DOI:
10.1039/C9RA09572A
(Paper)
RSC Adv., 2020,
10, 2216-2226
Analytical modeling framework for performance degradation of PEM fuel cells during startup–shutdown cycles†
Received
17th November 2019
, Accepted 31st December 2019
First published on 13th January 2020
Abstract
Startup–shutdown cycling is one of the main factors that contribute to fuel cell deterioration related to high cathode potential. In this study, a coupled model with the carbon corrosion model and agglomerate model of the cathode catalyst layer is built to predict performance degradation during startup–shutdown cycles. The carbon corrosion model calculates the carbon loading loss through the rate equations and material balance expressions of seven reactions, while the agglomerate model describes the catalyst layer performance according to the computed structural parameters. A set of operational and structural parametric studies are used to investigate their effects on initial performance and voltage degradation rate. The maximum voltage of the cyclic load is found to have a greater influence over the voltage degradation rate compared with relative humidity, pressure, and minimum voltage of the cyclic load. Among the structural parameters, the carbon loading and platinum loading have the greatest and least impact on voltage degradation rate, respectively, while ionomer fraction has a complex and nonmonotonic effect. An optimal design strategy is provided with a case demonstration. Results may provide a fundamental and important tool for degradation prediction in startup–shutdown conditions and guidance for catalyst layer design and operation.
1 Introduction
Faced with environmental pollution and resource shortage, the proton exchange membrane fuel cell (PEMFC) has considerable potential as an alternative to the traditional internal combustion engine1 due to its high energy density, high efficiency, and theoretically zero emission.2 In addition, FC is more reliable than lithium batteries which have many safety issues.3 However, the widespread marketing of FC generators in vehicles still faces two major bottlenecks: cost and durability. According to the roadmap of the U.S. Department of Energy, the lifetime of commercial PEMFCs needs to reach 8000 h by 2025.4 The challenge of PEMFC durability is the complex operating conditions of vehicles, including speed change, startup and shutdown, idling, and high power. Frequent startup and shutdown cycling contributes 33% to the degradation of PEMFC.5 This process causes an instantaneous condition and local potential in the catalyst layer (CL) can reach up to 1.5 V, which is abnormal in FC operations. Two critical issues in CL exist under this condition, namely, degradation mechanism and performance characterization, and they are investigated through experiment and simulation.
As shown in Fig. 1a, startup and shutdown cycles are represented normally in literature6–10 by a triangle wave voltage between 1 and 1.5 VRHE with a sweep rate of 500 mV s−1 to investigate its degradation mechanisms and phenomena. Carbon corrosion during cyclic startup and shutdown can lead to the collapse of porous structure and reduction of material transport capacity11 (see Fig. 1b), the increase in platinum particles, and reduced electrochemical surface area (ECSA).6,12,13 After an extended shutdown, a small amount of oxygen will appear at both electrodes due to pressure and sealing problems. Once FCs start working, hydrogen enters the anode channel, resulting in a hydrogen/oxygen interface. Takagi et al.14 reported that the excessive cathode potential and carbon corrosion are mainly caused by the formation of hydrogen/oxygen interface under the startup–shutdown cycles.
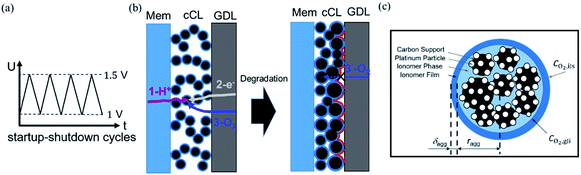 |
| Fig. 1 Schematic of (a) startup–shutdown cycles and (b) cathode structural changes in degradation, including reduction of thickness and coarsening of catalyst. The reaction processes in cathode comprise (1) proton transport in membrane and ionomer phase of CL; (2) electron conduction in the gas diffusion layer (GDL) and CL; (3) oxygen diffusion in the GDL and CL; (3′) blocked oxygen diffusion due to degradation of CL. (c) An enlarged view of a single agglomerate composed of carbon support, platinum particles, and ionomer phase. | |
Several models were proposed to explore the carbon corrosion mechanisms because an experiment would be costly and time-consuming. Meyers and Darling15 developed a 1D model of carbon corrosion in both steady-state and transient operation. Findings indicate that hydrogen maldistribution leads to oxygen evolution and carbon corrosion on the cathode. Uniform distribution of fuel and control of cathode potential can relieve degradation during startup–shutdown conditions. A more comprehensive model considering the reactions of platinum and their effect on carbon corrosion was developed by Chen et al.16 The effect of initial platinum state at the anode and cathode, temperature, and anode oxygen diffusivity was studied. A set of two-dimensional models17–19 were developed to take into consideration the difference of current density along the thickness direction. Carbon corrosion can reportedly be mitigated only by optimizing the catalyst. Other models focus solely on electrochemical reactions of the carbon support. Gallagher and Fuller20 proposed an electrochemical mechanism and numerical model for graphitized carbon. According to their study, carbon mass loss and surface oxide growth over time can be predicted. Their findings also indicate that three mechanisms contribute to current decay during electrochemical oxidation: carbon loss due to CO2 formation, reversible formation of a passive oxide, and irreversible formation of another oxide.
Another issue is the description of CL structure and simulation of CL performance. Models for the cathode CL can be classified into three categories according to their different complexity:21 interface models, homogeneous models, and agglomerate models (see Fig. 1c). Among them, the agglomerate model, which shows the best match with experimental data,22 has been used more often to study the related issues of CL in recent years.23–26 Moein-Jahromi et al.21 used the agglomerate model to simulate the CL, and the results were compared with those of the homogeneous one. A set of parametric studies were performed, in which the agglomerate size was found to be the most influential parameter. Ismail et al.26 studied the influence of the shape of agglomerates and found that slight changes in structure can substantially improve fuel cell performance.
