DOI:
10.1039/C9NH00380K
(Review Article)
Nanoscale Horiz., 2020,
5, 431-453
Organic–inorganic hybrids for CO2 sensing, separation and conversion
Received
5th June 2019
, Accepted 4th December 2019
First published on 11th December 2019
Abstract
Motivated by the air pollution that skyrocketed in numerous regions around the world, great effort was placed on discovering new classes of materials that separate, sense or convert CO2 in order to minimise impact on human health. However, separation, sensing and conversion are not only closely intertwined due to the ultimate goal of improving human well-being, but also because of similarities in material prerequisites – e.g. affinity to CO2. Partly inspired by the unrivalled performance of complex natural materials, manifold inorganic–organic hybrids were developed. One of the most important characteristics of hybrids is their design flexibility, which results from the combination of individual constituents with specific functionality. In this review, we discuss commonly used organic, inorganic, and inherently hybrid building blocks for applications in separation, sensing and catalytic conversion and highlight benefits like durability, activity, low-cost and large scale fabrication. Moreover, we address obstacles and potential future developments of hybrid materials. This review should inspire young researchers in chemistry, physics and engineering to identify and overcome interdisciplinary research challenges by performing academic research but also – based on the ever-stricter emission regulations like carbon taxes – through exchanges between industry and science.
1 Introduction
The steadily rising level of CO2 in the atmosphere and subsequent ocean acidification are environmental and health issues concerning everyone. Following the industrial revolution, human activities have significantly altered the global carbon cycle. Since the late 19th century, atmospheric CO2 concentrations have increased from 275 ppm to more than 400 ppm with a record increase of 3.3 ppm between 2015 and 2016. There are both natural and anthropogenic sources of CO2. Natural sources include animal and plant respiration or decomposition of organic matter, whereas anthropogenic sources include for example the combustion of fossil fuels, cement production, or power generation. Natural CO2 sinks on the other hand include ocean- and terrestrial-based regions. In fact, oceans absorb roughly 40% of atmospheric CO2.1 To decrease atmospheric CO2 levels and to mitigate the adverse effects related to it, CO2 needs to be efficiently separated and captured at its source, e.g. power plants or chemical factories, using for example amine scrubbers or gas separation membranes. CO2 can then be recycled, e.g. in modified atmosphere packaging,2 as a supercritical solvent and heat transfer fluid,3,4 or in enhanced oil recovery. Alternatively, CO2 can be converted to various reaction precursors or fuels such as carbon monoxide, methanol, ethanol, ethylene, formic acid, or formaldehyde. Particularly, solar irradiation can be utilized as an inexhaustible energy source to power electrocatalytic devices or to directly convert CO2 in a photocatalytic approach. Importantly, separation and conversion processes need to be monitored accurately, thus requiring precise CO2 sensors. Consequently, there is an ever-increasing need for functional materials that display application-specific interaction with CO2. In this context, the use of organic–inorganic hybrids is a viable option because they combine typical properties related to organic materials (gas diffusivity, mass transport, flexibility, or chemical reactivity) and inorganic materials (surface area, mechanical stability, conductivity, or magnetic and optical properties), thus yielding new or enhanced properties such as internal surface area, functionality, light harvesting, or mass transport. Additionally, the solution-processability of hybrids is a major advantage because it allows low-cost printing or roll-to-roll fabrication of flexible materials at industrial scales. Typical application areas include environmental gas sensors,5 solar cells,6 separation membranes,7 catalysts,8 proton conducting membranes,9 and supercapacitors.10 Various aspects of history, preparation, and design of hybrids have been covered previously.11–13
Here, we explicitly focus on current trends and novel ideas of how to take an advantage of synergistic interaction between organic and inorganic materials used for sensing, separation, and catalytic conversion of CO2. To this end, we first briefly introduce the most frequently utilized components of hybrid structures (Section 2) and their properties that make them suitable for CO2-based applications, as shown in Fig. 1. We discuss specific figures of merit and highlight the most exciting examples of hybrid structures utilized in sensing (Section 3), separation (Section 4), and catalytic conversion (Section 5) of CO2, respectively. However, in these sections we refrain from comparing performance metrics because these highly depend on the experimental setup and are difficult to compare without effective benchmarking. Instead, we highlight common synergistic benefits of organic–inorganic hybrid materials across multiple material classes and identify experimental obstacles that limit their performance comparison.
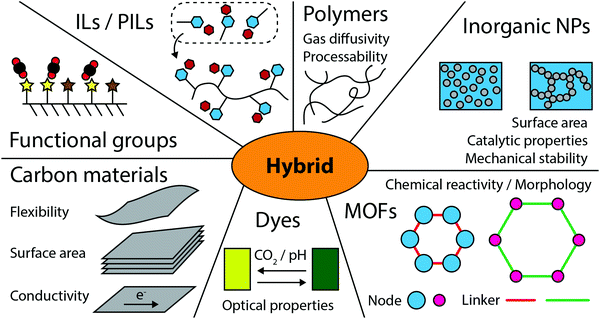 |
| Fig. 1 Most common building blocks utilized for designing hybrid materials for CO2 related applications. | |
Finally, we give an outlook on future trends in the development of CO2-active hybrid materials.
2 Individual organic & inorganic building blocks
Functional groups
Here, we discuss the chemical background behind the interaction of CO2 with commonly used functional groups that can be bonded onto or incorporated into solid materials in order to tune the chemical properties of otherwise unreactive surfaces. For example, amines interact with CO2 based on the hard soft acid base (HSAB) concept and dipole–quadrupole interactions.14 Indeed, CO2 (hard Lewis acid) has a strong affinity towards primary and secondary amines (hard Lewis base) under the formation of alkylammonium carbamate complexes: | CO2 + 2RNH2 ⇄ RNHCOO− + RNH3+ | (1) |
| CO2 + 2R2NH ⇄ R2NCOO− + R2NH2+ | (2) |
| CO2 + R2NH + RNH2 ⇄ R2NCOO− + RNH3+ | (3) |
| CO2 + R2NH + RNH2 ⇄ RNHCOO− + R2NH2+ | (4) |
The anhydrous carbamation reaction (eqn (1)–(4)) cannot occur for tertiary amines. Instead, in the presence of humidity hydrogen carbonate forms:
| CO2 + RNH2 + H2O ⇄ RNH3+ + HCO3− | (5) |
| CO2 + R2NH + H2O ⇄ R2NH2+ + HCO3− | (6) |
| CO2 + R3N + H2O ⇄ HCO3− + R3NH+ | (7) |
Note that primary amines (eqn (1)) are typically more reactive than secondary amines (eqn (2)). Compared to primary and secondary amines, tertiary amines (eqn (7)) are not as reactive, but can be regenerated at moderate temperatures.15 Further, CO2 loading is limited to 0.5 mol of CO2 per mol of primary or secondary amines, compared to 1 mol of CO2 per mol of tertiary amines.
At room temperature, the equilibrium of these reactions is typically on the right side of the reaction formula, while elevated temperatures promote desorption and evaporation of CO2, thus pushing it to the left side. Examples of chemicals with high primary and secondary amine loading include diethylenetriamine (DETA), triethylenetetramine (TETA), and tetraethylenepentamine (TEPA). Alternatively, CO2 also interacts with water (eqn (8) and (9)).
| CO2 + H2O ⇄ H2CO3 ⇄ HCO3− + H+ | (8) |
| HCO3− + H+ + H2O ⇄ HCO3− + H3O+ | (9) |
The formation of protons and hydronium ions is utilized for instance in colorimetric CO2 sensors based on pH indicators16,17 or tunable ion conductors.18
However, the presence of amines and/or water may lead to corrosion which not only triggers catalytic degradation of amine functional groups but also causes degradation of equipment.19,20 Therefore, corrosion inhibitors or alternatives to amines like ionic liquids are of major interest. Ionic liquids (ILs) are salts that have melting points below 100 °C. In particular, room temperature ILs (RTILs) are liquid at room temperature. Besides their negligible vapor pressure, ILs display good chemical and thermal stability. Generally, ILs are composed of organic cations such as imidazolium, pyridinium or ammonium ions and of anions ranging from halides, to polyatomic inorganic anions such as tetrafluoroborate [BF4]− and hexafluorophospate [PF6]− or to large organic anions like bistriflimide [Tf2N]−. Owing to the large variety of ions available, physicochemical properties and affinity towards CO2 can be readily adjusted.21–23 For example, ILs with high CO2 solubility were synthesized by using ammonium, imidazolium, or pyridinium cations.24–31 Besides minor interactions with the cation, CO2 (hard Lewis acid) primarily interact with [BF4]−, [PF6]−, or [Tf2N]− anions (hard Lewis base) by accepting partial electric charges from the electronegative fluorine atoms.21,32 Although the affinity of CO2 towards the respective cations seems to be marginal, the side chain length of a cation can control free volume to accommodate CO2.21
Polymers
The affinity of solid substrates towards CO2 can be increased through functionalization with amines or ionic liquids. However, as prepared materials are typically limited to silicas due to presence of surface hydroxyl groups and suffer from relatively low number of functional groups and insufficient efficiency.33 Therefore, polymers that directly interact with CO2 are interesting for uses requiring functional films with good gas selectivity and permeability, e.g. in gas sensing, separation or conversion. Many polymers show high amine loading and are used to selectively absorb CO2. Examples include amidine and guanidine-based polymers,34,35 poly(ether imide),36 or poly(ethylene-imine).37 Alternatively, by polymerizing ILs, so-called polymerized ionic liquids or poly(ionic liquid)s (PILs) are obtained. Unlike most IL precursor, PILs are usually solid at room temperature. Several PILs have been shown to selectively and reversibly absorb CO2.27,38,39 The use of PILs in capture and separation was recently reviewed elsewhere.40
Also, the polymer nanostructure, i.e. nanopores or segregated microphases can affect the diffusion of gases or reactants, thus proving useful in gas separation and catalysis. For example, polymers such as polystyrene (PS), polysulfone (PSf), or poly(ether-b-amide-6) (PEBA) are used owing to their controllable porosity as well as their good CO2 permeability and selectivity.41 PEBA is a block-copolymer composed of amorphous and permeable polyethylene oxide (PEO) units interacting with CO2via dipole–quadrupole interaction42 as well as crystalline polyamide (PA) segments giving mechanical stability.43
Alternatively, porous organic polymers synthesized from individual multidentate organic building blocks can be used for nanostructuring. Depending on polymerization conditions, cross-linking of polymer can be influenced to obtain nano (<2 nm), meso (2–50 nm), and macroporous (>50 nm) materials.44 Due to high surface area, large pore volume and wide range of building blocks, porous organic polymers display a huge potential as CO2 adsorbent and as a catalytic platform. Diverse synthetic routes and challenges related to the pore size distribution have been covered previously.45,46
Through thermal decomposition of polymeric structures with a well-defined porous structure, microporous carbon materials with precisely tuned structure can be obtained. Indeed, the pyrolysis of nitrogen-containing polymers, such as polypyrrole,47 melamine formaldehyde resin,48 polyacrylonitrile49 and polyaniline50 results in N-doped porous carbon materials, thus improving CO2 adsorption capabilities when compared to pure carbon frameworks. It is however still under debate whether the increased CO2 adsorption by N-doping is a result of facilitated CO2 adsorption by acid–base interaction, improved hydrogen-bonding capability or by residual ions.51,52 Owing to their low cost, high thermal and chemical stability, controllable pore structure, high surface area and abundant nitrogen surface atoms, N-doped carbon materials are gaining significant attention for CO2 capture and separation.53,54
Moreover, by incorporating inorganic building blocks within the polymer matrix inherent properties of inorganic nanomaterials or additional features of the formed polymer–nanoparticle-interface can be observed while maintaining flexibility and processability of polymeric materials.55 However, weak interfacial bonding is still an obstacle for obtaining uniform dispersions of nanomaterials in polymer matrices. For instance, functional groups that interact with the surface of particles can be integrated for steric stabilization of inorganic building blocks to avoid loss of functionality as a result of agglomeration, which is currently one of the major challenges for practical application.56
Dyes
Even though dyes usually do not interact directly with CO2, their pH-sensitivity can be used to detect and respond to proton concentration changes occurring upon the interaction of CO2 with water or humidity (see eqn (7) and (8)). This effect is utilized in various optical CO2 sensors. pH-Sensitive dyes include thymol blue (TB),57–59 bromothymol blue (BTB),58 phenol red (PSP),57,59 methyl red,59 cresol red,57 or luminescent 1-hydroxypyrene-3,6,8-trisulfonate (HPTS).60 Finally, porphyrine-based dyes can be used to promote the absorption of visible light in photocatalytic conversion of CO2.61
Carbon materials
Graphene exhibits exceptional mechanical, optical, thermal, and electrical properties while having a low density and it has become a real game changer in material science.62 It is a zero band gap semiconductor with symmetric band structure showing high electron mobility.63,64 Moreover, graphene displays a 2-dimensional nanosheet morphology resulting in high specific surface area and its π-conjugated structure supports the interaction with π-bonds in CO2.65 Therefore, graphene and its derivatives such as graphene oxide (GO) or reduced graphene oxide (rGO) are now utilized in sensing, separation, and catalytic conversion.66–69
Graphene oxide (GO) can be synthesized by chemical oxidation and exfoliation of graphite using strongly oxidizing agents. In contrast to graphene, the surface of GO is heavily decorated with electron-rich, oxygen-containing, and highly reactive polar groups such as hydroxyls, epoxides, or carboxyls, thus exhibiting oxygen contents of up to 40 at%.70 The electron-donating nature of these moieties leads to preferential adsorption of CO2 through dipole–quadrupole interaction.71 Furthermore, the interlayer spacing of GO can be adjusted, thus making it interesting for membrane applications.71–76 Moreover, exfoliated GO nanosheets are hydrophilic and thus stable in water. Therefore, organic solvents are not necessary and the environmental friendliness of industrial scale production and processing is enhanced.
By reducing GO, it is possible to partially restore the properties of graphene.77 The reduced graphene oxide (rGO) shows good electrical conductivity, dominated by positively charged carriers (holes).78 Consequently, when CO2 interacts with functional groups at the rGO surface by transferring an electron to rGO, the hole concentration decreases and the resistance increases. This effect is a chemical foundation for CO2 sensing.79,80
Graphitic carbon nitride (g-C3N4) is closely related to graphene, GO, and rGO. It is a 2-dimensional, semiconducting material with a band gap of about 2.7 eV and is composed of tri-s-triazine units that are interconnected by planar tertiary amino groups. It can be prepared from low-cost nitrogen- and carbon-rich precursors such as cyanamide, dicyandiamide, melamine, and urea.81–83 By altering the precursor or post-synthetic functionalization, the electronic band structure of the material and its light absorption properties can be tuned,84 thus making it attractive for various catalytic applications.85
Metal–organic frameworks
Metal organic frameworks (MOF) are an emerging class of crystalline hybrid materials consisting of metal containing nodes, also called secondary building units (SBU), that are interconnected by organic linkers forming a 1-, 2-, or 3-dimensional porous network. MOF crystals typically display large internal cavities that are interconnected by small channels.86 Owing to their highly ordered nanoporosity of up to 90%, MOFs provide correspondingly large internal surface area.18,87 Moreover, the molecular building blocks can be varied systematically, which is a major reason for the exceptionally high chemical and functional versatility as well as adjustable internal surface properties. Consequently, and in contrast to other porous materials such as zeolites or aerogels, MOFs show precisely tunable pore size in a range of 2–100 Å.88–92 For example it is possible to fit the size of molecular guests, thus even enabling H2/D2 isotope separation by quantum sieving.92 Furthermore, enzyme-like selectivity was demonstrated after postsynthetic modification with molecular recognition groups. Additionally, the incorporation of MOFs as fillers in polymeric matrices is facilitated by their partially organic nature that yields good compatibility. Therefore, MOFs are interesting for numerous applications including molecular sieving,93,94 CO2 separation,95,96 catalysis,97 sequestration,98,99 and chemical sensing.94,100–103 Owing to their tunability, it is possible to design MOFs specifically interacting with CO2.90,104,105 For example, zeolithic imidazole frameworks (ZIF) (Zn/Co SBUs, imidazole-based linkers) are interesting because of their good CO2 capture and sieving properties. For instance, ZIF-8 displays large cages (11.6 Å) and narrow pore apertures (3.4 Å),106,107 which can be tuned such that the large cages contain CO2 absorbing ILs while the narrow pores allow molecular sieving of CO2.108,109 Similarly, MFU-4 (Zn5Cl4 SBUs, benzobistriazolate linkers) displays alternating small (3.88 Å) and large cages (11.94 Å) connected by narrow apertures (2.52 Å).110 Alternatively, MIL-101 (Cr SBUs, terephthalic acid linkers) can easily be functionalized with CO2 sensitive molecules owing to numerous unsaturated chromium sites within the structure. Also, CuBDC (Cu SBUs, 2-hydroxyterephthalic acid linkers) and MIL-53 (Al SBUs, terephthalic acid linkers) display good CO2/CH4 selectivity.36,111,112 Moreover, by incorporating catalytic centers and photosensitizers in MIL-125 (Ti SBUs, 2-aminoterephthalate linkers), it was possible to combine the photocatalytic CO2 reduction activity with large surface area and open porosity of MOFs for improved mass transport.113 This list is by far not exhaustive, but it hints at the versatility of MOFs for application in CO2 separation and conversion. Nevertheless, certain MOFs are water sensitive, thus limiting stability in process environments. Water adsorption concepts, water stability of several MOFs and experimental characterization procedures have been discussed previously.114
Inorganic nanomaterials
Depending on the application, inorganic materials are generally selected based on their morphology or their surface and bulk properties. For instance, in applications that require high specific surface area or short charge carrier diffusion lengths, nano-sized building blocks are employed. Regarding the morphology, structures with various dimensionality such as spherical nanoparticles, nanowires or -chains, nanosheets, and nanocubes that show dimension- and shape-dependent optical and electrical properties can be distinguished.115,116 Moreover, given that different morphologies exhibit different crystallographic surfaces and surface energies, they also display different affinities to CO2. For example, nanoparticles of various shapes show varying activities and selectivities for identical catalytic reactions due to the exposure of different crystal facets.117,118 In addition, catalytic activity also depends on chemical composition. For electrocatalytic reduction of CO2, copper and noble metals like gold, silver and palladium showed to be most effective. However, based on varying ability to stabilize reaction intermediates, copper is uniquely active for reduction of CO2 to hydrocarbons, while gold, silver and palladium form CO as major product.119 For photocatalytic reduction, metal oxides like TiO2, Cu2O, WO3 and Bi2WO6 or metal sulfides as CdS, ZnS were intensively investigated due to their suitable band gap.120 Among these various semiconductor photocatalysts capable of driving CO2 reduction, perovskite oxides (ABO3) emerged as a promising class of materials because band structure, charge transfer and adsorption of CO2 can easily be manipulated and optimized by varying A- and B-site cation composition.121 Besides, more advanced synthesis protocols allow precise fabrication of colloidal semiconductor quantum dots or lead-halide perovskites that can be utilized for CO2 reduction due to the size-dependent band structure.122
These inorganic building blocks can be assembled to macroscopic objects e.g. by formation of aerogels which increases the dwell time of CO2 molecules in the confined space and enables interparticle charge transfer such that lifetime of photoexcited charge carriers is extended.123 Additionally, aerogels can be functionalized with organic groups, thus providing high reactive site densities. Moreover, by tuning the pore size in mesoporous silica materials, good sieving properties can be obtained.
