Unique calibration of passive air sampling for field monitoring of PAHs with polyethylene thin films across seasons and locations†
Received
23rd December 2020
, Accepted 5th May 2021
First published on 17th May 2021
Abstract
Although passive sampling has been applied to determine concentrations of semi-volatile organic chemicals such as polycyclic aromatic hydrocarbons (PAHs) in air and water for about two decades and is well established, several uncertainties and challenges remain. This study presents a comprehensive validation of polyethylene thin sheets as passive air samplers (PE-PAS), detailing alternative approaches to calibration and analysis. Besides measuring the uptake kinetics in the field using time series deployments of PE-PAS at 3 field sites under varying seasonal conditions (temperature, wind) with a performance reference compound (PRC), we implement a numerical model to fit uptake kinetics based on partition and diffusion coefficients adapted to hourly changes of temperature. This model follows similar approaches as reported in earlier studies, but we use loss curves of a spiked PRC (anthracene-D10) to fit the air-side boundary layer (δg), which is limiting mass transfer between air and PE, instead of calibrating the PAS sampling rate. Surprisingly, air side boundary layers, and thus inherent sampling rates, were almost equal at the different locations but varied for the different sampling campaigns by about a factor of two. This variation is probably due to short term effects, but a robust average over all seasons and locations could be obtained (1 mm ± 0.5 mm) which is characteristic for our sampling design. A well-known δg allows concentrations in air of compounds with very different uptake kinetics onto the PE-PAS to be estimated. Additionally, as in previous studies, our results indicate stable distribution patterns of PAHs in the environment of the study region in Germany. Given a practical chosen PE-PAS deployment time, representative compounds may be selected that integrate over the desired monitoring time period (e.g. fluorene for days, phenanthrene for weeks and pyrene or fluoranthene for many months to years). Measurement of one PAH applied with specific ratios derived from stable distribution patterns of PAHs, in the absence of influential local point sources, can then be used to estimate air concentrations of PAHs that are more difficult to quantify during the monitoring period.
Environmental significance
The investigation of Polycyclic Aromatic Hydrocarbons (PAHs) in air in general is environmentally relevant, since (i) PAHs are characterized as a class of contaminants with special concern due to their toxic behaviour and relatively long persistence and (ii) both emission and transport with subsequent distribution of these compounds in the environment mainly occur in the air. In our study we provide a method to determine PAH concentrations in air that can be easily, reproducibly and reliably applied. Thus, we aim for an improvement of the widely used passive air sampling of PAHs.
|
1. Introduction
Determining atmospheric concentrations of the broad range of chemicals being introduced into the environment is important because many chemicals show extreme persistence and potential for long-range transport, reaching even remote areas, and monitoring can improve our understanding of environmental fate, human and environmental health risks, and regulatory actions.1–3 Application of passive samplers has increased remarkably during the last years to detect contaminants in various environmental media,4–6 including in air as reviewed by Wania & Shunthirasingham.7 Passive sampling applications are diverse and include the study of contaminant spatial and temporal distribution over various scales as well as to determine cross-compartment fugacity gradients that enable observation of fluxes.8–13 In particular, in remote areas and for long-term sampling campaigns, passive air sampling (PAS) gets more attractive than active air sampling due to high effort for maintenance and energy supply.14 Application of PAS thus far is mainly semi-quantitative15 or used to examine prevailing flux direction rather than fluctuation in rates16,17 and still several uncertainties exist.7
The theory of passive sampling is well described in literature.4,18–22 Following a double-film diffusion conceptual model,23 equilibrium conditions exist at the interface of a PAS and air, with concentration gradients evolving on both sides leading to boundary layers at the air-side (δg) and the sampler-side (δp) which act as mass transfer resistances.21 Because of relatively high partitioning coefficients (Kpg), it is widely accepted that the air-side boundary layer is controlling the uptake process of semi- and low volatile atmospheric compounds onto various polymer passive samplers depending on deployment set-up.4,19,22,24 Uptake onto the passive sampler occurs in three phases: kinetic (linear), intermediate (curvilinear) and dynamic equilibrium (plateau).4,25 For the translation of measured concentrations on passive air samplers into gas concentrations in the atmosphere, the sampled uptake phase of the target compounds needs consideration; if the uptake of target compounds onto passive air samplers exceeds the linear phase but does not yet reach equilibrium between air and sampler, information on both the uptake kinetics and equilibrium (i.e. the partition coefficient) needs to be known.25,26 Both the exchange kinetics and the partition coefficient are influenced by environmental conditions; Kpg varies with temperature, while the kinetics are additionally influenced by the wind speed. For instance, high wind speeds result in thinner boundary layers and thus in faster kinetics meaning higher sampling rates and shorter equilibration time scales.27,28
Calibrating passive sampling kinetics in the lab or additionally using active samplers in the field is a commonly used approach but cannot account for varying atmospheric conditions.28–30 Therefore, often several performance reference compounds (PRCs) are used for in situ calibration of passive samplers.27,31,32 Based on the measured loss of PRCs the uptake of target compounds on PAS is calibrated with mathematical models (see below). Although PRC loss is a widely applied method for calibrating exchange kinetics in passive sampling, few studies focus on a quantification of the air-side boundary layer parameter.
Polycyclic aromatic hydrocarbons (PAHs) are a class of contaminants of concern due to being potential human carcinogens as well as exerting other toxicities, and having relatively long half-lives. They typically occur in much higher concentration in soils, sediments and the atmosphere than other persistent organic compounds. Anthropogenic emission of PAHs is mainly caused by the incomplete combustion of fuels, so typical emission sources are industrial processes, biomass/waste combustion, domestic heating and traffic.33–36 PAHs occur as mixtures and their physico-chemical properties range over orders of magnitude, which poses a challenge for environmental sampling. PAHs may undergo long range transport, they are stored and re-volatilized from terrestrial compartments37–39 and surface seawater12,40 and re-enter the atmosphere. Diffuse sources and mixing processes lead to rather stable distribution patterns of PAHs in air which may remain relatively constant in time and space for a region without strong influence of specific primary sources. Such stable characteristic ratios of individual PAHs sensed by PAS might be applied as a new approach to extrapolating measured concentrations of less volatile target compounds that require longer integration times to be detected on the sampler. This should enable concentration estimates for compounds that are routinely difficult to measure and quantify.
The objectives of this study were to (i) gain understanding of the kinetics and time scales for passive sampling of PAHs in air with PE thin sheets under field conditions in different seasons, (ii) determine air-side boundary layer thicknesses using numerical models to calculate concentrations of PAHs in air, and (iii) analyse the suitability of PAH distribution patterns to reduce actual pollutant measurements to one indicator PAH. Fluorene (Fln), phenanthrene (Phe), fluoranthene (Fth) and pyrene (Pyr) were chosen as representative PAHs within this study based on their partition coefficients (PE-air), as they cover a broad range of almost two log units (Table 1), and because they are commonly detected at relatively high concentrations on the sampler and thus offer reliability and lower uncertainty for measurements. Pyr is the only high molecular weight PAH which could be detected in sufficiently high concentrations during all monitoring periods. Anthracene-D10 (Ant-D10) was used as a performance reference compound for the planned deployments since it shows a significant release (>50%) from the sampler within a few days. Ant-D10 does not occur in the atmosphere and has similar physicochemical properties as phenanthrene, the most abundant PAH. We chose to study PE thin sheets because of their relative low cost, simplicity in handling in the field and the lab, and demonstrated promise for use as a PAS in previous studies.22,41 However, use of PE sheets may have disadvantages derived from its relatively low capacity in comparison to infinite sink samplers, which complicates the data evaluation in terms of the uptake phase of different compounds and their integration time span.
Table 1 Properties of fluorene (Fln), phenanthrene (Phe), fluoranthene (Fth) and pyrene (Pyr) (representative PAHs) and anthracene-D10 (Ant-D10) used as performance reference compound: molar volume (Vm), molecular weight (MW), diffusion coefficient in PE (p), diffusion coefficient in gas phase (Dg), polyethylene/gas phase partition coefficient (Kpg at 25 °C) and enthalpy of vaporization (ΔHvap)
Compound |
V
m [cm3 mol−1] |
MW [g mol−1] |
log Dpa [m2 s−1] |
log Dg [m2 s−1] |
log Kpgac [L kg−1] |
ΔHvapb [kJ mol−1] |
Lohmann.42
Roux, Temprado.36
K
pg has uncertainties of approx. 0.4 log units for the different approaches to determine this value.20,42,43
|
FLN |
193.9 |
166.22 |
−12.36 |
−5.23 |
6.3 |
72.1 |
Ant-D10 |
190.2 |
178.23 |
−12.44 |
−5.22 |
7.1 |
79.9 |
PHE |
190.2 |
178.23 |
−12.44 |
−5.22 |
7.0 |
78.3 |
FTH |
223.2 |
202.25 |
−12.96 |
−5.26 |
8.1 |
87.1 |
PYR |
203 |
202.25 |
−12.76 |
−5.24 |
8.1 |
89.4 |
2. Materials and methods
2.1. Air sampling
Materials.
