DOI:
10.1039/D0RA00722F
(Paper)
RSC Adv., 2020,
10, 25446-25455
Novel huperzine A based NMDA antagonists: insights from molecular docking, ADME/T and molecular dynamics simulation studies †
Received
23rd January 2020
, Accepted 7th May 2020
First published on 6th July 2020
Abstract
Huperzine A (HupA) is an alkaloidal natural product and drug isolated from Chinese herb Huperzia serrata, which is a potent selective anticholinesterase inhibitor. HupA has symptomatic, cognitive-enhancing and protective effect on neurons against amyloid beta-induced oxidative injury and antagonizing N-methyl-D-aspartate receptors by blocking the ion channels. The present study aimed to identify the docking, ADME/T and molecular dynamics simulation parameters of a library of 40 analogues which can correlate the binding affinity, conformational stability and selectivity of the ligands towards NMDA receptor through in silico approach. Glide molecular docking analysis was performed for the designed analogues to understand the binding mode and interactions. MD simulations were performed to explain the conformational stability and natural dynamics of the interaction in physiological environmental condition of protein–ligand complex affording a better understanding of chemical-scale interactions between HupA and its analogues with NMDA channel that could potentially benefit the development of new drugs for neurodegenerative diseases involving NMDA receptors.
1. Introduction
Alzheimer's disease (AD) is predicted to reach epidemic proportions, with an enormous human and economic burden, by the year 2050. Alzheimer's is a type of dementia that causes problems with memory, thinking and behavior and other cognitive abilities that interfere with daily life.1,2 Thus effective therapies that prevent AD or slow its progression or treat its cognitive and behavioral symptoms are urgently needed to avert this public health crisis.3,4 Several advances have been made recently to understand the neurobiology of AD. The major known cause for progression of disease is oligomerization of amyloid beta (Aβ) which is formed from amyloid precursor protein (APP) by the action of beta secretase and gamma secretase.5,6 An increased production of Aβ oligomers leads to synaptic depression and a reduced capacity for synaptic plasticity.7–9 Several studies have shown that a blockade of N-methyl-D-aspartate receptors (NMDARs) can mitigate the effects of Aβ on synapses.10–12 NMDARs are involved in learning and memory function in the CNS and play a major role in the synaptic plasticity.13–15 NMDARs can both promote neuronal protection and kill neurons depending on its site of location. Specifically NMDARs which are present in synaptic region promote neuronal protection. In contrast, activation of NMDARs which are localized at extrasynaptic region produces neuronal death.
NMDARs have been identified into different subtypes such as GluN1, GluN2A, GluN2B, GluN2C, GluN2D, GluN3A and GluN3B. NMDARs are hetero-tetramers associated with two GluN1 and two GluN2 subunits.16 GluN1 is gated by glycine, whereas GluN2 is gated by glutamate. Recent results indicate that Aβ oligomers increase the GluN2A to GluN2B ratio by selectively reducing the synaptic currents of GluN2B-containing NMDARs. Such a GluN2B to GluN2A switch also requires an alternative NMDAR function that involves glutamate-binding to GluN2B leading to conformational changes of the GluN2B-NMDAR, but does not involve ion flux through the NMDAR.17 This ion flux-independent glutamate binding to GluN2B leads to synaptic loss and an ultimate neuronal death. Hence selective GluN2B antagonists can be designed to target the amyloid beta induced dysfunction.18
NMDA receptor antagonists play a significant role for the treatment AD disease. Huperzine A (HupA) is an alkaloidal natural product isolated from chinese herb Huperzia serrata, which is a potent selective anticholinesterase inhibitor with antioxidant and neuroprotective properties. The availability of HupA from natural sources is scarce and trivial modifications on HupA, thus far, have led to less effective analogues. State Food and Drug Administration of China approved huperzine for Alzheimer's treatment in 1994. Earlier reports demonstrate that HupA is an inhibitor of NMDA receptors as well, with good efficacy in enriching memory in various animal models.19,20 HupA is proved to be a non-competitive NMDA antagonist, which inhibits the NMDA-induced toxicity by blocking ion channels of NMDA receptor through binding at MK-801 binding site.21 The scarcity of HupA from natural sources induced several groups to develop synthetic routes to this compound and its numerous analogues as cholinesterase inhibitors. The reported structures represent rather trivial and obvious modifications to the parent structure and were found to be either less effective than HupA by the in silico or in vitro studies.22–25 We surmised that more “creative” alterations of HupA will provide more active analogues, and thus we have designed some novel analogues based on the structure of the key intermediate for HupA serving as a starting point, in the present study.26–29 As per these previous reports, the key intermediate for HupA, ‘K’ could be obtained by asymmetric palladium catalyzed bicycloannulation of a β-keto ester. Thus we have designed the analogues I–X and NM (1–30) with subtle manipulation of the functionalities on the key intermediate K with a future focus to possibly obtain the analogues by simple chemical transformations of K (Scheme 1). In consequence, our present investigations are directed towards the design and in silico studies of the designed analogues to explore the ligand–enzyme interactions (Scheme 1).
