DOI:
10.1039/D0RA07786K
(Paper)
RSC Adv., 2020,
10, 39640-39653
Structural and conformational changes induced by missense variants in the zinc finger domains of GATA3 involved in breast cancer†
Received
10th September 2020
, Accepted 22nd October 2020
First published on 29th October 2020
Abstract
Breast cancer (BC) is the main cancer in women having multiple receptor based tumour subtypes. Large scale genome sequencing studies of BC have identified several genes among which GATA3 is reported as a highly mutated gene followed by TP53 and PIK3CA. GATA3 is a crucial transcription factor, and was initially identified as a DNA-binding protein involved in the regulation of immune cell functions. Different missense mutations in the region of the DNA-binding domain of GATA3 are associated with BC and other neoplastic disorders. In this study, computational based approaches have been exploited to reveal associations of various mutations on structure, stability, conformation and function of GATA3. Our findings have suggested that, all analysed missense mutations were deleterious and highly pathogenic in nature. A molecular dynamics simulation study showed that all mutations led to structural destabilisation by reducing protein globularity and flexibility, by altering secondary structural configuration and decreasing protein ligand stability. Essential dynamics analysis indicated that mutations in GATA3 decreased protein mobility and increased its conformational instability. Furthermore, residue network analysis showed that the mutations affected the signal transduction of important residues that potentially influenced GATA3-DNA binding. The present study highlights the importance of different variants of GATA3 which have potential impact on neoplastic progression in breast cancer and may facilitate development of precise and personalized therapeutics.
1. Introduction
Breast cancer (BC) is the main leading cancer, diagnosed more commonly among women worldwide. It arises from a terminal ductal lobular unit within the breast.1 Over the past decade, there has been increasing trends in the incidence of breast cancer. The recent GLOBOCAN, 2018 published by IACR (International Association of Cancer Registries) estimates incidence of 11.6% with mortality of 6.6% of total cancer cases worldwide irrespective of gender and age.2 Various factors such as increasing age, hormonal factors such as hyperestrogenic exposure of the body in the form of a long menstrual life due to early menarche and late menopause, nulliparity, limited/no breastfeeding, more use of contraceptive pills, etc. have been associated with increased risk of breast cancer.3 It may also develop due to accumulation of somatic mutations which affects ∼85% of women having no family history of the disease (https://www.breastcancer.org/). Thousands of mutations that contain small insertions/deletions or indels in various studies have novel genes involved in the breast cancer development.4 Whole exome sequencing studies suggested that ∼10% of mutations were found in p53 (TP53), GATA3 and phosphoinositide-3-kinase (PIK3CA) genes that acts as mutation driver genes in BC.5 Among them, GATA3 is an important gene as it plays dual roles in both development and oncogenesis.6
GATA gene encode proteins having less conserved transactivation domain and two highly conserved zinc finger DNA binding domains, which recognise consensus sequence 5′ [A/T] GATA [A/G] 3′ in the DNA.7 GATA family binding proteins are 6 members (from GATA1 to GATA6), identified as a regulator of immune cell lineage and each member have an organ specific function which regulate the fate of cell speciation.8 GATA3 involves in development of kidney, breast, T-lymphocytes, central nervous system and erythrocytes. Overexpression of GATA3 is associated with pancreatic carcinoma, Hodgkin's lymphoma and esophageal carcinoma and its under-expression may be associated with clear renal cell carcinoma and cervical cancer.9–11 However, both over and underexpression of GATA3 has been observed in BC.12 GATA3 is mutated in ∼5% of sporadic and ∼13% of familial breast tumors. It regulates different pathways involved in breast cancer via recruitment and interaction with other co factors such as FOXA1 and ERα.13 GATA3 targets mammary gland and facilitates differentiation of luminal epithelial cells. The expression of GATA3 is linked with subtypes of BC with higher expression observed in the luminal A and luminal B subtypes.14 In breast carcinoma, patient diagnosed with the higher expression of GATA3 shows good prognosis in comparison to lower GATA3 expression.
Mutations at both N and C fingers also known as zinc finger domains were reported in HDR (hypoparathyroidism, deafness and renal dysplasia) patients where protein fail to bind with DNA and other cofactor such as FOG2.15,16 Interestingly, in BC cases, most of the mutations were centred at C finger domain of GATA3.17,18 Experimental studies have suggested that frameshift mutation in this domain lead to loss of DNA binding that result in reprogramming of transcription network.19 Moreover, nonsynonymous missense mutations occurred at both N and C finger domains may be have been more deleterious and leads to worst prognosis. Molecular consequences of these nonsynonymous missense mutations of GATA3 have never been investigated in details. In the present study, we have adopted computational methods to explore structural and functional effect of human GATA3 reported missense mutations in BC and other associated disorders. Pathogenicity of different variants were predicted through multiple tools and structural and conformational studies were elucidated through molecular dynamics (MD) simulation, followed by residues network analysis. We found that all reported missense mutations were highly pathogenic, caused structure destabilisation, altered protein secondary properties and conformation and also affected residues network topology.
2. Materials and methods
2.1 Dataset collection
Protein sequence of human GATA3 was obtained from UniProt (Id: P23771).20 The nonsynonymous missense mutations such as W275R (tryptophan275 to arginine), C318R (cysteine318 to arginine), N320K (asparagine320 to lysine) and L344F (leucine344 to phenylalanine) were obtained from previous experimental studies15–17 and these mutations were further confirmed by dbSNP database (https://www.ncbi.nlm.nih.gov/snp/) of NCBI (National centre for biotechnology information).
2.2 Nonsynonymous SNPs annotations
Pathogenicity of various missense mutations were predicted by Predict SNP server, which host about 7 SNP prediction tools (Predict SNP, SNP&GO, MAPP, PhD-SNP, Polyphen2, SIFT and SNAP) in a common web interface.21 Each tool caters results as their expected confidence in the range between 0–100%. Predict SNP rely on the confidence score of an individual tool and the maximum tally of transformed confidence score is used to develop a common consensus classifier. All tools have been individually described in our previous study.22 Pathogenicity of above mutations were also predicted from PANTHER, PROVEN, FATHMM and Mutation Assessor servers. PANTHER is Protein analysis through evolutionary relationships, predict functional impact of missense variants on the basis of position-specific evolutionary preservation (PSEP). It estimates length (millions of years) of a variant that has been preserved in the protein and provides output as a probably damaging with threshold of 450 my (million year). The longer a variant has been conserved, the more expected that variant has damaging effect.23 PROVEAN is protein variation effect analyser that measures the impact of substitution of residue on the biological function of protein as either neutral or deleterious. The prediction is based on the changes, caused by given variations, in the similarity of query sequence to a set of its related protein sequences. It computes score with a threshold value −2.5.24 FATHMM is Functional analysis through hidden markov models, based on the mutation dataset reported in various database such as CanProVar, UniProt and used for both coding as well as non-coding variants to predict functional consequences, gives score with prediction threshold of −0.75.25 And, Mutation Assessor, attributes classes of SNPs on the footing of functional impact of amino acid substitution as neutral, low, medium or high. Its impact calculated on the basis of evolutionary conservation of polymorphic variants in protein homologs.26 Furthermore, stability and functional impact of different mutants were assessed through MutPred and I-Mutant2 tools as described previously.22
2.3 Protein 3D structures preparation and validation
3D structure of GATA3 in complex with DNA is available at PDB (Id: HC7).27,28 Non-protein molecules were removed by PyMOL and structure was represented as wildtype (WT) GATA3. Mutants (MTs) of GATA3 were prepared by replacing the target amino acid residues (W275R, C318R, N320K and L344F) in protein sequences and 3D structures were modelled through T-TASSER server.29 Briefly, after the submission of amino acid sequence, I-TASSER retrieves template from the PDB library by using LOMETS (locally installed meta-threading) approach.30 The aligned region reassembled into full length models through replica-exchange Monte Carlo simulations while the unaligned regions built by an ab initio modelling. It generated series of models (5 in most of cases) where the quality of predicted models is based on the account of C- and TM-scores. C-score lies in the range of −5 to 2, higher score signifies more confidence and TM score which shows the closest structural similarity and higher the TM score (≥0.5) higher the structural similarity between template and model. Protonation of residues is not deal by homology modelling. Therefore, we have used H++ server for missing hydrogen and maintain protonated states of delta and epsilon nitrogen of histidine residues.31
Further, structural similarity between templates and generated models were examined by calculating the RMSDs (root mean square deviation) between them. All structures (WT and MTs) were validated by estimating their geometry and stereochemical properties through SAVES server.32 Qualities of all the structures were also validated through ProSA (Protein Structure Analysis) and Q-MEAN (Qualitative Model Energy Analysis) web servers.33,34
2.4 Molecular dynamics simulation
All structures (WT, W275R, C318R, N320K and L344F) were subjected to atomistic molecular dynamics simulation by GROMACS 5.0. in conjunction with AMBER03 force field.35,36 At first, topology files were prepared using pdb2gmx by placing the structures in cubic box and maintained the periodic boundary distance of 1.2 nm from the edge of protein to the wall of box. All protein systems were solvated with TIP3P water model and neutralized by sodium and chloride ions. After that, systems were energy minimised by steepest-descent method. NVT and NPT equilibration simulations were performed for 100 and 500 ps, respectively using positional restrains. All bonds were restrained by LINCS algorithm and long range electrostatic were maintained by PME (Particle Mesh Ewald) method. Temperature and pressure of 300 K and 1 bar were maintained using v-scale (modified Berendsen thermostat) and Parrinello-Rahman barostat, respectively. Finally, production simulations of 100 ns were performed for all the systems.
