DOI:
10.1039/C9SC00961B
(Edge Article)
Chem. Sci., 2019,
10, 5495-5504
Multiplexed immunophenotyping of circulating exosomes on nano-engineered ExoProfile chip towards early diagnosis of cancer†
Received
25th February 2019
, Accepted 19th April 2019
First published on 22nd April 2019
Abstract
Circulating exosomes have been studied as a promising biomarker for non-invasive cancer diagnosis, as they are implicated in tumor initiation, progression, and metastasis. However, the clinical significance of circulating exosomes has not been revealed thoroughly, due to the technical limitation in sensitive and multiplexed detection of cargoes on exosomes, such as proteins and nucleic acids. Herein we developed an integrated exosome profiling platform (ExoProfile chip) to afford superior sensitivity and multiplexed capability for quantitative detection of a panel of surface protein markers on exosomes. To achieve this goal, we innovatively constructed 3D porous serpentine nanostructures via patterned colloidal self-assembly to provide enormous reaction sites and improve biosensing efficiency of exosomes. Meanwhile, the switchable microfluidic design enabled the simultaneous detection of eight markers on single addition of exosome samples. The ExoProfile chip was validated with purified exosomes from SKOV3 cells, which yielded a limit of detection of 21 exosomes per μL. We applied the ExoProfile chip to clinical analysis of circulating exosomes using only 10 μL ovarian cancer plasma and completing the analysis within 3 h. The diagnostic power of seven markers (EGFR, HER2, CA125, FRα, CD24, EpCAM, and CD9 plus CD63) were evaluated with receiver operator characteristic curve and heatmap clustering. Compared to single biomarker, the combined assessment of a biomarker panel was demonstrated to display improved accuracy in distinguishing early and late stage cancer. The results suggested the ExoProfile chip as a promising platform for molecular fingerprinting of circulating exosomes towards early cancer diagnosis.
Introduction
Exosomes of 30–150 nm in size are a distinct population of extracellular vesicles (EVs) actively secreted by nearly all eukaryotic cells.1,2 They are released by fusion of multivesicular endosomes with the plasma membrane, carry a cargo of proteins, nucleic acids and lipids from their parental cells, and participate in biological functions such as intercellular communication and immune response.3 Recent studies show exosomes correlated well with tumor progression, angiogenesis and metastasis,4,5 thus hold great potential to act as a liquid biopsy biomarker for cancer diagnosis.6–8 Recently, molecular probing of tumor-derived circulating exosomes has been demonstrated to aid in non-invasive cancer diagnosis and monitoring of treatment response,9–11 including CD147 for colorectal cancer,12 NY-ESO-1 for lung cancer,13 glypican-1 for pancreatic cancer,14 PSA for prostate cancer,15 CD24 and CA-125 for ovarian cancer.16,17 However, the discovery of more potential exosomal protein markers was limited by detection sensitivity of current exosome assays, especially for low abundance proteins on exosomes. Meanwhile, due to the phenotypic heterogeneity of circulating exosomes, a “biomarker panel” had improved accuracy and specificity for liquid biopsy diagnosis compared to single markers.18–20 Therefore, the development of innovative exosome assay with much improved sensitivity and multiplexed detection capacity was urgently needed to expand the clinical utility of circulating exosomes on routine diagnosis of cancer.
The conventional exosome assays consist of ultracentrifugation-based isolation and downstream Western blot & ELISA molecular characterization. Ultracentrifugation is time-consuming and yields low recovery and poor purity.21,22 The benchtop molecular characterization assays also hold poor sensitivity in the detection of exosomal proteins. As a result, large amount of biofluid samples (>1 mL plasma) were consumed for each assay, while most of low-abundance biomarkers were unable to detect. In the past decades, integrated microfluidic platforms emerged to carry out exosomes isolation and in situ molecular detection with much improved efficiency and sensitivity.16,20,23–32 Lee's group developed a nanoplasmonic exosome (nPLEX) assay platform for label-free, surface plasmon resonance (SPR) sensing of exosome surface proteins.17 The periodic nanoholes array enabled efficient capture and sensitive detection of ∼3000 exosomes. Stott et al. reported a sensitive analytical microfluidic platform (EVHB-Chip) with engineered nanointerfaces that enables tumor-specific EV isolation within 3 h and achieved 94% tumor-EV specificity and a limit of detection of 100 EVs per μL.33 In our group, we previously used nanomaterials to modify chip interface and developed a GO/PDA based exosome analysis platform (nano-IMEX).34 The 2D GO/PDA nanosheets increased the surface area and improved the surface binding efficiency, which endowed the platform with high sensitivity for integrated exosome analysis. We recently developed a novel strategy termed multiscale integration by designed self-assembly (MINDS)35 to overcome the fundamental limits of microfluidic biosensing in mass transfer, surface reaction and boundary effects simultaneously. Using the MINDS strategy, we built a 3D nanostructured herringbone (nano-HB) chip which immensely improved the sensitivity for exosome detection.35
In this work, we expand the MINDS approach to develop a multi-channel, integrated exosome profiling platform (ExoProfile) for multiplexed, sensitive and high-throughput immunophenotyping of circulating exosomes in plasma samples. The ExoProfile chip presents three distinctions from our previous nano-HB chip. First, we expanded the low cost, lithography-free MINDS approach to 3D nano-engineering of a classic 2D mixer, serpentine channel that induces secondary Dean Flow and local vortices to enhance microflow mixing.36 Compared to the 3D mixing microstructures like HBs, such planar mixers afford structural simplicity and easy fabrication to devise a robust, integrated lab-on-a-chip system. Second, the ExoProfile chip pneumatically automates the entire assay pipeline of exosome capture and molecular analysis, offering the “sample-in-answer-out” capability to enhance the rigor and reproducibility. Last, the new multi-channel microsystem enables high-throughput, multi-marker immunophenotyping of circulating exosomes to improve the accuracy of cancer diagnosis. We measured surface markers of exosomes from ovarian cancer cell line SKOV3 on ExoProfile chip, using sandwich structured enzymatic fluorescent amplification reaction to boost sensitivity. For exosomal markers such as CD9, CD63 and CD81, the limit of detection reaches to about 21 exosomes per μL, which is better than the nano-IMEX chip34 and other published work.17,33 The superior sensitivity and multiplexed property of ExoProfile chip also enabled us to profile other tumor related biomarkers quantitatively and simultaneously, such as EGFR, HER2, CA125, EpCAM, CD24 and FRα. We applied the ExoProfile chip for molecular profiling of circulating exosomes on clinical setting, using only 10 μL ovarian cancer plasma and completing the analysis within 3 h. The combined assessment of the seven biomarkers panel on exosomes not only discriminated patient and benign group on a cohort of 20 samples, but also classified early-stage and late-stage ovarian cancer patients successfully. The results indicated that the ExoProfile chip holds great potential for circulating exosome-based cancer diagnosis.
