg round, white film-coated tablets. Each 200 mg tablet contained the following inactive ingredients: microcrystalline cellulose NF, corn starch NF, L-hydroxypropyl cellulose NF, magnesium stearate NF and colloidal silicone dioxide NF. The film coating contained: methyl hydroxypropyl cellulose USP, polyethylene glycol 6000 and titanium dioxide USP.26 Therefore, the synthetic formulation was made by mixing the followings: 0.01 g of each of colloidal SiO2, TiO2, cellulose and sucrose with 0.01 g SFX powder. Then, an appropriate amount of the mixture (equivalent to 0.01 g of SFX) was accurately weighed, transferred to a 10 mL volumetric flask, and dissolved in acetonitrile. The same procedure for the pure drug was followed for the formulation (direct calibration).
2.4.1.3 Procedure for the standard addition method. Five samples were prepared by adding 250 μL of the formulation to each test tube (labelled S0–S4). Then, different solutions of the drug were added in the range of 5–50 μg mL−1. Finally, the optimum conditions were applied, and the obtained solutions were measured at λmax = 464 nm.
2.4.1.4 Procedure for Job's method. Equimolar solutions (2.58 mM) of SFX and TCNE were used to investigate the molar ratio implementing Job's method with continuous variation as an approach. A set of solutions was arranged, where the total volume was kept at 5.0 mL using acetonitrile.27
2.4.2. Determination of the thermodynamic parameters. Samples were prepared in such a way that the [Drug] was at least five times more than that of [TCNE], [D] ≫ [A]. Five sets were prepared, and each set comprised five samples in 10 mL volumetric flasks. Different volumes (1–3 mL) of 2.5 mM solution of SFX were added followed by 1.0 mL of 0.5 mM of TCNE solution. The prepared solutions were heated in a water bath for 10 min at 25 °C, 40 °C, 55 °C, 70 °C and 85 °C. The absorbance was recorded against a reagent blank at 464 nm.
2.4.3. Procedure for the kinetics approach. Seven sets were prepared (A–G) having a final concentration of 5–80 μg mL−1 SFX. The absorbance of each set was measured at varied time intervals of 0, 5, 10, 20, 30, 45 and 60 min. The samples were then analysed as described under the general procedure.
2.4.4. Computational details. Molecular geometry optimization, the charge density and the energy of molecular orbits were calculated using density functional theory (DFT) or time-dependent (TD) DFT at the B3LYP level of functional and with the 6-31+G basis set within Gaussian-09W software.28 A Microsoft Windows 10 Enterprise X64-based PC, with 16 GB RAM and Intel® Core™ i7-7500U CPU @ 2.70 GHz, 2 cores processor was used.
3 Results and discussion
3.1. Charge-transfer (CT) reaction
The product of interaction of SFX and TCNE is a charge-transfer complex (CTC), as seen in Scheme 2. In general, and as represented in the electronic spectra shown in Fig. 1, two main CT states (CTSs) are formed. The magnitude of both CTSs is greatly dependent on the instigated experimental conditions, as will be shown later under the optimization phase. As shown in Fig. 1, the formed yellowish coloured CTC showed two main absorption maxima, λmax = 390 and 464 nm, with two shoulders at 342 and 365 nm. Comparing the obtained spectra of the CTC with the spectra of SFX and TCNE alone (in acetonitrile), it was shown that TCNE does not possess any absorption bands in this region; yet SFX has four absorption bands: 200, 223, 308 and 365 nm. These peaks might be attributed to π–π* transitions (medium and low) in the aromatic moiety, piperazine ring and C
O transitions, respectively.29–31 The formation of two new bands at 390 and 464 nm can be explained based on the formation of a donor–acceptor (D–A) complex, since neither SFX nor TCNE alone absorb in this region. It is imperative to mention that the findings reported herein show that these two peaks, representing two CTSs, increase and decrease simultaneously depending on the experimental conditions, unlike our previously reported CT of synephrine, where two types of complexes were formed.22 Quantum mechanical calculations using DFT-B3LYP and 6-31+G levels of calculation showed that for TCNE (acceptor), the electron-deficient centre (receiving centre) is the two carbon atoms located on the sides of the double bond, and not the strong electron-attracting cyanide groups, Fig. 2. For SFX (donor), and using the same level of calculations, several electron-rich donation sites exist, as shown in Fig. 3. This finding explains the complex UV-Vis absorption spectrum, shown in Fig. 1. Absorbance measurements were taken at the two main wavelengths to assist us gaining a more reliable comprehension of the reaction mechanism.
