DOI:
10.1039/D0RA06730J
(Paper)
RSC Adv., 2020,
10, 40480-40488
The inactivation mechanism of chemical disinfection against SARS-CoV-2: from MD and DFT perspectives†
Received
4th August 2020
, Accepted 28th September 2020
First published on 6th November 2020
Abstract
Exploring effective disinfection methods and understanding their mechanisms on the new coronavirus is becoming more active due to the outbreak of novel coronavirus pneumonia (COVID-19) caused by severe acute respiratory coronavirus 2 (SARS-CoV-2). By combining molecular dynamics and first-principles calculations, we investigate the interaction mechanism of chemical agents with 3CL hydrolase of SARS-CoV-2. The radial distribution functions indicate that the biocidal ingredients are sensitive to the unsaturated oxygen atoms of 3CL hydrolase and their interactions remarkably depend on the concentration of the biocidal ingredients. Besides, we find that the adsorption performance of the active ingredients for the unsaturated oxygen atoms is superior to other styles of atoms. These computational results not only decipher the inactivation mechanism of chemical agents against SARS-CoV-2 from the molecule-level perspective, but also provide a theoretical basis for the development and application of new chemical methods with a high disinfection efficiency.
Introduction
Since the end of 2019, the continuous outbreak of new coronavirus pneumonia associated with a novel coronavirus in the world has become a serious threat to public health.1–3 At the end of June 28, 2020, there had been 9
843
073 confirmed cases of COVID-19 on a global scale, including 495
760 associated deaths giving a fatality of 5.0%.4 Clearly, SARS-CoV-2 is highly a pathogenic strain with a frightening fatality rate. Several important transmission events occurring in hospital and family settings have shown that SARS-CoV-2 can transmit via close human-to-human contact (e.g., respiratory droplets over a short distance or contaminated hands) or via fecal–oral routes.5–8 Furthermore, SARS-CoV-2 is highly stable in a favourable external environment and its infectiousness can remain for a few minutes to several days on different styles of materials.9,10 Compared to rough surfaces (e.g. wood and cloth), the surface stability of SARS-CoV-2 is higher on smooth surfaces such as glass, stainless steel, and plastic.11 This viability in vitro is conducive to coronavirus transmission between vector and humans. Consequently, adopting effective methods that are helpful in cleaning and disinfecting community settings, healthcare settings, and the home environment is extremely pressing for prevention and control of epidemic situations.
Chemical biocidal method has been acted as a powerful disinfection measure in minimizing the occurrence and spread of the viruses. Currently, it has been reported that 75% medical ethanol and 0.55% sodium hypochlorite can effectively reduce the infectivity of SARS-CoV-2 within recommended time.12,13 These two disinfectants play a prominent role in several global public health emergencies caused by SARS-CoV, MERS-CoV, and SARS-CoV-2. Silver, as a precious metal, has been consciously utilized to act as a biocidal agent since ancient times, and its biocidal property in ionic form shows a strong inactivation for influenza A virus.14 In 2003, silver ions based disinfectants are included in the SARS prevention and treatment manual that is compiled by No. 302 Hospital of Chinese People's Liberation Army. Meanwhile, the efforts in actions activity of silver ions on the coronaviruses further confirm that silver ions have significant antiviral activity for SARS-CoV.15 Therefore, silver ions could be a candidate for the prevention and therapeutic method of SARS-CoV-2 in the absence of specific drug therapy. The inactivation mechanisms of chemical disinfectants against bacteria and viruses have been studied in the experimental and theoretical level.16–19 Nevertheless, a theoretical and molecular-level understanding of interaction mechanism between chemical disinfectants and SARS-CoV-2 is still lacking. In addition, molecular dynamics and first principles simulations have become powerful tools in illustrating the delicate interplay of various interactions of biomaterials and electronic structure of many-body systems, and predicting novel materials for technology innovation, especially 2D materials.20–23 Therefore, the deciphering of molecular mechanisms of chemical disinfectants inactivating SARS-CoV-2 are worth investigating to provide a theoretical guidance for the actual treatment and prevention.
In this article, we theoretically study the dynamics behaviors, structural and electronic properties between chemical disinfectants and 3CL hydrolase of SARS-CoV-2 by combining molecular dynamics and first-principles calculations. The results show that the active ingredients of chemical disinfectants are anchored to the unsaturated oxygen atoms of amino acid residues by hydrogen bonds or chemical bonds. Meanwhile, the interactions of the active ingredients with amino acid residues exhibit a strong concentration dependence. Among anchored structures, the ingredient-S1 conformations are more favorable than other conformations that the active ingredients are anchored to S2 or S3, and their binding energies and charge transfer are larger than 0.5 eV and 0.1e, respectively. Furthermore, the calculations of charge density difference further demonstrate the strong interaction and considerable charge transfer between the active ingredients and amino acid residues.
