DOI:
10.1039/D0RA08769F
(Paper)
RSC Adv., 2021,
11, 2453-2461
Characterizing the structure–activity relationships of natural products, tanshinones, reveals their mode of action in inhibiting spleen tyrosine kinase†
Received
15th October 2020
, Accepted 5th January 2021
First published on 14th January 2021
Abstract
The cytosolic non-receptor protein kinase, spleen tyrosine kinase (SYK), is an attractive drug target in autoimmune, inflammatory disorder, and cancers indications. Here, we employed pharmacophore-based drug screening combined with biochemical assay and molecular dynamics (MD) simulations to identify and characterize inhibitors targeting SYK. The built pharmacophore model, phar-TanI, successfully identified tanshinone (TanI (IC50 = 1.72 μM)) and its analogs (TanIIA (IC50 = 3.2 μM), ST32da (IC50 = 46 μM), and ST32db (IC50 = 51 μM)) which apparently attenuated the activities of SYK in vitro. Additionally, the MD simulations followed by Ligplot analyses revealed that TanI and TanIIA interfered SYK activity through binding deeply into the active site. Besides, TanI and TanIIA mainly interact with residues L377, A400, V433, M448, M450, A451, E452, L453, G454, P455, and L501, which are functional hotspots for structure-based inhibitor optimization against SYK. The structure–activity relationships (SAR) study of the identified SYK inhibitors demonstrated that the pharmacophore model, phar-TanI is reliable and precise in screening inhibitors against SYK. This study disclosed the structure–function relationships of tanshinones from Traditional Chinese Medicine (Danshen), revealing their binding site and mode of action in inhibiting SYK and provides applicability in developing new therapeutic agents.
Introduction
Spleen tyrosine kinase (SYK) is a nonreceptor cytoplasmic tyrosinase kinase mainly expressed in mast cells, monocytes, neutrophils and B-cells.1–5 SYK is an essential mediator of immunoreceptor signaling and has been reported as a feasible therapeutic target in autoimmune, oncology, and allergic indications.6–8 Upon immunoreceptor stimulation, the cytoplasmic domain of immunoreceptor tyrosine-based activation motif (ITAMs) is activated by SRC family kinase, resulting in the recruitments of SYK through the SH2 domain.9–11 The activated SYK subsequently propagates signaling cascade to regulate immune cell function via phosphorylation of targets (e.g., B-cell linker (BLNK)), and later triggers the Bruton's tyrosine kinase (Btk), phosphatidylinositol 3-kinase (PI3K), phospholipase-C gamma (PLCγ), and mitogen-activated protein kinase (MAPK) pathways.10,12,13 SYK contributes to various cellular response, such as cell proliferation, differentiation, cytoskeletal rearrangement, cytokine release, histamine release, reactive oxygen species (ROS) production, and phagocytosis.14,15
Inhibition of SYK reveals the high potential to treat autoimmune and inflammatory disorder.16 This has led to academic institutions and pharmaceutical companies becoming involved in developing SYK inhibitors. Recently, several SYK inhibitors were reported, such as naphthyridines,17 pyrazolopyrazines,18 and heteroaryl carboxamides.15,19 Especially, GS-9973,14 PRT062607 (ref. 20) and TAK-659 (ref. 21) are in the progress of human clinical trials. For now, only one chemically synthetic inhibitor, Fostamatinib 4, is approved by FAD for the treatment of immune thrombocytopenia;22 the current available drugs and are very limited. Thus, developing new/novel and safe SYK inhibitors are needed and will benefit in the treatments of several diseases (allergic asthma, arthritis, chronic lymphocytic leukemia, rhinitis, and rheumatoid). Unlike the chemically synthetic inhibitors, natural products and Traditional Chinese Medicine (TCM) are safer and free from adverse side effects for new drug development. Particularly, the TCM is unique in Taiwan and China; remarkably different from the chemical drugs of Western countries. The natural products extracted from TCM, such as artemisinin, berberine, ginkgolide, and paclitaxel exert apparent activities in disease treatments.23–25 Therefore, TCM could be of great potential in developing novel and potent SYK inhibitors.
