DOI:
10.1039/D0RA03792C
(Paper)
RSC Adv., 2020,
10, 24463-24471
Development of an ultrasound-enhanced smartphone colorimetric biosensor for ultrasensitive hydrogen peroxide detection and its applications†
Received
27th April 2020
, Accepted 11th June 2020
First published on 26th June 2020
Abstract
In this work, we developed the first ultrasound technique enhanced smartphone application for highly sensitive determination of hydrogen peroxide (H2O2). The measurement technique is based on the change in color intensity due to the transformation of tetramethylbenzidine (TMB) to oxidized tetramethylbenzidine (oxTMB) by the oxidation process with hydroxyl radical (OH˙) from the oxidation etching of silver nanoparticles (AgNPs) and its ultrasound usability. The oxTMB product occurs without peroxidase and can be detected with a saturation channel using HSV methodology via the application of a smartphone. To prove the peroxidase mimic property, our proposed method was also validated by determination of certain biomolecules, including glucose, uric acid, acetylcholine and total cholesterol, of which the known amounts are a valuable diagnostic tool. The proposed method provided the lowest limits of detection (LOD) of 2.0, 5.0, 12.50, 7.50, and 10.0 nmol L−1 for H2O2, glucose, uric acid, acetylcholine, and cholesterol, respectively, when compared with LODs obtained from other smartphone colorimetric methods. Reproducibility was calculated from the detection of H2O2 at 25.0 and 50.0 nmol L−1 with the highest standard deviations of 3.47 and 4.58%, respectively. Additionally, the determination of all analytes in human urine samples indicated recoveries in the range of 96–104% with the highest relative standard deviation of 3.98%, offering high accuracy and precision. Our research shows the novel compatibility of basic technology and chemical methodology with green chemistry principles by reducing a high-power process and organic solvent as well as exhibiting good colorimetric performance and effective sensitivity and selectivity. Thus, our developed method can be applied for point-of-care medical diagnosis.
1. Introduction
Hydrogen peroxide (H2O2) is a redox compound which acts as both a powerful oxidant and reductant (E° = 1.80 V). Its properties have broadly applied in the biochemical, pharmaceutical, nutrition, clinical and textile industries. In addition, H2O2 plays an important role in organic and inorganic contaminant removal using the Fenton reaction during the water treatment process due to its strong formation of the hydroxyl radical (OH˙) (E° = 2.80 V), as well as its association with various synthesis processes.1 H2O2 is also a product of biological processes in the human body due to the oxidase enzymatic reaction of some biomolecules such as glucose, uric acid, cholesterol and acetylcholine, which are important compounds for medical care because their different types and quantities can indicate disease. Moreover, the influence of H2O2 can generate reactive oxygen species (ROS) that cause the pathogenesis of many diseases and effect heart failure and cardiac ischemia.2,3 Consequently, there is a major basis for the potential rapid and accurate measurement of trace H2O2 in biological samples for medical diagnosis. Considering all these facets, it is challenging to develop a novel method for the determination of trace H2O2 content because it lacks a chromogenic functional group, such as aromatic rings and nitro substituents. Currently, numerous studies have reported H2O2 detection by various techniques, such as titrimetric,4 spectrophotometric,5–7 fluorescence,8–10 chemiluminescence,11,12 chromatographic,13 electrochemical sensor14–17 and colorimetric methods.18,19 Although these techniques provide high sensitivity measurements, they require expensive instrumentation, the transmission of data from on-site to experts, long preparation and/or analysis time, large reagent consumption, and trained manpower. Hence, these methods are not useful for point-of-care monitoring. Among these analytical techniques, colorimetric method is convenient with good advantages, such as ease of measurement and low cost due to naked eye detection or evaluation of color intensity with simple devices. For this reason, a smartphone can perform as an essential colorimetric detector in research because of its cost efficiency and ease of use.20 Therefore, many studies have used smartphone applications for colorimetric assay of H2O2 and/or other biomolecules.21
Currently, nanomaterials are widely used to improve the characteristics of colorimetric biosensors due to their excellent optical properties and good peroxidase-like ability. Nonetheless, their capabilities depend on many factors, such as their preparation process, size, shape and type. Silver nanoparticles (AgNPs) are one of the most popular metallic nanoparticles for colorimetric measurement because of their easy synthesis method with low cost compared with other nanoparticles.22 Additionally, AgNPs have been used for H2O2 detection via localized surface plasmon resonance (LSPR);23 however, this method provided quite low sensitivity and selectivity as it was based on the existence of AgNPs. In order to increase the analytical performance, tetramethylbenzidine (TMB) can be used for colorimetric detection via their oxidation to produce the common chromogenic reagent oxidized tetramethylbenzidine (oxTMB). TMB has also been combined with many metallic nanoparticles to detect H2O2 and biomolecules as it shows a high-performance ability for determination of H2O2 and metallic nanoparticles show a peroxidase mimic property.24–26 These methods' lowest limits of detection for H2O2 and glucose were 20 nmol L−1 and 2.0 μmol L−1, respectively, but the method was a bi-enzymatic system and needed a long incubation time. Thus, it is essential to develop a new method to improve the sensitivity using a single enzymatic system which can be useful for medical diagnosis.