Carbon corrosion models focus on reactions and its influencing factors and the strategy for mitigating corrosion, while agglomerate models provide ways to calculate performance. In automobile startup–shutdown operations, a sequential relationship exists between carbon corrosion and performance degradation. However, models for establishing this connection are still lacking at present. Thus, this study aims to fill this gap to provide useful guidance for the better understanding of FC performance in automobiles.
In this study, the carbon corrosion model and CL agglomerate model are combined to predict the performance of CL during startup–shutdown cycles. Both models were validated versus experimental data from literature. A parametric study was presented to analyze the effect of operation condition (e.g., relative humidity [RH], pressure, and maximum and minimum voltage) and structural parameters (e.g., carbon loading, platinum loading, and ionomer fraction) in the agglomerate model on the initial performance and voltage degradation rate (VDR) at various numbers of cycles.
2 Methods
2.1 Carbon corrosion model
The electrochemical oxidation of carbon comprises seven reactions with the individual kinetic equation from eqn 1–7 in Table 1 with the basic framework adopted from ref. 14 and 20. θab represents the coverage of species b on site a. x is the stoichiometric number fitted by Gallagher et al.20 V is the electrode potential. g is a Frumkin factor that describes the change in activation energy when oxide coverage differs.
Table 1 Reaction and kinetic equations of carbon corrosion
Reaction equations |
Kinetic governing equations |
C# + H2O → C#OH + H+ + e− |
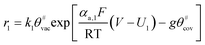 |
(1) |
C# + H2O ⇌ C# (H2O)ads |
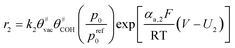 |
(2) |
C# (H2O)ads + C#OH → C#OC#OH + 2H+ + 2e− |
〃 |
2C#OC#OH + 3C* + 3H2O → C*O(C*OOH)2 + 2C# +2C#OH + 4H+ + 4e− |
〃 |
C*O(C*OOH)2 → C*OOH + C*O + * + CO2 + H+ + e− |
〃 |
C*OOH → * + CO2 + H+ + e− |
〃 |
xC# + H2O ⇌ C#xO + 2H+ + 2e− |
 |
(3) |
2C#OH + (x − 2) C# + H2O → C#xO3 + 4H+ + 4e− |
 |
(4) |
C#xO + C*O → C#xO2 + C* |
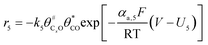 |
(5) |
2C#OH + (x − 2) C# + H2O + C#xO2 → C#xO3 + C#xO2 + 4H+ + 4e− |
 |
(6) |
C#x (OH)2 ⇌ C#xO2 + 2H+ + 2e− |
 |
(7) |
According to this model, carbon mass loss and surface oxide growth over time under different temperatures and potential conditions can be predicted.
2.1.1 Electrochemistry and kinetic expressions. Two types of reaction sites of C# and C* are consumed in this mechanism due to the separate location for water absorption and CO2 formation. The first reaction, eqn 1, denotes water functions as the oxidant to stimulate the growth of catalytic surface oxide. The adopted active center of C#OH is the only surface oxide proved by experimental evidence.20 The second reaction is more complex, involving the reversible absorption of water and subsequently the combination of C# (H2O)ads and C#OH, and a series of oxidation steps to produce CO2. The second step of this reaction is assumed to be the rate determining step. The reversible formation of carbon oxide C#x O and the irreversible formation of C#x O3, quinone C#x O2 and hydroquinone C#x (OH)2 is suggested to affect the equilibrium concentration of C#OH as shown in eqn 3–7. Of the seven reactions mentioned above, the second reaction (See eqn 2) to produce CO2 is the key step. We can take a preliminary look at the factors that influence the degradation rate. First of all, the reaction kinetics is greatly affected by temperature T, which can directly change the rate or indirectly by changing the equilibrium potential U2. In addition, carbon oxidation is dependent on the water partial pressure, so p0 directly affects the corrosion rate. Increasing the applied potential that is at the exponential position in the expression will greatly accelerate the corrosion rate. The detailed parameter interpretation and value are shown in Tables S1 and S2.†
2.2 Agglomerate model of the catalyst layer
The agglomerate model of the cathode CL in this study is based on the following assumptions:
• The model is one-dimensional and under steady state.
• The reaction condition is isothermal and isobaric.
• The reaction gasses are regarded as ideal.
• Void spaces in the CL are filled by liquid water.
• The agglomerate particles are spherical in shape with identical diameters.
Key processes and equations are introduced as follows, while other expressions of parameters are shown in Table 3.