 |
| Scheme 2 Charge-transfer (CT) complex formation, D is SFX (donor) and A is TCNE (acceptor). | |
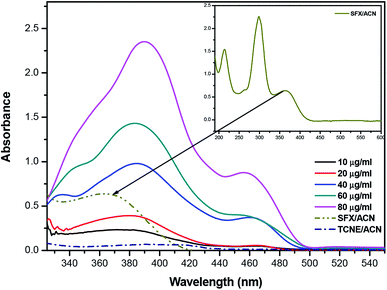 |
| Fig. 1 Absorption spectrum of the reaction product between SFX and TCNE at different concentration levels (10–90 μg mL−1). All the measurements were done against a reagent blank using the following conditions: temp 25 °C, RV 5.0 mL, RT 0 min and methanol as the diluting solvent. The inset shows the absorption spectrum of SFX in acetonitrile (ACN). | |
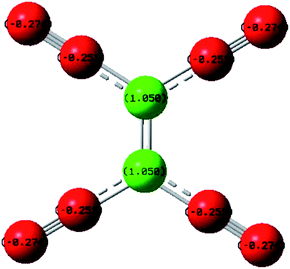 |
| Fig. 2 Electronic distribution of charges in TCNE, using DFT-B3LYP and 6-31+G levels of calculation. | |
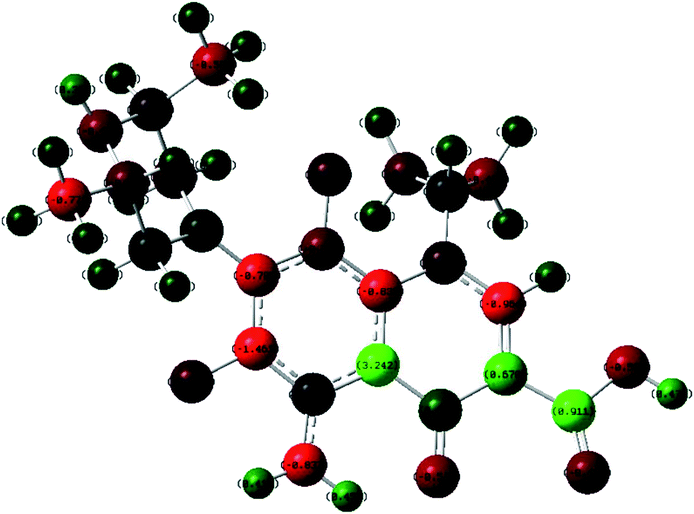 |
| Fig. 3 Electronic distribution of charges in SFX obtained using DFT-B3LYP and 6-31+G levels of calculation. | |
3.2. Assessment of the reaction conditions
3.2.1. Definitive screening design (DSD) model evaluation. As previously mentioned, DSD offers a cogent equilibrium between the number of variables to be modelled and the number of experimental runs to be conducted. With a capability to detect the main and quadratic effects without the need for effect aliasing, as well as the two-way interactions, DSD is currently attracting a great deal of attention.17–19 To develop a prediction model for the influence of the four variables on the developed CTSs and hence to get a better understanding of the interaction of SFX and TCNE, DSD was the approach of choice.All the numerical and 2-level categorical factors that might influence the investigated reaction are listed in Table 1. Factorial levels (upper and lower) were selected based on a literature survey combined with the findings from our preliminary studies, and in order to preclude intemperate conditions. One 2-level qualitative variable, namely the DS (diluting solvent), was considered. As DSD works better with the fewest number of variable and preferably 2-level categorical variables, preliminary studies were conducted to decide the DS. Three solvents were tested: methanol, ethanol and acetonitrile. The two best solvents (based on the maximum response) were selected. Usually, it is easy to decide upon a factorial impact by looking at the coefficient; however, since the physical scales for the selected variables were different, coding was the optimum approach to unify the scale. Table 2 shows the design matrix (coded and un-coded variables).
3.2.2. Data fitting and modelling. The response surface for each CTS was modelled using a general equation for DSD as follows: |
YCTS = m0 + m1X1 + m2X2 + … + mnXn + ∑mikXiXk + ∑miiXi2
| (1) |
where YCTS denotes the measured response at either charge-transfer state, m0, m1, etc. are the regression coefficients with m0 being a constant, Xi is an independent variable (A, B, C or D) and XiXk and Xi2 are the interaction and quadratic terms, respectively. Analysis of data was performed using a Box–Cox transformation together with a stepwise selection of terms (where α to be removed and α to be added were 0.15 and the model hierarchy was maintained in all cases) in the case of YCTS2, compared to only a forward selection using α = 0.25 in the case of YCTS1.32Table 2 shows that the predicted (fitted) values for the response for both CTSs were close to the experimentally obtained values. Values of the standard error of fit (SE Fit) were small (ranging between 0.0016–0.0035 and 0.003–0.109 for YCTS1 and YCTS2, respectively), implying good precision for the estimate of the mean response at a 95.0% confidence interval (CI).
The regression equations (eqn (2) and (3)) obtained following the response transformation are shown in Table 3 for both CTSs together with the values of R2, R2-Adj (R2-adjusted) and R2-Pred (R2-predicted). As shown in Table 3, the values of R2 were high, implying the good performance of the proposed models. The values of R2-Adj were in good agreement with R2-Pred, indicating the capability of the proposed models to predict the response for a new observation. The R2-Pred values were not noticeably less than R2, inferring that the model was not overfitting.
