DOI:
10.1039/C9SC02266J
(Edge Article)
Chem. Sci., 2019,
10, 6617-6623
Poly(ionic liquid)s as a distinct receptor material to create a highly-integrated sensing platform for efficiently identifying numerous saccharides†
Received
9th May 2019
, Accepted 22nd May 2019
First published on 5th June 2019
Abstract
Saccharides have strong hydrophilicities, and are complex molecular structures with subtle structure differences, and tremendous structural variations. The creation of one sensing platform capable of efficiently identifying such target systems presents a huge challenge. Using the integration of unique multiple noncovalent interactions simultaneously occurring in poly(ionic liquid)s (PILs) with multiple signaling channels, in this research an aggregation-induced emission (AIE)-doped photonic structured PIL sphere is constructed. It is found that such a sphere can serve as a highly integrated platform to provide abundant fingerprints for directly sensing numerous saccharides with an unprecedented efficiency. As a demonstration, 23 saccharides can be conveniently identified using only one sphere. More importantly, by using simple ion-exchanges of PIL receptors or/and increasing the AIE signaling channels, this platform is able to perform, on demand, different sensing tasks very efficiently. This is demonstrated by using it for the detection of difficult targets, such as greatly extended saccharides as well as mixed targets, in real-life examples on one or two spheres. The findings show that this new class of platform is very promising for addressing the challenges of identifying saccharides.
Introduction
The detection and identification of saccharides in aqueous media is fundamentally important, but intrinsically challenging because of their heavy hydration, complex structures with subtle regio- and stereo-chemical differences, and enormous structural diversity.1–3 It is quite impractical to design and synthesize all the specific receptors for the recognition of numerous saccharides.4,5 Fortunately, instead of making synthetic receptors with lock-and-key specificity, the creation of sensor arrays based on a set of readily available selective receptors is a very promising alternative way to detect such target systems.6 In an array-based sensing scheme, the development of suitable sensing elements to generate unique fingerprint patterns by the collection of the cross-reactive responses of each sensing element with the analytes of interest is the basis of realizing the identification of the samples.7 Over the past few years, a variety of recognition motifs and corresponding sensor arrays for the detection and identification of saccharides have been constructed by different groups, such as those of Chang,8a Anslyn,8b Suslick,8c Severin,8d Singaram,8e Yan,8f and Bunz,8g to name a few.9 Although significant progress has been achieved, a sensing platform that can identify saccharides in a very efficient way, in terms of array size, implementation procedure, extended targets and tolerance to complex interferents, is still limited.
Incorporation of diverse noncovalent molecular interactions into receptors to increase the cross-reactivity of the sensing elements is critical for the acquisition of abundant sensing information, and thus, the performance and efficiency of the devised sensor array.10 For example, De et al. separately introduced different types of noncovalent interactions into discrete receptors, including hydrogen bonding, electrostatic forces, hydrophobic interactions, and π–π interactions, to achieve the required cross-responses of sensor arrays for the successful identification of target analytes.11 It is possible, that if these interactions are integrated into one receptor material, the resulting synergy between them resembling the recognition strategy used by biological systems, would further promote analyte discrimination,3 leading to the enhancement of the efficiency of the devised array. But all possible molecular interactions seldom occur together in one normal receptor material without tedious synthetic effort.12 In this context, ionic liquids (ILs) seem to be an ideal material as they simultaneously maintain nearly all molecular interactions.13 As a consequence of the abundant interactions, ILs exhibit an extraordinary solvation effect on common compounds or materials, and have exceptional dissolution capability for saccharides and their derivatives, which are hard to solubilize in conventional solvents.14 Inspired by this unique feature, it was assumed that IL-based materials might become a brand-new receptor material for creating an efficient array for use with saccharides. However, the engineering signaling channel is another effective way to enhance the capability of acquiring differential sensing information from the sensor array.15 It is envisioned that merging the multiple interactions of ILs with multiple signaling units within one sensing element could maximize the cross-reactivity of the devised array, and therefore, dramatically enhance its efficiency using a minimal sized sensor array.16 In reality, it was found that the polymer form of ILs is a distinct receptor material which could be used to create a highly-integrated sensing platform, by which direct identification of numerous saccharides and their derivatives could be achieved with unprecedented efficiency.