Computational methods
Molecular dynamics (MD) simulations are performed with the current state-of-art Forcite program using the COMPASS (Condensed-phase Optimized Molecular Potentials for Atomistic Simulation Studies) forcefield parameters.24–26 The approximate atomic charges are assigned by utilizing the Gasteriger method for the ethanol–/hypochlorite–3CL hydrolase systems, being used to evaluate electrostatic interactions.27 For the silver ion–3CL hydrolase system, the charge of silver atom is set to 1.0e. The calculations of energy minimizations of all complex systems are conducted by adopting both the steepest descent and conjugate gradient algorithm at atmospheric pressure of 10−4 GPa. Concurrently, the electrostatic and van der Waals interactions are described by using Ewald and atom-based summation methods in all simulations, respectively. Considering the actual situations, the MD simulations of all complex systems are carried out within the framework of NVT ensemble (constant number of particles, constant volume and constant temperature). The system temperature is set as 300 K which is regulated by adopting the Berendsen thermostat.27,28
All calculations at the density functional theory (DFT) level are conducted using DMol3 package, as implemented in the Materials Studio.29 The exchange–correlation interaction is described by the Perdew–Burke–Ernzerhof (PBE) functional within the generalized gradient approximation (GGA).30,31 The vdW dispersion interaction has a significant influence on the interaction potential of intermolecular. This potential is a key ingredient in the adsorption structures and binding energies.32,33 For a proper treatment of the long-range van der Waals (vdW) interactions between the biocidal ingredients and amino acid residues, the dispersion corrected DFT (DFT-D) approach proposed by Grimme is employed.34,35 The atomic orbital is treated through a double numeric quality basis set including polarization functions (DNP) and the global orbital cutoff is kept as 5.0 Å.36,37 To quantitatively analyze the interactions of the biocidal ingredients with amino acid residues, the binding energies (ΔE) are determined by using the formula38
ΔE = Esystem − Eingredient − Eresidue |
where
Esystem,
Eingredient, and
Eresidue represent the total energy of the ingredient–residue system, ingredient molecule, and amino acid residue, respectively. The interaction process with a negative binding energy is spontaneous and energetically favorable, being expected to occur in the chemical reactions where a smaller value indicates a stronger constraint force.
39 Simultaneously, charge transfer (Δ
Q) is deduced by Mulliken population analysis, determining whether the biocidal ingredient act as acceptor or donor.
Results and discussion
Molecular dynamics calculations
The 3CL hydrolase, a crucial protein required for the maturation of coronaviruses, is extremely important for the life cycle of such viruses.2 This makes it the most attractive target for the development of anti-coronavirus drugs in addition to four structural proteins (i.e. nucleocapsid protein N, matrix glycoprotein M, envelope protein E, spike glycoprotein S).40–42 Therefore, the atomistic structure of 3CL hydrolase of SARS-CoV-2 is established to investigate interaction mechanism with the biocidal ingredients, being presented in Fig. 1a. The complex systems consisting of the ingredient molecules (i.e. ethanol, hypochlorite, and silver ion) and 3CL hydrolase are constructed within the framework of LAMMPS program and shown in Fig. 1b and d. Adding 1000 ingredient molecules ensures that all complex systems are small enough for dynamic simulations over picosecond time scales.26,43
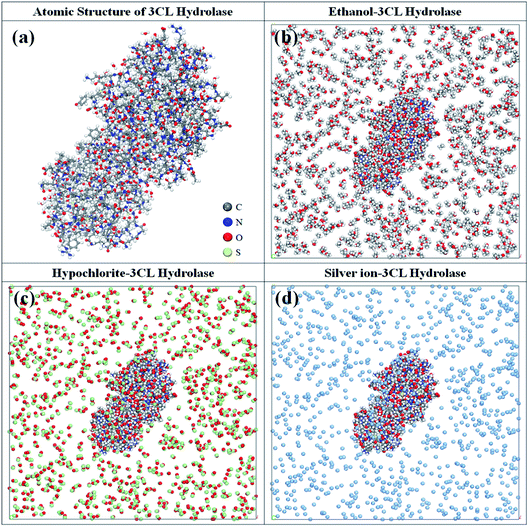 |
| Fig. 1 (a) The atomic structure of 3CL hydrolase of SARS-CoV-2. The complex systems consisting of 3CL hydrolase and (b) ethanol, (c) hypochlorite, and (d) silver ion. | |
Before investigating the dynamics behaviors of the ethanol–, hypochlorite–, and silver ion–3CL hydrolase systems, their geometric structures fully relaxed by combining the steepest descent and conjugate gradient algorithm are first discussed. After minimizing energies, it is found that 3CL hydrolase is almost surrounded by ethanol molecules and their minimum distance is less than 2.0 Å in the ethanol–3CL hydrolase system. Interestingly, the ethanol molecules are mainly concentrated in the vicinity of glutamate acid (GLU) and aspartic acid (ASP) residues of 3CL hydrolase. Although ethanol molecules also occur near other amino acid residues such as arginine (ARG), asparagine (ASN), and isoleucine (ILE) residues, the number of adsorbed molecules by them is relatively small compared with that by GLU and ASP residues. For the hypochlorite–3CL hydrolase system, it clearly shows that the distribution of hypochlorite molecules in the vicinity of 3CL hydrolase is similar to that of ethanol molecules. However, their concentrations near the above-mentioned residues is lower than that of ethanol molecules and the minimum distance is less than 3.0 Å. In contrast, in the case of the silver ion–3CL hydrolase system, there are only a small amount of silver ions near 3CL hydrolase within the range of 4.0 Å. Meanwhile, we find that silver ions are primarily clustered in the vicinity of threonine acid (THR) and phenylalanine acid (PHE) residues and partly occur near other residues (e.g. lysine, leucine, glutamate acid, etc.).