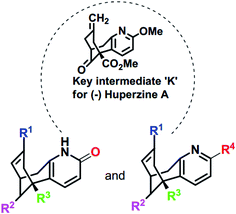 |
| Scheme 1 Skeletal structures of the molecules designed based on K [I–X and NM (1–30)]. The structures of designed analogues of K are furnished in ESI Fig. S4.† | |
2. Materials and methods
2.1 Rationale of work
To the best of our knowledge, molecular modeling studies were not explored either on HupA or its analogues as NMDA receptor antagonists. Recently, Song X. et al. solved the crystal structure of heterotetrameric GluN1/GluN2B NMDA receptor which proved the mechanistic inhibition of NMDA receptor by MK-801 via ion channel blocking.30 This gave an encouraging start to our computational exploration in identifying some of the synthetically possible HupA analogues as NMDA receptor antagonists. In the present work, we have designed novel compounds with basic skeleton cycloocta[b]pyridine structural framework of the HupA. Fig. 1 demonstrates the schematic version of the present work. The demands of this endeavor of designing and discovering efficient NMDA receptor antagonists were met by structural variations derived from the biologically viable functionalities that were visualized and carefully incorporated on the structure of the key intermediate K. Molecular docking simulation studies were conducted to examine the detailed protein–ligand interactions between the active site of NMDA receptor and the designed molecules. ADME/T prediction studies enabled us understand the drug-likeliness of the designed molecules, while molecular dynamics simulation studies explained the natural dynamics of interaction in physiological conditions, of the top potential hits among the designed molecules as NMDA receptor antagonist.
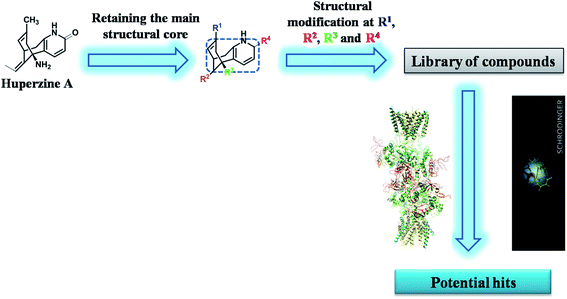 |
| Fig. 1 Schematic representation of the research protocol in the present study. | |
2.2 Computational methods
2.2.1 Selection of target protein. To the best of our knowledge, the crystal structure for GluN1/GluN2B NMDA receptor of humans with good resolution has not been reported yet. The crystallographic structures of Xenopus laevis GluN1/GluN2B NMDA receptor (PDB ID 5UN1) were selected for further studies. The protein sequence of Homo sapiens GluN1 (Uniprot, Entry Id: Q05586) and GluN2B (Uniprot, Entry Id: Q13224) was downloaded from the Universal Protein Resource. Further, targeted heterotetrameric GluN1/GluN2B NMDA protein search was performed using Blast Search tools against the PDB databank. Structure prediction wizard tool of Maestro was used for comparative analysis of Homo sapiens and Xenopus laevis NMDA receptor protein sequence.31 Fig. S1 and S2 (ESI)† demonstrate 92.1% and 82.6% identity in the protein sequence alignment of GluN1 and GluN2B from Homo sapiens with those from Xenopus laevis, respectively.
2.2.2 Protein structure preparation. The coordinates for the NMDA receptor were downloaded from the RCSB protein data bank (PDB ID 5UN1). The protein structure was processed using the Protein Preparation Wizard (PPW) and its integrity was checked and adjusted by adding the missing residues by employing Prime module of Schrödinger suite.32,33 The water molecules and all other heteroatoms were removed from the protein crystal structure. The H-bond network was optimized and the overlapping hydrogens were fixed under the refine tab of PPW. The most likely positions of thiol and hydroxyl hydrogen atoms, protonation states and tautomers of various amino acid residues were selected by the protein assignment script shipped by Schrodinger. The pH range was set to 7.0 and the protein was minimized by applying OPLS_2005 force field. Finally, restrained minimization was performed until the average root mean square deviation (RMSD) of the non-hydrogen atoms converged to 0.30 Å.
2.2.3 Identification of binding sites on NMDA receptor using SiteMap analysis. The prepared NMDA receptor was further subjected to SiteMap computer program to identify their potential binding sites. SiteMap combines a novel and highly effective algorithm for rapid binding site identification for various proteins.34,35 Table S1 (ESI)† depicts the SiteMap analysis results for top most potential binding sites of NMDA receptor. Site-I of NMDA receptor was found to possess the top most Site score (ligand binding ability) and D score (druggability). The size and volume of Site-I binding site is admirable. Hydrophobic (hydrophobic character of the binding site) score of Site-I is convincingly excellent. Fig. S3 (ESI)† illustrates the identified potential binding site (Site-I) on NMDA receptor. Site-I also covers the specific active site residues present at MK-801 binding site. Visualization and characterization of the catalytic binding site was done using the SiteMap module of Maestro 11.1. Further, preparation of the receptor grid for target protein was done utilizing OPLS_2005 force field. The grid center was set to be the centroid of the active site, and the size of the cubic grid was fixed at 20 Å.
2.2.4 Ligand preparation. A total of 40 molecules were built on Maestro Molecule Builder of Schrödinger and optimized using OPLS_2005 force field in LigPrep module of Schrödinger software 2017-1.36 All the possible protomers and ionization states were enumerated for ligands at a pH of 7.4 using Ionizer. The tautomeric states were generated for chemical groups with possible prototropic tautomerism.