Experimental section
Reagents and materials
Silica microbeads (1.0 μm) were ordered from Bangs Laboratories Inc. (3-Mercaptopropyl)trimethoxysilane (3-MPS) and 4-maleimidobutyric acid N-hydroxysuccinimide ester (GMBS) were purchased from Sigma-Aldrich; streptavidin conjugated β-galactosidase (SβG), fluorescein di-β-D-galactopyranoside (FDG) were received from Life Technology. The detailed information of various capture and detection antibodies in our experiment was list in Table S1.† The ExoTEST™ Ready-to-Use Kit for microplate ELISA testing was purchased from HansaBioMed, Ltd (Tallinn, Estonia). SuperBlock blocking buffer was ordered from Thermo Scientific; 1× phosphate-buffered saline (PBS) was from Mediatech, Inc.; all other solutions were prepared with deionized water (18.2 MΩ cm, Thermo Scientific). SβG and FDG were dissolved in PBS working solution (PBSW) at pH 7.4 PBS which contain 0.5 mM DL-dithiothreitol solution (Sigma-Aldrich), 2 mM MgCl2 (Fluka Analytical), and 0.5% bovine serum albumin (BSA) (Sigma-Aldrich).
Microfabrication of ExoProfile chip
Standard soft photolithography was used for the microfabrication of ExoProfile chip, which contains several PDMS layers, such as pneumatic layer, fluidic layer, beads pattern layer and antibody immobilization layer. The feature of each PDMS layer was shown in Fig. S1† and parameters were summarized in Table S2.† PDMS molds were prepared with SU-8 series photoresist, following the manual of products. The whole procedure consists of spin-coating of photoresist, pre-baking, UV exposure, post-baking, developing and hard baking. After that, the prepared molds were treated with trimethylchlorosilane under vacuum for 8 h. To fabricate PDMS chip of pneumatic layer, 30 g PDMS mixture at a 7 (base material)
:
1 (curing agent) ratio was poured on the mold and cured in the oven at 70 °C for 2 h. PDMS pieces were cut and peeled off from the mold. Meanwhile, the fluidic layer was prepared by mixing 5 g PDMS at a ratio of 15
:
1 and spincoating over the mold at 1000 rpm for 45 s, followed by curing on 70 °C hotplate for 30 min. The pneumatic layer was then manually aligned under a stereomicroscope and permanently bonded with the bottom fluidic layer by baking in the 70 °C oven overnight.
For the fabrication of serpentine nanostructures, beads pattern chip was sealed with clean glass slide to guide colloidal self-assembly. After 10 min sonication, 10% w/v aqueous suspensions of monodisperse silica beads were added into the buffer reservoir. The silica colloids filled the serpentine channels and then the buffer reservoir was sealed with small piece of PDMS chunk. Water will only evaporate from the other ending of serpentine channels and thus induce close packing of silica beads along serpentine channels. After the formation of self-assembled nanostructures, colloidal suspension in the reservoir was then replaced with 5% 3-MPS in ethanol. The silane reaction between silica beads connected them each other and thus strengthened the self-assembled structures sufficiently. After drying, the pattern chip was peeled off carefully.
A patterning chip was used to define the antibody immobilization area. After aligning and bonding with the self-assembled nanostructured channel arrays, 5% 3-MPS in anhydrous ethanol solution was filled the chip and reacted for 1 h. Excess silane was washed away with 70% ethanol for three times. 0.28 mg mL−1 GMBS was reacted for 0.5 h and used as linker to immobilize antibody. After wash with PBS, 0.1 mg mL−1 capture antibody CD81 was flowed through and reacted for 1 h at room temperature. Afterward, the pattern chip was removed and ExoProfile chip was aligned eventually. Before use, the chip was blocked with 5% BSA and stored at 4 °C.
Cell culture and exosome isolation
The ovarian cancer cell line SKOV3 was cultured at 37 °C under a 5% humidified CO2 atmosphere, using RPMI-1640 media supplemented with 10% (v/v) exosome-depleted FBS, recombinant insulin (7.5 μg mL−1), penicillin (100 U mL−1), and streptomycin (100 μg mL−1). Cell line was cultured until cellular sub-confluency of ∼70% and then the conditioned media was collected. Exosomes were isolated by standard ultracentrifugation following previously report. Briefly, conditioned media were collected and centrifuged at 2500 × g for 5 min to remove cellular debris. The supernatant was then ultracentrifuged at 4 °C for 45 minutes at 10
000 × g to remove microvesicles and again at 100
000 × g for 2 h to pellet exosomes. Exosome pellets were then resuspended in 10 mL of PBS for a wash step and then collected again with ultracentrifugation at 4 °C for 60 min at 110
000 × g in Beckman Coulter Quik-Seal Centrifuge Tubes. After aspiration of the PBS supernatant, the exosome pellet was resuspended in 100 μL PBS.
Molecular profiling assay of exosomes
The stock solution of isolated SKOV3 exosomes was aliquoted and stored at −80 °C. NTA was tested to estimate the concentration of exosomes. For single exosome detection assay, 50 μL of exosomes solution was pumped through chip by homemade pump system with a constant flow rate. Non-captured exosomes were washed away with the Superblock buffer. The on-chip captured exosomes were then detected by the biotinylated detection antibodies, which contained a mixture of biotin-CD9 (20 μg mL−1), biotin-CD63 (20 μg mL−1) and biotin-CD81 (20 μg mL−1). After attachment of detection antibodies, streptavidin conjugated β-galactosidase (SβG) prepared in PBSW buffer (20 ng mL−1) was introduced as the reporter enzyme for chemifluorescence detection. Subsequently, the enzyme substrate di-β-D-galactopyranoside (FDG) (500 μM) was introduced in to the assay chambers. Fluorescence images were taken after 0.5 h using a Zeiss Axiovert A1 inverted fluorescence microscope equipped with a LED excitation light source (Thorlabs, Newton, NJ). The digital images were processed and analyzed using ImageJ (NIH, http://rsbweb.nih.gov/ij/) to measure the fluorescence intensity.
SEM characterization
The SEM images of ExoProfile microchips were took in FEI Versa 3D Dual Beam scanning electron microscope. The chip was coated with 5 nm gold using a high-resolution ion bean coater to enhance conductivity. In order to take clear images of captured exosomes, extra fixing and staining steps were needed. Exosomes were fixed with 2.5% glutaraldehyde in PBS buffer for 30 minutes and then rinsed for 3 × 5 minutes. The samples were post-fix for 15 minutes in 1% osmium tetroxide and rinsed 10 minutes with water. The samples were dehydrated in a graded series of ethanols (30%, 50%, 70%, 95% and 100%) for 2 × 10 min at each step. After that, the samples were coated with gold and examined by SEM.