Commercial low-density polyethylene (LDPE, or short: PE) sheets of 80 μm thickness were used as passive samplers (JUFOL GmbH). All solvents used for extraction had a certified purity of 99.8% and were supplied by Merck Millipore (Darmstadt, Germany). Ultrapure water was obtained by a water purification system (Gen Pure Pro UV-TOC, Thermo Scientific). The external (PAH Mix 14, Dr Ehrenstorfer GmbH) and internal standards (PAH Mix 31, Dr Ehrenstorfer GmbH) had a certified purity of >98%, and both were used for quantification of the target compounds. The deuterated anthracene (Ant-D10, Dr Ehrenstorfer GmbH) had a certified purity of 99.0%.
Preparation of the PE passive samplers.
Sheets of PE thin film were cut into strips of 9 × 25 cm and perforated at two spots to allow easy and fast deployment on hangers in the field. For precleaning, PE was shaken for 24 h in cyclohexane followed by 24 h in ethylacetate followed by several rinsing steps with ultrapure water. In advance of the deployment, samplers were spiked with deuterated anthracene (Ant-D10) as PRC using a procedure adapted from Booij.44 In brief, PE was placed in a spiked solution of ultrapure-water
:
methanol (80
:
20% vol) with masses optimized to achieve a desired Ant-D10 starting concentration and mixed for seven days on an overhead shaker. The prepared PE-PAS were taken out of the spiking solution, wiped dry of solution and wrapped thoroughly in aluminium foil right before transportation into the field.
Deployment, sampling and extraction.
Three study sites (Entringen, Poltringen, Tailfingen – see Fig. S1†) in the hinterland of Stuttgart in southwestern Germany were chosen for regular monitoring campaigns. All sites were formerly or recently used for agriculture and local point sources of PAHs could be mostly excluded. In the field, PE-PAS were deployed within an open-bottomed aluminium box cover at 1.2 m height to reduce exposure to wind, direct sunlight and dust (Fig. S2†) Samplers (N = 21) were placed in two rows within one box with 4.5 cm distance between them. Sampling campaigns were initiated on 3-May 2016, 1-August 2016, 1-November 2016, 3-February 2017, 1-May 2017, 1-August 2017, 3-November 2017 and 1-February 2018. At the beginning of each sampling campaign, samples were taken in a high resolution (12, 24, 48, 72, 120 hours), followed by 10, 20 and 30 days (and 36 days for February 2017) to monitor the uptake kinetics of the target compounds and the loss of the PRC (during the second year of sampling, the sampling resolution varied slightly with an additional sampling after 15 days). At sampling, the PE was rinsed with ultrapure water and wiped dry with lint-free tissue to remove potential particles. For the extraction, PE was shaken with 50 mL of ethylacetate twice for 24 hours. Internal standard (PAH-Mix 31 deuterated, Dr Ehrenstorfer GmbH) was added to the combined extracts before reducing the volume to 10 mL using a rotational evaporator (at 40 °C and 215 mbar). In preliminary analyses we observed the clearest chromatograms, without superimposing peaks, when extracts were mixed with 800 mL ultrapure water before solvent exchange with an addition of 10 mL of cyclohexane shaken for 30 minutes. Subsequently, the solvent phase was transferred into clean vials, heated to 40 °C and reduced under a gentle nitrogen stream to 200 μL.
Active sampling.
Active sampling was performed over 3–5 days periods twice during November–December 2017 and twice during February 2018, aiming to sample about 4 m3 of air each time to provide enough gaseous PAHs for reliable quantification. Due to logistical reasons, active sampling was done in triplicates in Entringen instead of at each site. Personal air sampling pumps (Gilian 5000) were set at a flow rate of 1 L min−1, which was calibrated using a flow-through-meter (Rotameter, Fisher Bioblock). Since there was no power supply at the sampling site, pumps were brought into the lab every 16 hours for battery-recharge, and thus stopped regularly for intervals of up to 8 hours. Cartridges (2.5 cm in diameter) containing one glass fibre filter (GFF) (0.45 μm, Whatman) and two subsequent polyurethane foam (PUF) filters of 1 cm thickness each (TISCH Environmental) were used. Before deployment, the PUF filters were cleaned by Soxhlet extraction with acetone for 12 hours. After the sampling, all glass fibre and polyurethane filters were extracted separately with an Accelerated Solvent Extractor (ASE) using ethylacetate as solvent at 85 °C during 3, 20 min extraction cycles. Internal standard was added into the extracts before shaking with ultrapure water and transferring into cyclohexane in the process described above.
Quality control and uncertainty.
Several types of material controls were performed to check for background contamination. Precleaned, PRC-spiked PE-PAS were analyzed by extracting samplers in triplicate at the time of preparation. Additionally, to determine potential contamination during transport, triplicate field blanks taken to each field site on the day of deployment set-up were analyzed. Blank PUF filters were extracted to assess the potential for lab contamination right after the clean-up, and GFF as well as PUF field blanks were sampled from cartridges that were not attached to pumps but otherwise handled the same as the samples taken to field sites. Concentrations measured on PE-PAS, GFF and PUF during the monitoring period were corrected by the respective lab and field blanks.
Measurement uncertainties that propagate in the model were considered. Internal and external standard variation may affect target analyte quantitation by up to 10%. Manual integration of peak areas introduces another uncertainty, which gets most pronounced for small peaks and can reach up to 20%. Based on those observations, the confidence interval for comparing different sets of concentrations was generally set to ±30%. The relative standard deviation of the replicate samples was generally below 15%, with some exceptions for small concentrations. As expected, the standard deviation increased when combining the three locations, which was again most significant for low concentrations and high temperatures.
2.2. Analysis and evaluation
Measurement.
Concentrations of the target compounds were determined by gas chromatography with coupled mass spectrometry (Hewlett Packard [HP] 68909 and HP 5973) using a 25 m long capillary column (25 μm inner diameter, 0.25 μm thick film of 5% phenyl- and 95% dimethyl-polysiloxane; J&W Scientific). Samples were injected in pulsed splitless mode. The temperature program started with an initial temperature of 65 °C for 4 minutes, with temperature ramped 10 °C min−1 to 270 °C, held constant for 10 minutes, and then ramped again at the same rate to 310 °C and held for 6 minutes. Helium was used as inert carrier gas with a constant flow rate of 0.7 mL min−1. The mass spectrometer was operated in selected ion monitoring mode.
Mathematical models and data evaluation.
Our data evaluation follows the common approach of a first order exponential equation to describe the exchange kinetics between sampler (PE) and sampled medium (air).12,19,31,45 The loss of PRCs over time is defined by the loss rate constant ke [s−1] and can be described as: | CPRC,t = CPRC,te(−ket) | (1.1) |
CPRC,t [ng g−1] denotes the concentration of the PRC on the passive sampler at time t [s] and CPRC,0 [ng g−1] is the initial concentration. ke is obtained by fitting the slope of the release curves of the PRC15,31,42 and depends on the partition coefficient (PE-air), the geometry of the passive sampler as well as on mass transfer, i.e. diffusion through the air side boundary layer which is limiting for thin sheets and compounds with KOA > 106,46 as observed in earlier studies.47 The loss rate constant for the PRC is: | 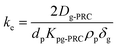 | (1.2) |
where Dg-PRC, Kpg-PCR, ρp and dp denote the diffusion coefficient in gas phase [m2 s−1] and the partition coefficient between sampler and air [L kg−1] of the PRC, and the density [kg L−1] and the thickness of the passive sampler [m], respectively. 2/dp represents the specific surface area of a thin sheet [m2 m−3]. This approach is equivalent to earlier studies, which use the inherent sampling rates (RS) of passive samplers as RS = Dg/δgAs (with As as the surface area of the samplers). The difference in our approach is that we account for the area and Dg separately. For compounds with similar diffusivities, the air-side boundary layer is independent of the target compound and can be determined from ke of the PRC: | 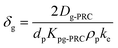 | (1.3) |
If δg is known, individual uptake rate constants (kup [s−1]) can be calculated using 1.2 but for diffusion and partitioning coefficients for each target compound, assuming a stable δg in the sampling period. Uptake of the target compounds onto the passive sampler is: | 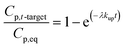 | (1.4) |
Atmospheric concentrations can also be calculated with the analytical solution,12,13,31 adapting eqn (1.4) accordingly to:
| 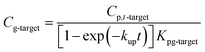 | (1.5) |
Note that for early times the argument of the exponential function is very small and may be used directly (1 − exp(−x) = x); thus, in this early stage the uptake of a compound is linear and becomes independent on the partitioning of the compound or the temperature (Kpg drops out).