2.2.5 Molecular docking. Molecular docking studies were performed by using GLIDE (Grid-based Ligand Docking with Energetics) docking module of Schrödinger suite, wherein, the prepared ligands were docked into the generated receptor grid using Glide SP docking precision.37,38 It uses a grid-based ligand docking method with energetic, that searches for favorable interactions between a ligand and a receptor molecule like protein. Van der Waals scaling factor and partial charge cutoff was selected to be 0.80 and 0.15, respectively for ligand atoms. The interactions of each complex were analyzed and the 3D poses demonstrating the molecular recognition interactions were obtained using Schrödinger suite 2017-1 and PyMOL v0.99.39
2.2.6 In silico physico-chemical and ADME/T studies. Physico-chemical and ADME/T properties, which help in predicting both physico-chemical significant descriptors and pharmacokinetically important properties of the molecules, were calculated using Qikprop module of Schrödinger suite 2017-1.40 QikProp enumerates the comparative ranges of a molecule's properties with those of known drugs. Major parameters of the designed molecules that enabled us to quickly evaluate the physico-chemical and ADME/T properties of designed HupA analogues are discussed in Section 3.2.
2.2.7 Molecular dynamics. In order to understand the dynamic interaction mechanism between the receptor and the ligand, we have performed molecular dynamics simulation study using Desmond module of Schrödinger suite 2017-1.41 Aqueous biological system was built by using OPLS_2005 force field and TIP3P model was used to stimulate the water molecules. Orthorhombic periodic boundary conditions were set up to specify the shape and size of the repeating unit buffered at 10 Å distances. Boundary conditions box volume was initially calculated as 2
223
364 Å3 (for 5UN1–HupA complex); 2
223
334 Å3 (for 5UN1–MK-801 complex); 2
223
382 Å3 (for 5UN1–NM28 complex) and then minimized to 2
136
731 Å3, 2
136
683 Å3 and 2
136
758 Å3 respectively. Required number of sodium and chloride ions was added to neutralize the system and osmotic effect of water was maintained by adding 0.15 mol L−1 sodium and chloride ions. After building the solvated system containing protein in complex with the ligand, the system was minimized and relaxed using default protocol integrated within Desmond module using OPLS_2005 force field parameters. 300 K temperature and 1 atmospheric pressure were maintained by using Nose–Hoover temperature coupling and isotropic scaling, respectively. Reversible reference system propagation algorithm (REPSA), a time stepping algorithm was used for far non-bonded, near non-bonded and bonded interactions (6, 2 and 2 fs, respectively) and finally, the molecular dynamics simulations (100 ns) were performed by using Desmond module of Schrödinger suite.
3. Results and discussion
3.1 Molecular docking study
Molecular docking studies were performed to elucidate the binding mode and type of interactions for designed HupA analogues at the active site of NMDA receptor (PDB ID 5UN1) using GLIDE docking module of Schrödinger suite 2017-1. To validate the docking protocol, the bound ligand (MK-801) was extracted from the prepared protein structure and was re-docked, after which the RMSD was determined. After validation of the docking protocol, the HupA analogues were docked into the active site of NMDA receptor.
This molecular docking simulation study suggested that the top ranked conformations of HupA analogues were well lodged at the active binding site of NMDA receptor. Table 1 showed the results of docking along with the major interactions for some of the designed HupA analogues with NMDA receptor with scores above −7.4, which is higher than that of the co-crystal. Interestingly, out of all the designed HupA analogues, NM28 was found to possess higher interaction energy (−7.830 kcal mol−1) than the co-crystalized ligand and HupA. Fig. 2 shows binding pose and detailed protein interaction of NM28 within the active site of NMDA receptor. Several hydrophobic interactions observed between the NM28 and the active site residues, such as Val634(A), Phe597(B), Val623(B), Leu626(B), Val634(C), Val623(D), Leu626(D) and Ala627(D) stabilize the binding of the NM28 in the binding site of NMDA receptor. Further, compounds NM14, NM16, NM20 and NM22 showed good interaction energy with target NMDA receptor i.e. −7.475 kcal mol−1, −7.542 kcal mol−1, −7.722 kcal mol−1, and −7.558 kcal mol−1, respectively. Fig. 3 demonstrates the docking model of compounds NM14, NM16, NM20 and NM22 at the binding site of NMDA receptor. Superimposition of native ligand, HupA and best docked pose of NM28 at the active pocket of NMDA receptor is depicted in Fig. 4, which reveals that NM28 and HupA share a common binding pattern with MK-801. Pyridin-2-one ring of the NM28 and HupA overlap with one of the benzene ring of iminodibenzo annulene of MK-801. The docking analysis, revealed good hydrophobic contacts of designed HupA analogues with all the four chains of heterotetrameric GluN1/GluN2B NMDA receptor, which may be responsible for the selective blockade of ion channel. Thus, the molecular docking results, allow us to believe that some of the HupA analogues have potential to inhibit NMDA receptor through an action on binding site.