Table 3 Regression equations for the response surface models and the analysis of variance findings following data transformation
Response variable |
Regression equation |
R2 |
R2-Adj |
R2-Pred |
Transformed YCTS1 |
YCTS1 = 0.55936 + 0.006138A + 0.05545B − 0.004674C − 0.47416D + 0.000082AB + 0.004595AD |
(2) |
0.9999 |
0.9998 |
0.9996 |
Transformed YCTS2 |
(YCTS2λ−1)/(λ × g(λ−1)) = −0.9965 − 0.0431B + 0.4361D − 0.1184BD, (λ = 3, g = 0.482318 is the geometric mean of YCTS2) |
(3) |
0.8659 |
0.8256 |
0.7746 |
A Pareto chart of standardized effects was plotted to determine the statistical significance of each factor before and after response transformation. As shown in Fig. 4 and considering the second CTS at 464 nm as an example, the diluting solvent (DS, D) and reagent volume (RV, B) were the most statistically effective factors (i.e. exceeding the reference line). The interaction (RV*DS) was also statistically significant. The same results were obtained using normal and half-normal probability plots (graphs are not shown herein). These findings were further confirmed by analysis of variance (ANOVA), where variables with p-value < 0.05 were considered as statistically significant. All the measurements were conducted at 95.0% CI. Regression eqn (2) and (3) showed the direction and magnitude of each variable on the measured response through the associated coefficient. For example, and for YCTS1, variables A (temp) and B (RV) possessed a positive standardized effect, with B being more effective than A, as compared to a negative effect of C (RT) and D (DS).
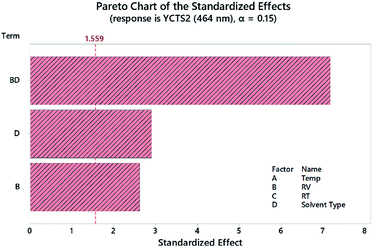 |
| Fig. 4 Pareto chart of the standardized effects for the DSD for the absorbance of coloured CTC measured at 464 nm (YCTS2) following response transformation. | |
Probability plots at 95.0% CI, Fig. 5, were used to assess whether the obtained data, following response transformation, were normally distributed. The decision was made by comparing p-values with the significance level (α = 0.05) and by visualizing how precisely the data points trailed the fitted distribution line (middle line in both panels). The shown panels were grouped based on the influence of the categorical factor, i.e. the diluting solvent (DS, D). In both cases, the obtained p-values were >0.05 and followed the fitted line well.
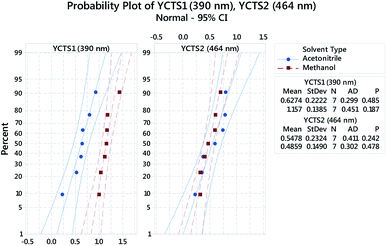 |
| Fig. 5 Multiple probability plot for the absorbance of the coloured complex measured at 390 nm (Left, YCTS1) and 464 nm (Right, YCTS2) following response transformation. Data points were grouped based on the categorical factor (DS, D). The outer lines on the plot are confidence intervals for the individual percentiles. | |
Histograms were sketched to investigate the shape and spread of the obtained data. Data were also categorized based on the influence of the DS used. Interestingly, the data showed a multi-modal distribution in the case of the peak at 390 nm compared to the peak at 464 nm. A 2-sample t-test was further used to assess whether the impact of a different DS on the absorbance of the CTC was statistically significant. Preliminary results showed that the difference between methanol and acetonitrile on YCTS2 was not statistically significant, whereby the p-value was >α (significance level). The opposite was comprehended in the case of YCTS1, where the mean response in the case of methanol was noticeably higher compared to acetonitrile, Table 4. This explains the bimodal distribution observed in Fig. 6 and infers the impact of solvent polarity on the formed complex at this stage. Keeping in mind that the sample size was less than 20 samples and that the histograms might not be capable of accurately describing the data distribution, individual value plots were used instead to assess the existence of any outliers as well as the distribution coverage, Fig. 7. As shown on this figure, and considering that the use of methanol as a DS would increase the response compared to acetonitrile in the case of YCTS1, the impact of the two solvents was almost the same.
Table 4 Descriptive statistics for the 2-sample t-tests
Response variable |
Solvent type |
N |
Mean |
SD |
SE mean |
p-value |
Transformed YCTS1 |
Acetonitrile |
7 |
0.627 |
0.222 |
0.084 |
0.000 |
Methanol |
7 |
1.157 |
0.139 |
0.052 |
Transformed YCTS2 |
Acetonitrile |
7 |
0.548 |
0.232 |
0.088 |
0.564 |
Methanol |
7 |
0.486 |
0.149 |
0.056 |
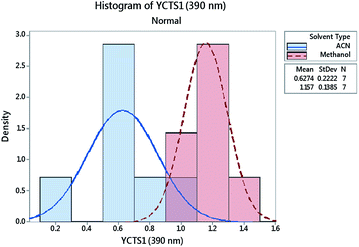 |
| Fig. 6 Histogram for the absorbance of the coloured complex measured at 390 nm (YCTS1) following response transformation. Data points were grouped based on the categorical factor (DS, D). | |
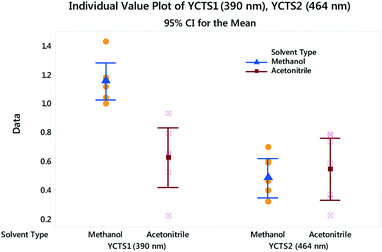 |
| Fig. 7 Individual value plot for both CTSs with added interval bars at 95.0% CI. Individual standard deviations are used to calculate the intervals. | |
Residual plots (e.g. normal probability plot of the residuals, histograms of residuals, versus fits and versus order, although note the figures are not shown) were also used to attest whether the ordinary least squares hypotheses were realized. Comparisons between models were performed based on the obtained values of the Anderson–Darling (AD) statistic as well as the probability (p-value).33,34 The results obtained showed that goodness-of-fit was achieved.