Table 3 Expressions of parameters in the carbon corrosion and agglomerate models
Parameters |
Expressions |
Ref. |
Oxygen diffusion coefficient in liquid water, DO2,w |
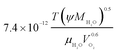 |
21 |
Effective oxygen diffusion coefficient in agglomerate,  |
DO2,i L1.5i,agg |
21 |
Thiele modulus, ϕL |
 |
29 |
Reaction effectiveness factor, Er |
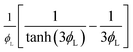 |
29 |
Local water content of the ionomer phase, λ |
0.3 + 10.8 RH − 16RH2 + 14.1 RH3 |
29 |
Protonic conductivity, κl |
 |
29 |
Effective protonic conductivity, κeffl |
κlL1.5l |
22 |
Effective electronic conductivity, κeffs |
κsL1.5Pt/C |
22 |
Reversible potential of the reaction of hydrogen and oxygen, Erev |
1.229 − 0.85 × 10−3 (T − 298.15) + 4.31 × 10−5 T[ln(χH2P) + 0.5 ln(χO2P)] |
22 |
Reversible potential of Pt decomposition reaction, Erev,Pt |
1.188 + 3.2376 × 10−4 (T − 298) |
30 |
2.2.1 Diffusion of oxygen. In the process of oxygen reduction, the dissolved oxygen is transported from the outer surface of the ionomer film to the catalyst sites. The mass transport process can be described using Fick's law:28 |
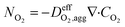 | (15) |
where
is the effective oxygen diffusion coefficient within an agglomerate particle. The penetrated oxygen flux into the ionomer film covering the agglomerate can be calculated by the following equation:29 |
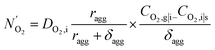 | (16) |
where CO2,g|i and CO2,i|s are the oxygen concentration at the gas/ionomer interface and the ionomer/solid interface, respectively. The conservation of oxygen at steady state can be expressed as |
 | (17) |
2.2.2 Oxygen reduction reaction within the agglomerate. The consumption rate of oxygen is linearly proportional to its concentration. Therefore, Ragg can be expressed aswhere Er is defined as an effectiveness factor for agglomerate.29 Consequently, the oxygen consumption rate RO2 can be expressed as |
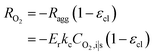 | (19) |
where εcl is the porosity of CL that is shown as Fig. S1.†
2.2.3 Electrochemical reaction rate. According to Faraday's law NO2 = −i/4F, the relationship between current density and oxygen consumption rate is shown in the following equation: |
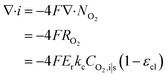 | (20) |
Combined with eqn (16), (17), and (19), we can write CO2,i|s in terms of CO2,g|i. Thus, the current density gradient is obtained as follows:
|
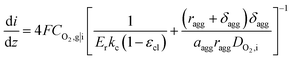 | (21) |
On the other hand, the current density in homogeneous view can be described by the Butler–Volmer equation:31
|
 | (22) |
where

is the reference oxygen concentration.
αa and
αc are anodic and cathodic transfer coefficient respectively. The homogeneous model can be also retrieved from
eqn (21) when
ragg and
δagg tend to 0:
|
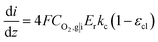 | (23) |
Therefore, kc can be expressed according to eqn (22) and (23)
|
 | (24) |
2.2.4 Local overpotential. The protonic and electronic resistance result in the distribution of local overpotential within the CL, which can be expressed as follows: |
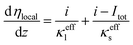 | (25) |
where κeffl and κeffs are the effective protonic conductivity and the effective electronic conductivity, respectively. Itot is the given cell current density. To obtain the cell voltage at a given current density Itot, the following equation was used:32 |
Ucell = Erev − ηlocal − ROhmItot
| (26) |
where Erev is the reversible potential of the reaction of hydrogen and oxygen; ROhm is treated as a constant in this study.
2.2.5 Mass transport of oxygen. The coupling relation between oxygen concentration and current density is as follows: |
 | (27) |
where
is the effective diffusion coefficient of oxygen. According to the assumption that the void spaces are filled by liquid water, oxygen can diffuse to the surface of the agglomerate by two routes: within the void spaces flooded by water and ionomer phase. The detailed derivation process of
is presented in ESI.†
2.2.6 Boundary conditions. At z = 0, the oxygen concentration is determined using Henry's law:22 |
 | (28) |
where HO2,w is Henry's constant of oxygen in water. Protons are assumed to be consumed before they reach the GDL/CL boundary.21 Hence, At z = tcl, the current density is equal to the total cell current density
2.3 Agglomerate radius and electrochemical surface area
According to Ostwald ripening theories, platinum particles undergo the process of dissolution (Pt ⇌ Pt2+ + 2e−) and redeposition (Pt2+ + 2e− → Pt), which are driven by the tendency to decrease in total surface free energy.33 Consequently, large particles grow at the expense of dissolution of the smaller ones, leading to the increase in the mean radius of platinum particles and the decrease of the particle number. The coarsening of platinum particles34,35 can be written as |
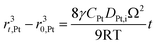 | (31) |
in which DPt,i is the diffusion coefficient of platinum in ionomer phase fitted to the experimental data of radius.9 Ω is molar volume of Pt. CPt is the equilibrium concentration (in units of mol m−3) of solute Pt2+, which is expressed as follows: |
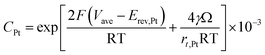 | (32) |
where Erev,Pt is the reversible potential of platinum decomposition reaction. With the combination of eqn (31) and (32), the radius of platinum particles at any time during startup–shutdown cycles can be calculated.
The exchange current density is calculated based on the effective catalyst surface area aeff, which is expressed by the platinum loading and ECSA
|
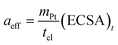 | (33) |
The electrochemical surface area has a great influence on the performance of the CL. Thus, its attenuation under startup–shutdown cycles needs to be reasonably quantified. In this study, ECSA at any time is calculated based on the radius of the Pt particle
|
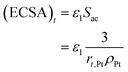 | (34) |
where
ρPt is the density of platinum.
The agglomerate radius also changes with the potential cycles, which can be related to the radius of the platinum particles. The radius of the agglomerate for degraded CL can be expressed as:35
|
 | (35) |
2.4 Computation strategy
A coupled carbon corrosion–CL model that considers the influence of carbon corrosion behavior to the structure change of CL was developed to evaluate the effect of the transient operation condition and main parameters of CL. Fig. 2 introduces the detailed solution procedure of the modeling framework built in this study. The initial value of the agglomerate radius, r0,agg, and the thickness of the ionomer film covering an agglomerate, δ0,agg, are determined by adjusting them until the output results of the agglomerate model comply with the results of experiments. Afterwards, ECSA, the radius of the agglomerate, and structural parameters after N startup–shutdown cycles are calculated to be the input of agglomerate model. Finally, the governing equations in agglomerate model are solved to obtain the performance of degraded CL.