Table 1 GLIDE docking results for some of the designed HupA analogues at the active site of NMDA receptor (PDB ID 5UN1)
S. no. |
Ligand name |
Ligand structure |
Docking score |
Hydrophobic interactions |
1 |
Huperzine A |
 |
−6.970 |
Met631(A), Val634(A), Ala635(A), Phe597(B), Leu626(B), Ala627(B), Tyr629(B), Val634(C), Ala635(C), Ala622(D), Val623(D), Leu626(D), Ala627(D) |
2 |
NM14 |
 |
−7.475 |
Met631(A), Val634(A), Ala635(A), Phe597(B), Val623(B), Leu626(B), Ala627(B), Tyr629(B), Val634(C), Val623(D), Leu626(D), Ala627(D) |
3 |
NM16 |
 |
−7.542 |
Met631(A), Val634(A), Ala635(A), Leu626(B), Ala627(B), Tyr629(B), Val634(C), Ala635(C), Val623(D), Leu626(D), Ala627(D), Ala631(D) |
4 |
NM20 |
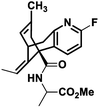 |
−7.722 |
Met631(A), Val634(A), Ala635(A), Val623(B), Leu626(B), Ala627(B), Tyr629(B), Val634(C), Ala622(D), Val623(D), Leu626(D), Ala627(D) |
5 |
NM22 |
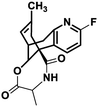 |
−7.558 |
Met631(A), Val634(A), Ala635(A), Val623(B), Leu626(B), Ala627(B), Val634(C), Ala635(C), Val623(D), Leu626(D), Ala627(D) |
6 |
NM28 |
 |
−7.830 |
Val634(A), Phe597(B), Val623(B), Leu626(B), Val634(C), Val623(D), Leu626(D), Ala627(D) |
7 |
MK-801 |
 |
−7.365 |
Met631(A), Val634(A), Ala635(A), Leu626(B), Ala627(B), Tyr629(B), Val623(D), Leu626(D), Ala627(D) |
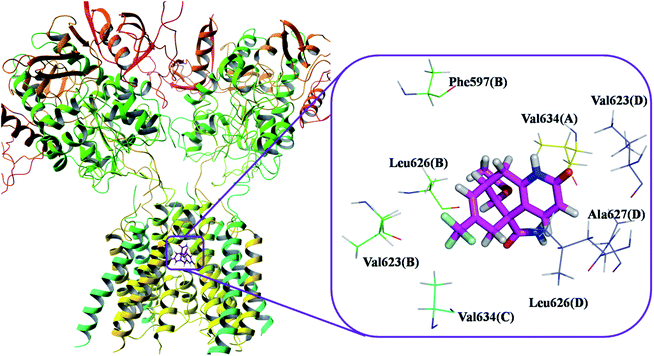 |
| Fig. 2 Binding pose and detailed protein interaction of the most potent NM28 (magenta colour stick) with NMDA receptor. All the amino residues represent hydrophobic interactions. | |
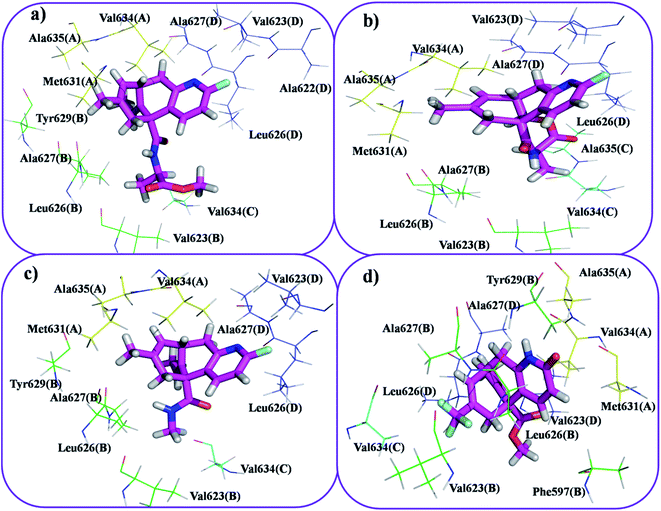 |
| Fig. 3 Docking model of compound NM20 (a), NM22 (b), NM16 (c) and NM14 (d) with NMDA receptor. All the amino residues represent hydrophobic interactions. | |
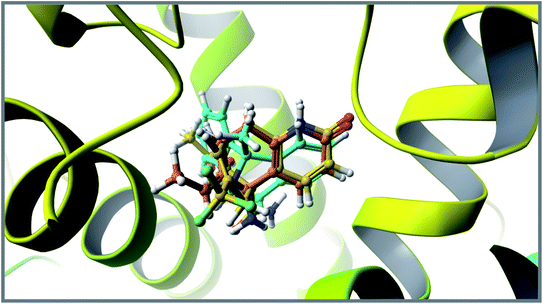 |
| Fig. 4 Superimposition of co-crystallized ligand (turquoise), HupA (orange) and best docked pose of NM28 (yellow) at the active site of NMDA receptor. | |
3.2 In silico ADME/Tstudies
The drug likeliness of the designed HupA analogues was evaluated in terms of the physicochemically important descriptors and key pharmacokinetic properties through the QikProp program of Schrödinger software. The lead molecules obtained from docking study (Fig. 5) were subjected for QikProp ADME/T profiling. Some of the computed ADME/T parameters are shown in Table 2. From this in silico study, we can compare the ADME/T properties of the known NMDA receptor channel blockers and our designed molecules.