For the above purposes, we present a simple device-assisted colorimetric smartphone method combined with an ultrasound application for the detection of H2O2 and certain biomolecules to prove its applications using the peroxidase mimic property. Our proposed method is the first reported to use an ultrasound-enhanced H2O2, AgNPs and TMB colorimetric reaction. Ultrasound provides a highly sensitive oxidation process for H2O2 using AgNPs as a peroxidase mimic for trace H2O2 measurement. Ultrasound helps generate OH˙ due to the disintegration of H2O2 in accordance with the advanced oxidation processes (AOPs) principle.27–29 The quantitative detection is based on the blue colorimetric saturation of oxTMB relative to the changing substance concentration which is captured via an application on a smartphone and indicates good accuracy and precision. This approach is suitable for the determination of certain biomolecules which are useful for clinical diagnosis, with benefits including simplicity, rapidity, cost effectiveness, portability, ruggedness, low reagent consumption and ultrasensitive and selective point of care monitoring.
2. Material and methods
2.1 Chemicals and solutions
30% hydrogen peroxide (H2O2) was purchased from Merck. Tetramethylbenzidine (TMB) was purchased from Tokyo Chemical Industry CO., LTD. Glucose, uric acid, acetylcholine, cholesterol, glucose oxidase (Type II from Aspergillus niger), uricase (from Candida sp.), acetylcholinesterase (from Electrophorus electricus), choline oxidase (from Alcaligenes) and cholesterol oxidase (from microorganisms), as well as all chemicals and the interference agents used in experiments were analytical reagent (AR) grade and purchased from Sigma-Aldrich (USA). Surine™ negative urine control samples were obtained from Sigma-Aldrich (USA). Preparation of all solutions is described in the ESI.†
2.2 Synthesis of silver nanoparticles and characteristics
Silver nanoparticles (AgNPs) were synthesized according to a previously reported method.22 Briefly, 1% (w/v) starch solution was heated to boiling and left standing at room temperature for 1 day. Next, 0.3150 g of silver nitrate (AgNO3) and 0.1050 g of sodium borohydride (NaBH4) were dissolved in the starch solution and AgNO3 was rapidly mixed into the NaBH4 solution under homogenization at about 1000 rpm for 30 min. Then, the solution was heated at 100 °C to boiling before it was kept standing at room temperature for 12 hours. Next, 30% H2O2 was slowly added into the solution under homogenization at about 1000 rpm for 20 min. The solution changed from yellow color to pink. Finally, the stock solutions were kept standing for about 2 days before use to allow H2O2 decomposition. Their characteristics were evaluated and are listed in Fig. S1 (described in ESI†).
2.3 Fabrication device and apparatus
An appropriate 3D closed system device was designed and built from matte black acrylic material, dimensions 6.0 × 22 × 6.5 cm (width, length and height), with visible LED strips of 6.0 cm (white light, constant 2.4 Watt, WorldSemi) for the light controller. The internal background was white acrylic to avoid light absorption which affects the detection system. The detecting channel was focused through the chamber's circular cavity, as indicated in Fig. S2.† A cuvette with a capacity of 4.0 mL (quartz cell, series no. 06-907, Coax Group Corporation Ltd) was used in the experiment. For ultrasound generation, earphones were used as generator probes to transfer ultrasound waves from the frequency generator application (Hoel Boedec, version 3.7), which can generate sound frequencies up to 22 kHz, from a smartphone to the detection solution. Color Grab (Loomatix, version 3.6.1) was used for real-time color intensity detection through the camera of the smartphone system because this application can be easily downloaded and widely used. Evaluation of the analytical acquisition was studied to decide the suitable channels for detection of the blue color intensity of oxTMB. Hue (H, degree unit), saturation (S) and value (V, percentage unit) channels in the Color Grab application were separately evaluated by comparing the slopes of the calibration curves generated from H2O2 concentrations in the range of 25.0 to 1000.0 nmol L−1 (Fig. S3†). Additionally, we validated the components of our fabricated device, such as color background and focusing distance, for H2O2 measurement, as indicated in Fig. S4 (described in ESI†). A UV-visible spectrophotometer (Shimadzu, UV2100) and transmission electron microscope (TEM, JEOL JEM-1400, 120 kV) were used to characterize the nanoparticle properties and TMB oxidation reaction in this experiment.
2.4 General procedure and optimization conditions
H2O2 solutions at various concentrations were prepared from the stock solution of 1.0 μmol L−1 by dilution using phosphate buffer solution (PBS, 50.0 mmol L−1, pH 7.5). 0.60 mL of each concentration was mixed with 1.80 mL of AgNPs (400.0 mg L−1), 0.30 mL of acetate buffer (0.20 mol L−1, pH 4) and 0.30 mL of TMB (15.0 mmol L−1); the final solution was transferred to the cuvette. This solution received ultrasound waves from the smartphone application for 5.0 min from earphones placed on top. The blue product solution in the cuvette was inserted into our designed device and the color intensity was detected by the Color Grab application acting as the analytical signal on the smartphone (Fig. 1). The optimum conditions were evaluated through sensitivity performance studies in which the sensitivity was obtained from the slope of the linear relationship between the concentration of H2O2 in the range of 25.0 to 125.0 nmol L−1 and the analytical signal.
