DOI:
10.1039/C9RA07328K
(Paper)
RSC Adv., 2019,
9, 40196-40202
A simple and robust reporter gene assay for measuring the bioactivity of anti-RANKL therapeutic antibodies
Received
12th September 2019
, Accepted 16th November 2019
First published on 3rd December 2019
Abstract
RANKL (receptor activator of nuclear factor κB ligand) plays a key role in the differentiation, activation and survival of osteoclasts. Denosumab, which targets RANKL, is approved for osteoporosis or bone loss that has a high risk for fracture and bone metastases from solid tumors. Bioactivity determination is essential for the safety and efficacy of therapeutic antibodies. At present, the mechanism of action (MOA) based bioassay for anti-RANKL monoclonal antibodies (mAbs) is the measurement of tartrate resistant acid phosphatase (TRAP) activity, which takes about five days and has complex operation and relatively high variation. In this study, we developed a reporter gene assay (RGA) based on a RAW264.7 cell line stably expressing luciferase reporter under the control of nuclear factor-κB (NF-κB) response elements. After optimizing the key parameters, the validation results based on ICH-Q2 not only show superior specificity, precision, linearity, accuracy and passage stability, but also a short duration and simple operation. These results demonstrate the RGA based on the RANKL–RANK–NF-κB pathway can be an excellent alternative for measuring the bioactivity of anti-RANKL mAbs.
1. Introduction
Bone tissue involves a variety of functions in vertebrates,1 and is constantly being remolded. Two major cell types, the bone-building osteoblasts and the bone-adsorbing osteoclasts, play essential roles during the remolding process, and the balance between them is well orchestrated to maintain the physiological conditions.2,3
RANKL/RANK regulates the bone adsorbing process by modulating osteoclast development.4,5 RANKL was identified both in transmembrane6,7 and soluble forms,8,9 while both forms interact with RANK in osteoclast membranes, and regulate their differentiation into multinucleated osteoclasts and further activation into mature osteoclasts.4,5 Various factors, such as parathyroid hormone-related peptide (PTHrP), corticosteroids and others, induce RANKL expression from osteoblasts.10 As a decoy receptor for RANKL, osteoprotegerin (OPG) interferes the interaction between RANKL and RANK, contributing to the inhibition of bone resorption.6,11 OPG can be induced by various factors, such as estrogen and IL-4.5 The balance between the level of RANKL and OPG is tightly regulated to maintain the physiological bone structure. However, in postmenopausal women, the decreased level of estrogen leads to the reduced OPG expression, resulting in the increased RANKL activity and the resultant elevated bone-resorbing activity of osteoclasts, hence osteoporosis.12
Although as the first line treatment for osteoporosis, the sole use of biphosphate is imperfect, which necessitates newer drug development.13 As for targeting RANKL/RANK/OPG system as discussed above, OPG-Fc development was the first attempt,14 but was discontinued due to the induction of immunogenicity. Anti-RANKL mAb, denosumab, was then successfully developed and marketed by Amgen due to its good pharmacokinetic profile and excellent clinical efficacy.15
Denosumab blocks the interaction between RANKL and RANK, with higher affinity to RANKL than OPG.15 With the trademark of Prolia, denosumab was approved to treat osteoporosis or bone loss with high risk for fracture, due to menopause, taking corticosteroid, and non-metastatic breast and prostate cancers (https://www.prolia.com). Furthermore, with the trademark of Xgeva, denosumab is indicated to prevent fracture, spinal cord compression, or the need for radiation or surgery to bone in patients with multiple myeloma and in patients with bone metastases from solid tumors (https://www.xgeva.com).
Proper high-order structure is essential for the function of complex molecules such as mAbs, while as for quality control strategies, the physicochemical information may be extensive but unable to confirm the higher-order structure which, however, can be inferred from the bioactivity.16 As a critical quality attribute (CQA), bioactivity assay should be fully validated and mechanism-of-action (MOA) based to meet the need of lot release and regulatory expectation.17 Bioactivity is also extensively applied in the screening, characterization, stability, comparability and biosimilarity study.