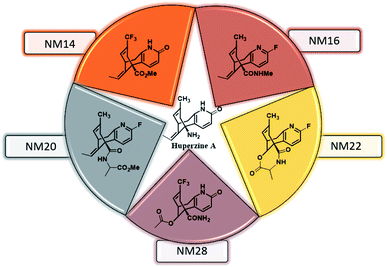 |
| Fig. 5 Structures of the lead molecules. | |
Table 2 Physico-chemical properties and ADME/T profile of designed HupA analoguesa
Compound name |
Parameters |
Rule of five |
PSA |
QP log Po/w |
QPpolrz |
QP log BB |
QP log Kp |
POA |
PSA = polar surface area, QP log Po/w = predicted octanol/water partition coefficient, QPpolrz = predicted polarizability, QP log BB = predicted brain/blood partition coefficient, QP log Kp = predicted skin permeability, POA = predicted human oral absorption. |
NM14 |
No violation |
71.606 |
3.384 |
34.117 |
−0.343 |
−3.340 |
100.000 |
NM16 |
No violation |
43.172 |
3.184 |
32.668 |
0.184 |
−1.947 |
100.000 |
NM20 |
No violation |
73.086 |
3.861 |
39.674 |
−0.207 |
−2.230 |
100.000 |
NM22 |
No violation |
81.467 |
1.469 |
33.519 |
−0.463 |
−3.421 |
81.681 |
NM28 |
No violation |
123.117 |
0.857 |
32.679 |
−1.123 |
−4.762 |
64.640 |
Huperzine A |
No violation |
63.754 |
1.466 |
27.760 |
−0.156 |
−5.346 |
75.744 |
MK-801 |
No violation |
15.003 |
3.310 |
27.642 |
0.770 |
−2.947 |
100.000 |
The lead molecules of HupA based designed library showed significant values for the properties analyzed and on the basis of physico-chemical properties, they exhibited drug-like characteristics. ADME/T prediction studies depicts that the designed HupA analogues conform to the Lipinski's rule of five. They have appropriate log
P values for desired biological efficacy and showed no violation in the recommended ranges of physico-chemical and ADME/T parameters. The other associated factors, such as blood–brain permeability and percent human oral absorption are also within the acceptable range defined for human use. Interestingly from this study we have observed compound NM28 to possess highest polar surface area amongst the designed HupA analogues, indicating the drug likeness of the designed HupA analogues.
3.3 Molecular dynamics simulation
From the molecular docking analysis, the best docked complexes were extracted to explore the conformational stability and time dependent performance of the ligands at the active pocket of NMDA receptor. Molecular docking predicts the spatial orientation of a ligand in the active pocket of the receptor. Subsequently, in addition to the fit of the binding pocket, other factors such as binding affinity and conformational stability should be taken into consideration for the development of compounds targeting NMDA receptor in the treatment of Alzheimer's disease. Conformational stability is crucially important for potent inhibition of NMDA receptors and an MD study provides conformational landscape of ligand–protein complexes at given temperature. The molecular dynamics simulation (MD) were performed for protein–ligand complex of compound NM28, MK-801 and HupA using Desmond module of Schrödinger suite 2017-1.
Molecular dynamics trajectory was used to examine the equilibration of dynamics over period of time. An insight about the convergences of simulated protein–ligand complexes can be obtained by taking into consideration the root-mean-square deviations (RMSD) of initial structure and average simulated structure of all the frames in MD trajectory. Configuration RMSD of all the selected complexes with respect to their initial structures were found to increase and then converge after 20 ns during equilibration phase. Initially, RMSD of the C-alphas, heavy atoms, backbone and side chains during MD simulation of NM28–5UN1 complex shows little fluctuations, but during course of simulation it attains constant RMSD value. In the beginning, RMSD values of C-alphas, heavy atoms, backbone and side chains are 4.23, 4.24, 4.23 and 4.50 Å, respectively. After MD simulation for 100 ns, we observed average RMSD values of C-alphas (Fig. 6), heavy atoms, backbone and side chains as 9.15, 8.96, 9.12 and 8.98 Å, respectively. NM28 has RMSD of 3.00 Å at the starting and after simulation it reached to constant value of 6.65 Å. Protein–ligand interactions of all the complexes have been examined during the course of MD simulation. Fig. 7 demonstrates the type of protein–ligand contacts exhibited by all the complexes employed in MD simulation. Mainly, there are three types of protein–ligand interactions: H-bonds, hydrophobic and water bridges. During the course of the MD simulation, H-bond interactions with residues Asn606(A), Thr638(A), Asn598(B) and Thr630(D) were observed, among which more contribution for H-bond interaction fraction was shown by Asn598(B) and Thr630(D). NM28 has good number of hydrophobic interactions with the active site residues Val634(A), Ala635(A), Leu626(B) and further strong interaction with Leu626(D) during MD simulation. In the results obtained from the MD studies, it was found that the carbonyl oxygen and nitrogen atoms belonging to the amidic groups of the lead analogue NM28, showed strong hydrogen bonding with Thr630(D) and Thr638(A) amino acid residues respectively. Similarly, nitrogen and carbonyl oxygen atoms of cycloocta[b]pyridine moiety of NM28 established H-bonds with Asn598(B) and Asn606(A) amino acid residues of the NMDA protein. These interactions strengthen the binding of the molecule at the active binding site, suggesting their potential NMDA inhibitory capability. Fig. 8–10 illustrates timeline representation of ligand–protein contacts including both H-bond and hydrophobic interactions for compound NM28, MK-801 and HupA with protein during 100 ns MD simulation period. Further, ligand–protein interactions of MK-801–5UN1 and HupA–5UN1 complexes were also analyzed during time period of MD simulation (100 ns). MK-801 has shown hydrophobic interactions with the residues Met631(A), Val634(A), Val634(C), Val623(D), Leu626(D) and hydrogen bond interaction with Phe597(B), Leu626(B). HupA has hydrophobic interactions with Val634(A), Leu626(B), Val623(D), Leu626(D) and hydrogen bond interactions with Asn598(B), Asn599(B) and Leu626(B). In addition, MD studies were also performed on NM14, NM16, NM20 and NM22 to understand their conformational stability at the active pocket of NMDA receptor. Fig. S6 and S7 (ESI)† demonstrate the RMSD values and type of protein–ligand contacts exhibited NM14, NM16, NM20 and NM22 with NMDA receptor during 100 ns MD simulation studies. Overall from this MD simulation study, we observed that the compound NM28 has little fluctuations in the beginning and is more stable at the active site of 5UN1 during course of MD simulation. NM28 further shows number of favorable contacts with the active site residues that might enhance its binding into the NMDA receptor.