3.2.3. Response optimization. Finding the optimal conditions for the formation of CTC at either wavelength could be achieved either by dealing with each state as a separate entity or with the CTC unabridged. Therefore, more than one set-up could be proposed. Thinking of the entire CTC and setting the goal to maximize both CTSs, the ideal conditions would be: temp 60 °C, RV 5.0 mL, RT 0 min and methanol as a DS, Fig. 8. The desirability of these conditions was confirmed by the Derringer function statistic (D), which was calculated using the following equation: |
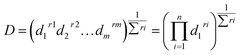 | (4) |
where D is the global desirability, d is the individual desirability, r is the importance of each response compared to the other and m is the number of responses to be optimized.35,36 The obtained value of D was close to 1.0000, implying the favourable influence of the selected variables' blend on the response. Optimization of the separate states (at the expense of one another) showed that YCTS1 could be favoured using the same set-up shown in Fig. 8. Yet, YCTS2 would be favoured if RV was reduced to 1.0 mL and acetonitrile was used as a DS. This finding reflects the impact of solvent polarity on the formation of the CTC. It is noteworthy to mention that changing the temperature from 60 °C to 25 °C did not greatly impact the value of the composite desirability. Therefore, and to avoid heating, the temperature was kept at 25 °C.
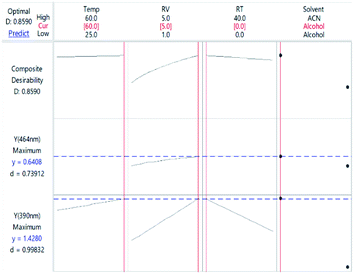 |
| Fig. 8 Optimization plot showing the optimal conditions for the CTC considering both states. | |
3.2.4. Contour and surface plots. Contour plots represent the relationship between a fitted response for one wavelength when considering the study of only two factors in each plot. The darkest zone on the graph, Fig. 9 (left panel), shows the highest absorbance, which could be obtained using 2.2–3.5 mL of TCNE, while keeping the RT at less than 7 min. For the surface plots, 3-dimensional graphs showing the relationship between a response value on the Z-axis and two variables on the X- and Y-axes are shown on the right panel of Fig. 9. The combinations of three axes produce peaks and valleys represented as local maxima or minima. Similar inferences could be obtained as in the left panel.
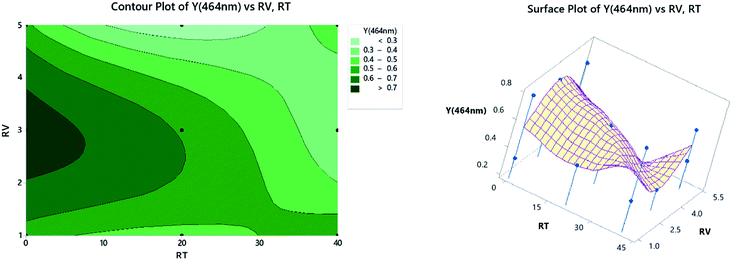 |
| Fig. 9 2D contour plots (left) and 3D surface plots (right). | |
3.3. Validation of the proposed method
The proposed method was validated executing the ICH guiding principles.25 The format includes the assessment of the method linearity, sensitivity, limits of detection (LOD) and quantification (LOQ), accuracy and precision. The analytical parameters are listed in Table 5.
Table 5 Spectral parameters, linearity and sensitivity data for the determination of SFX using the proposed method at 464 nm
Parameter |
Value |
Parameter |
Value |
Regression equation: A = bC + a, where A is the absorbance, C is the concentration in mM, a is the intercept, b is the slope, Sb = SD of slope, ±tSb = confidence limit for the slope, Sa = SD of the intercept ±tSa = confidence limit for the intercept Sy/x = SD of the regression, SS is the sum of the squares. LOD = limit of detection, LOQ = limit of quantification, r2 = coefficient of determination. |
Wavelength, λmax (nm) |
464 |
Slope (b) |
0.0103 |
Linear range, (μg mL−1)a |
10.00–90.00 |
Intercept (a) |
−0.0576 |
Sb |
0.00024 |
r2 |
0.9978 |
±tSb |
0.00019 |
LOD (μg mL−1)b |
1.97 |
Sa |
0.01388 |
LOQ (μg mL−1)b |
5.96 |
±tSa |
0.01110 |
Residual SS |
0.0012 |
Sy/x |
0.01724 |
Regression SS |
0.5749 |
3.3.1. Linearity and range. A linear relationship was found between the SFX concentration (10–90 μg mL−1) and the absorbance, Table 5, at λmax = 464 nm. The optimum conditions mentioned in Fig. 8 were executed to construct the calibration curve. The values for the CI of the slope and intercept are shown in Table 5. A statistical assessment of the results obtained from the analysis of SFX by the proposed method compared to those of a reference spectrophotometric method37 was performed using the variance ratio F-test and the student's t-test. The results obtained displayed a virtual agreement between both methods, Table 6.