3 Organic–inorganic hybrids for sensing of CO2
In the past years, the evolution of the internet of things (IoT) has yielded a steadily growing interest for different types of sensors and actuators.124 In particular, CO2 sensors that are technologically relevant in exhaust gas analysis, in breath analysis for medical diagnosis, or in indoor air quality monitoring for intelligent ventilation systems are attracting a lot of attention. Gaseous and dissolved CO2 is commonly detected and quantified using the non-dispersive infrared (NDIR) and the potentiometric Severinghaus125 techniques, respectively. The NDIR method is based on the Beer–Lambert law of absorption of infrared radiation by CO2 molecules and shows high selectivity, sensitivity, and cyclability in a large concentration range. In contrast, Severinghaus sensors measure the pH-change of a bicarbonate buffer solution induced by the CO2/H2O interaction. However, both sensor types have limited miniaturization potential, which impedes their use in portable devices. Consequently, considerable efforts were made to develop innovative CO2 sensors based on the resistive and capacitive read-out. The main advantage of this technology lies within its applicability in flexible and printed electronics as well as in battery-driven devices. For example, polymeric materials with tuned functionality were developed and have shown improved gas-sensing performance.126 However, despite many efforts in material design and morphology control, problems of long-term stability, moisture sensitivity and conductivity remain unsolved. Alternatively, inorganic metal-oxide semiconductors show pronounced CO2 sensitivity at operating temperatures of 250–350 °C.127–129 However, because of the high energy demand related to the elevated operating temperatures they are unsuited for applications in battery-driven devices. Moreover, heated sensors could act as ignition sources under flammable condition. Nevertheless, the issues related to organic and inorganic materials can be overcome by utilizing hybrids thereof.5,130,131,135,136
We discuss the main figures of merit used to characterize CO2 sensors and present a selection of hybrid CO2 sensors pooled by their respective transducing technology including electrical, optical, and acoustic sensors. Fig. 2 gives an overview of different sensors discussed herein. We finally summarize the main limitations of these sensors and how they can be tackled.
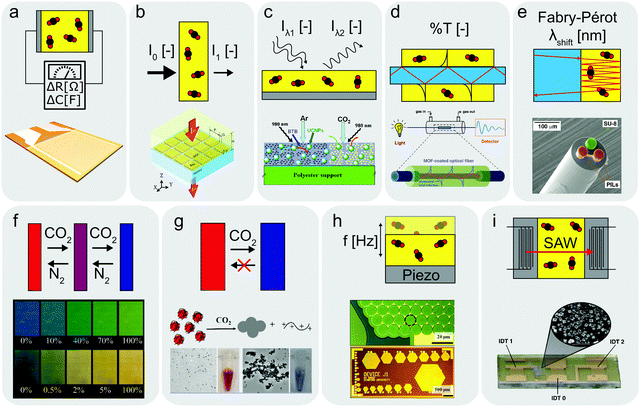 |
| Fig. 2 Schematics of operating principles for different sensors with examples from literature. (a) Resistive or capacitive-type sensor,135,136 (b) IR-type sensor with enhanced CO2 absorption layer,144 (c) optical sensor utilizing upconverting particles,41 (d) optical fibre cladded with CO2 sensitive MOF layer,138 (e) optical sensor based on Fabry–Pérot interferometer,139 (f) colorimetric sensor utilizing pH-sensitive dye,58 (g) unidirectional CO2 indicator,143 (h and i) CMUT35 and SAW142 sensors measuring resonance frequency changes upon CO2 absorption. (b) Reprinted with permission from ref. 144. Copyright 2017 IOP Publishing. (c) Adapted with permission from ref. 41. Copyright 2010 Elsevier. (d) Adapted with permission from ref. 138. Copyright 2018 American Chemical Society. (e) Adapted with permission from ref. 139. Copyright 2017 Elsevier. (f) Reprinted with permission from ref. 58. Copyright 2007 American Chemical Society. (g) Reprinted with permission from ref. 143. Copyright 2016 American Chemical Society. (h) Reprinted with permission from ref. 35. Copyright 2012 Elsevier. (i) Adapted with permission from ref. 142. https://pubs.acs.org/doi/10.1021/acssensors.7b00014 Copyright 2017 American Chemical Society. | |
Figures of merit
Depending on sensor type, different properties of the active material such as resistance, capacitance (electrical sensors), absorption, transmission, wavelength shift, luminescence (optical sensors), or resonance frequency (resonant sensors) are monitored as a function of CO2 concentration. The extent of the measured signal change determines the sensitivity that is typically given as an absolute or relative change with respect to the baseline signal. Sensor kinetics are commonly characterized by the response and recovery times of sensors. For instance, t90 is the time needed to reach 90% of the absolute change measured at equilibrium condition. Slower sensors are sometimes defined by their t50 value. The sensor kinetics strongly depend on CO2 adsorption and desorption rates of the active layer. Moreover, desorption substantially influences the cyclability of a sensor, which is a crucial property for industrial applications. Furthermore, the limit of detection as well as the dynamic range determine the lower and upper bound of the concentration range in which the sensor can be operated, respectively. It is worth mentioning that the necessitated dynamic range depends on the field of application and has therefore to be adjusted accordingly. For example, when controlling air quality, CO2 concentrations below 0.5% have to be detected whereas in food packaging, CO2 concentrations above 80% are usual.132 Finally, the cross-sensitivity of a CO2 sensor towards humidity and other gases as well as environmental effects including temperature and pressure is a key characteristic. For the sensors discussed next, some of these parameters are summarized in Table 1. We refrain from listing response and recovery times because these values strongly depend on gas flow and sample chamber volume, which are not directly comparable from one study to another.
Table 1 Sensor characteristics of hybrid CO2 sensors and indicators discussed within this section
Material |
Carrier gas |
Dynamic range |
Temperature/humidity |
Ref. |
PEI + starch@CNT |
Air |
500 ppm – 10% |
RT/80% rh |
133
|
Hydroquinone@3D-rGO |
Syn. air |
100–1000 ppm |
RT–80 °C/— |
134
|
La2O2CO3 + P[VBTMA][PF6] |
Syn. air |
150–2400 ppm |
RT/50% rh |
135
|
Al2O3 + P[VBTMA][PF6] |
Syn. air |
600–3200 ppm |
RT/30–70% rh |
136
|
PEI + SiO2 |
Syn. air |
400–3000 ppm |
RT/24–78% rh |
137
|
[BMIM][TOS] + silicone |
N2 |
0–100% |
10–50 °C/100% rh |
58
|
ZIF-8@optical fiber |
N2 |
0–100% |
RT/— |
138
|
PAVB@optical fiber |
N2 |
0–75% |
RT–50 °C/— |
139
|
CDMOF-2 |
N2 |
0–100% |
RT/— |
18
|
APTES@mesoporous layer |
N2 |
0–2% |
RT, regeneration at 120 °C/0–80% rh |
35
|
ZIF-8@SAW |
N2 |
5–100% |
RT/— |
140
|
PIL@SWCNT |
N2 |
500 ppt–50 ppm |
RT/42% rh |
141
|
MFU-4@SAW |
N2/He |
1 ppm – 100% |
−63 to 28 °C/— |
142
|
NaYF4:Yb:Er + BTB + PS |
Ar |
1–3% |
RT/100% rh |
41
|
PNAAN@AuNP |
— |
— |
RT/— |
143
|
Resistive and capacitive sensors
A resistive or capacitive CO2 sensor monitors changes of resistance or capacitance of a transducing material as a function of CO2 concentration as depicted in Fig. 2a. Most organic–inorganic hybrid gas sensors combine the chemical activity of a low-conducting organic material with the transducing functionality of a conductive inorganic constituent. The chemical interaction of CO2 with the organic material has a direct impact on charge carrier density or mobility in the transducer or causes relative permittivity variations in the dielectric layer, thus yielding resistance or capacitance changes. Unsurprisingly, carbon-based materials turn out to be ideal transducers.79,133,134,141 For example, PEI-modified carbon nanotube field-effect transistors (NTFETs) were designed for CO2 sensing.133 The selective interaction of CO2 with the primary and secondary amines of PEI leads to the formation of carbamates, which in turn lowers the pH of the polymer layer and reduces the electron-donating effect of PEI to the carbon nanotube channel. In addition, starch was used to improve the PEI/carbon nanotube interaction and because of its hygroscopic nature, permits higher CO2 absorption and results in a stronger protonation of the amino groups of PEI. Similarly, single-walled carbon nanotubes (SWCNT) can be functionalized with percolating networks of ammonium-based P[VBTMA][BF4].141 Owing to the strong interaction of P[VBTMA][BF4] with SWCNT through cation–π and π–π interactions, the polymer chains wrap around the SWCNTs while agglomeration of particles is prevented by repulsive forces among imidazolium cations. As CO2 interacts with [BF4]−, charge transfer from [BF4]− to CO2 will diminish electron donating-effect of [BF4]− to SWCNT, thus increasing the hole concentration and eventually lowering the electrical resistance. An important drawback is that the recovery has to be triggered by an UV illumination step, which decreases the CO2 desorption energy barrier. Others chemically modify 3-dimensional reduced graphene oxide (rGO) with hydroquinone, thus introducing doping and defect sites on the surface of rGO.134 In fact, charge transfer from CO2 to rGO leads to enriched hole concentration in rGO and lower resistance.
In our own research, we disperse inorganic filler particles in polymeric films to increase gas permeability. Even though it might seem counter-intuitive, the addition of nanoparticles leads to increased voids by hindering crystallization of polymers. We synthesize P[VBTMA][PF6]/La2O2CO3135 as well as P[VBTMA][PF6]/Al2O3136 hybrids for CO2 sensing. In the first study, La2O2CO3, which is inherently sensitive towards CO2 at elevated temperatures,127,145 was combined with P[VBTMA][PF6] that is able to reversibly absorb large amounts of CO2, eventually acting as a preconcentrator. In the follow-up study, the same principle was demonstrated, but we replace La2O2CO3 by Al2O3 particles because of recyclability, environmental, and ecological reasons and because of their inertness to other gases. We find that the role of particles mainly consists in increasing gas permeability and allowing fast and reversible sensing, while CO2 influences the electric and ionic conductivities of the polymer measured via DC resistance and AC impedance. Impedance studies highlight the importance of protons during CO2 sensing, supported by the fact that a minimum relative humidity around 40% is needed so that its interaction with CO2 yields protons and hydrogen carbonate (eqn (8)).
A similar behavior was observed in highly porous, γ-cyclodextrin-based CDMOF-2.18 The noncovalent bonding of CO2 with readily available free hydroxyl groups forms alkyl carbonic acid, which leads to a decrease of proton conductivity. CDMOF-2 is highly sensitive in the low CO2 concentration regime and displays good reproducibility over several cycles.
Besides measuring electrical resistance or ionic conductivity changes upon exposure to CO2, it is also possible to track the capacitance of a sensor as a function of time. For instance, silica nanoparticles that are combined with PEI by wet-impregnation display linear capacitance changes as a function of CO2 and humidity.137,146 The measurements show good reproducibility even after ageing for several weeks at ambient conditions.
Because sensors based on the electrical principle transduce a chemical signal that is induced by a certain number of adsorption sites into an electrical signal, the sensor operability is usually limited to low CO2 concentrations (<10%), while it saturates at higher concentrations. This is one of the reasons driving the search for alternative detection principles such as via optical read-out, which enables measurements also at high concentration (10–100%).
Optical sensors
Optical gas sensors typically measure either the infrared radiation absorption or colorimetric and fluorescence intensity changes of a sensing layer as illustrated in Fig. 2b–g.147 The main benefits of optical sensors are their large dynamic range, their high selectivity, and their usability for remote or electrically isolated sensing. Furthermore, they are applicable for the detection of dissolved CO2, which is needed for CO2 monitoring in blood or seawater and which makes them serious competitors to current Severinghaus detectors. Many efforts are made to find smaller alternatives to conventional NDIR sensors. Some studies focus on improving NDIR sensors e.g. by using enhanced thermal IR emitters148 or by using amine-functionalized structures to increase IR absorption, eventually reducing the light path length needed to reach a given sensitivity.144,149 For example, porous Al2O3 substrates were functionalized with a glutaraldehyde-interlinked PEI film that acts as an enrichment layer.149 Likewise, metal–organic frameworks can be combined with plasmonic gold nanopatch arrays, thus increasing the absorption path of on-chip sensors.144
Alternatively, CO2 sensors monitoring the color or the fluorescence of the sensing layer take advantage of the pH changes induced by the CO2/H2O interaction. Through adding a dye that changes its color upon protonation, the color is indicative of CO2 content. Additionally, the sensitivity can be adjusted by tuning the pKa value of the pH indicator, thus allowing precise CO2 measurement in a well-defined concentration range. As an example, the usability of silicone-encapsulated room temperature ILs was demonstrated.58 As CO2 dissolves in the buffer and reacts with [BMIM][TOS], the pH increases leading to a colorimetric change of the dye. The fluorescence intensity decreases exponentially with CO2 concentration and the sensitivity can be tuned by the choice of the room temperature ionic liquid (RTIL) counteranion. However, photobleaching in the presence of oxygen is a possible reason for fluorescence intensity decrease over time.
The use of optical fibers is attractive owing to their mechanical flexibility and in situ/in vivo operability.150,151 For example, the cladding can be removed by etching to enable subsequent surface functionalization with a sensitive coating. Since CO2 alters the refractive index of the sensing layer, the evanescent waves propagating into the layer as well as the transmission through the fiber will be affected.152 For instance, porous ZIF-8 films can be deposited on the surface of an optical fibre.138 CO2 molecules selectively interact with the MOF material, influencing pore filling and refractive index. As the refractive index of the MOF-film draws closer to the refractive index of the fiber, a larger portion of light propagates into the film causing a linear decrease of transmittance, which is in direct correlation with CO2 concentration. The challenge of this method lies in the detection of extremely small variations in refractive index induced by CO2. Others have used hybrids containing thymol blue (TB) dye and found logarithmic dependence of sensor signal with CO2 concentration.17 Alternatively, the sensitive layer can be deposited onto the fiber tip, giving rise to a Fabry–Pérot interferometer (FPI)153 for example by direct μ-printing of poly(allyl-3-vinylimidazolium bromide) (PAVB) on the fiber tip.139 Indeed, the reversible CO2 absorption of PAVB induces refractive index changes measurable by a shift of the interference peak. Interestingly, because a multi-cored optical fiber can be employed, it is possible to simultaneously measure temperature using SU-8 epoxy control sample. This points towards the possibility to detect and monitor multiple parameters in parallel which is important for signal calibration in electronic noses.
Another interesting approach consists in mixing NaYF4:Yb,Er upconverting nanoparticles together with longwave absorbing and pH-sensitive BTB dye in a PS matrix.41 Upconversion takes advantage of a nonlinear optical process involving the absorption of two or more low-energy photons in long-living energy states with subsequent emission of a single higher-energy photon. The composite film is excited using a NIR-laser, and the dye-induced luminescence can be recorded as a measure of CO2 concentration.