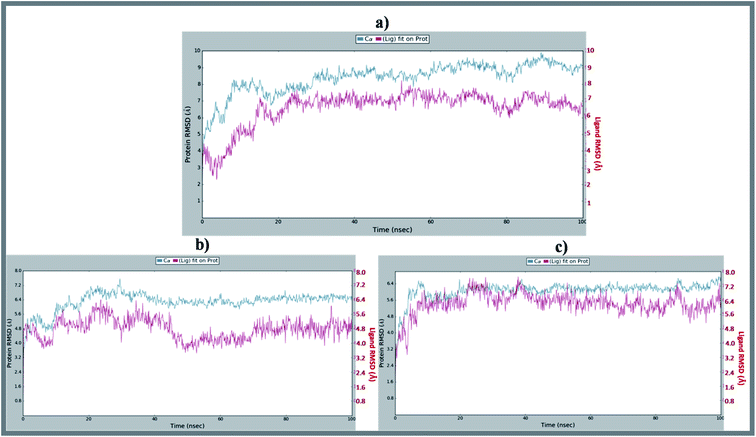 |
| Fig. 6 Average RMSD of C-alphas of protein and ligand complexes (a) NM28–5UN1, (b) MK-801–5UN1 and (c) HupA–5UN1 during molecular dynamics simulation (100 ns). | |
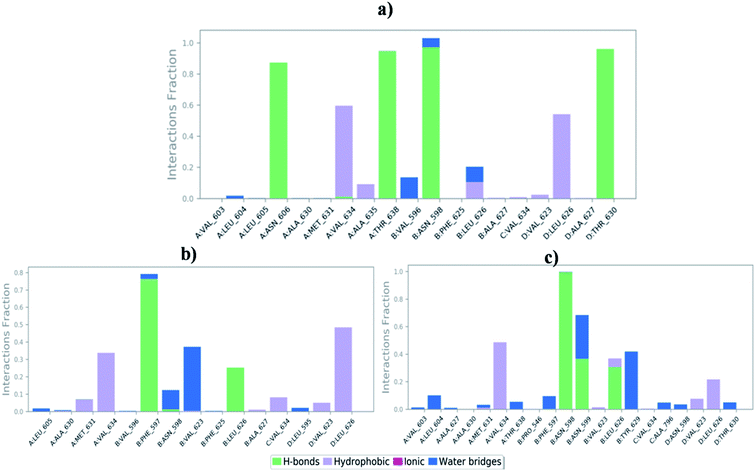 |
| Fig. 7 Bar-chart representation of protein–ligand contacts of (a) NM28–5UN1 complex, (b) MK-801–5UN1 complex and (c) HupA–5UN1 complex during molecular dynamics simulation. | |
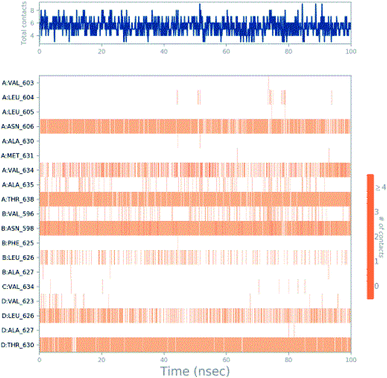 |
| Fig. 8 Timeline representation of ligand–protein contacts for compound NM28–5UN1 complex observed during molecular dynamics simulation. | |
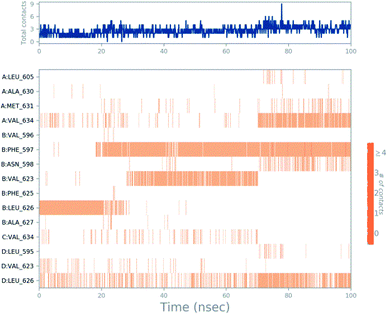 |
| Fig. 9 Timeline representation of ligand–protein contacts for compound MK-801–5UN1 complex observed during molecular dynamics simulation. | |
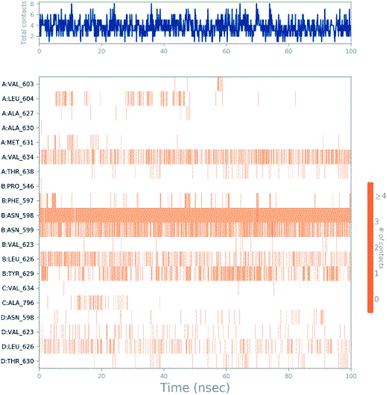 |
| Fig. 10 Timeline representation of ligand–protein contacts for compound HupA–5UN1 complex observed during molecular dynamics simulation. | |
3.3.1 Simulation quality analysis. Further, simulation quality analysis tool of Desmond module was used to analyze the thermodynamic properties which includes energy values with standard deviation was depicted in Tables S3–S5 (ESI).† The tables display valuable information about MD simulation parameters and show the statistical properties of basic thermodynamic quantities. From this analysis, we understood that during the course of MD simulation study, standard deviation become its minimum, it indicates that simulation has equilibrated and its fluctuations was stabilized.