2.5 Principle component and free energy landscape analyses
Principle components analysis (PCA) also known as essential dynamics was conducted to obtained the major motions during MD simulation that are significant to the biological function of a given protein.37 After removing the translational and rotational motions, the covariance matrix was constructed and diagonalized which generated a set of eigenvectors with corresponding eigenvalues.38 PCA were restricted to backbone atoms and utilised the stabilised MD simulation trajectories with PCs having low cosine values (≤0.2).39 Free energy landscape (FEL) analyses were performed by taking the reaction coordinates of PC1 and PC2 from the respective systems.
2.6 Residues network and interaction analyses
Residue interaction network (RIN) was used to understand the impact of missense mutations on structure and function of proteins. RIN was constructed from represented structures of all proteins. Initially, MD optimized protein structures for each system were used to predict the interaction network by RING server 2.0 (residues interaction network generator) and obtained networks were visualized in Cytoscape3.4 plugin with RINalyzer.40,41 Network centrality in residue–residue interaction network were constructed through weighted graph theory where residues represented the nodes and weights denoted the number of hydrogen bonds between the residues. Furthermore, betweenness centrality was calculated to identify the central node using Brandes algorithm.42 Additionally, different types of physico-chemical interactions or bonding such as hydrogen bonds, van der Waals and ionic interactions involved in WT and MT proteins were also studied in which residues were represented as node and interactions between the residues were denoted as edges in RIN.43
2.7 Analysis
Most of the analyses were performed in GROMACS suite. Secondary structures were obtained through DSSP (dictionary of secondary structure of protein) method using do_dssp module.44 PCA and FEL were analysed by gmx analyze, gmx anaeig and gmx sham modules of GROMACS package. All 3D structures of protein for visualizations were rendered in PyMOL (The PyMOL Molecular Graphics System, Version 1.3 Schrodinger, LLC) and UCSF chimera (Computer Graphics Laboratory, University of California, San Francisco).
3. Results
3.1 Pathogenicity prediction of various mutants
Protein sequence of WT GATA3 was taken from Uniprot database (P23771) and sequence of different mutants (W275R, C318R, N320K and L344F) were created by replacing tryptophan273 with arginine (W275R), cysteine316 with arginine (C318R), aspargine318 with lysine (N320K) and leucine342 with phenylalanine (L344F) in the WT protein sequence. Sequence based pathogenic prediction of different MTs were monitored through Predict SNP server and found that all MTs were deleterious and diseased with high prediction rate (ESI Table S1†). Predict SNP predicted that all MTs were deleterious with prediction rate ranged from 76% (minimum) for C318R and N320K MTs to 87% (maximum) for W275R and L344F MTs. Similar results were also observed when using other tools such as MAPP, PhD-SNP, Polyphen, SIFT, SNAP and SNPs&GO, which predicted all variants as deleterious and had deceased phenotype with prediction rate ranging from 59% to 89% (ESI Table S1†). Further, impact and pathogenicity of all variants were also monitored through PROVEN, Mutation Assessor, FATHMM and PANTHER-PSEP tools, which showed all MTs were deleterious, high impact, cancer causing and probably have damaging effect (ESI Table S2†).
3.2 Functional impact of variants and 3D structure modelling
Structural and functional impacts of various MTs were examined through I-mutant and MUpro servers and found that all MTs have decreased structural stabilities. ΔΔG < 0 or > 0 denoted decreased and increased stabilities. W275R, C318R and N320K MTs exhibited −1.78, −0.62 and −0.19 of ΔΔG which were <0, indicated decreased stabilities (Table 1). Similar results were also observed in MUpro tools where all MTs were having low stabilities (Table 2). Different structural deformations were found due to different variants where loss of stabilities and alterations in the protein structures were existed with high probabilities (Table 1).
Table 1 Stability analysis of different GATA3 variants
|
I-Mutant |
MUpro |
Variant |
ΔΔGa |
Stability |
ΔΔGa |
Stability |
ΔΔG = Free energy change. |
W275R |
−1.78 |
Decrease |
−1.1046698 |
Decrease |
C318R |
−0.62 |
Decrease |
−0.56893866 |
Decrease |
N320K |
−0.19 |
Decrease |
−1.1932192 |
Decrease |
L344F |
0.11 |
Decrease |
−0.66260087 |
Decrease |
Effects of various mutations on the structure and conformation of GATA3 were elucidated at 3D structural level by structural modelling and MD simulation. 3D structure of GATA3 was taken from PDB as described in method and 3D MT models were generated through I-TASSER server. PDB structure of GATA3 consists of N-finger domain on the N-terminal side, C-finger domain on C-terminal and a linker which connected both N and C finger domains (also known as Zn finger domains). N-finger domain consists of single α-helix, 2 β-sheets and short N-terminal flanking region.27 While C finger domain consists of single α-helix, 2 β-sheets and long C-terminal flanking region known as C-tail (Fig. 1A). W275R mutation was found in the boundary of first β-sheet of N finger domain while all other mutations (C318R, N320K and L344F) were lied at or near to β-sheets and α-helix of C finger domain (Fig. 1A). All MT models along with WT were validated through different structure validation tools. Initially, validations of all MT models were performed by superimposing the generated models with reference templates and measured the RMSDs between them. We have obtained 1.2, 1.1, 1.5 and 1.6 Å values of RMSD of W275R, C318R, N320K and L344F MTs models, respectively when aligned with their reference templates which demonstrated that structural qualities of MT models were almost overlapped with template structures (ESI Fig. S1 and Table S3†). Further, stereochemical properties of WT and MTs were examined through inspection of dihedral angles in Ramachandran plot and found that all WT and MTs exhibited high percentage of residues to be placed in favoured and allowed regions (ESI Table S3†). ERRAT, ProSA and QMEAN results suggested that WT and MTs have better 3D structure qualities. WT and MTs were further proceeded for all atomic MD simulation.