In order to better decipher the underlying mechanism of interaction between the biocidal ingredients and 3CL hydrolase, the radial distribution function (RDF) of three complex systems are investigated by analyzing the MD simulation trajectories. RDF is also referred to the pair correlation function, characterizing the probability of the existence of an atom at the origin of an arbitrary reference frame.44–46 The RDFs g(r)O–O, g(r)N–O, and g(r)S–O of O(GLU residues)–/O(ASP residues)–/O(ARG residues)–/O(ASN residues)–/O(MET residues)–O(ethanol), N(ARG residues)–/N(ASN residues)–O(ethanol), and S(MET residues)–O(ethanol) pairs for the ethanol–hydrolase system are shown in Fig. 2a and b. It is well known that the peak of RDF curve within 3.5 Å represents the hydrogen bonds and chemical bonds, and that outside 3.5 Å is contributed by the non-bond (Coulomb and vdW) interactions.47 As shown in Fig. 2a, the first peak of g(r)O–O of O(GLU residues)–/O(ASP residues)–O(ethanol) pairs appear around 2.6 Å that is smaller than 3.5 Å, indicating the bond interactions between ethanol and these two residues. Meanwhile, there is a second peak illustrated in the range 4–5 Å for GLU and ASP residues, which can be explained as the non-bond interactions. For ARG and ASN residues, it is found that the first peak of their g(r)O–O is located within the range of 6.5–7.5 Å. Thus, the non-bond interactions are responsible for the constraint of ARG and ASN residues with ethanol molecules, which is further confirmed by the g(r)N–O of N(ARG residues)–/N(ASN residues)–O(ethanol) pairs (see Fig. 2b). In addition, MET residue is also considered due to the presence of S and O atoms in it. In Fig. 2b, the locations of the first peak of g(r)O–O and g(r)S–O clearly shows that the O–O interactions are stronger than the interactions between S atom of MET residue and O atom of ethanol. Furthermore, we find that the peak height of g(r)O–O of O(ARG residues)–/O(ASN residues)–/O(MET residues)–O(ethanol) pairs is lower than that of O(GLU residues)–/O(ASP residues)–O(ethanol), which indicates a higher binding energy between GLU residues, ASP residues and ethanol molecules.
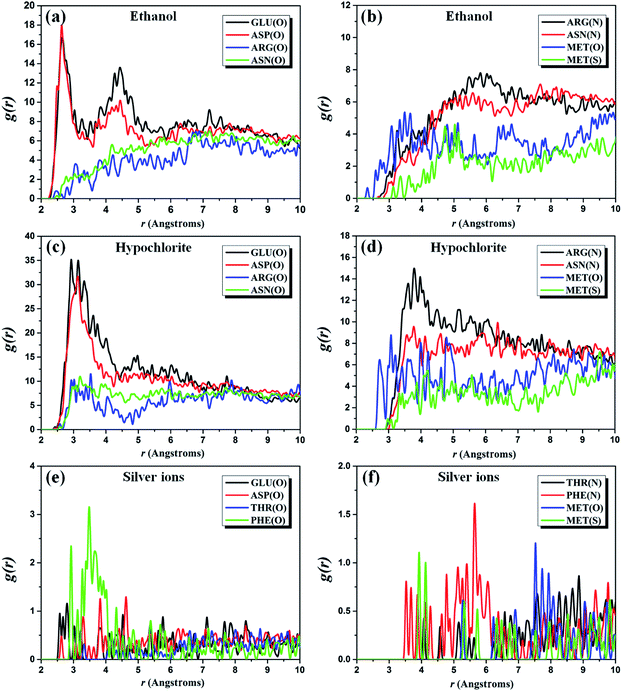 |
| Fig. 2 Pair correlation function (a and b) g(r) of ethanol, (c and d) g(r) of hypochlorite and (e and f) g(r) of silver ions with 3CL hydrolase of SARS-CoV-2. | |
Owing to the similar distribution of ingredient molecules near 3CL hydrolase, five amino acid residues discussed in the ethanol–3CL hydrolase system are also used for the RDF analysis of the hypochlorite–3CL hydrolase system. In Fig. 2c, it can be observed that the first peak of g(r)O–Cl of O(GLU residues)–/O(ASP residues)–Cl(hypochlorite) pairs is found around 3.0 Å which is less than 3.5 Å. This indicates that the constraints between these two residues and hypochlorite molecules are contributed by hydrogen bonds or chemical bonds. The position of the first peak of g(r)O–Cl of O(ARG residues)–/O(ASN residues)–Cl(hypochlorite) pairs is close to 3.2 Å, but their second peaks occur in the range from 7.0 Å to 8.0 Å. As a result, the hypochlorite–GLU/ASP residue interactions are stronger than that attracted by ARG and ASN residues. It is further demonstrated by the non-bond constraints of Cl atoms with N atoms of ARG and ASN residues, as depicted in Fig. 2d. For MET residues, it can be noted that the first peak of g(r)S–Cl is around 3.0 Å, whereas that of g(r)O–Cl is outside 3.5 Å. Thus, the S–Cl interactions is stronger than the interactions between O atoms and Cl atoms. Among these five residues, there are large binding energies in the O(GLU residues)–/O(ASP residues)–Cl(hypochlorite) pairs due to their higher peak height.
Unfortunately, the distribution behaviors of the biocidal ingredients occurring in the ethanol– and hypochlorite–3CL hydrolase systems cannot be well-preserved in the case of silver ions. The above-discussed results have shown that silver ions are mainly concentrated near THR and PHE residues. Therefore, these two residues are the protagonists in the calculations of RDFs. For comparison, we also consider GLU, ASP, and MET residues. Fig. 2e presents the RDFs of silver ions appearing around the O atoms of GLU, ASP, THR, and PHE residues. It can be found that the first peak of O(GLU residues)–/O(ASP residues)–Ag(silver ions) pairs is observed at ∼3.0 Å, which is less than that (>3.0 Å) of O–Ag pairs from THR and PHE residues.
