DOI:
10.1039/C9RA04530A
(Paper)
RSC Adv., 2019,
9, 22980-22986
Exploration of a high-efficiency and low-cost technique for maximizing the glucoamylase production from food waste†
Received
17th June 2019
, Accepted 11th July 2019
First published on 25th July 2019
Abstract
This study was aimed at the exploration of high-efficiency and low-cost technique for glucoamylase (GA) production from food waste; moreover, the produced GA could be directly used in the hydrolysis of food waste. A mixture of food waste, rice waste and cake waste as a sole feedstock was investigated for the production of GA via solid-state fermentation. The highest GA activity of 458.3 U g−1 was obtained from the rice waste after 9 days of incubation. The cake waste also demonstrated a high GA production, achieving 406.5 U g−1 dry substrate. However, the most practical substrate for GA production that could be integrated in the food waste treatment was the mixed food waste, which could effectively produce GA without any additives or adjustments using the technique developed in this study. The optimum conditions for GA production from the mixed food waste were determined through a response surface methodology: the temperature of 31.16 °C, the inoculum amount of 1.54 mL, and the time of fermentation of 7.81 days. The maximum GA activity of 180.59 U g−1 could be achieved under these optimum conditions, which was actually much higher than those reported in the literature. This study showed that the mixed food waste could be an ideal feedstock for the on-site production of high-activity GA, and the produced GA could be directly applied in food waste hydrolysis, which significantly reduced the process cost.
1. Introduction
An estimated 1.6 gigatons of food are wasted annually according to the Food and Agriculture Organization (FAO). Food waste is usually landfilled or incinerated; this leads to many environmental problems.1–3 Landfilled food waste often results in the generation of methane gas, which facilitates global warming since methane has a global warming potential 28 times that of carbon dioxide and demands a significant landfill capacity.4,5 The incineration of food waste is costly due to its high moisture content and leads to severe air pollution.6 Food waste indeed is rich in carbohydrates, proteins, fats, etc., which can be converted into high-value products, such as platform chemicals and biofuels, through fermentation.7–9 As is known, starch is the major component of food waste, accounting for 30–60%.10 Hence, the saccharification of food waste is the key step in its bioconversion into value-added products and external enzymes,11,12 especially glucoamylase (GA), which can hydrolyze starch to produce glucose and is necessary in this process.
Commercial enzymes are usually needed for the treatment of food waste. However, the expenditure on commercial enzymes is the main contributor to the overall production cost of the treatment process.13,14 The overall cost could be significantly reduced if enzymes could be produced on-site; this would decrease the costs of enzyme purchase, transportation and storage. Furthermore, if the activity of the crude enzyme is high enough, it would be possible and economical to directly apply the enzyme in the food waste hydrolysis without a recovery process. These efforts have been made by some researchers before. For example, Wang et al. (2009) have integrated the GA production with lactic acid production from food waste,15 and Kiran et al. (2015) have pretreated food waste with an in situ-produced enzyme to enhance the hydrolysis and methane production potential.16 Since the major component of enzyme cost lies in the raw material, a proper and cheap substrate should be used to produce enzymes. Agro-industrial residues, such as wheat bran and rice bran, are generally considered the best substrates for enzyme production and are the most commonly used feedstocks in the GA production.17–19 However, agro-industrial residues are not suitable for the production of enzymes that can be integrated in food waste hydrolysis. On the one hand, the overall treatment cost increases due to the purchase, transportation and storage of agro-industrial residues. On the other hand, the introduction of exogenous substrates into food waste would aggravate the difficulty of treatment. Therefore, this study was aimed at finding an appropriate substrate that could produce effective GA, which could be directly used in the subsequent food waste hydrolysis without any extraction or treatment process. Solid-state fermentation has many advantages, such as a simpler operation, requirement of less space, superior productivity, low consumption of water, lower production of wastewater, easier control of microorganism contamination, absence of the rigorous control of fermentation parameters, and lower cost for downstream treatment, over submerged fermentation.20 Thus, in this study, solid-state fermentation was employed.