In most applications, reversibility of CO2 sensors is highly desired. However, unidirectional optical CO2 indicators that switch color upon onetime exposure are conceivable, e.g. as an alert sign similar to mechanical shock indicators. For instance, gold NPs were functionalized with CO2-responsive poly(N-(3-amidino)-aniline) (PNAAN).143,154 Because the amidine groups from PNAAN capture protons generated by the CO2/H2O interaction, the polymer becomes hydrophilic, swells and detaches from the gold surface. The subsequent irreversible aggregation of gold NPs leads to a unidirectional color change from red to blue.
Resonant sensors
The resonant gas sensors take advantage of the mass-loading mechanism (see Fig. 2h and i). This effect exploits the fact that whenever a resonant oscillator absorbs CO2, its mass increases, thus leading to a decrease of resonance frequency. For this method to work, high mass sensitivity has to be achieved. This is possible with surface acoustic wave (SAW),155 quartz crystal microbalance (QCM), or capacitive micromachined ultrasound transducers (CMUT).35,156 A major advantage of these transducers lies within their good CMOS compatibility making them interesting, cost-effective candidates for miniaturized gas sensors. For example, an APTES-functionalized mesoporous silica and guanidine-based polymer layer was deposited onto a CMUT substrate.35 The device is operated at its mechanical resonance frequency and shows linear dependence of frequency shift with CO2 concentration without saturating. Alternatively, SAW-based acoustic transducers monitor the propagation of SAWs in terms of amplitude, phase, and wave velocity. Any mass, conductivity, or stiffness change of the sensitive layer will affect these parameters.157 SAW-based sensors have already been utilized for the detection of humidity and organic analytes.158,159 More recently, porous MFU-4 films were directly grown from solution onto SAW substrates for the adsorption and detection of CO2.142 However, because CO2 interacts with MOFs solely by physisorption, the differentiation between CO2 and other adsorbates remains a major issue. Nevertheless, owing to the fast response time and picogram accuracy, it is possible to differentiate absorbed gases by their uptake rate. Furthermore, temperature-dependent measurements allow the evaluation of the activation energy of CO2 uptake and show that at low temperatures, the phase shift increases due to a higher mass loading. In another study, ZIF-8 is deposited onto SAW and QCM transducers and used as the CO2 sensitive material with SAW sensors outperforming QCM devices.140
Common limitations of sensors
A notable issue of many sensors is the cross-sensitivity towards interfering gases. Therefore, it is necessary to design sensors depending on their application field. Conceivable approaches include the use of coordinated sensor arrays that enable calibration procedures or sensor filters and coatings for the encapsulation of the sensing material. Alternatively, environmental effects can be compensated by specially designed circuitry, e.g. Wheatstone bridges.
In addition, sensors should ideally be reversible and recover rapidly under mild conditions. Sensors that display high selectivity typically interact chemically with the analyte resulting in high activation energy for CO2 desorption. As a consequence, pulsed or continuous heating as well as UV illumination are still required for many sensors.134 Possible solutions include the optimization of sensing layer morphology as well as the use of physisorbing materials showing enhanced reversibility but with the drawback of lower selectivity. Regarding the response and recovery times of sensors, direct comparison of these characteristics from different studies is difficult because they are strongly dependent on gas flow as well as the volume of the respective flow chambers. Apart from this, an interesting approach using magnetic nanostirrers for enhanced response in gas sensors has been demonstrated recently.160
Cyclability and long-term stability are usually tested on a small number of pulses within a relatively short time scale, i.e. hours and days. However, it is necessary to test the sensors in a longer time scale to prove their usability in real-life applications. This is particularly important for sensors working in aggressive environments that are detrimental to the service life of the sensing layer. An alternative approach consists in protecting the sensor layers by employing coatings with selective gas permeability. Furthermore, the temperature and pressure-dependence on sensitivity, foremost for absorption-based sensors, requires calibration of both temperature and pressure using reference sensors. Finally, there is a traditional trade-off between slow desorption at low temperatures and low sensitivity at high temperatures.
4 Organic–inorganic hybrids for separation of CO2
The efficient separation of CO2 from gas mixtures is important e.g. in flue gas treatment (CO2/N2), natural gas sweetening (CO2/CH4), or syngas cleaning (CO2/H2).161–165 Conventional separation processes include amine scrubbing by aqueous alkanolamine solutions, pressure swing absorption, or cryogenic distillation.166–168 However, these processes are costly and/or energy intensive during regeneration, thus lowering plant efficiency and impeding the use in large-scale industrial applications. Furthermore, amine-based processes suffer from the volatility and corrosivity of amine solutions. A promising alternative is the use of solid separation membranes, which are characterized by simple operation, low energy consumption, and environmental friendliness.169–172 For instance, dense polymeric membranes show reasonable selectivity but an inherent permeability–selectivity trade off limits their use.173–175 Alternatively, inorganic membranes show enhanced permeability–selectivity,93 but these membranes are difficult to fabricate, expensive, and brittle. In fact, the low mechanical strength makes them particularly vulnerable to the high transmembrane pressure drop that is required for efficient separation. Recently, mixed matrix membranes (MMM), which are fabricated by incorporating filler particles such as zeolites, MOFs, carbon-based materials, or mesoporous silica into polymeric matrices, have been demonstrated to overcome permeability and stability issues.163,176,177
Here, we present the key parameters used to characterize gas separation membranes and describe recent advances in the development of hybrid materials for CO2 separation. Finally, we discuss common limitations and solutions thereto.
Figures of merit
Permeability and selectivity are the most important parameters utilized to characterize gas separation membranes: | Permeabilityi = Diffusivityi·Solubilityi | (10) |
| Selectivityij = Permeabilityi/Permeabilityj | (11) |
While selectivity is unitless, permeability is measured in barrer with 1 barrer = 10−10 cm3 (STP) cm cm−2 s−1 cmHg−1 which is related to the flow rate (cm3 (STP) s−1), membrane thickness (cm) and area (cm2), as well as pressure difference across the membrane (cmHg). Note that one cm3 (STP) is not a volume but the amount of gas in one cm3 at standard temperature and pressure (STP). Importantly, there is a traditional permeability–selectivity trade-off exemplified by Robeson's upper bound178,179 restricting gas separation performance (see Fig. 3). Because the permeability of a gas through a membrane is determined by its diffusivity as well as its solubility (see eqn (10)), membranes based on size exclusion and solution–diffusion can be utilized taking advantage of physical and chemical differences between the gases, respectively. This is schematically shown in Fig. 4. Moreover, because permeation is a thermally activated process following the Arrhenius equation, the temperature during the separation process needs to be controlled. Table 2 shows key properties of hybrid separation membranes.
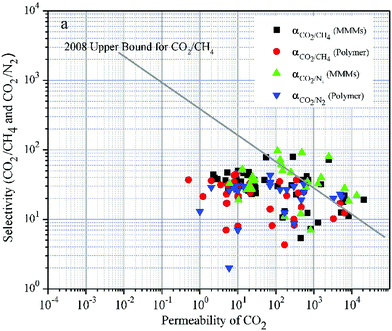 |
| Fig. 3 Selectivity versus permeability for the separation of CO2 from CH4 and N2. It appears that MMMs (black squares, green triangles pointing up) generally perform better than the respective neat polymeric matrices (red circles, blue triangles pointing down). The straight line corresponds to Robeson's upper bound, which shows the experimentally defined permeability-selectivity limit. Note that both permeability and selectivity are plotted on a logarithmic axis. Adapted with permission from ref. 180. Copyright 2017 Elsevier. | |
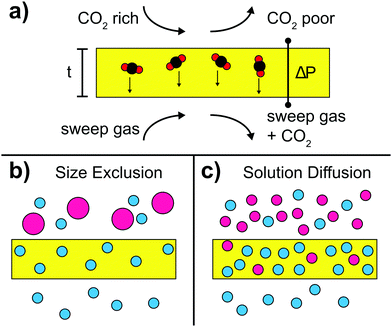 |
| Fig. 4 (a) Schematic drawing of the gas separation process. On the top, a CO2 rich gas reaches the membrane surface while on the bottom, the CO2 that permeated through the membrane is removed using a sweep gas. The permeation is enhanced for small membrane thickness t and high transmembrane pressure drop ΔP. (b) Separation via size exclusion. In contrast to gas molecules with a small kinetic diameter (blue circles), gas molecules with a large kinetic diameter (pink circles) do not permeate through the membrane. (c) Separation via solution–diffusion. The membrane preferentially absorbs a particular gas (blue circles). The resulting concentration gradient with respect to the permeate side leads to higher permeability. | |
Table 2 Key characteristics of different hybrid materials used for CO2 separation
Material |
Carrier gas/selectivity |
CO2 permeability [barrer] |
ΔP [bar] |
Ref. |
ZIF-8@UiO-66-NH2 in PSf |
N2/39 |
45.2 |
— |
181
|
PEG + organosilane@SiO2 |
N2/10 |
158 |
— |
182
|
MoS2 + PEBA + PDMS in PSf |
N2/93 |
64 |
— |
183
|
Poly(PEA-montmorillonite-TMC) in PSf |
N2/37 |
16 |
1 |
115
|
Poly(PEA-hydrotalcite-TMC) in PSf |
N2/40 |
15 |
1 |
[C2mim][B(CN)4] + SiO2 in PDMAAm |
N2/38 |
2600 |
6 |
184
|
[C2mim][Tf2N] + SiO2 in PIL |
N2/24 |
500 |
— |
185
|
[bmim][Tf2N] + ZIF-8 in PEBA |
N2/42 |
231 |
1 |
186
|
MIL-53 + aminosilane in poly(ether imide) |
N2/41 |
24 GPU |
— |
187
|
CuBDC + polyimide |
CH4/85 |
3–6 |
7.5 |
163
|
[bmim][Tf2N]@ZIF-8 in PSf |
N2/152 |
310 |
10 |
188
|
CH4/66 |
20 |
[emim][BF4] + GO in PEBA |
N2/44 |
981 GPU |
— |
189
|
CH4/11 |
GO in PEO–PBT |
N2/73 |
143 |
— |
190
|
CH4/21 |
H2/12 |
Size exclusion mechanism
The size exclusion mechanism is based on the size-dependence of permeability. In fact, when CO2 is separated using molecular sieves, a smart balance of pore size has to be found such that pores are small enough to efficiently separate the different gases and large enough to allow reasonable gas flow. Pore sizes of 5–10 Å were found to be suitable for separation of CO2 whose kinetic diameter is smaller than many light gases, CO2 (330 pm) < N2 (364 pm) < CO (376 pm) < CH4 (380 pm).191
In particular, MOFs play an important role in molecular sieving and they are often used as filler material in hybrid membranes owing to their precisely tunable pore size. It is also possible to incorporate ILs into MOFs to fine-tune the effective aperture size. For instance [bmim][Tf2N] was incorporated into ZIF-8 particles via in situ ionothermal synthesis.188 The [bmim]+ cation is used because of its size, which enables efficient cavity occupancy, whereas the [Tf2N]− anion promotes CO2 absorption. Because 1–2 IL molecules are confined in each sodalite cage of ZIF-8, they lead to a strong decrease of surface area of the MOF host. By incorporating as prepared IL-modified ZIF-8 particles into a PSf matrix, CO2 permeability as well as CO2/CH4 and CO2/N2 selectivities was remarkably increased. This example shows that it is possible to achieve a target-oriented alteration of MOF cut-off size through choosing ILs with proper chain length and by controlling the confinement ratios.
Alternatively, dual-channel pore engineering, i.e. the utilization of two different pore sizes in a single composite material, is an attractive method that combines precise separation with high permeability. For example, particles composed of a UiO-66-NH2 core with large pores surrounded by a ZIF-8 shell with small pores were fabricated and embedded in a PSf matrix as shown in Fig. 5a.181 The advantage of such core/shell particles is that the large pores of the MOF provide facilitated transport pathways whereas the smaller pores of the ZIF as well as incommensurately overlapping pores at the MOF/ZIF interface act as the sieving layer. Furthermore, it is possible to optimize permeability and selectivity by adjusting the shell thickness. Similar observations were made utilizing porous liquids as dual-channel molecular sieves where hollow silica spheres coated with a microporous shell were used for gas separation.182 As obtained core/shell particles can further be functionalized with organosilane moieties that react with hydroxy groups on the shell surface. Eventually, a poly(ethylene glycol)-tailed sulfone canopy is added. Such organic–inorganic particles, which remain liquid even under vacuum condition, can be embedded in a polymeric matrix to obtain solid membranes. In these materials, two effects are actively taking part in the separation process: the acid/base interaction of CO2 with ether groups in the canopy and the diffusion process through the cavities of the porous liquid that provide free volume.
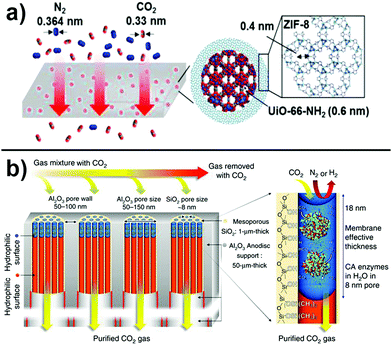 |
| Fig. 5 (a) Dual channel pore engineering. The gases are separated according to their kinetic size using ZIF/MOF core–shell structures embedded in a polymeric matrix. Adapted with permission from ref. 181. Copyright 2017 American Chemical Society. (b) Approach to decrease layer thickness, tortuosity, and thus increase permeability. Reprinted from ref. 192. Distributed under a Creative Commons Attribution 4.0 International License http://creativecommons.org/licenses/by/4.0/. | |
A useful strategy to increase membrane selectivity involves the decrease of permeability of larger gas molecules by homogeneously incorporating exfoliated nanosheets or layered materials into the gas separation layer. Moreover, the layered structure forces the CO2 molecules to diffuse along 2-dimensional voids, thus free diffusion pathways are efficiently eliminated. For instance, MoS2, montmorillonite, Mg–Al hydrotalcite, or CuBDC MOF were dispersed in various polymers such as PSf or PI.115,163,183 Note that a main disadvantage of this approach is the strong filler orientation-dependence of overall gas permeability.
Finally, many efforts are made targeting at the enhancement of CO2 permeability by tuning the geometry of membranes, especially by reducing the membrane thickness. This leads to less tortuosity for the diffusing species and diminution of gas diffusion resistance. For example, ultrathin asymmetric MMM layers have been created.36 Alternatively, it has been shown that capillary-based structures can be chemically modified in a way that only a small fraction of the capillary is filled by the gas separation medium, yielding μm thin membranes (see Fig. 5b).192
Solution–diffusion mechanism
Separation via solution–diffusion utilizes the high and selective CO2 solubility of the organic matrices in hybrids that is often related to the comparably high quadrupolar moment of CO2 with respect to other light gases such as N2 or CH4. For example, CO2/N2 selectivity can be adjusted by focusing on CO2-philicity or N2-phobicity of the membrane material. It is important to note that in membranes based on the solution–diffusion mechanism, the permeance is not necessarily following the kinetic diameter of gases.
Many ILs have inherent CO2/N2 permeability–selectivity and can be inserted into polymeric network membranes for separation applications.26,193 However, a common issue with such supported ionic liquid membranes (SILM) is the leakage of ILs and blow-out effect occurring at high transmembrane pressure drops. Consequently, membranes with chemically bound functional groups are considered more durable than their counterparts with mobile carriers. A possible approach is the fabrication of ion gel membranes combining RTIL and a 3D network of inorganic nanoparticles in a stabilizing polymeric matrix, thus forming so-called double network gels where inorganic nanoparticles lead to toughening of the membranes.194–197 For instance, [C2mim][Tf2N]185 and [C2mim][B(CN)4]184 ILs were combined with silica and incorporated into a polymeric matrix. It turns out that the ionic liquid promotes CO2 solubility and enhances the adhesion between the silica particles and the polymeric matrix. Concurrently, the fragile inorganic network can easily be ruptured, thus dissipating the energy loaded upon the ion gel, which in turn leads to increased toughness. Furthermore, as prepared membranes show good compression stress behavior and are able to withstand high pressure difference over several days, suggesting good IL holding property. The observed effects are in strong contrast with standard SILMs, thus underlining the utility of double network gels.
Common issues observed when filler particles are dispersed in an organic matrix include the aggregation and poor particle dispersion as well as voids between the filler and the matrix material. All of these effects are detrimental to efficient separation. Nevertheless, these issues can be tackled by chemically modifying the filler particles. For instance, MIL-53 particles were functionalized with aminosilanes and blended into poly(ether imide).187 MIL-53 increases the permeance while the aminosilanes act as interfacial bridges to the polymer and enhance adhesion. This prevents nonselective transport through defects in the skin layer at the MOF/matrix interface. On the other hand, the poly(ether imide) provides mechanical, thermal, and chemical stability together with high selectivity. Furthermore, CO2 accumulates at adsorption sites and thus leads to a crowding out effect by the formation of a large concentration gradient that accelerates the diffusion of CO2. Alternatively, MOF structures can be decorated with IL moieties and incorporated into polymeric matrices. In the case of [bmim][Tf2N]/ZIF-8/PEBA composites, the addition of ZIF-8 particles results in both a stiffening of the PEBA matrix as well as an increase of CO2 permeability by the formation of narrow voids.186 Furthermore, the addition of IL improves the compatibility of ZIF-8 with the polymeric matrix, thus lowering the crystallinity and yielding higher free volume that in turn is beneficial for gas diffusion. Moreover, by employing [BF4]− and [DCA]− anions, the importance of the anion choice is demonstrated. Indeed, the use of [Tf2N]− yields highest permeability, whereas [BF4]− yields best CO2/CH4 selectivity and [DCA]− yields best CO2/N2 selectivity. Interestingly, the composites show relative diffusivities CO2 > N2 > CH4 which is in accordance with their kinetic diameter. Also, GO can be utilized to tune the CO2 selectivity, solubility, and permeability. For example, [emim][BF4]/GO/PEBA thin film membranes are suitable for CO2/N2 and CO2/CH4 separation.189 Indeed, CO2 binds to oxygen-containing functional groups on the GO surface whereas diffusion of other gases is impeded by increased tortuosity as a result of the insertion of GO nanosheets. With increasing humidity and temperature, the overall permeance increases, but at a higher rate for N2 than CO2, thus decreasing the selectivity of the hybrid. Regarding long-term operability, it is noteworthy to mention that even though the permeance decreases over time, the selectivity is retained for at least half a year. Furthermore, it is believed that the use of a PDMS matrix is a possible solution to avoid increased transport resistance after ageing. Similarly, separation membranes made from GO embedded in PEO–PBT copolymer were tested.190 However, it appears that if the content of GO is too high, the nanosheets begin to stack, eventually hindering the efficient diffusion of CO2.
