DOI:
10.1039/C9AN01715A
(Paper)
Analyst, 2020,
145, 277-285
Klarite as a label-free SERS-based assay: a promising approach for atmospheric bioaerosol detection†
Received
3rd September 2019
, Accepted 6th November 2019
First published on 12th November 2019
Abstract
Detecting atmospheric bioaerosols in a quantitative way is highly desirable for public health and safety. This work demonstrates that surface-enhanced Raman spectroscopy (SERS) is a simple and rapid analytical technique for the detection of atmospheric bioaerosols, on a Klarite substrate. For both simulated and ambient bioaerosols, this detection assay results in an increase in the enhancement factor of the Raman signal. We report a strong SERS signal generated by bioaerosols containing living Escherichia coli deposited on Klarite. Furthermore, we demonstrate that SERS mapping can be used to estimate the percentage of airborne, living Escherichia coli. Moreover, Klarite provides differently distinct SERS spectra at different bacterial growth phases, indicating its potential to identify changes occurring in the bacterial envelope. Finally, we applied SERS for the rapid detection of Escherichia coli in ambient bioaerosols without using time-consuming and laborious culture processes. Our results represent rapid, culture-free and label-free detection of airborne bacteria in the real-world environment.
Introduction
Atmospheric bioaerosols are a class of aerosol particles that range in size from a few nanometers (nm) to tens of micrometers (μm).1,2 They are composed of living and dead cells, fungal spores, and microorganisms including algae, viruses and bacteria that affect the atmospheric chemistry through ice nucleation and cloud droplet formation, which eventually play a major role in global temperature.3 Generally, their presence in the atmosphere is due to anthropogenic activities, such as solid waste reuse, agricultural activities and waste water treatment.4,5 Several atmospheric bioaerosols can cause severe infections and allergies in humans, upon inhalation of the contaminated air or upon skin contact with them, and are related to increased mortality.6,7 Sensitive and efficient detection techniques for atmospheric bioaerosols are very important for public health and safety.8 The standard detection assay for bioaerosols is plating and culturing. Although this method is sensitive and selective, the necessary incubation step renders it laborious and time-consuming.9 In addition, several other methods, for example, the polymerase chain reaction (PCR),10 matrix assisted laser desorption/ionization mass time of flight spectrometry (MALDI-TOF),11 enzyme-linked immunosorbent assay (ELISA),12 and loop-mediated isothermal amplification (LAMP),13 have been developed. These methods can provide qualitative data, but are unable to distinguish between live and dead bacteria. Learning about the concentration of live bacteria could predict the potential of infection. Therefore, a sensitive and rapid method for atmospheric bioaerosol detection and living condition determination is highly desirable.
For the study and characterization of aerosol particles, various mass spectrometry techniques including aerosol time-of-flight mass spectrometry and single-particle aerosol mass spectrometry have been employed.14,15 In the case of atmospheric bioaerosols, Raman spectroscopy as a nondestructive vibrational spectroscopy technique has proven to be useful. When it is combined with an electrodynamic balance, acoustic levitation and optical tweezers, it can be useful to study individual aerosol particles.16,17 Because of its sensitivity and scattering efficiency, surface-enhanced Raman spectroscopy (SERS) constitutes an analytical technique of choice for the rapid detection of molecules over traditional Raman spectroscopy. Recently, SERS has attracted increasingly more attention for the study of atmospheric aerosols, due to its ability to detect the chemical composition of individual aerosol particles.18,19 For instance, Craig et al. applied SERS to characterize atmospheric particles.20 It was also demonstrated that SERS is a powerful tool for determining the mixture state of sulfate-nitrate-ammonium components in atmospheric particles.21 The commonly used substrates for SERS are precious metals such as gold, silver and copper.22,23 Sengupta et al. first reported the use of SERS for bioaerosol characterization using silver or silver colloids.24,25 However, the lack of uniformity in the hotspot distribution results in a low enhancement factor (EF). The enhancement of the SERS signals depends on the surface enhanced effect between the analyte and substrate. A high EF requires a large number of SERS-active electromagnetic hotspots, the distributions in intensity and in space of which are consistent throughout the substrate.26 Consequently, the synthesis and fabrication of ultra-sensitive SERS substrates are critically important. Klarite is a commercially prepared SERS substrate, consisting of gold-coated inverted pyramid nanostructures. It is assembled through a silicon (Si) fabrication process in which a thin gold layer is deposited on an oriented Si surface by using vapor deposition. Subsequently, a combination of chemical etching and optical lithography is used. The inverted pyramid nanostructures are formed due to differential etching that represents a fixed apex angle. Klarite exploits the enhancement in the Raman signal intensity that originates from its specialized inverted pyramid structure. Our previous work established the successful application of Klarite for studying individual abiotic atmospheric aerosols.18 To the best of our knowledge, there has been no research on the study of atmospheric bioaerosols using Klarite.
