DOI:
10.1039/D0RA07659G
(Paper)
RSC Adv., 2021,
11, 1320-1331
A molecular journey to check the conformational dynamics of tau tubulin kinase 2 mutations associated with Alzheimer's disease†
Received
7th September 2020
, Accepted 28th November 2020
First published on 5th January 2021
Abstract
Proteins are one of the most vital components of biological functions. Proteins have evolutionarily conserved structures as the shape and folding pattern predominantly determine their function. Considerable research efforts have been made to study the protein folding mechanism. The misfolding of protein intermediates of large groups form polymers with unwanted aggregates that may initiate various diseases. Amongst the diseases caused by misfolding of proteins, Alzheimer's disease (AD) is one of the most prevalent neuro-disorders which has a worldwide impact on human health. The disease is associated with several vital proteins and single amino acid mutations. Tau tubulin kinase 2 (TTBK2) is one of the kinases which is known to phosphorylate tau and tubulin. The literature strongly supports that the mutations-K50E, D163A, R181E, A184E and K143E are associated with multiple important cellular processes of TTBK2. In this study, to understand the molecular basis of the functional effects of the mutations, we have performed structural modeling for TTBK2 and its mutations, using computational prediction algorithms and Molecular Dynamics (MD) simulations. The MD simulations highlighted the impact of the mutations on the Wild Type (WT) by the conformational dynamics, Free Energy Landscape (FEL) and internal molecular motions, indicating the structural de-stabilization which may lead to the disruption of its biological functions. The destabilizing effect of TTBK2 upon mutations provided valuable information about individuals carrying this mutant which could be used as a diagnostic marker in AD.
1 Introduction
Microtubule-Associated Protein (MAP) includes Tau, majorly responsible for stabilizing microtubules which are abundantly expressed in neuron cytoskeletons.1 Tau protein is divided into four sections such as, N-terminal region, a proline-rich domain, three/four repeat microtubule-binding domain (MBD), and the C-terminal region which consists of more than 85 phosphorylation sites.2 The hyper-phosphorylation of tau is one of the signatures of Alzheimer's disease (AD) occurrence. The result of tau hyper-phosphorylation is aggregation which leads to reduced affinity to microtubules and in turn destroys tau-associated cellular activities which abnormally affects axonal growth, vesicle transport, and signal propagation mediated by microtubules.3,4 Many scientific studies reported more than 20 kinases that are involved in this phosphorylation of AD sites involved in neurodegeneration associated with tau.5,6 Tau tubulin kinase 2 (TTBK2) belongs to the casein kinase 1 (CK1) superfamily which is a highly expressed in the several parts like granular cell layer, hippocampus, midbrain, cerebellum Purkinje cells, and the substantia nigra.6 The protein can phosphorylate tau and tubulin at numerous different sites that are majorly associated with AD. TTBK2 has two specific phosphorylation sites at S208 and S210 and can be expressed in high levels in many tissues including liver, pancreas, skeletal muscle, kidney, heart, and largely found in the cerebellum of the brain. Apart from AD, the protein TTBK2 has been identified as a crucial target in various diseases like cancer progression, TDP-43 accumulation and transporter stimulation and thereby to be an important pharmaceutical target.6,7
The amino acid optimizing in enzyme/proteins is one of the challenging tasks in modern scientific applications. There are several well-known methodologies that are successful in correlating the role of mutations associated with various diseases.8–10 The mutations at different sites on the protein sequence are one of the underpinning mechanisms for these correlations. The mutations at kinase domain of the TTBK2 protein, namely K50E, K143E, D163A, R181E, and A184E are majorly involved in AD and other neurodegenerative disorders.11,12 The selected mutations have been strongly implicated in the inhibition of endogenous protein kinase activity through a systematic pathological role in AD (Fig. 1).
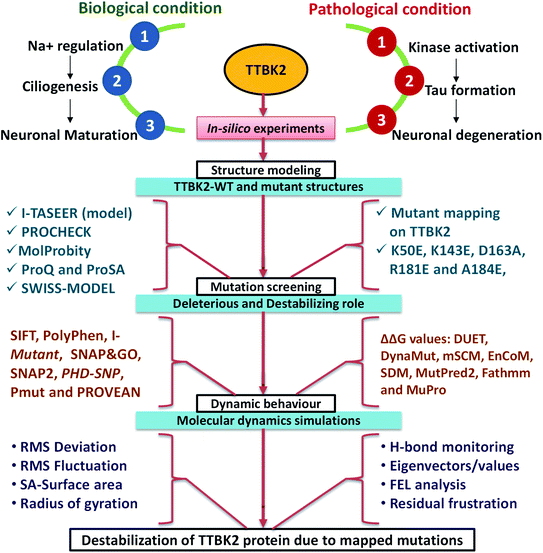 |
| Fig. 1 The schematic representation of TTBK2 in biological role, pathological condition and in silico analysis followed in the current study to know the de-stabilization caused by mapped mutants. | |
TTBK2 protein is structurally encoded with 1244 amino acid length (kinase domain from 1–300 residues) which lacks amino acid residues from 1–7 and residue 30 in the available crystal structure.13 Herein, we implemented a computational model to stereo-chemically characterize the effects of mutations on protein 3D structure. The good quality homology-based 3D structure of TTBK2 was generated using I-TASSER, the reliability of the model was checked using PROCHECK, ProSA (Protein structure analysis), ProQ (Protein Quality) and MolProbity.14 SwissPDB viewer was used to introduce the mutations on TTBK2 protein.15–19 To study the effect of mutations on TTBK2 protein structure, we used various online screening algorithms.20 To understand the phenotypic effect of the five TTBK2 mutations, we used computational predictions, which can provide a better understanding of deleterious effect, damaging role, and disease pathophysiology due to the mutations. We relied on GROMACS for MD simulations,21 to understand the stability and dynamical behavior of mutants on TTBK2 protein. MD simulations were conducted for the WT and the mutant structures to study the effects of the mutation on protein stability which revealed a significant impact on the native TTBK2 structure in aid to the prediction algorithms results.