Results and discussion
Creation of a highly integrated sensing platform
In this paper, aggregation-induced emission (AIE)-doped photonic structured poly(ionic liquid) (PIL) spheres used as a highly integrated sensing platform are presented. In this platform, the multiple interactions of ILs with the photonic and FL signaling channels were integrated. A three-step procedure was developed for the fabrication of AIE-doped photonic PIL spheres, including the preparation of opal (photonic) spheres, the infiltration of the interstitial spaces of photonic spheres with IL monomer, crosslinker as well as AIE luminogen (AIEgen) followed by polymerization, and the removal of photonic templates with hydrogen fluoride. The IL monomer, crosslinker as well as the AIEgens used are shown in Fig. 1a and their synthetic details can be found in the ESI.† High-quality silicon dioxide- based photonic spheres (Fig. S1a and b†) were used as templates and were produced using a microfluidic approach. The resulting AIE-doped inverse opal photonic spheres (Fig. 1b and c) exhibited well-defined interconnected macropore structures (Fig. S1c and d†) and high surface area (Fig. S2†), which were favorable for the diffusion and interaction of analyte molecules within the PIL scaffold. The crosslinked density and thermal stability of the PIL materials were also well-characterized (Fig. S3†). Both of the photonic (PhC) property arising from the periodic pore structures and the fluorescence (FL) of the doped AIEgens can serve as signaling transduction mechanisms (Fig. 1d) for recognition purposes. To satisfy different detection demands, the signaling channel structure of the spheres was readily tunable from dual-(1 × PhC, 1 × FL) to triple-(1 × PhC, 2 × FL) and quadruple-(1 × PhC, 3 × FL), and even higher channels, which were dependent on the types of the introduced AIEgens.
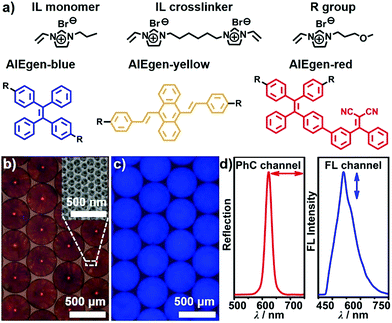 |
| Fig. 1 (a) Structures of IL monomer, crosslinker and AIE luminogens. (b) Reflection image and inset: scanning electron microscopy image, and (c) the FL image of the parent AIEgen-blue doped photonic structured PIL spheres. (d) The corresponding reflection (left) and the FL spectra (right). | |
Sphere-based arrays for efficiently discriminating saccharides
As a proof-of-concept, 23 different saccharides (for structures see Fig. S4†) at a concentration of 100 mM in neutral aqueous solution, the largest set of saccharides discriminated by the arrays reported so far, were chosen as target molecules to assess the performance of the integrated probe system. In this case, a dual channel sphere (AIEgen-blue doped photonic PIL sphere with a dicyanamide anion (DCA) as counteranion, Fig. S5†) was used and exposed to the samples mentioned previously. It was clear to see that the different interactions between the PIL sphere and these analytes induced the hydrogel spheres to physically swell or shrink to varying extents, leading to diversified photonic band shifts accompanied by remarkable color changes (Fig. 2a and S6†).17 At the same time, because of the swelling or shrinking of the PIL skeleton, as well as the local environment changes caused by the analytes, the FL intensity of the doped AIEgen-blue also altered to different extents (Fig. 2b).18 Quantitatively, the PhC band shift and the FL intensities of the doped dye at 515 nm for the peak (F1) and at 554 nm for the shoulder (F2) were collected and are shown in Fig. 2c and d, indicating that for 23 different saccharides the utilization of one sphere as a probe could generate abundant cross-responsive information to differentiate between them. Notably, even for the diastereomers, which differ only in the number and position of axial hydroxyl groups (e.g., allose, glucose, galactose, and mannose), one sphere could respond quite differently in terms of PhC and FL signals.
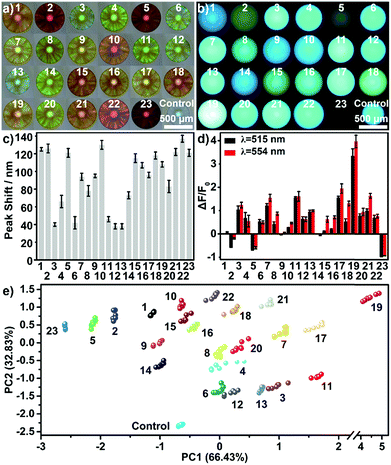 |
| Fig. 2 One dual channel sphere (DCA as counteranion) for identifying 23 saccharides at a concentration of 100 mM in a buffer of pH 7: (a) reflection images, (b) the FL images, (c) bar plot for the Bragg diffraction peak shifts, (d) bar plot for FL changes, and (e) the resultant PCA plot. Saccharides: allose,1 2-deoxyribose,2 fructose,3 fucose,4 galactose,5 glucose,6 lyxose,7 mannose,8 methyl α-glucopyranoside,9 rhamnose,10 ribose,11 sorbose,12 xylose,13 cellobiose,14 lactose,15 maltose,16 maltulose,17 melibiose,18 sucrose,19 trehalose,20 melezitose,21 raffinose,22 acarbose.23 | |
The previous complicated multivariate data (PhC, F1 and F2) were analyzed using principal component analysis (PCA). The Fig. 2e shows the resultant PCA plot, which clearly demonstrates that all 23 saccharides and one buffer control were discriminated well. The Jackknife cross-validation procedure was used to verify the complete discrimination with 100% accuracy (Table S1†). The successful discrimination of the similar 23 saccharides at a concentration of 100 mM were reported by Lee et al., in which six pH-indicator/boronic acid probe pairs screened from 100 organic indicator dyes and over 10 boronic acids were used.8a In this research, the results indicated that the extraction of differential sensing information from one highly-integrated sphere was sufficient to achieve the same goal. Undoubtedly, this virtual sensor array using one sensing element, significantly simplifies the sensor system physically and enhanced the experimental efficiency. Furthermore, it was found that the resolution of the detection and discrimination was also greatly improved using the highly integrated system. The implementation of identifying multi-saccharides at a concentration of 10 mM and even at 1 mM in neutral aqueous solution is possible (Fig. S7 and S8†).