Here, we demonstrate that the functionalization of the Klarite SERS substrate not only achieves a stable and high-intensity spectrum for robust detection, but also label-free in situ rapid discrimination of live from dead airborne bacteria. This interface can be used to study the changes that occur in the bacterial envelope during different bacterial growth phases. Furthermore, we proved how this SERS-based assay can be implemented for the rapid culture-free and label-free detection of bacteria in the real atmosphere.
Experimental
Bacterial strains
E. coli (DH5 alpha) was used in this study. The protocols for the preparation of this bacterial strain are provided in the ESI.†
Preparation of different percentages of dead bacterial suspension
Two 240 mL batches of E. coli were washed twice with phosphate buffered saline (PBS) by centrifuging at 3750 rpm, for 10 min, at 4 °C. The supernatant was removed, and the pellets were resuspended in PBS because it is isotonic and nontoxic to the cells. Saline helps to maintain the osmotic balance between the internal and external environment of the cell, and also keeps the cells intact. Meanwhile, phosphate buffer maintains the physiological pH. Afterward, one batch of E. coli was autoclaved to kill the bacteria therein. Subsequently, different volumes of live and dead bacterial suspensions were mixed, thereby obtaining different percentages of dead bacteria. For each reaction, 80 mL of bacterial suspension was used to generate bioaerosols.
Laboratory generated bioaerosol samples
The whole system comprises a bioaerosol generator, an Andersen sampler (TISCH Environmental, Inc. USA) and a turbulator enclosed in a closed rectangular chamber (Fig. 1). A bioaerosol generator coupled with three air filter systems, was charged with 80 mL of the bacterial suspension. Air flows out from the compressor towards two air-filter systems (0.1 and 0.01 μm) for the removal of impurities. Then, air flows towards the air dryer system, followed by another air-filter (0.01 μm) for the complete removal of impurities. After that, bioaerosols with an aerodynamic diameter range from 0.5–20 μm were generated by a nebulizer. A tabulator was placed in the center of the chamber for rapid distribution and maintenance of the bioaerosols.27 An eight-stage Andersen sampler was used to collect the bioaerosols at a flow rate of 28.3 L min−1. For each sampling process, Klarite was placed into the Andersen sampler and then simulated (i.e., lab-made) bioaerosols were generated for 10 min. Afterward, the bioaerosols were collected for 10 min with a constant flow rate of 28.3 L min−1. Before sampling for different bacterial strains, the inside of the chamber was disinfected with 70% alcohol, each time.
 |
| Fig. 1 Schematic illustration exhibiting the different stages of bioaerosol generation, collection and detection under SERS. | |
Ambient bioaerosol samples
Airborne bacterial particles were collected at the cafeteria (31°20′09.0′′N, 121°30′14.9′′E) of Fudan University on October 14, 2018. The sampling site was inside the cafeteria after lunch time. Bioaerosols were collected on Klarite using the Andersen sampler with a constant flow rate of 28.3 L min−1, and the sampling time was 10 min.
SERS analyses
Once collected on the Klarite, the airborne bacterial particles were analyzed by using an XploRA confocal Raman spectrometer (Jobin Yvon, HORIBA, France). The Raman spectra measurements were recorded using 785 nm laser excitation at 10 mW with a holographic diffraction grating with 1200 groves per millimeter coupled with a 50x LWD Olympus microscope objective lens (Olympus, 0.5 Numerical Aperture). The confocal slit width was 100 μm, with 10 accumulations at 10 s acquisition time, detecting a spectral region of 600 to 1500 cm−1. When Raman mapping data were collected, nearly 100 spectra were recorded in a 10 × 10 μm2 area with a step size of 1.1 μm. The exposure time and accumulation numbers were 5 s and 10, respectively.
Enhancement factors (EFs) are used to quantify the vibrational modes of interest. According to the following equation,
, where ISERS and INRS are defined as the intensities related to SERS and normal Raman spectroscopy (NRS), respectively, and NSERS and NNRS are defined as the number of molecules involved in both SERS and NRS, respectively.28 As the sample preparation steps in both the SERS and NRS scenarios are identical, INRS and NNRS are assumed to be similar. The calculations for the peak intensities were performed using peak height analysis, after baseline correction.
All the Raman spectral data and peak intensities were measured using LabSpec 6 (HORIBA Scientific) software, whereas data handling was performed using Origin 9.5 (OriginLab).