Competitive ELISA was reported to determine the bioactivity of anti-RANKL mAbs.18 However, ELISA only involves the initial step of binding, which is far from the end point of the biological response, so it could not completely represent the MOA of anti-RANKL mAbs. As for bioactivity determination of anti-RANKL mAbs with cell based methods, calcification of porcine valvular interstitial cells was checked by Alizarin red staining,19 and maturation of patients-derived and mice-derived osteoclast were indicated by tartrate resistant acid phosphatase (TRAP) activity,20 while both of which are unsuitable for routine bioassay due to the employment of primary cells and the resultant high variability. RANKL-induced osteoclastogenesis of RAW264.7 cells was suppressed by anti-RANKL mAbs by measurement of TRAP activity, but it took five to seven days to perform the whole bioassay, making the bioassay less favorable for the release of anti-RANKL mAbs.
It was reported that RANKL-induced NF-κB-driven luciferase assay based on RAW264.7 cells could reflect the RANKL bioactivity as the osteoclastogenesis assay reflected by TRAP activity in the same cell type.21–31 However, this simple assay format was only applied in the research field for the study of RANKL, but never established, optimized or validated to determine whether it is suitable or not to measure the bioactivity of anti-RANKL mAbs. In the study, we screened out a highly RANKL-responsive RAW264.7 cell variant stably transfected with NF-κB-driven luciferase. After the establishment of RGA, we optimized and validated the methodology, and proved that the method had good assay performance such as specificity, linearity, precision and accuracy, which is suitable for the bioactivity determination of anti-RANKL mAbs.
2. Materials and methods
2.1 Cell lines and reagents
RAW264.7 cells were purchased from ATCC. Recombinant Human TRANCE (sRANKL) was purchased from TONBO biosciences, PBS, FBS, DMEM, 0.05% trypsin–EDTA (1×), penicillin–streptomycin solution (P/S) and glutamine from Gibco, pCM1.1 (Luc/NF-κB/Hygro) plasmid from Promega, hygromycin B from Roche, Bright-Glo™ luciferase detection reagent from Promega, and denosumab from Amgen.
2.2 Cell culture
The parental RAW264.7 cells were maintained in DMEM with 10% FBS, 1% P/S, 1% glutamine, while the transgenic cells NF-κB-Luci RAW264.7 cells were cultured in DMEM with 10% FBS, 1% P/S, 1% glutamine and 150 μg ml−1 hygromycin B. During the bioassay, DMEM medium containing 10% FBS was employed as the assay medium.
2.3 Establishment of NF-κB-Luci RAW264.7 cells
RAW264.7 cells were transfected with the vector of pCM1.1 (Luc/NF-κB/Hygro), followed by selection of 150 μg ml−1 hygromycin B 24 h post transfection. The hygromycin resistant stable pool was then subcloned by limited dilution, and various single cell derived variants were further screened by the addition of RANKL and the measurement of luciferase expression to choose the best responsive variant (NF-κB-Luci RAW264.7 cells).
2.4 RGA method
NF-κB-Luci RAW264.7 cells were seeded at the density of 8 × 104 cells in 50 μl assay medium per well. Anti-RANKL mAbs were sequentially diluted in 1
:
3 for two dilutions, 1
:
1.5 for five dilutions, and 1
:
3 for another two dilutions with the starting concentration of 6000 ng ml−1. The ten serially diluted samples were added at the volume of 50 μl per well, followed by incubation at 37 °C and 5% CO2 for 24 h. After addition of 100 μl per well of the reconstituted Bright-Glo™, relative luciferase units (RLU) were recorded with luminometer.
2.5 Linearity, accuracy and precision
Five potency levels were designed to validate linearity, accuracy and precision, i.e., 50%, 75%, 100%, 125% and 150%, which corresponded to the concentrations of 3000, 4500, 6000, 7500 and 9000 ng ml−1 denosumab respectively, with 6000 ng ml−1 as the reference standard. Under the optimized assay conditions, each concentration of the samples was tested for three times.
2.6 The classical TRAP activity measurement assay
The parental RAW264.7 cells were seeded to 96-well plates with the cell number of 1500 in 150 μl assay medium per well, followed by incubation for 2–3 hours at 37 °C and 5% CO2. Both 40 μl of the serially diluted denosumab and 40 ng ml−1 sRANKL were added. After incubation for four days, 100 μl citrate buffer containing 0.2% Triton-X-100 per well was added, and the plate was shaken for 45 minutes to perforate the cells. Then 50 μl p-nitrophenyl phosphate (pNPP) substrate solution per well was added to the plates, and incubated for 80 minutes. The reaction was terminated with 50 μl of 2 M NaOH solution per well. Finally, the absorbance values were measured at 405 nm wavelength.