However, the peak height of g(r)O–O of O(PHE residues)–Ag(silver ions) pairs is larger than that of other three residues. Consequently, the binding energy of O atoms of PHE residues with silver ions is relatively strong, which is further confirmed by the RDF curves plotted in Fig. 2f. The first peaks of g(r) of N(THR residues)–/N(PHE residues)–/O(MET residues)–/S(MET residues)–Ag(silver ions) pairs are located in the scope of the non-bond interactions.
Previous studies focusing on the disinfection effectiveness of ethanol with different concentrations have clearly shown that the inactivation of coronaviruses is sensitive to the ethanol concentrations.48–51 Ethanol with the concentration of from 75% to 95% readily inactivate coronavirus infectivity by approximately 4
log10 or more and outside this concentration range is less effective.52,53 Although this phenomenon is well-proven in the experiment level, its molecular-level mechanism is still unknown. As a result, two complex systems with ethanol of 30% and 75% are discussed to gain the insight into the molecular-level mechanism of this phenomenon, being depicted in Fig. 3. The relaxation calculations indicate that the main distribution of ethanol molecules near 3CL hydrolase is similar with in the case of pure ethanol (Fig. 1b). Nevertheless, there are some differences in these two complex systems. For the case of 30% ethanol, water (H2O) molecules are the main aggregation in the vicinity of 3CL hydrolase, which may reduce the probability of ethanol contacting with amino acid residues. The ethanol molecules cannot effectively interact with amino acid residues, thereby the bioactivity of 3CL hydrolase cannot be disturbed. On the contrary, an expected distribution phenomenon for ethanol molecules is exhibited in the complex system with 75% ethanol. We find that there are more ethanol molecules near 3CL hydrolase compared to that case of 30% ethanol, which suggests that ethanol molecules are more likely to interact directly with amino acid residues. H2O molecules have a weak impact on the interactions between ethanol molecules and 3CL hydrolase. Additionally, ethanol solvent with a high concentration can readily damage to the structure of 3CL hydrolase. By comparison, it is found that 3CL hydrolase is completely enclosed by ethanol molecules when the alcoholicity is set to 75% and the amount of molecules attracted by 3CL hydrolase is smaller than that of pure ethanol. However, ethanol molecules that first interact with 3CL hydrolase could impede the subsequent interaction processes of other molecules with 3CL hydrolase in the system of pure ethanol. This could be the underlying reason that 75% ethanol has more remarkable effect in terms of disinfection compared with pure ethanol.
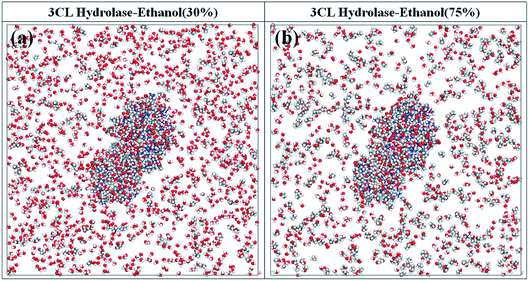 |
| Fig. 3 The complex systems with ethanol of (a) 30% and (b) 75%. | |
Similarly, RDFs of two complex systems with the alcoholicity of 30% and 75% are also considered to provide further evidence for the above-discussed results. We investigate the same five amino acid residues (i.e. GLU, ASP, ARG, ASN, and MET residues) as for the pure ethanol system and the g(r) plots of the corresponding atom pairs are presented in Fig. 4. It can be noted that the first peak of g(r)O–O of O(GLU)–/O(ASP)–O(ethanol) pairs occurs around 2.5 Å, which indicates that the position of the first peak is independent of ethanol content. The height of g(r)O–O curve of O(GLU)–O(ethanol) pairs increases significantly when the alcoholicity changes from 30% to 75%. However, its peak height within the range of 4.5–10.0 Å has an apparent decrease when the ethanol concentration up to 100%, which is expected to be observed in the molecular level. Simultaneously, we note that the peak height of g(r)O–O curves of O(ARG)–/O(ASN)–O(ethanol) pairs firstly increases and then decrease with the increasing of the concentration of ethanol, especially in the range from 2.0 Å to 4.0 Å. The similar behavior also observed in the g(r)N–O curves of N(ARG)–/N(ASN)–O(ethanol) pairs and the most obvious section is in the range of 4.5–7.5 Å. For MET residues, the ethanol content has a slightly influence on the overall tendency of g(r)O–O and g(r)S–O of O(MET)– and S(MET)–O(ethanol) pairs, but the peak height of these two curves (4.5–5.5 Å) exhibits a relationship of first rise and then drop with the ethanol concentration. These results are in good agreement with the reported phenomenon in the experiments where the desired inactivation effect cannot be achieved under low concentrations and/or pure ethanol.