Table 6 Determination of SFX using TCNE as bulk and in a synthesized preparation contrasted to a reference method37
Parameter |
Proposed method |
Reported method37 |
Synthetic formulation |
Calibration method |
Standard addition method |
Average of 3 determinations; a and b are the tabulated t-values and F-ratios at p = 0.05. |
Mean % recoverya |
100.01 |
99.77 |
100.29 |
99.48 |
±SD |
0.81 |
1.25 |
3.90 |
3.96 |
RSD |
0.81 |
1.25 |
3.89 |
3.98 |
N |
6 |
5 |
5 |
5 |
±SE |
0.331 |
0.312 |
1.59 |
1.62 |
t |
0.385 (2.262)a |
|
|
|
F |
2.38 (6.26)b |
|
|
|
The impact of the formulation additives was tested through the recovery calculations. The average % recoveries were 100.29 and 99.48 for the calibration and standard addition methods, respectively. These numbers indicate that the matrix effect was absent, and that the proposed method was successful in determining SFX in its formulation, Table 6.
3.3.2. LOD and LOQ. The limits of detection (LOD) and quantification (LOQ) were calculated according to the following equation: |
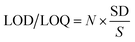 | (5) |
where SD is the standard deviation for the blank under the optimum conditions and S is the slope of the calibration curve. Here, N = 3.3 for the LOD and 10 for the LOQ. The LOD and LOQ values were small enough to indicate the method sensitivity, Table 5.
3.3.3. Accuracy and precision. The accuracy and precision of the proposed technique were concluded following the optimization phase and at 95.0% CI for three different concentrations of SFX as per se and in its synthesized formulation. Evaluation of the precision was done three times within the same day, i.e. within the course of measurements (intra-day) made for each sample and on three different days (inter-day).Precision is expressed as the percentage relative standard deviation (% RSD). The results indicated the good precision of the developed methods and its suitability for the quality control analysis of SFX. The accuracy of the suggested methods was assessed as the percentage relative error (% RE).38 The data obtained are disclosed in Table 7 and indicate a reasonable accuracy and precision of the proposed procedures.
Table 7 Accuracy and precision of the suggested reaction (intra- and inter-day)
Added (μg mL−1) |
Recovery%a |
Mean ± SD |
RSD |
% SE |
Average of three determinations. |
Intra-day |
5.00 |
98.87 |
4.94 ± 1.22 |
1.24 |
1.13 |
20.0 |
99.15 |
19.83 ± 0.52 |
0.53 |
0.85 |
40.0 |
99.69 |
39.88 ± 0.45 |
0.46 |
0.31 |
![[thin space (1/6-em)]](https://www.rsc.org/images/entities/char_2009.gif) |
Inter-day |
5.00 |
99.47 |
4.97 ± 0.90 |
0.91 |
0.53 |
20.0 |
100.20 |
20.04 ± 0.80 |
0.80 |
−0.22 |
40.0 |
100.33 |
40.13 ± 1.85 |
1.84 |
−0.33 |
3.4. Determination of the stoichiometry of the reaction
To determine the molar ratio, Job's method of continuous variation was applied as described under the experimental section.27 The obtained plot reached a maximum value at a mole fraction of nearly 0.56, indicating the formation of a 1
:
1 complex between SFX and TCNE.
3.5. Thermodynamic parameters
The formation constant for the CT reaction between SFX and TCNE was calculated using the Benesi–Hildebrand equation:39 |
[Ao]/AAD = 1/εAD + 1/εADKADc × 1/[Do]
| (6) |
where [Ao] and [Do] are the initial concentrations of the acceptor and donor, respectively, εAD is the molar absorptivity of the formed complex, AAD is the absorbance of the charge-transfer complex and KADc is the formation constant of the complex. The application of the Benesi–Hildebrand equation for 1
:
1 CTC required that the [Ao] must be fixed and lower than [Do]. The [Do] was higher than [Ao] by 5 to 10 times. For different temperatures (25 °C, 40 °C, 55 °C, 70 °C and 85 °C), graphs of [Ao]/AAD (y-axis) against 1/[Do] (x-axis) were plotted. Straight lines were obtained at the different temperatures, supporting the formation of a 1
:
1 complex. The obtained straight lines had a slope and intercept equal to 1/εAD and 1/εADKADc, respectively. The different values of εAD and KADc calculated at different temperatures at 464 nm are illustrated in Table 8.