Traditional one-factor-at-a-time approach is laborious, time consuming and often misses the interaction effects between multiple components.21 Recently, many complex statistical designs have been successfully employed in bioprocess optimization, which can effectively overcome the disadvantages of single factor test and minimize the error in the determination of the effect of factors and interactions between factors.22,23 Among them, response surface methodology (RSM) is suitable for identifying the effect of each variable and interactive effect between variables as well as seeking optimum conditions for a multivariable system. Thus, in this study, RSM was applied to optimize the fermentation conditions to obtain GA with high activity.
2. Materials and methods
2.1 Substrates
Mixed food waste used in this study was obtained from the student canteen located in Nanyang Technological University, Singapore. The mixed food waste mainly consisting of rice, meat and vegetables was immediately blended in the laboratory using a domestic kitchen blender and then stored at −20 °C until use. The rice waste was leftover rice obtained from the same canteen and stored at −20 °C directly without any pretreatment. The cake waste obtained from a local supermarket was ground and stored in zipped plastic bags at −20 °C for further experiments. The compositions of all substrates are shown in Table S1.†
2.2 Microorganism and inoculum preparation
All experiments were conducted using Aspergillus oryzae obtained from ABM Chemicals Ltd (Cheshire, England) to produce GA through solid-state fermentation. The propagation of fungus was conducted in 500 mL flasks containing a sterilized solid sporulation medium containing 5% (w/v) whole wheat flour and 2% (w/v) agar (Sigma-Aldrich) via inoculation with 0.5 mL of spore suspension. The flasks were incubated for 4 days at 30 °C. After the incubation period, the fungal spores were harvested using a 1% NaCl solution. The spore solution of A. oryzae (4.75 × 106 spores per mL) was used in the further experiments.
2.3 Effect of various substrates on GA production
The mixed food waste, rice waste and cake waste were chosen to produce GA through solid-state fermentation. The total solids of cake waste and rice waste were 67.44% and 40.04%, respectively, and then, their moisture contents were adjusted to 70% before use. This moisture content has been proved to be optimum for GA production through solid-state fermentation in previous studies.16,24 The moisture content of mixed food waste was not adjusted since its value was already 77.20%. At first, 10 g substrate (dry weight) was weighed and placed in a 250 mL flask. After sterilization by autoclaving at 121 °C for 20 min, the flask was cooled down and then inoculated with a 1 mL A. oryzae spore solution. Fermentation was conducted at 30 °C for 6 days under stationary conditions, and then, the GA content was measured.
2.4 Optimization of process parameters for GA production from mixed food waste
The central composite design obtained using response surface methodology was employed in the optimization of GA production from mixed food waste. To simplify the fermentation process, the substrate was not changed, and only the operation parameters were optimized; thus, fermentation time (X1, day), amount of inoculum (X2, mL) and fermentation temperature (X3, °C) were chosen as independent variables, and GA production (Y, U g−1, based on dry solid) was used as the dependent output variable. Each variable was assessed at five different levels: combining axial points (−α, +α), factorial points (−1, +1) and central point (0), as shown in Table 1. This experiment design could provide information about the significant effects of the selected operation parameters and the interactions between them on the GA production to identify the optimal value of each parameter that could produce maximum GA. The effects of the chosen variables on the GA production were estimated by the following second-order quadratic equation: |
Y = b0 + b1X1 + b2X2 + b3X3 + b11X12 + b22X22 + b33X32 + b12X1X2 + b23X2X3 + b13X1X3
| (1) |
where Y is the predicted response, X1, X2, and X3 are the independent variables, b0 is the offset term, b1, b2, and b3 are the linear effects, b11, b22, and b33 are the squared effects, and b12, b23, and b13 are the interaction effects between variables.