Besides qualitative identification, one dual channel sphere was also found to be powerful for performing semiquantitative and quantitative assays. Acarbose, an oligosaccharide which is difficult recognize using boronic acid-containing receptors,19 was chosen to be examined in a wide concentration range (Fig. 3a). The PCA plot in Fig. 3b clearly shows that the clusters of acarbose at 11 varying concentrations were well separated and a good linear correlation between the Euclidean distances of these clusters and the concentrations (from 5 μM to 10 mM) could be established (Fig. 3c). The limit of detection for acarbose was estimated to be as low as 1.4 μM, showing an incredible sensitivity. In addition, in contrast to the majority of boronic acid-containing recognition motifs which were very sensitive to the changes in pH and often required a high pH in order to work, the discriminatory power of the PIL-based sensing platform described here was unaffected by pH values from 4 to 9 with the samples tested (Fig. S9†). This feature implied that the sensing system should be directly applicable for use with biological samples, such as blood, tears, urine, saliva, and sweat, whose pH values range from 4.5 to 8.0.20 Furthermore, the sensing performance of the PIL spheres were not affected by organic solvents, acids or alkalis, high temperatures and long periods of time, exhibiting a high stability to such conditions (Fig. S10†). Thanks to the reversible binding between the PILs and saccharides through the noncovalent interactions, the PIL spheres exhibited excellent reusability (Fig. S11†).
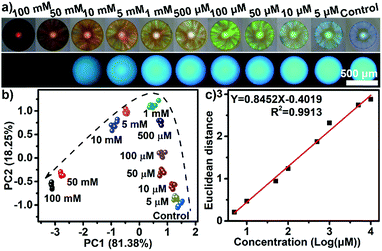 |
| Fig. 3 (a) Reflection images and fluorescence images of dual channel spheres (DCA as counteranion) for acarbose at 11 varying concentrations. (b) PCA plot for the semiquantitative analysis. (c) Linear regression for the quantitative analysis. | |
To gain a sound understanding of the interactions that the PIL recognition motif may involve, in this work, quantum calculations were performed using glucose and its two diastereomers (allose and galactose) as demonstration substances. Fig. 4a–c show the plotted reduced density gradient isosurfaces of the weak interactions occurring between the IL moiety used (imidazolium cation with DCA anion) and the analytes.21 Clearly, the multiple noncovalent interactions, including repulsive interactions (red regions), van der Waals, π-stacking and hydrophobic interactions (green regions), as well as the predominant hydrogen bonding (blue regions), exist between the IL moiety and the saccharides. The study using density functional theory (DFT) further illustrated that the multiple interactions involved lead to different binding energies (Fig. 4d–f). Currently, there is no solid evidence to support the hypothesis that only one specific interaction triggers the binding of saccharide molecules with PIL, despite the more significant role of hydrogen bonding than other secondary interactions. Thus, it is widely accepted that the binding event should be attributed to the synergetic effects of all the previously described noncovalent interactions.22 This multivalency resembles the key working principle of molecular recognition in biological systems. Lectins, the natural carbohydrate binding proteins, make cumulative use of individually weak interactions in their recognition processes.3 Because of the simultaneous existence of abundant noncovalent interactions in ILs, the PIL motif, in principle, should be generally applicable for any given saccharide. Indeed, based on the same sphere described previously, various saccharide derivatives such as sugar alcohols, phosphor sugars, amino sugars, sulfated sugars, glycosaminoglycans and glycoproteins, could also be successfully recognized from each other (for selected examples see Fig. S12–S15†).