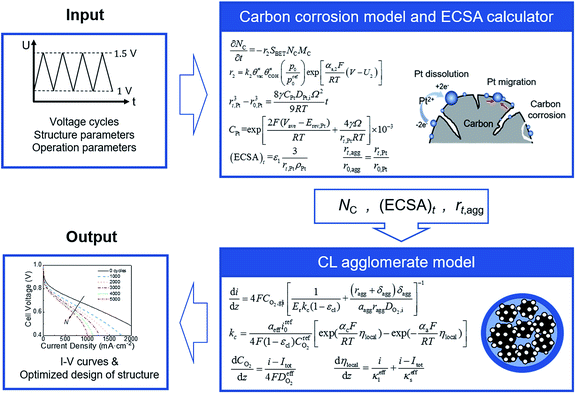 |
| Fig. 2 Schematic of the model framework for predicting the performance of the cathode CL undergoing startup–shutdown cycles and structural parameter optimization. A potential cycling between 1 and 1.5 VRHE with a sweep rate of 500 mV s−1 is used to simulate startup–shutdown conditions. | |
The carbon corrosion model is used to calculate the loss of mass loading per area of the cathode under different operating conditions and structure parameters. Based on the detachment of Pt particles6 and collapse of porous structure11 caused by carbon corrosion, the platinum loading and volume of void space were assumed to decrease proportionally to the carbon load loss for the sake of simplicity. Meanwhile, the ECSA and radius of agglomerate during voltage cycles are obtained according to eqn (34) and (35), respectively, in Section 2.3. The agglomerate model of CL is a performance calculator to obtain the distribution of overpotential, current density, and other parameters. The model establishes the relationship between structural parameters and performance.
3 Results and discussion
3.1 Model validation
In this study, the modeling framework for degradation prediction consists of the carbon corrosion model and the agglomerate model. To have a more rigorous validation process and take the advantage of available test data, two separate experiments from previous literatures will be used to validate two sub-models respectively. In addition, the experimental results of 1000 and 2000 cycles are used to validate the coupled model considering the possible synergies between the two sub-models.
3.1.1 Carbon loss validation. According to the carbon corrosion model, carbon mass loss under different potential conditions can be predicted. Fig. 3 shows the comparison between the simulation results and the experimental data36 during potential cycles. It was found that the compatibility between experiment data and the model results in ref. 36 is a bit better than this study. We still use a comprehensive model rather than a simplified model because the comprehensive one considers the reactions of several carbon surface oxides which play an important role in the electrochemical oxidation and are necessary for more in-depth research.
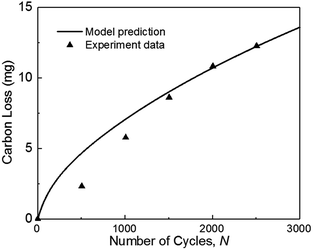 |
| Fig. 3 Validation of carbon mass loss with experiments of ref. 36 during square-wave potential cycles between 1 and 1.5 VRHE with a rest time of 5 s at each potential. (operation condition: T = 70 °C, P = 1.5 atm, RH = 100%). | |
Fig. 3 also indicates that the model result is larger than the experimental data when the number of cycles N is smaller than 1500. As the number of cycles increases, the predicted carbon loss follows the experimental results. This situation probably occurred because the complex reactions of carbon corrosion in the experiment require a transition time for reaction species intake and exhaust, thereby causing the experimental results to be smaller initially. In the experiment, the mass of carbon loss was calculated from the detected concentration of CO2 measured by non-dispersive infrared spectroscopy (NDIR). The detection of gas is lagging the degradation test (see Fig. 14(a) in ref. 9 and Fig. 6 in ref. 37), but this deviation is not considered in the model, which leads to the simulation results being larger than the experimental results at the beginning.
3.1.2 Performance validation. The performance of cathode CL during startup–shutdown cycles is calculated using the agglomerate model. According to the recommendations of The Fuel Cell Commercialization Conference of Japan,38 the potential cycling between 1 and 1.5 VRHE with a sweep rate of 500 mV s−1 simulates startup–shutdown conditions. As shown in Fig. 4, the polarization curves after 0, 1000, and 2000 cycles are validated. The model results match well with the experimental data9 for both fresh and degraded CL. It illustrates the performance loss of degraded CL compared with the initial one due to the ECSA decline and radius growth of agglomerate with the increasing number of cycles. Carbon loss rate, as well as ECSA and agglomerate radius are factors affecting simulated performance.
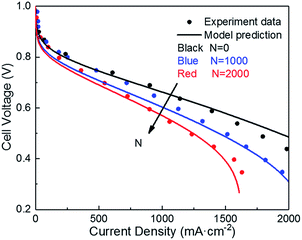 |
| Fig. 4 Validation of polarization curve with experiments of ref. 9 during the potential cycling between 1 and 1.5 VRHE with a sweep rate of 500 mV s−1 (operating conditions: T = 80 °C, P = 1 atm, RH = 100%). | |
3.2 Effect of operating conditions
3.2.1 Relative humidity. RH is an important parameter in PEMFC operation and affects output performance and degradation during operation. In this study, the minimum value of the range of RH is 40% at which the proton exchange membrane lacks moisture, while the maximum value is 100%, a commonly used value in simulations and experiments.With decreasing RH, the water content of the proton exchange membrane reduces, leading to reduced protonic conductivity of the membrane phase. As shown in Fig. 5a, a small RH corresponds to poor initial performance of CL. However, RH has the opposite effect on performance degradation under startup–shutdown cycles. As shown in Fig. 5b, increasing RH will increase the VDR. In the carbon corrosion model, RH affects the parameter p0 (see eqn 2). An increase in RH accelerates the carbon corrosion reaction rate, resulting in increased performance losses. Similar conclusions can be found in the article by Takeuchi et al.14 Therefore, RH is an important parameter for controlling performance and VDR. A PEMFC needs to work at an optimal value of RH. In addition, in the actual situation, a low RH causes the proton exchange membrane to be insufficiently wetted, resulting in a large increase in membrane resistance and contact resistance, which is not considered in this model.