The rate constant allows comparison of the sampled time spans for the individual compounds since the characteristic times to reach 63% equilibrium may be estimated as the inverse of the respective rate constant:
|  | (1.6) |
This characteristic time is chosen for convenience of calculation and does not represent a specific uptake phase. Since gaseous diffusion coefficients of PAHs are similar, the time scales over which a passive sampler integrates consequently increases with increasing Kpg(1.2). Thus, linear and curvilinear uptake phases (and t0.63) occur at very different time scales for different compounds, which is a major difficulty for PAS sampling using thin PE sheets.7 Since partition coefficients between PE and gas phase of the 16 U.S. EPA priority PAHs cover a range of more than four orders of magnitude,42 high molecular weight PAHs equilibrate 10
000 times slower than low molecular weight compounds. Kpg depends on temperature (to some minor extent this also applies to Dg), which may be different in each time interval; additionally, the exchange kinetics are influenced by wind speed,28,30 although this is reduced by the wind-protected sampling design and is accounted for by calibrating δg.
To account for the temperature-dependent varying values of Kpg and Dg, Fick's first law can be solved numerically using the differential equation for uptake of a compound from the gas phase:
| 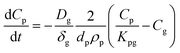 | (1.7) |
Solving this equation step-wise provides discrete Cp values after each time step (dt):
| 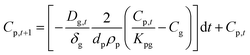 | (1.8) |
Cp/
Kpg denotes the gas phase concentration at the polymer/air interface which allows to calculate the concentration difference across the air-side boundary layer.
Dg/
δg is the mass transfer coefficient [m s
−1].
Kpg and
Dg can be adjusted for each time step according to temperature conditions. Partition coefficients can be calculated for different temperatures (
T1 and
T2 in kelvin) following the van't Hoff equation and using the enthalpy of vaporization, according to Khairy and Lohmann:
22,48 | 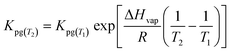 | (1.9) |
Kpg(T1) is the partitioning coefficient at standard temperature
T1 (
e.g. 298.15 K, here taken from Khairy and Lohmann
22) and
R denotes the ideal gas constant (8.314 × 10
−3 kJ (mol
−1 K
−1)). The evaporation enthalpy Δ
Hvap is typically ∼80 kJ mol
−1 (see
Table 1) and thus a decrease of temperature by 20 °C would cause an increase of
Kpg by a factor of 10. The diffusion coefficient in the gas phase
Dg (in [m
2 s
−1]) can be calculated for the respective temperature following the Fuller–Schettler–Giddings-method:
| 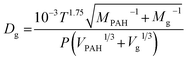 | (1.10) |
MPAH,
Mg,
VPAH,
Vg,
P denote molecular mass [g mol
−1] of the compound of interest and of air, their molar volumes [m
3 mol
−1] and atmospheric pressure [atm].
During PRC loss, the air side boundary layer thickness δg is the only unknown parameter in our setup (see eqn (1.7)). In a first step, the numerical model was applied to calibrate this air-side boundary layer as a constant value for each sampling campaign by location. A Gauß–Newton type least-square method implemented in the optimization toolbox of Matlab (lsqcurvefit) was utilized to correlate the calculated concentrations on the PE to the measured data set by fitting δg. Diffusion and partition coefficients were adapted to the current temperatures during each sampling period based on hourly measurements, provided by the German Weather Survey, measured at the close-by airport in Stuttgart-Echterdingen (48.6915°, 9.1939°). A residual concentration of Ant-D10 (ca. 5% of the initial spiking) on PE-PAS remained even after several weeks in the field, which was considered in the fitting routine calculating δg, but did not influence the resulting δg. For comparison, δg was also fitted to the uptake of Phe onto the sampler. Since concentrations on PE were very similar for the three sites, data were merged to obtain one average δg for each monitoring, which is discussed in Section 3.3. Phenanthrene was chosen since its physicochemical properties are almost the same as for Ant, the linear uptake phase of Phe onto PE is long enough to be sampled, and Phe reaches equilibrium on the sampler during each monitoring. At equilibrium, the atmospheric concentrations of Phe can be determined simply by using the equilibrium partition coefficient (Cg,eq = Cp,eq/Kpg) and δg remains as the only unknown fitting parameter.
For the other target compounds, a fixed air-side boundary layer thickness was implemented into the numerical model (eqn (1.7)) and Cg remains as the only unknown parameter, which was fitted with the same fitting routine as applied for δg. As in other approaches, Cg is assumed to be stable during the monitoring, as our model fits only one (average) value of Cg to the uptake curve onto PE. This adds some uncertainty to the resulting Cg, since concentrations of some PAHs in air likely show short-term fluctuations and diurnal changes.49–51 Depending on the partition coefficients between air and sampler (or the sampling capacity of the sampler), PE integrates over different time scales for the different compounds26 (see characteristic times, eqn (1.1)), which additionally varies with season. For instance, lower molecular weight PAHs (e.g. Fln) reach chemical equilibrium on PE within hours to days, and the different sampling times could be used to capture short-term variations in air. In contrast, higher molecular weight PAHs, like Fth and Pyr, do not reach equilibrium on PE during the monthly deployment time and Cp integrates over the whole monitoring period. In comparison to the numerical approach (eqn (1.7)), the analytical solution (eqn (1.4)) uses constant values for Kpg and Dg over the monitoring period to calculate again an average value for Cg. The resulting Cg varies only slightly from the numerical model, but fluctuations on the PE cannot be captured this way.
Our approach takes no PAH degradation processes into account, as previous tests in the field (sampling with different set-ups, various PRCs and a day versus night sampling) confirmed that degradation of PAHs on the sampler is negligible (see ESI†). Many previous studies track loss of PRCs and assume that the loss rate constant of the chosen PRCs equals the uptake rate of target compounds and therefore use several PRCs with a range of physicochemical properties in parallel for kinetics calibration.13,28,48 Alternatively, sampling rates of the target compounds are calibrated using active and passive samplers simultaneously.52–56 We compared the air concentrations derived by passive sampling and the numerical model using PRC calibration with concentrations given by active air samplers not for calibration purposes but to test the robustness of the passive sampling approach. The advantage of our approach is that we need only one PRC, as we focus on the air-side boundary layer as the limiting parameter for the exchange of PAHs between air and PE.
3. Results and discussion
3.1. Air-side boundary layers
Fig. 1 shows the measured PRC-loss at each location and the fit to the predicted loss after merging the three sites, which seems valid for the comparable conditions at the study sites. Air-side boundary layer thicknesses (δg) determined based on the loss of Ant-D10 from PE or the uptake of phenanthrene for each location and for 7 time periods (30 to 36 days each) over 2 years are listed in Table 2 together with the average wind speed, air temperature, global radiation and average Kpg during the first three days – the period which was used for calibrating δg. Values of δg determined from PRC analysis were very similar for the three sites and varied over time likely due to a combination of factors including weather conditions and analytical uncertainties. For instance, in August 2016 and August 2017 almost equal wind speeds (2.1 m s−1) and average temperatures (difference less than ∼4 °C) were measured at the nearby airport but fitted average values for δg differ by a factor of two (1.6 ± 0.34 and 0.80 ± 0.06 mm). Overall, values of δg stay in a rather small range with thicknesses between 0.4 mm and 2.3 mm during the two consecutive years and without a seasonal pattern. No obvious correlation could be observed for the thickness of the air-side boundary layer either as a function of air temperature (which varied between −1 °C and 20 °C), wind speed (which was rather stable with 2–3 m s−1 during all sampling campaigns) or the solar radiation (which showed higher values during summer). The latter indicates that the sampling design limits photodegradation of PAHs on passive samplers which is a potential issue discussed in literature.57–59 If photodegradation was causing significant loss of PAHs from PE, we would expect a correlation to solar radiation leading to faster losses of the PRC and thus (artificially) thinner air-side boundary layer in summer, which was not the case. Since Phe and Ant differ significantly in their photosensitivity,58–60 the agreement in δg calculated using either Ant-D10 loss or Phe uptake also indicates that photodegradation of PAHs on PE was not relevant. For a more thorough test on adverse influences on our PAS (like photodegradation), we compared different sampling designs, controlled diurnal variations of PAHs on PE and used Pyr-D10 as additional PRC for comparison during some monitorings. None of those tests gave evidence of photodegradation of PAH on PE (see ESI†). Thus, the small range in values of δg is likely due to the sampling set-up, with boxes minimizing the influence of wind and solar radiation on the samplers throughout the monitoring periods. This suggests the use of an average value of δg independent of the season (here 1 mm) which corresponds to a general calibration of passive sampling kinetics for a specific set-up, such as used before within the Global Atmospheric Passive Sampling network.7,61 This would then also apply for long-term monitoring of higher molecular weight PAHs which have characteristic time scales for equilibration of several months (Fig. 2). At lower temperatures, characteristic times increase for all compounds due to Kpg increasing by up to a factor of ten. Thus, in this case (with rather stable sampling conditions) the exchange between PE and air over seasonal time-scales is more influenced by the physical–chemical properties of the target compounds than by temporal variation of δg. Yet, the observed variation of δg (±50%) illustrates its uncertainty.