Table 1 The experimental levels of the variables in the central composite design
Variable |
Low axial (−α) |
Low factorial (−1) |
Centre (0) |
High factorial (+1) |
High axial (+α) |
Time (d) |
2 |
4 |
6 |
8 |
10 |
Inoculum (mL) |
0.5 |
1 |
1.5 |
2 |
2.5 |
Temperature (°C) |
25 |
30 |
35 |
40 |
45 |
The Design Expert (Stat-Ease Inc., Minneapolis, USA) (Version 8.0.5.0) software was employed for designing experiments and for the regression analysis of the experimental data obtained. All experiments were carried out in triplicates, and the results were the average data of three trials. The analysis of variance (ANOVA), including Fisher test (F-test), its associated probability P(F) and the coefficient of determination (R2), which evaluates the accuracy of fitting of the regression model, have been calculated according to the experimental data. The response surfaces as well as the contour plots of the predicted responses of the model were used to assess the interactions between these significant operation parameters. Moreover, numerical optimization could provide the optimum operation parameters to achieve maximum GA production from the mixed food waste.
2.5 Analytical methods
At the end of fermentation, 100 mL distilled water was added to the fermented food waste, and the entire content was mixed thoroughly. Then, the solid suspensions were centrifuged at 10
000 rpm for 10 min (4 °C), and the supernatant was used for the GA activity measurement. The GA activity of the enzyme solution was determined by measuring the amount of glucose released from starch per minute. Moreover, one-milliliter of supernatant was incubated with 20 mL 2% (w/v) soluble starch (Sigma-Aldrich) dissolved in 100 mM sodium acetate buffer (pH 5) at 60 °C. The starch solution was first boiled and then cooled down just before use to increase the hydrolytic susceptibility of the starch to the enzyme mixture. The GA activity of the enzyme solution was determined by measuring the amount of glucose released per minute. Glucose concentration in the supernatants was measured using the DNSA assay. The GA activity throughout the study was expressed as U g−1 on dry basis. Moreover, one unit (U) is defined as the amount of the enzyme required to produce 1 mg of glucose per minute under the assay conditions.
Starch content was measured using the Megazyme's TN kit (Bray, Ireland), and the lipid content was determined by the hexane/isopropanol (3
:
2) method.25 Protein was measured using KjelFlex K-360 (BUCHI, Switzerland) and then calculated based on the Kjeldahl method with the conversion factor of 6.25. Moisture and ash contents of the samples were determined by analytical gravimetric methods (AOAC, 2001).
3. Results and discussion
3.1 GA production from various substrates
Fig. 1 shows the GA produced from the cake waste, rice waste and mixed food waste, and highest GA activities have been obtained after 8–9 days of fermentation. The incubation time is usually determined by the type of fungi, characteristic of substrate as well as the incubation conditions.26 As shown in Fig. 1a, the GA produced from cake waste had the significantly higher activity of 406.5 U g−1 dry substrate after 8 days of fermentation, and then, the activity of GA decreased with a further increase in the fermentation time. The possible reason for the reduction of GA activity is the depletion of nutrients in the cake waste. The highest GA activity of 458.3 U g−1 was