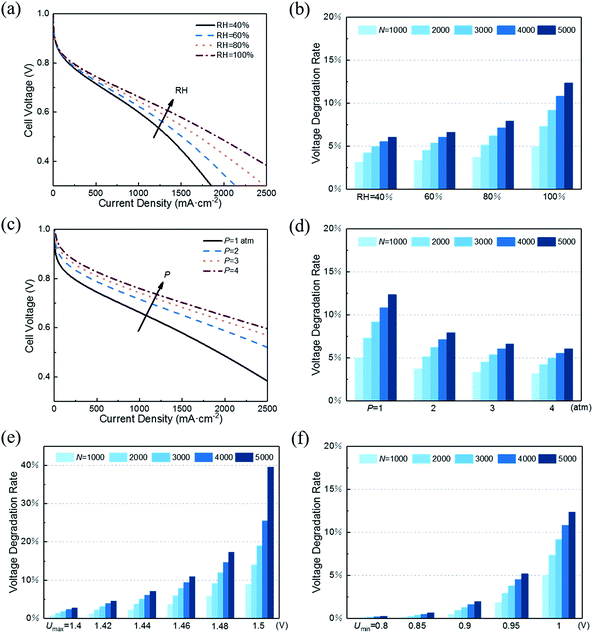 |
| Fig. 5 Effects of operating parameters such as (a and b) RH, (c and d) pressure, (e) maximum voltage of the cyclic load, and (f) minimum voltage of cyclic load on initial performance of CL and VDR during cycles. The VDR is the ratio of voltage loss to initial voltage at a current density of 500 mA cm−2 (b, d and f) or 1000 mA cm−2 (e). | |
3.2.2 Pressure. Pressure is the second operation parameter that is studied. Pressure appears to have a great influence on the performance. Fig. 5c shows that as the pressure increases from 1 atm to 4 atm, the voltage at a current density of 500 mA cm−2 increases from 0.74 V to 0.83 V. Such a result can be explained by the increase in the equilibrium potential of oxygen reduction reaction (ORR) and the supplied oxygen concentration. In the simulation of Khajeh-Hosseini-Dalasm et al.,22 oxygen can only penetrate the CL for a short distance, indicating that most of the catalyst is not utilized. Therefore, increasing the gas pressure can increase the oxygen concentration, thereby increasing the utilization of platinum in the CL and obtaining better performance.As for performance degradation, pressure does not affect carbon corrosion in this study. The different results shown in Fig. 5d indicate that the initial performance vary under different pressures while the voltage loss is the same.
3.2.3 Maximum and minimum voltage of the cyclic load. In this section, the effects of varying maximum or minimum values on performance degradation were studied, although the startup–shutdown cycle potential is between 1 and 1.5 VRHE with a sweep rate of 500 mV s−1 according to the standard of the accelerated stress test. Note that when the maximum or minimum value changes, the sweep speed changes while the period is fixed at 2 s. For instance, when Umax decreases from 1.5 V to 1.48 V, Umin remains constant at 1 V, and the sweep speed is set to 480 mV s−1.As shown in Fig. 5e, the maximum voltage Umax has a great influence on VDR. The slight decrease in Umax causes VDR to drop sharply. The same influence trend was also proposed in the research of Dhanushkodi et al.36 When PEMFC starts and stops, the generated hydrogen–oxygen interface causes the cathode to suffer from an abnormally high potential of about 1.5 V. As Umax decreases, the rate of carbon corrosion reactions decreases exponentially. Moreover, the increase in the radius of platinum particles will reduce the ECSA and increase the radius of the aggregates, which will decrease the CL performance. The increase rate of the platinum particle radius decreases with the reduction of Umax. The combination of the above two points results in slow performance degradation as Umax decreases.
Fig. 5f illustrates that reducing Umin can also reduce performance degradation. The same reason is that its decrease reduces carbon corrosion and slows the growth of platinum particle radius. Similarly, in the study of Gallagher et al.,20 the carbon corrosion caused by lower voltage is weaker.
3.3 Effect of structural parameters
In this section, a parametric study is performed to provide guidance for the optimum design of CL expected to possess both excellent initial performance and resistance to degradation. Therefore, the effect of following key structural parameters on initial voltage and VDR are studied: (1) carbon loading, mC; (2) platinum loading, mPt; (3) ionomer fraction in agglomerate, Li,agg.
3.3.1 Carbon loading. Carbon is used to support the catalyst through which electrons are transferred and is a major component in the CL. The electronic conductivity increases as the carbon loading increases. However, this improvement has been limited by the high electron conductivity in the solid phase. More obviously, the porosity reduction caused by the increase in carbon loading results in the increased resistance of oxygen transmission, thereby increasing the overpotential and reducing the cell performance. To study the effect of carbon loading on the CL performance and degradation, simulations are performed at different carbon loadings in the range of 0.3–0.5 mg cm−2. The results are shown in Fig. 6.