Several parameters allied with MD simulations such as Root Mean Square Deviation (RMSD), Root Mean Square Fluctuation (RMSF), energy parameters, intramolecular hydrogen (H) bonds, solvent accessibility surface area (SASA), radius of gyration (Rg) and secondary structure assessment (SS), analysis of conformational dynamics, residual motion, and free energy landscape (FEL) analysis were implemented to assess the effect of mutations on the TTBK2 (Fig. 1). The study highlighted that the substitution of amino acids from K→E at position 50, K→E at position 143, D→A at position 163, R→E at position 181, A→E position at 184 could significantly impact the structure and function of TTBK2. The change of amino acids from aliphatic polar (Lys) to the positively charged polar residue (Glu), negatively charged polar residue (Asp) to aliphatic non-polar (Ala), positively charged polar residue (Arg) to negatively charged polar residue (Glu), aliphatic non-polar (Ala) residue to negatively charged polar residue (Glu), can affect the binding of small molecules, induce conformational changes, secondary structure deterioration on native TTBK2 structure. Summarily, the in silico analysis of the selected mutations of TTBK2 confirmed the structural and conformational destabilization of native TTBK2 structure by hindering the downstream processes of the protein function in AD. Therefore, the insightful findings from the study will provide direction for further investigations into the function and regulation of TTBK2, and the significant effect of K50E, K143E, D163A, R181E, and A184E on the protein stability.
2 Materials and methods
2.1. Bioinformatics tools and software
The online tools such as Sorting Intolerant From Tolerant (SIFT), PolyPhen, I-mutant, PROVEAN, SNP&GOs, SNAP2 and Pmut tools were explored to affirm the damaging role and disease associated mutants on TTBK2 protein.22 UniProt was approached to acquire the FASTA sequence further I-TASSER was employed to perform structural modeling. Protein Data Bank (PDB) database was used to retrieve the 3D structures of template, and PyMOL was used to visualize the complexes. DynaMut, MutPred2, Fathmm, and MuPro web servers were utilized to check the destabilizing effect of mutation on protein.23 MD simulation was performed using GROMACS 5.13.3 software package installed on High Performance Computing (HPC) Dell server with 128 GB RAM, and CUDA enabled NVIDIA (Model: Nvidia Tesla V100) graphics processing units (GPUs) 1 GPU Memory: Total 16 GB X 4.
2.2. Annotation of sequence and structural information
Three-dimensional (3D) structure of the protein is crucial in order to study its functionality, especially when trying to understand the effect of mutant on its overall structure and function. The primary FASTA sequence of TTBK2 consisting of 1244 native amino acids was retrieved from UniProt database (Q6IQ55) which includes the relevant information including the protein name, gene name, organism, sequence information, taxonomy, family and domains, and related molecular functions, biological processes, associated pathway and corresponding diseases.13
2.3. Protein models of WT and mutant structures
Computational modeling for TTBK2 was performed using I-TASSER to execute the 3D structure and was considered as Wild Type (WT) in the study. The reliable template for TTBK2 was obtained from PDB (6U0K) to generate homology models.13 The 3D structure was built through I-TASSER online tool using the template selected in the previous step.24 The top ranked models with high confidence score are scrutinized based on their resolution, RMSD, TM score and query coverage. The best model was evaluated using web-based quality checking tools, such as Ramachandran plot (PROCHECK), MolProbity, ProSA, ProQ and SWISS-MODEL.25,26 We mapped the deleterious mutations K50E, K143E, D163A, R181E, and A184E on TTBK2 at corresponding positions and mutant structure were constructed using in silico mutagenesis plug-in SWISS-Pdb Viewer.27
2.4. Functional relevance of mutant with in silico analysis
To predict the functional impact of selected mutations on the protein, we have explored the most widely accepted tools, such as SIFT, PolyPhen, I-mutant 2.0, PROVEAN, SNP & Go, SNAP2, PhD SNP and Pmut tool. SIFT algorithm was used to predict the effect of amino acid upon substitution that depicts protein function, by tolerance scores between 0 and 1. PolyPhen 2.0 predicts the possible impact of an amino acid change on the structure and functions of a protein and provides a high-quality sequence alignment pipeline to combine both sequence and structure. I-mutant 2.0 is a support vector machine (SVM) based server used for predicting protein stability changes arising from a single point mutation. PROVEAN server identifies the non-synonymous or indel variants that could be functionally important on protein. SNP & GO identified the effect of single point protein mutations responsible for the cause of in humans. SNAP2 and Pmut servers were implemented to understand the functional effects of the mutations and annotation of pathological variants on protein. DUET, mCSM, ENCoM, DynaMut, SDM, MutPred2, Fathmm, and MuPro online tools were also implemented to visualize and analyze the protein dynamics to calculate the structure-based stability changes upon mutations on TTBK2. The tools SIFT and I-mutant de-stability role of the mutations, PROVEAN and DynaMut examine the deleterious effect and PolyPhen, SNAP2 were used to understand the damaging role. Pmut and PhD SNP analysis was performed to understand the diseased state of the mutants on TTBK2 protein. Hence, we used different methods to evaluate the TTBK2 mutants to understand different and consensus effects on the native protein structure.
2.5. Molecular dynamics simulations
MD simulations were carried out by GROMACS 5.18.3 software package.21,28,29 The 3D structure of TTBK was collected and modeled by the I-TASSER online server30 to accurate the missing residues in the native PDB structure. The determination of charge states for the ionizable residues was performed using H++ calculation to understand protonation state of the protein.31 The WT and mutant topology parameter files were created by the CHARMM27 force field included with CMAP correction.32 The intermolecular (non-bonded) potential, represented as the sum of Lennard–Jones (LJ) force-based switching with cut-off distance range of 8 to 10 Å, pairwise Coulomb interaction and the long-range electrostatic force were determined by particle mesh Ewald (PME) approach.33 The real-space cutoff was set to 1.2 nm for the PME calculations.34 The velocity Verlet algorithm was applied for the numerical integrations and the initial atomic velocities were generated with a Maxwellian distribution at the given absolute temperature. Then the system immersed with the default TIP3P water model, and protein was placed at the center of the cubic grid box (1.0 nm3).35 The neutralization was performed to make concentration of the system to 0.15 M. The neutralized system was then subjected to energy minimization using the Steepest Descent (SD) and Conjugate Gradient (CG) algorithms utilizing a convergence criterion of 0.005 kcal mol−1 Å−1. The two-standard equilibration phase was carried out separately NVT (atom, volume, temperature) and NPT (atom, pressure–temperature) ensemble conditions such as constant volume and constant pressure for each complex similar time scale with LINear Constraint Solver (LINCS) to restrain the bonds involving hydrogen atoms.21 Nosé-Hoover thermostat and Parinello–Rahman barostat was applied to maintain the temperature and the pressure of the system, respectively. The system was maintained constant at 1 bar and 300 K, with a coupling time of τP = 2 ps, and τT = 1 ps, respectively. The Periodic Boundary Condition (PBC) used for integrating the equation of motion by applying the leap-frog algorithm with a 2 fs time step. Finally, to make the system ready for production the fixing of constraints is achieved with the relaxation of the grid box with water along with the counter ions.