2.7 Data analysis
Four-parameter model was employed to fit the dose–response relationship between RLU and log10 of the antibody concentration, while the relative potency is expressed as half maximal effective concentration (EC50) ratio of the reference standard versus the sample.
3. Results
3.1 Generation of NF-κB-Luci RAW264.7 cells
We transfected the vector of pCM1.1 (Luc/NF-κB/Hygro) into the parental RAW264.7 cells. Various cell variants cloned from single cell derived from the hygromycin B resistant pool were analyzed for luciferase expression induced by sRANKL.
As shown in Fig. 1, among the twelve individual clones, clone 1D6 showed the highest fold induction (FI) of luciferase, and was named NF-κB-Luci RAW264.7 cells for the subsequent optimization and validation of the RGA.
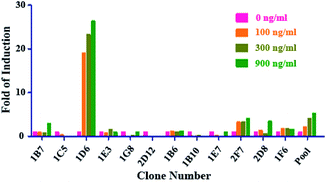 |
| Fig. 1 The establishment of NF-κB-Luci RAW264.7 cells. After transfection of vector containing NF-κB driven luciferase gene, various single cell derived variants survived the hygromycin selection pressure were further screened by the addition of three different concentrations of sRANKL (100, 300, and 900 ng ml−1) and measurement of luciferase expression. | |
3.2 Optimization of the RGA
Firstly, we generated the full dose response curve with serial dilution of sRANKL, as shown in Fig. 2A. We chose EC90, EC75, EC50 and EC25 of sRANKL, which corresponded to about 1000, 200, 100, 50 ng ml−1, as the fixed concentration for the next denosumab neutralization bioassay as shown in Fig. 2B. Due to steep curve slope of 1000 ng ml−1, and similar signal-to noise ratio between 200, 100, 50 ng ml−1, we set 50 ng ml−1 of sRANKL as the fixed concentration for the next step of optimization of denosumab RGA.
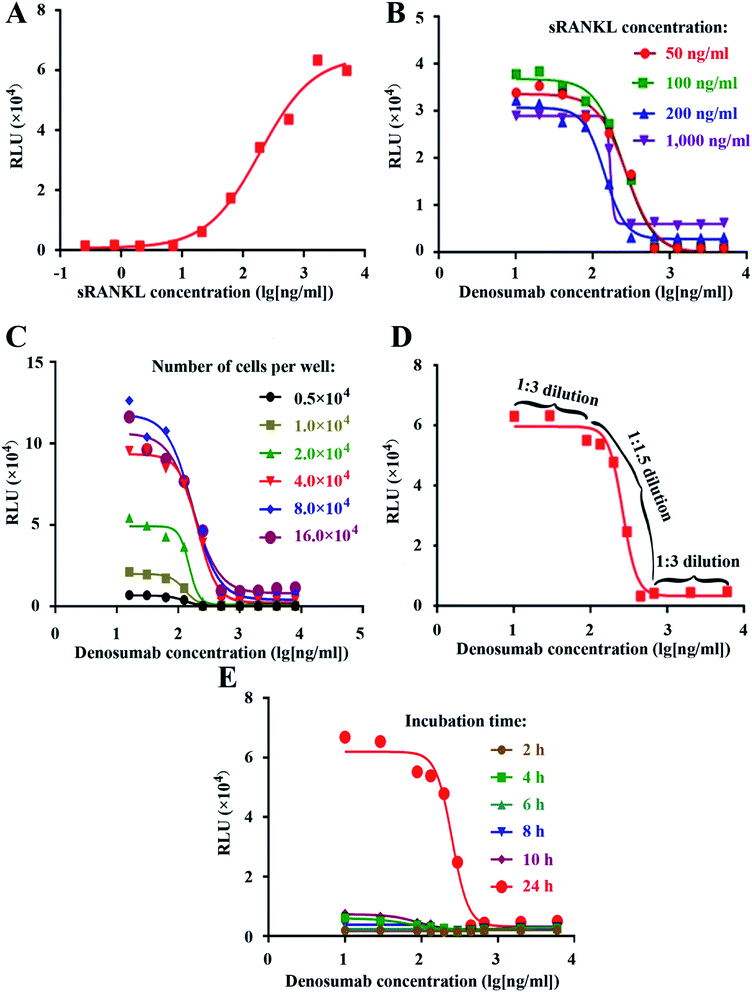 |
| Fig. 2 Establishment and optimization of the RGA. The dose–responsive curves of sRANKL (A) and anti-RANKL mAb in the presence of different fixed concentrations of sRANKL as indicated (B). In the presence of 50 ng ml−1 sRANKL, the dose–responsive curves of anti-RANKL mAb with the different cell number per well (C), anti-RANKL mAb with optimized starting concentration and unequally serial dilution as indicated (D), and anti-RANKL mAb with the different time of incubation as indicated before the addition of luciferase substrate and luciferase intensity measurement (E). | |
Next, we optimized several key experimental parameters one by one, including cell number per well, the initial concentration and dilution ratio of denosumab, and incubation time. NF-κB-Luci RAW264.7 cells were seeded into 96-well plate at the density of 0.5 × 104, 1.0 × 104, 2.0 × 104, 4.0 × 104, 8.0 × 104 and 16.0 × 104 cells per well, followed by addition of 50 ng ml−1 of sRANKL and serial dilution of denosumab, while no big difference of signal-to-noise ratio was observed as shown in Fig. 2C. For cost effectiveness, the condition of 4.0 × 104 cells per well was chosen.