Results and discussion
Standard bioaerosol samples
To test the SERS ability during the bioaerosol detection assay, laboratory-generated airborne bacterial particles were used to investigate various SERS substrates. Fig. S1† shows the comparison of the SERS enhanced substrate with respect to non-enhanced substrates of simulated bioaerosols of E. coli. Herein, vibrational signals are assigned based on previous studies. The main SERS peaks originate from different cell wall components. The typical SERS peaks of E. coli at 735, 955, 1330 and 1446 cm−1 can be observed (Table S1 in the ESI†). The pronounced peak at 735 cm−1 has been assigned to adenine and glycosidic ring modes in previous studies.29,30 Following contact with the non-enhanced substrates, the bioaerosols exhibit a weak signal at 735 cm−1. By comparison, the peak intensity is significantly enhanced at 735 cm−1 on Klarite (Fig. S1a†). This peak can hardly be detected using the alternative substrates: Cu/Ag film on glass, Au nanoparticles on silicon wafer, Au film on glass and Au film on Cu foil. It can be assumed that the uniform distribution of large quantities of hotspots in Klarite provides a high enhancement factor and good measurement reproducibility for bioaerosol particles, as well as for differentiating between live and dead bioaerosols. To find out how Klarite is an efficient substrate for aerosol detection, we performed finite-difference time-domain (FDTD) simulations in our previous study.18 As our group already reported, the E-field hotspots are strongly localized within these pyramids, specifically toward the apex of the pyramids and close to their sides. Therefore, there is a direct chemical interaction between the substrate and analyzed molecule, which results in a high EF. To further study the enhancement factor of the Klarite substrate as compared to others, we calculated its value at 735 cm−1 and obtained an average EF of 61.77. Thus, the greater EF value demonstrates the advantage of Klarite over the alternative substrates, this advantage being attributable to Klarite's uniform hotspots. Compared to other research on SERS, Klarite is employed as a bioaerosol detection platform for the first time in the present study.
The size and morphology of the simulated bioaerosols of E. coli can be studied under a scanning electron microscope (SEM). Fig. 2 shows the SEM images of E. coli before and after deposition on Klarite. In Fig. 2b, we can see that several bacteria are situated well within the Klarite inverted pyramids. In other words, the Klarite substrate channels some of the E. coli bacteria into the electromagnetic hotspots, which is the precise reason for observing an enhanced Raman signal. Fig. 3 illustrates the intensity of the SERS spectra against the control. Almost no signal can be detected in the control, which is PBS. These results validate the sensitivity of Klarite for the detection of airborne particles of E. coli.
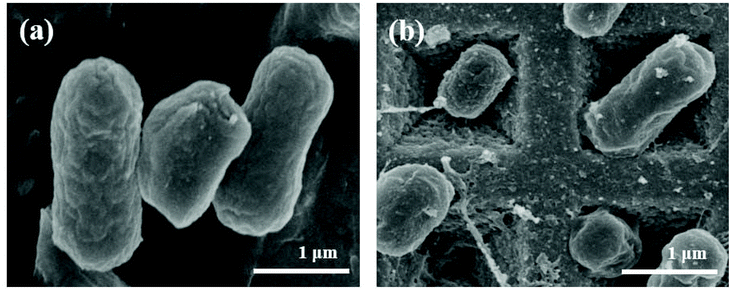 |
| Fig. 2 Scanning electron micrographs of E. coli (a) before and (b) after nebulization on Klarite. Scale bars: 1 μm for both (a) and (b). | |
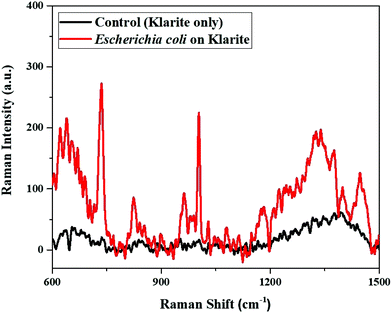 |
| Fig. 3 SERS spectra obtained from E. coli. The SERS spectrum when detecting E. coli (red), and the control spectrum (black) where no bacteria were present. The concentration of E. coli used was 1.8 × 109 cells per mL. | |
Furthermore, the analytical performance of SERS was investigated using different percentages of dead E. coli (Fig. 4a). It can be observed that there is indirect proportionality between the peak intensity and the percentage of dead E. coli cells deposited on Klarite after nebulization. The SERS signal intensity of E. coli gradually decreases with the increase in the percentage of dead bacteria; there is hardly any observable SERS signal once the entire population of bacteria is dead. The plot of the intensity of the 735 cm−1 peak in the SERS spectra versus the percentage of dead E. coli is illustrated in Fig. 4b, and can be fitted to a line with a correlation coefficient of 0.9923. This result shows that there is a good linear relationship between the SERS intensity and the analyte concentration, further revealing that different percentages of dead airborne bacteria can be quantified by using SERS. However, the signal intensity drops when the cell wall/cell envelope is altered due to the modification in surface chemistry and the cellular collapse, which leads to the loss of a subset of proteins with key functions.31–33 We considered that E. coli couldn't complete its metabolism as usual, and it broke down into fractions after sterilization. These fractions couldn't retain their molecular structure. Besides, sterilization may have an impact on the attachments between the E. coli fractions and the substrate, which may also influence the signal intensity. Since dead cells did not attach to the substrate as well as live cells, SERS electromagnetic theory suggests that the enhancement in their Raman signal would not be as good as that for the live cells. However, with live bacteria, most hot spots were generated when the cell wall came into contact with the pyramid structures.18,30 Hence, the distinct shape distribution of Klarite is favorable for calculating the bacterial concentration in a quantitative manner.