The run of 200 ns were performed for each the WT and five mutant structures of TTBK2 and all the trajectories were recorded at every 4 ps for further validation. The calculations of RMSD, RMSF, SASA, Rg, intra-molecular hydrogen bond, FEL and density distributions were analyzed. The MD analysis with the above-mentioned parameters were conducted using GROMACS toolsets. The energy plots and graphs were marked and visualized using QtGrace visualization tool.
2.6. Principal component analysis (PCA)
PCA is one of the well-known statistical techniques that help in the reduction of complexity in analyzing the rigorous motion information in simulations which is essentially correlated with biological function. The eigenvectors calculations and eigenvalues that are identified by diagonalize matrix along with the projections of first two Principal Components (PC) were carried out using Essential Dynamics (ED) method. The free energy (Gα) was defined:
Gα = −kT ln[(qα)Pm(q)] |
where, k is the Boltzmann constant, T is the temperature of simulation. P(qα) corresponds to the estimate of probability density function extracted from histogram of the MD data that was constructed using joint probability distribution (PC1 and PC2 as reaction coordinates). Pmax(q) represents the probability of the most probable state.
The protein movements and the dynamic motions of WT and K50E, K143E, D163A, R181E, and A184E mutant structures throughout the trajectories in the subspace were further identified by Cartesian trajectory coordinates.
3 Results
The study was hypothesized to understand the underlying mechanism and molecular basis of TTBK2 mutations K50E, K143E, D163A, R181E, and A184E on the protein stability to aid AD. The various analysis results enumerated in this section show how the loss of TTBK2 stability may be an important factor for the occurrence of AD progression.
3.1. Prediction of the impact of the mutants
The SIFT and PolyPhen scores found that K50E, D163A, R181E, A184E and K143E specifically K50E showed high scores to reflect deleterious effects on the protein. The results revealed that the mutations K50E, K143E, D163A, R181E, and A184E are predicted to be producing functional impact, where K50E is predicted to be benign. All the mutants were showing high impact on the on TTBK2 protein function and pathophysiology of the disease which was confirmed from the obtained results of SNP&GO (3 to 7), SNAP2 (>80%) and Pmut tool (>70%-diseased) (Table 1). The impact of K50E, K143E, D163A, R181E, and A184E by amino acid substitutions on the biological function of the protein was predicted by PROVEAN has been at higher risk with high deleterious effects. I-mutant upshot the TTBK2 protein stability was showing destabilizing with alterations in the confidence score respectively, revealing protein damage effects. The stability changes due to the mutations on TTBK2 was further evaluated using Gibbs free energy (ΔΔG) by DUET, mCSM, DynaMut, SDM, MutPred2, Fathmm and MuPro web servers depicted a significant role in the destabilization (Table 2). Summarily, all the mutants were showing deleterious effects upon the amino acid alteration on TTBK2 protein and found to be deleterious, damaging and destabilizing on TTBK2 altering the structure and pathophysiology.
Table 1 Prediction scores of mutants on TTBK2 protein by in silico tools
S. no. |
Mutant |
SIFT |
PROVEAN |
PolyPhen |
SNP & GOs |
SNAP2 |
Pmut |
I-mutant |
PhD SNP |
1 |
K50E |
0.99 destabilizing |
−3.780 deleterious |
1.000 damaging |
7 diseased |
95% damaging |
0.88 diseased |
−0.36 destabilizing |
2 diseased |
2 |
K143E |
1.00 destabilizing |
−3.699 deleterious |
0.999 damaging |
6 diseased |
95% damaging |
0.88 diseased |
−0.80 destabilizing |
1 diseased |
3 |
D163A |
0.98 destabilizing |
−7.601 deleterious |
1.000 damaging |
7 diseased |
91% damaging |
0.88 diseased |
−0.02 destabilizing |
2 diseased |
4 |
R181E |
0.75 destabilizing |
−4.778 deleterious |
1.000 damaging |
6 diseased |
80% damaging |
0.82 diseased |
−1.16 destabilizing |
1 diseased |
5 |
A184E |
0.74 destabilizing |
−4.778 deleterious |
0.999 damaging |
3 diseased |
80% damaging |
0.70 diseased |
−0.87 destabilizing |
2 diseased |
Table 2 Dynamic protein stability prediction scores of mutants on TTBK2 protein
S. no. |
Mutant |
DUET |
mCSM |
ENCoM |
DynaMut |
SDM |
MutPred2 |
Fathmm |
MuPro |
1 |
K50E |
−0.521 kcal mol−1 |
−0.203 kcal mol−1 |
−4.127 kcal mol−1 |
−0.233 kcal mol−1 |
−0.57 destabilizing |
0.897 kcal mol−1 |
2.04 kcal mol−1 |
−0.46 destabilizing |
2 |
K143E |
−0.869 kcal mol−1 |
−1.141 kcal mol−1 |
−4.719 kcal mol−1 |
−0.255 kcal mol−1 |
0.02 destabilizing |
0.884 kcal mol−1 |
−0.21 kcal mol−1 |
−0.61 destabilizing |
3 |
D163A |
−0.269 kcal mol−1 |
−0.713 kcal mol−1 |
−4.893 kcal mol−1 |
−0.533 kcal mol−1 |
1.11 destabilizing |
0.920 kcal mol−1 |
−0.21 kcal mol−1 |
−0.70 destabilizing |
4 |
R181E |
−0.085 kcal mol−1 |
−0.039 kcal mol−1 |
−4.245 kcal mol−1 |
−0.165 kcal mol−1 |
−1.08 destabilizing |
0.927 kcal mol−1 |
2.55 kcal mol−1 |
−0.47 destabilizing |
5 |
A184E |
−1.447 kcal mol−1 |
−1.297 kcal mol−1 |
−4.024 kcal mol−1 |
0.814 kcal mol−1 |
−2.27 destabilizing |
0.909 kcal mol−1 |
2.48 kcal mol−1 |
−0.05 destabilizing |
3.2. Sequence alignment and homology modeling
BLAST-P search for TTBK2 protein was performed and the sequences with identity >70% and query coverage of >90% matches were subjected to homology modeling.36 Among a list of similar protein structures, 6U0K was selected as template as it was sharing best identity (86%) and query coverage (99%). Multiple sequence alignment of TTBK2 also projected that the selected template was sharing 99% of query coverage revealing the reliability of the template for modeling (Fig. SF1†). I-TASSER was used to generate target-template alignments and models for the TTBK2 protein. Top 10 models were analyzed and first model was selected with respect to the low confidence (C)-score, which is typically should be in range of −5 to 2, for a good model (Table ST1†). Our model had a C-score of 1.222 with high confidence of 100% revealed that the TTBK2 model is reliable, could be used in future molecular modeling studies.