Exosome analysis using microplate ELISA
100 μL, 106 μL−1 purified SKOV3 EVs were added into each well of a 96-well plate. The plate was sealed with parafilm and incubated at room temperature while shaking for 30 min, then transferred into a 4 °C fridge for overnight incubation (12 h). 200 μL of washing buffer was added into each well, mixed by shaking, and discard by pouring out. The washing step was repeated for 3 times. The same biotinylated detection antibody as in the chip analysis was diluted to 2 μg mL−1 and 100 μL was added into wells. The plate was sealed and incubated at room temperature while shaking for 20 min, then incubated for 2 h at 4 °C. The plate was washed as above for 3 times, followed by adding 100 μL of 1
:
5000 diluted HRP-streptavidin conjugate, shaking at room temperature for 15 min, and incubation at 4 °C for 1 h. Excess enzyme was washed away with the washing buffer for 3 times. Lastly, 100 μL of chromogenic substrate solution was added to each well and incubated for 10 min at room temperature in dark. The reactions were stopped by adding 100 μL of stop solution. Absorbance was measured at 450 nm on a CYTATION 5 imaging reader (BioTek) and subtracted by the background measured with PBS.
Statistical analysis
For one-way ANOVA of each biomarker, the fluorescent intensities measured for individual subjects were corrected by the blank signals and then normalized by the 99th percentile value of all background-corrected signals. The unweighted sum of seven markers provides the SUM signature of each plasma sample. Comparison of the control and cancer groups was tested by two-tailed Student's t-test was used for comparison. ROC curves were constructed for individual markers and the SUM signature to evaluate their performance for diagnosis of ovarian cancer. Linear discriminant analysis was conducted to assess the six-marker exosomal signature for cancer diagnosis and staging.20 The 95% CIs were calculated using a binomial distribution. Non-supervised hierarchical clustering of six tumor markers was performed with Ward linkage and Euclidean distance. For all statistical analyses, the significance level was set at p < 0.05. Statistical comparison, ROC/AUC analysis, and hierarchical clustering were performed using Origin 2016 and GraphPad Prism 7.
Results and discussion
Microfluidic design and working principle of ExoProfile chip
Due to the heterogeneity of cancer phenotypes, the expression level of single protein marker on circulating exosomes is variable across individual patient. The combined profiling of a panel of proteins on circulating exosomes will improve the accuracy of liquid biopsy-based diagnosis. To achieve this goal, the ExoProfile chip was designed to measure up to eight surface markers of exosomes simultaneously with high sensitivity and minimum variation. There are two strategies in the microfluidic design for multiplexed profiling of exosomes. The one is exosomes are captured in single chamber, and then labelled with a mixture of unique fluorescent dye conjugated detection antibodies. The excitation and emission wavelength of each conjugated dye is different, thus multiplexed detection of exosome surface markers are carried out simultaneously in single chamber. This strategy is straightforward and used in our previous study,16 while the throughput of detection is limited, contributing to the wavelength overlap of fluorescent dyes. The other is that several parallel channels are integrated on chip simply, and each channel is assigned to capture exosomes and detect one marker individually.37 Although the throughput is unlimited theoretically, it need to consume much more samples and reagents, which is not compatible with clinical application due to the limited volume of biofluid samples in liquid biopsy. Meanwhile, the individual sample loading process leads to large batch-to-batch variation and affect the accuracy of molecular detection. To address this issue, the ExoProfile chip takes advantage of both strategies and harnesses a switchable design, which works in a single-channel mode for exosomes capture and switches to the parallel-channel mode for afterward multiplexed molecular detection. This innovative design endowed the chip with multiplexed detection capacity and minimized sample consuming.
As shown in Fig. 1a, the ExoProfile chip consists of two PDMS layers which are aligned together. The bottom is fluidic layer for bioassay, consists of pump region, assay chambers and several sample reservoirs. 3D serpentine nanostructures were constructed on eight parallel assay chambers for in situ exosomes molecular profiling. The top is pneumatic control layer, which contains three sets of valves. The left three circular pump valves were used to drive assay solution flow through the channels. We introduced five-step stop-flow pumping method to actuate the three circular valves consecutively, thus pump the flow delivery forward. The flow rate was controlled preciously by adjusting the time of each actuating step, and flow direction can also be changed if we reverse the five-step pumping program.38 The middle and right valves were used to switch the chip from the single-channel mode to the parallel-channel mode. When middle valves were closed and right valve open, exosomes were flowed through whole channels and captured evenly on eight assay chambers (top of Fig. 1b). After finishing the capture and washing process, we opened middle valves and closed right valve, as illustrated in bottom of Fig. 1b. The chip was operated in the parallel-channel mode, and different detection antibodies were added in middle reservoirs to react in eight assay chambers for individual molecular detection of captured exosomes.
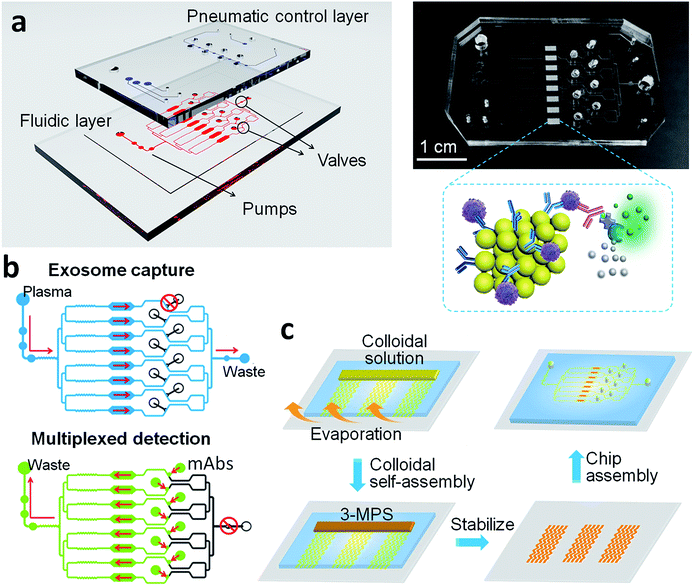 |
| Fig. 1 ExoProfile chip. (a) Schematic illustration and photo of the ExoProfile chip, consists of pneumatic and fluidic layer and 3D serpentine nanostructures, for in situ immunophenotyping of exosomes. (b) The working principle and flow manipulation of ExoProfile chip in the exosome immunocapture mode and the multiplexed immunodetection mode. Arrows indicated the flow direction. Blue channel: sample injection; green channel: injection of monoclonal detection antibodies (mAbs); black valve/channel: closed valve/channel. (c) The workflow for fabrication of an ExoProfile chip by microfluidic colloidal self-assembly. | |
Characterization of 3D self-assembled serpentine nanostructures
Surface area and capture probe density are critical factors governing the performance of on-chip biosensing. In order to improve the sensitivity of exosomes profiling, we introduced 3D porous nanostructures to expand the nanointerface of chip. The 3D serpentine nanostructures were constructed by microchannel guided evaporation-driven colloidal self-assembly, which was illustrated in Fig. 1c. Silica beads with 1 μm diameter were closely packed along the pattern microchannels, and then treated with (3-mercaptopropyl)trimethoxysilane (3-MPS) to connect the assembled beads and strengthen the nanostructures. SEM image (Fig. 2a) displays the intact 3D serpentine nanostructures without any crack fabricated by this bottom-up strategy. The serpentine morphology has been demonstrated to enhance the flow mixing previously, thus was used to increase the surface binding efficiency of exosomes. The magnified images (Fig. 2b and S2†) show the highly ordered crystalline structures and silica necks gluing the contacted beads. The closely packed beads possess the connected pores with size of about 150 nm (15% of the beads size), which allows the penetration of exosomes (<150 nm) and other assay reagents. The integrated porous network structures provide sufficient reaction sites for molecular profiling of exosomes.