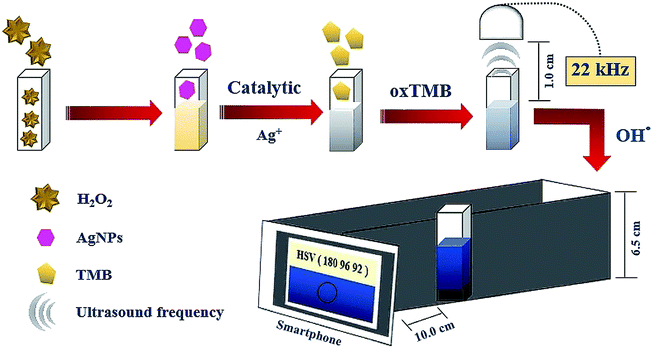 |
| Fig. 1 General process of this proposed method for H2O2 detection. | |
2.5 Biosensor measurement
Solutions of the analytes (glucose, uric acid, acetylcholine and cholesterol) at various concentrations were prepared from stock (1.50 μmol L−1) by dilution with PBS. To generate H2O2, 0.45 mL of various concentrations of each analyte were incubated for 10.0 minutes with 0.15 mL (2.5 U mL−1) of their specific enzymes, including glucose oxidase, uricase, acetylcholinesterase/choline oxidase and cholesterol oxidase for glucose, uric acid, acetylcholine, and cholesterol, respectively. Then, the solutions were detected in accordance with Section 2.4. 0.20 mL of the human negative urine sample, non-glucose, uric acid, acetylcholine, and cholesterol content were spiked with a standard of each analyte at three various concentration levels to determine the accuracy of the proposed method.
2.6 Analytical characteristics
Each calibration curve was obtained using various concentrations of the analytes following the process in Sections 2.4 and 2.5. Repeatability and reproducibility (intra-day and inter-day) were determined by the analytical detection of H2O2 at 25.0 and 50.0 nmol L−1. The limit of detection (LOD) of each analyte was evaluated as the lowest detectable concentration of analyte which promoted transformation of the color from the dark yellow color of AgNPs (blank) to the blue color of oxTMB. Additionally, the developed method was performed to investigate its ruggedness for the detection of H2O2 by varying the brands of smartphone and earphones used as generator probes. Then, the sensitivities of the linearity ranges from different smartphones and earphones were compared with common smartphone and earphone tools.
The interference effect was studied by measuring the analytical signal of a mixed solution of each analyte, 0.60 mL of H2O2 (1.0 μmol L−1), 0.45 mL of glucose (1.0 μmol L−1), uric acid (0.75 μmol L−1), acetylcholine (0.90 μmol L−1), and cholesterol (0.70 μmol L−1), with 0.50 mL of a different interference agent at 2.0 mmol L−1; then, the experimental process was performed according to Sections 2.4 and 2.5.
3 Results and discussion
3.1 Detection principle of the sensor
The measurement principle of the developed method relates to the decomposition and oxidation process of H2O2 according to these equations. |
AgNPs + H2O2 → Ag+ + OH˙ + OH−
| (1) |
|
 | (2) |
Thus,
|
AgNPs + 2H2O2 → Ag+ + 3OH˙ + OH−
| (3) |
Eqn (3) presents the overall reaction process. AgNPs were applied as the catalyst for the developed method because they can be strongly oxidized in the presence of H2O2 to generate OH˙ and Ag+ in the “oxidation-etching” procedure (eqn (1)), which is similar to the Fenton reaction.30 Additionally, the proposed method applied the ultrasound wave from the smartphone application to enhance the production of OH˙ in the presence of H2O2 because ultrasound can enhance the homolytic fission of the O–O bond (eqn (2)).31–33 Therefore, more OH˙ may be produced due to the combination of the AgNPs' oxidation-etching procedure and ultrasound application (eqn (3)). This process was used for the oxidation process of TMB substrate to oxTMB for colorimetric detection of trace amounts of H2O2 (eqn (4)). To evaluate the proposed method, we studied the oxidation efficiency of the developed method with H2O2 at 25.0 nmol L−1 (Fig. S5†). The blue color intensity of oxTMB (maximum wavelength at 650 nm) increased within 5 min with ultrasound, whereas an absorbance peak at 650 nm was not found without ultrasound. It can be supposed that the solution without ultrasound was not able to change color from dark brown to blue because this concentration was insufficient to generate OH˙ from only the oxidation-etching reaction with AgNPs. Meanwhile, it was possible to measure using ultrasound application because of the improvement in the oxidation performance as well as in oxidation-etching owing to the change in the size of AgNPs from 43 ± 4 nm (without ultrasound) to 35 ± 2 nm (with ultrasound), as indicated in Fig. 2. It was clearly observed in the TEM image of H2O2 that ultrasound application created a rough surface background caused by the slight bubbles of acoustic cavitation during the ultrasound process.34 We also examined the oxidation performance at a high concentration of H2O2 (1.0 μmol L−1), with the results shown in Fig. S6.† The highest analytical signal was found with the combination of ultrasound and AgNPs. This result confirmed that ultrasound provides oxidation ability in this proposed method. Thus, ultrasound application can enhance our experiment for the determination of H2O2 with higher measuring capability.