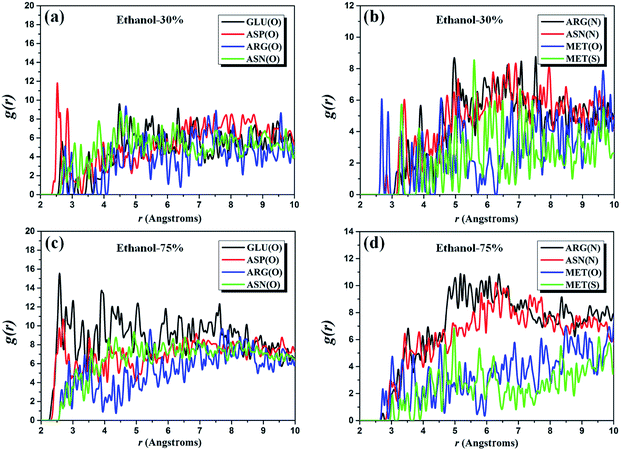 |
| Fig. 4 RDFs g(r) of the complex system with ethanol of (a and b) 30% and (c and d) 75%. | |
First principles calculations
According to the above-described MD results, four amino acid residues, namely, GLU, ASP, THR, and PHE residues, are used for further estimating the interaction with ethanol, hypochlorite, and silver ion. To explore the most favorable binding structure amino acid between residues and the active ingredients, we consider three possible anchoring sites for each residue, as illustrated in Fig. 5. For GLU and ASP residues, owing to the similar terminal structure (–CH2CO2), two O atoms and one H atom of –CH2CO2 group are set as the anchoring positions of ethanol and hypochlorite molecules. In the THR residue, the binding sites include the H atom of –CH3 group, the O atom of –OH group, and the O atom of –CO group, whereas for PHE residue they are the H atom and C atom of –C6H5 group and the O atom of –CO group. It should be noted that the C and H atoms of ethanol molecule and the O atom of hypochlorite are not tested on these anchoring positions. On the basis of the aforementioned DFT approach, we perform the geometric optimization of the ingredient–residue configurations. The computational results of the most favorable conformation are listed in Table 1.
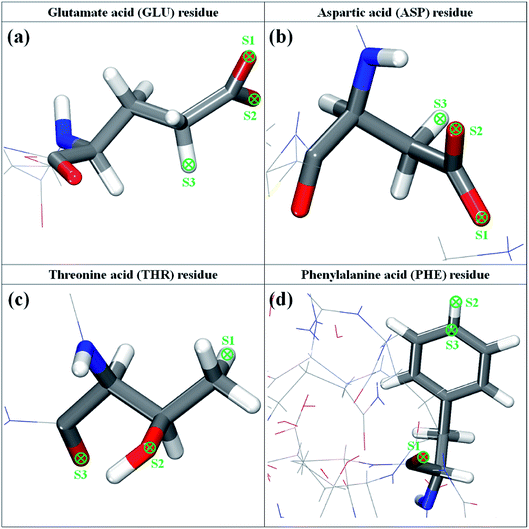 |
| Fig. 5 The molecular structure of glutamate, aspartic, threonine, and phenylalanine residues of 3CL hydrolase of SARS-CoV-2. | |
Table 1 Binding energy (ΔE), charge transfer (ΔQ) from ingredient to amino acid residue, minimum distance (dmin) of the atom belonging to composition molecule to amino acid residue
Composition |
Residue |
Site |
ΔE (eV) |
ΔQ (e) |
dmin (Å) |
Ethanol |
GLU |
S1 |
−0.78 |
0.240 |
1.63 |
Hypochlorite |
GLU |
S1 |
−0.64 |
0.115 |
2.39 |
Silver ion |
PHE |
S1 |
−1.44 |
0.402 |
2.16 |
Ethanol molecule
There are six possible configurations depending on the anchoring positions of GLU and ASP residues. Among six anchoring positions, the most favorable binding site is the S1 of GLU residue and the corresponding conformation with the lowest energy is displayed in Fig. 6a. As we can see, the O–H bond of ethanol molecule points to the S1 site and the structural parameters of have some slight variations. The minimum distance (dmin) of the atom of ethanol molecule to GLU residue ranges from 1.63 Å to 2.20 Å for six binding configurations. The magnitude is slightly close to that (1.87 Å) of ethanol on graphene oxide.54,55 The binding energies (ΔE) of six configurations are lower than −500 eV, confirming that ethanol molecule is bound by GLU or ASP residue though the chemical interaction. The reference length of O–H covalent bond is 0.95 Å obtained by summing the covalent radii of O and H atoms.35,56 Meanwhile, a strong hydrogen bonding force is defined in the range from 1.5 Å to 2.5 Å.57 As a result, the interaction process between ethanol molecule and GLU (or ASP) residue is dominated by a strong hydrogen bonding force. To understand the interaction nature, we represent the charge density difference (CDD) image for the ethanol/GLU structure with the lowest energy by using the below formula:
Δρ = ρethanol/residue − ρethanol − ρresidue |
where ρethanol/residue, ρethanol, ρresidue are the total charge density of the ethanol/residue structure, isolated ethanol molecule, and amino acid residue, respectively. It should be noted that ρethanol and ρresidue are calculated with each fragment of the ethanol/residue structure at the same positions. From the CDD graph (Fig. 6b), we can observe that the majority of the charges are significantly depleted on the H atoms of –CH2OH group of ethanol molecule, while the charges are mainly accumulated on the –CO2 group of GLU residue. This reveals that the charges are transferred from ethanol molecule to GLU residue. The charge transfer (ΔQ) from ethanol molecule to GLU residue based on the Mulliken population analysis is 0.240e per molecule (Table 1), suggesting that there is a strong electrostatic interaction in the ethanol/GLU residue structure.