Since the linear part of the fitting curve was within only one log of magnitude, to generate an optimal and stable curve, we set the dilution fold as 1
:
1.5 within the five serial dilutions in the linear parts, while as 1
:
3 within the each of the two dilutions flanking the linear parts in the upper and lower asymptotes. The experimental curve with a total of ten serial dilutions demonstrated to be an optimal one (Fig. 2D), proving the theoretical design. The attempt was also made to set the constant dilution fold as 1
:
1.5, 1
:
2 and 1
:
3, but failed (data not shown).
The luciferase intensity and signal-to-noise ratio were proved to be the highest at 24 h other than 2, 4, 6, 8, and 10, so we chose and set this incubation time for the RGA.
3.3 Validation of the RGA
Based on the ICH-Q2 guidelines, we performed validation on the optimized RGA method, including specificity, linearity, accuracy, and precision.
Native but denatured denosumab could inhibit the luciferase expression induced by sRANKL (Fig. 3A), and bevacizumab, trastuzumab, basiliximab, rituximab, or infliximab all failed to generate the dose–response curve (Fig. 3B), which proved the specificity of the established RGA.
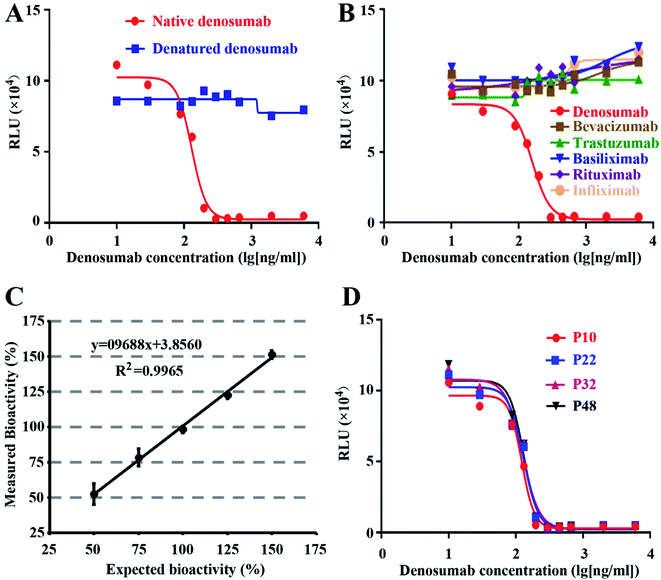 |
| Fig. 3 Specificity, linearity, accuracy, and stability of the bioassay. Dose–response curve of the native and denatured denosumab (A), and various mAbs as indicated (B). The closeness of agreement between the measured and the expected bioactivity of denosumab, and each point represents the mean of three replicates (C). The dose–responsive generated with different passages of NF-κB-Luci RAW264.7 cells (D). | |
Precision was accessed at two levels, i.e., intra-assay (repeatability) and inter-assay precision. Three repeated analyses demonstrated the plate-to-plate variability as 7.76%. Three repeated analyses conducted in three different days displayed the inter-assay precision as 1.58%. Our data proved the excellent precision of the established RGA.