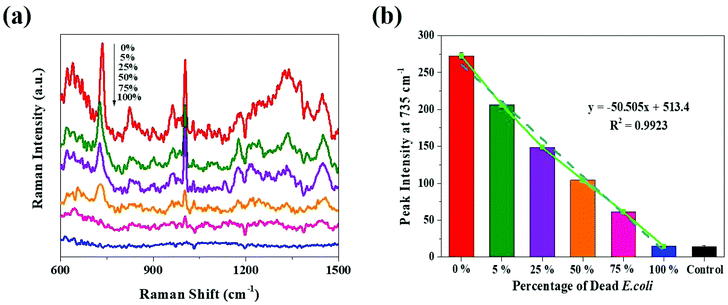 |
| Fig. 4 SERS spectra obtained from different percentages of dead E. coli. (a) SERS spectra and (b) SERS peak intensity at 735 cm−1 from 0% to 100% dead E. coli from top to bottom. The SERS spectrum of the control exhibits that no bacteria were present. The tendency chart shows the linear SERS response with respect to the percentage of dead E. coli. The concentration of E. coli used was 1.8 × 109 cells per mL. | |
To further study the distribution of different percentages of dead bacteria on Klarite, we turned to Raman mapping technology. SERS mapping was conducted with bioaerosols containing different proportions of alive and dead E. coli. Therefore, bioaerosols were deposited homogeneously on the SERS substrate and a thin bacterial film formed at the concentration of 1.8 × 109 cells per mL. The SERS maps in Fig. 5 represent the point by point mapping of the deposited different concentrations of airborne E. coli particles in a region of about 10 × 10 μm2 with a mapping step size of 1.1 μm. The maps were quantified by peak intensity at 735 cm−1. Each black spot indicates dead bacteria, whereas living bacteria are represented by other spots depending upon their intensity. There is a clear correlation between the proportions of alive and dead bacteria, depending upon the varying number of black spots in SERS mapping.
 |
| Fig. 5 SERS mapping of different percentages of dead E. coli. The percentages of dead E. coli are (a) 0%, (b) 5%, (c) 25%, (d) 50%, (e) 75% and (f) 100%. The black squares indicate dead bacteria. The concentration of E. coli used was 1.8 × 109 cells per mL. The objective lens was ×50LWD. | |
In addition, the SERS-based Klarite technique can be applied to discriminate between individual live and dead airborne bacteria. As shown in the bright field microscopy image in Fig. 6a, after the deposition of E. coli airborne particles on Klarite, two bacteria were selected for this detection assay (inset Fig. 6a). The results are shown in Fig. 6c and d. A SERS signal indicates a living bacterium and no SERS signal indicates that the bacteria are dead. Fig. 6b shows the corresponding SERS mapping that differentiates living from dead bacteria. A suspension containing equal proportions of live and dead bacteria was used in this experiment. The total number of bacteria was counted from the bright field microscopy image. A total of 31 bacteria were identified within the field of view of the bright field microscope. SERS signals were obtained for 16 bacteria, upon mapping a region of about 10 × 10 μm2 with a mapping step size of 1.1 μm. From this mapping experiment, we conclude that 16/31 = 51% of the bacteria were alive on Klarite. The data clearly confirm the expected equal proportions of alive versus dead bacteria. Furthermore, we can also calculate the bacterial concentrations depending upon their peak intensity. We can infer that there is a relation between bacterial concentration and disease severity. As the bacterial concentration increases, the disease will become more severe, and the bacterial load is higher in those patients that died as compared to those patients that were diagnosed with a milder disease form.34