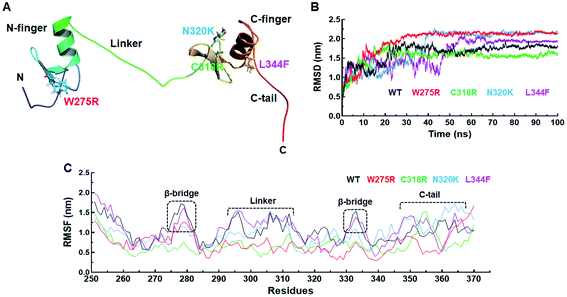 |
| Fig. 1 Structure and MD simulation. (A) Tertiary structure of GATA3, (B) root mean square deviation of backbone atoms of GATA3 protein and (C) root mean square fluctuation of backbone atoms of GATA3 protein. Tertiary structure of GATA3 was shown in cartoon mode and mutant residues were displayed in stick mode. Different domains of GATA3 were also shown in figure. WT, W275R, C318R, N320K and L344F MTs were labelled in black, red, green, blue and magenta lines in 2D graphs, respectively. | |
Table 2 Functional annotation of different GATA3 variants
Variant |
MutPred2 |
MutPred2 score |
Molecular consequences |
Probability |
P-value |
W275R |
0.874 |
Gain of intrinsic disorder |
0.42 |
6.0 × 10−3 |
Altered transmembrane protein |
0.11 |
0.03 |
Altered stability |
0.10 |
0.04 |
C318R |
0.938 |
Gain of intrinsic disorder |
0.38 |
0.01 |
Altered disordered interface |
0.32 |
7.0 × 10−3 |
Altered transmembrane protein |
0.16 |
0.01 |
N320K |
0.869 |
Altered disordered interface |
0.21 |
0.03 |
Altered transmembrane protein |
0.16 |
0.01 |
L344F |
0.841 |
Altered ordered interface |
0.24 |
0.04 |
Altered transmembrane protein |
0.10 |
0.05 |
3.3 Destabilisation of GATA3 mutants (MTs)
Prior to investigate the stabilisation effects of different MTs (W275R, C318R, N320K and L344F) along with WT, minimum energies of all the respective WT and MT systems were monitored through gmx energy module of Gromacs. WT, W275R, C318R, N320K and L344F MTs exhibited −798
138, −798
688, −798
571, −798
557 and −797
937 kJ mol−1, respectively of potential energies (ESI Fig. S2A†). Minimum energy values for all the systems were obtained suggesting that all systems were stabled and can be proceeded for further analysis. The overall structural stabilities of WT and all MTs have been assessed through measuring the RMSD (root mean square deviation) of protein backbone. RMSD of WT and all MTs were stabilised after 70 ns of simulation period (Fig. 1B). The RMSD of WT, W275R, C318R, N320K and L344F MTs exhibited average values ∼1.63, ∼1.95, ∼1.53, ∼1.79, and ∼1.64 nm, respectively. High RMSD values of W275R, N320K and L344F MTs as compared to WT and C318R MT indicating the destabilisation of GATA3 proteins upon these mutations. Further, we examined the protein flexibility at residues levels of WT and all MTs by calculating RMSF (root mean square fluctuation). The variations in the RMSF pattern and values suggested that WT and MTs displayed similar flexibilities except β-bridges of N and C finger domains, linker region, and C-tail of GATA3 (Fig. 1C). RMSFs of WT and L344F were higher at both β-bridges and linker regions with average values ≥1.5 nm as compared to the rest of MTs. Deeper inspection at these regions suggested that residues 26–29 (N finger domain), Ser303, Arg306 and Arg312 (linker), Ala332 and Asn333 (C finger domain) remained to be highly flexible in WT and L344F (Fig. 1C). However, as expected ∼15 residues spanned at C-tail region remained fluctuated in WT and all MT proteins with RMSF values ≥1.6 nm. C-tail of GATA3 is an extreme C-terminal flanking region which tend to be more exposed on surface with high mobility. Flexibilities of β-bridges and linker of GATA3 is necessary as these regions were assisted in binding with double standard DNA or ligand.27 RMSF results of WT and MTs were articulated, MTs (W275R, C318R, N320K) became rigid and less flexible, resulted unstable 3D structure configuration of GATA3 as compared to WT and L344F MT.
Size and structural compactness of protein upon different mutations were measured through Rg (radius of gyration) at function of time. Rg of WT and C318R were stabilised after ∼78 and ∼65 ns time, while Rg of W275R, N320K and L344F MTs showed equilibration after ∼50 ns (ESI Fig. S2B†). Rg values of WT, W275R, C318R, N320K and L344F MTs were ∼2.10, ∼1.65, ∼1.94, ∼1.98, and ∼1.87 nm, respectively. All MTs displayed less Rg than WT, indicating that all MTs decreased the compactness of GATA3 protein. To further explore the structure compactness, we measured the distance between N- and C-terminals of WT and MTs. In GATA3 protein, two residues at N and C-terminal positions were serine, therefore we have measured the carbon α distance of these two serine residues by gmx distance script of Gromacs. N- and C-terminal distance were maintained after ∼75 ns in WT and ∼90 ns in all MTs (ESI Fig. S3A†). Moreover, the average distance between N- and C-terminals were ∼4.16, ∼2.77, ∼3.77, ∼3.78 and ∼3.37 nm in WT, W275R, C318R, N320K and L344F, respectively. Distance between N- and C-terminals were reduced in all MTs as compared to WT, resulted decrease in the compactness of protein. Rg analyses demonstrated that structural compactness and globularity of GATA3 were reduced upon different mutations. RMSD, RMSF and Rg analyses cumulatively suggested, mutations in the zinc or N and C finger domains of GATA3 led to 3D structures destabilisation that may influence the GATA3–DNA interaction.
3.4 Structural properties analyses
Structural properties of various MTs and WT were elucidated by measuring the SASA (solvent accessible surface area), by monitoring the pattern of secondary structures formation, and by calculating the intra and inter H-bonding (hydrogen bonding) during MD simulation. SASA is a crucial parameter for determining the protein conformation in water and provide the areas exposed to solvent molecules by mediating the interactions through hydrophobic and hydrophilic residues.22 Total SASA of WT and MTs showed fluctuations at the starting of MD simulation, as simulation proceed it came down and stabilised till the end of simulation period (ESI Fig. S3B†). Similar results were also observed in hydrophobic SASA (ESI Fig. S3C†), but hydrophilic SASA showed variations W275R, N320K and L344F MTs as compared to WT (ESI Fig. S3D†). The average values of total SASA were 97.13, 91.17, 94.30, 99.57 and 92.71 nm2 in WT and W275R, C318R, N320K and L344F MTs, respectively (Fig. 2A). Lower values of total SASA in W275R, C318R and L344F MTs as compared to WT accompanied by the reduction of hydrophilic SASA. W275R, C318R and L344F MTs exhibited 56.96, 58.45, 57.06 nm2 of average values of hydrophilic SASA which is lower than WT (97.13 nm2) and N320K (62.24 nm2) MT (Fig. 2A). Variations and low values of SASA in case of MTs indicated that mutations induced the conformational changes of GATA3 protein, which in turn affects the protein ligand interactions.
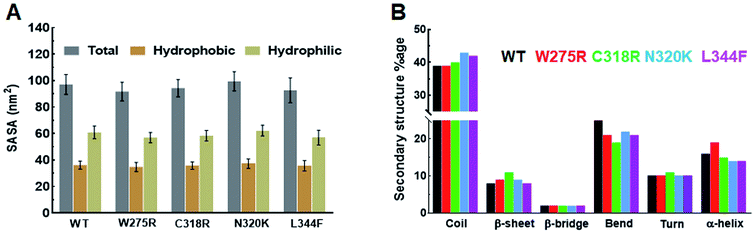 |
| Fig. 2 Secondary structural properties analyses of GATA3. (A) Total, hydrophobic and hydrophilic SASA of WT and MTs and (B) secondary structures formation during MD simulation. Refer Fig. 1 for different colour codes for WT and MTs. | |
Secondary structures formation during simulations were performed through DSSP method, where different structural moieties such as α-helix, β-sheet, bridge, bend, turn and 3-helix were analysed individually (Fig. 2B and ESI Fig. S4†). The secondary structure evolutions with time of WT and all MTs displayed an increased in coil and sheet contents in MTs with concomitant decreased of bend and α-helix (Fig. 2B). In W275R MT, coils remain unchanged while both α-helix, β-sheet were increased throughout the simulation period (Fig. 2B and ESI Fig. S4B†). WT exhibited 39, 8, 2, 25, 10 and 16% of coil, β-sheet, β-bridge, bend, turn and α-helix, respectively while W275R MT consists of 39, 9, 2, 21, 10 and 19% of coil, β-sheet, β-bridge, bend, turn and α-helix, respectively. Secondary structural components of C318R, N320K MTs exhibited 40, 11, 2, 19, 11, 15 and 43, 9, 2, 22, 10, 14% of coils, β-sheets, β-bridges, bends, turns and α-helices, respectively. And L344F MT contains 42, 8, 2, 21, 10 and 14% of coil, β-sheet, β-bridge, bend, turn and α-helix, respectively (Fig. 2B & Table S4†). DSSP results suggested that flexible moieties such as turns were reduced with increase in the sheets and helices in case of MTs, implying that GATA3 protein acquired rigidity and less flexibilities upon mutations, thus led to destabilisation of secondary structures, which were also corroborated with RMSF results.
Hydrogen bonds (H-bond) play a major role in formation of protein secondary structures and aid in protein ligand binding. Intra H-bonding or protein–protein H-bonds contribute to the protein structure integrity while inter H-bonding or protein-water H-bonds provide stability of protein ligand complex.22 Here, we calculated both intra and inter H-bonds and observed that all MTs exhibited high number of intra H-bonds as compared to WT and low number of inter H-bonds than WT (ESI Fig. S5†). Average intra H-bonds of WT and W275R, C318R, N320K and L344F MTs were 63.9, 72.95, 64.24, 61.43 and 65.77, respectively, inter H-bonds of WT and W275R, C318R, N320K and L344F MTs were 271.94, 253.16, 267.14, 279.97 and 266.17, respectively. The increase in protein–protein H-bonds in W272R, C318R and L344F MTs indicated MTs had acquired highly stabled structural moieties, thud led to increase in the overall structure rigidity (ESI Fig. S5A†). Conversely, decreased in protein–water H-bonds in W275R, C318R and L344F MTs indicated MTs had less protein ligand binding capacities, which in turn loss of overall protein ligand stability (ESI Fig. S5B†). Structural properties analyses of WT and MTs GATA3 were suggested that GATA3 protein had reduced surface area, acquired high rigid structure configuration and reduced protein interaction toward ligand upon mutations.