|
 | (4) |
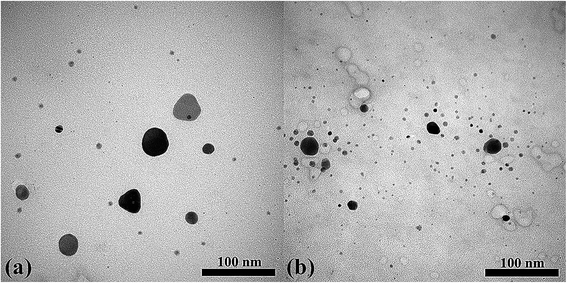 |
| Fig. 2 TEM image of AgNPs for H2O2 detection at 25.0 nmol L−1 (a) without ultrasound and (b) with ultrasound application in the proposed method. | |
3.2 Optimization of the conditions
We chose hexagonal AgNPs because their shape provides ease of oxidization, which occurs at the corners of the hexagonal nanoparticles.35 Thus, the volume of the AgNPs is associated with the efficiency of the analysis due to the oxidation-etching performance. For this reason, we studied various volumes. The sensitivity of the proposed technique tended to increase directly proportionally to the volume of AgNPs, but not when the volume was more than 1.80 mL, since higher concentrations are not related to higher peroxidase mimic capability (Fig. S7†). Therefore, we selected 1.80 mL as a suitable volume of AgNPs because it was adequate for the proposed experiment. In addition, acetate buffer was a suitable buffer medium for the formation of the free radical because of its improvement of the stability and dispersion of AgNPs. However, the acetate buffer also acts as a reducing agent for AgNPs, reducing their size from 45 ± 3 nm to 43 ± 4 nm due to the localized surface plasmon resonance (LSPR).36 Hence, some of them are changed to a spherical shape (showing a dark brown color) that is associated with a noticeable increase in absorbance at 420 nm and a decrease at 520 nm in the AgNPs spectrum, as indicated in Fig. S8.† Other buffer solution types were studied and showed less efficiency compared with acetate buffer, as shown in Fig. S9(a).† Furthermore, different pH values and concentrations of acetate buffer are related to the generation of free radicals and, thus, the sensitivity of the proposed method tended to rise quickly as the pH of acetate buffer rose from 2 to 4 and to drop rapidly when pH was more than 4.0, as indicated in Fig. S9(b).† The formation of OH˙ was favorable in the weakly acidic medium because H2O2 can be transformed to H3O2+ (oxonium ion) in a strongly acid medium (eqn (5)), which leads to reduced OH˙ formation.37 The fast transformation of OH˙ in the neutral and alkaline media can occur and generate O2˙– form from the sonolysis of water (eqn (6)), which reduced the analytical signal because OH˙ can also react extremely fast with O2˙− to create hydroxyl ions and oxygen (K = 1 × 10−10 mol L−1 s−1), decreasing the OH˙ in the system (eqn (7)). Additionally, O2˙− has less oxidation ability than OH˙.38,39 Hence, acetate buffer at pH 4.0 was selected for the developed experiment. Additionally, the concentration of acetate buffer affected the sensitivity of the analysis, as indicated in Fig. S9(c).† Its increasing concentration led to higher sensitivity owing to the increasing efficiency of OH˙ formation40 until concentrations greater than 0.20 mol L−1 showed immediate constant sensitivity. Thus, in order to reduce reagent consumption, we selected 0.20 mol L−1 of acetate buffer for our experiment. |
OH˙ + H2O2 → O2˙− + H2O + H+
| (6) |
|
O2˙− + OH˙ → OH− + O2
| (7) |
In order to quantify the color-based reaction that occurs between OH˙ and the chromogenic agent, a sufficient concentration of TMB was reacted to form oxTMB and show a blue color in the proposed analysis. The results demonstrated that the sensitivity of this method gradually increased with increasing chromogenic substrate, as seen in Fig. S9(d).† However, the sensitivity was constant when TMB concentration was more than 15.0 mmol L−1, at which point there is more than enough chromogenic agent for the reaction with analyte to form oxTMB. Hence, the 15.0 mmol L−1 concentration of chromogenic agent was chosen as sufficient for the proposed method to follow green chemistry principles.
3.3 Ultrasound conditions of the smartphone application
We studied the conditions suitable for enhancing the analysis performance. Normally, ultrasound waves have an oscillation frequency level of more than 20 kHz, but the smartphone application can generate frequency levels only to 22 kHz. Thus, frequency levels were optimized in the range from 20 to 22 kHz, as indicated in Fig. 3a. The frequency level of 22 kHz provided the highest sensitivity because the higher frequency levels affect the sound intensity I (W m−2) due to the increasing power P (W) of the sound, which increases the production efficiency of OH˙, as can be seen from the relation of the equations below, |
 | (9) |