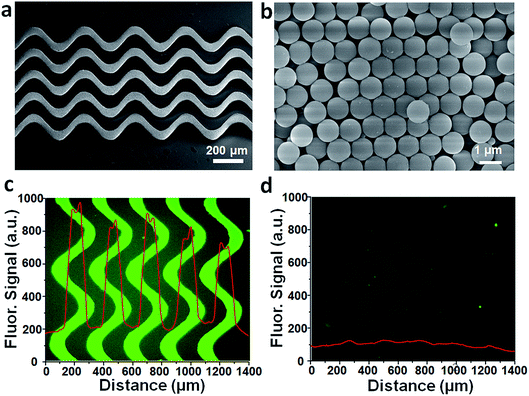 |
| Fig. 2 Characterization of 3D self-assembled serpentine nanostructures. (a) SEM image of large scale continuous serpentine nanostructures without any cracks and defects. (b) Amplified SEM image showing the uniform close packing of beads on nanostructures. (c) Quantification of immobilized anti-CD81 antibodies on ExoProfile chip with FITC-labeled anti-IgG antibody and (d) BSA negative control. | |
To verify the huge reaction sites of 3D porous nanostructures, we measured the probe intensity on ExoProfile chip firstly. Exosome capture antibodies were immobilized on nanostructures and adjacent glass surface via silane chemistry, and then quantitatively detected with fluorescent labelled anti-IgG antibodies. Compared to the flat glass substrate, the fluorescent signal on nanostructures was much higher (Fig. 2c). The measured fluorescent intensity indicates the amount of immobilized antibodies increases about 10 folds, consistent with theoretical calculation. The nanostructure is 25 μm in height and consists of 25 beads layers, thus is able to provide several dozens of more surface area for antibody immobilization than flat glass surface. The BSA control testing (Fig. 2d) demonstrated the minimum non-specific adsorption on nanostructures, after 3-MPS modification and BSA blocking.
Capture and molecular detection of exosomes on ExoProfile chip
EVs isolated from ovarian cancer SKOV3 cell culture media by ultracentrifugation (UC) were used to evaluate the performance of ExoProfile chip. For immunocapture of exosomes, tetraspanin proteins which are commonly expressed on the surface of exosomes were chosen as capture antibodies in this study. We compared three members of tetraspanin family (CD9, CD63, CD81) and demonstrated that CD81 displays the best performance for capture of ovarian cancer exosomes (Fig. S3†). The captured exosomes on ExoProfile chip were confirmed by SEM imaging. Compared to BSA coated control chip (Fig. 3b), large amount of exosomes were captured on anti-CD81 antibodies modified serpentine nanostructures (Fig. 3a). The capture efficiency of the ExoProfile chip was evaluated with DiO stained SKOV3 cell-derived exosomes, following the approach described before.35 In contrast to ultracentrifugation isolation that yielded a recovery rate of 17.3%, the capture efficiency of our chip was measured to be 75.4 ± 3.2% (Fig. S4†). This capture efficiency was slightly lower than that of the nano-HB chip, which can be attributed to its smaller chip size and less effective flow mixing by the 2D serpentine channel than a HB-based 3D mixer. Nonetheless, our nano-engineered ExoProfile chip outperforms the solid-HB chips for exosome isolation33 and afford sufficient capture efficiency to ensure high sensitivity of downstream exosome detection, as demonstrated below. The captured exosomes show typical round cup and spherical shapes with a size of less than 150 nm (inset, Fig. 3a). On the contrast, the UC-isolated EVs displayed two major peaks on nanoparticle tracking analysis (Fig. S5†), which corresponds to exosomes (50–150 nm) and microvesicles (200–300 nm). Size is a commonly acceptable criterion to differentiate exosomes from other vesicles in biofluid samples. This observed size discrepancy between UC and ExoProfile chip isolation demonstrated the specificity of immunocapture for exosomes, and ensured the accuracy of downstream molecular analysis of exosomes. Exosomes captured on the adjacent glass surface (Fig. 3c) were obviously less, due to the lower antibody density and capture efficiency. Notably, exosomes were also observed on silica beads below the surface layer of serpentine nanostructures (Fig. 3d). This phenomenon confirmed our original hypothesis, the 3D porous serpentine nanostructures allow exosomes to penetrate and thus provide ideal reaction sites for sensitive biosensing of exosomes.