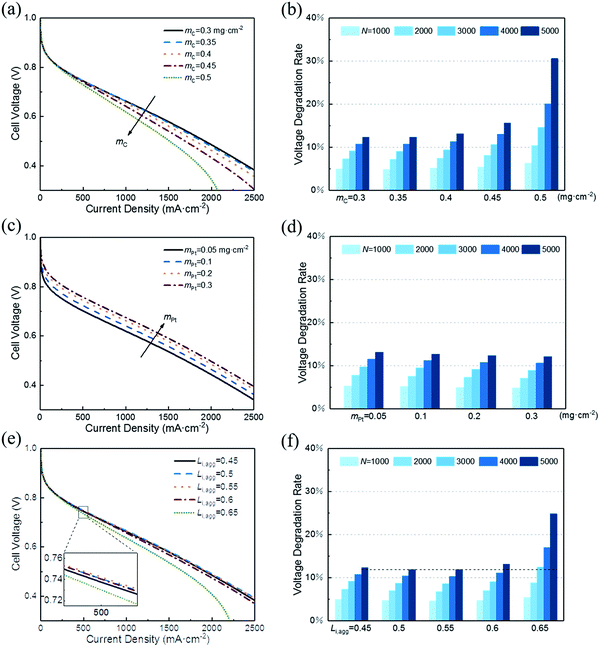 |
| Fig. 6 Effect of (a and b) carbon loading, (c and d) platinum loading, and (e and f) ionomer fraction in agglomerate on the initial performance of CL and VDR during cycles. The VDR is the ratio of voltage loss to initial voltage at a current density of 500 mA cm−2. | |
Fig. 6a shows that the initial performance decreases with the increase in carbon loading when other parameters are fixed. The increase in carbon loading leads to the increase in solid phase (Pt/C) and the reduction in CL porosity. As a result, electronic conductivity increases and the diffusion coefficient of oxygen decreases. Their net effect is a lower cell voltage at different current density. Moreover, the polarization curves corresponding to 0.3 and 0.35 mg cm−2 are close to overlap. This finding suggests that excessive reduction of carbon loading has little effect on performance.
In addition, small porosity of the CL corresponds to a great impact when recession occurs. Fig. 6b illustrates that the VDR after different startup–shutdown cycles increases as the carbon loading increases. This condition occurs because the CL with low porosity is more likely to produce closed pores, resulting in a high resistance of oxygen transport. Notably, the VDR for cases of 0.3 and 0.35 mg cm−2 are almost the same.
3.3.2 Platinum loading. The noble metal platinum together with the carbon support constitutes the solid phase in CL. Platinum is scarce and costly; thus, many studies aim to reduce platinum load while ensuring excellent performance. Currently, the platinum loading of many commercial catalysts can be reduced to about 0.2 mg cm−2. For low platinum loading MEA, this value can be 0.05 mg cm−2 or even lower. In this parametric study, the effect of platinum load was studied with a range of 0.05–0.3 mg cm−2. With the increase in platinum loading, the performance of CL was slightly improved, but was less sensitive than that of carbon loading. That's the reason to increase the data interval. The increase in platinum loading also leads to an increase in solid phase and a decrease in CL porosity. However, these two volumetric changes are less obvious than those in the study on carbon loading because platinum is much denser than carbon.In addition, the effective platinum surface area per unit CL volume increases with increased platinum loading. Therefore, the ORR rate increases and hence enhances the performance. Likewise, increasing platinum load continuously has less impact on performance improvement, as shown in Fig. 6c. When platinum loading changes from 0.1 to 0.2 mg cm−2, the voltage at 500 mA cm−2 increases by 22 mV, while from 0.2 to 0.3 mg cm−2, the value is 12 mV. This result indicates that boosting performance by increasing platinum load is not optimal when considering the cost. Cho et al.39 and Khajeh-Hosseini-Dalasm et al.22 have made similar conclusions.
As shown in Fig. 6d, the VDR decreased slightly with the increase in platinum loading. Actually, the voltage loss of different platinum loading has little difference. The difference in initial voltage is what causes VDR to vary. The VDR of 0.05 and 0.3 mg cm−2 after 5000 cycles is 13.17% and 12.13%, respectively. Compared with the data in Fig. 6a, the effect of platinum loading on voltage loss is less sensitive than carbon loading.
3.3.3 Ionomer fraction in the agglomerate. The ionomer phase in the CL is the site of proton conduction. In this model, the volume fraction of ionomer in CL is calculated using eqn S5.† Thus, the ionomer fraction in agglomerate was studied instead of the overall fraction in CL. The performance curve and a bar chart of voltage decay for various ionomer fraction in agglomerate are shown in Fig. 6e. Different from the case of carbon loading and platinum loading, the effect of ionomer fraction in agglomerate on CL is more complex; an optimal value of Li,agg exists for both the best initial performance and lowest degradation rate.A rise in the cell voltage at 500 mA cm−2 is observed when Li,agg is increased from 0.45 to 0.5. As its value continues to increase, the voltage drops back to a level that is much lower than in the case of 0.45. The simulation of Srinivasarao et al. obtained similar results.40 The influence of ionomer fraction on the performance has positive and negative effects; with the increase in ionomer fraction, the proton transfer capacity of CL is improved. However, the high ionomer fraction can lead to water affinity of the electrode, which prevents the reaction gas from reaching the active site of the catalyst and causes the water produced by the cathode to be discharged, thus reducing the utilization rate of platinum and causing the electrode to be flooded. In addition, excessive ionomer fraction increases the electron transfer resistance and oxygen diffusion resistance of CL.
The effect of Li,agg on VDR during cycles is shown in Fig. 6f. Similar to the initial performance, the VDR decreases first and then increases with increasing Li,agg. The protonic resistance of CLs with higher ionomer fraction increases more slowly than that of CLs with lower ionomer fraction.41 However, high ionomer fraction results in less porosity, making CLs more likely to produce closed pores, which then leads to the higher resistance of oxygen transport and more serious degradation of performance.
3.4 Design of CL structural parameters
In the parametric study, a variable is changed within a range by keeping other parameters constant. However, the optimum value of each variable is supposed to vary with changes in the other structural parameters. Therefore, cases of different combinations of parameters are performed for integrated optimum design of CL. As shown in Fig. 7, the initial performance and resistance of degradation are described by the initial voltage U0 and the normalized voltage U′/U0, respectively. U0 and U′ are the voltage at 500 mA cm−2 of pristine CL and degraded CL after 5000 cycles, respectively.