Common limitations of sorbents and separation membranes
Humidity has a strong influence on separation performance and many membranes need the CO2-carrying gas stream to be humidified in order to avoid drying-out. Furthermore, because high pressure drops across the membrane are required for efficient separation, mechanical strength of membranes is important. Due to the trade-off between mechanical strength and diffusivity, practical solutions such as the use of strong polymer networks and dual-network structures need to be utilized. In addition, the performance stability over time is a major issue in hybrid separation membranes. This is particularly important for membranes based on ILs. Furthermore, it will be necessary to develop new regeneration methods in order to optimize long-term stability. Also, the large majority of hybrid membranes are being used for CO2/N2 separation. However, gas purification processes usually contain gas mixtures and impurities as SO2, H2S, CO, H2. Therefore, it will be important to study selectivity of CO2 compared to diverse gas components and to focus on other separation processes such as CO2/CO, CO2/CH4 or CO2/H2 as well. Finally, an enormous potential lies within the utilization of computational methods to predict the CO2 separation characteristics of potential MMMs.198
5 Organic–inorganic hybrids for catalytic conversion of CO2
Nature has established a dynamic equilibrium between generation and recovery of carbon dioxide. Indeed, CO2 is emitted e.g. by decomposition of organic matter or by respiration199 while plants and algae capture gaseous or dissolved CO2 and photosynthetically convert it back to carbohydrates. However, there is a man-made imbalance between emission and recovery that should be reverted by considering CO2 as a renewable carbon feedstock. In fact, by combining CO2 capture and storage technology with CO2 conversion on industrial scale, an artificial, carbon-neutral life cycle can be mimicked. Chemical fuels obtained from the conversion of CO2 are attractive in terms of energy storage owing to their 10 to 100-fold higher energy density as compared to batteries.200,201
After the first attempts to catalytically reduce CO2 in 1870,202 it took nearly one century until research in this field intensified between the 1970s and 1980s where photo- and electrocatalytic reduction of CO2 to methane,203,204 ethylene,203 formic acid,204,205 methanol,204,205 and formaldehyde204,205 were demonstrated. Among various photocatalytically active semiconductor materials, TiO2 has been investigated most extensively owing to its high availability, low cost, and high chemical stability.206 However, photocatalytic efficiency for CO2 conversion is often limited by high electron–hole recombination rates and low visible light absorption that is related to the wide-bandgap of TiO2. Consequently, several strategies were developed to enhance visible light absorption including doping, deposition of metal co-catalysts that act as electron sinks and suppress electron–hole recombination, or formation of heterostructures where two semiconductor materials are coupled to promote charge carrier separation.120,207–213 Furthermore, the role of surface reactions,208,214 morphology and crystal phase of semiconductors,209 inorganic–inorganic hybrid materials,215 metal oxides,120,210 or size dependency of metal catalysts212 were studied. Alternatively, organic–inorganic hybrid materials can be utilized because the organic part allows introduction of functional groups like amines or ionic liquids, which act as mediators for the electro- and photochemical reduction of CO2. Recently, imidazolium-based ionic liquids were used as additives in electrolytes to enhance electrocatalytic reduction of CO2 due to increased solubility of CO2 and significantly lower energy barrier for electron-transfer processes.212,216,217 Additionally, amine functionalization of titania nanoparticles,218 zinc oxide,219 or titanate nanosheets220 substantially improved CO2 adsorption—thus enhancing photocatalytic activity.218
In this section, we briefly present the theoretical background of CO2 conversion by electro- and photocatalytic processes and discuss different hybrids based on MOFs, g-C3N4, carbon materials and organically doped metals. We then present and discuss future perspectives of organic–inorganic hybrid materials for CO2 reduction.
Thermodynamics and kinetics of CO2 conversion
CO2 is a product of the combustion (oxidation) process of organic matter and its carbon atom displays an oxidation state of +IV. Because the bonding energy of a C
O double bond is 750 kJ mol−1, which is considerably larger than C–C (336 kJ mol−1), C–O (327 kJ mol−1) or C–H bonds (411 kJ mol−1), the photo- or electrocatalytic reduction of CO2 (see Fig. 6) is challenging from a thermodynamic point of view. Typical products of the CO2 reduction process display oxidation states of carbon ranging from +II (CO) to −IV (CH4) and can be formed by different pathways involving the transfer of multiple electrons. One-electron reduction is complicated by big structural differences between the linear CO2 and the bent CO2− molecule (eqn (12) in Table 3).221 On the contrary, proton-assisted multi-electron transfer processes exhibit significantly lower redox potentials and yield manifold reaction products like CO, HCOOH (eqn (13)), MeOH (eqn (17)), or CH4 (eqn (18)). The redox potential limits the products that are obtained since reactions with more positive redox potential are more likely to happen. On the other hand, the number of electrons dictates the reduction kinetics. For example, the reduction of CO2 to CH4 is thermodynamically favoured but kinetically suppressed because eight electrons need to be transferred simultaneously. Consequently, it is hard to achieve high selectivity in electro- or photocatalytic reduction of CO2.222
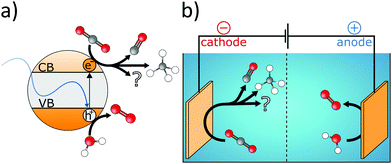 |
| Fig. 6 Schematic representation of (a) the photo- and (b) the electrocatalytic reduction of CO2. During photocatalytic reduction, a photon is absorbed by a semiconductor under the formation of an electron–hole pair. While the electron in the conduction band (CB) can be utilized to reduce CO2, the hole in the valence band (VB) commonly drives the oxidation of water. Similar reactions take place during the electrocatalytic reduction of CO2. However, in this case, electrons and holes are provided by an external power supply and the cathodic reduction and anodic oxidation processes are spatially separated by a membrane. | |
Table 3 Redox potentials of CO2 reduction and side reactions ordered by their redox potential. Redox potentials are tabulated versus normal hydrogen electrode (NHE) under standard conditions in aqueous solution (pH 7, 25 °C, 1 atm)225
Reaction |
Redox potential vs. NHE, pH 7.0 |
Eqn |
CO2 + e− → CO2− |
E = −1.90 V |
(12) |
CO2 + 2e− + 2H+ → HCOOH |
E = −0.61 V |
(13) |
CO2 + 2e− + 2H+ → CO + H2O |
E = −0.53 V |
(14) |
CO2 + 4e− + 4H+ → HCHO + H2O |
E = −0.48 V |
(15) |
2H+ + 2e− → H2 |
E = −0.41 V |
(16) |
CO2 + 6e− + 6H+ → CH3OH + H2O |
E = −0.38 V |
(17) |
CO2 + 8e− + 8H+ → CH4 + 2H20 |
E = −0.24 V |
(18) |
2H2O + 4h+ → O2 + 4H+ |
E = +0.82 V |
(19) |
Because CO2 is inert under UV-vis and at RT, semiconductor catalysts are required for its photocatalytic reduction. The bandgap of the catalyst affects the portion of the absorbed solar spectrum, and the respective positions of the valence band (VB) and conduction band (CB) determine the photocatalytic performance. Consequently, band structure engineering enables the enhancement of photon efficiency. For instance, if the CB is at more negative potential than the redox potential of the corresponding CO2 reduction reaction, the transfer of photogenerated electrons to surface-adsorbed CO2 molecules is facilitated. On the other hand, if the VB is at more positive potential than the H2O oxidation reaction (eqn (19)), the consumption of photogenerated holes is enabled. Although H2O seems well suited for the neutralization of photogenerated holes (eqn (19)) and for providing protons for the reduction reactions, protons also compete with electrons in the CB for the generation of hydrogen (eqn (16)).223 This dual behaviour of H2O is one of the major challenges in electrocatalytic reduction of CO2. While the extent of the photocatalytic reduction is determined by the position of the CB, it additionally depends on the applied bias in the case of the electrocatalytic reduction. Consequently, the selectivity of the reduction reaction can be influenced by the applied potential224 and in order to increase electrocatalytic conversion efficiency the inherent charge carrier mobility has to be optimized. Both approaches require facile transfer of multiple electrons from the catalyst to the CO2 molecule. Furthermore, the surface of the catalyst should facilitate adsorption of reactants, while efficient desorption of reaction products avoids catalyst poisoning. Moreover, the nature of the products and their purity are determined by the chemical properties of the catalyst material like CO2 binding affinity and redox behaviour. However, combining selectivity, activity, and efficiency into a single catalyst material is very challenging.
Nevertheless, organic–inorganic hybrids were shown to enhance the electro- and photocatalytic activity by optimizing light harvesting, charge separation as well as mass transport and CO2 affinity.
Figures of merit
The photo- or electrocatalytic reduction of CO2 is a complicated, multi-step process in which different aspects as light absorption, charge carrier separation and movement to surface reaction sites or adsorption and desorption of molecules determine the overall efficiency. Besides these multi-step processes, also sample geometry and experimental parameters such as temperature, illumination, gas flow and composition play a decisive role.214,226–228 However, currently there is no single quantity which considers this complexity and allows distinct comparison of several catalyst materials. Instead, there are measures which relate the performance to either amount of catalyst, illumination power or amount of catalytic active sites. For electrocatalytic reduction, turnover frequency (TOF) and faradaic efficiency are commonly used as performance metrics to evaluate CO2 reduction electrocatalysts. TOF is defined as rate of electrochemical conversions per number of catalytic active sites and illustrates it's activity. In order to reflect selectivity of electrocatalysts, the faradaic efficiency of a certain product is defined as the ratio of transferred charges to obtain the product relative to the number of total charges. The faradaic efficiency is often restricted by H2 formation. Therefore, faradaic efficiencies >80% are considered for good CO2 reduction electrocatalysts.229
For photocatalytic reduction, the most often used quantity is the rate-per-weight of a product that is usually given in mol of product per hour of illumination and per gram of catalysts (mol h−1g−1). However, the rate-per-weight data is specific for the material and experimental setup and does not reflect the intrinsic catalytic activity. Moreover, TOF values can be calculated based on the specific area of the photocatalyts obtained by Brunauer–Emmett–Teller (BET) measurements. Nevertheless, due to internal shading not the complete surface area is uniformly illuminated, which means that the surface area may not accurately describe the number of available catalytic active sites. Additionally, even if the number of catalytic sites can be accurately described, it is unclear how many are actually active throughout the entire experiment. At present there is no straight-forward quantity which can be used to compare the catalytic performance of different materials obtained by different experimental setups. To avoid misleading interpretations of experimental data and to allow better comparison within the CO2 reduction community, rigorous testing protocols including advanced scientific techniques like 13CO2 isotope labelling are mandatory when studying CO2 reduction.230 Since the discussion of merits is beyond the scope of this review we would like to refer to publications which exclusively deal with objective evaluation of catalyst's performance.231–237
Metal–organic frameworks and their hybrids
By choosing adequate building blocks of MOFs, it is possible to incorporate photosensitizers or catalytic centres directly in the MOF structure. For example, it was demonstrated that CO2 is reduced to formate anions under visible light irradiation using MIL-125.113 The amine functional group within the structure led to improved CO2 adsorption capability and extended the upper bound of visible light absorption from 350 to 550 nm. More recently, it was demonstrated that photocatalytic properties of MOFs can be tuned by small variations of the backbone. Indeed, a series of MIL-125 materials whose organic linker feature N-alkyl substituents with increasing chain length and varying connectivity (primary or secondary amines) were prepared.238 In fact, inductive electron donating ability depends on chain length and reduces the optical band gap from 2.56 to 2.29 eV, whereas the utilization of secondary amines enhances the lifetime of excited states. In particular, MIL-125-NH-cyclopentyl shows optimum activity due to its long-living excited state and narrow band gap. These observations demonstrate that small variations in the MOF backbone strongly influence photocatalytic properties for CO2 reduction.
Also MOF-based hybrids are fabricated to boost selectivity, activity, and efficiency of CO2 reduction catalysts.239 For example, Re3-UiO-67 was deposited onto Ag nanocubes (see Fig. 7a) to confine photoactive Re centres to the plasmon-intensified electric field at the surface of Ag nanocubes, resulting in a 7-fold enhancement of CO2 to CO conversion under visible light as compared to bare Re3-UiO-67.240 To enable plasmon-enhanced reduction, the localized surface plasmon resonance (LSPR) peak of the metallic nanoparticle has to overlap with the absorption range of the linker molecule of the MOF. Due to the material- and size-dependency of the LSPR, plasmon-enhanced photocatalytic activity should be transferable to different MOF materials that show enhanced selectivity to higher-valued hydrocarbons.
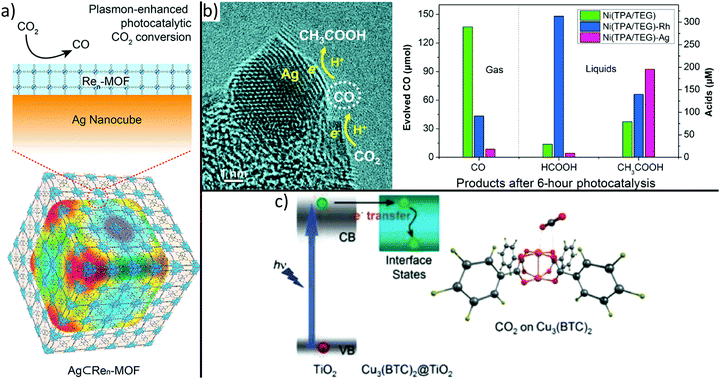 |
| Fig. 7 MOF-based hybrids synthesized to boost activity, selectivity and efficiency of CO2 photoreduction. Integration of metal nanoparticles, (a) incorporates a plasmon-intensified electric field leading to higher photonic efficiency within the MOF building block or (b) controls selectivity by loading different type of metals.240,243 Moreover, (c) superior CO2 adsorption on Cu sites of HKUST-1 causes dramatically improved performance and selectivity compared to bare TiO2.242 (a) Adapted with permission from ref. 240. Copyright 2017 American Chemical Society. (b) Adapted from ref. 243 ©The Authors, some rights reserved; exclusive licensee American Association for the Advancement of Science. Distributed under a Creative Commons Attribution NonCommercial License 4.0 (CC BY-NC) http://creativecommons.org/licenses/by-nc/4.0/. (c) Reprinted with permission from ref. 242. Copyright 2014 John Wiley and Sons. | |
Another attractive alternative used to improve light absorption is the combination of MOFs with semiconductor materials. For example, ZIF-8 nanoparticles were grown onto semiconducting Zn2GeO4 nanorods or HKUST-1 was coated with a porous TiO2 shell, thus resulting in a strong enhancement of photocatalytic CO2 to CH3OH or CO2 to CH4 conversion as compared to bare Zn2GeO4 and TiO2, respectively.241,242 These observations are explained by the successful combination of the CO2 adsorption property of ZIF-8 and HKUST-1 and the light harvesting ability of Zn2GeO4 and TiO2 (Fig. 7c), respectively.
Noble metal nanoparticles can also be used to tune the CO2 conversion selectivity of catalysts (see Fig. 7b).243 First, by replacing rigid linker molecules of a Ni-based MOF with softer molecules, a spongy, disordered, and defective metal–organic material with high selectivity in photocatalytic CO2 to CO conversion can be obtained. Second, the selectivity can be tuned by decorating the spongy material with Ag or Rh nanoparticles resulting in the formation of formic acid or acetic acid as a major product, respectively. These observations were attributed to further reduction of the intermediate CO molecules, as depicted in Fig. 7b.