3.5 Collective motions of WT and MTs GATA3
To assess the dominant motions and conformational changes that were induced due to mutations, we performed essential dynamics or principle component analysis (PCA) on last 25 ns (75–100 ns) stabled MDS trajectories of each system. Initially, low values of cosine content (≤0.2) were obtained to ensure the motions were not due to random diffusion (ESI Table S5†). PCA of WT and MTs indicated that the first few principle components (PCs) or eigenvectors (10–15) had >1 nm2 eigenvalues (Fig. 3A and B). The diagonalized co-variance matrix trace values of WT and W275R, C318R, N320K and L344F MTs were 386.56, 196.18, 178.54, 366.9 and 400.9 nm2, respectively (ESI Table S6†). Low trace values of W275R, C318R, N320K MTs relative to the WT indicated that these MTs have less mobile or more rigid than WT. Cumulative percentage of mean square fluctuations for first 10 eigenvectors (or PCs) were 93.26, 93.04, 92.02, 94.51 and 94.52% for WT, W275R, C318R, N320K and L344F MTs, respectively (Fig. 3A and B; Table S6†). In addition, the first three eigenvectors account for larger motions and fluctuations such as 72.5, 78.9, 75.7, 81.9 and 75.32% for WT, W275R, C318R, N320K and L344F MTs, respectively (ESI Fig. S6 and Table S6†).
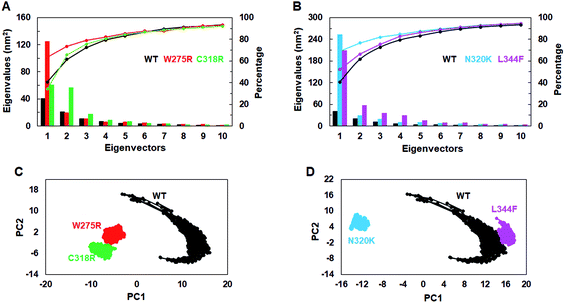 |
| Fig. 3 Collective mode of motions and essential dynamics analyses. (A) and (B) first 15 eigenvectors with different eigenvalues with cumulative percentage of WT and all MTs. (C) and (D) Projection of principle component 1 and 2 of WT and all MTs. Refer Fig. 1 for different colour codes for WT and MTs. | |
The trajectories obtained from first 3 PCs of WT and MTs were projected on the phase space, indicated W275R, C318R, N320K and L344F MTs occupied smaller subspace corresponding to their lower trace values (Fig. 3C and D; ESI Fig. S7†). Furthermore, dynamic nature of WT and MTs were inspected by taking 30 frames from first PC of respective systems and sequentially superimposed to elucidate the variations in collective motions (ESI Fig. S8†). From this, all MTs showed negligible or no motions at N and C finger domains and linker region (ESI Fig. S8B–E†). Unlike MTs, WT displayed larger motions at N and C finger domains and the linker region (ESI Fig. S8A†). Since, motions in the N and C finger domains and linker region of GATA3 is crucial for its function as these motifs assisted in DNA binding, therefore we examined the nature of motions demonstrated by WT and MTs through porcupine structures which were extracted from first PC of respective systems (ESI Fig. S9†). In porcupine structures, length and direction of cone indicated magnitude and direction of motions. WT exhibited larger motions at linker, N and C finger domains. Linker displayed outward motion while both N and C finger domains showed inward and downward motions (ESI Fig. S9A†). On the other hand, no such motions at linker, N and C finger domains were found in MTs indicating MTs restricted the movement of functional motifs of GATA3 and have huge impact on its structural conformation (ESI Fig. S9B–E†). No motions were observed in case of all MTs suggested, these MTs altered structure organisation of GATA3, which may have an impression on the symmetry required for GATA3-DNA complex formation.
The global minimum conformations of different MTs along with WT were studied by assessing the low energy structures through free energy landscape (FEL) analyses. 2D and 3D plots of FEL for WT and MTs were obtained to displayed the different energy barriers (Fig. 4 and ESI Fig. S10†). 2D FEL plots for WT showed two minimal energy clusters and wide structural distribution (Fig. 4A and A′). On the other hand, all MTs showed only one cluster and restricted structural distribution (Fig. 4B–C′′). Moreover, all MTs displayed constraints conformation. FEL analyses of WT, W275R, C318R, N320K and L344F MTs revealed that they access to the lowest energy conformers at 89
290, 96
360, 89
210, 98
180 and 95
870 ps snapshots, respectively that were isolated from the most populated minimum free energy clusters (Fig. 4B–C′′). Importantly, all MTs underwent large conformational changes when aligned with WT. PCA results suggested that all MTs had rigid structures with no motions and displayed constraints conformations as compared to WT, demonstrating that mutations in the finger domains of GATA3 affect its structure and conformation, which led to malfunctioning of GATA3 protein.
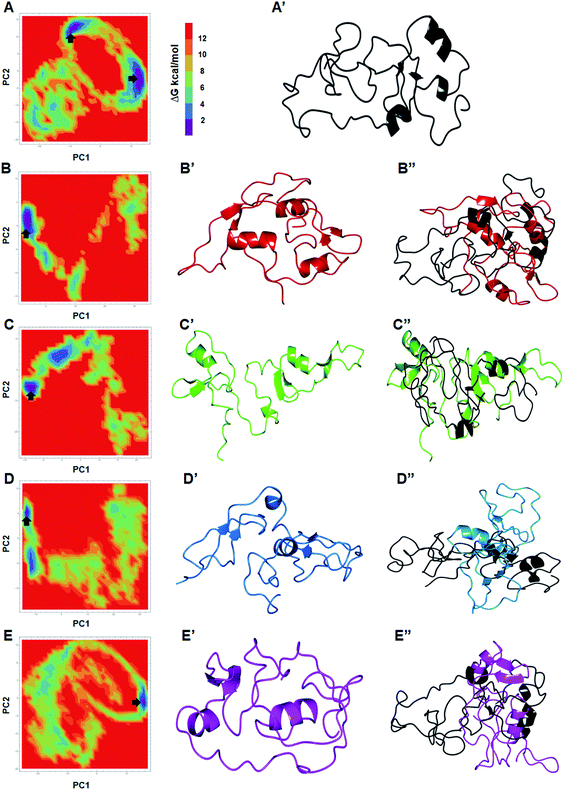 |
| Fig. 4 Free energy landscapes analyses. (A & A′) 2D FEL plot and 3D structure extracted from minimum energy state of WT. (B, B′ & B′′) 2D FEL plot, 3D structure extracted from minimum energy state of W275R and its superimposition with WT structure. (C, C′ & C′′) 2D FEL plot, 3D structure extracted from minimum energy state of C318R and its superimposition with WT structure. (D, D′ & D′′) 2D FEL plot, 3D structure extracted from minimum energy state of N320K and its superimposition with WT structure and (E, E′ & E′′) 2D FEL plot, 3D structure extracted from minimum energy state of L344F and its superimposition with WT structure. ΔG were measured in kilocalorie per mol. Structure were displayed in uniform cartoon mode. | |
3.6 Network centrality and RINs analyses
Residues interaction network analysis was conducted to identify the important residues involved in function of protein.45 To understand the signalling in between the residues and variations existed in WT and all MTs (W275R, C318R, N320K and L344F), betweenness centrality (CB), closeness (CC) and degree (CD) were calculated. Out of this, CB (between centrality) is a crucial parameter for identifying functionally important residues involved in signal transduction within the protein.46 We considered the residues with CB value ≥0.05 in order to understand the differences between WT and all MTs (Fig. 5). WT exhibited 7 residues having CB values ≥0.05 (Fig. 5A and 6A and Table 3), while W275R, C318R, N320K and L344F MTs exhibited 4, 8, 6 and 7 residues with CB values ≥0.05, respectively (Fig. 5B–E, 6B–E and Table 3). Importantly, these residues spanned at the sheet and helix moieties of both N and C finger domains. Interestingly, tryptophan275 (W275) has large effect on the signal transduction as substitution of this residue (W275R) diminished the flow of information on both N and C finger domains of protein (Fig. 6B and Table 3). Also, substitution of asparagine residue (N320K) affected signal strength on C finger domain while N finger domain remained unaffected (Fig. 6D and Table 3). Rest of MTs such as C318R and L344F exhibited similar residues as in WT. Different types of bonding such as hydrogen bonds, van der Waals and ionic interactions involved in WT and MT proteins were also studied by monitoring the interaction of mutant residues to its surrounding residues (Fig. 7 & ESI Table S7†). In this residue network, nodes and edges were represented as residues and different types of bonding, respectively. From the results, it was shown that substitution of tryptophan to arginine (W275R) abolished binding strength as the number of residues involved in interactions were largely reduced (Fig. 7A & ESI Table S7†). Similarly, substitution of cysteine to arginine (C318R) and leucine to phenylalanine (L344F) have also shown in the reduction of binding strength (Fig. 7B and D). In contrast, substitution of asparagine to lysine (N320K) enhanced binding strength as compared to WT as maximum number of interactions were attained (Fig. 7C). Additionally, individual missense variants also affected the overall stabilities of other variants as larger deviations in the RMSDs of WT and all MTs were observed (ESI Fig. S11†). Above results indicated that W275R and N320K variants abolished the residues interaction network, reduced residues signalling and affected overall topology of protein which ultimately affected the structure and function of GATA3. Furthermore, both N and C finger domains amenable for signal transduction by assisting the conformational switching of GATA3 during ligand binding.