where ω is the angular frequency (radians), f is the sound frequency (Hz), μ is the linear mass density (kg m−1), A is the amplitude of the sound waves (m), υ is the wave speed (m s−1) and r is the distance from the source (m).41 Therefore, we selected this frequency for the proposed method. Afterward, the timing of the ultrasound application was studied between 3.0–10.0 min. We found that the development process at 5.0 min was sufficient to form OH˙ due to it showing the highest sensitivity (Fig. 3b). However, it was observed that times of more than 8.0 min reduced the sensitivity due to sonolysis of the water. Different characteristics of the generated ultrasound waveforms were evaluated, as shown in the diagram in Fig. S10.† The results showed that the sawtooth waveform had the best performance for the proposed method because it was the richest in terms of harmonics, partial tones that are a whole multiple of the fundamental frequency (Fig. 4a).42 Hence, the sawtooth waveform was chosen as the suitable waveform for frequency oscillation. The effect of the distance between the solution surface and the generator probes at various values from 1.0 to 4.0 cm was optimized (Fig. 4b). It was found that the sensitivity dropped slightly in proportion to the increasing distance because an increase in distance leads to a decrease in sound intensity, as described in eqn (10). Hence, we selected 1.0 cm as a suitable distance for our proposed method. Intensity levels are another important parameter associated with sound intensity. We evaluated this parameter by detecting the various loudness levels of the smartphone system. The values cannot be transformed as the decibel (β) levels of the sound unit, but as loudness levels related to intensity levels from eqn (11), where I0 is 10−12 due to the sound intensity at which humans begin to hear.41 |
β = 10 log(I/I0)
| (11) |
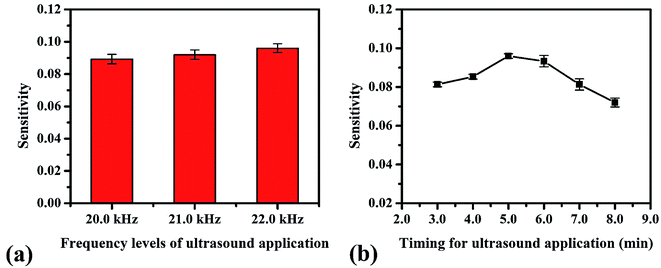 |
| Fig. 3 Optimum conditions for ultrasound application in the proposed method: (a) frequency levels of oscillation and (b) timing. | |
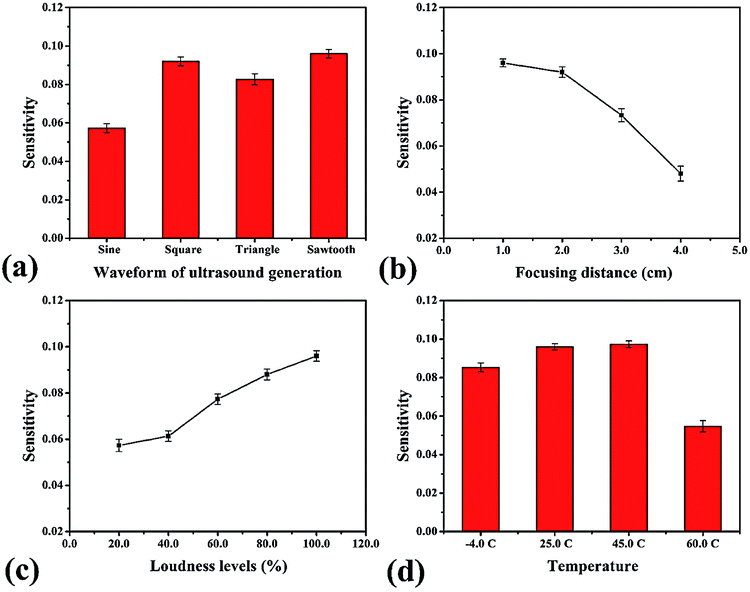 |
| Fig. 4 Optimum conditions for ultrasound application in the proposed method: (a) waveform of ultrasound generation, (b) focusing distance of generator probe, (c) loudness level of smartphone system and (d) temperature. | |
This method provided the greatest sensitivity at the highest loudness level of the smartphone system due to the increase of the sound intensity (Fig. 4c). Next, the temperature effect on the formation of OH˙ was examined. Our experimental simulation studied the temperatures −4, 25, 45, and 60 °C (Fig. 4d). We found that rising temperatures led to higher sensitivity because the velocity of sound in air depends on temperature, as seen in eqn (12) (θ = temperature, °C).41
|
V = 331(1 + θ/273)1/2
| (12) |
When the temperature is 60 °C, the sensitivity noticeably decreases due to the transformation of OH˙ by the sonolysis of water. However, our practical implementation was used at room temperature (approximately 25–32 °C). Therefore, we selected 25 °C as a sufficient temperature for analysis.
3.4 Analytical characteristics of H2O2 analysis
The proposed smartphone sensor was applied to H2O2 detection in accordance with the parameters in Table S1.† A linearity range for H2O2 was found from 25.0 to 1000 nmol L−1, with an R2 of 0.9998 (Fig. 5), as well as a spectrum of oxTMB and the gradually increasing color saturation in our designed device (Fig. S11 and S12†). The limit of detection (LOD) was calculated as 2.0 nmol L−1 from the lowest concentration of H2O2, which changes from the dark brown color of the blank solution to blue. Examination of this concentration showed a signal with more than 3-fold the standard deviation of the blank signal (n = 10). Repeatability was determined at H2O2 concentrations of 25.0 and 50.0 nmol L−1 (n = 10) with relative standard deviation values of 3.47% and 2.85%, respectively. Reproducibility tests at H2O2 concentrations of 25.0 and 50.0 nmol L−1 (n = 10) found respective relative standard deviation values of 4.58% and 3.76% for intra-day and 4.58% and 4.53% for inter-day. Ruggedness evaluations of the proposed method (both smartphone and earphone) were also studied. The results clearly show that various brand smartphones and earphones are unaffected by the proposed method and, although the smartphone cameras have different resolutions and the earphones have different sensitivities, there is no significant difference in method sensitivity according to F-test and T-test statistics values at the 95% confidence level (Fcritical = 3.78 and Tcritical = 2.36), as shown Tables S2–S5.†
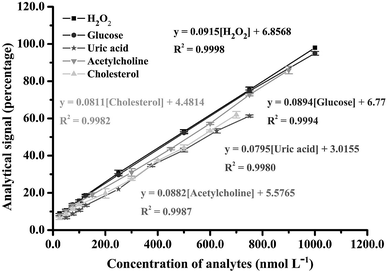 |
| Fig. 5 Analytical linearity range obtained for H2O2 and biomolecule detection in the proposed method. | |
3.5 Biosensor applications
To evaluate the usability of the proposed technique, the ultrasound application combined with smartphone sensor was used for the determination of biomolecules including glucose (Glu), uric acid (UA), acetylcholine (Ach) and cholesterol (Cho). Each biomolecule can generate H2O2 in a specific enzymatic process. Thus, their analytical characteristics were similar to the H2O2 system at the same concentration of all enzymes. Linearity ranges for the proposed smartphone biosensor were obtained at 25.0–1000.0 nmol L−1 (R2 = 0.9994) for Glu, 50.0–750.0 nmol L−1 (R2 = 0.9980) for UA, 25.0–900.0 nmol L−1 (R2 = 0.9987) for Ach and 25.0–700.0 nmol L−1 (R2 = 0.9982) for Cho (Fig. 5). In addition, the developed smartphone biosensor provided limits of detection for these biomolecules at 5.0, 12.50, 7.50, and 10.0 nmol L−1 for Glu, UA, Ach, and Cho, respectively. The activities of these enzymes for the biomolecules affected the production of H2O2, which caused their different analytical performances.