3.3. Validation of modeled structure
The TTBK2 structure model obtained by I-TASSER was further validated with Ramachandran Plot (PROCHECK), ProSA, ProQ, MolProbity and SWISS-MODEL. PROCHECK analysis confirmed that the residues of the modeled structure were present in 99.2% of favored and allowed regions, whereas 0.8% of residues (2 residues) were observed in the disallowed region, revealing the good stereo-chemical quality. The MolProbity analysis of Ramachandran Plot also confirmed with the presence of 99.7% of residues were found in favored and allowed regions (1 residue in outlier) revealing the quality of the model to be reliable (Fig. 2). The ProSA analysis resulted Z-score of −7.54, which was greater than −4 indicating that modeled structure was refined with a minimum range of conformations of native crystal structure. Further, ProQ analysis provided a good resemblance to the most validated model with −p(LG) score of 5.716 with threshold score >4.0 suggesting the model with high quality. The obtained results from the validation tools were further corroborated with the SWISS-MODEL online tool which showed less than 3% stereo-chemical analysis, revealing the TTBK2 model is reliable (Fig. 3A).
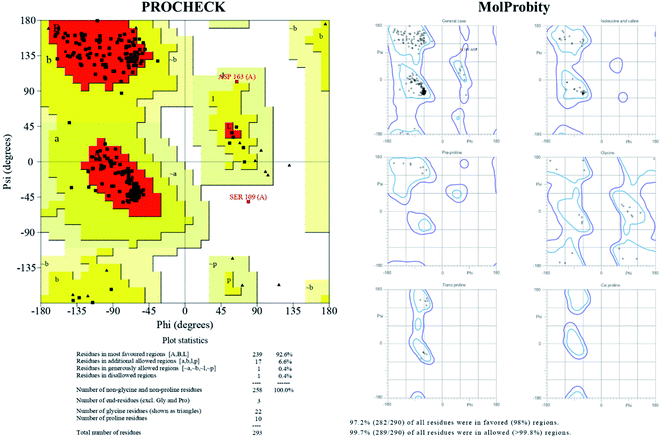 |
| Fig. 2 Ramachandran Plot statistics, stereo-chemical properties of TTBK2 model generated by I-TASSER and depiction of psi(φ)–phi(ψ) angle degrees validated through PDB-sum (PROCHECK) and MolProbity. | |
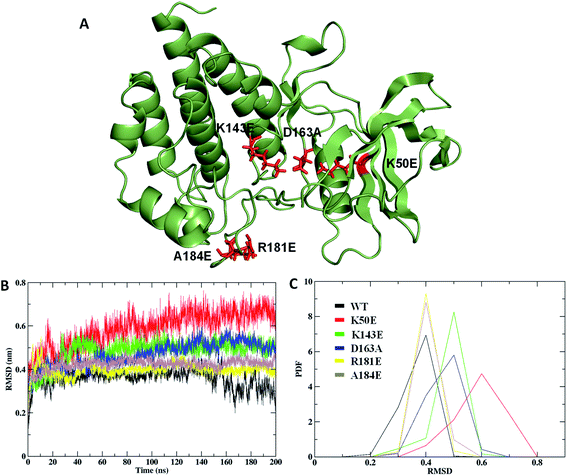 |
| Fig. 3 Modeled 3D structure elucidating the mutations, RMSD analysis of WT and mutants structures, (A) representation of mutations on TTBK2 modeled structure with green-wire model and mutations are illustrated by red color-sticks, (B) C-alpha conformation of RMSD, (C) comparative PDF average RMSD functions of WT and mutations. | |
3.4. Molecular Dynamics on WT and mutant TTBK2
3.4.1. Structural stability analysis. The effect of mutants on the native TTBK2 structure was studied in detail with the help of MD simulation allied parameters. The RMSD analysis revealed that the mutations K50E, K143E, D163A, R181E, and A184E were unstable with average range of ∼0.4 to 0.5 Å when compared with WT, which was found to be stable with ∼0.3 Å. Mutant K50E noticeably tends to show a high fluctuation of ∼0.5 nm, D163A and R181E shown a drift of ∼0.5 nm, followed by K143E with ∼0.4 nm, and A184E was with∼0.4 nm respectively (Fig. 3B). The study was further subjected to Probability Distribution Function (PDF) and revealed that the WT was within the threshold average PDF-RMSD values of 0.36 nm, whereas K50E, K143E, D163A, R181E and A184E structures revealed high fluctuations of 0.58 nm, 0.48 nm, 0.46 nm, 0.40 nm and 0.42 nm, respectively (Fig. 3C). The instability caused by the mutations are confirmed with the deviation analysis on TTBK2 which gives us space to think that there is deformation, leading to conformational changes on the protein leading to instability (Table ST2†).
3.4.2. Relative residue fluctuation. Relative residue fluctuations were determined to understand the flexibility of WT and mutants of TTBK2 to acquire the effect of Cα-RMSF of each residue. The declined drift of RMSF graph indicated huge increase in atomic movements of mutants causing instability when compared to WT. The mutant K50E presented high negative fluctuations at positions K50-S53. The mutant K143E displayed maximum negative drifts at residue positions such as L42, T43, E45, L49-A54, D141, K143, F170-G175, P180-A184, F186, R187, followed by the mutant D163A showed fluctuations at D40-V47, N46, V47, L49-A54, R140K143, R169-R181 and V183-I195. Further, the mutation R181E revealed maximum fluctuations at residue positions D40-E45, K50-A54, R140, D141, G165-A182, R187 and the mutant A184E displayed high negative drifts at positions L42-N46, L49-D141, K143, S145, M149, A167-T189 and I195, respectively (Fig. 4A–E). Summarily, the comparative residue fluctuation analysis revealed high degree of flexibility on mutants. Overall negative and positive values obtained from the RMSF analysis portrayed high impact on the rigidity of TTBK2 mutant structures.