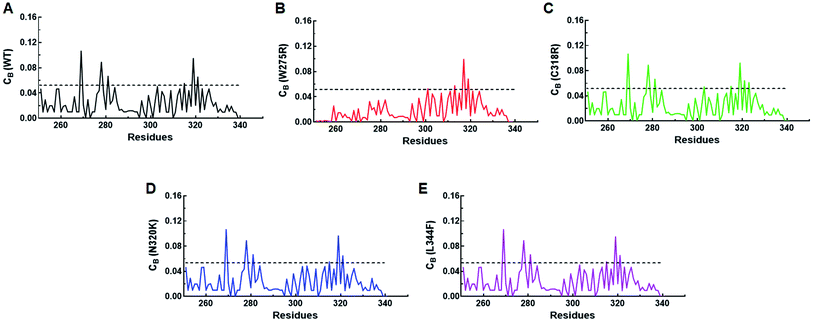 |
| Fig. 5 Network centrality analyses. (A) Betweenness (CB) centrality of WT, (B) betweenness (CB) centrality of W275R, (C) betweenness (CB) centrality of C318R, (D) betweenness (CB) centrality of N320K and (E) betweenness (CB) centrality of L344F. Black solid line denoted the cut-off (≥0.05) value used to select the functionally crucial residues. | |
Table 3 Residues with high CB values in protein network analysis
|
WT |
W275R |
C318R |
N320K |
L344F |
Residues |
W275, C285, C288, C318, N332, V338, N340 |
C318, N332, V338, N340 |
W275, C285, C288, R320, N332, V338, N340, C342 |
W275, C285, C288, N332, V338, N340 |
W275, C285, C288, C318, N332, V338, N340 |
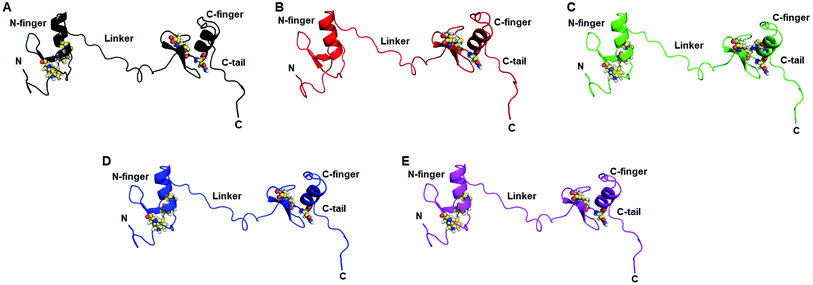 |
| Fig. 6 Network centrality analyses contd. Residues with high CB (≥0.5) were shown as spheres on representative structures of (A) WT, (B) W275R, (C) C318R, (D) N320K and (E) L344F. Structures were displayed in cartoon with uniform colour. | |
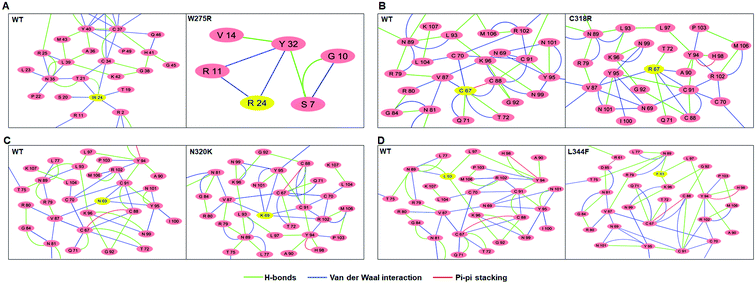 |
| Fig. 7 Residue interaction networks of (A) WT, (B) W275R, (C) C318R, (D) N320K and (E) L344F. In these networks, residues number were started from 1 so W24R, C67R, N69K and L93F correspond to W275R, C318R, N320K and L344F, respectively. Mutant residues were highlighted in yellow colour. | |
4. Discussion
The global burden of breast cancer is arising with increasing incidence in higher age group, after attaining menopause etc.3 At molecular level, various genes such as TP53, PIK3CA, ERBB2, MYC, and GATA3 are known to frequently mutated in breast cancer and largely contributed its progression.5 GATA3 is a transcription factor and its expression in breast suggest its role in growth and differentiation of breast epithelial cells. GATA3 shows clinical relevance in terms of prognosis.2 Expression of GATA3 is more in luminal type breast cancer and it shows good prognosis in comparison to other non-luminal types. Non synonymous substitution in GATA3 can affect not only the structure and stability but also abolish its function.17 Thus, GATA3 acts as a prognostic marker for breast carcinoma and other associated diseases.47 Domain architecture of GATA3 comprises two transcriptional activation (TA1 and TA2) and two N and C finger (containing zinc ions) domains also known as zinc finger domains, that are connected with linker.11 Among these, N finger alone can bind with DNA independently but participation of both N and C fingers in the binding of palindromic GATA motif markedly increased the affinity.48,49 Mutations in the C finger or second Zn finger domain of GATA3 result in loss of DNA binding which contributed in breast cancer.19 Additionally, mutations in first Zn finger or N finger domain also affect the DNA binding affinity and led to development of complex diseases like hypoparathyroidism, sensory neural deafness and renal dysplasia.50 Previous computational study has suggested that mutation in the zinc finger 1 domain of GATA3 induced the conformational changes that led to closure binding of zinc finger 2 domain in wrapping architecture. Study has provided significant insights into the GATA3-DNA binding but due to short simulation time, the conformational study of GATA3 remain limited.51
Experimental study suggested that most of the mutations were clustered at C finger and few were located in N finger domains as reported in breast cancer and other diseased samples.17,18 Additionally, recent large-scale genomic profiling of breast cancer samples has identified various mutations associated with GATA3 gene.9,10 However, molecular consequences of GATA3 mutations are poorly understood due to lacking of studies associated with the function of GATA3 mutants. Experimental testing to reveal the functional consequences of individual GATA3 variants is a promising approach but it is very costly and also time consuming. Hence, computational methods are deemed to be the best approach for characterising the missense variants. Therefore, in the current study, we have elucidated molecular (sequence, structural and functional) consequences of various nonsynonymous missense mutations of GATA3 using computational approaches. Pathogenic effects of different mutations at sequence level were identified through multiples tools. Structural and functional impact of above mutations were examined through MD and essential dynamics simulations. Furthermore, network centrality and residues interaction network studies were conducted to delineated signalling among the residues which provided structure-function relationships of GATA3 upon various mutations.
Single amino acid substitution is a type of non-synonymous mutation that change the amino acid sequence of protein which may lead to alteration in protein structures, stability, conformation and ultimately the function.22 Disease causing or pathogenic mutations are often to be observed in the core or interface residues of protein that participating in structural integrity and ligand interactions.52 Mutations at core regions lead to structure destabilisation while mutations at interface regions affect the binding affinity of protein–ligand (protein/DNA/drug) complex which contributes in the development of disease.53 Hence, missense mutations at functional domain of the protein acts as a driving factor in the progression of disease. Various tools were used to potentiated the consequential analysis of driver non synonymous mutations by providing amino acid sequences of wildtype and mutants. A group of 11 servers were used to predict the deleterious effect of missense mutations (W275R, C318R, N320K and L344F) at sequence level. Our findings showed that all missense mutations were predicted to be deleterious, highly damaging with high prediction rate. Mutational effects on structure and stability of protein were examined through I-mutant, MUpro and MutPred2 servers, and found that all mutant (MT) proteins had decreased stabilities and altered protein structures.