Carbon materials
Due to their intrinsic advantages such as abundant natural resources, low cost and variety of dimensions, carbon-based materials like fullerenes (0D), carbon nanotubes (1D) and graphene (2D) have attracted great attention as alternatives to costly noble-metal-based electrocatalysts in recent years.244–246 Although carbon catalysts show excellent activity for electrocatalytic conversion of CO2, effect of heteroatom doping and structural defects as an origin for its activity are currently under debate and not fully understood.246 Moreover, quality of commercial graphene flakes is rather poor and irregular as they contain different type of impurities, thus hindering comparison between different studies and real-world applications of final products.247 Nevertheless, the π–π interaction between graphene and CO2 can cause destabilization and activation of CO2 for facilitated reduction reactions.248 Moreover, through its 2-dimensional structure with high specific surface area and conductivity, graphene has been widely used to promote electron–hole separation during photocatalytic reduction of CO2.213,249–253 For example, graphene–TiO2 hybrid thin films yield strong enhancement in the photo-reduction.251
Besides graphene, GO was demonstrated to significantly enhance CO2 photoreduction. Recently, it was shown that the band gap of GO can be tuned by the carbon to oxygen ratio, an effect that can be exploited to optimize CO2 to MeOH conversion.254 Although pristine GO shows six-fold enhancement of CO2 to MeOH conversion efficiency as compared to commercial TiO2 nanoparticles, even higher conversion rates are required for practical applications. To this end, GO was decorated with Cu nanoparticles, thus yielding high and tuneable methanol and acetaldehyde production rates.255 This tendency was attributed to enhanced electron–hole separation and to a change in the GO CB position with increasing Cu loading as shown in Fig. 8a.
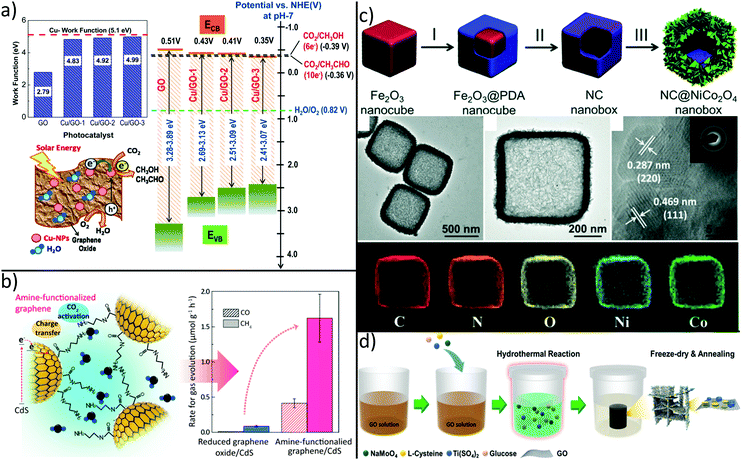 |
| Fig. 8 Carbon-based hybrids synthesized for improved CO2 conversion by tuning (a) selectivity, (b) affinity and (c + d) by creating hierarchical architectures. (a) Decoration of GO with increasing amounts of Cu nanoparticles leads to a decrease of CB position and eventually to different products.255 (b) Grafting of ethylenediamine leads to higher CO2 adsorption capacity and photocatalytic activity.256 Synthesis of hierarchical (c) graphene/MoS2/TiO2262 and (d) N-doped carbon/NiCo2O4 double-shell nanoboxes263 enable improved CO2 reduction capabilities by enhanced mass transport and light harvesting. (a) Adapted with permission from ref. 255. Copyright 2014 American Chemical Society. (b) Reprinted with permission from ref. 256. Copyright 2017 American Chemical Society. (c) Adapted with permission from ref. 262. Copyright 2018 American Chemical Society. (d) Adapted from ref. 263 with permission from The Royal Society of Chemistry. | |
However, graphene hybrids are not only prominent for high electron mobility which relieves charge transfer of photogenerated electrons, but also for preventation of catalyst degradation. In detail, attack of active species like OH radicals on photoactive CdS NPs can be avoided by wrapping GO around positively charged CdS NPs as shown in Fig. 8b.256 By subsequent grafting of ethylenediamine via coupling reaction between one amine group and the carboxylic acid group of GO, CO2 adsorption capacity was several times higher than in rGO/CdS, thus resulting in an enhanced photocatalytic activity. There is a strong need for alternatives to typical II–VI semiconductors like CdS since their toxicity often prevents practical use. In fact, perovskite materials have attracted great interest in the field of optoelectronic devices such as LEDs or photovoltaic cells owing to high extinction coefficients, wide absorption ranges, and long charge carrier diffusion lengths.257,258 These outstanding optical properties ensue perovskites as interesting candidates for efficient photocatalytic CO2 reduction. Recently, GO was decorated with CsPbBr3 quantum dots (QD).259 Compared to individual CsPbBr3 QDs, photocatalytic activity of CsPbBr3/GO composites was increased mainly because of fast separation and suppressed recombination of photogenerated electron–hole pairs. Nevertheless, insufficient stability and toxicity of CsPbBr3 also limits their practical application. Therefore, the development of lead-free halide perovskites where Pb is replaced by less toxic Sn, Sb or Bi is of significant academic and industrial interest.260
As an alternative to noble metals or toxic semiconductor materials, transition metal disulfides such as tungsten disulfide (WS2) or molybdenum disulfide (MoS2) are increasingly attracting attention due to low price and outstanding electrocatalytic performance. The latter is commonly attributed to the metallic character and high d-electron density at Mo-terminated edges.261 For example, graphene/MoS2/TiO2 hybrids with hierarchical porosity were prepared by self-assembly in a simple one-pot hydrothermal synthesis and applied for photocatalytic reduction of CO2.262 The 3-dimensional, graphene-based aerogel decreases the electron–hole recombination by creating an electron-conduction channel from TiO2 to MoS2, while the macroporous structure offers efficient mass transport, large surface area, mechanical stability, and enhanced light harvesting via multiple reflections. This noble metal-free approach offers a high CO selectivity and higher production yield than bare TiO2 by integrating structural and functional merits of TiO2, MoS2 and graphene into a 3-dimensional material, as shown in Fig. 8d. In another inspiring approach, the catalytic performance of a hierarchical N-doped carbon/NiCo2O4 double-shell nanobox benefits from an unique composition and structure.263 For this, sacrificial Fe2O3 nanocubes were coated with a thin layer of polydopamine, which was subsequently pyrolyzed under N2 atmosphere to create an N-doped carbon shell. In a further step, the oxide core was selectively etched to generate hollow nanoboxes while in a last step, NiCo2O4 nanosheets were grown on the nanoboxes via a hydrothermal reaction (Fig. 8c). Regarding photoreduction of CO2, such hollow nanoboxes combine structural and functional features like catalytic activity of NiCo2O4, high electrical conductivity of the carbon-based inner layer, large active surface area, and enhanced light absorption by multiple light scattering events within the hollow structure.
Graphitic carbon nitride
g-C3N4 shows increased absorbance in the visible-light range as compared to commonly used catalysts such as TiO2. However, the photocatalytic efficiency is limited by fast electron–hole pair recombination.83,264 Therefore, many attempts were made to overcome this challenge by combining g-C3N4 with inorganic materials.215 For example, charge carrier separation is facilitated by utilizing noble metals or other semiconductors.215 Moreover, the deposition of Pt nanoparticles on g-C3N4 not only boosts CO2 reduction activity, but also affects the selectivity for CO2 conversion to methane, methanol, and formaldehyde.265 In detail, Pt acts as a sink for photogenerated electrons and therefore prevents the electron–hole recombination. By investigating the role of single Pd or Pt atoms deposited on g-C3N4 using DFT calculations, it turns out that during the reduction reaction, the noble metal atoms act as active sites while the g-C3N4 provides hydrogen from hydrogen evolution reaction.266 Furthermore, it appears that HCOOH and CH4 are preferentially produced by Pd/g-C3N4 and Pt/g-C3N4, respectively. In another study, Ag-decorated g-C3N4 shows direct generation of syngas during electrocatalytic reduction of CO2 in water.267 Furthermore, the H2/CO ratio can be adjusted by varying the applied potential and the Ag particle loading. Moreover, no significant loss of selectivity or activity was observed, thus demonstrating high stability of g-C3N4 in electrocatalytic conditions. Note that the produced syngas can directly be reacted to industrially relevant chemical fuels via Fischer–Tropsch process or MeOH synthesis.
Alternatively, g-C3N4 can be decorated with semiconducting materials, which facilitate electron–hole pair separation. For instance, Ag3PO4/g-C3N4 hybrids efficiently separate electron–hole pairs based on a z-scheme mechanism, where holes from the VB of g-C3N4 combine with electrons from the CB of Ag3PO4, resulting in the generation of electrons and holes in the CB of g-C3N4 and VB of Ag3PO4, respectively. Consequently, the recombination of photocatalytically active electrons is strongly suppressed.268 The same mechanism is observed in hierarchical structures that combine 3D urchin-like α-Fe2O3 and g-C3N4.269 Such hybrids display facilitated electron–hole pair separation, increased binding energy, and increased CO2 adsorption capacity, thus demonstrating the utility of z-scheme g-C3N4 based photocatalysts. Moreover, many other materials were combined with g-C3N4 including CdS,270 ZnO,271 CeO2,272 TiO2,273 WO3,274 In2O3,275 BiOI,276 LaPO4,277 CdMoO4,278 and Bi2WO6.279
Because the electron transfer from catalytic active site to CO2 requires an intimate contact, adsorption of CO2 on the catalyst surface is essential for efficient CO2 reduction. For this, g-C3N4 was combined with ZIF-9280 and UiO-66281 cocatalysts that enhance the adsorption of CO2 and separation of photogenerated charge carriers. Furthermore, besides exploiting the high specific surface area of MOFs, CO2 adsorption of g-C3N4 can be facilitated by chemical functionalisation. Recently, amino-rich g-C3N4 containing carbon defects and intercalated [K]+ ions was synthesized.282 Amino-groups on the surface of g-C3N4 strongly enhance CO2 adsorption and conversion, and activate the CO2 molecule for subsequent reduction processes,283 while [K]+ and carbon defects enhance the separation and transfer of photogenerated charge carriers.
Organically doped metals
The research field of organically doped metals is relatively new and their application for CO2 electroreduction is still in its infancy. For example, pyridine-functionalized Pd electrodes have been used to electrochemically reduce CO2.284 Others entrap pyridine derivatives within Pd285 or Cu–Pd alloy cages286 in order to combine the catalytic activity for CO2 to MeOH conversion of pyridine with the large surface area of the porous and electrically conductive metal cage. This approach prevents the use of large amounts of pyridine that are commonly needed for the reduction of CO2 to MeOH.285 Besides showing constant current density for several hours, such hybrids can easily be recycled after washing with water. It appears that Pd and Cu catalysts facilitate the generation of MeOH and EtOH, respectively. Consequently, the Cu–Pd alloy combines two catalytically active sites within one material, thus allowing electrochemical reduction of CO2 to MeOH or EtOH depending on the applied potential. To conclude, the entrapment of pyridine derivatives within metallic nanoparticles allows effective electrochemical reduction of CO2 with high stability, reusability, and tuneable selectivity.
Common limitations of catalysts
Established concepts used to enhance CO2 reduction performance of purely inorganic materials such as semiconductor/semiconductor or semiconductor/metal combinations are also applicable to different classes of organic–inorganic hybrids. To date, research mainly focussed on improving the electro- and photocatalytic activity by optical or electronic efficiency optimisation including bandgap engineering, plasmonic enhancement, or facilitated charge carrier mobility. However, the process of catalytic conversion starts with adsorption of CO2 on the catalysts surface and is completed with desorption of the product molecule. These intermediate steps of the overall conversion process can be tackled by the appropriate choice of an organic component of organic–inorganic hybrids allowing chemical functionalization that results in significantly increased CO2 affinity, suppressed competitive hydrogen evolution reactions, and enhanced selectivity towards higher-valued hydrocarbons. Nevertheless, the organic components can act as catalysts on their own, block reagents from reaching catalytic centres, or suppress charge transfer. Moreover, the origin of catalytic reaction products need further attention, e.g. through investigations comprising isotope labelling of 13CO2 to avoid misleading interpretations of the detected products.223,230,287 Consequently, the organic component harbours many opportunities but also extra complexity for catalytic applications.
Because CO2 reduction is closely associated with water oxidation, which is the second half reaction of the redox process, further investigations in this field need to be promoted. In detail, insufficient oxidation diminishes hydrogen formation and causes hole accumulation, both of which negatively affect the product yield. Finally, an enormous potential still lies within biological/inorganic hybrids that have recently demonstrated CO2 reduction efficiencies exceeding natural photosynthetic systems.288–290
Ultimately, to enable real-world application of organic–inorganic hybrid materials for CO2 conversion, catalysts have to exhibit a long term stability over thousands of hours, cyclability, non-toxicity, and economic feasibility besides showing good catalytic activity. Consequently, better understanding of the catalyst degradation mechanism is crucial to improve future catalysts. Recently, the general degradation mechanism of metallic CO2 electrocatalysts was revealed and correlated to the catalytic performance by monitoring structural changes of different sized copper nanocubes and performing density functional theory calculations.291
Furthermore, to promote cost-effective real-world applications, CO2 conversion to higher valued hydrocarbons can be broken into a CO2 to CO and a separate CO to hydrocarbon reduction step as this circumvents the kinetically suppressed transfer of multiple electrons and allows usage of different catalysts which are highly selective for a single redox reaction and tailor-made for its specific environmental conditions.
6 Conclusion and outlook
This review discusses the most common components of state-of-the-art organic–inorganic hybrid materials designed for sensing, separation, and catalytic conversion of CO2. In fact, organic–inorganic hybrids are generating a strong excitement in the field of materials science and new building blocks are constantly synthesized at high-rate, thus yielding an ever-growing toolbox of materials. Nevertheless, many challenges have yet to be overcome to enable the breakthrough of hybrid materials for the production at industrial scale and utilization in real-world applications. For instance, the loss of functionality during long-time exposure to detrimental operating conditions and subsequent durability and stability issues need to be addressed. Additionally, conventional synthesis routes need to be optimized and extended in order to yield superior control over composition, morphology, and intermixing of organic and inorganic phases down to the nanometer scale.292
In the future, computational methods that are currently still in their infancy, will become a promising tool to simulate and predict material properties and electrical or chemical interaction of hybrids with CO2.198,224,293 Moreover, in operando characterization of working devices will yield more detailed knowledge of working mechanisms.129 Furthermore, researchers will have to take environmental compatibility, renewability, and sustainability of new organic–inorganic hybrids into account. It is quite certain that such efforts will in turn lead to their industrial viability and accelerate their utilization in CO2-related applications, ultimately enabling to close the artificial carbon cycle. For instance, owing to steadily advancing fabrication techniques, one can imagine fabrication of multifunctional devices that combine multiple hybrid materials into a single unit. For example, CO2 can be separated from flue gas and reduced to CO and higher valued hydrocarbons using multiple catalyst materials which are highly selective for a specific reduction process. Those catalysts can easily be combined with CO2/CO or CO/CH4 separation materials to enable recirculation of unreacted educts and storage of products with very high purity. It is self-evident that all these processes have to be controlled with accurate sensors. Consequently, this topic holds many challenges and opportunities and constitutes a versatile playground for chemists, physicists, and material scientists.
Conflicts of interest
There are no conflicts to declare.
Acknowledgements
This work is supported by the Cluster of Excellence ‘CUI: Advanced Imaging of Matter’ of the Deutsche Forschungsgemeinschaft (DFG) – EXC 2056 – project ID 390715994.
Notes and references
- T. DeVries, M. Holzer and F. Primeau, Nature, 2017, 542, 215 CrossRef CAS PubMed.
- P. Singh, A. Wani Ali, A. A. Karim and H. C. Langowski, Int. J. Dairy Technol., 2011, 65, 161–177 CrossRef.
- X. Zhang, S. Heinonen and E. Levanen, RSC Adv., 2014, 4, 61137–61152 RSC.
- L. F. Cabeza, A. de Gracia, A. I. Fernández and M. M. Farid, Appl. Therm. Eng., 2017, 125, 799–810 CrossRef CAS.
- A. Kaushik, R. Kumar, S. K. Arya, M. Nair, B. D. Malhotra and S. Bhansali, Chem. Rev., 2015, 115, 4571–4606 CrossRef CAS PubMed.
- M. Wright and A. Uddin, Sol. Energy Mater. Sol. Cells, 2012, 107, 87–111 CrossRef CAS.
- F. Peng, L. Lu, H. Sun, Y. Wang, J. Liu and Z. Jiang, Chem. Mater., 2005, 17, 6790–6796 CrossRef CAS.
-
N. Hüsing and S. Hartmann, in Hybrid Nanocomposites for Nanotechnology: Electronic, Optical, Magnetic and Biomedical Applications, ed. L. Merhari, Springer US, Boston, MA, 2009, pp. 131–171 DOI:10.1007/978-0-387-30428-1_3.
- M. Aparicio and A. Durán, J. Sol-Gel Sci. Technol., 2004, 31, 103–107 CrossRef CAS.
- J. Tao, N. Liu, W. Ma, L. Ding, L. Li, J. Su and Y. Gao, Sci. Rep., 2013, 3, 2286 CrossRef PubMed.
- C. Sanchez, P. Belleville, M. Popall and L. Nicole, Chem. Soc. Rev., 2011, 40, 696–753 RSC.
- L. Nicole, C. Laberty-Robert, L. Rozes and C. Sanchez, Nanoscale, 2014, 6, 6267–6292 RSC.
- M. Faustini, L. Nicole, E. Ruiz-Hitzky and C. Sanchez, Adv. Funct. Mater., 2018, 1704158 CrossRef.
- K. D. Vogiatzis, A. Mavrandonakis, W. Klopper and G. E. Froudakis, ChemPhysChem, 2009, 10, 374–383 CrossRef CAS PubMed.
- P. D. Vaidya and E. Y. Kenig, Chem. Eng. Technol., 2007, 30, 1467–1474 CrossRef CAS.