obtained with the rice waste after 9 days of incubation (Fig. 1b). As can be observed in Table S1,† the starch content in rice waste was much higher than that in other wastes, which reached up to 82.3%. It is known that starch is a key factor that influences the GA production; thus, the highest GA activity has been obtained via rice waste fermentation. The comparison of the results presented in Table 2 clearly suggests that the GA produced in this study possesses significantly higher activity than those reported in the literature;24,26 for example, Kiran et al. (2014) have reported GA produced from bread waste, savory waste, cake waste, cafeteria waste, fruits, vegetables and potatoes, and the highest GA activity has been obtained for the cake waste at the level of 108.5 U g−1, which is about 4.5 times lower than that obtained in this study (e.g., 458.3 U g−1, Table 2); Anto et al. (2006) have also applied rice flake manufacturing waste products as a substrate to produce GA, and the GA activity of 264 U g−1 has been obtained.27 Note that Kunamneni et al. (2005) have reported the GA activity of 534 U g−1 obtained by wheat bran but with a substantial supplementation of minerals. In addition, Fig. 1c shows that the GA produced from food waste obtained from a university canteen without the addition of any supplements and adjustments exhibits much higher activity (169.8 U g−1) than those reported in the literature.24,26,28 As shown in Table S1,† both cake and rice wastes were rich in starch, which was advantageous for the production of GA by fungi. The starch content of rice waste is 1.45 times that of the cake waste, whereas the GA activity of the fermented rice waste is only 1.13 times higher than that measured for the cake waste. Further analysis revealed that the protein content (12.8%) in the cake waste was higher than that in the rice waste (6.8%). These results suggest that although starch is crucial for GA production, the protein in the substrate is also important, promoting the growth of fungi and subsequently enhancing the GA production. In addition to starch and protein, food waste contains very rich nutrients including lipid and minerals. These materials would provide abundant nutrients for the growth of fungi and production of GA. The appearances of mixed food waste at different fermentation times are shown in Fig. 2. Although high-activity GA could be produced from the cake and rice wastes, these wastes could not be easily separated from the mixed food waste or obtained at large quantities. From a practical and economical viewpoint, the mixed food waste is more suitable for the massive on-site production of GA for food waste saccharification. Therefore, the mixed food waste was directly used as a substrate for the production of glucoamylase in the following optimization procedure.
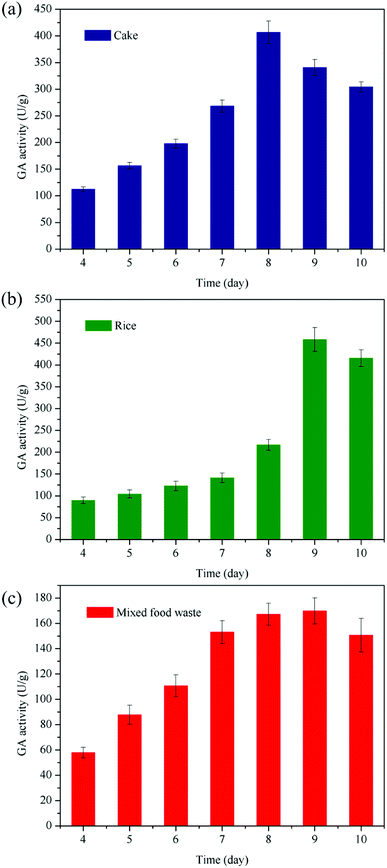 |
| Fig. 1 The GA production from the cake waste, rice waste and mixed food waste substrates. | |
Table 2 GA production under the solid-state fermentation conditions
Substrate |
Inoculum |
Supplemental materials |
Activitya (U g−1) |
Reference |
Transformed by the same activity definition. |
Wheat bran |
Thermomyces lanuginosus |
KH2PO4, MgSO4, peptone |
534.0 |
17 |
Bran, cottonseed powder |
Mixture strains of Aspergillus niger |
(NH4)2SO4, KH2PO4 |
223.0 |
29 |
Defatted rice bran |
Aspergillus niger |
CaCO3, K2SO4, (NH4)2HPO4, and urea; rice straw as medium support |
125.1 |