MD simulation gives further insight into the motions of atoms or molecules which in turns reflect the structural integrity, stability and conformational changes arise due to missense mutations in the protein.22 Therefore, MD simulations of 100 ns time were conducted for WT and MT proteins to unravel the effect of mutations on the structure and conformation of GATA3 protein. For MD simulation, 3D structure of all MTs were constructed through hybrid modelling followed by model validations and observed that all MT models had better geometrical properties. During MD simulation, gmx rmsd, gmx rmsf and gmx gyrate modules of Gromacs were used to measure the RMSD, RMSF and Rg of WT and MTs, respectively. Our results suggested that RMSD of WT and MTs were stabilised after ∼70 ns time with higher RMSD values were observed in W275R, N320K and L344F MTs as compared to WT and C318R MT demonstrating that MTs (W275R, N320K and L344F) destabilised the GATA3 protein. To measure the protein structural flexibility, RMSF analysis was performed. Flexibility of protein assistant in folding, ligand binding and to help in acquiring the stable conformation. Neither too flexibility, nor too rigidity or appropriate flexibility/rigidity of protein is required for proper functioning.54 During RMSF analysis, it was observed that WT and L344F MT have higher flexibilities at β-bridges of N and C finger domains and linker region of protein. By contrast, low flexibilities or high rigidities were observed in W275R, N320K and C318R MTs, demonstrating that these MTs reduced the flexibility of GATA3 protein that caused unstable 3D structure. Rg was used to measure the size and compactness of protein due to mutations and found that Rg for WT and MTs were stabilised almost at ∼78 ns time with deceased values in MTs as compared to WT. Low values of Rg in MTs were accompanied with the decreased distance of N- and C-terminals of protein. Hence, mutations in W275, C318, N320 and L344 residues caused reduction in size and globularity of GATA3 protein.
Time dependent structural properties and variations due to mutations were examined through monitoring the solvent accessible surface area (SASA), by performing quantitative and qualitative analyses of secondary structures and the number of hydrogen bond (H-bond) formation during entire simulation period. SASA is an important structural feature of a protein as it measures the interaction surface available for water and/or ligand, thus provide protein ability to interact with other molecules. The interaction of protein is mediated through hydrophobic and hydrophilic residues.55 During SASA analysis, we found that total SASA of MTs W275R: 91.17, C318R: 94.30, and L344F: 92.71 nm2 were reduced as compared to WT (97.13 nm2) and N320K (99.57 nm2). Total reduction of SASA of MTs were accompanied with decreased in hydrophilic SASA. Our findings suggested that SASA of almost all MTs were lower than WT, indicating mutations in GATA3 decreased the interaction capacity of protein which led to destabilisation of protein ligand complexes. Impact of mutations on protein secondary structures were elucidated through DSSP method and observed that flexible moieties such as turns were reduced with concomitantly increased in sheets and coils contents in MTs, articulating that mutations reduced the required flexibility of GATA3 protein and changing the secondary structures configurations, in turn disturbed protein stability. Similar results were also observed during sequence-based analyses of MTs as alterations in protein stability were found. Further, protein and protein ligand stabilities were assessed through formation of protein–protein (intra) and protein–water (inter) H-bonding. During analysis it was observed that intra H-bonds were increased and inter H-bonds were decreased in MTs as compared to WT, implying that MTs acquired rigid structures and having low protein ligand stabilities.
Functions of proteins like binding of substrate, adaptation to different environment, conformational adjustment during binding and allosteric effects are accomplished through certain types of internal motions that enable them to perform different biological functions.56 Essential dynamics or principle components analysis elucidate the essential degree of freedom that describe motions of WT and MT proteins which are relevant to their functions. During ED analyses, we found that MTs showed constraint motions with less essential subspace during phase sampling as compared to WT. Moreover, 3D structures extracted from ED analysis displayed less or negligible motions in the N and C finger domains and linker region of all MTs compared with larger motions in those regions of WT. Furthermore, free energy landscape studies showed that all MTs exhibited only single energy minima as compared to WT that restricted the conformations of MT proteins. Above results demonstrating, MTs of GATA3 affected motions and conformations of protein, consequently altered its functions.
To gain further insights into the structure-function relationships of WT and MT GATA3, we have conducted network centrality and residues interaction network analyses to elucidated the flow of information among different residues. Network centrality was calculated to identified the functionally crucial residues, like those helps in protein allosteric communication, present in the active or binding site of protein, metabolic and diseased networks.45 In this network, three centralities such as betweenness (CB), closeness (CC) and degree (CD) were measured. It was found that the node having high CB values correlates with the functional residues that regulates protein ligand interaction or allosteric signals, indicating the significance of CB over CC and CD.46 Therefore, CB was measured to find out the residues crucial for signalling in WT and all MTs. Network centrality results suggested that the residues located near to sheets and helices of both N and C finger domains were functionally important. In case of few MTs such as W275R and N320K affected the overall distribution of residues resulted the alteration in flow of signal caused function impairment of GATA3. Moreover, residues network analysis results articulated the changing in residues–residue interactions of MT with the surrounding residues indicated, MT residues affected the local topologies of GATA3, in further affected its structure and function.
GATA3 protein comprised 3 domains namely, N-finger, linker and C-finger domains. Appropriate flexibility or mobility is essential for both finger domains for its binding with double helix DNA. N-finger domain spanned around 264–288 amino acid residues and bind with GATG site of DNA.27 In W275R MT, tryptophan which is an aromatic residue, replaced by arginine (R), a basic or positively charge residue located at N-finger domain. Positively charged amino acids such as arginine (R), lysine (L), histidine (H) have significant effect on DNA recognition site and favour binding preference with guanine over other bases. They interact with nucleotide bases via multiple hydrogen bonds which result conformational changes in the protein.57 Therefore, W275R mutation in this region distort the conformation of N-finger domain, which in turn affect its interaction with DNA. On the other hand, C-finger domain spanned around 316–249 amino acid residues with C-tail around 350–366 residues showed conservation during DNA binding mechanism. C-finger domain not only bind with major groove but its C-tail also bind with minor groove of DNA by interacting with N-finger domain.27 Therefore, mutations centred at C-finger domains largely affect the structure and conformation of GATA3 protein. In L344F MT, leucine, a hydrophobic residue changed into phenylalanine (F), also having hydrophobic nature but containing aromatic group that altered the size of protein. Phenylalanine participated in the binding of DNA bases by ring stacking between bases through van der wall interactions, which limits the nucleic acid molecule to gain a proper shape with respect to protein.57 Therefore, L344F MT affect the interaction of C-finger domain of GATA3 with DNA by disrupting the overall structure configuration required during stable binding. Based on the results obtained from this study, it is quite possible that missense mutations of GATA3 affect its structure organisation which ultimately affect its interaction with DNA.
5. Conclusion
In conclusion, the present computational work has explored the mutational effect on structure, dynamics, conformation and function of GATA3. Mutations in N and C finger domains of GATA3 impaired structure, conformation and residues interaction network which might be affect its interaction with DNA that result the development of various malignant phenotypes and associated disorders. Current study has great significance in understanding the molecular basis of malignancies and associated disorders caused and would provide a clue for the development of personalise therapy.
Author contributions
Rakesh K. and P. T. conceptualized and designed the study. Rakesh K. and Rahul K. performed the experiments. S. D., S. M., U. A. and S. H. help in literature study. Rakesh K. and Rahul K. analysed the data and wrote manuscript. P. T. provided laboratory infrastructure. Rakesh K., Rahul K. and P. T. read and approved the final version of manuscript.
Conflicts of interest
There are no conflicts of interest to declare.
Acknowledgements
Rakesh and Rahul thank Indian Council of Medical Research and University Grant Commission, respectively for financial support. This work was supported by Indian Council of Medical Research through grant 5/13/1/TF/AIIMS/2016/NCD-III.
References
- J. D. Figueroa, R. M. Pfeiffer, D. A. Patel, L. Linville, L. A. Brinton, G. L. Gierach, X. R. Yang, D. Papathomas, D. Visscher, C. Mies, A. C. Degnim, W. F. Anderson, S. Hewitt, Z. G. Khodr, S. E. Clare, A. M. Storniolo and M. E. Sherman, Terminal Duct Lobular Unit Involution of the Normal Breast: Implications for Breast Cancer Etiology, J. Natl. Cancer Inst., 2014, 106, 10 CrossRef.