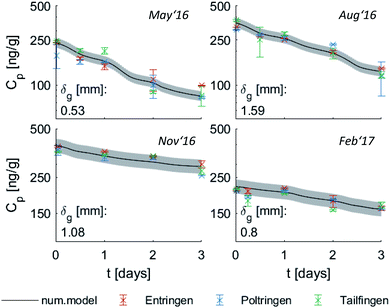 |
| Fig. 1 Seasonal PRC-loss during the first year of monitoring, measured at each location and fitted (black solid line) based on measurements averaging over all locations. The air-side boundary layer thickness δg was fitted for the average concentrations on PE. Error bars refer to triplicate samples at each location and the grey area indicates the standard deviation of the predicted concentration. | |
Table 2 Air-side boundary layers (δg) in mm, fitted with the numerical model to the loss of Ant-D10 from PE sheets during the first three days of each sampling period and for each location individually and average values fitted to the linear uptake of Phe (δg,Phe) as well as average values of Kpg, Dg, temperature (T), wind speed and the sum of the global radiation during the first three days; the overall average of δg is 1 mm ± 0.5 mm
Monitoring |
May-16 |
Aug-16 |
Nov-16 |
Feb-17 |
Aug-17 |
Nov-17 |
Feb-18 |
Entringen δg,Ant [mm] |
0.5 ± 0.1 |
1.7 ± 0.1 |
1.2 ± 0.2 |
1.2 ± 0.3 |
0.9 ± 0.1 |
2.2 ± 0.2 |
0.8 ± 0.2 |
Poltringen δg,Ant [mm] |
0.7 ± 0.1 |
2.1 ± 0.3 |
0.9 ± 0.2 |
1.5 ± 0.2 |
0.7 ± 0.1 |
1.3 ± 0.2 |
0.8 ± 0.1 |
Tailfingen δg,Ant [mm] |
0.5 ± 0.1 |
1.2 ± 0.2 |
1.4 ± 0.4 |
1.0 ± 0.3 |
0.8 ± 0.1 |
1.4 ± 0.1 |
0.8 ± 0.2 |
Avg. δg,Ant [mm] |
0.6 ± 0.1 |
1.6 ± 0.3 |
1.1 ± 0.3 |
1.4 ± 0.3 |
0.8 ± 0.1 |
1.5 ± 0.3 |
0.8 ± 0.3 |
Avg. δg,Phe [mm] |
0.8 ± 0.1 |
1.3 ± 0.1 |
1.2 ± 0.2 |
1.1 ± 0.4 |
0.9 ± 0.2 |
1.0 ± 0.1 |
0.8 ± 0.1 |
log Kpg [L kg−1] |
7.78 |
7.33 |
8.04 |
8.12 |
7.15 |
7.88 |
8.26 |
log Dg [m2 s−1] |
−5.26 |
−5.24 |
−5.28 |
−5.28 |
−5.23 |
−5.27 |
−5.29 |
T [K] |
283.7 |
292.7 |
278.8 |
277 |
296.45 |
281.68 |
275.27 |
Radiation [J cm−2] |
5130 |
4090 |
1450 |
4760 |
4310 |
1100 |
1400 |
Wind speed [m s−1] |
3.1 |
2.1 |
1.6 |
1.3 |
2.1 |
1.4 |
2.7 |
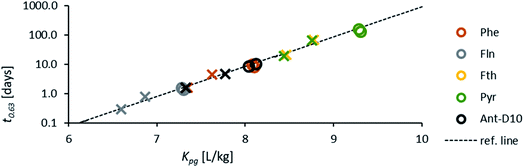 |
| Fig. 2 Characteristic times of fluorene (Fln), phenanthrene (Phe), fluoranthene (Fth) and pyrene (Pyr) to reach 63% of equilibrium on PE for a mean air-side boundary layer thickness δg (=1 mm) versus PE-air partitioning constant Kpg; the dotted line covers the range from naphthalene to benzo(a)pyrene at a temperature of 20 °C; symbols indicate seasonal sampling values for Kpg during autumn and winter (circles) and summer (crosses). Due to their similar properties, Fth and Pyr plot above each other, similar to Phe and Ant during winter. | |
3.2. Uptake of target compounds – comparison of numerical model and analytical solution
Uptake curves of the four PAHs onto PE were fitted both with the numerical model and the analytical solution using the mean δg of 1 mm for all sampling campaigns and are shown in Fig. 3. Only the last measurement on PE was used to fit the analytical solution to Fth and Pyr. PE integrates the air concentrations for these two compounds in the linear uptake phase over the entire monitoring campaign, as their long characteristic times indicate. Additionally, higher mass accumulation at the end of the sampling improves certainty in analytical measurement. In contrast, for Fln and Phe all measurements on PE were used to integrate similarly over each time interval during monitoring.
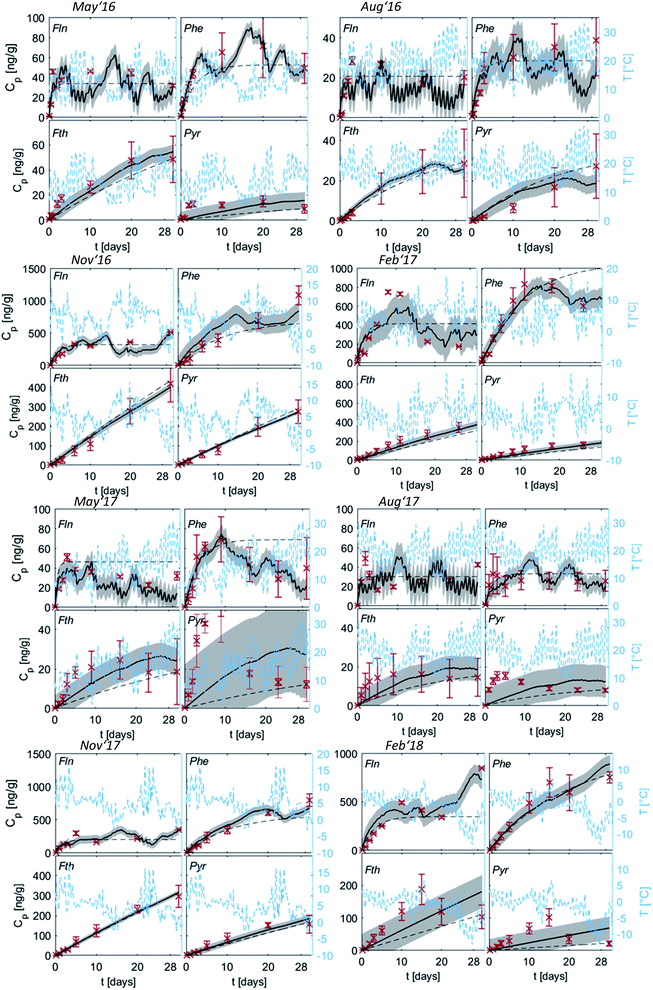 |
| Fig. 3 Measured (red crosses) and modelled uptake curves of the four representative PAHs (averaged for all three sites) along with the temperature changes (blue); grey areas indicate standard deviations calculated with the numerical model (solid line), error bars refer to the standard deviation of the measured replicates, dashed lines indicate the analytical solution (exponential function, eqn (1.4)). For a better illustration of low values, concentrations of Fln and Pyr are multiplied with a factor of five. For Fln this is done for all seasons, while for Pyr this was necessary only during May and August 2017. | |
Fig. 3 shows diurnal and seasonal variations in uptake kinetics of target compounds on PE from air. The seasonal dependency of t0.63 is clearly visible with shorter timescales during May and August. In those warmer periods, Fln and Phe reach air-PE equilibrium during the first ∼10 days of the sampling campaign. Subsequently, PE concentrations of both compounds fluctuate in response to changing Kpg driven by changes in the ambient temperature. This fluctuation is dampened during November and February when characteristic equilibration times are longer. In those colder months, Fth and Pyr demonstrate a linear uptake during the 30-d monitoring periods. Thus, the PE-PAS operate as either equilibrium or kinetic samplers during the deployment of the same duration depending on the compound, and possibly the season.17 This observation can also be linked directly to the variation of the partition coefficient with temperature driving change in sorption capacity, since the air-side boundary layer (and thus the inherent sampling rate) is not affected by temperature and was observed in our deployments to be as almost constant throughout the year. Significantly higher concentrations on PE were observed during November and February, indicating higher atmospheric concentrations likely due to the increased fuel demand for heating in the winter season (note different scales of Cp in Fig. 3). Both seasonal variations confirm our expectations and support previous studies on PAHs in the atmosphere62–64 as well as passive sampling kinetics.61
Since the numerical model accounts for temperature variation by adapting Kpg over time, it is able to capture the fluctuation of the target compounds on the PE, if equilibration is fast. This was clearly observed during February '17 with a decrease of Cp for Fln and Phe induced by an increase of temperature towards the end of the monitoring. The analytical solution, however, averages Kpg over the whole sampling period and thus cannot reflect temporal variations on the sampler. By accounting for the temperature variation in Kpg throughout each monitoring period, the numerical model can indicate whether a change of concentration on PE is due to a change of temperature alone or in addition to a change in atmospheric concentrations. Large differences between measured and numerically-calculated concentrations on PE indicate a large deviation from average Cg and thus a change of the emission scenario.