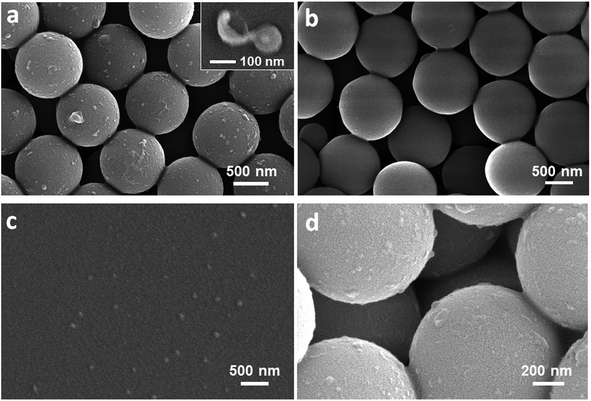 |
| Fig. 3 SEM images of captured exosomes on ExoProfile chip. (a) Large amount of exosomes captured on anti-CD81 antibodies immobilized 3D beads assembled nanostructures, and (b) minimized non-specific adsorption of exosomes on BSA coated control chip. (c) Less exosomes captured on the flat glass surface adjacent to the nanostructures. (d) Silica beads beneath the surface layer of nanostructures also captured exosomes. | |
To detect surface protein markers of captured exosomes quantitatively, we employed an on-chip sandwich ELISA assay as illustrated in Fig. 4a. Unlike the universal capture of exosomes with anti-CD81 antibodies, biotin conjugated detection antibodies enable the specific recognition of individual marker. Subsequently, streptavidin conjugated β-galactosidase (SβG) was introduced to assist fluorescent amplification reaction and further boost the detection sensitivity of exosomal marker. We used mixed detection antibodies of three highly expressed exosomal markers (CD9, CD63, CD81) to access the fluorescent detection performance of the ExoProfile chip. Intense fluorescent signal was measured on representative fluorescent image (Fig. 4b left) with high concentration of SKOV3 exosomes (105 μL−1), while low non-specific background was obtained by using PBS as blank control (Fig. 4b right). The enhanced signal should attribute to improved exosomes capture and biosensing efficiency on 3D porous serpentine nanostructures, indicating high sensitivity for molecular profiling of exosomes.
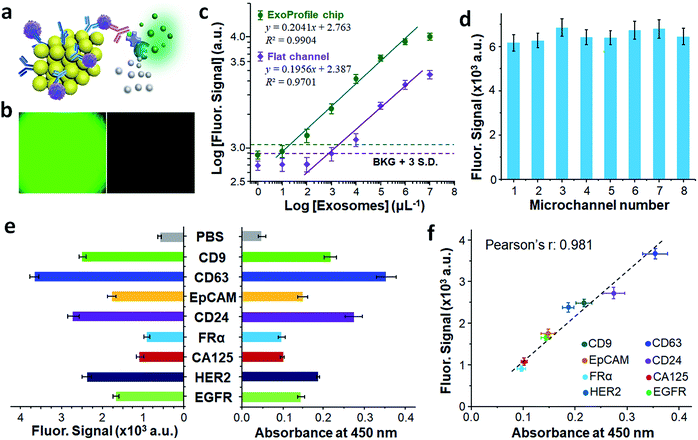 |
| Fig. 4 Molecular profiling of SKOV3 exosomes on ExoProfile chip. (a) Illustration of on-chip sandwiched ELISA detection of exosomes. (b) Enzymatic amplified fluorescent images of SKOV3 exosomes (left) and PBS control (right) using a mixture of detection antibodies (CD9, CD63 and CD81). (c) log–log calibration curve for quantifying total exosomes on ExoProfile and flat channels chip. LOD was calculated by 3σ/S, in which σ is the standard deviation (S.D.) of blank control, and S is the slope of standard curve within the linear range. (d) Homogeneity of fluorescence signal in eight parallel channels. (e) Profiling of seven surface markers SKOV3 exosomes: CD9, CD63, EGFR, HER2, CA125, FRα, CD24 and EpCAM, using the ExoProfile chip (left) and a commercial microplate ELISA kit (right). (f) Correlation of eight SKOV3 exosomal markers analyzed by the ExoProfile and commercial microplate ELISA. Error bars: S.D. from three independent measurements. | |
To the end of exosomes quantification, we calibrated the ExoProfile chip by detecting overall expressing of CD9, CD63 and CD81 on 10× diluted SKOV3 standard exosomes. The obtained calibration curve (Fig. 4c) showed quantitative detection over a 4-log dynamic range, with a low limit of detection (LOD) of ∼21 exosomes per μL, calculated by dividing three standard deviations of the background with the slope of the calibration plot. For comparison, a control chip without 3D serpentine nanostructures yielded much lower signal intensity and a LOD of 2219 exosomes per μL. The direct comparison demonstrated the advantages of the self-assembled 3D nanostructures in providing sufficient reaction sites and thus increasing sensitivity of on-chip biosensing. The superior sensitivity of ExoProfile chip also make it possible to profile some low-abundant markers on circulating exosomes.
The multiplexed detection capacity of configurable ExoProfile chip was further evaluated. The key concern is that there is no interference between the eight parallel channels as each chamber is an independent assay corresponding to unique marker. When chip was switched into the parallel channel mode, the outlets of eight channels were still connected together. For on chip ELISA detection, the fluorescent products of enzymatic amplification reaction may diffuse into side channels and cause signal interference. Hence, we added a long serpentine channel at the end of each assay chamber to delay the solution diffusion. To validate the effect, we injected red and green ink into these eight chambers separately and simulated the on-chip diffusion. We observed from Fig. S6† that there is no visible color mixing even after 0.5 h. This phenomenon indicated that the solution diffusion between parallel channels was under control and will not affect the accuracy of downstream fluorescent detection. We also tested the system deviations of these eight parallel on-chip ELISA assays. Using same detection antibodies (anti-CD9, CD63, CD81) for each channel, the achieved fluorescent signal was homogenous (Fig. 4d) and standard deviation of eight channels was measured to be 3.90%, which owes to the symmetrical channel design and minimized flow bias. The minimum interference and assay variation among the eight channels ensure the accuracy of multiplexed molecular profiling of exosomes.
Eight surface protein markers of SKOV3 exosomes were quantitatively profiled on ExoProfile chip simultaneously. Tetraspanin proteins (CD9 and CD63) were highly abundant on exosomes, thus held intense fluorescent signal in Fig. 4e. The other six markers were EGFR, HER2, CA125, FRα, CD24 and EpCAM, which have been studied as liquid biopsy biomarkers of ovarian cancer. All the six markers were detected successfully with variable expressing level on captured exosomes. It's worth noting that while FRα was relative low abundance,17,35 our ExoProfile chip achieved the accurate quantification of FRα on SKOV3 exosomes, which demonstrated FRα holds great potential serving as a candidate biomarker on circulating exosomes of ovarian cancer. The exosomal protein patterns were also confirmed by commercial microplate chromogenic ELISA, which consumed much more exosome samples (800 μL, 107 exosomes per μL) than ExoProfile chip (50 μL, 105 exosomes per μL). The results were highly consistent with our on-chip fluorescent detection, and the regression analysis of all the eight markers shows a strong linear correlation between the two methods (Fig. 4f, Pearson's r = 0.981). The successful profiling of eight proteins on SKOV3 exosomes paved the way for clinical diagnosis of ovarian cancer based on molecular profiling of circulating exosomes on ExoProfile chip.