Table 8 Physical parameters of the CTC measured at different temperatures, λCT 464 nm
Temp (K) |
λCT (nm) |
KADc(L mol−1) |
ξ (L mol−1 cm−1) |
ΔG° (kJ mol−1) |
ΔH° (kJ mol−1) |
ΔS° (J K−1 mol−1) |
298 |
464 |
7.14 × 103 |
2.0 × 103 |
−21.989 |
−53.10 |
55.970 |
313 |
2.00 × 104 |
2.5 × 103 |
−25.776 |
65.386 |
328 |
1.67 × 104 |
2.0 × 103 |
−26.514 |
64.646 |
343 |
1.00 × 103 |
3.3 × 103 |
−19.702 |
57.441 |
358 |
6.67 × 102 |
5.0 × 103 |
−19.356 |
54.069 |
Complex formation was found to be thermodynamically favoured based on the large negative value of standard Gibbs free energy change (ΔG°). The standard Gibbs free energy decreased (less negative) as the temperature increased, indicating that complex formation was not favoured at higher temperature. This also could be concluded from the values of the formation constant, KADc.The calculated values of the formation constant KADc at different temperatures were used to calculate the standard enthalpy of formation (ΔH°) using van't Hoff plots and using the following equation:40
|
log KADc = ΔH°/2.03RT + constant
| (7) |
where
R is the gas constant (8.314 J mol
−1 K
−1), and
T is the temperature in Kelvin. A linear graph was obtained with a slope = −Δ
H/2.303
R, after plotting the log of the formation constant
KADc against the reciprocal of the absolute temperature. The calculated standard enthalpy change (Δ
H°) was a large negative value, denoting that the complex formation was exothermic in nature. The standard entropy change (Δ
S°) was obtained by applying the following equation:
The positive values of ΔS° indicated an increase in the entropy, upon the formation of the CTC.
3.6. Evaluation of the kinetics methods
The absorbance of the produced complex was found to rise as the heating time increased, till it reached saturation. This inference was used as a basis for a kinetic evaluation of the interaction between SFX and TCNE. Under the previously delineated optimal conditions, the initial rates of the proposed reaction were determined from the slopes of absorption–time curves. The reaction rate can be described by the following equation: |
Rate = K′[acceptor]m[donor]n
| (9) |
Under the optimal experimental conditions, the rate of reaction was found to be [SFX] dependent, and could be best described by the pseudo-first order rate equation:
where
K′ is the pseudo-first order rate constant, and
n is the order of reaction with respect to [SFX]. Numerous outlines were then verified to find [SFX] from the rate data and taking into consideration
eqn (9) and
(10). The investigated techniques included the initial rate, rate constant, fixed concentration and fixed time methods.
41–44 The four methods were assessed, and the choice was made based on the applicability, LOD, LOQ and linearity of the obtained data.
3.6.2. Fixed-time method. In this technique, calibration graphs were plotted for the concentration range 5–60 μg mL−1 at fixed reaction times of 0, 5, 10, 20, 30, 45 and 60 min. The regression equations were calculated at each time. The results are illustrated in Table 9. The best correlation coefficient was obtained at a reaction time of 0–5 min. A distortion of linearity was observed in the cases involving heating for longer times.
Table 9 Regression equations at different fixed times for the determination of SFX using TCNE
Time (min) |
Regression equation |
R2 value |
0 |
A = 0.0101C − 0.0621 |
0.9965 |
5 |
A = 0.0077C − 0.0115 |
0.9993 |
10 |
A = 0.0119C − 0.0548 |
0.9705 |
20 |
A = 0.0087C − 0.0014 |
0.9955 |
30 |
A = 0.0031C − 0.0225 |
0.8462 |
45 |
A = 0.0091C − 0.1230 |
0.8461 |
60 |
A = 0.0094C − 0.0976 |
0.7784 |
3.6.3. Rate constant method. In this method, plots of log absorbance (log
A) vs. time in (s) were drawn for a concentration range of 5–60 μg mL−1 (1.27 × 10−5 to 1.52 × 10−4 M) of SFX. Then, a plot of K′ (pseudo-first-order rate constant) on the y-axis vs. concentration (M), on the x-axis was constructed. The regression equation was: |
K′ = 0.4373x − 0.0004 with R2 = 0.9264
| (13) |
The equation shows good linearity, yet less than that shown for fixed-time and fixed-concentration methods.
3.6.4. Fixed-concentration method. In this method, a plot of absorbance versus time (min) was drawn. A straight line was drawn to cut as many curves as possible. Then, another plot of 1/t (s) vs. concentration (M) was drawn. The regression equation was: |
1/t = −0.5.0608C + 0.0013, R2 = 0.9562
| (14) |
The method was applicable over a narrow concentration range of 12–16 μg mL−1, and therefore, it is not recommended for the determination of SFX.