18 |
Waste bread |
Aspergillus awamori |
None |
114.0 |
30 |
Cake waste |
Aspergillus awamori |
None |
108.5 |
24 |
Waste bread |
Aspergillus awamori |
None |
102.8 |
31 |
Wheat pieces |
Aspergillus awamori |
None |
81.3 |
32 |
Waste bread |
Aspergillus awamori |
None |
78.4 |
32 |
Rice waste |
Aspergillus oryzae |
None |
458.3 |
This study |
Cake waste |
Aspergillus oryzae |
None |
406.5 |
This study |
Mixed food waste |
Aspergillus oryzae |
None |
169.8 |
This study |
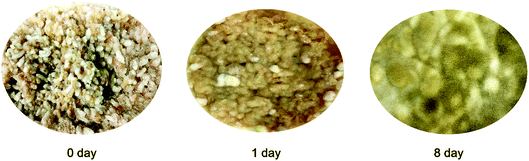 |
| Fig. 2 Appearances of the mixed food waste at different fermentation times. | |
3.2 Optimization of the fermentation conditions for GA production from the mixed food waste
In this study, RSM was employed to optimize the fermentation conditions towards maximizing the GA production from the mixed food waste. For determining the optimal fermentation time, amount of inoculum and fermentation temperature, twenty experiments were designed using a central composite design (Table S2†), and a quadratic model was chosen for data fitting and the analysis of variance (ANOVA). Consequently, a regression equation of GA activity as a function of fermentation time (X1, day), amount of inoculum (X2, mL) and fermentation temperature (X3, °C) was obtained as follows: |
GA = −997.10 + 79.81X1 + 257.29X2 + 42.90X3 + 6.35X1X2 − 0.77X1X3 − 0.29X2X3 − 4.20X12 − 97.04X22 − 0.59X32
| (2) |
As shown in Table 3, the F-value of 79.00 implies that this model is significant. Moreover, all the terms with the values of “Prob > F” below 0.0500 are significant, i.e. the fermentation time and temperature are important than the inoculum amount for the GA production. The coefficient of determination (R2) for the GA production was calculated as 0.9861, meaning that 98.61% of the sample variation in the GA production could be attributed to the independent variables. The value of R is more than 0.99, implying that only less than 1% of the variation in GA production is not explained by the model. The value of the adjusted determination coefficient (adj. R2 = 0.9736) is also high, advocating a high significance of the model. Moreover, a relatively lower value of the coefficient of variation (CV = 5.79%) represents better precision and reliability of the conducted experiments. The adequate precision ratio measures the signal to noise ratio, which is desirable if greater than 4.33 In this case, the value of this ratio was 23.65, indicating an adequate signal and that this model could be used to navigate the design space. Consequently, the chosen quadratic model and the obtained equation were able to represent the GA production system under the specified experimental domain. The activities of GA predicted by the abovementioned equation are presented along with the experimental values in Table S2, and Fig. S1† further shows a good correlation between the experimental and predicted values of the responses. The point cluster is close to the diagonal line; this indicates a good fit between the experimental and predicted data.
Table 3 ANOVA for GA production as a function of fermentation time (X1, day), amount of inoculum (X2, mL) and fermentation temperature (X3, °C)a
Source |
Sum of squares |
Degree of freedom |
Mean square |
F value |
p-Value prob > F |
R2 = 0.9861, R = 0.9930, adjusted R2 = 0.9736, CV = 5.79%, adequate precision = 23.651. |
Model |
33 908.37 |
9 |
3767.597 |
78.99915 |
<0.0001 |
X1-time |
9321.243 |
1 |
9321.243 |