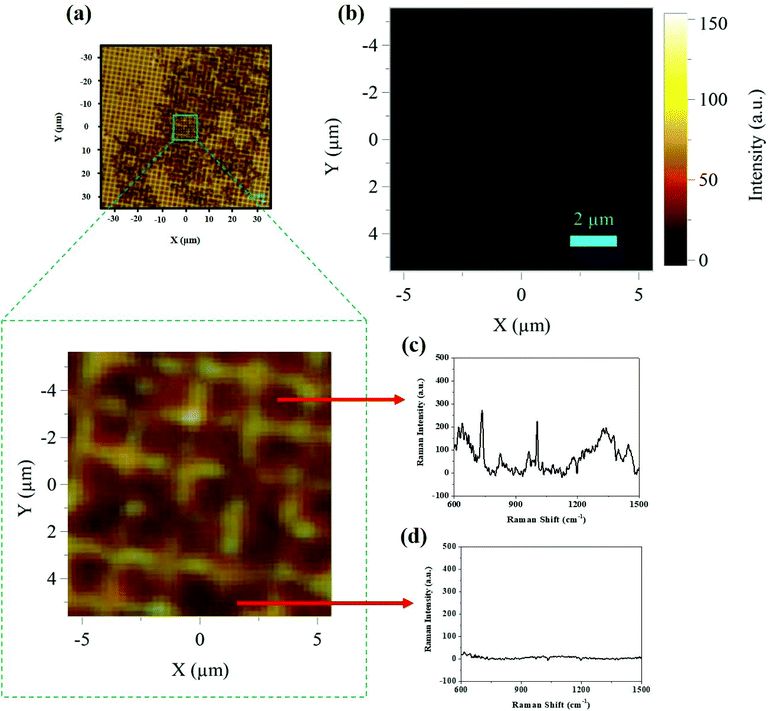 |
| Fig. 6 SERS spectra for the discrimination of live and dead bioaerosols. (a) Bright field image; the inset image shows the mapping region, the area is 10 × 10 μm2 and a 50× objective lens was used. (b) SERS mapping performed at 735 cm−1. (c) SERS spectrum of alive E. coli. (d) SERS spectrum of dead E. coli. The concentration of E. coli used was 1.8 × 109 cells per mL. | |
Moreover, the ultra-high SERS sensitivity can be used to study the changes occurring in the bacterial envelope, as the bacteria divide at different growth phases. Fig. S2† depicts the optical density of E. coli at three distinct growth phases measured at 600 nm (OD600) 0.4, 1.5 and 2.5, which illustrates the growth of bacteria at the beginning of the exponential phase, middle to late exponential phase and stationary phase, respectively. Notably there is a change in the SERS spectra of E. coli (Fig. 7), as the bacterial growth moves from the exponential phase starting (OD600 = 0.4) towards the end (OD600 = 1.5) and to the stationary phase (OD600 = 2.5).35 The change in the SERS profile with respect to the bacterial growth phase may indicate a change in the morphology of the E. coli cells.
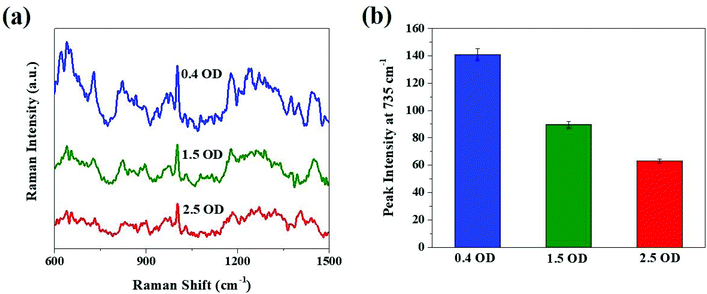 |
| Fig. 7 SERS spectra obtained from E. coli at different growth phases. (a) SERS spectra and (b) SERS peak intensities at 735 cm−1 for this assay. | |
Ambient bioaerosol samples
In this study, we have taken a step forward towards the real application of the SERS-based Klarite assay for the detection of E. coli. in ambient bioaerosols. Bioaerosols were sampled at the cafeteria (31°20′09.0′′N, 121°30′14.9′′E) of Fudan University. The sampled bioaerosols used for culture-free detection of E. coli. under SERS are labeled as the “positive sample” (Fig. 8). Its main peak is in good agreement with results from our previous, laboratory-generated bioaerosols. In contrast, the “negative sample” was collected from the air-flow chamber exposed under ultraviolet (UV) light, which could inactivate airborne microorganisms.36 At 735 cm−1, there is a significant difference between the SERS spectra of our positive and negative samples (Fig. 8). Furthermore, the total analysis time was ∼1 h from sample collection to obtaining SERS results, in contrast to culture-based techniques37 that can take at least 24 h for the results to be obtained.