An analytical performance comparison of our proposed method and other colorimetric methods for H2O2 and biomolecule analysis is shown in Table 1.21,43–48 In recent literature, most of the smartphone colorimetric sensors are paper-based devices. Although paper-based devices for smartphone-based colorimetric detection provide rapidity and simplicity, these methods have low sensitivity. Our proposed method showed the lowest detection limit for all analytes compared with the other methods and our proposed method does not require horseradish peroxidase in the analysis system. Our analysis time was less than the co-doped carbon dots sensor which also performed the experiment in the solution phase. Additionally, our method is the first technique for acetylcholine determination with a smartphone colorimetric application. The validity of our proposed method was confirmed in real samples by spiking human urine samples with analyte standard at three different concentrations and detecting with our smartphone biosensor process (Table 2). This technique demonstrated high accuracy with total recoveries ranging from 96 to 104% and a highest relative standard deviation of 3.98%.
Table 1 Comparison of analytical characteristics of our proposed method and other colorimetric methods for H2O2 and biomolecule detection
Analyte |
Substrate |
Linear range (μmol L−1) |
Limit of detection (μmol L−1) |
Analysis time (min) |
Reference |
This work did the experiment in the solution phase. Poly(aniline-co-anthranilic acid) (ANI-co-AA). B, N, and S co-doped carbon dots (BNd-CDs). Mesoporous carbon-dispersed Pd nanoparticles (meso-C). Glucose oxidase (GOx). Horseradish peroxidase (HRP). 4-Amino antipyrine (4-AAP). 3,5-Dichloro-2-hydroxy acid sodium (DHBS). Cholesterol oxidase (Chox). |
H2O2 |
HRPf/ANI-co-AAb |
25–500 |
11.0 |
2.0 |
21 |
BNd-CDsc/TMB |
3–30 |
0.8 |
30.0 |
43a |
Pd NPs/meso-Cd/TMB |
5–300 |
1.0 |
0.5 |
44 |
Ultrasound/AgNPs/TMB |
0.005–0.2 |
0.002 |
5.0 |
This work |
Glucose |
GOxe/HRPf/ANI-co-AAb |
25–200 |
20.0 |
8.0 |
21 |
GOxe/Co3O4–CeO2 nanosheets/TMB |
5–1500 |
0.21 |
10.0 |
45 |
GOxe/HRPf/4-AAPg/DHBSh |
10–15 000 |
3.0 |
10.0 |
46 |
GOxe/HRPf/TMB |
20–4000 |
14.0 |
10.0 |
47 |
GOxe/HRPf/DHBS |
3000–8000 |
800.0 |
15.0 |
48 |
Ultrasound/GOxAgNPs/TMB |
0.005–0.2 |
0.005 |
15.0 |
This work |
Uric acid |
Uricase/HRPf/TMB |
10–1000 |
3.0 |
10.0 |
47 |
Ultrasound/Uricase/AgNPs/TMB |
0.01–0.15 |
0.0125 |
15.0 |
This work |
Cholesterol |
Choxi/HRPf/DHBS |
3000–8000 |
800.0 |
15.0 |
48 |
Ultrasound/Choxi/AgNPs/TMB |
0.005–0.14 |
0.01 |
15.0 |
This work |
Table 2 Recoveries obtained by the proposed method for the determination of all analytes in human urine samples (n = 3)
Analyte |
Standard added (nmol L−1) |
Total found (nmol L−1) |
% Recovery |
% RSD |
H2O2 |
250.0 |
257.71 |
103 ± 0.6 |
1.90 |
500.0 |
516.08 |
103 ± 1.0 |
1.85 |
750.0 |
759.89 |
101 ± 0.6 |
0.76 |
Glucose |
250.0 |
258.47 |
103 ± 1.0 |
3.33 |
500.0 |
512.86 |
102 ± 0.6 |
1.10 |
750.0 |
771.00 |
103 ± 0.6 |
0.76 |
Uric acid |
375.0 |
386.53 |
103 ± 1.0 |
2.94 |
500.0 |
504.37 |
101 ± 1.2 |
2.66 |
625.0 |
647.47 |
104 ± 0.6 |
1.06 |
Acetylcholine |
450.0 |
438.14 |
97 ± 1.2 |
2.60 |
600.0 |
592.57 |
99 ± 1.0 |
1.72 |
750.0 |
769.59 |
103 ± 0.6 |
0.78 |
Cholesterol |
300.0 |
288.34 |
96 ± 1.0 |
3.57 |
400.0 |
415.29 |
104 ± 1.5 |
3.98 |
500.0 |
517.66 |
104 ± 1.5 |
3.27 |
3.6 Interference evaluation
The performance of the smartphone biosensor was studied for the effect of interference agents expected to be found in real samples, such as sodium chloride (NaCl), sodium nitrate (NaNO3), potassium chloride (KCl), potassium dihydrogen phosphate (KH2PO4), magnesium chloride (MgCl2), magnesium sulfate (MgSO4), calcium chloride (CaCl2), calcium carbonate (CaCO3), salicylic acid, ascorbic acid, urea, creatinine and citric acid. No interference in analysis was observed when evaluated at 1.0 μmol L−1 of H2O2 and 2.0 mmol L−1 of the interference agent, with the highest change in analytical signal being 5.53% (Fig. S13†). In addition, we studied the influence of interference agents on the enzymatic system for each biomolecule (Fig. 6). The results showed no difference over ±10% from the normal beginning signal. Hence, no interference was observed in human urine samples, which showed high selectivity for analysis of these biomolecules with our proposed smartphone biosensor.