195.4483 |
<0.0001 |
X2-inoculum |
133.8718 |
1 |
133.8718 |
2.80703 |
0.1248 |
X3-temperature |
3825.441 |
1 |
3825.441 |
80.21204 |
<0.0001 |
X1X2 |
322.1213 |
1 |
322.1213 |
6.754255 |
0.0265 |
X1X3 |
470.7139 |
1 |
470.7139 |
9.869953 |
0.0105 |
X2X3 |
4.092068 |
1 |
4.092068 |
0.085803 |
0.7756 |
X12 |
7100.208 |
1 |
7100.208 |
148.8775 |
<0.0001 |
X22 |
14 798.88 |
1 |
14 798.88 |
310.3037 |
<0.0001 |
X32 |
5380.93 |
1 |
5380.93 |
112.8276 |
<0.0001 |
Residual |
476.9161 |
10 |
47.69161 |
|
|
Pure error |
46.59526 |
5 |
9.319051 |
|
|
Cor total |
34 385.29 |
19 |
|
|
|
Fig. 3–5 represent the three-dimensional response surfaces and contour plots for the optimum level of each fermentation condition and the effect of their interactions on the production of GA. By keeping one variable constant at its optimum level and varying the other two variables within the experimental range based on eqn (2), these figures can be obtained. As observed in Fig. 3–5, all response surfaces for the GA production are indicated by clear peaks, meaning that all the optimum points are inside the design boundary levels. Moreover, the shape of the corresponding contour plot indicates whether the mutual interactions between independent factors are significant or not.2
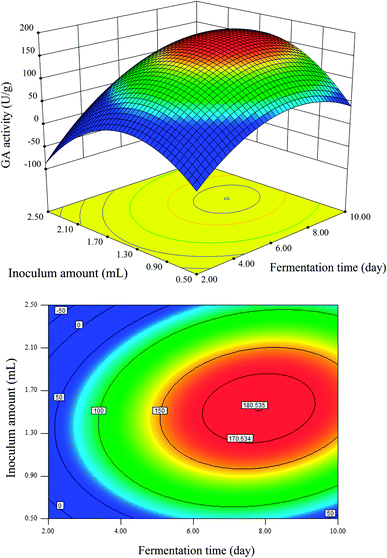 |
| Fig. 3 Response surface and contour plot for the effect of the interaction between the inoculum amount and fermentation time on the GA production (fermentation temperature was kept constant at 31.16 °C). | |
As shown in Fig. 3, the maximum GA activity was obtained at the inoculum amount of 1.5 mL and the fermentation time of 8 days. A further increase in the inoculum amount or fermentation time was found to adversely affect the GA production. As observed in Fig. 3, the effect of the fermentation time on GA production was more significant than that of the inoculum amount. Fermentation time is often determined by the type of fungi, characteristic of substrate, etc.26 In addition, the two-dimensional contour plot showed an elongated running diagonal pattern, suggesting that the effect of the interaction between the fermentation time and the inoculum amount was significant on the GA production (Y).
The effects of fermentation time and temperature on the GA yield while keeping the inoculum amount at 1.54 mL are depicted in Fig. 4. The response surface plot indicated that the optimum production of GA could be attained when the fermentation time was long and the fermentation temperature was low. The two-dimensional contour plot showed a uniformly elongated diagonal running pattern, further suggesting that the effect of the interaction between the fermentation time and temperature was significant on the GA production.
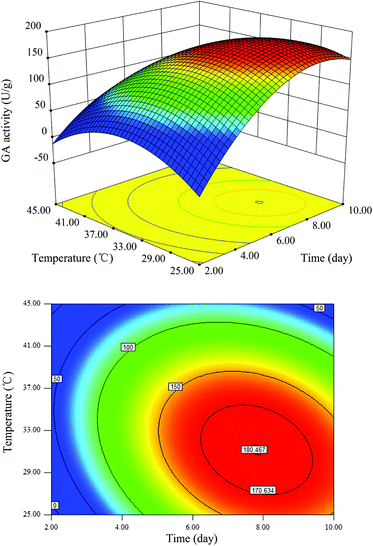 |
| Fig. 4 Response surface and contour plot for the effect of the interaction between fermentation temperature and fermentation time on the GA production (the inoculum amount was kept constant at 1.54 mL). | |
The effect of inoculum amount and fermentation temperature on GA is shown in Fig. 5. In the response surface plot, it can be observed that the maximum GA production can be obtained at low temperatures when the inoculum amount is kept at a zero level. Moreover, the two-dimensional contour plot showed a circular pattern, implying that the effect of the interaction between the inoculum amount and fermentation temperature was not significant on the GA production.