- R. N. Dansby-Sparks, J. Jin, S. J. Mechery, U. Sampathkumaran, T. W. Owen, B. D. Yu, K. Goswami, K. Hong, J. Grant and Z.-L. Xue, Anal. Chem., 2010, 82, 593–600 CrossRef CAS PubMed.
- H. Segawa, E. Ohnishi, Y. Arai and K. Yoshida, Sens. Actuators, B, 2003, 94, 276–281 CrossRef CAS.
- J. J. Gassensmith, J. Y. Kim, J. M. Holcroft, O. K. Farha, J. F. Stoddart, J. T. Hupp and N. C. Jeong, J. Am. Chem. Soc., 2014, 136, 8277–8282 CrossRef CAS PubMed.
- F. L. Bernard, F. Dalla Vecchia, M. F. Rojas, R. Ligabue, M. O. Vieira, E. M. Costa, V. V. Chaban and S. Einloft, J. Chem. Eng. Data, 2016, 61, 1803–1810 CrossRef CAS.
- I. R. Soosaiprakasam and A. Veawab, Int. J. Greenhouse Gas Control, 2008, 2, 553–562 CrossRef CAS.
- J. L. Anthony, J. L. Anderson, E. J. Maginn and J. F. Brennecke, J. Phys. Chem. B, 2005, 109, 6366–6374 CrossRef CAS PubMed.
- Y. Hou and R. E. Baltus, Ind. Eng. Chem. Res., 2007, 46, 8166–8175 CrossRef CAS.
- N. M. Yunus, M. I. A. Mutalib, Z. Man, M. A. Bustam and T. Murugesan, Chem. Eng. J., 2012, 189–190, 94–100 CrossRef CAS.
- L. A. Blanchard, D. Hancu, E. J. Beckman and J. F. Brennecke, Nature, 1999, 399, 28 CrossRef.
- Z. Lei, C. Dai and B. Chen, Chem. Rev., 2014, 114, 1289–1326 CrossRef CAS PubMed.
- Z. Dai, R. D. Noble, D. L. Gin, X. Zhang and L. Deng, J. Membr. Sci., 2016, 497, 1–20 CrossRef CAS.
- J. Tang, H. Tang, W. Sun, M. Radosz and Y. Shen, J. Polym. Sci., Part A: Polym. Chem., 2005, 43, 5477–5489 CrossRef CAS.
- P. G. Mineo, L. Livoti, M. Giannetto, A. Gulino, S. Lo Schiavo and P. Cardiano, J. Mater. Chem., 2009, 19, 8861–8870 RSC.
- J. Yuan and M. Antonietti, Polymer, 2011, 52, 1469–1482 CrossRef CAS.
- J. Yuan, D. Mecerreyes and M. Antonietti, Prog. Polym. Sci., 2013, 38, 1009–1036 CrossRef CAS.
- W. Fang, Z. Luo and J. Jiang, Phys. Chem. Chem. Phys., 2013, 15, 651–658 RSC.
- S. G. Kazarian, B. J. Briscoe and T. Welton, Chem. Commun., 2000, 2047–2048, 10.1039/B005514J.
- G. Qi, L. Fu and E. P. Giannelis, Nat. Commun., 2014, 5, 5796 CrossRef CAS PubMed.
- T. Endo, D. Nagai, T. Monma, H. Yamaguchi and B. Ochiai, Macromolecules, 2004, 37, 2007–2009 CrossRef CAS.
- H. J. Lee, K. K. Park, M. Kupnik and B. T. Khuri-Yakub, Sens. Actuators, B, 2012, 174, 87–93 CrossRef CAS.
- H. Zhu, L. Wang, X. Jie, D. Liu and Y. Cao, ACS Appl. Mater. Interfaces, 2016, 8, 22696–22704 CrossRef CAS PubMed.
- X. Wang, V. Schwartz, J. C. Clark, X. Ma, S. H. Overbury, X. Xu and C. Song, J. Phys. Chem. C, 2009, 113, 7260–7268 CrossRef CAS.
- S. Supasitmongkol and P. Styring, Energy Environ. Sci., 2010, 3, 1961–1972 RSC.
- E. I. Privalova, E. Karjalainen, M. Nurmi, P. Mäki-Arvela, K. Eränen, H. Tenhu, Y. Murzin Dmitry and J. P. Mikkola, ChemSusChem, 2013, 6, 1500–1509 CrossRef CAS PubMed.
- S. Zulfiqar, M. I. Sarwar and D. Mecerreyes, Polym. Chem., 2015, 6, 6435–6451 RSC.
- R. Ali, S. M. Saleh, R. J. Meier, H. A. Azab, I. I. Abdelgawad and O. S. Wolfbeis, Sens. Actuators, B, 2010, 150, 126–131 CrossRef CAS.
- A. Car, C. Stropnik, W. Yave and K. V. Peinemann, Adv. Funct. Mater., 2008, 18, 2815–2823 CrossRef CAS.
- J. Lilleparg, P. Georgopanos, T. Emmler and S. Shishatskiy, RSC Adv., 2016, 6, 11763–11772 RSC.
- D. Wu, F. Xu, B. Sun, R. Fu, H. He and K. Matyjaszewski, Chem. Rev., 2012, 112, 3959–4015 CrossRef CAS PubMed.
- P. Bhanja, A. Modak and A. Bhaumik, ChemCatChem, 2019, 11, 244–257 CrossRef CAS.
- P. Kaur, J. T. Hupp and S. T. Nguyen, ACS Catal., 2011, 1, 819–835 CrossRef CAS.
- M. Sevilla, P. Valle-Vigón and A. B. Fuertes, Adv. Funct. Mater., 2011, 21, 2781–2787 CrossRef CAS.
- Y. Wu, Y. Li, L. Qin, F. Yang and D. Wu, J. Mater. Chem. B, 2013, 1, 204–212 RSC.
- M. Zhong, E. K. Kim, J. P. McGann, S.-E. Chun, J. F. Whitacre, M. Jaroniec, K. Matyjaszewski and T. Kowalewski, J. Am. Chem. Soc., 2012, 134, 14846–14857 CrossRef CAS PubMed.
- A. Silvestre-Albero, J. Silvestre-Albero, M. Martínez-Escandell and F. Rodríguez-Reinoso, Ind. Eng. Chem. Res., 2014, 53, 15398–15405 CrossRef CAS.
- Y. Zhao, X. Liu and Y. Han, RSC Adv., 2015, 5, 30310–30330 RSC.
- G.-P. Hao, W.-C. Li, D. Qian and A.-H. Lu, Adv. Mater., 2010, 22, 853–857 CrossRef CAS PubMed.
- A. Alabadi, H. A. Abbood, Q. Li, N. Jing and B. Tan, Sci. Rep., 2016, 6, 38614 CrossRef CAS PubMed.
- A. Rehman and S.-J. Park, Chem. Eng. J., 2018, 352, 539–548 CrossRef CAS.
- L. S. Schadler, L. C. Brinson and W. G. Sawyer, JOM, 2007, 59, 53–60 CrossRef CAS.
- G. Dong, H. Li and V. Chen, J. Mater. Chem. A, 2013, 1, 4610–4630 RSC.
- N. Nakamura and Y. Amao, Sens. Actuators, B, 2003, 92, 98–101 CrossRef CAS.
- S. M. Borisov, M. C. Waldhier, I. Klimant and O. S. Wolfbeis, Chem. Mater., 2007, 19, 6187–6194 CrossRef CAS.
- K. Wysokiński, M. Napierała, T. Stańczyk, S. Lipiński and T. Nasiłowski, Sensors, 2015, 15, 31888–31903 CrossRef PubMed.
- O. Oter, K. Ertekin, D. Topkaya and S. Alp, Anal. Bioanal. Chem., 2006, 386, 1225–1234 CrossRef CAS PubMed.
- D.-I. Won, J.-S. Lee, Q. Ba, Y.-J. Cho, H.-Y. Cheong, S. Choi, C. H. Kim, H.-J. Son, C. Pac and S. O. Kang, ACS Catal., 2018, 8, 1018–1030 CrossRef CAS.
- K. S. Novoselov, A. K. Geim, S. V. Morozov, D. Jiang, Y. Zhang, S. V. Dubonos, I. V. Grigorieva and A. A. Firsov, Science, 2004, 306, 666 LP–669 CrossRef PubMed.
- H. Hirai, H. Tsuchiya, Y. Kamakura, N. Mori and M. Ogawa, J. Appl. Phys., 2014, 116, 083703 CrossRef.
- K. M. Borysenko, J. T. Mullen, E. A. Barry, S. Paul, Y. G. Semenov, J. M. Zavada, M. B. Nardelli and K. W. Kim, Phys. Rev. B: Condens. Matter Mater. Phys., 2010, 81, 121412 CrossRef.
- A. D. Smith, K. Elgammal, X. Fan, M. C. Lemme, A. Delin, M. Råsander, L. Bergqvist, S. Schröder, A. C. Fischer, F. Niklaus and M. Östling, RSC Adv., 2017, 7, 22329–22339 RSC.
- F. Schedin, A. K. Geim, S. V. Morozov, E. W. Hill, P. Blake, M. I. Katsnelson and K. S. Novoselov, Nat. Mater., 2007, 6, 652 CrossRef CAS PubMed.
- E. Singh, M. Meyyappan and H. S. Nalwa, ACS Appl. Mater. Interfaces, 2017, 9, 34544–34586 CrossRef CAS PubMed.
- G. Liu, W. Jin and N. Xu, Chem. Soc. Rev., 2015, 44, 5016–5030 RSC.
- F. Perreault, A. Fonseca de Faria and M. Elimelech, Chem. Soc. Rev., 2015, 44, 5861–5896 RSC.
- D. R. Dreyer, S. Park, C. W. Bielawski and R. S. Ruoff, Chem. Soc. Rev., 2010, 39, 228–240 RSC.
- J. H. Lee, H. J. Lee and J. W. Choi, Phys. Chem. Chem. Phys., 2017, 19, 22743–22748 RSC.
- R. R. Nair, H. A. Wu, P. N. Jayaram, I. V. Grigorieva and A. K. Geim, Science, 2012, 335, 442 CrossRef CAS PubMed.
- P. Sun, M. Zhu, K. Wang, M. Zhong, J. Wei, D. Wu, Z. Xu and H. Zhu, ACS Nano, 2013, 7, 428–437 CrossRef CAS PubMed.
- H. W. Kim, H. W. Yoon, S.-M. Yoon, B. M. Yoo, B. K. Ahn, Y. H. Cho, H. J. Shin, H. Yang, U. Paik, S. Kwon, J.-Y. Choi and H. B. Park, Science, 2013, 342, 91 CrossRef CAS PubMed.
- H. Huang, Y. Ying and X. Peng, J. Mater. Chem. A, 2014, 2, 13772–13782 RSC.
- B. Mi, Science, 2014, 343, 740 CrossRef CAS PubMed.
- S. Pei and H.-M. Cheng, Carbon, 2012, 50, 3210–3228 CrossRef CAS.
- C. Gómez-Navarro, R. T. Weitz, A. M. Bittner, M. Scolari, A. Mews, M. Burghard and K. Kern, Nano Lett., 2007, 7, 3499–3503 CrossRef PubMed.
- H. Kim Jung, S. Chang Won, D. Kim, R. Yang Jong, T. Han Joong, G. W. Lee, T. Kim Ji and K. Seol Seung, Adv. Mater., 2015, 27, 157–161 CrossRef PubMed.
- L. Ganhua, E. O. Leonidas and C. Junhong, Nanotechnology, 2009, 20, 445502 CrossRef PubMed.
- X. Wang, K. Maeda, A. Thomas, K. Takanabe, G. Xin, J. M. Carlsson, K. Domen and M. Antonietti, Nat. Mater., 2008, 8, 76 CrossRef PubMed.
- S. C. Yan, Z. S. Li and Z. G. Zou, Langmuir, 2009, 25, 10397–10401 CrossRef CAS PubMed.
- F. Dong, L. Wu, Y. Sun, M. Fu, Z. Wu and S. C. Lee, J. Mater. Chem., 2011, 21, 15171–15174 RSC.
- K. Wang, Q. Li, B. Liu, B. Cheng, W. Ho and J. Yu, Appl. Catal., B, 2015, 176-177, 44–52 CrossRef CAS.
- F. Goettmann, A. Fischer, M. Antonietti and A. Thomas, Angew. Chem., Int. Ed., 2006, 45, 4467–4471 CrossRef CAS PubMed.
- A. Bétard and R. A. Fischer, Chem. Rev., 2012, 112, 1055–1083 CrossRef PubMed.
- H.-C. Zhou, J. R. Long and O. M. Yaghi, Chem. Rev., 2012, 112, 673–674 CrossRef CAS PubMed.
- M. Eddaoudi, J. Kim, N. Rosi, D. Vodak, J. Wachter, M. Keeffe and O. M. Yaghi, Science, 2002, 295, 469 CrossRef CAS PubMed.
- Y.-S. Bae, O. K. Farha, J. T. Hupp and R. Q. Snurr, J. Mater. Chem., 2009, 19, 2131–2134 RSC.
- J. A. Thompson, N. A. Brunelli, R. P. Lively, J. R. Johnson, C. W. Jones and S. Nair, J. Phys. Chem. C, 2013, 117, 8198–8207 CrossRef CAS.
- M. S. Denny Jr, J. C. Moreton, L. Benz and S. M. Cohen, Nat. Rev. Mater., 2016, 1, 16078 CrossRef.
- J. Teufel, H. Oh, M. Hirscher, M. Wahiduzzaman, L. Zhechkov, A. Kuc, T. Heine, D. Denysenko and D. Volkmer, Adv. Mater., 2012, 25, 635–639 CrossRef PubMed.
- Z. Kang, Y. Peng, Y. Qian, D. Yuan, M. A. Addicoat, T. Heine, Z. Hu, L. Tee, Z. Guo and D. Zhao, Chem. Mater., 2016, 28, 1277–1285 CrossRef CAS.
- J.-R. Li, R. J. Kuppler and H.-C. Zhou, Chem. Soc. Rev., 2009, 38, 1477–1504 RSC.
- A. Ö. Yazaydın, R. Q. Snurr, T.-H. Park, K. Koh, J. Liu, M. D. LeVan, A. I. Benin, P. Jakubczak, M. Lanuza, D. B. Galloway, J. J. Low and R. R. Willis, J. Am. Chem. Soc., 2009, 131, 18198–18199 CrossRef PubMed.
- L. Cao, K. Tao, A. Huang, C. Kong and L. Chen, Chem. Commun., 2013, 49, 8513–8515 RSC.
- Y.-B. Huang, J. Liang, X.-S. Wang and R. Cao, Chem. Soc. Rev., 2017, 46, 126–157 RSC.
- K. Sumida, D. L. Rogow, J. A. Mason, T. M. McDonald, E. D. Bloch, Z. R. Herm, T.-H. Bae and J. R. Long, Chem. Rev., 2012, 112, 724–781 CrossRef CAS PubMed.
- Y. Lin, C. Kong, Q. Zhang and L. Chen, Adv. Energy Mater., 2016, 7, 1601296 CrossRef.
- K. Li, D. H. Olson, J. Seidel, T. J. Emge, H. Gong, H. Zeng and J. Li, J. Am. Chem. Soc., 2009, 131, 10368–10369 CrossRef CAS PubMed.
- L. E. Kreno, K. Leong, O. K. Farha, M. Allendorf, R. P. Van Duyne and J. T. Hupp, Chem. Rev., 2012, 112, 1105–1125 CrossRef CAS PubMed.
- D. J. Wales, J. Grand, V. P. Ting, R. D. Burke, K. J. Edler, C. R. Bowen, S. Mintova and A. D. Burrows, Chem. Soc. Rev., 2015, 44, 4290–4321 RSC.
- I. Stassen, N. Burtch, A. Talin, P. Falcaro, M. Allendorf and R. Ameloot, Chem. Soc. Rev., 2017, 46, 3185–3241 RSC.
- S. M. Cohen, Chem. Sci., 2010, 1, 32–36 RSC.
- S. M. Cohen, Chem. Rev., 2012, 112, 970–1000 CrossRef CAS PubMed.
- K. S. Park, Z. Ni, A. P. Côté, J. Y. Choi, R. Huang, F. J. Uribe-Romo, H. K. Chae, M. O’Keeffe and O. M. Yaghi, Proc. Natl. Acad. Sci. U. S. A., 2006, 103, 10186 CrossRef CAS PubMed.
- G. Lu and J. T. Hupp, J. Am. Chem. Soc., 2010, 132, 7832–7833 CrossRef CAS PubMed.
- K. Fujie, T. Yamada, R. Ikeda and H. Kitagawa, Angew. Chem., Int. Ed., 2014, 53, 11302–11305 CrossRef CAS PubMed.
- S. Shahid, K. Nijmeijer, S. Nehache, I. Vankelecom, A. Deratani and D. Quemener, J. Membr. Sci., 2015, 492, 21–31 CrossRef CAS.
- S. Biswas, M. Grzywa, H. P. Nayek, S. Dehnen, I. Senkovska, S. Kaskel and D. Volkmer, Dalton Trans., 2009, 6487–6495, 10.1039/B904280F.
- X. Y. Chen, V.-T. Hoang, D. Rodrigue and S. Kaliaguine, RSC Adv., 2013, 3, 24266–24279 RSC.