3.3. Calculation of average Cg from passive sampling data
To check on the effect of the variation in δg for the individual sampling sites and seasons, atmospheric concentrations of the target PAHs were determined both by fitting for each location individually and as an average over the three sites. The outcome for both approaches was in close agreement (Fig. 4(a)) as expected based on the good fit for δg (Fig. 1). Only low concentrations (Fth and Pyr) show some deviation from the reference line in excess of a confidence interval of ±30%. The best fit was determined for the more volatile compounds, Fln and Phe, which are already in or close to equilibrium at the end of the sampling campaign (see Fig. 2 for characteristic times). The main deviation was observed for the study site in Entringen with slightly higher concentrations fitted. This site is located close to a railway (diesel fuelled) and is thus likely influenced by this additional emission source. This observation indicates that the uncertainty in data evaluation is rather caused by atmospheric variability than differing air-side boundary layers. The generally good agreement for each site confirmed the reproducibility of the chosen method. Measured concentrations on PE result in 6–9 replicates per sampling time and 72 samples available in total to numerically fit average values of Cg. Fig. 4 compares atmospheric concentrations fitted two ways: (1) using concentrations on PE combined for all locations for the first year of monitoring with seasonal values for δg and (2) based on the mean δg of 1 mm. As shown in Fig. 4(b) concentrations determined by these two approaches are within a confidence interval of ±30%; although Cg fluctuates significantly throughout the year, the average δg is still able to capture those atmospheric variations.
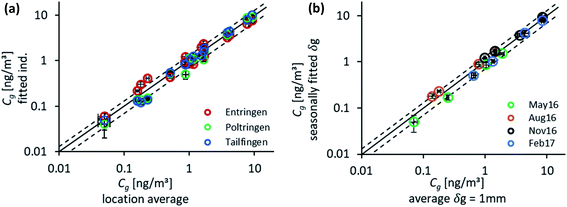 |
| Fig. 4 Scatter plots of fitted atmospheric concentrations (numerical model) of the four target PAHs for (a) Cg fitted for each location individually versus Cg averaging over the three sites and (b) combining the three sites with Cg based on a seasonal δgversus the average δg of 1 mm. A reference line (1 : 1) as well as a 30% confidence interval is included, and the error bars of the measurement refer to the standard deviation calculated for the numerical model. | |
Similarly, atmospheric concentrations determined with the numerical model and the analytical solution applying a constant air-side boundary layer of 1 mm agreed reasonably well (Fig. S8†). Only for Pyr some clear deviations could be observed during the warmer periods when atmospheric concentrations of Pyr as well as the concentrations on the sampler are very low. This is already visible for the uptake onto the sampler in Fig. 3 with unexpectedly high concentrations in the beginning, followed by a decrease towards the end of the monitoring. Hence, the numerical model, which accounts for all sampling times, leads to a higher concentration in air than the analytical solution which is based only on the last sampling point.
Earlier studies have illustrated how complex the determination of precise sampling rates is and how easily errors can be made when calibrating passive samplers.7,65 Sampling rates, or the amount of target compound sampled usually expressed as air volume per time,66–68 for a given PAS configuration are reported to vary over several orders of magnitude, depending on the environmental conditions, the considered time span, the target compound, and even the concentrations on the passive samplers.15,56,69,70 Since they are significantly influenced by the ambient temperature, wind speed and wind angle, sampling rates can vary considerably within a sampling campaign. The air-side boundary layer would be influenced by ambient conditions like wind speed and wind angle in the same way as the inherent sampling rate. In the present field study, we observed a good reproducibility of this parameter due to the similarity of sites and conditions. Thus, for stable sampling conditions the general calibration of PE-passive samplers using δg seems justified, as for sampling rate. Yet, an ease and transparency of comparison is provided by using δg as it does not change with the surface area of the sampler, nor for the target compounds.
3.4. Comparison of Cg from passive to active sampling
Active sampling was conducted at one location (Entringen) in November 2017 and February 2018 to reference against air concentrations determined by PE-PAS. Active samplers filtered about 4 m3 of air during 3–5 days, sampling periodically for 16 hours with intervals (of up to 8 hours) to recharge the batteries. For both monitorings we sampled two time-spans to check on short-term variations of Cg. During November the respective time-spans were 18.11.17 to 22.11.17 and 1.12.17 to 4.12.17, and during February the time-spans were 15.2.18 to 20.2.18 and 25.2.18 to 28.2.18. LMW PAHs (Fln, Phe) are expected to have reached equilibrium state on PE at this point and thus are better suited for comparison. As listed in Table 3, significant variation of Cg during this cold period was only observed for Fth and Pyr with a decrease of Cg for a factor of ten towards the last sampling period (25. to 28.2.18). Although PAHs were below quantification limit on the glass fibre filters, the influence of particles on Cg determined on active samplers (PUF filters) cannot be completely excluded as shown in earlier studies,70 where Phe, Fth and Pyr sorbed to ultrafine particles under 100 nm (smaller than the pore size of the glass fibre filters) were found on PUF filters. As no PAHs were detected on glass fibre filters during the second sampling in February 2018, perhaps this indicates that particulates in air were at a lower level and therefore leading to a lower contribution of particle influence on PUF. Due to the strong sorption of Fth and Pyr to particles, a small amount of (accidentally) sampled particles could cause a significant measurement bias on the resulting atmospheric concentrations.56,70
Table 3 Atmospheric concentrations [ng m−3] of Fln, Phe, Fth and Pyr determined with active samplers covering two individual time spans in November 2017/December 2017 and February 2018
Monitoring |
Fln |
Phe |
Fth |
Pyr |
Active: 18.11. to 22.11.17 |
2.8 ± 0.3 |
9.0 ± 4.3 |
2.2 ± 0.1 |
1.4 ± 0.1 |
Active: 1.12. to 4.12.17 |
3.3 ± 0.1 |
13.0 ± 0.1 |
2.1 ± 0.1 |
1.7 ± 0.1 |
Active: 15.2. to 20.2.18 |
2.6 ± 0.8 |
8.9 ± 0.9 |
2.1 ± 0.2 |
1.0 ± 0.2 |
Active: 25.2. to 28.2.18 |
0.9 ± 0.4 |
12.5 ± 2.9 |
0.2 ± 0.1 |
0.1 ± 0.1 |
Fig. 5 compares results from passive samplers (evaluated with the numerical model) and Cg measured with active samplers during November 2017 (18.11.17 to 22.11.17) and February 2018 (15.2.18 to 20.2.18). A very good agreement was obtained for Fln and Phe during both monitoring periods. For Fth and Pyr, a reasonable agreement within the range of uncertainty can be observed for November, while during February the active samplers provide higher atmospheric concentrations than determined with passive samplers. This can be due to the different time spans, and thus different air masses, which are reflected by the two methods. A direct comparison of Cg based on different sampling methods can only be valid if the same air masses are sampled, otherwise the different methods can be influenced by varying atmospheric concentrations.53,56 In our study, due to the short 3–5 days duration of the active sampling, active and passive samplers integrate only for Fln over the same time span and reflect the same concentration in air. This is the same observation as reported by Melymuk et al.15 Again, the calculated t63 can be used to identify the compound-specific time span to reach 63% equilibration by the passive sampler. With a t63 on the order of days (Fig. 1), Fln is the most reliable of the chosen PAHs for a comparison of those two sampling methods. An average ratio of one for Cg,active/Cg,passive for Fln confirms the presented passive sampling approach as a valid method to measure gaseous concentrations of PAHs.