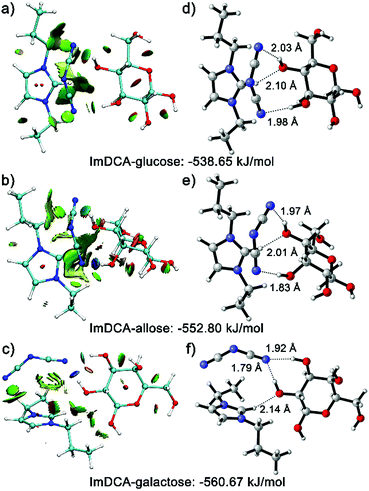 |
| Fig. 4 (a–c) Reduced density gradient isosurfaces for the visualization of multiple weak interactions between the ion pair (imidazolium cation and DCA anion) and three different isomeric saccharides: glucose, allose and galactose on a blue-green-red scale where blue represents strong attractive interaction, and red represents a strong nonbonding overlap. (d–f) Density functional theory results for the optimized structures [C atoms (gray), N atoms (blue), H atoms (white), and O atoms (red)]. The dashed lines denote the hydrogen bonds. | |
The theoretical calculations also help to explain the choice of the imidazolium cation rather than the phosphonium or ammonium cation in the PILs. In addition, the aromaticity of imidazolium ring, the relatively strong acidity of C2–H in the imidazolium cation ring can act as a hydrogen bonding donor to form a hydrogen bond with oxygen atoms in the saccharides. This hydrogen bonding can be clearly seen in the previous DFT calculations. However, the C–H of the alkyl side chains in the phosphonium or ammonium cations shows extremely weak acidity, and thus, no obvious hydrogen bonding can be observed. Furthermore the experimental results were used to validate that the PIL containing an imidazolium cation rather than a phosphonium or ammonium cation was a more promising candidate for use in saccharide detection (Fig. S16†). Taking the detection of 13 saccharides at 10 mM as an example, the use of one single dual channel sphere with an imidazolium cation could achieve 100% classification accuracy (Fig. S7†), but the classification accuracy for the spheres with ammonium or phosphonium cations was only 71% and 83%, respectively, (Fig. S16†).
As mentioned in introduction, one of the most characteristic features of carbohydrates is the tremendous structural variations. Thus, it is highly desirable to extend the devised sensing platform to address this challenge. In this case, the AIE-doped photonic PIL sphere appears very unique. Firstly, the structural feature of ILs allows rapid formation of a huge and dynamic sensing element library by using simple ion–exchange reactions. In principle, from one parent sphere, an unlimited number of sensing elements in the library could be easily derived without tedious synthetic research, because of the nearly endless combination of cations and anions. Furthermore, on the basis of the unique task specific concept of ILs,23 the functional counterions can be deliberately incorporated into the PIL receptor to influence the affinity of the sensing element to special target molecules. As a demonstration, Fig. 5 shows a 20 membered library of sensing elements created using a simple counteranion exchange from the parent AIE-doped photonic PIL sphere (for relevant counteranion structures see Fig. S17†). In this scenario, 32 different saccharides were used to test the capability of the sensing platform for the detection of largely extended analytes. Compared to the sample of 23 saccharides, the addition of nine new compounds (for structures see Fig. S18†) in the detection system ruined the discriminatory power of using one single sensing sphere as mentioned previously (Fig. S19 and Table S2†). However, the adoption of three sensing elements selected from our library could complete the detection mission on the relatively larger set of analytes (Fig. S20–S22 and Table S3†). In fact, it was found that an array consisting of any two sensing elements could serve as a minimum probe set for the full detection of 32 carbohydrates without error (Fig. S23–S25 and Table S4–S6†).
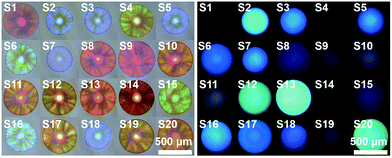 |
| Fig. 5 Reflection images (left) and FL images (right) for the 20 membered sensor library of sensing elements with differential PIL receptors. Counteranions for sensing elements S1–S20: bromide (S1), DCA (S2), bis(trifluoromethane)sulfonimide (S3), tripolyphosphate (S4), tetrafluoroborate (S5), hexafluorophosphate (S6), trifluoromethanesulfonate (S7), formate (S8), acetate (S9), carbonate (S10), chloride (S11), citrate (S12), glutamate (S13), ascorbate (S14), nitrate (S15), perchlorate (S16), tartrate (S17), thiocyanate (S18), sulfate (S19) and phosphate (S20). | |
In addition, engineering the signaling channel in the PIL photonic sphere offers another route to further extend the sensing platform. In a comparison with the dual channel (1 × PhC, 1 × FL) PIL sphere used previously, increasing the types of doped AIEgen (see Fig. 1a and S26, S27†) to form triple (1 × PhC, 2 × FL) or quadruple channel (1 × PhC, 3 × FL) spheres, can dramatically enhance the capability of acquiring the differential sensing information of the spheres. As shown in Fig. S28,† using only one quadruple channel photonic sphere (doped with AIEgen-blue, AIEgen-yellow, and AIEgen-red), seven dimensional sensing information can be extracted including one from the PhC channel and six from the FL channels (F1: AIEgen-blue peak emission at 515 nm, F2: AIEgen-blue shoulder emission at 554 nm, F3: AIEgen-yellow peak emission at 560 nm, F4: AIEgen-yellow shoulder emission at 528 nm, F5: AIEgen-red peak emission at 614 nm, F6: AIEgen-red shoulder emission at 642 nm). It was found that the previous multivariate data from one quadruple channel sphere was sufficient for the successful implementation of identifying the same extended sample system (Fig. 6 and S29, S30 and Table S7†). Based on these results, it was reasonable to assume that the combined use of ion–exchange reactions and modulation of AIE signaling channels could generate diverse and suitable sensing elements on demand for use with different demanding tasks in a very efficient way.