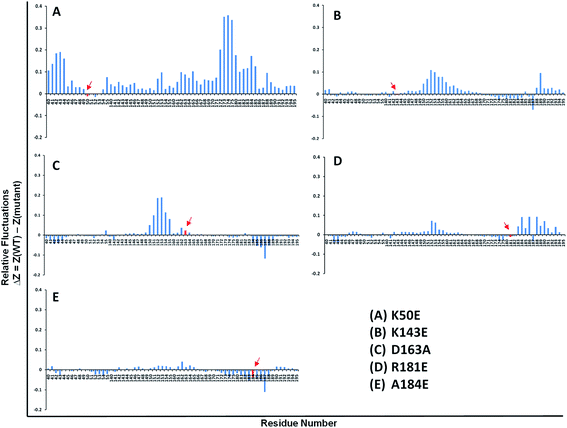 |
| Fig. 4 Relative residue fluctuations graphs of the TTBK2 mutants depicting high negative and positive drifts noted at total simulation time. The graphs (A)–(E) represent the relative residue fluctuations in the mutants K50E, K143E, D163A, R181E and A184E, respectively. | |
3.4.3. SASA and Rg analysis. The WT and mutant structures were subjected to SASA analysis where the core hydrophobic regions of both the systems were examined and found a significant alteration SASA. The mutants K50E, K143E, D163A, R181E and A184E revealed high fluctuations of SASA values with ranges from 161 nm2 to 165 nm2 in contrast to WT (165 nm2) (Fig. 5A). The PDF analysis of SASA on mutants revealed unsteady changes with the values of 161.52 nm2, 162.21 nm2, 164.37 nm2, 165.08 nm2, and 162.92 nm2, distinguishing with WT with 165.38 nm2 (Fig. 5B and Table ST2†). The fluctuations from the SASA and PDF-SASA results justified a high loss of hydrophobic contacts between the residues on the TTBK2 protein due to the substitution of amino acid.
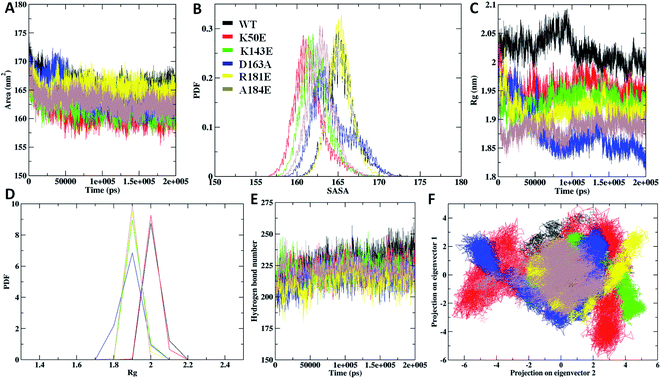 |
| Fig. 5 SASA and Rg analysis between WT and mutants of TTBK2 (A) SASA fluctuations per residue variation for WT and mutations, (B) comparative probability distribution-SASA, (C) the radius of gyration projection plot, (D) average PDF–Rg of WT and mutant structures, (E) the time evaluation of intra-molecular hydrogen bonds throughout total run time of 200 ns each, (F) the 2D projection plot between eigen vectors to mark conformational space of Cα-atom on TTBK2-WT and mutant structures. | |
The Rg analysis was carried out to understand the differences in compactness between WT and the mapped mutations. The results revealed K50E with highest Rg deviation of ∼1.9 nm, followed by K143E, D163A, R181E, and A184E mutants with average Rg range of ∼1.8 nm to ∼1.9 nm, differentiating with WT Rg value of ∼1.8 nm (Fig. 5C). The average PDF–Rg analysis accurate a high drift noticed on K50E with 1.95 nm, K143E with 1.92 nm, R181E with 1.88 nm, D163A with 1.91 nm, A184E with 1.89 nm, whereas WT with 2.02 nm, respectively (Fig. 5D and Table ST2†). Results from the above analysis suggested that mutant K50E is highly damaging the native TTBK2 leading to structural destabilization followed by K143E, D163A, R181E, and A184E could be responsible for the loss of compactness, suggesting a cumulative effect on core protein structure.
3.4.4. Secondary structure, H-bond and PCA analysis. The analysis was further made stronger with the secondary structural elements (SSE) which contribute to the shape of protein 3D structure. It was evident from the SSE analysis that mutants K50E projected deformation from helix to coil, sheets to coil and helix to bend around residues 145–150, 200, followed by K143E deformation from helix to coil/bend/turn around residue 100–105. The mutants D163A, R181E and A184E displayed conversation of helix to bend/turn/sheets positioned at 100–110, 150–200 and 250–200 residues, consequently, revealing differential changes when compared to WT structural elements (Fig. SF2†). The change in the number of intra-molecular hydrogen bonds formed in the WT and mutant structures were investigated (Fig. 5E). The overall analysis showed K50E with 221.75 H-bonds, followed by K143E with 221.81, D163A with 215.28, R181E with 210.91, and A184E with 221.60, whereas in the WT there were 227.25 H-bonds (Table ST3†). The analysis revealed the alterations in the hydrogen bonds in the mutants have a significant impact on the structure dynamics and instability of TTBK2.The study was investigated further for the essential subspace where the protein dynamics are noted with eigenvectors which are associated with the eigen-values. The dynamic behavior of WT and mutants were compared with the clusters and the results revealed that the clusters are well defined in the WT structures by covering minimum region where as the K50E, K143E, D163A, R181E and A184E mutants occupied maximum regions (Fig. 5F). Further the eigenvector1 and vector2 were plotted to understand the atomic fluctuation which enables the type of motion. The results revealed that K50E, K143E, D163A, R181E, and A184E mutations showed maximum fluctuation were noticed in comparison to WT TTBK2 throughout the MD simulations (Fig. SF3A and B†). A porcupine plot was generated to explain the differences in motions and magnitude between the WT and mutants (Fig. SF4†). The larger deviation patterns observed in mutants might be due to the unfold structure of TTBK2 which is crucial for proper function. The comparative study of WT and mutant TTBK2 amply high conformational changes in mutants of TTBK2 leading to consequential disturbance in internal atomic motion causing loss of protein stability.