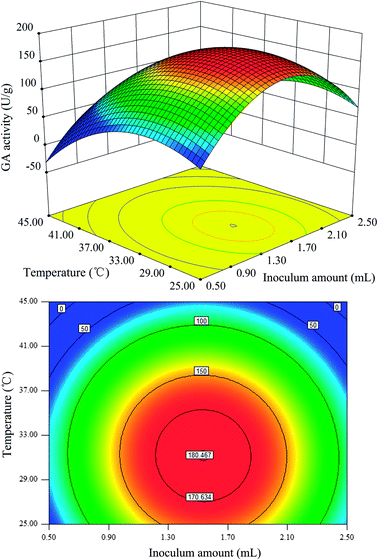 |
| Fig. 5 Response surface and contour plot for the effect of the interaction between fermentation temperature and inoculum amount on the GA production (the fermentation time was kept constant at 7.81 day). | |
The optimum conditions for the maximum GA activity were as follows: the temperature of 31.16 °C, the inoculum amount of 1.54 mL and the time of fermentation of 7.81 days. The maximum GA activity of 180.59 U g−1 could be achieved under these optimum conditions. Thus, using the solid fermentation of mixed food waste, GA with satisfactory activity can be obtained, which will be very useful in the subsequent food waste hydrolysis process.
4. Conclusions
This study demonstrated the feasibility of effective production of GA via solid-state fermentation using food waste as the sole feedstock. Cake waste, rice waste and mixed food waste were explored for the production of GA. GA with the highest activity of 458.3 U g−1 was obtained from the rice waste, whereas GA with the activities of 406.5 U g−1 and 169.8 U g−1 was obtained from the cake waste and the mixed food waste, respectively, under non-optimal conditions. However, considering the convenience of application, the production of GA directly from the mixed food waste was further optimized, and optimal GA production was achieved under the following conditions: the temperature of 31.2 °C, inoculum amount of 1.54 mL and fermentation time of 7.81 days. The maximum GA activity of 180.59 U g−1 could be achieved under these optimum conditions. The activity of GA produced in this study was generally much higher than those reported in the literature. Moreover, this study demonstrated that mixed food waste could be used without any supplements for the production of highly active GA for the saccharification of mixed food waste.
Conflicts of interest
There are no conflicts of interest to declare.
References
- Q. Wu, W. Guo, S. Yang, H. Luo, S. Peng and N. Ren, RSC Adv., 2015, 5, 103876–103883 RSC.
- N. Liu, J. Jiang, F. Yan, Y. Xu, M. Yang, Y. Gao, A. Aihemaiti and Q. Zou, RSC Adv., 2018, 8, 10457–10464 RSC.
- J. Wang, M. Gao, Q. Wang, W. Zhang and Y. Shirai, RSC Adv., 2016, 6, 104354–104358 RSC.
- A. M. Becker Jr, K. Yu, L. B. Stadler and A. L. Smith, Bioresour. Technol., 2017, 223, 131–140 CrossRef PubMed.
- S. Shi, D. Zou, Q. Wang, X. Xia, T. Zheng, C. Wu and M. Gao, RSC Adv., 2016, 6, 9541–9548 RSC.
- M. El-Fadel, A. N. Findikakis and J. O. Leckie, J. Environ. Manage., 1997, 50, 1–25 CrossRef.
- W. Guo, Q. Wu, S. Yang, H. Luo, S. Peng and N. Ren, RSC Adv., 2014, 4, 53321–53326 RSC.
- Y. Ma and Y. Liu, Biotechnol. Adv., 2019 DOI:10.1016/j.biotechadv.2019.06.013.
- Y. Ma, W. Cai and Y. Liu, Appl. Energy, 2017, 206, 83–89 CrossRef CAS.
- D. Pleissner and C. S. K. Lin, Sustainable Chem. Processes, 2013, 1, 21 CrossRef.
- F. Kallel, D. Driss, F. Bouaziz, L. Belghith, S. Zouari-Ellouzi, c. Fatma, A. Haddar, S. E. Chaabouni and R. Ghorbel, RSC Adv., 2015, 5, 6728–6741 RSC.