Accuracy expresses the closeness of agreement between the measured and expected values. Five different denosumab samples were subject to the analysis of bioactivity, with the initial concentration of 50%, 75%, 100%, 125% and 150% respectively as that of the established RGA. With another sample with 100% level as the reference, the relative bioactivity of the five samples was measured. The recovery rates varied from 98.10% to 105.24%. The closeness of agreement between the measured and expected bioactivity proved the excellent accuracy and linearity of the established bioassay (Fig. 3C). Furthermore, the accuracy evaluation was repeated three times, and the maximum RSD value of the five measured relative bioactivity is 7.58%.
3.4 Stability of cell lines
To evaluate the stability of the cell line, cells frozen at different passages during continuous culture were thawed at the same time, and subject to the performance evaluation of the established RGA. As shown in Fig. 3D, the dose–response curves of the cells at passage 10, 22, 32 and 48 nearly coincided with one another, suggesting the negligible effect of the passage number on responsiveness of NF-κB-Luci RAW264.7 cells.
3.5 Wide applicability of the RGA
As shown in Fig. 4A, all the six mAbs from six companies exhibited good dose-dependent responses, demonstrating the wide applicability of RGA to the mAbs targeting RANKL.
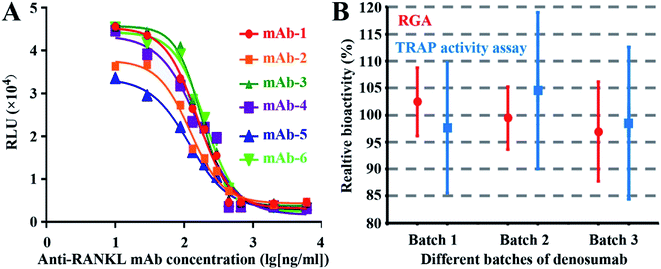 |
| Fig. 4 The wide applicability of the RGA and the comparison between the RGA and traditional TRAP activity measurement assay. Dose–response curve of six different anti-RANKL mAbs from six companies (A), the mean values and standard deviations of relative bioactivities (six independent assays) of three batches of denosumab with both the established RGA and the traditional TRAP activity measurement assay (B). | |
3.6 Advantage of the RGA over the anti-proliferation assay
To evaluate the agreement between the novel RGA and classical TRAP activity measurement assay, relative bioactivities of three batches of denosumab were determined against another batch as the reference for six times by both assays. No significant difference was found, indicating the consistency of the two assays. However, the smaller CV value suggests that the RGA is more precise (Fig. 4B) and time-saving (Table 1), and the wider linear range and the higher maximal FI (fold of induction) of the dose–response curves proved the higher sensitivity of the RGA upon the TRAP activity measurement assay.
Table 1 Comparison of the RGA with the TRAP activity assay
Parameter |
RGA |
TRAP activity assay |
Experiment duration |
1 day |
5 days |
Coefficient of correlation (R2) |
>0.99 |
>0.97 |
Linear range (ng ml−1) |
88–2000 |
100–1500 |
Maximal FI |
∼15 |
∼2.5 |
Precision (intra- and inter-) |
<9% |
>11% |
4. Discussion
Upon interaction, soluble RANKL trimer induces the trimerization of RANK on osteoblast membrane,32,33 contributing to the recruitment and activation of TNF receptor-associated factors 6 (TRAF6).34 The activated TRAF6 binds to the scaffolding protein p62, and p62 further interacts with atypical protein kinase C (aPKC). The activated TRAF6 also associates with TGFβ-activated kinase 1 (TAK1), and TAK1 further interacts with the adaptor proteins TAK1-binding protein 1 (TAB1) and TAB2. The two multimeric complexes, TRAF6–p62–aPKC and TRAF6–TAK1–TABs, can both activate the IκB kinase (IKK) complex, which contains IKK1/IKKα, IKK2/IKKβ, and the non-catalytic accessory protein NF-κB Essential Modulator (NEMO)/IKKγ. After phosphorylation by IKK2, the NF-κB inhibitory protein, IκB, is ubiquitinated and undergoes proteasomal degradation. The release of IκB results in the translocation of p65/RelA and p50/NF-κB1 dimer to nucleus and translation of various genes related to osteoclastogenesis.35,36 RANK trimer also competitively binds with TRAF2/3 and induces their degradation, leading to the release of NF-κB-inducing kinase (NIK) from the degradative complex which consists of cellular inhibitors of apoptosis 1 and 2 (cIAP1/2) and TRAF2/3. The stabilized NIK from constitutive ubiquitination and degradation induces the phosphorylation and activation of IKKα, while both NIK and IKKd stimulate the proteolysis of p100 to p52/NF-κB2. Heterodimer of NF-κB2 and RelB then translocate to the nucleus and mediates the transcription of osteoclastogenesis-related genes.37 Besides the canonical NF-κB1 and the noncanonical NF-κB2 pathway, various signal transduction pathways are also activated upon the RANK and RANKL interaction.38–40
It was reported that mice with combined deletion of NF-κB1/p50 and NF-κB2/p52 subunits displayed severe osteopetrosis due to complete deficiency of osteoclasts.41,42 Inhibition of NF-κB pathway also suppressed the RANKL-induced osteoclastogenesis of RAW264.7 cells.23,43 All these documentations underlie the indispensability of NF-κB pathways during the osteoclastogenesis, which proves the validity of the use of NF-κB activation as the surrogate of end point of osteoclastogenesis. RAW264.7 cells, naturally expressing receptor RANK,44 are mouse-derived osteoclast precursor cells originally derived from tumors caused by Abelson mouse leukemia virus.45 The stable transfection of NF-κB driven luciferase gene into the cells renders the cells an excellent model to study the bioactivity of RANKL and anti-RANKL mAbs, since NF-κB-driven luciferase intensity is proportional to the extent of differentiation of RAW264.7 cells.