Multiplexed profiling of circulating exosomes for diagnosis of ovarian cancer
Ovarian cancer has the highest mortality rate among gynecologic malignancies, because of the lack of early symptoms and signs. The 5 year survival rate for early-stage ovarian cancer patients is about 90%, whereas at late stage the survival rate is less than 20%, underscoring the importance of early diagnosis.39 A large number of diagnostic biomarkers have been investigated in the past decades, including CA125 which is the most commonly used ovarian tumor biomarker.40–42 However, there is no single biomarker that can detect early stage ovarian cancer with desired sensitivity and specificity, due to the heterogeneity of tumor phenotypes.43 The combination of biomarker panel was proposed to improve the precision of tumor diagnosis.19,20 As circulating exosomes were enriched resources of tumor biomarkers, we employed ExoProfile chip to detect several surface protein markers of circulating exosomes for liquid biopsy diagnosis of ovarian cancer. The cohort of clinical analysis consist of the plasma samples collected from 15 ovarian cancer patients (samples 1–15) and 5 benign controls (samples 16–20), with detailed information listed in Table S3.† 10 μL of plasma diluted to 50 μL were used for single test and six ovarian cancer biomarkers (EGFR, HER2, CA125, FRα, CD24 and EpCAM) were profiled simultaneously. The remaining two channels were assigned to positive control (CD9 + CD63) and negative control (PBS). Due to the dilution of plasma matrix and high specificity of our assay, the non-specific signal of free proteins in plasma was negligible (Fig. S7†).
As shown in Fig. 5a, the expressing level of these markers varied significantly across individual patients. Five markers, EGFR, CA125, FRα, CD24 and EpCAM, showed a significantly increased level in patients (Fig. S8†). There is no significant difference between ovarian cancer patients and healthy controls for HER2 and the generic exosome marker CD9 and CD63 combined, which is consistent with the previous studies reporting that counting exosomes is insufficient for tumor diagnosis. Receiver operator characteristic curve (ROC) and the area under ROC curve (AUC) were used to assess the diagnostic power of individual exosomal markers and their unweighted sum (SUM), as presented in Fig. 5b. It was seen that CD24, FRα and the SUM signature provide the best diagnosis accuracy (AUC = 1) to differentiate the patient and control groups. We assessed the ExoProfile analyses of these markers for early cancer diagnosis using one-way ANOVA with post hoc Tukey's test (Fig. 5c). FRα, CA125, EpCAM, and CD24 was able to individually detect at least one of the control (n = 5), early-stage (stage I/II, n = 5), and advanced OvCa (stage III/IV, n = 10) groups in the training set. For comparison, the SUM signature greatly outperformed the individual markers and differentiated all three groups significantly.
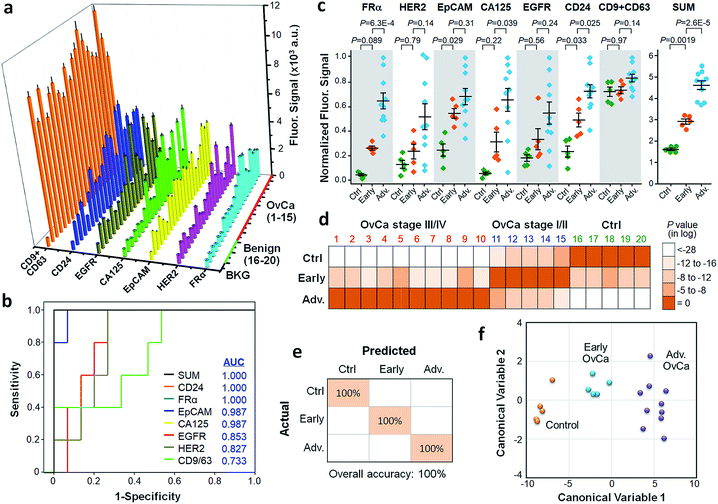 |
| Fig. 5 Molecular diagnosis of circulating exosomes from plasma of ovarian cancer (OvCa) patients. (a) Quantification of six tumor markers (EGFR, HER2, CA125, FRα, CD24, EpCAM) and exosomal markers (CD9 + CD63) from 15 patients and 5 benign controls plasma samples. Error bars: S.D. (n = 3). (b) ROC analysis to evaluate the diagnostic power of individual exosomal markers and the SUM signature to detect OvCa cases from the benign controls. (c) Scatter plots of the seven exosomal markers in the plasma samples from the controls and the early-stage (stage I/II) and advanced (stage III/IV) OvCa patients measured by the ExoProfile chip. For each marker, the fluorescent intensities measured for individual subjects were corrected by the blank signals and then normalized by the 99th percentile value of all background-corrected signals. Error bars are the mean and one standard error of the mean (s.e.m.). Statistical comparison of three groups was performed by one-way ANOVA with post hoc Tukey's test. (d) Heatmap of classification probabilities, (e) confusion matrix, and (f) canonical score plot summarizing the results of LDA for accurate classification of benign control, early stage and late stage OvCa patients. Significance level was set at p < 0.05 for all statistic analyses. | |
To demonstrate the feasibility of using a multi-marker exosomal signature for cancer diagnosis and staging, we conducted the linear discriminant analysis of the measured exosomal profile. The LDA on all six markers was seen to correctly classify each subject in the training cohort into one of the three groups (Fig. 5d). The classification results were quantitatively summarized in the confusion matrix, which showed an overall accuracy of 100% (95% CI: 83–100%) for identifying control, early-stage and advanced cases (Fig. 5e). The canonical score plot provides a succinct view of clear separation of the subjects using the first two linear discriminant variables (Fig. 5f). In addition to the supervised LDA, non-supervised hierarchical clustering analysis was also employed to assess the diagnostic potential of the exosomal markers (Fig. S9†). Consistently, the combined panel yielded much improved accuracy than the individual markers (CD24 and FRα) to detect the OvCa patients and to cluster them correctly into two disease conditions. It is noted that a small number of samples was used here for technology assessment and the clinical promise of these exosomal markers necessitates large-scale clinical validation. Nonetheless, our results collectively demonstrated the potential of the ExoProfile chip for multiplexed immunophenotyping of circulating exosomes as a liquid biopsy for non-invasive cancer diagnosis.
Conclusions
Here we demonstrated simple, lithography-free 3D nano-engineering of a classic serpentine channel micromixer to devise a robust, automated ExoProfile system for multiplexed exosome immunophenotyping. The ExoProfile chip affords highly efficient exosome immunocapture, superior exosome detection sensitivity, and high-throughput quantitative profiling of as many as eight biomarkers simultaneously. We profiled EGFR, HER2, CA125, FRα, CD24, EpCAM and CD9 + CD63 on circulating exosomes from plasma of 15 ovarian cancer patients and 5 benign controls. Significant heterogeneity in exosomal expression was observed for these biomarkers among patients. Compared to single biomarkers, multiplexed analysis of a combined biomarker panel was demonstrated to improve the diagnostic power, enabling classification of early and advanced-stage tumors. These preclinical validation studies corroborated the advantages of our technology for highly sensitive, multiplexed profiling of tumor-derived circulating exosomes with minimal sample consumption, which are particularly compelling for applications in preclinical screening, early diagnosis, and molecular stratification of cancer.