3.5. Diagonalized covariance matrix and FEL analysis
The WT and mutant structures were further examined for diagonalized covariance matrix to know the positional fluctuations on protein Cα atoms. The atomic behavior of the residual motions was served with this analysis between WT and mutant structures. The analysis was differentiated with red and blue color representation where red implies small fluctuations between the atoms and blue imply large fluctuations. The amplitude and the intensity of WT was magnified with value of 0.276 nm2 whereas, higher differences were observed in mutants K50E, K143E, D163A, R181E, and A184E with 0.541 nm2, 0.355 nm2, 0.299 nm2, 0.314 nm2, 0.933 nm2, respectively (Fig. SF5†). The comparison of residual displacement between WT and mutants revealed the molecular deformation of TTBK2 on native structure. Further, the free energy changes of WT and K50E, K143E, D163A, R181E and A184E were explored with the MD simulations using FEL analysis through eigenvectors plots. The comparison studies indicated the presence of stable global free energy minima confined within one basin in WT. However, mutants including K50E, K143E, D163A, R181E, and A184E had wider basins of sample conformations with numerous meta-stable conformations associated with multiple energy minima (Fig. 6A–F). From the observations, it was evident that the mutations triggered structural destabilization of TTBK2, which in turn provoking the dysfunction of protein dynamics. The marked significant differences in the folding behavior of mutants indicated an unsteady conformation with altered energy minima leading to an unfolding behavior on the TTBK2 protein, leading to protein stability loss.
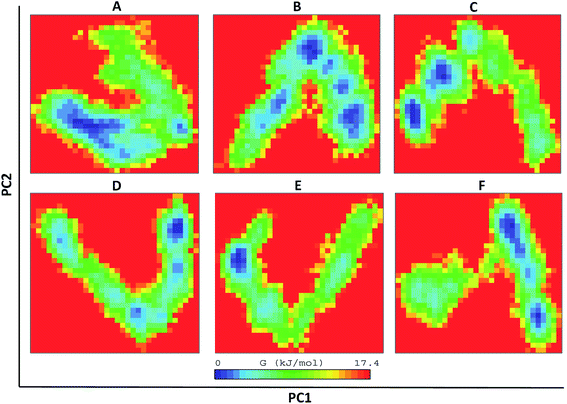 |
| Fig. 6 The direction of motion and magnitude analysis of WT and mutant structures with FEL analysis of (A) TTBK2, (B) K50E, (C) K143E, (D) D163A, (E) R181E and (F) A184E. | |
3.5.1. Density distribution and frustration analysis. The atomic density distribution analysis was investigated for WT and mutations of TTBK2 to know the changes in atomic orientation and distribution plotted using densmap. The partial density area of WT was observed to be stable with minimum values of 2.74 nm−3 whereas high density was noticed on mutations K50E, K143E, D163A, R181E and A184E with a range of 3.25 nm−3, 4.05 nm−3, 3.25 nm−3, 3.55 nm−3, 3.19 nm−3, respectively (Fig. 7A–F). The comparison of density distribution affirmed high impact of structural transition on TTBK2 leading to stability loss upon amino acid substitution.
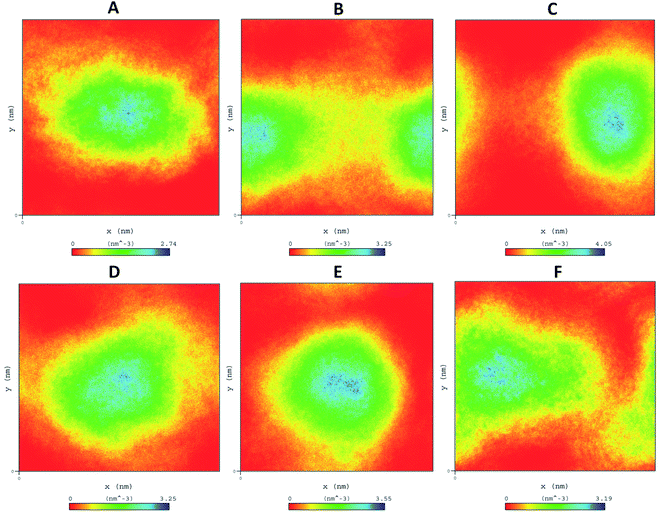 |
| Fig. 7 Density distribution of WT and mutant comparison throughout MD simulations (A) WT-TTBK2, (B) K50E, (C) K143E, (D) D163A, (E) R181E, (F) A184E. | |
The residue frustrations of TTBK2-WT and mutations resulted with a well global and local energy minima occurrence in WT when compared to mutants.37,38 The results showed maximum frustrations leading to loss of contacts in mutations that was noticed from ∼50–70 positions for K50E, ∼150–250 positions for K143E, ∼170–300 positions for D163A, ∼270–250 positions for R181E, and ∼170–200 positions for A184E, whereas gain of frustration was perceived on the WT structure at occurrence positions (Fig. SF6†). The residue frustration analysis showed an unsteady shift in the patterns at substitution positions of mutant with significant loss of contacts, leading to deterioration of TTBK2.
3.5.2. Dynamic cross-correlation matrices analysis. The conformational motions of the WT and mutant structures of TTBK2 were monitored by the dynamic cross-correlation matrices (DCCM) analysis (Fig. 8). The analysis were elucidated with two colour representations, where red indicates positive correlation of residue movements in same direction and blue indicates anti-correlation of residue movements in opposite direction.39 The intensity of the colour located in the diagonal lane signify the degree of atom movements.40 The results revealed that the mutants K50E, K143E, D163A, R181E, and A184E presented least correlation/anti-correlation movements of the residues in contrary to WT with strong correlation caused instability on native structure of TTBK2. The overall comparative dynamic correlation matrix enabled loss of contacts among the residues which hinted destabilization of TTBK2 structure upon mutation occurrence positions.