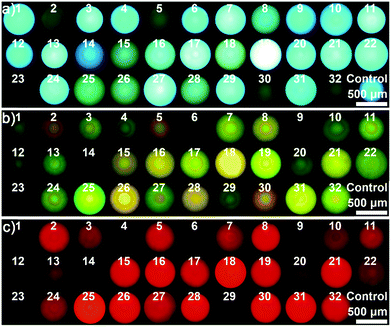 |
| Fig. 6 The FL responses of one quadruple channel PIL photonic sphere (doped with AIEgen-blue, AIEgen-yellow, and AIEgen-red; DCA as counteranion) to 32 saccharides: (a) FL-B channel images, (b) FL-Y channel images, and (c) FL-R channel images (exposure time 30 ms). Saccharides: allose (1), 2-deoxyribose (2), fructose (3), fucose (4), galactose (5), glucose (6), lyxose (7), mannose (8), methyl α-glucopyranoside (9), rhamnose (10), ribose (11), sorbose (12), xylose (13), cellobiose (14), lactose (15), maltose (16), maltulose (17), melibiose (18), sucrose (19), trehalose (20), melezitose (21), raffinose (22), acarbose (23), arabinose (24) 2-deoxy-lyxohexos (25), tagatose (26), gentiobiose (27), lactulose (28), palatinose (29), turanose (30), maltotriose (31) and maltopentose (32). | |
Real world applications
The encouraging results prompted the testing of the feasibility of the sensing platform in real world applications. In this work, two types of real life samples were chosen. One type was 12 commonly accessible sugary beverages, and another was complex mixtures of saccharides in tea. Fig. S31† shows the responsive fingerprint obtained when using one single dual channel sphere (doped with AIEgen-blue) as a probe to directly test sugary beverages without any change of their pH values. Not surprisingly, because of the large differences of overall ingredients between these beverages (Table S8†), this determination task was accomplished solely by using one sphere (Fig. 7a). Additionally, these 12 beverage samples after adjusting their pH values to the same level (pH = 7) were also tested and the experiments performed confirmed full discrimination between the samples (Fig. S32†), indicating that the pH values of the beverages had little influence on the sensing performance of the PIL spheres. To further demonstrate the high performance of the sensing platform in complex backgrounds, the detection of sugars in teas was performed. Thus, five often used saccharides: fructose, galactose, glucose, lactose and sucrose were selected as five components for the preparation of saccharide mixtures in the same tea solution. In total, 26 equimolar mixtures (10 two-component mixtures, 10 three-component mixtures, 5 four-component mixtures, and 1 five-component mixture) were used as target systems for detection. Obviously, for such complex target systems one single dual channel or quadruple channel sphere no longer has the capability to achieve complete discrimination (Fig. S33, S34 and Tables S9, S10†). Nevertheless, it was found that from the sensor array consisting of two quadruple channel PIL spheres (DCA and BF4− as counteranions, respectively), sufficient sensing information (Fig. S35 and S36†) could be collected to obtain the desired discrimination. As shown in Fig. 7b, the PCA plot indicated the full discrimination of the tested mixtures (five unitary systems, 26 mixed systems and one control system) with 100% accuracy (Table S11†). In order to study the relevance of the other background solutions, two usually occurring solutions such as sugars in urine and sugars in serum were selected as target systems for testing the performance of the sensing platform. It was found that in both backgrounds, the saccharides dissolved in solutions could also be identified with no error as shown in the PCA plots (Fig. S37†), indicating that the identification of saccharides might not be susceptible to complex backgrounds by using this system. According to the basic principle of the array-based sensing scheme, as long as the training sets were established, it was possible to detect the unknowns by identifying their locations in the score plot space.24 Following this lead, 32 samples in the previous 32 training sets were also correctly predicted (Table S12†). To our knowledge, none of the previous arrays has yet been reported to fulfill a similar difficult detection task.