We selected commercial denosumab for optimization and validation of the RGA. Various assay performances were evaluated according to the ICH-Q2 guidelines for analytical method validation.16 Excellent specificity, precision, linearity, accuracy, and passage stability of the bioassay were demonstrated, proving the good applicability of the RGA to the bioactivity determination of anti-RANKL mAbs.
The activity measurement of TRAP46 has been used as an index of osteoclast activity in more than 50 years.47 We compared our novel RGA method to the traditional TRAP activity measurement method,48 which demonstrates the simpler operation, much shorter duration, higher signal-to noise ratio and relatively lower variation of the RGA.
5. Conclusions
This study is the first to measure the biological activity of anti-RANKL mAb based on the NF-κB-driven luciferase. The novel RGA not only shows superior sensitivity, precision, linearity and robustness, but also has significant advantage on assay simplicity (shorter assay time and simpler operation), compared to the traditional TRAP activity measurement assay. Our study provides a suitable RGA to measure the bioactivity of anti-RANKL mAbs, which would surely benefit the R&D and quality control of anti-RANKL mAbs.
Conflicts of interest
There are no conflicts to declare.
Acknowledgements
This work was supported by National Major Scientific and Technological Special Project for ‘Significant New Drugs Development’ (Grant number: 2018ZX09736016-007) and Research Project for Research Institutes for Public Welfare of the Chinese Academy of Medical Sciences.
References
- P. Grabowski, Endocr. Dev., 2009, 16, 32–48 CrossRef CAS.
- R. Florencio-Silva, G. R. Sasso, E. Sasso-Cerri, M. J. Simoes and P. S. Cerri, BioMed Res. Int., 2015, 2015, 421746 CrossRef.
- B. J. Kim and J. M. Koh, Cell. Mol. Life Sci., 2019, 76, 1243–1253 CrossRef CAS.
- V. Nagy and J. M. Penninger, Gerontology, 2015, 61, 534–542 CrossRef CAS PubMed.
- S. Rao, S. J. F. Cronin, V. Sigl and J. M. Penninger, Trends Cell Biol., 2018, 28, 213–223 CrossRef CAS.
- W. S. Simonet, D. L. Lacey, C. R. Dunstan, M. Kelley, M. S. Chang, R. Luthy, H. Q. Nguyen, S. Wooden, L. Bennett, T. Boone, G. Shimamoto, M. DeRose, R. Elliott, A. Colombero, H. L. Tan, G. Trail, J. Sullivan, E. Davy, N. Bucay, L. Renshaw-Gegg, T. M. Hughes, D. Hill, W. Pattison, P. Campbell, S. Sander, G. Van, J. Tarpley, P. Derby, R. Lee and W. J. Boyle, Cell, 1997, 89, 309–319 CrossRef CAS.
- D. L. Lacey, E. Timms, H. L. Tan, M. J. Kelley, C. R. Dunstan, T. Burgess, R. Elliott, A. Colombero, G. Elliott, S. Scully, H. Hsu, J. Sullivan, N. Hawkins, E. Davy, C. Capparelli, A. Eli, Y. X. Qian, S. Kaufman, I. Sarosi, V. Shalhoub, G. Senaldi, J. Guo, J. Delaney and W. J. Boyle, Cell, 1998, 93, 165–176 CrossRef CAS.