- W. C. Lam, D. Pleissner and C. S. K. Lin, Biomolecules, 2013, 3, 651–661 CrossRef PubMed.
- Twenty-Sixth Symposium on Biotechnology for Fuels and Chemicals, ed. B. H. Davison, Springer Science & Business Media, 2005, vol. 121, pp. 485–499 Search PubMed.
- A. L. Woiciechowski, S. Nitsche, A. Pandey and C. R. Soccol, Braz. Arch. Biol. Technol., 2002, 45, 393–400 CrossRef CAS.
- X. Q. Wang, Q. H. Wang, H. Zhi Ma and W. Yin, J. Chem. Technol. Biotechnol., 2009, 84, 139–143 CrossRef CAS.
- E. Uçkun Kiran, A. P. Trzcinski and Y. Liu, Bioresour. Technol., 2015, 183, 47–52 CrossRef PubMed.
- A. Kunamneni, K. Permaul and S. Singh, J. Biosci. Bioeng., 2005, 100, 168–171 CrossRef CAS PubMed.
- S. T. Silveira, M. S. Oliveira, J. A. V. Costa and S. J. Kalil, Appl. Biochem. Biotechnol., 2006, 128, 131–139 CrossRef CAS PubMed.
- A. Pandey, Biol. Wastes, 1990, 34, 11–19 CrossRef CAS.
- P. Ellaiah, K. Adinarayana, Y. Bhavani, P. Padmaja and B. Srinivasulu, Process Biochem., 2002, 38, 615–620 CrossRef CAS.
- V. V. R. Bandaru, S. R. Somalanka, D. R. Mendu, N. R. Madicherla and A. Chityala, Enzyme Microb. Technol., 2006, 38, 209–214 CrossRef CAS.
- S. M. T. Gharibzahedi, S. H. Razavi, S. M. Mousavi and V. Moayedi, Ind. Crops Prod., 2012, 40, 345–354 CrossRef CAS.
- S. K. Ahuja, G. M. Ferreira and A. R. Moreira, Biotechnol. Bioeng., 2004, 85, 666–675 CrossRef CAS PubMed.
- E. U. Kiran, A. P. Trzcinski and Y. Liu, Biofuel Res. J., 2014, 1, 98–105 CrossRef CAS.
- A. Hara and N. S. Radin, Anal. Biochem., 1978, 90, 420–426 CrossRef CAS.
- Q. Wang, X. Wang, X. Wang and H. Ma, Process Biochem., 2008, 43, 280–286 CrossRef CAS.
- H. Anto, U. B. Trivedi and K. C. Patel, Bioresour. Technol., 2006, 97, 1161–1166 CrossRef CAS PubMed.
- X. Q. Wang, Q. Wang, Y. Y. Liu and H. Z. Ma, Waste Manage. Res., 2010, 28, 539–544 CrossRef CAS PubMed.
- X.-J. Wang, J.-G. Bai and Y.-X. Liang, Appl. Microbiol. Biotechnol., 2006, 73, 533–540 CrossRef CAS PubMed.
- M. Melikoglu, C. S. K. Lin and C. Webb, Food Bioprod. Process., 2013, 91, 638–646 CrossRef CAS.
- M. Melikoglu, C. S. K. Lin and C. Webb, Biochem. Eng. J., 2013, 80, 76–82 CrossRef CAS.
- R. Wang, L. C. Godoy, S. M. Shaarani, M. Melikoglu, A. Koutinas and C. Webb, Enzyme Microb. Technol., 2009, 44, 223–228 CrossRef CAS.
- M. Muthukumar, D. Mohan and M. Rajendran, Cem. Concr. Compos., 2003, 25, 751–758 CrossRef CAS.
Footnote |
† Electronic supplementary information (ESI) available. See DOI: 10.1039/c9ra04530a |
|
This journal is © The Royal Society of Chemistry 2019 |
Click here to see how this site uses Cookies. View our privacy policy here.