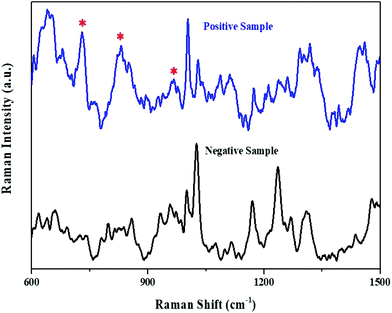 |
| Fig. 8 SERS spectra of ambient bioaerosol samples. SERS spectra of a positive sample (blue) collected from the real-world environment and a negative sample collected from an air flow chamber, where the bacteria were exposed to harmful UV light. The asterisks highlight characteristic peaks that are used to identify the E. coli. | |
On the basis of the above results, SERS mapping shown in Fig. S3† with a 1.1 μm step size covering an area of about 10 × 10 μm2 was further used to detect E. coli in ambient bioaerosols. The maps were quantified by measuring the peak intensity at 735 cm−1. The procedure was similar to that followed for Fig. S3(a):† the number of different spots other than black corresponds to the number of E. coli bound to the Klarite surface. From Fig. S3(b),† it can be clearly seen that no bacteria were detected. All these results confirm that the SERS-based Klarite assay can be used for the detection of bacteria in ambient bioaerosols. This demonstration of detecting bacteria on Klarite under SERS with high sensitivity has the potential for culture-free detection of airborne bacteria in ambient bioaerosols. We believe that further optimization of this method is required, such as finding substrates with greater enhancement factor that may improve the sensitivity further.
Conclusions
SERS is demonstrated to be an ultra-sensitive and rapid analytical technique for atmospheric bioaerosol detection when using Klarite as a substrate. We found that living E. coli generate strong SERS signals, whereas no such signals are observed from dead airborne bacteria. The SERS-based Klarite interface not only detected the airborne bacteria in a qualitative manner, but it also differentiated different percentages of dead airborne bacteria in a quantitative manner. This enabled us to count single live and dead individual bacteria using SERS mapping. Furthermore, we applied this method to study the changes that occur in the bacterial envelope at different growth phases. This interface proved to be successful for culture-free detection of airborne bacteria in ambient bioaerosols. Additionally, SERS also involves short assay time and higher sensitivity in comparison to other commercially available techniques.
Although in general SERS has huge potential for detecting airborne bacteria, both qualitatively and quantitatively, optimizing the substrate enhancement factor remains a challenge. The enhancement and reproducibility of the SERS signals mainly depend on the particle-film spacing, but fully understanding the enhancement mechanism is necessary for the fabrication and development of future SERS substrates. With progress in nanofabrication technology, we can anticipate substrates with much greater enhancement factors, which also maintain reproducibility and present greater aging factors. In time, we envision that portable and efficient SERS devices will be developed and applied for in-field detection of various biological species suspended in the air.
Conflicts of interest
The authors declare no competing financial interest.
Acknowledgements
This material is based upon work jointly supported by the National Natural Science Foundation of China (No. 21976030 and 21677037), the Ministry of Science and Technology of the People's Republic of China (2016YFE0112200 and 2016YFC0202700), the Natural Science Foundation of Shanghai (No. 19ZR1471200 and 17ZR1440200), and the China Postdoctoral Science Foundation funded project (No. KLH1829019). V. K. V. acknowledges support from the Royal Society through the University Research Fellowships and grants URF\R\191016, RGF\EA\180228 and PEF2E\100000.
References
- V. Ramanathan, P. Crutzen, J. Kiehl and D. Rosenfeld, Aerosols, climate, and the hydrological cycle, Science, 2001, 294, 2119–2124 CrossRef CAS.
- U. Lohmann and J. Feichter, Global indirect aerosol effects: a review, Atmos. Chem. Phys., 2005, 5, 715–737 CrossRef CAS.
- D. K. Farmer, C. D. Cappa and S. M. Kreidenweis, Atmospheric processes and their controlling influence on cloud condensation nuclei activity, Chem. Rev., 2015, 115, 4199–4217 CrossRef CAS.
- R. S. Dungan and A. B. Leytem, Qualitative and quantitative methodologies for determination of airborne microorganisms at concentrated animal-feeding operations, World J. Microbiol. Biotechnol., 2009, 25, 1505–1518 CrossRef.