- F. Bray, J. Ferlay, I. Soerjomataram, R. L. Siegel, L. A. Torre and A. Jemal, Global Cancer Statistics 2018: GLOBOCAN Estimates of Incidence and Mortality Worldwide for 36 Cancers in 185 Countries, CA, Ca-Cancer J. Clin., 2018, 68, 394–424 CrossRef.
- K. Rojas and A. Stuckey, Breast Cancer Epidemiology and Risk Factors, Clin. Obstet. Gynecol., 2016, 59, 651–672 CrossRef.
- P. J. Stephens, P. S. Tarpey, H. Davies, P. V. Loo, C. Greenman, D. C. Wedge, S. Nik-Zainal, S. Martin, I. Varela, G. R. Bignell, L. R. Yates, E. Papaemmanui, D. Beare, A. Butler, A. Cheverton, J. Gamble, J. Hinton, M. Jia, A. Jayakumar, D. Jones, C. Latimer, K. W. Lau, S. McLaren, D. J. McBride, A. Menzies, L. Mudie, K. Raine, R. Rad, M. S. Chapman, J. Teague, D. Easton, A. Langerød, OSBREAC, M. T. Lee, C. Y. Shen, B. T. Tee, B. W. Huimin, A. Broeks, A. C. Vargas, G. Turashvili, J. Martens, A. Fatima, P. Miron, S. Chin, G. Thomas, S. Boyault, O. Mariani, S. R. Lakhani, M. Vijver, L. Veer, J. Foekens, C. Desmedt, C. Sotiriou, A. Tutt, C. Caldas, J. S. Reis-Filho, S. A. J. R. Aparicio, A. V. Salomon, A. L. Børresen-Dale, A. L. Richardson, P. J. Campbell, P. A. Futreal and M. R. Stratton, The landscape of cancer genes and mutational processes in breast cancer, Nature, 2012, 486, 400–404 CrossRef CAS.
- Cancer Genome Atlas Network, Comprehensive molecular portraits of human breast tumours, Nature, 2012, 490, 61 CrossRef.
- J. Chou, S. Provot and Z. Werb, GATA3 in Development and Cancer Differentiation: Cells GATA Have It!, J. Cell. Physiol., 2010, 222, 42–49 CrossRef CAS.
- D. L. Bates, Y. Chen, G. Kim, L. Guo and L. Chen, Crystal Structures of Multiple GATA Zinc Fingers Bound to DNA Reveal New Insights into DNA Recognition and Self-Association by GATA, J. Mol. Biol., 2008, 381, 1292–1306 CrossRef CAS.
- R. Zheng and G. A. Blobel, GATA Transcription Factors and Cancer, Genes, Chromosomes Cancer, 2010, 1, 1178–1188 CrossRef CAS.
- C. Atayar, S. Poppema, T. Blokzijl, G. Harms, M. Boot and A. Berg, Expression of the T-Cell Transcription Factors, GATA-3 and T-bet, in the Neoplastic Cells of Hodgkin Lymphomas, Am. J. Pathol., 2005, 166, 1 CrossRef.
- A. Gulbinas, P. O. Berberat, Z. Dambrauskas, T. Giese, N. Giese, F. Autschbach, J. Kleeff, S. Meuer, M. W. Buchler and H. Friess, Aberrant Gata-3 Expression in Human Pancreatic Cancer, J. Histochem. Cytochem., 2006, 54, 161–169 CrossRef CAS.
- H. W. Tun, L. A. Marlow, C. A. Roemeling, S. J. Cooper, P. Kreinest, K. Wu, B. A. Luxon, M. Sinha, P. Z. Anastasiadis and J. A. Copland, Pathway Signature and Cellular Differentiation in Clear Cell Renal Cell Carcinoma, PloS One, 2010, 5, e10696 CrossRef.
- T. Sørlie, R. Tibshirani, J. Parker, T. Hastie, J. S. Marron, A. Nobel, S. Deng, H. Johnsen, R. Pesich, S. Geisler, J. Demeter, C. M. Perou, P. E. Lønning, P. O. Brown, A. L. Børresen-Dale and D. Botstein, Repeated observation of breast tumor subtypes in independent gene expression data sets, Proc. Natl. Acad. Sci. U.S.A., 2003, 100, 8418–8423 CrossRef.
- V. Theodorou, R. Stark, S. Menon and J. S. Carroll, GATA3 acts upstream of FOXA1 in mediating ESR1 binding by shaping enhancer accessibility, Genome Res., 2013, 23, 12–22 CrossRef CAS.
- Y. Z. Jiang, K. Yu, W. J. Zuo, W. T. Peng and Z. M. Shao, GATA3 Mutations Define a Unique Subtype of Luminal-Like Breast Cancer With Improved Survival, Cancer, 2014, 120, 1329–1337 CrossRef CAS.
- M. A. Nesbit, M. R. Bowl, B. Harding, A. Ali, A. Ayala, C. Crowe, A. Dobbie, G. Hampson, I. Holdaway, M. A. Levine, R. Mc Williams, S. Rigden, J. Sampson, A. J. Williams and R. V. Thakker, Characterization of GATA3 Mutations in the Hypoparathyroidism, Deafness, and Renal Dysplasia (HDR) Syndrome, J. Biol. Chem., 2004, 279, 22624–22634 CrossRef CAS.
- K. Muroya, T. Hasegawa, Y. Ito, T. Nagai, H. Isotani, Y. Iwata, K. Yamamoto, S. Fujimoto, S. Seishu, Y. Fukushima, Y. Hasegawa and T. Ogata, GATA3 abnormalities and the phenotypic spectrum of HDR syndrome, J. Med. Genet., 2001, 38, 374–380 CrossRef CAS.
- J. Usary, V. Llaca, G. Karaca, S. Presswala, M. Karaca, X. He, A. Langer, R. Karesen, D. S. Oh, L. G. Dressler, P. E. Lonning, R. L. Strausberg, S. Chanock, A. L. B. Dale and C. M. Perou, Mutation of GATA3 in human breast tumors, Oncogene, 2004, 23, 7669–7678 CrossRef CAS.
- A. B. Adomas, S. A. Grimm, C. Malone, M. Takaku, J. K. Sims and P. A. Wadem, Breast tumor specific mutation in GATA3 affects physiological mechanisms regulating transcription factor turnover, BMC Canc., 2014, 14, 278 CrossRef.
- M. Takaku, S. A. Grimm, J. D. Roberts, K. Chrysovergis, B. D. Bennett, P. Myers, L. Perera, C. J. Tucker, C. M. Perou and P. A. Wade, GATA3 zinc finger 2 mutations reprogram the breast cancer transcriptional network, Nat. Commun., 2018, 9, 1–14 CrossRef CAS.
- The UniProt Consortium, UniProt: the universal protein knowledgebase, Nucleic Acids Res., 2017, 45, D158–D169 CrossRef.
- J. Bendl, J. Stourac, O. Salanda, A. Pavelka, E. D. Wieben, J. Zendulka, J. Brezovsky and J. Damborsky, PredictSNP: Robust and Accurate Consensus Classifier for Prediction of Disease-Related Mutations, PLoS Comput. Biol., 2014, 10, e1003440 CrossRef.
- R. Kumar, R. Kumar, P. Tanwar, G. K. Rath, R. Kumar, S. Kumar, N. Dash, P. Das and S. Hussain, Deciphering the impact of missense mutations
on structure and dynamics of SMAD4 protein involved in pathogenesis of gall bladder cancer, J. Biomol. Struct. Dyn., 2020, 1–15 Search PubMed.
- H. Tang and P. D. Thomas, PANTHER-PSEP: predicting disease-causing genetic variants using position-specific evolutionary preservation, Bioinformatics, 2016, 32, 2230–2232 CrossRef CAS.
- Y. Choi and A. P. Chan, PROVEAN web server: a tool to predict the functional effect of amino acid substitutions and indels, Bioinformatics, 2015, 31, 2745–2747 CrossRef CAS.
- H. A. Shihab, J. Gough, D. N. Cooper, P. D. Stenson, G. L. A. Barker, K. J. Edwards, I. N. M. Day and T. R. Gaunt, Predicting the Functional, Molecular, and Phenotypic Consequences of Amino Acid Substitutions using Hidden Markov Models, Hum. Mutat., 2013, 34, 57–65 CrossRef CAS.
- B. Reva, Y. Antipin and C. Sander, Predicting the functional impact of protein mutations: application to cancer genomics, Nucleic Acids Res., 2011, 39, e118 CrossRef CAS.