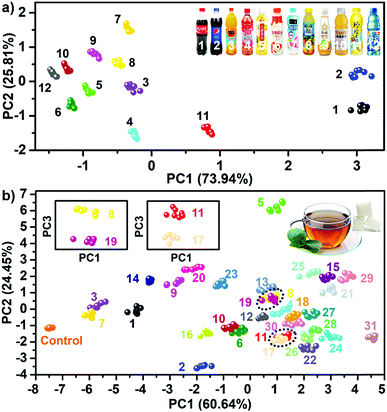 |
| Fig. 7 (a) PCA plot of one dual channel sphere (DCA as counteranion) for directly identifying 12 sugary beverages without any change of their pH values. (b) PCA plot of two quadruple channel spheres (DCA and BF4− as counteranions, respectively) for identifying one control system, five unitary systems and 26 mixed systems for all saccharides at a concentration of 20 mM in tea. A (1), B (2), C (3), D (4), E (5), AB (6), AC (7), AD (8), AE (9), BC (10), BD (11), BE (12), CD (13), CE (14), DE (15), ABC (16), ABD (17), ABE (18), ACD (19), ACE (20), ADE (21), BCD (22), BCE (23), BDE (24), CDE (25), ABCD (26), ABCE (27), ABDE (28), ACDE (29), BCDE (30) and ABCDE (31). A–E typify fructose, galactose, glucose, lactose and sucrose, respectively. | |
In the past few years, two main strategies for constructing sensor arrays have been developed so far: to obtain one type of signal from the multiprobes (multiple sensing elements) or to get multiple signals from one type of probe (sensing element). The former always required the synthesis of several probes with different functional groups, whereas the latter usually relies on different signals of the single probe known as “lab-on-a-molecule” or “lab-on-a-particle”.15 The strategy of merging all possible non-covalent interactions (functional groups) with multichannel signaling sets in one single element has not yet been reported. These results indicated that such a convergence strategy described here allowed for dramatic enhancement of the capability of one single element to acquire abundant sensing information, demonstrating a very promising route to tackle tricky detection problems in an efficient way.
Conclusions
In summary, for the first time it was discovered that poly(ionic liquid)s could act as distinct receptor materials for complexing saccharides to create high-performance sensor arrays. Through the integration of the unique multiple noncovalent interactions simultaneously occurring in ionic liquids and the multichannel signaling structure, AIE-doped photonic PIL spheres were developed which were a new class of sensing platform for directly identifying numerous saccharides. The extremely high efficiency and generality of the discrimination were the highlights of the highly integrated sensing platform, as demonstrated by several experiments. With these distinctive features, it is believed that this sensing platform has great potential for addressing the challenges associated with saccharide detection.
Live subject statement
The human urine and serum experiments were conducted in compliance with the laws and institutional guidelines for the Academic Norms System established by the Academic Committee of Tsinghua University. This committee approved the experiments and informed consent from the person who provided urine and serum samples was obtained for the experimentation with human subjects.
Conflicts of interest
There are no conflicts to declare.
Acknowledgements
The authors gratefully acknowledge the financial support from the NSF China (No. 21773135, 21473098, 21121004, and 21421064), MOST (2017YFA0204501, 2013CB834502), and the Deutsche Forschungsgemeinschaft DFG (TRR61). The authors wish to thank Prof. Dr Xiaomin Liu and Prof. Dr Suojiang Zhang at Institute of Process Engineering, Chinese Academy of Sciences for help with the quantum calculations. The authors also wish to thank Prof. Dr Yuchun Han and Prof. Dr Yilin Wang at the Institute of Chemistry, Chinese Academy of Sciences for useful discussions.
Notes and references
-
(a) R. A. Tromans, T. S. Carter
, L. Chabanne, M. P. Crump, J. V. Matlock,
M. G. Orchard and A. P. Davis, Nat. Chem., 2019, 11, 52–56 CrossRef CAS PubMed;
(b) P. Ríos, T. J. Mooibroek, T. S. Carter, C. Williams, M. R. Wilson, M. P. Crump and A. P. Davis, Chem. Sci., 2017, 8, 4056–4061 RSC.
- T. D. James, K. R. A. S. Sandanayake and S. Shinkai, Angew. Chem., Int. Ed., 1996, 35, 1910–1922 CrossRef.
- S. Jin, Y. Cheng, M. Li and B. Wang, Med. Res. Rev., 2010, 30, 171–257 CAS.
- M. Rauschenberg, S. Bomke, U. Karst and B. J. Ravoo, Angew. Chem., Int. Ed., 2010, 49, 7340–7345 CrossRef CAS PubMed.