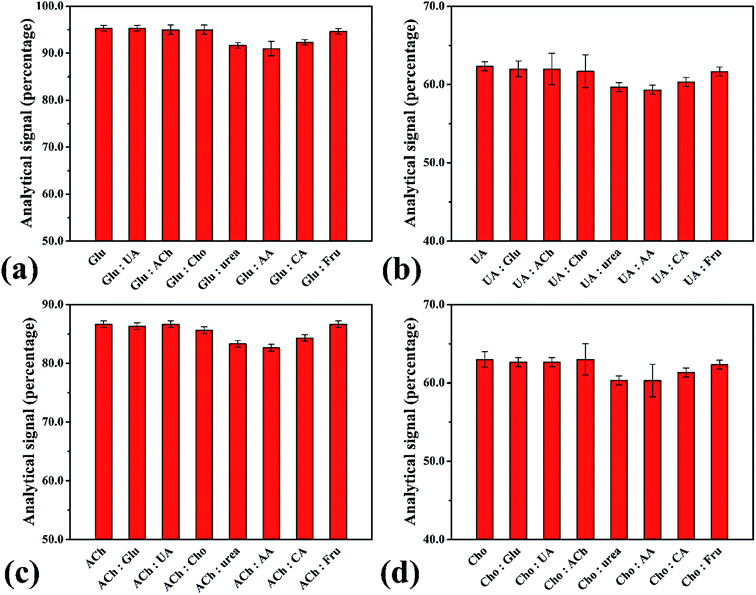 |
| Fig. 6 Analytical signals for interference evaluation of the biomolecules: interference agents (2.0 mmol L−1) such as ascorbic acid (AA), citric acid (CA) and fructose (Fru) for detection of (a) glucose (Glu) at 1.0 μmol L−1, (b) uric acid (UA) at 0.75 μmol L−1, (c) acetylcholine (Ach) at 0.90 μmol L−1 and (d) cholesterol (Cho) at 0.70 μmol L−1. | |
4 Conclusion
A simple ultrasound application combined with a smartphone colorimetric biosensor was successfully established to enhance oxidation performance and the peroxidase mimic process for the rapid and accurate quantitative detection of H2O2 and biomolecules in human urine samples. The developed device offers cost-effectiveness, low reagent consumption and waste generation, portability, and high stability, accuracy and precision, as well as ultra-sensitivity and selectivity. The ultrasound application on the smartphone device formed OH˙ from H2O2 and color saturation of the proper product was successfully detected with the smartphone camera. Compared with previous techniques described in the literature, our method showed the best analytical performance with the lowest limits of detection, including the first report of acetylcholine analysis with a smartphone colorimetric biosensor. Additionally, our method is suggested for the determination of some biomolecules in point of care medical-clinical analysis for immediate diagnosis in patients and for quality control routines.
Conflicts of interest
There are no conflicts to declare.
Acknowledgements
The authors are sincerely grateful to the financial support from Petchra Pra Jom Klao PhD Research Scholarship from King Mongkut’s University of Technology Thonburi.
References
- J. Zou, H. Cai, D. Wang, J. Xiao, Z. Zhou and B. Yuan, Chemosphere, 2019, 224, 646–652 CrossRef CAS PubMed.
- Y. Su, H. Song and Y. Lv, Microchem. J., 2019, 146, 83–97 CrossRef CAS.
- J.-L. Vincent, E. Bogossian and M. Menozzi, Crit. Care Clin., 2020, 36(1), 177–187 CrossRef PubMed.
- C. Liang and B. He, Chemosphere, 2018, 198, 297–302 CrossRef CAS PubMed.
- M. Hoshino, et al., Spectrochim. Acta, Part A, 2014, 117, 814–816 CrossRef CAS PubMed.
- H. Cai, et al., Chemosphere, 2018, 193, 833–839 CrossRef CAS PubMed.
- D. Wang, S. Qiu, M. Wang, S. Pan, H. Ma and J. Zou, Spectrochim. Acta, Part A, 2019, 221, 117138 CrossRef CAS PubMed.
- J. Chen, Y. Gao, Q. Ma, X. Hu, Y. Xu and X. Lu, Sens. Actuators, B, 2018, 268, 270–277 CrossRef CAS.
- F. Meng, H. Yin, Y. Li, S. Zheng, F. Gan and G. Ye, Microchem. J., 2018, 141, 431–437 CrossRef CAS.
- Y. Wu, Y. Gao and J. Du, Talanta, 2019, 197, 599–604 CrossRef CAS PubMed.
- A. Tahirović, A. Čopra, E. Omanović-Mikličanin and K. Kalcher, Talanta, 2007, 72(4), 1378–1385 CrossRef PubMed.
- M. Luo, et al., Eur. Polym. J., 2017, 91, 307–314 CrossRef CAS.
- A. S. Ivanova, A. D. Merkuleva, S. V. Andreev and K. A. Sakharov, Food Chem., 2019, 283, 431–436 CrossRef CAS PubMed.
- A. Benvidi, M. T. Nafar, S. Jahanbani, M. D. Tezerjani, M. Rezaeinasab and S. Dalirnasab, Mater. Sci. Eng., C, 2017, 75, 1435–1447 CrossRef CAS PubMed.