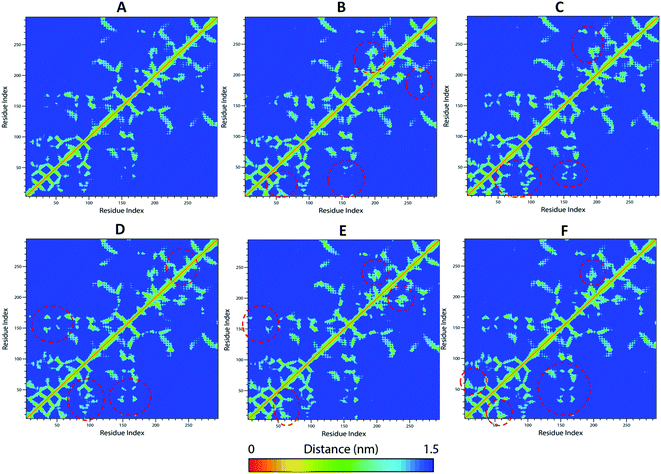 |
| Fig. 8 Cross-correlation matrix of mean smallest distance between the Cα-atoms of each amino acid residues of WT and mutants (A) WT-TTBK2, (B) K50E, (C) K143E, (D) D163A, (E) R181E, and (F) A184E. The major differences in the mutant structures with WT were marked with dashed red circles in their corresponding matrix. | |
4 Discussion
There are more than 20 kinases that are responsible to phosphorylate tau, in which most of them are involved in phosphorylation of AD sites. TTBK2 is a 1244 amino acid length with a kinase domain from 21–280 amino acid residues. It was essential to design a homology model to uncover the missing residues of TTBK2 protein where the kinase domain persists. I-TASSER generated a reliable and validated model of TTBK2 and the mutations were broadly studied through computational protocol to predict their impact on the native structure. The substitution of K50E, D163A, R181E, A184E and K143E of TTBK2 mutations at kinase N-terminal domain is the one of the crucial cause of un-stability of the protein.11 At biological level the mutations K50E, K143E, D163A, R181E, and A184E of TTBK2 affect the active site geometry and the interaction between the protein and the kinase domain is dented leading to misfolding of TTBK2 native structure.11–13
From the computational mutational analysis it was predicted that the mutations mapped on TTBK2 presented a significant perturbation on the native structure leading to stability loss promoting AD progression. Long-scale molecular simulations implemented on WT and mutants of the TTBK2, helped in studying the molecular level effects of the TTBK2 mutants with the help of monitoring various parameters like RMSD, RMSF, Rg, SASA, SSE and FEL analysis. The mutants K50E, K143E, D163A, R181E, and A184E categorized by the substitution of highly hydrophobic amino acids to hydrophilic amino acids and vice versa increased the flexibility and deviations which is evident in the RMSF and RMSD comparative analysis with the WT. The interchange of charges might be the cause of loss in helix upon the point mutations at respective occurrence, which corroborated with the prediction algorithm outcome too. Based on the obtained results it was evident that more flexible conformations were caused due to the mutations, this information will be useful for the ligand/drug binding the TTBK2 cleft and its inhibitor designing. The large oscillations in Rg and low SASA values displayed that the native protein TTBK2 might be undergoing a significant structural transition which was further supported by the outcomes of conformational dynamics, residual motion and atomic density maps. The longer variations depicted from the above results might be the reason for the endurance of structural transition in the mutants, differentiated with WT. The time dependent H-bond formation during the simulations on WT and mutants comprehend a strong relation between the bond formation and flexibility of the protein. The analysis also demonstrated that the mutant structures were forming a less number of H-bonds throughout the stipulated simulation time period.
Moreover, the results from the corresponding eigenvalues also projected the level of variation and dynamic nature of TTBK2 in the simulation system and were completely restricted on WT and the wide basins with multiple wells were observed in mutants indicating loss of stability. The secondary structure changes in mutants were showing high structural plasticity reflecting a high degree of flexibility on TTBK2 protein. The differences in WT and the mutations indicate that the transition path of all the mutant structures from starting conformations to the final conformations were not being identical, revealing the influence of amino acid change from K50, K143, D163, 181E, A184 to 50E, 143E, 163A, 181E, 184E at respective positions, which also affirmed the destabilization effect of the mutations on the structural and functional dynamics of TTBK2. Overall the results illustrated that the substitution of amino acids were highly affecting the structural behavior of protein to cause significant structural disruptions. Thus, the study offers a dynamic and molecular level insight on the effects of TTBK2 protein upon mutations that are associated with Alzheimer's disease consequential, to aim the studies on designing of novel drugs.
Author contributions
SA contributed to extensive in silico exercises, MD simulations investigations, and write-up in the manuscript. HK and VK contributed to write-up and corrections in the manuscript. DG contributed to the designing the hypothesis, major inputs, correspondence, and entire corrections in the manuscript.
Conflicts of interest
The authors declare no conflict of interest
Acknowledgements
SA, Research Associate, is thankful for the support and financial assistance (2019-6039 File No. ISRM/11(83)/2019) from Indian Council of Medical Research, India. HK is the recipient of Women Scientist awarded from DBT-BioCARe (No. BT/PR31715/BIC/101/1233/2019), Department of Biotechnology, India. DG is endowed by the Department of Biotechnology (Grant No. BT/BI/04/001/2018), Government of India.
References
- H. Shigematsu, T. Imasaki, C. Doki, T. Sumi, M. Aoki, T. Uchikubo-Kamo, A. Sakamoto, K. Tokuraku, M. Shirouzu and R. Nitta, J. Cell Biol., 2018, 217, 4155–4163 CrossRef CAS.
- K. Liu, Y. Liu, L. Li, P. Qin, J. Iqbal, Y. Deng and H. Qing, Biochim. Biophys. Acta, Mol. Basis Dis., 2016, 1862, 192–201 CrossRef CAS.
- Y. Kitagishi, A. Nakanishi, Y. Ogura and S. Matsuda, Alzheimer's Res. Ther., 2014, 6, 35 CrossRef.
- M. Pawlowski, S. G. Meuth and T. Duning, Diagnostics, 2017, 7, 42 CrossRef.
- N. F. Liachko, P. J. McMillan, T. J. Strovas, E. Loomis, L. Greenup, J. R. Murrell, B. Ghetti, M. A. Raskind, T. J. Montine and T. D. Bird, PLoS Genet., 2014, 10, e1004803 CrossRef.
- J.-C. Liao, T. T. Yang, R. R. Weng, C.-T. Kuo and C.-W. Chang, BioMed Res. Int., 2015, 2015, 575170 Search PubMed.
- S. Ikezu and T. Ikezu, Front. Mol. Neurosci., 2014, 7, 33 Search PubMed.
- H. Kanipakam, K. Sharma, T. Thinlas, G. Mohammad and M. Q. Pasha, J. Biomol. Struct. Dyn., 2020, 1–16 Search PubMed.