-
(a) W. J. Ramsay and H. Bayley, Angew. Chem., Int. Ed., 2018, 57, 2841–2845 CrossRef CAS PubMed;
(b) M. Yamashina, M. Akita, T. Hasegawa, S. Hayashi and M. Yoshizawa, Sci. Adv., 2017, 3, e1701126 CrossRef PubMed;
(c) Y.-J. Huang, W.-J. Ouyang, X. Wu, Z. Li, J. S. Fossey, T. D. James and Y.-B. Jiang, J. Am. Chem. Soc., 2013, 135, 1700–1703 CrossRef CAS PubMed;
(d) N. Chandramouli, Y. Ferrand, G. Lautrette, B. Kauffmann, C. D. Mackereth, M. Laguerre, D. Dubreuil and I. Huc, Nat. Chem., 2015, 7, 334–341 CrossRef CAS;
(e) J. Yoon and A. W. Czarnik, J. Am. Chem. Soc., 1992, 114, 5874–5875 CrossRef CAS;
(f) C. Zhang, G. G. Cano and P. V. Braun, Adv. Mater., 2014, 26, 5678–5683 CrossRef CAS PubMed;
(g) K. T. Kim, J. J. L. M. Cornelissen, R. J. M. Nolte and J. C. M. van Hest, J. Am. Chem. Soc., 2009, 131, 13908–13909 CrossRef CAS;
(h) H.-C. Wang, H. Zhou, B. Chen, P. M. Mendes, J. S. Fossey, T. D. James and Y.-T. Long, Analyst, 2013, 138, 7146–7151 RSC;
(i) X.-P. He, X.-W. Wang, X.-P. Jin, H. Zhou, X.-X. Shi, G.-R. Chen and Y.-T. Long, J. Am. Chem. Soc., 2011, 133, 3649–3657 CrossRef CAS PubMed.
-
(a) L. You, D. Zha and E. V. Anslyn, Chem. Rev., 2015, 115, 7840–7892 CrossRef CAS PubMed;
(b) A. Akdeniz, T. Minami, S. Watanabe, M. Yokoyama, T. Ema and P. Anzenbacher Jr, Chem. Sci., 2016, 7, 2016–2022 RSC;
(c) Y. Qi, W. Xu, R. Kang, N. Ding, Y. Wang, G. He and Y. Fang, Chem. Sci., 2018, 9, 1892–1901 RSC.
-
(a) C. Zhou, W. Xu, P. Zhang, M. Jiang, Y. Chen, R. T. K. Kwok, M. M. S. Lee, G. Shan, R. Qi, X. Zhou, J. W. Y. Lam, S. Wang and B. Z. Tang, Adv. Funct. Mater., 2019, 29, 1805986 CrossRef;
(b) C. Bravin, A. Guidetti, G. Licini and C. Zonta, Chem. Sci., 2019, 10, 3523–3528 RSC;
(c) Z. Zheng, W.-C. Geng, J. Gao, Y.-Y. Wang, H. Sun and D.-S. Guo, Chem. Sci., 2018, 9, 2087–2091 RSC;
(d) J.-S. Moon, W.-G. Kim, D.-M. Shin, S.-Y. Lee, C. Kim, Y. Lee, J. Han, K. Kim, S. Y. Yoo and J.-W. Oh, Chem. Sci., 2017, 8, 921–927 RSC.
-
(a) J. W. Lee, J.-S. Lee and Y.-T. Chang, Angew. Chem., Int. Ed., 2006, 45, 6485–6487 CrossRef CAS PubMed;
(b) N. Y. Edwards, T. W. Sager, J. T. McDevitt and E. V. Anslyn, J. Am. Chem. Soc., 2007, 129, 13575–13583 CrossRef CAS PubMed;
(c) S. H. Lim, C. J. Musto, E. Park, W. Zhong and K. S. Suslick, Org. Lett., 2008, 10, 4405–4408 CrossRef CAS PubMed;
(d) F. Zaubitzer, A. Buryak and K. Severin, Chem.–Eur. J., 2006, 12, 3928–3934 CrossRef CAS PubMed;
(e) A. Schiller, R. A. Wessling and B. Singaram, Angew. Chem., Int. Ed., 2007, 46, 6457–6459 CrossRef CAS PubMed;
(f) J. Tan, H.-F. Wang and X.-P. Yan, Anal. Chem., 2009, 81, 5273–5280 CrossRef CAS PubMed;
(g) N. M. Bojanowski, M. Bender, K. Seehafer and U. H. F. Bunz, Chem.–Eur. J., 2017, 23, 12253–12258 CrossRef CAS PubMed.
-
(a) M. Qin, Y. Huang, Y. Li, M. Su, B. Chen, H. Sun, P. Yong, C. Ye, F. Li and Y. Song, Angew. Chem., Int. Ed., 2016, 55, 6911–6914 CrossRef CAS PubMed;
(b) J. F. Teichert, D. Mazunin and J. W. Bode, J. Am. Chem. Soc., 2013, 135, 11314–11321 CrossRef CAS PubMed;
(c) X. Bi, D. Li and Z. Liu, Anal. Chem., 2015, 87, 4442–4447 CrossRef CAS PubMed;
(d) X.-T. Zhang, S. Wang and G.-W. Xing, ACS Appl. Mater. Interfaces, 2016, 8, 12007–12017 CrossRef CAS PubMed.
- Z. Li, J. R. Askim and K. S. Suslick, Chem. Rev., 2019, 119, 231–292 CrossRef CAS PubMed.
- M. De, S. Rana, H. Akpinar, O. R. Miranda, R. R. Arvizo, U. H. F. Bunz and V. M. Rotello, Nat. Chem., 2009, 1, 461–465 CrossRef CAS PubMed.