4 Conclusions
Sparfloxacin was analysed using a green spectrophotometric approach, both as a pure material and as a pharmaceutical formulation. The method was based on the formation of a CTC between SFX and TCNE. Two charge-transfer states were observed, at 390 and 464 nm. A quantum mechanical calculation using density functional theory, with the B3LYP level of calculation and 6-31G(+) basis set was used to calculate the charge distribution of the electron cloud for the donor and acceptor. For the donor, the results showed multiple donating atoms and sites with a relatively high electron density. The results showed that the donor was not a simple single atom donor, instead it was a combination of several atoms. Such molecules usually show a complex CTC with multi absorption features. For the acceptor, the calculation showed that the C
C site had the highest electron deficiency, and consequently, was the receptor of the electron charge and not the strong electron-withdrawing cyano groups, as would be expected. As a three-level factorial design, DSD was used to optimize the response. A Pareto chart was used to find the most statistically significant factors that favoured the formation of the CTC. The diluting solvent was found to be the most statistically significant variable. The thermodynamics and kinetics of the reaction were studied. The calculated standard enthalpy change (ΔH°) was a large negative, implying that the reaction was exothermic, and the complex formation was spontaneous. Following the reaction kinetics, the reaction was found to follow a pseudo-first order. It was found that the molar ratio was 1
:
1 (D
:
A) by applying Job's method and this was further confirmed by the Benesi–Hildebrand equation. The kinetic study was evaluated through the initial rate, the rate constant, the fixed absorbance and fixed-time methods. The developed method was validated employing the ICH guidelines, and the obtained results were found to be satisfactory.
Conflicts of interest
There are no conflicts to declare.
References
- N. X. Chin, J. W. Gu, K. W. Yu, Y. X. Zhang and H. C. Neu, Antimicrob. Agents Chemother., 1991, 35, 567–571 CrossRef CAS PubMed.
- V. T. Andriole, in The Quinolones, ed. V. T. Andriole, Academic Press, San Diego, 3rd edn, 2000, ch. 16, pp. 477–495 Search PubMed.
- J. K. Aronson and M. N. G. Dukes, in Meyler's Side Effects of Drugs : The International Encyclopedia of Adverse Drug Reactions and Interactions, ed. J. K. Aronson and M. N. G. Dukes, Elsevier, Amsterdam, 15th edn, 2006, pp. 3172–3174 Search PubMed.
- J. K. Aronson, in Meyler's Side Effects of Drugs, ed. J. K. Aronson, Elsevier, Oxford, 16th edn, 2016, pp. 465–467 Search PubMed.
- L. M. Dembry, J. M. Farrington and V. T. Andriole, Fluoroquinolone Antibiotics: Adverse Effects and Safety Profiles, Infectious Diseases in Clinical Practice, 1999, 8, 421–428 CrossRef.
- E. M. Scholar and W. Pratt, in The Antimicrobial Drugs, ed. E. M. Scholar and W. Pratt, Oxford Univ. Press, Oxford, U.K., 2nd edn, 2000, pp. 257–279 Search PubMed.
- J. Li, X. Ren, Y. Diao, Y. Chen, Q. Wang, W. Jin, P. Zhou, Q. Fan, Y. Zhang and H. Liu, Food Chem., 2018, 257, 259–264 CrossRef CAS PubMed.
- Z. Pan, J. Peng, Y. Chen, X. Zang, H. Peng, L. Bu, H. Xiao, Y. He, F. Chen and Y. Chen, Microchem. J., 2018, 136, 71–79 CrossRef CAS.
- A. F. Faria, M. V. N. de Souza, M. V. de Almeida and M. A. L. de Oliveira, Anal. Chim. Acta, 2006, 579, 185–192 CrossRef CAS PubMed.
- Y. Wang, W. R. G. Baeyens, C. Huang, G. Fei, L. He and J. Ouyang, Talanta, 2009, 77, 1667–1674 CrossRef CAS PubMed.
- S. Jain, N. K. Jain and K. S. Pitre, J. Pharm. Biomed. Anal., 2002, 29, 795–801 CrossRef CAS PubMed.
- H.-J. Zeng, R. Yang, B. Liu, L.-F. Lei, J.-J. Li and L.-B. Qu, J. Pharm. Anal., 2012, 2, 214–219 CrossRef CAS PubMed.
- M. Hou, X. Yan and L. Xiong, J. Lumin., 2015, 157, 58–62 CrossRef CAS.
- H. R. N. Marona and E. E. S. Schapoval, J. Pharm. Biomed. Anal., 2001, 26, 501–504 CrossRef CAS PubMed.
- H. Askal, I. Refaat, I. Darwish and M. Marzouq, Chem. Pharm. Bull., 2007, 55, 1551–1556 CrossRef CAS PubMed.
- A. M. El-Didamony, Anal. Lett., 2007, 40, 2708–2720 CrossRef CAS.
- B. Jones and C. J. Nachtsheim, J. Qual. Technol., 2011, 43, 1–15 CrossRef.
- B. Jones and C. J. Nachtsheim, J. Qual. Technol., 2013, 45, 121–129 CrossRef.
- C. Zhang, W. Chen, J. Xian and D. Fu, RSC Adv., 2018, 8, 3934–3940 RSC.
- M. Belfaragui, A. Seridi, J.-Y. Winum, M. Abdaoui and M. Kadri, Spectrochim. Acta, Part A, 2013, 108, 55–61 CrossRef CAS PubMed.
- A. M. A. Adam, M. S. Refat, M. S. Hegab and H. A. Saad, J. Mol. Liq., 2016, 224, 311–321 CrossRef CAS.