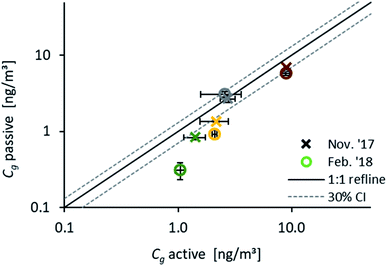 |
| Fig. 5 log–log scatter plot of atmospheric concentrations of Fln (grey), Phe (brown), Fth (yellow) and Pyr (green) determined with passive samplers in deployments over 3-November to 3-December and 1–15 February using the numerical model based on the seasonal δg (1.6 mm) versus concentrations measured with active samplers in deployments over 18–22 November 2017 and 15–20 February 2018 in Entringen. Error bars show the standard deviation of the triplicate samples (passive and active). | |
3.5. The use of characteristic ratios
Fig. 6 shows the distribution pattern of the four PAHs in air during each of the seasonal sampling campaigns between May 2016 and February 2018. Concentrations included within Fig. 6(a) were fitted with the numerical model to the respective uptake curves on the PE. As described above, the three study sites were combined as they reflect the same geographic area with only slight concentration differences. Therefore, up to nine replicates were considered per sampling time (and 72 samples per fitting routine), resulting in robust average values, particularly with respect to the observed pattern. Fig. 6(a) illustrates two characteristic features: (i) atmospheric concentrations are higher in the heating period during autumn and winter during both years (confirming the observation of Fig. 3) and (ii) Phe has the highest concentrations in air throughout the whole monitoring period, followed by Fln, while Fth and Pyr show only low atmospheric concentrations. The remainder of the 16 U.S. EPA priority PAHs targeted in the analytical method have lower concentrations than these four, except for naphthalene, which is in the same range as Fln. Fig. 6(b) demonstrates the very good reproducibility of this characteristic distribution pattern throughout all sampling campaigns. This also holds for active samplers, except for the second campaign in February (Fig. S9†), which may be explained by a lower influence of particles on PUF filters during this period. Stable characteristic distribution patterns allow the calculation of concentrations of several compounds based on just a few or one reference compound, e.g. Phe. This approach was presented for soils by Wilcke71 and Bucheli et al.,72 who determined very stable distribution patterns of PAHs in a broad range of soils and used specific ratios to calculate concentrations of several PAHs based on the measurement of one reference PAH. Earlier studies on PAH atmospheric deposition in southwestern Germany also report very consistent distribution patterns with highest amounts for Phe.73,74
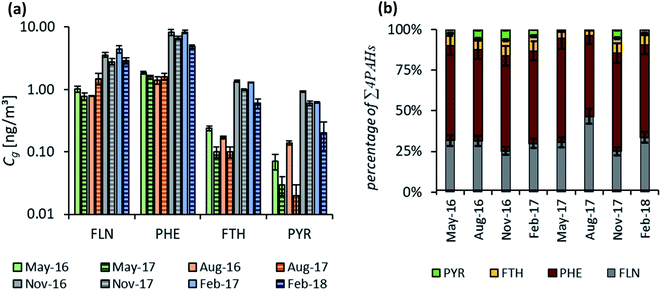 |
| Fig. 6 (a) Atmospheric concentrations of the four representative PAHs determined by passive sampling averaged over all sites. Error bars indicate standard deviation of 9 replicates. (b) Distribution pattern of the four representative PAHs, error bars for FLN and PHE are set to a standard deviation of 10%. | |
Based on these findings, Phe may be applied as a reference to calculate the concentrations of other PAHs within soil or the atmosphere. Phenanthrene was chosen as a reference compound, as we observed highest concentrations and lowest standard deviation for replicate samples. For this approach, the ratios of Fln/Phe, Fth/Phe and Pyr/Phe were calculated for Cg during summer 2016. Subsequently, those ratios were used in combination with the fitted Cg of Phe during all other monitoring periods to estimate Cg for Fln, Fth and Pyr. A very good agreement, with the majority within ±30%, was obtained for all atmospheric concentrations above 0.1 ng m−3 (Fig. 7). As discussed above, lower concentrations near analytical detection limits are more uncertain, and this issue is most pronounced for Pyr during the warmest sampling campaigns. This results in distinctively higher concentrations of Pyr estimated than those fitted with the numerical model (marked in the figure). For unknown reasons, the observed uptake of Pyr onto PE is considerably faster than calculated with the numerical model (Fig. 3) except for August 2016, which might lead to an underestimation of the corresponding Cg. Using an average ratio of Pyr/Phe to account for all sampling campaigns with Cg > 0.1 ng m−3 leads to slightly lower atmospheric concentrations (factor 0.8) than the specific ratio during summer 2016. For Fln and Fth no difference was observed for an average ratio (Fig. S10†). Interestingly, Melymuk et al.15 also observed unexpectedly high passive sampling rates during the first half of the year for Fth and Pyr. However, the generally good agreement of estimated and fitted concentrations can be accepted to confirm the reliability of this approach to use one reference compound and stable ratios to determine the whole range of atmospheric concentrations. This allows to overcome exceedingly long monitoring times otherwise required for high molecular weight PAHs on the passive samplers and can be used to reduce the uncertainty caused by the measurement of very low concentrations. Yet, it should be noted, that such an approach is only valid in certain areas for which homogenously mixed air masses or stable distribution patterns have been observed.
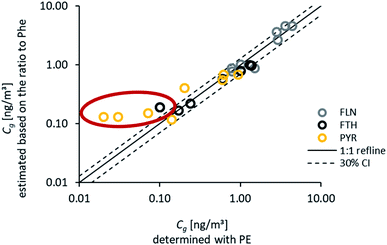 |
| Fig. 7 Atmospheric concentrations of Fln, Fth and Pyr for all seasons and all locations calculated from Phe and the average distribution pattern during August 2016. The red circle denotes outliers of Pyr during the warm periods as well as Fth during August 2017. | |
4. Conclusions
As the uptake kinetics of passive samplers in the atmosphere vary significantly for the different compounds and seasons, the characteristic times to 63% equilibration (t0.63) provide a useful and convenient measure of the time span over which PAS integrate individual target compounds. Knowledge of the characteristic times helps to improve the planning of sampling campaigns as well as the evaluation of measured concentrations. Providing a consistent design for the passive samplers in the field allows the use of one (average) air-side boundary layer for the data evaluation, which is specific for the sampling set-up, but according to our results is (almost) independent of location and season. Thus, a set-up specific value may be taken (here 1 mm) for all compounds. Numerical modelling shows that even diurnal temperature changes affect the loading of the passive samplers for compounds with log
Kpg < 8 L kg−1 because of the temperature sensitivity of partition coefficients. Hence, combining measured uptake curves (time series) with such a modelling approach allows for the identification of (short-term) concentration changes in the atmosphere. Cg probably fluctuates rapidly (diurnal) and significantly for many SVOCs of interest for passive air sampling and if the goal is to get average concentrations then for compounds with fast equilibration with PE-PAS (Fln, Phe) several measurements are needed during the considered time span. Compounds with log
Kpg > 9 L kg−1 only reach equilibrium after long time periods (at least 100 days) and yield a stable average during the linear uptake period for shorter deployments (in principle just one data point is needed), but for these compounds the uptake is very low and often below the limit of quantification. One way to solve this dilemma would be focusing on a representative PAH covering the desired monitoring time span and using PAH ratios to calculate other compounds of interest. Based on the present study a good candidate could be Phe, which shows the highest concentrations in air and on the sampler and thus the lowest uncertainty for the data-evaluation.
Conflicts of interest
There are no conflicts to declare.
Acknowledgements
We would like to thank Renate Seelig and Thomas Wendel for their support with laboratory work as well as Wolfgang Kürner for providing the set-up in the field. Bettina Rüdiger was a great support in the field and laboratory work. This work was funded by the Deutsche Forschungsgemeinschaft (DFG) under the grant agreement GR 971/30-1.