- S. Karra and E. Katsivela, Microorganisms in bioaerosol emissions from wastewater treatment plants during summer at a Mediterranean site, Water Res., 2007, 41, 1355–1365 CrossRef CAS.
- W. O. Cookson, M. J. Cox and M. F. Moffatt, New opportunities for managing acute and chronic lung infections, Nat. Rev. Microbiol., 2018, 16, 111 CrossRef CAS.
- P. Stather, M. Salji, S. Hassan, M. Abbas, A. Ahmed, H. Mills, T. Elston, C. Backhouse, A. Howard and S. Choksy, A comparison of airborne bacterial fallout between orthopaedic and vascular surgery, Ann. R. Coll. Surg. Engl., 2017, 99, 295–298 CrossRef CAS.
- O. Žukovskaja, S. Kloß, M. G. Blango, O. Ryabchykov, O. Kniemeyer, A. A. Brakhage, T. W. Bocklitz, D. Cialla-May, K. Weber and J. r. Popp, UV-Raman Spectroscopic Identification of Fungal Spores Important for Respiratory Diseases, Anal. Chem., 2018, 90, 8912–8918 CrossRef.
- A. K. Deisingh and M. Thompson, Detection of infectious and toxigenic bacteria, Analyst, 2002, 127, 567–581 RSC.
- A. J. Alvarez, M. P. Buttner and L. D. Stetzenbach, PCR for bioaerosol monitoring: sensitivity and environmental interference, Appl. Environ. Microbiol., 1995, 61, 3639–3644 CAS.
- A. Wieser, L. Schneider, J. Jung and S. Schubert, MALDI-TOF MS in microbiological diagnostics—identification of microorganisms and beyond (mini review), Appl. Micribiol. Biotechnol., 2012, 93, 965–974 CrossRef CAS.
- B. L. Dylla, E. A. Vetter, J. G. Hughes and F. Cockerill, Evaluation of an immunoassay for direct detection of Escherichia coli O157 in stool specimens, J. Clin. Microbiol., 1995, 33, 222–224 CAS.
- Q. Liu, Y. Zhang, W. Jing, S. Liu, D. Zhang and G. Sui, First airborne pathogen direct analysis system, Analyst, 2016, 141, 1637–1640 RSC.
- E. Gard, J. E. Mayer, B. D. Morrical, T. Dienes, D. P. Fergenson and K. A. Prather, Real-time analysis of individual atmospheric aerosol particles: Design and performance of a portable ATOFMS, Anal. Chem., 1997, 69, 4083–4091 CrossRef CAS.
- W. Li, L. Shao, D. Zhang, C.-U. Ro, M. Hu, X. Bi, H. Geng, A. Matsuki, H. Niu and J. Chen, A review of single aerosol particle studies in the atmosphere of East Asia: morphology, mixing state, source, and heterogeneous reactions, J. Cleaner Prod., 2016, 112, 1330–1349 CrossRef CAS.
- C. Cai, D. J. Stewart, J. P. Reid, Y.-h. Zhang, P. Ohm, C. S. Dutcher and S. L. Clegg, Organic component vapor pressures and hygroscopicities of aqueous aerosol measured by optical tweezers, J. Phys. Chem. A, 2015, 119, 704–718 CrossRef CAS PubMed.
- M. I. Cotterell, B. J. Mason, T. C. Preston, A. J. Orr-Ewing and J. P. Reid, Optical extinction efficiency measurements on fine and accumulation mode aerosol using single particle cavity ring-down spectroscopy, Phys. Chem. Chem. Phys., 2015, 17, 15843–15856 RSC.
- Y. Fu, C. Kuppe, V. K. Valev, H. Fu, L. Zhang and J. Chen, Surface-enhanced Raman spectroscopy: A facile and rapid method for the chemical component study of individual atmospheric aerosol, Environ. Sci. Technol., 2017, 51, 6260–6267 CrossRef CAS PubMed.
- M. Gen and C. K. Chan, Electrospray surface-enhanced Raman spectroscopy (ES-SERS) for probing surface chemical compositions of atmospherically relevant particles, Atmos. Chem. Phys., 2017, 17, 14025–14037 CrossRef CAS.
- R. L. Craig, A. L. Bondy and A. P. Ault, Surface enhanced Raman spectroscopy enables observations of previously undetectable secondary organic aerosol components at the individual particle level, Anal. Chem., 2015, 87, 7510–7514 CrossRef CAS PubMed.
- Z. Sun, F. Duan, K. He, J. Du and L. Zhu, Sulfate–nitrate–ammonium as double salts in PM2. 5: Direct observations and implications for haze events, Sci. Total Environ., 2019, 647, 204–209 CrossRef CAS.