- M. Amir, S. Ahamad, T. Mohammad, D. S. Jairajpuri, G. M. Hasan, R. Dohare, A. Islam, F. Ahmad and M. I. Hassan, J. Biomol. Struct. Dyn., 2019, 1–10 Search PubMed.
- S. Ahamad, A. Islam, F. Ahmad, N. Dwivedi and M. I. Hassan, Comput. Biol. Chem., 2019, 78, 398–413 CrossRef CAS.
- M. Bouskila, N. Esoof, L. Gay, E. H. Fang, M. Deak, M. J. Begley, L. C. Cantley, A. Prescott, K. G. Storey and D. R. Alessi, Biochem. J., 2011, 437, 157–167 CrossRef CAS.
- H. Houlden, J. Johnson, C. Gardner-Thorpe, T. Lashley, D. Hernandez, P. Worth, A. B. Singleton, D. A. Hilton, J. Holton and T. Revesz, Nat. Genet., 2007, 39, 1434 CrossRef CAS.
- D. J. Marcotte, K. A. Spilker, D. Wen, T. Hesson, T. A. Patterson, P. R. Kumar and J. V. Chodaparambil, Acta Crystallogr., Sect. F: Struct. Biol. Commun., 2020, 76, 103–108 CrossRef CAS.
- V. B. Chen, W. B. Arendall, J. J. Headd, D. A. Keedy, R. M. Immormino, G. J. Kapral, L. W. Murray, J. S. Richardson and D. C. Richardson, Acta Crystallogr., Sect. D: Biol. Crystallogr., 2010, 66, 12–21 CrossRef CAS.
- Y. Zhang, BMC Bioinf., 2008, 9, 40 CrossRef.
- R. A. Laskowski, M. W. MacArthur, D. S. Moss and J. M. Thornton, J. Appl. Crystallogr., 1993, 26, 283–291 CrossRef CAS.
- M. Wiederstein and M. J. Sippl, Nucleic Acids Res., 2007, 35, W407–W410 CrossRef.
- P. Larsson, M. J. Skwark, B. Wallner and A. Elofsson, Proteins: Struct., Funct., Bioinf., 2009, 77, 167–172 CrossRef CAS.
- N. Guex, M. C. Peitsch and T. Schwede, Electrophoresis, 2009, 30, S162–S173 CrossRef.
- M. Amir, S. Ahmad, S. Ahamad, V. Kumar, T. Mohammad, R. Dohare, M. F. Alajmi, T. Rehman, A. Hussain and A. Islam, J. Biomol. Struct. Dyn., 2020, 38, 1514–1524 CrossRef CAS.
- M. J. Abraham, T. Murtola, R. Schulz, S. Páll, J. C. Smith, B. Hess and E. Lindahl, SoftwareX, 2015, 1, 19–25 CrossRef.
- G. R. C. Pereira, G. D. B. Tavares, M. C. de Freitas and J. F. De Mesquita, PLoS One, 2020, 15, e0229730 CrossRef CAS.
- J. J. Galano-Frutos, H. García-Cebollada and J. Sancho, Briefings in bioinformatics, 2019 Search PubMed.
- J. Yang and Y. Zhang, Nucleic Acids Res., 2015, 43, W174–W181 CrossRef CAS.
- R. A. Laskowski, J. A. C. Rullmann, M. W. MacArthur, R. Kaptein and J. M. Thornton, J. Biomol. NMR, 1996, 8, 477–486 CrossRef CAS.
- S. Bienert, A. Waterhouse, T. de Beer, G. Tauriello, G. Studer, L. Bordoli and T. Schwede, Nucleic Acids Res., 2018, 46, W296–W303 CrossRef.
- N. Guex and M. C. Peitsch, Electrophoresis, 1997, 18, 2714–2723 CrossRef CAS.
- S. Pronk, S. Páll, R. Schulz, P. Larsson, P. Bjelkmar, R. Apostolov, M. R. Shirts, J. C. Smith, P. M. Kasson and D. van der Spoel, Bioinformatics, 2013, btt055 Search PubMed.
- B. Hess, C. Kutzner, D. van der Spoel and E. Lindahl, J. Chem. Theory Comput., 2008, 4, 435–447 CrossRef CAS.
- T. Schwede, J. Kopp, N. Guex and M. C. Peitsch, Nucleic Acids Res., 2003, 31, 3381–3385 CrossRef CAS.
- J. C. Gordon, J. B. Myers, T. Folta, V. Shoja, L. S. Heath and A. Onufriev, Nucleic Acids Res., 2005, 33, W368–W371 CrossRef CAS.
- K. Vanommeslaeghe, E. Hatcher, C. Acharya, S. Kundu, S. Zhong, J. Shim, E. Darian, O. Guvench, P. Lopes and I. Vorobyov, J. Comput. Chem., 2010, 31, 671–690 CAS.
- J. Lee, X. Cheng, J. M. Swails, M. S. Yeom, P. K. Eastman, J. A. Lemkul, S. Wei, J. Buckner, J. C. Jeong and Y. Qi, J. Chem. Theory Comput., 2016, 12, 405–413 CrossRef CAS.
- H. Wang, H. Nakamura and I. Fukuda, J. Chem. Phys., 2016, 144, 114503 CrossRef.
- D. J. Price and C. L. Brooks III, J. Chem. Phys., 2004, 121, 10096–10103 CrossRef CAS.
- S. F. Altschul, W. Gish, W. Miller, E. W. Myers and D. J. Lipman, J. Mol. Biol., 1990, 215, 403–410 CrossRef CAS.
- J. Vannimenus and G. Toulouse, Journal of Physics C: Solid State Physics, 1977, 10, L537 CrossRef.
- J. D. Bryngelson, J. N. Onuchic, N. D. Socci and P. G. Wolynes, Proteins: Struct., Funct., Bioinf., 1995, 21, 167–195 CrossRef CAS.
- L. Dorosh and M. Stepanova, Mol. BioSyst., 2017, 13, 165–182 RSC.
- Q. Shao, J. Mater. Chem. B, 2020, 8(31), 6934–6943 RSC.
Footnote |
† Electronic supplementary information (ESI) available. See DOI: 10.1039/d0ra07659g |
|
This journal is © The Royal Society of Chemistry 2021 |
Click here to see how this site uses Cookies. View our privacy policy here.