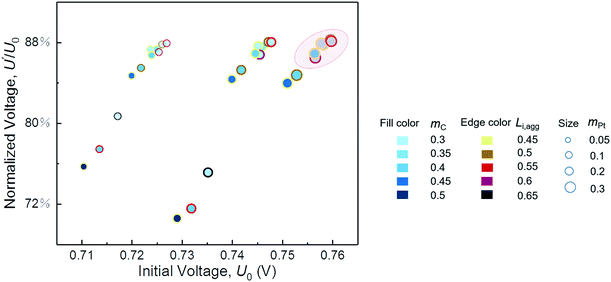 |
| Fig. 7 Performance and degradation comparison of different combinations of parameters. The normalized voltage is the ratio of degraded voltage to initial voltage at a current density of 500 mA cm−2. | |
We expect to prepare CL with both excellent initial performance and resistance to degradation. Fig. 7 indicates that, regardless of cost, a satisfactory CL requires proper ionomer fraction, low carbon loading, and high platinum loading within the range of our parametric study. If the structure parameters of CL are in circle range, then its performance and ability to resist degradation will be satisfactory. Furthermore, the optimal value of the ionomer fraction in agglomerate depends on the values of carbon loading and platinum loading. In this study, the parameters of the best CL are mC = 0.3 mg cm−2, mPt = 0.3 mg cm−2, and Li,agg = 0.55. Table 4 shows the comparison of the model results with the optimization parameters in some literatures. The model predicts the optimal structural parameters well. It should be noted that the Nafion content Li of this study is calculated by Li,agg (see eqn S5† for specific calculation). What's more, few factors can be studied in a single experiment limited by time and cost. Simulation work of this study just makes up for this shortcoming.
Table 4 Comparison of simulation results with reported optimized parameters in experimental study
Source |
Factors studied |
mPt/mg cm−2 |
mC/mg cm−2 |
Li |
This study |
mPt/mC/Li |
0.3 |
0.3 |
0.35 |
Ref. 42 |
mPt/Li |
0.5 |
— |
0.3 |
Ref. 43 |
mPt |
0.4 |
— |
— |
Ref. 44 |
Li |
— |
— |
0.34 |
Ref. 45 |
mPt |
0.28 |
— |
— |
4 Conclusions
In this study, an analytical modeling framework is established to predict the performance degradation of cathode CL during startup–shutdown cycles. The carbon corrosion model, together with assumptions that the platinum loading and volume of void space decrease proportionally to the carbon load loss, is used to calculate the changes in structural parameters. The ECSA is calculated by its relation with platinum radius. The output of structural parameters and ECSA is then used in the agglomerate model to predict the performance of CL after any number of cycles. The results of the model match well with the experimental data. After model validation, the effect of several operational and structural parameters is investigated in a parametric study. The operating parameters include RH, pressure, and maximum and minimum voltage of the cyclic load, while structural parameters cover carbon loading, platinum loading, and ionomer fraction in agglomerate. On the basis of this work, the following conclusions can be made:
• Increasing gas pressure can improve CL performance and reduce VDR due to the increase in oxygen supply concentration. A suitable value of RH is required in practical operation. Higher maximum voltage and minimum voltage of the cyclic load lead to more significant performance degradation.
• Low carbon loading and high platinum loading can increase initial performance and decrease VDR in general. However, their influence is weak when the carbon loading is smaller than 0.4 mg cm−2 and the platinum loading is higher than 0.2 mg cm−2.
• Degradation forecast of CL performance during startup–shutdown cycles and guidance for the optimal design of CL can also be achieved through this established computational framework.
The presented modeling framework offers guidelines for the next-generation design and development of a durable catalyst for automobiles with optimized performance and cost to meet the requirements of the ever-demanding industry applications of the future.
Conflicts of interest
There are no conflicts to declare.
Acknowledgements
This work was financially supported by The National Key Research and Development Program of China (2018YFB0105400).
Notes and references
- L. Lin, W. Zhou, R. Gao, S. Yao, X. Zhang, W. Xu, S. Zheng, Z. Jiang, Q. Yu, Y. W. Li, C. Shi, X. D. Wen and D. Ma, Nature, 2017, 544, 80 CrossRef CAS PubMed.
- O. H. Kim, Y. H. Cho, S. H. Kang, H. Y. Park, M. Kim, J. W. Lim, D. Y. Chung, M. J. Lee, H. Choe and Y. E. Sung, Nat. Commun., 2013, 4, 2473 CrossRef PubMed.
- B. Liu, Y. Jia, J. Li, S. Yin, C. Yuan, Z. Hu, L. Wang, Y. Li and J. Xu, J. Mater. Chem. A, 2018, 6, 21475 RSC.
- U. S. Department of Energy and U. S. DRIVE Fuel Cell Technical Team, Fuel Cell Technical Team Roadmap, 2017 Search PubMed.
- P. Pei, Q. Chang and T. Tang, Int. J. Hydrogen Energy, 2008, 33, 3829 CrossRef CAS.
- J. Speder, A. Zana, I. Spanos, J. J. K. Kirkensgaard, K. Mortensen, M. Hanzlik and M. Arenz, J. Power Sources, 2014, 261, 14 CrossRef CAS.
- L. Castanheira, W. O. Silva, F. H. B. Lima, A. Crisci, L. Dubau and F. Maillard, ACS Catal., 2015, 5, 2184 CrossRef CAS.
- S. Ghosh, H. Ohashi, H. Tabata, Y. Hashimasa and T. Yamaguchi, J. Power Sources, 2017, 362, 291 CrossRef CAS.
- N. Macauley, D. D. Papadias, J. Fairweather, D. Spernjak, D. Langlois, R. Ahluwalia, K. L. More, R. Mukundan and R. L. Borup, J. Electrochem. Soc., 2018, 165, F3148 CrossRef CAS.