- H. Guan, J. Zhang, Y. Liu, Y. Zhao and B. Zhang, Electrochim. Acta, 2019, 295, 997–1005 CrossRef CAS.
- X. Liang, W. Li, H. Han and Z. Ma, J. Electroanal. Chem., 2019, 834, 43–48 CrossRef CAS.
- Y. Kosto, et al., Appl. Surf. Sci., 2019, 488, 351–359 CrossRef CAS.
- B. Gökdere, A. Üzer, S. Durmazel, E. Erçağ and R. Apak, Talanta, 2019, 202, 402–410 CrossRef PubMed.
- P. J. Rivero, E. Ibañez, J. Goicoechea, A. Urrutia, I. R. Matias and F. J. Arregui, Sens. Actuators, B, 2017, 251, 624–631 CrossRef CAS.
- S. Kanchi, M. I. Sabela, P. S. Mdluli, Inamuddin and K. Bisetty, Biosens. Bioelectron., 2018, 102, 136–149 CrossRef CAS PubMed.
- O. Hosu, et al., Talanta, 2019, 204, 525–532 CrossRef CAS PubMed.
- K. Phoonsawat, N. Ratnarathorn, C. S. Henry and W. Dungchai, Analyst, 2018, 143(16), 3867–3873 RSC.
- S. Basiri, A. Mehdinia and A. Jabbari, Colloids Surf., A, 2018, 545, 138–146 CrossRef CAS.
- A. Üzer, S. Durmazel, E. Erçağ and R. Apak, Sens. Actuators, B, 2017, 247, 98–107 CrossRef.
- Y. Ding, et al., Mater. Sci. Eng., C, 2017, 80, 558–565 CrossRef CAS PubMed.
- Y. Zhang, et al., Sens. Actuators, B, 2018, 275, 155–162 CrossRef CAS.
- Y. Segura, F. Martínez, J. A. Melero, R. Molina, R. Chand and D. H. Bremner, Appl. Catal., B, 2012, 113–114, 100–106 CrossRef CAS.
- A. Abou Dalle, et al., Electrochim. Acta, 2017, 246, 1–8 CrossRef CAS.
- M. Soltani Firouz, A. Farahmandi and S. Hosseinpour, Ultrason. Sonochem., 2019, 57, 73–88 CrossRef CAS PubMed.
- G.-L. Wang, X.-Y. Zhu, Y.-M. Dong, H.-J. Jiao, X.-M. Wu and Z.-J. Li, Talanta, 2013, 107, 146–153 CrossRef CAS PubMed.
- H. N. McMurray and B. P. Wilson, J. Phys. Chem. A, 1999, 103(20), 3955–3962 CrossRef CAS.
- E. Dalodière, M. Virot, P. Moisy and S. I. Nikitenko, Ultrason. Sonochem., 2016, 29, 198–204 CrossRef PubMed.
- D. B. Tata, J. Biglow, J. Wu, T. R. Tritton and F. Dunn, Ultrason. Sonochem., 1996, 3(1), 39–45 CrossRef CAS.
- R. Xiao, D. Diaz-Rivera, Z. He and L. K. Weavers, Ultrason. Sonochem., 2013, 20(3), 990–996 CrossRef CAS PubMed.
- T. Parnklang, C. Lertvachirapaiboon, P. Pienpinijtham, K. Wongravee, C. Thammacharoen and S. Ekgasit, RSC Adv., 2013, 3(31), 12886–12894 RSC.
- K. Ranoszek-Soliwoda, et al., J. Nanopart. Res., 2017, 19(8), 1–15 CrossRef CAS PubMed.
- G. Lyngsie, L. Krumina, A. Tunlid and P. Persson, Sci. Rep., 2018, 8(1), 1–9 CrossRef CAS PubMed.
- F. Collin, Int. J. Mol. Sci., 2019, 20(10) CAS.
- J. L. Roberts, M. M. Morrison and D. T. Sawyer, J. Am. Chem. Soc., 1978, 100(1), 329–330 CrossRef CAS.
- W. He, Y. T. Zhou, W. G. Wamer, M. D. Boudreau and J. J. Yin, Biomaterials, 2012, 33(30), 7547–7555 CrossRef CAS PubMed.
- “Some Basic Principles.”, Available: https://www.harada-sound.com/sound/handbook/basicterms.html, Accessed: 13-Mar-2020 Search PubMed.
- “The Difference Between Waveforms and Why It Matters.”, Available: https://www.perfectcircuit.com/signal/difference-between-waveforms, Accessed: 29-May-2020 Search PubMed.
- B. Peng, et al., Anal. Bioanal. Chem., 2020, 412(4), 861–870 CrossRef CAS PubMed.
- Y. Zhang, et al., Sens. Actuators, B, 2018, 275, 155–162 CrossRef CAS.
- N. Alizadeh, A. Salimi and R. Hallaj, Sens. Actuators, B, 2019, 288, 44–52 CrossRef CAS.
- F. Li, X. Wang, J. Liu, Y. Hu and J. He, Sens. Actuators, B, 2019, 288, 266–273 CrossRef CAS.
- X. Wang, et al., Anal. Bioanal. Chem., 2018, 410(10), 2647–2655 CrossRef CAS PubMed.
- J. Li, Y. Sun, C. Chen, T. Sheng, P. Liu and G. Zhang, Anal. Chim. Acta, 2019, 1052, 105–112 CrossRef CAS PubMed.
Footnote |
† Electronic supplementary information (ESI) available. See DOI: 10.1039/d0ra03792c |
|
This journal is © The Royal Society of Chemistry 2020 |
Click here to see how this site uses Cookies. View our privacy policy here.