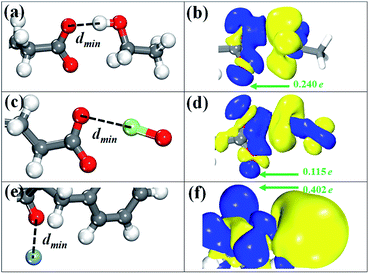 |
| Fig. 6 The atomic structure and charge density difference of most favorable conformation. (a and b) Ethanol–GLU configuration, (c and d) hypochlorite–GLU configuration, and (e and f) silver ion–PHE configuration. The isosurface is set to 5 × 10−3e Å−1. The yellow and blue color represent depletion and accumulation of charges, respectively. The green arrow denotes the direction of charge transfer. | |
Hypochlorite
Similar to the case of ethanol molecule, six possible binding configurations are tested. Fig. 6c shows the most favorable conformation in which the Cl–O bond of hypochlorite are almost on the same horizontal line as the S1 site. In this configuration, the geometric parameters of –CO2 group are 1.268 Å for the C–O bond and 125.7° for the O–C–O angle. Meanwhile, the plane constituted by three atoms of –CO2 group of GLU residue is obviously twisted to 54.3° from 19.1°. The ΔE values of all binding configurations are less than −500 eV with the exception of the Cl atom-S3 site (originating from ASP residue) configuration that is −0.497 eV. Nevertheless, their binding stability is inferior to that of the ethanol/GLU structures due to the larger ΔE values. This result indicates that the interaction between hypochlorite and GLU residue is relatively weak compared with the adsorption of ethanol molecule. In addition, the dmin in the hypochlorite/GLU residue configuration with the lowest energy is 2.39 Å, being similar to that (2.28 Å) of Cl2 molecule adsorbed on ZnO nanocluster.58 Based on the reference length (1.62 Å) of O–Cl covalent bond and the defined scope of hydrogen bonds, we can get a conclusion that the hydrogen bonding is responsible for the constraint of hypochlorite with GLU residue. The CDD image in Fig. 6d clearly reflects the main depletion of charges in the region around the Cl atom, indicating the accumulation of charges on the O atoms of –CO2 group and partly on the hypochlorite. This is in accordance with expectation because elemental O is more electronegative than Cl.59 Quantitative charge analysis (Table 1) shows that hypochlorite serves as a charge donor to GLU residue with a ΔQ of 0.115e per molecule, validating the relatively molecule–GLU residue interaction again.
Silver ion
Regarding the adsorption of silver ion, we examine six initial binding configurations where THR and PHE residues act as adsorbent. The most favorable conformation is found when the silver ion is aligned to the S1 site of PHE residue and depicted in Fig. 6e. Although the bond length and bond angle of PHE residue have slight changes after adsorption, the carbon ring is twisted by 6°. We find that the distance of the silver ion and the O atom of PHE residue is 2.16 Å which is in accordance with that of silver nanoparticle on graphene oxide.60 The dmin value is only 0.23 Å larger than the reference length (1.93 Å) of Ag–O covalent bond. Consequently, the constraint of silver ion with PHE residue is contributed by a pseudo-covalent bond. From Table 1, it can be found that the ΔE (−1.44 eV) is almost twofold of that (−0.78 eV) of the case of ethanol molecule, which characterizes a strong binding force. The CDD isosurface diagram of the silver ion-S1 site (in the PHE residue) configuration is shown in Fig. 6f. It clearly indicates a depletion of charges in the spatial region near silver ion and accumulation of charges in the proximity of –NH and –CO groups of PHE residue. The total ΔQ from the silver ion to the PHE residue is found to be 0.402e per silver ion, suggesting that the silver ion acts as a donor.
Conclusions
In summary, we have carried out molecular dynamics and first-principles calculations to study the interaction mechanism of three chemical agents with 3CL hydrolase of SARS-CoV-2. It is found that ethanol and hypochlorite molecules are more sensitive to the GLU and ASP residues of 3CL hydrolase compared to other residues, whereas for silver ion it is readily adsorbed by THR and PHE residues. The RDF results reveal that the unsaturated oxygen atoms of GLU, ASP, THR, and PHE residues play an important role in the adsorption process of three biocidal ingredients. Besides, the interaction performance as function of the concentrations is also investigated. We find that the interactions of ethanol molecules with 3CL hydrolase increase with increasing concentration. However, when the concentration reaches a critical value, the probability of ingredient molecules occurring in a certain range will decrease. This indicates that concentrations determine the disinfection efficiency. Our DFT calculations confirm that ethanol, hypochlorite, and silver ion strongly bound to the unsaturated oxygen atom of GLU, ASP, THR, and PHE residues. The binding energies provide sufficient evidence to conclude that the adsorption of three biocidal ingredients by amino acid residues is an exothermic process. Furthermore, the calculated minimum distances clearly show that the pseudo-covalent bond is responsible for the constraint of silver ion with PHE residue and the molecule–residue interaction for ethanol and hypochlorite is mainly contributed by the hydrogen bonds. Meanwhile, we observe that ethanol, hypochlorite, and silver ion act as charge donor providing considerable charges for residues. The present findings provide possible information for a well-grounded understanding of inactivation mechanism of chemical agents against SARS-CoV-2.
Conflicts of interest
There are no conflicts to declare.
Acknowledgements
This work was supported by the Key-Area Research and Development Program of GuangDong Province (2019B010131001), the National Natural Science Foundation of China (No. 51706029), and the National Key R&D Program of China (2018YFE0204600).