References
- C. J. Halsall,
et al., Spatial and Temporal Variation of Polycyclic Aromatic Hydrocarbons in the Arctic Atmosphere, Environ. Sci. Technol., 1997, 31(12), 3593–3599 Search PubMed.
- A. Cabrerizo,
et al., Climatic and biogeochemical controls on the remobilization and reservoirs of persistent organic pollutants in Antarctica, Environ. Sci. Technol., 2013, 47(9), 4299–4306 Search PubMed.
- S. M. Bengtson Nash,
et al., Persistent Organic Pollutants in the East Antarctic Atmosphere: Inter-Annual Observations from 2010 to 2015 Using High-Flow-Through Passive Sampling, Environ. Sci. Technol., 2017, 51(23), 13929–13937 Search PubMed.
- L. Tuduri,
et al., Passive air sampling of semi-volatile organic compounds, TrAC, Trends Anal. Chem., 2012, 31, 38–49 Search PubMed.
- B. Vrana,
et al., Passive sampling techniques for monitoring pollutants in water, TrAC, Trends Anal. Chem., 2005, 24(10), 845–868 Search PubMed.
- A. Kot-Wasik,
et al., Advances in passive sampling in environmental studies, Anal. Chim. Acta, 2007, 602(2), 141–163 Search PubMed.
- F. Wania and C. Shunthirasingham, Passive air sampling for semi-volatile organic chemicals, Environ. Sci.: Processes Impacts, 2020, 22(10), 1925–2002 Search PubMed.
- A. Sobek,
et al., Coastal sediments in the Gulf of Bothnia as a source of dissolved PCDD/Fs and PCBs to water and fish, Sci. Total Environ., 2014, 487, 463–470 Search PubMed.
- N. C. Niehus, B. Brockmeyer and G. Witt, Bioavailability and distribution of PAHs and PCBs in the sediment pore water of the German Bight and Wadden Sea, Mar. Pollut. Bull., 2019, 138, 421–427 Search PubMed.
- B. Beckingham and U. Ghosh, Polyoxymethylene passive samplers to monitor changes in bioavailability and flux of PCBs after activated carbon amendment to sediment in the field, Chemosphere, 2013, 91(10), 1401–1407 Search PubMed.
- Y. Liu,
et al., Gaseous and Freely-Dissolved PCBs in the Lower Great Lakes Based on Passive Sampling: Spatial Trends and Air–Water Exchange, Environ. Sci. Technol., 2016, 50(10), 4932–4939 Search PubMed.
- R. Lohmann,
et al., Determining air–water exchange, spatial and temporal trends of freely dissolved PAHs in an urban estuary using passive polyethylene samplers, Environ. Sci. Technol., 2011, 45(7), 2655–2662 Search PubMed.
- E. J. Morgan and R. Lohmann, Detecting air–water and surface-deep water gradients of PCBs using polyethylene passive samplers, Environ. Sci. Technol., 2008, 42(19), 7248–7253 Search PubMed.
- B. Zabiegała,
et al., Passive sampling as a tool for obtaining reliable analytical information in environmental quality monitoring, Anal. Bioanal. Chem., 2010, 396(1), 273–296 Search PubMed.
- L. Melymuk,
et al., Evaluation of passive air sampler calibrations: Selection of sampling rates and implications for the measurement of persistent organic pollutants in air, Atmos. Environ., 2011, 45(10), 1867–1875 Search PubMed.
- L. A. Schifman and T. B. Boving, Spatial and seasonal atmospheric PAH deposition patterns and sources in Rhode Island, Atmos. Environ., 2015, 120, 253–261 Search PubMed.
- T. Harner,
et al., Passive air sampling for persistent organic pollutants: introductory remarks to the special issue, Environ. Pollut., 2006, 144(2), 361–364 Search PubMed.
- J. N. Huckins,
et al., Lipid-containing semipermeable membrane devices for monitoring organic contaminants in water, Environ. Sci. Technol., 1993, 27(12), 2489–2496 Search PubMed.
- M. Shoeib and T. Harner, Characterization and Comparison of Three Passive Air Samplers Persistent Organic Pollutants, Environ. Sci. Technol., 2002, 36(19), 4142–4151 Search PubMed.
- M. E. Bartkow,
et al., Characterizing Uptake Kinetics of PAHs from the Air Using Polyethylene-Based Passive Air Samplers of Multiple Surface Area-to-Volume Ratios, Environ. Sci. Technol., 2004, 38(9), 2701–2706 Search PubMed.
- M. E. Bartkow,
et al., Passive air sampling theory for semivolatile organic compounds, Chemosphere, 2005, 60(2), 170–176 Search PubMed.
- M. A. Khairy and R. Lohmann, Field validation of polyethylene passive air samplers for parent and alkylated PAHs in Alexandria, Egypt, Environ. Sci. Technol., 2012, 46(7), 3990–3998 Search PubMed.
- W. G. Whitman, The two film theory of gas absorption, Int. J. Heat Mass Transfer, 1962, 5(5), 429–433 Search PubMed.
- K. Pozo,
et al., Seasonally Resolved Concentrations of Persistent Organic Pollutants in the Global Atmosphere from the First Year of the GAPS Study, Environ. Sci. Technol., 2009, 43(3), 796–803 Search PubMed.
- P. Mayer,
et al., Equilibrium sampling devices, Environ. Sci. Technol., 2003, 37(9), 184A–191A Search PubMed.
- T. Harner,
et al., Passive air sampling for persistent organic pollutants: Introductory remarks to the special issue, Environ. Pollut., 2006, 144(2), 361–364 Search PubMed.
- C. Moeckel,
et al., Use of Depuration Compounds in Passive Air Samplers: Results from Active Sampling-Supported Field Deployment, Potential Uses, and Recommendations, Environ. Sci. Technol., 2009, 43(9), 3227–3232 Search PubMed.
- H. S. Söderström and P.-A. Bergqvist, Passive Air Sampling Using Semipermeable Membrane Devices at Different Wind-Speeds in Situ Calibrated by Performance Reference Compounds, Environ. Sci. Technol., 2004, 38(18), 4828–4834 Search PubMed.
- W. A. Ockenden, F. M. Jaward and K. C. Jones, Atmospheric sampling of persistent organic pollutants: needs, applications and advances in passive air sampling techniques, Sci. World J., 2001, 1, 557–575 Search PubMed.
- L. Tuduri, T. Harner and H. Hung, Polyurethane foam (PUF) disks passive air samplers: Wind effect on sampling rates, Environ. Pollut., 2006, 144(2), 377–383 Search PubMed.
- M. E. Bartkow,
et al., Evaluation of performance reference compounds in polyethylene-based passive air samplers, Environ. Pollut., 2006, 144(2), 365–370 Search PubMed.
- J. N. Huckins,
et al., Determination of Uptake Kinetics (Sampling Rates) by Lipid-Containing Semipermeable Membrane Devices (SPMDs) for Polycyclic Aromatic Hydrocarbons (PAHs) in Water, Environ. Sci. Technol., 1999, 33(21), 3918–3923 Search PubMed.
- R. D. Wijayaratne and J. C. Means, Sorption of Polycyclic aromatic hydrocarbons by natural estuarine colloids, Mar. Environ. Res., 1984, 11(2), 77–89 Search PubMed.
- K. C. Jones,
et al., Organic contaminants in Welsh soils: polynuclear aromatic hydrocarbons, Environ. Sci. Technol., 1989, 23(5), 540–550 Search PubMed.
-
M. Howsam and K. C. Jones, Sources of PAHs in the Environment, in PAHs and Related Compounds: Chemistry, ed. A. H. Neilson, Springer Berlin Heidelberg, Berlin, Heidelberg, 1998, pp. 137–174 Search PubMed.
- M. V. Roux,
et al., Critically Evaluated Thermochemical Properties of Polycyclic Aromatic Hydrocarbons, J. Phys. Chem. Ref. Data, 2008, 37(4), 1855 Search PubMed.
- K. C. Jones, Observations on long-term air–soil exchange of organic contaminants, Environ. Sci. Pollut. Res., 1994, 1(3), 172 Search PubMed.
- Y. Dumanoglu,
et al., Spatial and seasonal variations, sources, air–soil exchange, and carcinogenic risk assessment for PAHs and PCBs in air and soil of Kutahya, Turkey, the province of thermal power plants, Sci. Total Environ., 2017, 580, 920–935 Search PubMed.