- R. Adams, C. Carson, J. Ward, R. Tannenbaum and W. Koros, Microporous Mesoporous Mater., 2010, 131, 13–20 CrossRef CAS.
- Y. Fu, D. Sun, Y. Chen, R. Huang, Z. Ding, X. Fu and Z. Li, Angew. Chem., Int. Ed., 2012, 51, 3364–3367 CrossRef CAS PubMed.
- N. C. Burtch, H. Jasuja and K. S. Walton, Chem. Rev., 2014, 114, 10575–10612 CrossRef CAS PubMed.
- Y. Zhang, H. Wang, Y. Zhang, X. Ding and J. Liu, Sep. Purif. Technol., 2017, 189, 128–137 CrossRef CAS.
- X. Peng, L. Manna, W. Yang, J. Wickham, E. Scher, A. Kadavanich and A. P. Alivisatos, Nature, 2000, 404, 59 CrossRef CAS PubMed.
- S. Cao, F. Tao, Y. Tang, Y. Li and J. Yu, Chem. Soc. Rev., 2016, 45, 4747–4765 RSC.
- A. Loiudice, P. Lobaccaro, E. A. Kamali, T. Thao, B. H. Huang, J. W. Ager and R. Buonsanti, Angew. Chem., Int. Ed., 2016, 55, 5789–5792 CrossRef CAS PubMed.
- D. Raciti and C. Wang, ACS Energy Lett., 2018, 3, 1545–1556 CrossRef CAS.
- S. N. Habisreutinger, L. Schmidt-Mende and J. K. Stolarczyk, Angew. Chem., Int. Ed., 2013, 52, 7372–7408 CrossRef CAS PubMed.
- R. Shi, G. I. N. Waterhouse and T. Zhang, Sol. RRL, 2017, 1, 1700126 CrossRef.
- J. Hou, S. Cao, Y. Wu, Z. Gao, F. Liang, Y. Sun, Z. Lin and L. Sun, Chem. – Eur. J., 2017, 23, 9481–9485 CrossRef CAS PubMed.
- L. He, T. E. Wood, B. Wu, Y. Dong, L. B. Hoch, L. M. Reyes, D. Wang, C. Kübel, C. Qian, J. Jia, K. Liao, P. G. O’Brien, A. Sandhel, J. Y. Y. Loh, P. Szymanski, N. P. Kherani, T. C. Sum, C. A. Mims and G. A. Ozin, ACS Nano, 2016, 10, 5578–5586 CrossRef CAS PubMed.
- M. Benammar, A. Abdaoui, S. H. M. Ahmad, F. Touati and A. Kadri, Sensors, 2018, 18, 581 CrossRef PubMed.
- J. W. Severinghaus and A. F. Bradley, J. Appl. Physiol., 1958, 13, 515–520 CrossRef CAS PubMed.
- H. Liu, S. Lin, Y. Feng and P. Theato, Polym. Chem., 2017, 8, 12–23 RSC.
- I. Djerdj, A. Haensch, D. Koziej, S. Pokhrel, N. Barsan, U. Weimar and M. Niederberger, Chem. Mater., 2009, 21, 5375–5381 CrossRef CAS.
- D. R. Miller, S. A. Akbar and P. A. Morris, Sens. Actuators, B, 2014, 204, 250–272 CrossRef CAS.
- O. Hirsch, K. O. Kvashnina, L. Luo, M. J. Süess, P. Glatzel and D. Koziej, Proc. Natl. Acad. Sci. U. S. A., 2015, 112, 15803–15808 CrossRef CAS PubMed.
- R. A. Potyrailo, C. Surman, N. Nagraj and A. Burns, Chem. Rev., 2011, 111, 7315–7354 CrossRef CAS PubMed.
- C. M. Hangarter, N. Chartuprayoon, S. C. Hernández, Y. Choa and N. V. Myung, Nano Today, 2013, 8, 39–55 CrossRef CAS.
- P. Puligundla, J. Jung and S. Ko, Food Control, 2012, 25, 328–333 CrossRef CAS.
- A. Star, T. R. Han, V. Joshi, J. C. P. Gabriel and G. Grüner, Adv. Mater., 2004, 16, 2049–2052 CrossRef CAS.
- J. Wu, K. Tao, J. Zhang, Y. Guo, J. Miao and L. K. Norford, J. Mater. Chem. A, 2016, 4, 8130–8140 RSC.
- C. Willa, J. Yuan, M. Niederberger and D. Koziej, Adv. Funct. Mater., 2015, 25, 2537–2542 CrossRef CAS.
- C. Willa, A. Schmid, D. Briand, J. Yuan and D. Koziej, ACS Appl. Mater. Interfaces, 2017, 9, 25553–25558 CrossRef CAS PubMed.
- J. Boudaden, A. Klumpp, H.-E. Endres and I. Eisele, Proceedings, 2017, 1, 472 CrossRef.
- K.-J. Kim, P. Lu, J. T. Culp and P. R. Ohodnicki, ACS Sens., 2018, 3, 386–394 CrossRef CAS PubMed.
- J. Wu, M.-J. Yin, K. Seefeldt, A. Dani, R. Guterman, J. Yuan, A. P. Zhang and H.-Y. Tam, Sens. Actuators, B, 2018, 259, 833–839 CrossRef CAS.
- J. Devkota, K.-J. Kim, P. R. Ohodnicki, J. T. Culp, D. W. Greve and J. W. Lekse, Nanoscale, 2018, 10, 8075–8087 RSC.
- Y. Li, G. Li, X. Wang, Z. Zhu, H. Ma, T. Zhang and J. Jin, Chem. Commun., 2012, 48, 8222–8224 RSC.
- B. Paschke, A. Wixforth, D. Denysenko and D. Volkmer, ACS Sens., 2017, 2, 740–747 CrossRef CAS PubMed.
- Y. Ma, K. Promthaveepong and N. Li, Anal. Chem., 2016, 88, 8289–8293 CrossRef CAS PubMed.
- X. Chong, K.-J. Kim, Y. Zhang, E. Li, P. R. Ohodnicki, C.-H. Chang and A. X. Wang, Nanotechnology, 2017, 28, 26LT01 CrossRef PubMed.
- A. Haensch, D. Koziej, M. Niederberger, N. Barsan and U. Weimar, Procedia Eng., 2010, 5, 139–142 CrossRef CAS.
- J. Boudaden, A. Klumpp, H.-E. Endres and I. Eisele, Nanomaterials, 2019, 9, 1097 CrossRef CAS PubMed.
- J. Hodgkinson and R. P. Tatam, Meas. Sci. Technol., 2013, 24, 012004 CrossRef.
- A. Pusch, A. De Luca, S. S. Oh, S. Wuestner, T. Roschuk, Y. Chen, S. Boual, Z. Ali, C. C. Phillips, M. Hong, S. A. Maier, F. Udrea, R. H. Hopper and O. Hess, Sci. Rep., 2015, 5, 17451 CrossRef CAS PubMed.
- S. Moumen, I. Raible, A. Krauß and J. Wöllenstein, Sens. Actuators, B, 2016, 236, 1083–1090 CrossRef CAS.
- C. Caucheteur, T. Guo, F. Liu, B.-O. Guan and J. Albert, Nat. Commun., 2016, 7, 13371 CrossRef CAS PubMed.
- X.-d. Wang and O. S. Wolfbeis, Anal. Chem., 2016, 88, 203–227 CrossRef CAS PubMed.
- X. Chong, K.-J. Kim, E. Li, Y. Zhang, P. R. Ohodnicki, C.-H. Chang and A. X. Wang, Sens. Actuators, B, 2016, 232, 43–51 CrossRef CAS.
- M. Nazari, M. A. Forouzandeh, C. M. Divarathne, F. Sidiroglou, M. R. Martinez, K. Konstas, B. W. Muir, A. J. Hill, M. C. Duke, M. R. Hill and S. F. Collins, Opt. Lett., 2016, 41, 1696–1699 CrossRef CAS PubMed.
- Y. Ma and L.-Y. L. Yung, Anal. Chem., 2014, 86, 2429–2435 CrossRef CAS PubMed.
- S. Sivaramakrishnan, R. Rajamani, C. S. Smith, K. A. McGee, K. R. Mann and N. Yamashita, Sens. Actuators, B, 2008, 132, 296–304 CrossRef CAS.
- H. J. Lee, K. K. Park, M. Kupnik, N. A. Melosh and B. T. Khuri-Yakub, Anal. Chem., 2012, 84, 3063–3066 CrossRef CAS PubMed.
- J. Devkota, R. P. Ohodnicki and W. D. Greve, Sensors, 2017, 17, 801–828 CrossRef PubMed.
- A. L. Robinson, V. Stavila, T. R. Zeitler, M. I. White, S. M. Thornberg, J. A. Greathouse and M. D. Allendorf, Anal. Chem., 2012, 84, 7043–7051 CrossRef CAS PubMed.
- P. Davydovskaya, A. Ranft, B. V. Lotsch and R. Pohle, Anal. Chem., 2014, 86, 6948–6958 CrossRef CAS PubMed.
- R. Gondosiswanto, D. B. Hibbert, Y. Fang and C. Zhao, ACS Appl. Mater. Interfaces, 2017, 9, 43377–43385 CrossRef CAS PubMed.
- D. Aaron and C. Tsouris, Sep. Sci. Technol., 2005, 40, 321–348 CrossRef CAS.
- T. E. Rufford, S. Smart, G. C. Y. Watson, B. F. Graham, J. Boxall, J. C. Diniz da Costa and E. F. May, J. Pet. Sci. Technol., 2012, 94–95, 123–154 CAS.
- T. Rodenas, I. Luz, G. Prieto, B. Seoane, H. Miro, A. Corma, F. Kapteijn, F. X. Llabrés i Xamena and J. Gascon, Nat. Mater., 2014, 14, 48 CrossRef PubMed.
- A. Kılıç, Ç. Atalay-Oral, A. Sirkecioğlu, Ş. B. Tantekin-Ersolmaz and M. G. Ahunbay, J. Membr. Sci., 2015, 489, 81–89 CrossRef.
- T. Pröll and H. Hofbauer, Fuel Process. Technol., 2008, 89, 1207–1217 CrossRef.
- E. Favre, J. Membr. Sci., 2007, 294, 50–59 CrossRef CAS.
- G. T. Rochelle, Science, 2009, 325, 1652 CrossRef CAS PubMed.
- M. Tagliabue, D. Farrusseng, S. Valencia, S. Aguado, U. Ravon, C. Rizzo, A. Corma and C. Mirodatos, Chem. Eng. J., 2009, 155, 553–566 CrossRef CAS.
- S. Basu, A. L. Khan, A. Cano-Odena, C. Liu and I. F. J. Vankelecom, Chem. Soc. Rev., 2010, 39, 750–768 RSC.
- T. C. Merkel, H. Lin, X. Wei and R. Baker, J. Membr. Sci., 2010, 359, 126–139 CrossRef CAS.
- D. L. Gin and R. D. Noble, Science, 2011, 332, 674 CrossRef CAS PubMed.
- M. Zhou, D. Korelskiy, P. Ye, M. Grahn and J. Hedlund, Angew. Chem., Int. Ed., 2014, 53, 3492–3495 CrossRef CAS PubMed.
- S. D. Kenarsari, D. Yang, G. Jiang, S. Zhang, J. Wang, A. G. Russell, Q. Wei and M. Fan, RSC Adv., 2013, 3, 22739–22773 RSC.
- D. F. Sanders, Z. P. Smith, R. Guo, L. M. Robeson, J. E. McGrath, D. R. Paul and B. D. Freeman, Polymer, 2013, 54, 4729–4761 CrossRef CAS.
- M. Carta, R. Malpass-Evans, M. Croad, Y. Rogan, J. C. Jansen, P. Bernardo, F. Bazzarelli and N. B. McKeown, Science, 2013, 339, 303 CrossRef CAS PubMed.
- A. F. Bushell, P. M. Budd, M. P. Attfield, J. T. A. Jones, T. Hasell, A. I. Cooper, P. Bernardo, F. Bazzarelli, G. Clarizia and J. C. Jansen, Angew. Chem., Int. Ed., 2012, 52, 1253–1256 CrossRef PubMed.
- B. Seoane, J. Coronas, I. Gascon, M. E. Benavides, O. Karvan, J. Caro, F. Kapteijn and J. Gascon, Chem. Soc. Rev., 2015, 44, 2421–2454 RSC.
- L. M. Robeson, J. Membr. Sci., 1991, 62, 165–185 CrossRef CAS.
- L. M. Robeson, J. Membr. Sci., 2008, 320, 390–400 CrossRef CAS.
- M. Vinoba, M. Bhagiyalakshmi, Y. Alqaheem, A. A. Alomair, A. Pérez and M. S. Rana, Sep. Purif. Technol., 2017, 188, 431–450 CrossRef CAS.
- Z. Song, F. Qiu, E. W. Zaia, Z. Wang, M. Kunz, J. Guo, M. Brady, B. Mi and J. J. Urban, Nano Lett., 2017, 17, 6752–6758 CrossRef CAS PubMed.
- J. Zhang, S. H. Chai, Z. A. Qiao, S. M. Mahurin, J. Chen, Y. Fang, S. Wan, K. Nelson, P. Zhang and S. Dai, Angew. Chem., Int. Ed., 2015, 127, 946–950 CrossRef.
- Y. Shen, H. Wang, X. Zhang and Y. Zhang, ACS Appl. Mater. Interfaces, 2016, 8, 23371–23378 CrossRef CAS PubMed.
- F. Ranjbaran, E. Kamio and H. Matsuyama, J. Membr. Sci., 2017, 544, 252–260 CrossRef CAS.
- M. G. Cowan, D. L. Gin and R. D. Noble, Acc. Chem. Res., 2016, 49, 724–732 CrossRef CAS PubMed.
- M. Li, X. Zhang, S. Zeng, L. bai, H. Gao, J. Deng, Q. Yang and S. Zhang, RSC Adv., 2017, 7, 6422–6431 RSC.
- H. Zhu, X. Jie, L. Wang, G. Kang, D. Liu and Y. Cao, RSC Adv., 2016, 6, 69124–69134 RSC.
- Y. Ban, Z. Li, Y. Li, Y. Peng, H. Jin, W. Jiao, A. Guo, P. Wang, Q. Yang, C. Zhong and W. Yang, Angew. Chem., Int. Ed., 2015, 54, 15483–15487 CrossRef CAS PubMed.
- W. Fam, J. Mansouri, H. Li, J. Hou and V. Chen, ACS Appl. Mater. Interfaces, 2018, 10, 7389–7400 CrossRef CAS PubMed.
- M. Karunakaran, R. Shevate, M. Kumar and K. V. Peinemann, Chem. Commun., 2015, 51, 14187–14190 RSC.
- J.-R. Li, Y. Ma, M. C. McCarthy, J. Sculley, J. Yu, H.-K. Jeong, P. B. Balbuena and H.-C. Zhou, Coord. Chem. Rev., 2011, 255, 1791–1823 CrossRef CAS.
- Y. Fu, Y.-B. Jiang, D. Dunphy, H. Xiong, E. Coker, S. Chou, H. Zhang, J. M. Vanegas, J. G. Croissant, J. L. Cecchi, S. B. Rempe and C. J. Brinker, Nat. Commun., 2018, 9, 990 CrossRef PubMed.
- J. Deng, L. Bai, S. Zeng, X. Zhang, Y. Nie, L. Deng and S. Zhang, RSC Adv., 2016, 6, 45184–45192 RSC.
- K. Ueno, S. Imaizumi, K. Hata and M. Watanabe, Langmuir, 2009, 25, 825–831 CrossRef CAS PubMed.
- J. P. Gong, Soft Matter, 2010, 6, 2583–2590 RSC.
- L. Viau, M.-A. Néouze, C. Biolley, S. Volland, D. Brevet, P. Gaveau, P. Dieudonné, A. Galarneau and A. Vioux, Chem. Mater., 2012, 24, 3128–3134 CrossRef CAS.
- Q. Chen, H. Chen, L. Zhu and J. Zheng, J. Mater. Chem. B, 2015, 3, 3654–3676 RSC.
- C. Altintas, G. Avci, H. Daglar, A. Nemati Vesali Azar, S. Velioglu, I. Erucar and S. Keskin, ACS Appl. Mater. Interfaces, 2018, 10, 17257–17268 CrossRef CAS PubMed.
- P. Falkowski, R. J. Scholes, E. Boyle, J. Canadell, D. Canfield, J. Elser, N. Gruber, K. Hibbard, P. Högberg, S. Linder, F. T. Mackenzie, B. Moore Iii, T. Pedersen, Y. Rosenthal, S. Seitzinger, V. Smetacek and W. Steffen, Science, 2000, 290, 291 LP–296 CrossRef PubMed.
- M. Aresta, A. Dibenedetto and A. Angelini, Chem. Rev., 2014, 114, 1709–1742 CrossRef CAS PubMed.
- H. Büttner, L. Longwitz, J. Steinbauer, C. Wulf and T. Werner, Top. Curr. Chem., 2017, 375, 50 CrossRef PubMed.
- M. E. Royer, Compt. Rend., 1870, 731–732 Search PubMed.
- Y. Hori, K. Kikuchi, A. Murata and S. Suzuki, Chem. Lett., 1986, 897–898 CrossRef CAS.
- T. Inoue, A. Fujishima, S. Konishi and K. Honda, Nature, 1979, 277, 637 CrossRef CAS.