Notes and references
- J. F. W. Chan, S. Yuan, K. H. Kok, K. K. W. To, H. Chu, J. Yang, F. Xing, J. Liu, C. C. Y. Yip and R. W. S. Poon, Lancet, 2020, 395, 514–523 CrossRef CAS.
- P. Sang, S. Tian, Z. Meng and L. Yang, ChemRxiv, 2020 DOI:10.26434/chemrxiv.11932995.
- T. Singhal, Indian J. Pediatr., 2020, 87, 281–286 CrossRef.
- WHO, available from: https://who.sprinklr.com/.
- C. I. Paules, H. D. Marston and A. S. Fauci, JAMA, J. Am. Med. Assoc., 2020, 323, 707–708 CrossRef CAS.
- J. Riou and C. L. Althaus, Eurosurveillance, 2020, 25, 1–5 CrossRef.
- L. T. Phan, T. V. Nguyen, Q. C. Luong, T. V. Nguyen, H. T. Nguyen, H. Q. Le, T. T. Nguyen, T. M. Cao and Q. D. Pham, N. Engl. J. Med., 2020, 382, 872–874 CrossRef.
- J. Gu, B. Han and J. Wang, Gastroenterology, 2020, 158, 1518–1519 CrossRef CAS.
- N. van Doremalen, T. Bushmaker, D. H. Morris, M. G. Holbrook, A. Gamble, B. N. Williamson, A. Tamin, J. L. Harcourt, N. J. Thornburg and S. I. Gerber, N. Engl. J. Med., 2020, 382, 1564–1567 CrossRef.
- J. Bu, D.-D. Peng, H. Xiao, Q. Yue, Y. Han, Y. Lin, G. Hu and J. Chen, medRxiv, 2020 DOI:10.1101/2020.02.13.20022715.
- A. Chin, J. Chu, M. Perera, K. Hui, H.-L. Yen, M. Chan, M. Peiris and L. Poon, The Lancet Microbe, 2020, 411–412 Search PubMed.
- Y. Yi, P. N. Lagniton, S. Ye, E. Li and R.-H. Xu, Int. J. Biol. Sci., 2020, 16, 1753–1766 CrossRef CAS.
- C. Bryson-Cahn, J. Duchin, V. A. Makarewicz, M. Kay, K. Rietberg, N. Napolitano, C. Kamangu, T. H. Dellit and J. B. Lynch, Clin. Infect. Dis., 2020 DOI:10.1093/cid/ciaa256.
- M. Minoshima, Y. Lu, T. Kimura, R. Nakano, H. Ishiguro, Y. Kubota, K. Hashimoto and K. Sunada, J. Hazard. Mater., 2016, 312, 1–7 CrossRef CAS.
- J. Han, L. Chen, S. Duan, Q. Yang, M. Yang, C. Gao, B. Zhang, H. He and X. Dong, Biomed. Environ. Sci., 2005, 18, 176–180 CAS.
- K. Ito, T. Kajiura and K. Abe, Jpn. J. Infect. Dis., 2002, 55, 117–121 CAS.
- J. Van Bueren, R. A. Simpson, H. Salmax, H. D. Farrelly and B. D. Cookson, Epidemiol. Infect., 1995, 115, 567–579 CrossRef CAS.
- B. T. Robert, P. G. Charles and B. Gabriel, Crit. Rev. Environ. Control, 1989, 14, 295–315 Search PubMed.
- A. Nalian and A. V. Iakhiaev, Thromb. Haemostasis, 2008, 100, 18–25 CrossRef CAS.
- J. Wang, P. Cieplak and P. A. Kollman, J. Comput. Chem., 2000, 21, 1049–1074 CrossRef CAS.
- N. Argaman and G. Makov, Am. J. Phys., 2000, 68, 69–79 CrossRef CAS.
- B. Song, Y. Zhou, H.-M. Yang, J.-H. Liao, L.-M. Yang, X.-B. Yang and E. Ganz, J. Am. Chem. Soc., 2019, 141, 3630–3640 CrossRef CAS.
- L.-M. Yang, V. Bacic, I. A. Popov, A. I. Boldyrev, T. Heine, T. Frauenheim and E. Ganz, J. Am. Chem. Soc., 2015, 137, 2757–2762 CrossRef CAS.
- P. Chhetri, C. Lee and L. Rakesh, J. Cancer Sci. Ther., 2011, 3, 220 CAS.
- C. Reece, D. J. Willock and A. Trewin, Phys. Chem. Chem. Phys., 2015, 17, 817–823 RSC.
- Y. Yu, S. Chen, X. Li, J. Zhu, H. Liang, X. Zhang and Q. Shu, RSC Adv., 2016, 6, 20034–20041 RSC.
- X. Wu, C. Dai, S. Fang, H. Li, Y. Wu, X. Sun and M. Zhao, Phys. Chem. Chem. Phys., 2017, 19, 16047–16056 RSC.
- Y. Li, S. Wang, B. Arash and Q. Wang, Carbon, 2016, 100, 145–150 CrossRef CAS.
- P. Wang, X. Jiang, J. Hu, B. Wang, T. Zhou, H. Yuan and J. Zhao, Phys. Chem. Chem. Phys., 2020, 22, 11045–11052 RSC.
- S. Mukherjee, A. Banwait, S. Grixti, N. A. Koratkar and C. V. Singh, ACS Appl. Mater. Interfaces, 2018, 10, 5373–5384 CrossRef CAS.