4. Conclusions
The present study marks the beginning of our efforts to obtain more potential NMDA receptor antagonists by exploring the structural behavior and binding affinities of novel HupA analogues by molecular docking, ADME/T and molecular dynamics simulation studies for virtual identification of NMDA receptor antagonists. While NM28 displayed the highest molecular docking score, NM14, NM16, NM20, NM22 along with NM28 showed better correlation in terms of binding affinities and interactions. Comparative MD analysis with time period of 100 ns on these lead molecules very well explains the conformational stability and natural dynamics of the interaction in physiological environmental condition. ADME/T prediction on the lead molecules enabled us to analyze their physicochemical and pharmacokinetic profiles. The obtained results will aid in the launch of new research protocols for discovery of potential NMDA receptor antagonists in the treatment of Alzheimer's disease.
Conflicts of interest
There are no conflicts to declare.
Acknowledgements
Authors are thankful to NIPER Hyderabad for facilities and Vinod Devaraji, Schrodinger, Bengaluru for molecular dynamics studies. Authors are also thankful to Dr Sreedhara Voleti, CEO, BioNest, UoH for timely help and advice. DKS thanks Department of Pharmaceuticals (DoP), Govt. of India, New Delhi, for the award of research fellowship.
References
- H. Hsieh, J. Boehm, C. Sato, T. Iwatsubo, T. Tomita, S. Sisodia and R. Malinow, Neuron, 2006, 52, 831–843 CrossRef CAS PubMed.
- F. Kamenetz, T. Tomita, H. Hsieh, G. Seabrook, D. Borchelt, T. Iwatsubo, S. Sisodia and R. Malinow, Neuron, 2003, 37, 925–937 CrossRef CAS PubMed.
- P. N. Lacor, M. C. Buniel, P. W. Furlow, A. S. Clemente, P. T. Velasco, M. Wood, K. L. Viola and W. L. Klein, J. Neurosci., 2007, 27, 796–807 CrossRef CAS PubMed.
- M. P. Lambert, A. K. Barlow, B. A. Chromy, C. Edwards, R. Freed, M. Liosatos, T. E. Morgan, I. Rozovsky, B. Trommer, K. L. Viola, P. Wals, C. Zhang, C. E. Finch, G. A. Krafft and W. L. Klein, Proc. Natl. Acad. Sci. U. S. A., 1998, 95, 6448–6453 CrossRef CAS PubMed.
- G. M. Shankar, B. L. Bloodgood, M. Townsend, D. M. Walsh, D. J. Selkoe and B. L. Sabatini, J. Neurosci., 2007, 27, 2866–2875 CrossRef CAS PubMed.
- L. Texidó, M. Martín-Satué, E. Alberdi, C. Solsona and C. Matute, Cell Calcium, 2011, 49, 184–190 CrossRef PubMed.
- J. T. Ting, B. G. Kelley, T. J. Lambert, D. G. Cook and J. M. Sullivan, Proc. Natl. Acad. Sci. U. S. A., 2007, 104, 353–358 CrossRef CAS PubMed.
- D. M. Walsh, I. Klyubin, J. V. Fadeeva, W. K. Cullen, R. Anwyl, M. S. Wolfe, M. J. Rowan and D. J. Selkoe, Nature, 2002, 416, 535–539 CrossRef CAS PubMed.
- H. W. Wang, J. F. Pasternak, H. Kuo, H. Ristic, M. P. Lambert, B. Chromy, K. L. Viola, W. L. Klein, W. B. Stine, G. A. Krafft and B. L. Trommer, Brain Res., 2002, 924, 133–140 CrossRef CAS PubMed.
- N. W. Hu, I. Klyubin, R. Anwyl and M. J. Rowan, Proc. Natl. Acad. Sci. U. S. A., 2009, 106, 20504–20509 CrossRef CAS PubMed.
- S. Li, M. Jin, T. Koeglsperger, N. E. Shepardson, G. M. Shankar and D. J. Selkoe, J. Neurosci., 2011, 31, 6627–6638 CrossRef CAS PubMed.
- J. Liu, L. Chang, F. Roselli, O. F. Almeida, X. Gao, X. Wang, D. T. Yew and Y. Wu, J. Alzheimer's Dis., 2010, 22, 541–556 CAS.