Recently, Wang et al. conducted a combining approached which utilized the docking-based virtually screening to identify SYK inhibitors.26 They found tanshinone I (TanI) showed activity in inhibiting SYK and exerted anti-mast cell degranulation activity in vitro. However, the mode of action of TanI against SYK was not well-characterized and unclear. Here, we employed structure-based pharmacophore modeling to explore the binding site of TanI towards SYK. We constructed a precise pharmacophore model, phar-TanI revealing key functional groups of TanI interacting with SYK. Additionally, ligand–pharmacophore mapping against natural products databases identified 5 natural products showing comparable SYK inhibitory abilities to that of TanI. Our molecular dynamics simulations along with Ligplot analyses characterized and depicted the structure–activity relationships (SAR) of the identified inhibitors to SYK. The observed results demonstrated that the built pharmacophore model (phar-TanI) is efficient and precise in screening potent SKY inhibitors. Also, SAR analysis annotated the binding mode of SYK and TanI. Our study provides evidence-proved information for structural optimization and modification to enhance the activity of inhibitors against SYK and will be helpful in developing new therapeutics.
Materials and methods
Natural product databases and ligands preparations for computer-aided drug screening
A total of 105
911 compounds were retrieved from natural product databases, including 1496 compounds from Specs (http://www.specs.net), 11
247 from ACD (http://www.ac-discovery.com/), 144 compounds from ICC (http://indofinechemical.com/), 14
084 compounds from PNP (http://www.princetonbio.com/), 74
940 compounds from the InterBioScreen (IBS, http://www.ibscreen.com) diversity set, and 4000 compounds from TCM (Taiwan Chinese medicine, http://www.nricm.edu.tw/bin/home.php). The sketch molecules and prepare ligands modules implemented in Discovery Studio 3.5 (Accelrys Software, Inc., San Diego, CA, USA) were used to create the molecular structures of all compounds. The preparation of compounds for docking analysis was processed by three steps: (1) two-dimensional (2D) structures were converted into three-dimensional (3D) structures, (2) charges were calculated, and (3) H atoms were added.
Molecular docking
The molecular docking was performed using GOLD docking program (Cambridge Crystallographic Data Center (CCDC), version 5.1). The complex structure of SYK-CG9 (PDB ID 4PUZ) was used as the protein model for docking. The residues within 0.5 Å of CG9 were selected and defined as the docking site. The performance of the docking process was validated by isolating the coordinate of the co-crystallized inhibitor, CG9 and redocked into the active site of SYK (with the GoldScore scoring function was employed for rigid docking where the protein structure was held rigid and the ligand conformations were generated by GoldScoring function). The root-mean-square deviation (RMSD) between the docked and the co-crystallized CG9 was <0.5 Å. Later the modelled SYK-TanI complex structure was also built by GOLD docking program with all the parameter same with that of re-docked procedure of CG9.
Receptor–ligand pharmacophore generation
We used the determined SYK-inhibitor complex structures (SYK-CG9 (PDB ID 4PUZ)) to build the pharmacophore models, containing the interactive features, for screening SYK inhibitors. The receptor–ligand pharmacophore generation module of Discovery Studio 3.5 (Accelrys Software, Inc., San Diego, CA, USA) was employed to build the pharmacophore models. The complex structure of SYK-CG9 (PDB ID 4PUZ) was used to generate the receptor–ligand pharmacophore models. The SYK structure was served as the “Input Receptor”, and the inhibitor, CG9, was utilized as the “Input Ligand”, separately. The “Minimum Features” and “Maximum Features” were set to 4 and 10, respectively, and the “Maximum Pharmacophores” set was to 10. The “fast method” was applied for conformation generation with “rigid fitting method”. The rest parameters were set as default during the receptor–ligand pharmacophore generation process.
Ligand pharmacophore mapping
Consequently, the built pharmacophore model, phar-TanI, was subjected to ligand-pharmacophore mapping (pharmacophore-based drug screening). All the molecules from natural products databases (105
911 molecules) were fit to the built pharmacophore model, phar-TanI, with the fitting method set to “flexible” and all other parameter were remained as default setting.