In principle, our MINDS approach is applicable to a broad spectrum of microfluidic mixer structures, opening new opportunities to developing innovative biosensing microdevices equipped with 3D nano-engineered sensing elements. We plan to investigate other 2D mixer geometries that have been reported to improve the mixing performance compared to the simple serpentine mixer examined here.44 The ExoProfile provides a versatile platform to implement different immunoassays to target various cancer types. It was observed by us and others that patients diagnosed of the same or different cancers exhibit significant heterogeneity in exosomal phenotype.17,20,31,35 Thus, identifying a panel of potent exosomal biomarkers is crucial to developing a diagnostic kit based on this microfluidic device for early cancer diagnosis and classification. The current ExoProfile chip is limited to profiling the surface protein markers of exosomes. Expanding the technology to measure both surface and internal protein contents of exosomes can leverage the adaptability to clinical applications. Finally, in this proof-of-concept study, our technology was validated with a small cohort of patient samples. A systematic validation study using the samples from a large cohort of patients is required to move the ExoProfile technology towards future clinical utilities.
Conflicts of interest
The authors declare no competing financial interest.
Acknowledgements
We thank the microfabrication core facility at the KU COBRE Center for Molecular Analysis of Disease Pathways for device fabrication and KU Cancer Center's Biospecimen Repository Core Facility funded in part by the National Cancer Institute Cancer Center Support Grant (P30 CA168524). This study was supported by 1R21CA186846, 1R21CA207816, 1R21EB024101, 1R33CA214333, and P20GM103638 from the NIH. P. Z. was partially supported by the postdoc award from the Kansas IDeA Network of Biomedical Research Excellence (K-INBRE) under the grant P20GM103418 from NIH/NIGMS.
References
- C. V. Harding, J. E. Heuser and P. D. Stahl, J. Cell Biol., 2013, 200, 367–371 CrossRef CAS PubMed.
- G. Raposo and W. Stoorvogel, J. Cell Biol., 2013, 200, 373–383 CrossRef CAS PubMed.
- A. Abramowicz, P. Widlak and M. Pietrowska, Mol. BioSyst., 2016, 12, 1407–1419 RSC.
- S. Mathivanan, H. Ji and R. J. Simpson, J. Proteomics, 2010, 73, 1907–1920 CrossRef CAS.
- C. Thery, L. Zitvogel and S. Amigorena, Nat. Rev. Immunol., 2002, 2, 569–579 CrossRef CAS.
- G. Chen, A. C. Huang, W. Zhang, G. Zhang, M. Wu, W. Xu, Z. L. Yu, J. G. Yang, B. K. Wang, H. H. Sun, H. F. Xia, Q. W. Man, W. Q. Zhong, L. F. Antelo, B. Wu, X. P. Xiong, X. M. Liu, L. Guan, T. Li, S. J. Liu, R. F. Yang, Y. T. Lu, L. Y. Dong, S. McGettigan, R. Somasundaram, R. Radhakrishnan, G. Mills, Y. L. Lu, J. Kim, Y. H. H. Chen, H. D. Dong, Y. F. Zhao, G. C. Karakousis, T. C. Mitchell, L. M. Schuchter, M. Herlyn, E. J. Wherry, X. W. Xu and W. Guo, Nature, 2018, 560, 382–386 CrossRef CAS.
- M. Poggio, T. Hu, C. C. Pai, B. Chu, C. D. Belair, A. Chang, E. Montabana, U. E. Lang, Q. Fu, L. Fong and R. Blelloch, Cell, 2019, 177, 414–427 CrossRef CAS PubMed.
- M. Poudineh, E. H. Sargent, K. Pantel and S. O. Kelley, Nat. Biomed. Eng., 2018, 2, 72–84 CrossRef PubMed.
- W. H. Li, C. Y. Li, T. Zhou, X. H. Liu, X. N. Liu, X. H. Li and D. X. Chen, Mol. Cancer, 2017, 16, 145–157 CrossRef PubMed.
- A. Yokoi, Y. Yoshioka, Y. Yamamoto, M. Ishikawa, S. Ikeda, T. Kato, T. Kiyono, F. Takeshita, H. Kajiyama, F. Kikkawa and T. Ochiya, Nat. Commun., 2017, 8, 14470 CrossRef PubMed.
- G. Brock, E. Castellanos-Rizaldos, L. Hu, C. Coticchia and J. Skog, Transl. Cancer Res., 2015, 4, 280–290 CAS.
- Y. Yoshioka, N. Kosaka, Y. Konishi, H. Ohta, H. Okamoto, H. Sonoda, R. Nonaka, H. Yamamoto, H. Ishii, M. Mori, K. Furuta, T. Nakajima, H. Hayashi, H. Sugisaki, H. Higashimoto, T. Kato, F. Takeshita and T. Ochiya, Nat. Commun., 2014, 5, 3591–3599 CrossRef PubMed.
- B. Sandfeld-Paulsen, N. Aggerholm-Pedersen, R. Boek, K. R. Jakobsen, P. Melolgaard, B. H. Folkersen, T. R. Rasmussen, K. Varming, M. M. Jorgensen and B. S. Sorensen, Mol. Oncol., 2016, 10, 1595–1602 CrossRef CAS PubMed.
- S. A. Melo, L. B. Luecke, C. Kahlert, A. F. Fernandez, S. T. Gammon, J. Kaye, V. S. LeBleu, E. A. Mittendorf, J. Weitz, N. Rahbari, C. Reissfelder, C. Pilarsky, M. F. Fraga, D. Piwnica-Worms and R. Kalluri, Nature, 2015, 523, 177–182 CrossRef CAS.
- M. Logozzi, D. F. Angelini, E. Iessi, D. Mizzoni, R. Di Raimo, C. Federici, L. Lugini, G. Borsellino, A. Gentilucci, F. Pierella, V. Marzio, A. Sciarra, L. Battistini and S. Fais, Cancer Lett., 2017, 403, 318–329 CrossRef CAS PubMed.
- Z. Zhao, Y. Yang, Y. Zeng and M. He, Lab Chip, 2016, 16, 489–496 RSC.
- H. Im, H. L. Shao, Y. I. Park, V. M. Peterson, C. M. Castro, R. Weissleder and H. Lee, Nat. Biotechnol., 2014, 32, 490–495 CrossRef CAS PubMed.