- S. Zhang, J. Zhang, Y. Zhang and Y. Deng, Chem. Rev., 2017, 117, 6755–6783 CrossRef CAS PubMed.
-
(a) W. Qian, J. Texter and F. Yan, Chem. Soc. Rev., 2017, 46, 1124–1159 RSC;
(b) J. Yuan, D. Mecerreyes and M. Antonietti, Prog. Polym. Sci., 2013, 38, 1009–1036 CrossRef CAS;
(c) M. Armand, F. Endres, D. R. MacFarlane, H. Ohno and B. Scrosati, Nat. Mater., 2009, 8, 621–629 CrossRef CAS PubMed;
(d) S. Zhang, K. Dokko and M. Watanabe, Chem. Sci., 2015, 6, 3684–3691 RSC;
(e) A. Ito, T. Yasuda, T. Yoshioka, A. Yoshida, X. Li, K. Hashimoto, K. Nagai, M. Shibayama and M. Watanabe, Macromolecules, 2018, 51, 7112–7120 CrossRef CAS;
(f) W. Zhang, Q. Zhao and J. Yuan, Angew. Chem., Int. Ed., 2018, 57, 6754–6773 CrossRef CAS PubMed.
-
(a) R. P. Swatloski, S. K. Spear, J. D. Holbrey and R. D. Rogers, J. Am. Chem. Soc., 2002, 124, 4974–4975 CrossRef CAS;
(b) H. Wang, G. Gurau and R. D. Rogers, Chem. Soc. Rev., 2012, 41, 1519–1537 RSC;
(c) O. A. El Seoud, A. Koschella, L. C. Fidale, S. Dorn and T. Heinze, Biomacromolecules, 2007, 8, 2629–2647 CrossRef CAS PubMed.
-
(a) M. Schmittel and H.-W. Lin, Angew. Chem., Int. Ed., 2007, 46, 893–896 CrossRef CAS PubMed;
(b) P. Wu, L.-N. Miao, H.-F. Wang, X.-G. Shao and X.-P. Yan, Angew. Chem., Int. Ed., 2011, 50, 8118–8121 CrossRef CAS PubMed.
- W. Zhang, N. Gao, J. Cui, C. Wang, S. Wang, X. Dong, G. Zhang, D. Zhang and G. Li, Chem. Sci., 2017, 8, 6281 RSC.
-
(a) Y. Zhao, X. Zhao and Z. Gu, Adv. Funct. Mater., 2010, 20, 2970–2988 CrossRef CAS;
(b) G. von Freymann, V. Kitaev, B. V. Lotsch and G. A. Ozin, Chem. Soc. Rev., 2013, 42, 2528–2554 RSC;
(c) T. M. Choi, K. Je, J.-G. Park, G. H. Lee and S.-H. Kim, Adv. Mater., 2018, 30, 1803387 CrossRef PubMed.
-
(a) J. Mei, N. L. C. Leung, R. T. K. Kwok, J. W. Y. Lam and B. Z. Tang, Chem. Rev., 2015, 115, 11718–11940 CrossRef CAS PubMed;
(b) K. Li and B. Liu, Chem. Soc. Rev., 2014, 43, 6570–6597 RSC;
(c) M. C. Heffern, L. M. Matosziuk and T. J. Meade, Chem. Rev., 2014, 114(8), 4496–4539 CrossRef CAS PubMed.
- G. Fukuhara and Y. Inoue, J. Am. Chem. Soc., 2011, 133, 768–770 CrossRef CAS PubMed.
- J. Axthelm, S. H. C. Askes, M. Elstner, G. U. Reddy, H. Görls, P. Bellstedt and A. Schiller, J. Am. Chem. Soc., 2017, 139, 11413–11420 CrossRef CAS PubMed.
-
(a) E. R. Johnson, S. Keinan, P. Mori-Sánchez, J. Contreras-García, A. J. Cohen and W. Yang, J. Am. Chem. Soc., 2010, 132, 6498–6506 CrossRef CAS PubMed;
(b) T. Lu and F. Chen, J. Comput. Chem., 2012, 33, 580–592 CrossRef CAS PubMed.
- Y. Li, J. Wang, X. Liu and S. Zhang, Chem. Sci., 2018, 9, 4027–4043 RSC.
- R. Giernoth, Angew. Chem., Int. Ed., 2010, 49, 2834–2839 CrossRef CAS PubMed.
- M. E. Germain and M. J. Knapp, J. Am. Chem. Soc., 2008, 130, 5422–5423 CrossRef CAS PubMed.
Footnotes |
† Electronic supplementary information (ESI) available: Preparation of AIE luminogens with different emission colors, experimental sections, fingerprint data, and chemometric data analysis. See DOI: 10.1039/c9sc02266j |
‡ These authors contributed equally to this work. |
|
This journal is © The Royal Society of Chemistry 2019 |
Click here to see how this site uses Cookies. View our privacy policy here.