- Y. Chen, D. L. Bates, R. Dey, P. Chen, A. C. D. Machado, I. A. L. Offringa, R. Rohs and L. Chen, DNA Binding by GATA Transcription Factor Suggests Mechanisms of DNA Looping and Long-Range Gene Regulation, Cell Rep., 2012, 2, 1197–1206 CrossRef CAS.
- P. W. Rose, B. Beran, C. Bi, W. F. Bluhm, D. Dimitropoulos, D. S. Goodsell, A. Prlic, M. Quesada, G. B. Quinn, J. D. Westbrook, J. Young, B. Yukich, C. Zardecki, H. M. Berman and P. E. Bourne, The RCSB Protein Data Bank: redesigned web site and web services, Nucleic Acids Res., 2011, 39, D392–D401 CrossRef CAS.
- Y. Zhang, I-TASSER server for protein 3D structure prediction, BMC Bioinf., 2008, 9, 40 CrossRef.
- R. Kumar, R. Maurya and S. Saran, Introducing a simple model system for binding studies of known and novel inhibitors of AMPK: a therapeutic target for prostate cancer, J. Biomol. Struct. Dyn., 2019, 37, 781–795 CrossRef CAS.
- J. C. Gordon, J. B. Myers, T. Folta, V. Shoja, L. S. Heath and A. Onufriev, H++: a server for estimating pKas and adding missing hydrogens to macromolecules, Nucleic Acids Res., 2005, 33, W368–W371 CrossRef CAS.
- R. A. Laskowski, M. W. MacArthur, D. S. Moss and J. M. Thornton, PROCHECK: a program to check the stereochemical quality of protein structures, J. Appl. Crystallogr., 1993, 26, 283–291 CrossRef CAS.
- M. Wiederstein and M. J. Sippl, ProSA-web: interactive web service for the recognition of errors in three-dimensional structures of proteins, Nucleic Acids Res., 2007, 35, W407–W410 CrossRef.
- P. Benkert, S. C. E. Tosatto and D. Schomburg, QMEAN: A comprehensive scoring function for model quality assessment, Proteins, 2008, 71, 261–277 CrossRef CAS.
- D. V. D. Spoel, E. Lindahl, B. Hess, G. Groenhof, A. E. Mark and H. J. C. Berendsen, GROMACS: fast, flexible, and free, J. Comput. Chem., 2005, 26, 1701–1718 CrossRef.
- C. Oostenbrink, A. Villa, A. E. Mark and W. F. V. Gunsteren, A biomolecular force field based on the free enthalpy of hydration and solvation: the GROMOS force-field parameter sets 53A5 and 53A6, J. Comput. Chem., 2004, 25, 1656–1676 CrossRef CAS.
- A. Amadei, A. B. M. Linssen and H. J. C. Berendsen, Essential Dynamics of Proteins, Proteins, 1993, 17, 412–425 CrossRef CAS.
- R. Kumar and S. Saran, Structure, molecular dynamics simulation, and docking studies of Dictyostelium discoideum and human STRAPs, J. Cell. Biochem., 2018, 119, 7177–7191 CrossRef CAS.
- B. Hess, Similarities between principal components of protein dynamics and random diffusion, Phys. Rev. E: Stat., Nonlinear, Soft Matter Phys., 2000, 62, 8438–8448 CrossRef CAS.
- D. Piovesan, G. Minervini and S. C. E. Tosatto, The RING 2.0 web server for high quality residue interaction
networks, Nucleic Acids Res., 2016, 44, W367–W374 CrossRef CAS.
- P. Shannon, A. Markiel, O. Ozier, N. S. Baliga, J. T. Wang, D. Ramage, N. Amin, B. Schwikowski and T. Ideker, Cytoscape: A Software Environment for Integrated Models of Biomolecular Interaction Networks, Genome Res., 2003, 13, 2498–2504 CrossRef CAS.
- U. Brandes, A faster algorithm for betweenness centrality, J. Math. Sociol., 2001, 25, 163–177 CrossRef.
- N. T. Doncheva, Y. Assenov, F. S. Domingues and M. Albrecht, Topological analysis and interactive visualization of biological networks and protein structures, Nat. Protoc., 2012, 7, 670 CrossRef CAS.
- W. Kabsch and C. Sander, Dictionary of Protein Secondary Structure: Pattern Recognition of Hydrogen-Bonded and Geometrical Features, Biopolymers, 1983, 22, 2577–2637 CrossRef CAS.
- G. Amitai, A. Shemesh, E. Sitbon, M. Shklar, D. N. I. Venger and S. Pietrokovski Network, Analysis of Protein Structures Identifies Functional Residues, J. Mol. Biol., 2004, 344, 1135–1146 CrossRef CAS.
- T. E. Saldaño, S. C. E. Tosatto, G. Parisi and S. F. Alberti, Network analysis of dynamically important residues in protein structures mediating ligand-binding conformational changes, Eur. Biophys. J., 2019, 48, 559–568 CrossRef.
- A. S. Fararjeh, S. H. Tu, L. C. Chen, Y. Liu, Y. K. Lin, H. L. Chang, H. W. Chang, C. H. Wu, W. W. H. Verslues and Y. S. Ho, The impact of the effectiveness of GATA3 as a prognostic factor in breast cancer, Hum. Pathol., 2018, 80, 219–230 CrossRef CAS.
- K. U. Gaynor, I. V. Grigorieva, M. D. Allen, C. T. Esapa, R. A. Head, P. Gopinath, P. T. Christie, M. A. Nesbit, J. L. Jones and R. V. Thakker, GATA3 mutations found in breast cancers may be associated with aberrant nuclear localization, reduced transactivation and cell invasiveness, Horm. Cancer, 2013, 4, 123–139 CrossRef CAS.
- B. Mair, T. Konopka, C. Kerzendorfer, K. Sleiman, S. Salic, V. Serra, M. K. Muellner, V. Theodorou and S. M. Nijman, Gain-and loss-of-function mutations in the breast cancer gene GATA3 result in differential drug sensitivity, PLoS Genet., 2016, 12, e1006279 CrossRef.
- H. V. Esch, P. Groenen, M. A. Nesbit, S. Schuffenhauer, P. Lichtner, G. Vanderlinden, B. Harding, R. Beetzk, R. W. Bilous, I. Holdaway, N. J. Shaw, J. P. Fryns, W. V. de Ven, R. V. Thakker and K. Devriendt, GATA3 haplo-insufficiency causes human HDR syndrome, Nature, 2000, 406, 419–422 CrossRef.
- R. Karn and I. A. Emerson, Breast cancer mutation in GATA3 zinc finger 1 induces conformational changes leading to the closer binding of ZnFn2 with a wrapping architecture, J. Biomol. Struct. Dyn., 2020, 38, 1810–1821 CAS.
- J. Andreani, G. Faure and R. Guerois, Versatility and invariance in the evolution of homologous heterometric interfaces, PLoS Comput. Biol., 2012, 8, e1002677 CrossRef CAS.
- M. Guharoy and P. Chakrabarti, Conservation and relative importance of residues across protein-protein interfaces, Proc. Natl. Acad. Sci. U.S.A., 2005, 102, 15447–15452 CrossRef CAS.
- P. Craveur, A. P. Joseph, J. Esque, T. J. Narwani, F. Noël, N. Shinada, M. Goguet, S. Leonard, P. Poulain, O. Bertrand, G. Faure, J. Rebehmed, A. Ghozlane, L. S. Swapna, R. M. Bhaskara, J. Barnoud, S. Téletchéa, V. Jallu, J. Cerny, B. Schneider, C. Etchebest, N. Srinivasan, J. C. Gelly and A. Brevern, Protein flexibility in the light of structural alphabets, Front. Mol. Biosci., 2015, 2, 20 Search PubMed.
- Md. Aftabuddin and S. Kundu, Hydrophobic, hydrophilic, and charged amino acid networks within protein, Biophys. J., 2007, 93, 225–231 CrossRef CAS.
- I. Daidone and A. Amadei, Essential dynamics: foundation and applications, Wiley Interdiscip. Rev.: Comput. Mol. Sci., 2012, 5, 762–770 Search PubMed.
- N. M. Luscombe, R. A. Laskowski and J. M. Thornton, Amino acid–base interactions: a three-dimensional analysis of protein–DNA interactions at an atomic level, Nucleic Acids Res., 2001, 29, 2860–2874 CrossRef CAS.
Footnote |
† Electronic supplementary information (ESI) available. See DOI: 10.1039/d0ra07786k |
|
This journal is © The Royal Society of Chemistry 2020 |
Click here to see how this site uses Cookies. View our privacy policy here.