- C. C. Lynch, A. Hikosaka, H. B. Acuff, M. D. Martin, N. Kawai, R. K. Singh, T. C. Vargo-Gogola, J. L. Begtrup, T. E. Peterson, B. Fingleton, T. Shirai, L. M. Matrisian and M. Futakuchi, Cancer Cell, 2005, 7, 485–496 CrossRef CAS.
- A. Hikita, I. Yana, H. Wakeyama, M. Nakamura, Y. Kadono, Y. Oshima, K. Nakamura, M. Seiki and S. Tanaka, J. Biol. Chem., 2006, 281, 36846–36855 CrossRef CAS.
- C. A. O'Brien, Bone, 2010, 46, 911–919 CrossRef.
- T. J. Martin and N. A. Sims, Rev. Endocr. Metab. Disord., 2015, 16, 131–139 CrossRef CAS PubMed.
- S. H. Tella and J. C. Gallagher, J. Steroid Biochem. Mol. Biol., 2014, 142, 155–170 CrossRef CAS PubMed.
- K. E. Ensrud and C. J. Crandall, Ann. Intern. Med., 2017, 167, ITC17–ITC32 CrossRef PubMed.
- P. J. Bekker, D. Holloway, A. Nakanishi, M. Arrighi, P. T. Leese and C. R. Dunstan, J. Bone Miner. Res., 2001, 16, 348–360 CrossRef CAS.
- S. C. Pageau, mAbs, 2009, 1, 210–215 CrossRef.
- http://www.ich.org/fileadmin/Public_Web_Site/ICH_Products/Guidelines/Quality/Q2_R1/Step4/Q2_R1__Guideline.pdf, 2005.
- https://www.ich.org/fileadmin/Public_Web_Site/ICH_Products/Guidelines/Quality/Q6B/Step4/Q6B_Guideline.pdf, 1999.
- R. Pourvasei, E. Lee, M. Eschenberg, V. Patel, C. Macaraeg, K. Pandya, J. Shih, M. Ma, J. W. Lee and B. DeSilva, AAPS J., 2013, 15, 70–77 CrossRef CAS.
- D. A. Lerman, S. Prasad and N. Alotti, Int. J. Cardiovasc. Res., 2016, 5(1) DOI:10.4172/2324-8602.1000249.
- I. Shibuya, M. Takami, A. Miyamoto, A. Karakawa, A. Dezawa, S. Nakamura and R. Kamijo, Pathol. Oncol. Res., 2019, 25, 409–419 CrossRef CAS.
- J. Huang, L. Zhou, H. Wu, N. Pavlos, S. M. Chim, Q. Liu, J. Zhao, W. Xue, R. X. Tan, J. Ye, J. Xu, E. S. Ang, H. Feng, J. Tickner, J. Xu and Y. Ding, Mol. Cell. Endocrinol., 2015, 399, 346–353 CrossRef CAS.
- X. Zeng, Y. Zhang, S. Wang, K. Wang, L. Tao, M. Zou, N. Chen, J. Xu, S. Liu and X. Li, Biochem. Pharmacol., 2017, 124, 57–68 CrossRef CAS.
- Q. Liu, H. Wu, S. M. Chim, L. Zhou, J. Zhao, H. Feng, Q. Wei, Q. Wang, M. H. Zheng, R. X. Tan, Q. Gu, J. Xu, N. Pavlos, J. Tickner and J. Xu, Biochem. Pharmacol., 2013, 86, 1775–1783 CrossRef CAS.
- M. Yamaguchi, M. N. Weitzmann and T. Murata, Mol. Cell. Biochem., 2012, 359, 193–203 CrossRef CAS PubMed.
- H. Y. Tsai, H. Y. Lin, Y. C. Fong, J. B. Wu, Y. F. Chen, M. Tsuzuki and C. H. Tang, Eur. J. Pharmacol., 2008, 588, 124–133 CrossRef CAS PubMed.
- I. Voronov, K. Li, H. C. Tenenbaum and M. F. Manolson, Biochem. Pharmacol., 2008, 75, 2034–2044 CrossRef CAS PubMed.