- E. Kaya,
et al., Spatial and temporal variation and air–soil exchange of atmospheric PAHs and PCBs in an industrial region, Atmos. Pollut. Res., 2012, 3(4), 435–449 Search PubMed.
- Z. Wu,
et al., Air–sea exchange and gas–particle partitioning of polycyclic aromatic hydrocarbons over the northwestern Pacific Ocean: Role of East Asian continental outflow, Environ. Pollut., 2017, 230, 444–452 Search PubMed.
- I. J. Allan,
et al., Effect of sampler material on the uptake of PAHs into passive sampling devices, Chemosphere, 2010, 79(4), 470–475 Search PubMed.
- R. Lohmann, Critical review of low-density polyethylene's partitioning and diffusion coefficients for trace organic contaminants and implications for its use as a passive sampler, Environ. Sci. Technol., 2012, 46(2), 606–618 Search PubMed.
- K. E. Kennedy,
et al., A field comparison of ethylene vinyl acetate and low-density polyethylene thin films for equilibrium phase passive air sampling of polycyclic aromatic hydrocarbons, Atmos. Environ., 2007, 41(27), 5778–5787 Search PubMed.
- K. Booij, S. Foppe and E. M. van Weerlee, Spiking of performance reference compounds in low density polyethylene and silicone passive water samplers, Chemosphere, 2002, 46, 1157–1161 Search PubMed.
- J. N. Huckins,
et al., Development of the Permeability/Performance Reference Compound Approach for In Situ Calibration of Semipermeable Membrane Devices, Environ. Sci. Technol., 2002, 36(1), 85–91 Search PubMed.
- T. Harner,
et al., Characterization of Polymer-Coated Glass as a Passive Air Sampler for Persistent Organic Pollutants, Environ. Sci. Technol., 2003, 37(11), 2486–2493 Search PubMed.
- Y.-G. Ma,
et al., Critical Review and Recommended Values for the Physical–Chemical Property Data of 15 Polycyclic Aromatic Hydrocarbons at 25 °C, J. Chem. Eng. Data, 2010, 55(2), 819–825 Search PubMed.
- M. A. Khairy and R. Lohmann, Field calibration of low density polyethylene passive samplers for gaseous POPs, Environ. Sci.: Processes Impacts, 2014, 16(3), 414–421 Search PubMed.
- Z. Bao,
et al., Modeling short-term concentration fluctuations of semi-volatile pollutants in the soil–plant–atmosphere system, Sci. Total Environ., 2016, 569–570, 159–167 Search PubMed.
- R. G. M. Lee,
et al., Measurement and Modeling of the Diurnal Cycling of Atmospheric PCBs and PAHs, Environ. Sci. Technol., 1998, 32(14), 2172–2179 Search PubMed.
- J. Liu,
et al., Diurnal and nocturnal variations of PAHs in the Lhasa atmosphere, Tibetan Plateau: Implication for local sources and the impact of atmospheric degradation processing, Atmos. Res., 2013, 124, 34–43 Search PubMed.
- S. J. Hayward, T. Gouin and F. Wania, Comparison of Four Active and Passive Sampling Techniques for Pesticides in Air, Environ. Sci. Technol., 2010, 44(9), 3410–3416 Search PubMed.
- T. Gouin,
et al., Passive and Active Air Samplers as Complementary Methods for Investigating Persistent Organic Pollutants in the Great Lakes Basin, Environ. Sci. Technol., 2005, 39(23), 9115–9122 Search PubMed.
- J. M. Armitage, S. J. Hayward and F. Wania, Modeling the Uptake of Neutral Organic Chemicals on XAD Passive Air Samplers under Variable Temperatures, External Wind Speeds and Ambient Air Concentrations (PAS-SIM), Environ. Sci. Technol., 2013, 47(23), 13546–13554 Search PubMed.
- C. Chaemfa,
et al., Field calibration of polyurethane foam (PUF) disk passive air samplers for PCBs and OC pesticides, Environ. Pollut., 2008, 156(3), 1290–1297 Search PubMed.
- K. M. Ellickson,
et al., Analysis of polycyclic aromatic hydrocarbons (PAHs) in air using passive sampling calibrated with active measurements, Environ. Pollut., 2017, 231, 487–496 Search PubMed.
- I. J. Allan,
et al., Photodegradation of PAHs in passive water samplers, Mar. Pollut. Bull., 2016, 105(1), 249–254 Search PubMed.
- M. E. Bartkow,
et al., Photodegradation of polyaromatic hydrocarbons in passive air samplers: field testing different deployment chambers, Environ. Pollut., 2006, 144(2), 371–376 Search PubMed.
- T. D. Behymer and R. A. Hites, Photolysis of polycyclic aromatic hydrocarbons adsorbed on simulated atmospheric particulates, Environ. Sci. Technol., 1985, 19(10), 1004–1006 Search PubMed.
- W. W. Brubaker and R. A. Hites, OH Reaction Kinetics of Polycyclic Aromatic Hydrocarbons and Polychlorinated Dibenzo-p-dioxins and Dibenzofurans, J. Phys. Chem. A, 1998, 102(6), 915–921 Search PubMed.
- J. Klánová,
et al., Assessing the Influence of Meteorological Parameters on the Performance of Polyurethane Foam-Based Passive Air Samplers, Environ. Sci. Technol., 2008, 42(2), 550–555 Search PubMed.
- K. Prevedouros,
et al., Seasonal and long-term trends in atmospheric PAH concentrations: evidence and implications, Environ. Pollut., 2004, 128(1), 17–27 Search PubMed.
- J. Masih,
et al., Seasonal Variation and Sources of Polycyclic Aromatic Hydrocarbons (PAHs) in Indoor and Outdoor Air in a Semi Arid Tract of Northern India, Aerosol Air Qual. Res., 2012, 12(4), 515–525 Search PubMed.
- L.-Y. Liu,
et al., Differences in spatiotemporal variations of atmospheric PAH levels between North America and Europe: Data from two air monitoring projects, Environ. Int., 2014, 64, 48–55 Search PubMed.
- S. Hazrati and S. Harrad, Calibration of polyurethane foam (PUF) disk passive air samplers for quantitative measurement of polychlorinated biphenyls (PCBs) and polybrominated diphenyl ethers (PBDEs): Factors influencing sampling rates, Chemosphere, 2007, 67(3), 448–455 Search PubMed.
- K. Pozo,
et al., Toward a Global Network for Persistent Organic Pollutants in Air:
Results from the GAPS Study, Environ. Sci. Technol., 2006, 40(16), 4867–4873 Search PubMed.
- L. B. Paulik,
et al., Emissions of Polycyclic Aromatic Hydrocarbons from Natural Gas Extraction into Air, Environ. Sci. Technol., 2016, 50(14), 7921–7929 Search PubMed.
- N. J. Herkert,
et al., Calibration and evaluation of PUF-PAS sampling rates across the Global Atmospheric Passive Sampling (GAPS) network, Environ. Sci.: Processes Impacts, 2018, 20(1), 210–219 Search PubMed.
- C. Persoon and K. C. Hornbuckle, Calculation of passive sampling rates from both native PCBs and depuration compounds in indoor and outdoor environments, Chemosphere, 2009, 74(7), 917–923 Search PubMed.
- P. Bohlin,
et al., Outdoor passive air monitoring of semi volatile organic compounds (SVOCs): a critical evaluation of performance and limitations of polyurethane foam (PUF) disks, Environ. Sci.: Processes
Impacts, 2014, 16(3), 433–444 Search PubMed.
- W. Wilcke, Synopsis Polycyclic Aromatic Hydrocarbons (PAHs) in Soil—a Review, J. Plant Nutr. Soil Sci., 2000, 163(3), 229–248 Search PubMed.
- T. D. Bucheli,
et al., Polycyclic aromatic hydrocarbons, black carbon, and molecular markers in soils of Switzerland, Chemosphere, 2004, 56(11), 1061–1076 Search PubMed.
- T. Gocht,
et al., Indications for pedogenic formation of perylene in a terrestrial soil profile: Depth distribution and first results from stable carbon isotope ratios, Appl. Geochem., 2007, 22(12), 2652–2663 Search PubMed.
- T. Gocht, O. Klemm and P. Grathwohl, Long-term atmospheric bulk deposition of polycyclic aromatic hydrocarbons (PAHs) in rural areas of Southern Germany, Atmos. Environ., 2007, 41(6), 1315–1327 Search PubMed.
Footnote |
† Electronic supplementary information (ESI) available. See DOI: 10.1039/d0ea00022a |
|
This journal is © The Royal Society of Chemistry 2021 |
Click here to see how this site uses Cookies. View our privacy policy here.