- K. R. Jakobsen, B. S. Paulsen, R. Baek, K. Varming, B. S. Sorensen and M. M. Jorgensen, J. Extracell. Vesicles, 2015, 4, 26659 CrossRef PubMed.
- S. Gulati, P. Martinez, T. Joshi, N. J. Birkbak, C. R. Santos, A. J. Rowan, L. Pickering, M. Gore, J. Larkin, Z. Szallasi, P. A. Bates, C. Swanton and M. Gerlinger, Eur. Urol., 2014, 66, 936–948 CrossRef CAS.
- C. Liu, J. X. Zhao, F. Tian, L. L. Cai, W. Zhang, Q. Feng, J. Q. Chang, F. N. Wan, Y. J. Yang, B. Dai, Y. L. Cong, B. Q. Ding, J. S. Sun and W. H. Tan, Nat. Biomed. Eng., 2019, 3, 183–193 CrossRef.
- B. J. Tauro, D. W. Greening, R. A. Mathias, H. Ji, S. Mathivanan, A. M. Scott and R. J. Simpson, Methods, 2012, 56, 293–304 CrossRef CAS PubMed.
- F. Momen-Heravi, L. Balaj, S. Alian, P. Y. Mantel, A. E. Halleck, A. J. Trachtenberg, C. E. Soria, S. Oquin, C. M. Bonebreak, E. Saracoglu, J. Skog and W. P. Kuo, Biol. Chem., 2013, 394, 1253–1262 CAS.
- R. Vaidyanathan, M. Naghibosadat, S. Rauf, D. Korbie, L. G. Carrascosa, M. J. A. Shiddiky and M. Trau, Anal. Chem., 2014, 86, 11125–11132 CrossRef CAS PubMed.
- L. Zhu, K. Wang, J. Cui, H. Liu, X. L. Bu, H. L. Ma, W. Z. Wang, H. Gong, C. Lausted, L. Hood, G. Yang and Z. Y. Hu, Anal. Chem., 2014, 86, 8857–8864 CrossRef CAS.
- S. Gholizadeh, M. S. Draz, M. Zarghooni, A. Sanati-Nezhad, S. Ghavami, H. Shafiee and M. Akbari, Biosens. Bioelectron., 2017, 91, 588–605 CrossRef CAS.
- H. L. Shao, J. Chung, K. Lee, L. Balaj, C. Min, B. S. Carter, F. H. Hochberg, X. O. Breakefield, H. Lee and R. Weissleder, Nat. Commun., 2015, 6, 6999 CrossRef CAS PubMed.
- M. He, J. Crow, M. Roth, Y. Zeng and A. K. Godwin, Lab Chip, 2014, 14, 3773–3780 RSC.
- S. S. Kanwar, C. J. Dunlay, D. M. Simeone and S. Nagrath, Lab Chip, 2014, 14, 1891–1900 RSC.
- K. Lee, H. L. Shao, R. Weissleder and H. Lee, ACS Nano, 2015, 9, 2321–2327 CrossRef CAS PubMed.
- K. Liang, F. Liu, J. Fan, D. L. Sun, C. Liu, C. J. Lyon, D. W. Bernard, Y. Li, K. Yokoi, M. H. Katz, E. J. Koay, Z. Zhao and Y. Hu, Nat. Biomed. Eng., 2017, 1, 21 CrossRef PubMed.
- Y. Wan, G. Cheng, X. Liu, S. J. Hao, M. Nisic, C. D. Zhu, Y. Q. Xia, W. Q. Li, Z. G. Wang, W. L. Zhang, S. J. Rice, A. Sebastian, I. Albert, C. P. Belani and S. Y. Zheng, Nat. Biomed. Eng., 2017, 1, 58 CrossRef PubMed.
- C. Liu, J. X. Zhao, F. Tian, J. Q. Chang, W. Zhang and J. S. Sun, J. Am. Chem. Soc., 2019, 141, 3817–3821 CrossRef CAS PubMed.
- E. Reategui, K. E. van der Vos, C. P. Lai, M. Zeinali, N. A. Atai, B. Aldikacti, F. P. Floyd, A. H. Khankhel, V. Thapar, F. H. Hochberg, L. V. Sequist, B. V. Nahed, B. S. Carter, M. Toner, L. Balaj, D. T. Ting, X. O. Breakefield and S. L. Stott, Nat. Commun., 2018, 9, 175–186 CrossRef PubMed.
- P. Zhang, M. He and Y. Zeng, Lab Chip, 2016, 16, 3033–3042 RSC.
- P. Zhang, X. Zhou, M. He, Y. Q. Shang, A. L. Tetlow, A. K. Godwin and Y. Zeng, Nat. Biomed. Eng., 2019 DOI:10.1038/s41551-41019-40356-41559.
- M. Khosravi Parsa, F. Hormozi and D. Jafari, Comput. Fluids, 2014, 105, 82–90 CrossRef CAS.
- Y. Q. Shang and Y. Zeng, Sci. Rep., 2016, 6, 20297 CrossRef CAS PubMed.
- T. Y. Wang, M. Zhang, D. D. Dreher and Y. Zeng, Lab Chip, 2013, 13, 4190–4197 RSC.
- R. C. Bast, B. Hennessy and G. B. Mills, Nat. Rev. Cancer, 2009, 9, 415–428 CrossRef CAS PubMed.
- Z. Zhang, R. C. Bast, Y. H. Yu, J. N. Li, L. J. Sokoll, A. J. Rai, J. M. Rosenzweig, B. Cameron, Y. Y. Wang, X. Y. Meng, A. Berchuck, C. van Haaften-Day, N. F. Hacker, H. W. A. de Bruijn, A. G. J. van der Zee, I. J. Jacobs, E. T. Fung and D. W. Chan, Cancer Res., 2004, 64, 5882–5890 CrossRef CAS PubMed.
- K. R. Kozak, M. W. Amneus, S. M. Pusey, F. Su, M. N. Luong, S. A. Luong, S. T. Reddy and R. Farias-Eisner, Proc. Natl. Acad. Sci. U. S. A., 2003, 100, 12343–12348 CrossRef CAS PubMed.
- S. Sharma, F. Zuniga, G. E. Rice, L. C. Perrin, J. D. Hooper and C. Salomon, Oncotarget, 2017, 8, 104687–104703 Search PubMed.
- A. C. Li, T. B. Zhang, M. Zheng, Y. N. Liu and Z. Chen, J. Hematol. Oncol., 2017, 10, 175–184 CrossRef PubMed.
- G. Cai, L. Xue, H. Zhang and J. Lin, Micromachines, 2017, 8, 274–300 CrossRef PubMed.
Footnote |
† Electronic supplementary information (ESI) available. See DOI: 10.1039/c9sc00961b |
|
This journal is © The Royal Society of Chemistry 2019 |