- T. Tamaki, H. Wang, N. Oka, I. Honma, S.-H. Yoon and T. Yamaguchi, Int. J. Hydrogen Energy, 2018, 43, 6406 CrossRef CAS.
- J. H. Park, S. M. Hwang, G. G. Park, S. H. Park, E. D. Park and S. D. Yim, Electrochim. Acta, 2017, 260, 674 CrossRef.
- Z. Hu, L. Xu, Y. Huang, J. Li, M. Ouyang, X. Du and H. Jiang, Appl. Energy, 2018, 212, 1321 CrossRef CAS.
- M. Chen, S. Hwang, J. Li, S. Karakalos, K. Chen, Y. He, S. Mukherjee, D. Su and G. Wu, Nanoscale, 2018, 10, 17318 RSC.
- N. Takeuchi and T. F. Fuller, J. Electrochem. Soc., 2010, 157, B135 CrossRef CAS.
- J. P. Meyers and R. M. Darling, J. Electrochem. Soc., 2006, 153, A1432 CrossRef CAS.
- J. Chen, J. Hu and J. R. Waldecker, J. Electrochem. Soc., 2015, 162, F878 CrossRef CAS.
- J. Hu, P. C. Sui, S. Kumar and N. Djilali, Electrochim. Acta, 2009, 54, 5583 CrossRef CAS.
- A. A. Kulikovsky, J. Electrochem. Soc., 2011, 158, B957 CrossRef CAS.
- C.-Y. Jung, W.-J. Kim, C.-S. Yoon, D.-H. Kim and S.-C. Yi, Int. J. Energy Res., 2013, 37, 1981 CAS.
- K. G. Gallagher and T. F. Fuller, Phys. Chem. Chem. Phys., 2009, 11, 11557 RSC.
- M. Moein-Jahromi and M. J. Kermani, Int. J. Hydrogen Energy, 2012, 37, 17954 CrossRef CAS.
- N. Khajeh-Hosseini-Dalasm, M. J. Kermani, D. G. Moghaddam and J. M. Stockie, Int. J. Hydrogen Energy, 2010, 35, 2417 CrossRef CAS.
- X. Zhang, Y. Gao, H. Ostadi, K. Jiang and R. Chen, J. Power Sources, 2015, 289, 114 CrossRef CAS.
- X. Zhang and Y. Gao, J. Power Sources, 2016, 318, 251 CrossRef CAS.
- M. Ahadi, A. Putz, J. Stumper and M. Bahrami, J. Power Sources, 2017, 354, 215 CrossRef CAS.
- M. S. Ismail, D. B. Ingham, L. Ma, K. J. Hughes and M. Pourkashanian, Energy, 2017, 122, 420 CrossRef CAS.
- K. S. Walton and R. Q. Snurr, J. Am. Chem. Soc., 2007, 129, 8552–8556 CrossRef CAS PubMed.
- F. Barbir, PEM fuel cells: theory and practice, Elsevier Academic Press, University of California, 2005 Search PubMed.
- W. Sun, B. A. Peppley and K. Karan, Electrochim. Acta, 2005, 50, 3359 CrossRef CAS.
- Y. Shao-Horn, W. C. Sheng, S. Chen, P. J. Ferreira, E. F. Holby and D. Morgan, Top. Catal., 2007, 46, 285 CrossRef CAS.
- A. Rowe and X. Li, J. Power Sources, 2001, 102, 82 CrossRef CAS.
- M. Khakbaz Baboli and M. J. Kermani, Electrochim. Acta, 2008, 53, 7644 CrossRef CAS.
- A. V. Virkar and Y. Zhou, J. Electrochem. Soc., 2007, 154, B540 CrossRef CAS.
- A. Baldan, J. Mater. Sci., 2002, 37, 2171 CrossRef CAS.
- M. Moein-Jahromi, M. J. Kermani and S. Movahed, J. Power Sources, 2017, 359, 611 CrossRef CAS.
- S. R. Dhanushkodi, S. Kundu, M. W. Fowler and M. D. Pritzker, J. Power Sources, 2014, 267, 171 CrossRef CAS.
- G. Lee, H. Choi and Y. Tak, Nanotechnology, 2019, 30, 085402 CrossRef CAS PubMed.
- A. Ohma, K. Shinohara, A. Iiyama, T. Yoshida and A. Daimaru, J. Electrochem. Soc., 2011, 41, 775 CAS.
- Y.-H. Cho, H.-S. Park, Y.-H. Cho, D.-S. Jung, H.-Y. Park and Y.-E. Sung, J. Power Sources, 2007, 172, 89 CrossRef CAS.
- M. Srinivasarao, D. Bhattacharyya, R. Rengaswamy and S. Narasimhan, J. Power Sources, 2010, 195, 6782 CrossRef CAS.
- A. P. Young, J. Stumper and E. Gyenge, J. Electrochem. Soc., 2009, 156, B913 CrossRef CAS.
- J. Thepkaew, A. Therdthianwong and S. Therdthianwong, Energy, 2008, 33, 1794 CrossRef CAS.
- C.-Y. Liu and C.-C. Sung, J. Power Sources, 2012, 220, 348 CrossRef CAS.
- T. Suzuki, S. Tsushima and S. Hirai, Int. J. Hydrogen Energy, 2011, 36, 12361 CrossRef CAS.
- T. Suzuki, S. Tsushima and S. Hirai, J. Power Sources, 2013, 233, 269 CrossRef CAS.
Footnote |
† Electronic supplementary information (ESI) available. See DOI: 10.1039/c9ra09572a |
|
This journal is © The Royal Society of Chemistry 2020 |
Click here to see how this site uses Cookies. View our privacy policy here.