- G. Rammes, A. Hasenjäger, K. Sroka-Saidi, J. M. Deussing and C. G. Parsons, Neuropharmacology, 2011, 60, 982–990 CrossRef CAS PubMed.
- R. Rönicke, M. Mikhaylova, S. Rönicke, J. Meinhardt, U. H. Schröder, M. Fändrich, G. Reiser, M. R. Kreutz and K. G. Reymann, Neurobiol. Aging, 2011, 32, 2219–2228 CrossRef PubMed.
- F. Roselli, P. Hutzler, Y. Wegerich, P. Livrea and O. F. Almeida, PLoS One, 2009, 4, e6011 CrossRef PubMed.
- P. Paoletti, C. Bellone and Q. Zhou, Nat. Rev. Neurosci., 2013, 14, 383–400 CrossRef CAS PubMed.
- A. Barria and R. Malinow, Neuron, 2002, 35, 345–353 CrossRef CAS PubMed.
- K. Erkan, S. Noriko and F. Hiro, EMBO J., 2009, 28, 3910–3920 CrossRef PubMed.
- P. K. Alan and T. Werner, Acc. Chem. Res., 1999, 32, 641–650 CrossRef.
- K. G. Richard, V. N. Savita, A. W. Julie, R. D. Jitendra, P. D. Bhupendra and S. V. Haresh, J. Appl. Toxicol., 2001, 21, S47–S51 CrossRef PubMed.
- H. Z. Yun, Y. Z. Xiao, Q. C. Xue, W. Yuan, H. Y. Hui and Y. H. Guo, Neurosci. Lett., 2002, 319, 107–110 CrossRef.
- H. Gunosewoyo, S. K. Tipparaju, M. Pieroni, Y. Wang, B. P. Doctor, M. P. Nambiar and A. P. Kozikowski, Bioorg. Med. Chem. Lett., 2013, 23, 1544–1547 CrossRef CAS PubMed.
- P. K. Alan, X. Yan, R. E. Rajarathnam, T. Werner, H. Israel and X. C. Tang, J. Org. Chem., 1991, 56, 4636–4645 CrossRef.
- C. Pelayo, M. Diego and S. Montserrat, Synth. Commun., 2001, 31, 3507–3516 CrossRef.
- A. K. Seán, F. Yann, M. John and M. B. Jonathan, Org. Biomol. Chem., 2003, 1, 2865–2876 RSC.
- S. Kaneko, T. Yoshino, T. Katoh and S. Terashima, Tetrahedron: Asymmetry, 1997, 8, 829–832 CrossRef CAS.
- S. Kaneko, T. Yoshino, T. Katoh and S. Terashima, Tetrahedron, 1998, 54, 5471–5484 CrossRef CAS.
- X. C. He, B. Wang and D. Bai, Tetrahedron Lett., 1998, 39, 411–414 CrossRef CAS.
- X. C. He, B. Wang, G. Yu and D. Bai, Tetrahedron: Asymmetry, 2001, 12, 3213–3216 CrossRef CAS.
- X. Song, M. O. Jensen, V. Jogini, R. A. Stein, C. H. Lee, H. S. Mchaourab, D. E. Shaw and E. Gouaux, Nature, 2018, 556, 515–519 CrossRef CAS PubMed.
- Maestro (Version 11.1), Schrödinger, LLC, New York, NY, 2017 Search PubMed.
- G. M. Sastry, M. Adzhigirey, T. Day, R. Annabhimoju and W. Sherman, J. Comput. Aided Mol. Des., 2013, 27, 221–234 CrossRef PubMed.
- Schrödinger Release 2017-1: Schrödinger Suite 2017, Protein Preparation Wizard; Epik (Version 3.9), Schrödinger, LLC, New York, NY, 2017 Search PubMed; Impact (Version 7.4), Schrödinger, LLC, New York, NY, 2017 Search PubMed.
- T. Halgren, Chem. Biol. Drug Des., 2007, 69, 146–148 CrossRef CAS PubMed.
- T. Halgren, J. Chem. Inf. Model., 2009, 49, 377–389 CrossRef CAS PubMed.
- LigPrep, Schrödinger, LLC, New York, NY, 2017 Search PubMed.
- R. A. Friesner, J. L. Banks, R. B. Murphy, T. A. Halgren, J. J. Klicic, D. T. Mainz, M. P. Repasky, E. H. Knoll, D. E. Shaw, M. Shelley, J. K. Perry, P. Francis and P. S. Shenkin, J. Med. Chem., 2004, 47, 1739–1749 CrossRef CAS PubMed.
- GLIDE (Version 7.4), Schrödinger, LLC, New York, NY, 2017 Search PubMed.
- W. L. DeLano, PyMOL, DeLano Scientific, San Carlos, CA, 700, 2002 Search PubMed.
- Qikprop (Version 5.1), Schrödinger, LLC, New York, NY, 2017 Search PubMed.
- Desmond (Version 4.9), Schrödinger, LLC, New York, NY, 2017 Search PubMed.
Footnote |
† Electronic supplementary information (ESI) available. See DOI: 10.1039/d0ra00722f |
|
This journal is © The Royal Society of Chemistry 2020 |
Click here to see how this site uses Cookies. View our privacy policy here.