- M. S. Elazazy, K. Ganesh, V. Sivakumar and Y. H. A. Huessein, RSC Adv., 2016, 6, 64967–64976 RSC.
- N. Holzmann, L. Bernasconi, K. M. Callaghan, R. H. Bisby and A. W. Parker, Chem. Phys. Lett., 2018, 692, 146–151 CrossRef CAS.
- M. S. Elazazy, in Spectroscopic Analyses - Developments and Applications, ed. E. Sharmin and F. Zafar, IntechOpen, 2017, 69891, ch. 11, pp. 213–230 Search PubMed.
- International Conference on Harmonization, ICH Harmonized Tripartite Guideline – Validation of Analytical Procedures: text and methodology, Q2 (R1) Complementary Guideline on Methodology, Fed. Register, London, 2005 Search PubMed.
- RxList, https://www.rxlist.com/zagam-drug.htm#description, accessed February 2018.
- P. Job, Ann. Chim., 1928, 9, 113–203 CAS.
- M. J. Frisch, G. W. Trucks, H. B. Schlegel, G. E. Scuseria, M. A. Robb, J. R. Cheeseman, G. Scalmani, V. Barone, B. Mennucci, G. A. Petersson, H. Nakatsuji, M. Caricato, X. Li, H. P. Hratchian, A. F. Izmaylov, J. Bloino, G. Zheng, J. L. Sonnenberg, M. Hada, M. Ehara, K. Toyota, R. Fukuda, J. Hasegawa, M. Ishida, T. Nakajima, Y. Honda, O. Kitao, H. Nakai, T. Vreven, J. A. Montgomery Jr, J. E. Peralta, F. Ogliaro, M. Bearpark, J. J. Heyd, E. Brothers, K. N. Kudin, V. N. Staroverov, T. Keith, R. Kobayashi, J. Normand, K. Raghavachari, A. Rendell, J. C. Burant, S. S. Iyengar, J. Tomasi, M. Cossi, N. Rega, J. M. Millam, M. Klene, J. E. Knox, J. B. Cross, V. Bakken, C. Adamo, J. Jaramillo, R. Gomperts, R. E. Stratmann, O. Yazyev, A. J. Austin, R. Cammi, C. Pomelli, J. W. Ochterski, R. L. Martin, K. Morokuma, V. G. Zakrzewski, G. A. Voth, P. Salvador, J. J. Dannenberg, S. Dapprich, A. D. Daniels, O. Farkas, J. B. Foresman, J. V. Ortiz, J. Cioslowski and D. J. Fox, Gaussian 09, Gaussian, Inc., Wallingford CT, 2009 Search PubMed.
- R. M. Issa, A. A. Hassanein, I. M. El-Mehasseb and R. I. A. El-Wadoud, Spectrochim. Acta, Part A, 2006, 65, 206–214 CrossRef PubMed.
- N. T. Abdel Ghani and A. M. Mansour, J. Mol. Struct., 2011, 991, 108–126 CrossRef CAS.
- O. R. Shehab and A. M. Mansour, J. Mol. Struct., 2015, 1093, 186–194 CrossRef CAS.
- G. E. P. Box and D. A. Cox, J. Royal Stat. Soc. B, 1964, 26, 211–252 Search PubMed.
- T. W. Anderson and D. A. Darling, J. Am. Stat. Assoc., 1954, 49, 765–769 CrossRef.
- G. Marsaglia and J. C. W. Marsaglia, J. Stat. Softw., 2004, 9, 1–5 Search PubMed.
- G. Derringer and R. Suich, J. Qual. Technol., 1980, 12, 214–219 CrossRef.
- R. H. Myers, D. C. Montgomery and C. M. Anderson-Cook, Response Surface Methodology: Process and Product Optimization Using Designed Experiments, Wiley, New York, 4th edn, 2009 Search PubMed.
- S. P. Sujitha, J. P. Asha and K. Tejaswi, Int. J. Innov. Pharm. Sci. Res., 2013, 4, 306–309 Search PubMed.
- J. N. Miller and J. C. Miller, Statistics and Chemometrics for Analytical Chemistry, Prentice Hall/Pearson, 2010 Search PubMed.
- H. A. Benesi and J. H. Hildebrand, J. Am. Chem. Soc., 1949, 71, 2703–2707 CrossRef CAS.
- A. N. Martin, J. Swarbrick and A. Cammarata, Physical Pharmacy, Lee and Febiger, Philadelphia, PA, 2nd edn, 1969, pp. 344–346 Search PubMed.
- K. B. Yatsimirskii, Kinetic Methods of Analysis, Pergamon Press, Oxford, 1966 Search PubMed.
- H. Laitinen and W. Harris, Chemical Analysis, McGraw-Hill, New York, 2nd edn, 1975 Search PubMed.
- M. S. Elazazy, Int. J. Pharma Sci. Res., 2015, 6, 1000–1011 Search PubMed.
- M. S. Elazazy, H. M. Khalil, M. N. Elbolkiny and A. Shalaby, Chin. Pharm. J., 2004, 56, 37–47 CAS.
|
This journal is © The Royal Society of Chemistry 2019 |
Click here to see how this site uses Cookies. View our privacy policy here.