- M. Halmann, Nature, 1978, 275, 115 CrossRef CAS.
- H. Abdullah, M. M. R. Khan, H. R. Ong and Z. Yaakob, J. CO2 Util., 2017, 22, 15–32 CrossRef CAS.
- J. L. White, M. F. Baruch, J. E. Pander, Y. Hu, I. C. Fortmeyer, J. E. Park, T. Zhang, K. Liao, J. Gu, Y. Yan, T. W. Shaw, E. Abelev and A. B. Bocarsly, Chem. Rev., 2015, 115, 12888–12935 CrossRef CAS PubMed.
- X. Chang, T. Wang and J. Gong, Energy Environ. Sci., 2016, 9, 2177–2196 RSC.
- S. Xie, Q. Zhang, G. Liu and Y. Wang, Chem. Commun., 2016, 52, 35–59 RSC.
- Y. Ma, X. Wang, Y. Jia, X. Chen, H. Han and C. Li, Chem. Rev., 2014, 114, 9987–10043 CrossRef CAS PubMed.
- M. B. Gawande, A. Goswami, F.-X. Felpin, T. Asefa, X. Huang, R. Silva, X. Zou, R. Zboril and R. S. Varma, Chem. Rev., 2016, 116, 3722–3811 CrossRef CAS PubMed.
- L. Liu and A. Corma, Chem. Rev., 2018, 118, 4981–5079 CrossRef CAS PubMed.
- W. Tu, Y. Zhou and Z. Zou, Adv. Mater., 2014, 26, 4607–4626 CrossRef CAS PubMed.
- L. B. Hoch, T. E. Wood, P. G. O'Brien, K. Liao, L. M. Reyes, C. A. Mims and G. A. Ozin, Adv. Sci., 2014, 1, 1400013 CrossRef PubMed.
- J. K. Stolarczyk, S. Bhattacharyya, L. Polavarapu and J. Feldmann, ACS Catal., 2018, 8, 3602–3635 CrossRef CAS.
- T. Benedetti, S. Naficy, A. Walker, D. L. Officer, G. G. Wallace and F. Dehghani, Energy Technol., 2018, 6, 702–709 CrossRef CAS.
- A. Weilhard, M. I. Qadir, V. Sans and J. Dupont, ACS Catal., 2018, 8, 1628–1634 CrossRef CAS.
- Y. Liao, S.-W. Cao, Y. Yuan, Q. Gu, Z. Zhang and C. Xue, Chem. – Eur. J., 2014, 20, 10220–10222 CrossRef CAS PubMed.
- Y. Liao, Z. Hu, Q. Gu and C. Xue, Molecules, 2015, 20, 18847 CrossRef CAS PubMed.
- S. Liu, J. Xia and J. Yu, ACS Appl. Mater. Interfaces, 2015, 7, 8166–8175 CrossRef CAS PubMed.
- J. Mao, K. Li and T. Peng, Catal. Sci. Technol., 2013, 3, 2481–2498 RSC.
- L. Yuan and Y.-J. Xu, Appl. Surf. Sci., 2015, 342, 154–167 CrossRef CAS.
- K. Li, B. Peng and T. Peng, ACS Catal., 2016, 6, 7485–7527 CrossRef CAS.
- Z. D. Dong, L. J. Long and Q. S. Zhang, Adv. Mater., 2016, 28, 3423–3452 CrossRef PubMed.
- P. D. Tran, L. H. Wong, J. Barber and J. S. C. Loo, Energy Environ. Sci., 2012, 5, 5902–5918 RSC.
- M. Schreck and M. Niederberger, Chem. Mater., 2019, 31, 597–618 CrossRef CAS.
- W. Sun, C. Qian, L. He, K. K. Ghuman, A. P. Y. Wong, J. Jia, A. A. Jelle, P. G. O’Brien, L. M. Reyes, T. E. Wood, A. S. Helmy, C. A. Mims, C. V. Singh and G. A. Ozin, Nat. Commun., 2016, 7, 12553 CrossRef CAS PubMed.
- K. K. Ghuman, T. E. Wood, L. B. Hoch, C. A. Mims, G. A. Ozin and C. V. Singh, Phys. Chem. Chem. Phys., 2015, 17, 14623–14635 RSC.
- J. Wu, Y. Huang, W. Ye and Y. Li, Adv. Sci., 2017, 4, 1700194 CrossRef PubMed.
- C.-C. Yang, Y.-H. Yu, B. van der Linden, J. C. S. Wu and G. Mul, J. Am. Chem. Soc., 2010, 132, 8398–8406 CrossRef CAS PubMed.
- H. Kisch, Angew. Chem., Int. Ed., 2010, 122, 9782–9783 CrossRef.
- H. Kisch and D. Bahnemann, J. Phys. Chem. Lett., 2015, 6, 1907–1910 CrossRef CAS PubMed.
- T. Maschmeyer and M. Che, Angew. Chem., Int. Ed., 2010, 49, 1536–1539 CrossRef CAS PubMed.
- J. M. Buriak, P. V. Kamat and K. S. Schanze, ACS Appl. Mater. Interfaces, 2014, 6, 11815–11816 CrossRef CAS PubMed.
- M. Qureshi and K. Takanabe, Chem. Mater., 2017, 29, 158–167 CrossRef CAS.
- J. G. Chen, C. W. Jones, S. Linic and V. R. Stamenkovic, ACS Catal., 2017, 7, 6392–6393 CrossRef CAS.
- E. L. Clark, J. Resasco, A. Landers, J. Lin, L.-T. Chung, A. Walton, C. Hahn, T. F. Jaramillo and A. T. Bell, ACS Catal., 2018, 8, 6560–6570 CrossRef CAS.
- M. W. Logan, S. Ayad, J. D. Adamson, T. Dilbeck, K. Hanson and F. J. Uribe-Romo, J. Mater. Chem. A, 2017, 5, 11854–11863 RSC.
- C. S. Diercks, Y. Liu, K. E. Cordova and O. M. Yaghi, Nat. Mater., 2018, 17, 301–307 CrossRef CAS PubMed.
- K. M. Choi, D. Kim, B. Rungtaweevoranit, C. A. Trickett, J. T. D. Barmanbek, A. S. Alshammari, P. Yang and O. M. Yaghi, J. Am. Chem. Soc., 2017, 139, 356–362 CrossRef CAS PubMed.
- Q. Liu, Z.-X. Low, L. Li, A. Razmjou, K. Wang, J. Yao and H. Wang, J. Mater. Chem. A, 2013, 1, 11563–11569 RSC.
- R. Li, J. Hu, M. Deng, H. Wang, X. Wang, Y. Hu, H.-L. Jiang, J. Jiang, Q. Zhang, Y. Xie and Y. Xiong, Adv. Mater., 2014, 26, 4783–4788 CrossRef CAS PubMed.
- K. Niu, Y. Xu, H. Wang, R. Ye, H. L. Xin, F. Lin, C. Tian, Y. Lum, K. C. Bustillo, M. M. Doeff, M. T. M. Koper, J. Ager, R. Xu and H. Zheng, Sci. Adv., 2017, 3, 1–9 Search PubMed.
- X. Duan, J. Xu, Z. Wei, J. Ma, S. Guo, S. Wang, H. Liu and S. Dou, Adv. Mater., 2017, 29, 1701784 CrossRef PubMed.
- S. Zhao, D.-W. Wang, R. Amal and L. Dai, Adv. Mater., 2019, 31, 1801526 CrossRef PubMed.
- R. Paul, F. Du, L. Dai, Y. Ding, Z. L. Wang, F. Wei and A. Roy, Adv. Mater., 2019, 31, 1805598 CrossRef PubMed.
- A. P. Kauling, A. T. Seefeldt, D. P. Pisoni, R. C. Pradeep, R. Bentini, R. V. B. Oliveira, K. S. Novoselov and A. H. Castro Neto, Adv. Mater., 2018, 30, 1803784 CrossRef PubMed.
- W.-J. Ong, L.-L. Tan, S.-P. Chai and S.-T. Yong, Chem. Commun., 2015, 51, 858–861 RSC.
- W. Tu, Y. Zhou and Z. Zou, Adv. Funct. Mater., 2013, 23, 4996–5008 CrossRef CAS.
- W. Tu, Y. Zhou, Q. Liu, S. Yan, S. Bao, X. Wang, M. Xiao and Z. Zou, Adv. Funct. Mater., 2012, 23, 1743–1749 CrossRef.
- Y. T. Liang, B. K. Vijayan, K. A. Gray and M. C. Hersam, Nano Lett., 2011, 11, 2865–2870 CrossRef CAS PubMed.
- Y. T. Liang, B. K. Vijayan, O. Lyandres, K. A. Gray and M. C. Hersam, J. Phys. Chem. Lett., 2012, 3, 1760–1765 CrossRef CAS PubMed.
- L.-L. Tan, W.-J. Ong, S.-P. Chai and A. R. Mohamed, Nanoscale Res. Lett., 2013, 8, 465 CrossRef PubMed.
- H.-C. Hsu, I. Shown, H.-Y. Wei, Y.-C. Chang, H.-Y. Du, Y.-G. Lin, C.-A. Tseng, C.-H. Wang, L.-C. Chen, Y.-C. Lin and K.-H. Chen, Nanoscale, 2013, 5, 262–268 RSC.
- I. Shown, H.-C. Hsu, Y.-C. Chang, C.-H. Lin, P. K. Roy, A. Ganguly, C.-H. Wang, J.-K. Chang, C.-I. Wu, L.-C. Chen and K.-H. Chen, Nano Lett., 2014, 14, 6097–6103 CrossRef CAS PubMed.
- K. M. Cho, K. H. Kim, K. Park, C. Kim, S. Kim, A. Al-Saggaf, I. Gereige and H.-T. Jung, ACS Catal., 2017, 7, 7064–7069 CrossRef CAS.
- Y. Wang, X. Li, J. Song, L. Xiao, H. Zeng and H. Sun, Adv. Mater., 2015, 27, 7101–7108 CrossRef CAS PubMed.
- S. A. Veldhuis, Y. K. E. Tay, A. Bruno, S. S. H. Dintakurti, S. Bhaumik, S. K. Muduli, M. Li, N. Mathews, T. C. Sum and S. G. Mhaisalkar, Nano Lett., 2017, 17, 7424–7432 CrossRef CAS PubMed.
- Y.-F. Xu, M.-Z. Yang, B.-X. Chen, X.-D. Wang, H.-Y. Chen, D.-B. Kuang and C.-Y. Su, J. Am. Chem. Soc., 2017, 139, 5660–5663 CrossRef CAS PubMed.
- J. Sun, J. Yang, J. I. Lee, J. H. Cho and M. S. Kang, J. Phys. Chem. Lett., 2018, 9, 1573–1583 CrossRef CAS.
- M. Asadi, B. Kumar, A. Behranginia, B. A. Rosen, A. Baskin, N. Repnin, D. Pisasale, P. Phillips, W. Zhu, R. Haasch, R. F. Klie, P. Král, J. Abiade and A. Salehi-Khojin, Nat. Commun., 2014, 5, 4470 CrossRef CAS PubMed.
- H. Jung, K. M. Cho, K. H. Kim, H.-W. Yoo, A. Al-Saggaf, I. Gereige and H.-T. Jung, ACS Sustainable Chem. Eng., 2018, 6, 5718–5724 CrossRef CAS.
- S. Wang, B. Y. Guan and X. W. Lou, Energy Environ. Sci., 2018, 11, 306–310 RSC.
- H. Shi, G. Chen, C. Zhang and Z. Zou, ACS Catal., 2014, 4, 3637–3643 CrossRef CAS.
- J. Yu, K. Wang, W. Xiao and B. Cheng, Phys. Chem. Chem. Phys., 2014, 16, 11492–11501 RSC.
- G. Gao, Y. Jiao, E. R. Waclawik and A. Du, J. Am. Chem. Soc., 2016, 138, 6292–6297 CrossRef CAS PubMed.
- F. Sastre, M. J. Muñoz-Batista, A. Kubacka, M. Fernández-García, W. A. Smith, F. Kapteijn, M. Makkee and J. Gascon, ChemElectroChem, 2016, 3, 1497–1502 CrossRef CAS.
- Y. He, L. Zhang, B. Teng and M. Fan, Environ. Sci. Technol., 2015, 49, 649–656 CrossRef CAS PubMed.
- Z. Jiang, W. Wan, H. Li, S. Yuan, H. Zhao and K. Wong Po, Adv. Mater., 2018, 30, 1706108 CrossRef.
- X. Yang, W. Xin, X. Yin and X. Shao, Chem. Phys. Lett., 2016, 651, 127–132 CrossRef CAS.
- W. Yu, D. Xu and T. Peng, J. Mater. Chem. A, 2015, 3, 19936–19947 RSC.
- M. Li, L. Zhang, M. Wu, Y. Du, X. Fan, M. Wang, L. Zhang, Q. Kong and J. Shi, Nano Energy, 2016, 19, 145–155 CrossRef CAS.
- M. Reli, P. Huo, M. Šihor, N. Ambrožová, I. Troppová, L. Matějová, J. Lang, L. Svoboda, P. Kuśtrowski, M. Ritz, P. Praus and K. Kočí, J. Phys. Chem. A, 2016, 120, 8564–8573 CrossRef CAS PubMed.
- T. Ohno, N. Murakami, T. Koyanagi and Y. Yang, J. CO2 Util., 2014, 6, 17–25 CrossRef CAS.
- S.-W. Cao, X.-F. Liu, Y.-P. Yuan, Z.-Y. Zhang, Y.-S. Liao, J. Fang, S. C. J. Loo, T. C. Sum and C. Xue, Appl. Catal., B, 2014, 147, 940–946 CrossRef CAS.
- J.-C. Wang, H.-C. Yao, Z.-Y. Fan, L. Zhang, J.-S. Wang, S.-Q. Zang and Z.-J. Li, ACS Appl. Mater. Interfaces, 2016, 8, 3765–3775 CrossRef CAS PubMed.
- M. Li, L. Zhang, X. Fan, M. Wu, M. Wang, R. Cheng, L. Zhang, H. Yao and J. Shi, Appl. Catal., B, 2017, 201, 629–635 CrossRef CAS.
- L. Zhao, L. Zhang, H. Lin, Q. Nong, M. Cui, Y. Wu and Y. He, J. Hazard. Mater., 2015, 299, 333–342 CrossRef CAS PubMed.
- M. Li, L. Zhang, X. Fan, Y. Zhou, M. Wu and J. Shi, J. Mater. Chem. A, 2015, 3, 5189–5196 RSC.
- S. Wang, J. Lin and X. Wang, Phys. Chem. Chem. Phys., 2014, 16, 14656–14660 RSC.
- L. Shi, T. Wang, H. Zhang, K. Chang and J. Ye, Adv. Funct. Mater., 2015, 25, 5360–5367 CrossRef CAS.
- Z. Sun, S. Wang, Q. Li, M. Lyu, T. Butburee, B. Luo, H. Wang, J. M. T. A. Fischer, C. Zhang, Z. Wu and L. Wang, Adv. Sustainable Syst., 2017, 1, 1700003 CrossRef.
- H. Wang, Z. Sun, Q. Li, Q. Tang and Z. Wu, J. CO2 Util., 2016, 14, 143–151 CrossRef CAS.
- G. Seshadri, C. Lin and A. B. Bocarsly, J. Electroanal. Chem., 1994, 372, 145–150 CrossRef CAS.
- H.-P. Yang, S. Qin, H. Wang and J.-X. Lu, Green Chem., 2015, 17, 5144–5148 RSC.
- H.-P. Yang, S. Qin, Y. N. Yue, L. Liu, H. Wang and J.-X. Lu, Catal. Sci. Technol., 2016, 6, 6490–6494 RSC.
- T. Yui, A. Kan, C. Saitoh, K. Koike, T. Ibusuki and O. Ishitani, ACS Appl. Mater. Interfaces, 2011, 3, 2594–2600 CrossRef CAS PubMed.
- C. Liu, B. C. Colón, M. Ziesack, P. A. Silver and D. G. Nocera, Science, 2016, 352, 1210 LP–1213 CrossRef PubMed.
- K. K. Sakimoto, A. B. Wong and P. Yang, Science, 2016, 351, 74 LP–77 CrossRef PubMed.
- E. M. Nichols, J. J. Gallagher, C. Liu, Y. Su, J. Resasco, Y. Yu, Y. Sun, P. Yang, M. C. Y. Chang and C. J. Chang, Proc. Natl. Acad. Sci. U. S. A., 2015, 112, 11461 LP–11466 CrossRef PubMed.
- J. Huang, N. Hörmann, E. Oveisi, A. Loiudice, G. L. De Gregorio, O. Andreussi, N. Marzari and R. Buonsanti, Nat. Commun., 2018, 9, 3117 CrossRef PubMed.
- L. Chen, X. Tang, P. Xie, J. Xu, Z. Chen, Z. Cai, P. He, H. Zhou, D. Zhang and T. Fan, Chem. Mater., 2018, 30, 799–806 CrossRef CAS.
- G. Sastre, J. van den Bergh, F. Kapteijn, D. Denysenko and D. Volkmer, Dalton Trans., 2014, 43, 9612–9619 RSC.
Footnote |
† Contributed equally to this work. |
|
This journal is © The Royal Society of Chemistry 2020 |
Click here to see how this site uses Cookies. View our privacy policy here.