- P. P. Singh, A. G. van der Kraan, J. Xu, M. T. Gillespie and J. M. Quinn, Biochem. Biophys. Res. Commun., 2012, 422, 48–53 CrossRef CAS.
- E. S. Ang, N. J. Pavlos, S. M. Chim, H. T. Feng, R. M. Scaife, J. H. Steer, M. H. Zheng and J. Xu, J. Cell. Biochem., 2012, 113, 946–955 CrossRef CAS.
- X. Wu, G. Pan, M. A. McKenna, M. Zayzafoon, W. C. Xiong and J. M. McDonald, J. Bone Miner. Res., 2005, 20, 107–116 CrossRef CAS.
- S. C. Liu, S. M. Chuang and C. H. Tang, Int. Immunopharmacol., 2012, 12, 494–500 CrossRef CAS.
- C. H. Tang, C. S. Chang, T. W. Tan, S. C. Liu and J. F. Liu, Eur. J. Pharmacol., 2010, 648, 59–66 CrossRef CAS.
- S. Zhang, C. Liu, P. Huang, S. Zhou, J. Ren, Y. Kitamura, P. Tang, Z. Bi and B. Gao, Arch. Biochem. Biophys., 2009, 487, 49–53 CrossRef CAS.
- S. Ito and T. Hata, Vitam. Horm., 2004, 67, 19–33 CrossRef CAS.
- N. Kim, Y. Kadono, M. Takami, J. Lee, S. H. Lee, F. Okada, J. H. Kim, T. Kobayashi, P. R. Odgren, H. Nakano, W. C. Yeh, S. K. Lee, J. A. Lorenzo and Y. Choi, J. Exp. Med., 2005, 202, 589–595 CrossRef CAS.
- N. S. Soysa, N. Alles, H. Shimokawa, E. Jimi, K. Aoki and K. Ohya, J. Bone Miner. Metab., 2009, 27, 131–139 CrossRef CAS.
- J. Xu, H. F. Wu, E. S. Ang, K. Yip, M. Woloszyn, M. H. Zheng and R. X. Tan, Cytokine Growth Factor Rev., 2009, 20, 7–17 CrossRef CAS.
- A. Sabokbar, D. J. Mahoney, F. Hemingway and N. A. Athanasou, Clin. Rev. Allergy Immunol., 2016, 51, 16–26 CrossRef CAS PubMed.
- Y. Abu-Amer, Osteoporosis Int., 2013, 24, 2377–2386 CrossRef CAS PubMed.
- B. F. Boyce, Y. Xiu, J. Li, L. Xing and Z. Yao, Endocrinol. Metab., 2015, 30, 35–44 CrossRef CAS PubMed.
- J. H. Park, N. K. Lee and S. Y. Lee, Mol. Cells, 2017, 40, 706–713 CAS.
- V. Iotsova, J. Caamano, J. Loy, Y. Yang, A. Lewin and R. Bravo, Nat. Med., 1997, 3, 1285–1289 CrossRef CAS PubMed.
- G. Franzoso, L. Carlson, L. Xing, L. Poljak, E. W. Shores, K. D. Brown, A. Leonardi, T. Tran, B. F. Boyce and U. Siebenlist, Genes Dev., 1997, 11, 3482–3496 CrossRef CAS.
- X. Z. Zeng, L. G. He, S. Wang, K. Wang, Y. Y. Zhang, L. Tao, X. J. Li and S. W. Liu, Acta Pharmacol. Sin., 2016, 37, 255–263 CrossRef CAS.
- B. L. Cuetara, T. N. Crotti, A. J. O'Donoghue and K. P. McHugh, In Vitro Cell. Dev. Biol.: Anim., 2006, 42, 182–188 CrossRef CAS.
- W. C. Raschke, S. Baird, P. Ralph and I. Nakoinz, Cell, 1978, 15, 261–267 CrossRef CAS.
- A. J. Janckila and L. T. Yam, Calcif. Tissue Int., 2009, 85, 465–483 CrossRef CAS.
- A. R. Hayman, Autoimmunity, 2008, 41, 218–223 CrossRef CAS.
- J. M. Halleen, H. Kaija, J. J. Stepan, P. Vihko and H. K. Vaananen, Arch. Biochem. Biophys., 1998, 352, 97–102 CrossRef CAS PubMed.
Footnote |
† These authors contributed equally to this work. |
|
This journal is © The Royal Society of Chemistry 2019 |
Click here to see how this site uses Cookies. View our privacy policy here.