- J. P. Perdew, K. Burke and M. Ernzerhof, Phys. Rev. Lett., 1996, 77, 3865–3868 CrossRef CAS.
- T. Lu and F. Chen, J. Mol. Model., 2013, 19, 5387–5395 CrossRef CAS.
- A. M. Reilly and A. Tkatchenko, Chem. Sci., 2015, 6, 3289–3301 RSC.
- C. Tan, Q. Yang, R. Meng, Q. Liang, J. Jiang, X. Sun, H. Ye and X. Chen, J. Mater. Chem. C, 2016, 4, 8171–8178 RSC.
- X. Chen, C. Tan, Q. Yang, R. Meng, Q. Liang, M. Cai, S. Zhang and J. Jiang, J. Phys. Chem. C, 2016, 120, 13987–13994 CrossRef CAS.
- Y. Inada and H. Orita, J. Comput. Chem., 2008, 29, 225–232 CrossRef CAS.
- S. Zhang, Y. Zhang, S. Huang, H. Liu, P. Wang and H. Tian, J. Phys. Chem. C, 2010, 114, 19284–19288 CrossRef CAS.
- X. Ye, X. Jiang, L. Chen, W. Jiang and S. Ma, Appl. Surf. Sci., 2020, 521, 146477 CrossRef CAS.
- S. Mukherjee, A. Banwait, S. Grixti, N. A. Koratkar and C. V. Singh, ACS Appl. Mater. Interfaces, 2018, 6, 5373–5384 CrossRef.
- A. D. Reddy, S. B. Suh, R. Ghaffari, S. N. Jiten and K. S. Kim, Bull. Korean Chem. Soc., 2003, 24, 899–900 CrossRef CAS.
- B. Xia and X. Kang, Protein Cell, 2011, 2, 282–290 CrossRef CAS.
- I.-L. Lu, N. Mahindroo, P.-H. Liang, Y.-H. Peng, C.-J. Kuo, K.-C. Tsai, H.-P. Hsieh, Y.-S. Chao and S.-Y. Wu, J. Med. Chem., 2006, 49, 5154–5161 CrossRef CAS.
- D. Van Niekerk and J. P. Mathews, Fuel Process. Technol., 2011, 92, 729–734 CrossRef CAS.
- N. Li, Z. Chang, X. Wu, C. Sun and W. Li, IOP Conf. Ser. Earth Environ. Sci., 2018, 199, 032002 CrossRef.
- Z. Luo and J. Jiang, Polymer, 2010, 51, 291–299 CrossRef CAS.
- L. Liu, R. Zhang, Y. Liu, W. Tan and G. Zhu, J. Mol. Model., 2018, 24, 137 CrossRef.
- J. P. Zeng, F. H. Wang and X. D. Gong, Mol. Simul., 2013, 39, 169–175 CrossRef CAS.
- A. Siddharta, S. Pfaender, N. J. Vielle, R. Dijkman, M. Friesland, B. Becker, J. Yang, M. Engelmann, D. Todt and M. P. Windisch, J. Infect. Dis., 2017, 215, 902–906 CrossRef CAS.
- H. F. Rabenau, J. Cinatl, B. Morgenstern, G. Bauer, W. Preiser and H. W. Doerr, Med. Microbiol. Immunol., 2005, 194, 1–6 CrossRef CAS.
- H. F. Rabenau, G. Kampf, J. Cinatl and H. W. Doerr, J. Hosp. Infect., 2005, 61, 107–111 CrossRef CAS.
- M. Saknimit, I. Inatsuki, Y. Sugiyama and K. Yagami, Exp. Anim., 1988, 37, 341–345 CrossRef CAS.
- Z.-M. Chen, J.-F. Fu, Q. Shu, Y.-H. Chen, C.-Z. Hua, F.-B. Li, R. Lin, L.-F. Tang, T.-L. Wang and W. Wang, World J. Pediatr., 2020, 16, 1–7 CrossRef.
- G. Kampf, D. Todt, S. Pfaender and E. Steinmann, J. Hosp. Infect., 2020, 104, 246–251 CrossRef CAS.
- J.-S. Kim, C.-J. Yu, K.-C. Ri, S.-H. Choe and J.-C. Ri, J. Phys. Chem. C, 2019, 123, 8932–8942 CrossRef CAS.
- A. A. Kistanov, Y. Q. Cai, D. R. Kripalani, K. Zhou, S.V. Dmitriev and Y. W. Zhang, J. Mater. Chem. C, 2018, 6, 4308–4317 RSC.
- P. Pyykk and M. Atsumi, Chem.–Eur. J., 2009, 15, 186–197 CrossRef.
- G. A. Jeffrey, An introduction to hydrogen bonding, Oxford University Press, 1997 Search PubMed.
- J. Beheshtian, A. A. Peyghan and Z. Bagheri, Appl. Surf. Sci., 2012, 258, 8171–8176 CrossRef CAS.
- A. Kistanov, Y. Cai, D. Kripalani, K. Zhou, S. Dmitriev and Y. W. Zhang, J. Mater. Chem. C, 2018, 6, 4308–4317 RSC.
- S. Tang, W. Wu and J. Yu, Phys. Chem. Chem. Phys., 2016, 18, 7797–7807 RSC.
Footnote |
† Electronic supplementary information (ESI) available. See DOI: 10.1039/d0ra06730j |
|
This journal is © The Royal Society of Chemistry 2020 |