- Y. Jiao, J. D. Ryckman, P. N. Ciesielski, C. A. Escobar, G. K. Jennings and S. M. Weiss, Patterned nanoporous gold as an effective SERS template, Nanotechnology, 2011, 22, 295302 CrossRef.
- S. Laing, K. Gracie and K. Faulds, Multiplex in vitro detection using SERS, Chem. Soc. Rev., 2016, 45, 1901–1918 RSC.
- A. Sengupta, M. Laucks, N. Dildine, E. Drapala and E. Davis, Bioaerosol characterization by surface-enhanced Raman spectroscopy (SERS), J. Aerosol Sci., 2005, 36, 651–664 CrossRef CAS.
- A. Sengupta, N. Brar and E. J. Davis, Bioaerosol detection and characterization by surface-enhanced Raman spectroscopy, J. Colloid Interface Sci., 2007, 309, 36–43 CrossRef CAS.
- S. Schlücker, Surface-Enhanced raman spectroscopy: Concepts and chemical applications, Angew. Chem., Int. Ed., 2014, 53, 4756–4795 CrossRef.
- X. Li, X. Zhang, Q. Liu, W. Zhao, S. Liu and G. Sui, Microfluidic System for Rapid Detection of Airborne Pathogenic Fungal Spores, ACS Sens., 2018, 3, 2095–2103 CrossRef CAS.
- S. L. Kleinman, R. R. Frontiera, A.-I. Henry, J. A. Dieringer and R. P. Van Duyne, Creating, characterizing, and controlling chemistry with SERS hot spots, Phys. Chem. Chem. Phys., 2013, 15, 21–36 RSC.
- J. Chen, X. Wu, Y.-w. Huang and Y. Zhao, Detection of E. coli using SERS active filters with silver nanorod array, Sens. Actuators, B, 2014, 191, 485–490 CrossRef CAS.
- H. Zhou, D. Yang, N. P. Ivleva, N. E. Mircescu, S. r. Schubert, R. Niessner, A. Wieser and C. Haisch, Label-free in situ discrimination of live and dead bacteria by surface-enhanced Raman scattering, Anal. Chem., 2015, 87, 6553–6561 CrossRef CAS.
- B. Mackey, C. Miles, S. Parsons and D. Seymour, Thermal denaturation of whole cells and cell components of Escherichia coli examined by differential scanning calorimetry, J. Gen. Microbiol., 1991, 137, 2361–2374 CrossRef CAS.
- J. Lee and G. Kaletunç, Evaluation of the heat inactivation of Escherichia coli and Lactobacillus plantarum by differential scanning calorimetry, Appl. Environ. Microbiol., 2002, 68, 5379–5386 CrossRef CAS.
- P. Leuenberger, S. Ganscha, A. Kahraman, V. Cappelletti, P. J. Boersema, C. von Mering, M. Claassen and P. Picotti, Cell-wide analysis of protein thermal unfolding reveals determinants of thermostability, Science, 2017, 355, eaai7825 CrossRef.
- S. Hackett, M. Guiver, J. Marsh, J. Sills, A. Thomson, E. Kaczmarski and C. Hart, Meningococcal bacterial DNA load at presentation correlates with disease severity, Arch. Dis. Child., 2002, 86, 44–46 CrossRef CAS.
- T.-T. Liu, Y.-H. Lin, C.-S. Hung, T.-J. Liu, Y. Chen, Y.-C. Huang, T.-H. Tsai, H.-H. Wang, D.-W. Wang and J.-K. Wang, A high speed detection platform based on surface-enhanced Raman scattering for monitoring antibiotic-induced chemical changes in bacteria cell wall, PLoS One, 2009, 4, e5470 CrossRef.
- A. A. Aliabadi, S. N. Rogak, K. H. Bartlett and S. I. Green, Preventing airborne disease transmission: review of methods for ventilation design in health care facilities, Adv. Prev. Med., 2011, 2011, 21 CrossRef.
- W. M. Fedio, K. C. Jinneman, K. J. Yoshitomi, R. Zapata, C. N. Wendakoon, P. Browning and S. D. Weagant, Detection of E. coli O157: H7 in raw ground beef by Pathatrix™ immunomagnetic-separation, real-time PCR and cultural methods, Int. J. Food Microbiol., 2011, 148, 87–92 CrossRef CAS.
Footnote |
† Electronic supplementary information (ESI) available. See DOI: 10.1039/c9an01715a |
|
This journal is © The Royal Society of Chemistry 2020 |
Click here to see how this site uses Cookies. View our privacy policy here.