Molecular dynamics simulations
The crystal structure of SYK (PDB ID 4PUZ) was employed for GOLD docking and molecular dynamics (MD) simulations. However, there are two segments (N406-D410 and T530-G532) missing in the structure. Therefore, we firstly modeled the missing loop by using Homology Modeling module of Discovery Studio 3.5 (Accelrys Software, Inc., San Diego, CA, USA). During the structural modeling, the protein sequence of SYK was downloaded from the FASTA sequence of Protein Data Bank (PDB ID 4PUZ). The crystal structure of SYK (PDB ID 4PUZ) was used as the template for homology modeling. Subsequently, the built structure model with lowest energy was chosen for further ligand-pharmacophore (phar-TanI) mapping and MD simulations. The SYK-inhibitor complexes obtained from ligand-pharmacophore mapping were firstly subjected to solvation (Discovery Studio 3.5) with orthorhombic cell shape under CHARMm forcefield. Consequently, the SYK-inhibitor complex was solvated with 6680 waters, 17 sodium atoms, and 19 chloride atoms. Furthermore, the solvated complex structure was subjected to Standard Dynamics Cascade (Discovery Studio 3.5) with default parameter setting and followed by Dynamics (Production) for 10 ns simulation times with 2 ps as save results interval. Finally, the total energy and potential energy changes as a function of time were analyzed and plotted by Trajectory analysis (Discovery Studio 3.5). The binding affinities of SYK and inhibitors after MD simulations were determined by Calculate Binding Energies module of Discovery Studio 3.5.
In vitro kinase inhibition assays
The luminescent kinase assay was carried out to determine the ADP produced in ADP-Glo™ kinase assay kit (Promega, Madison, WI, USA). During the assay, ADP generated by SYK will be converted to ATP, which then interact with luciferase and producing light. Thus, inhibitors attenuate SYK activity will reduce the luminescent signals. The inhibitory assay was conducted in white 384-well plate (Corning #3824, Corning Glass Works, Corning, NY, USA). The buffer (40 mM Tris pH 7.5, 20 mM MgCl2, 0.1 mg ml−1 BSA and 50.0 μM DTT) was used to conducted SYK kinase reaction. About 2 μl tested compound (in kinase buffer with 5% DMSO) and 4 μl SYK (1 ng μl−1 in kinase buffer) were mixed and incubated at 25 °C for 15 min. Subsequently, 4.0 μl substrate (0.2 μg μl−1 polypeptide (4
:
1 Glu, Tyr) in kinase buffer with 10.0 μM ATP) was added to initiate the kinase reaction at 37 °C for 1 hour. Later, 5 μl AGP-Glo™ reagent was added into SYK reaction solution to terminate the reaction and then incubate for another 40 min to complete deplete all ATP. Eventually, the kinase detection reagent (10.0 μl) was added and incubated for one more hour. The luminescent signal was determined using microplate reader (CLARIOstar® Plus, BMG LABTECH).
Results
Receptor–ligand pharmacophore generation
For now, only one highly selective and orally efficacious inhibitor, CG9 of SYK is currently being examined in clinical trials.27,28 Additionally, the complex structure of SYK-CG9 was determined, providing significant information for developing new SYK inhibitors. The computer-aided drug design (CADD)—virtual high-throughput screening and pharmacophore modeling—is powerful in screening bioactive inhibitors. CADD is with low cost and less time consuming, considerably accelerating the pace of investigations of inhibitors with specific biological activity. In silico virtual high-throughput screening (molecular docking) is able to evaluate the potential biological activities of compounds based on their structural properties. However, to more precisely screen potent inhibitors against SYK, the functionally essential features responsible for the interactions between SYK and known inhibitors (such as, CG9 (PDB ID 4PUZ)) should be considered. The receptor–ligand pharmacophore generation is precise to explore the functionally essential features required for the interactions of protein and ligand. Here, we employed pharmacophore modeling to explore the bioactive pharmacophore scaffold of SYK interacting with CG9. The complex structure of SKY-CG9 was presented and compared with the reported model of SYK-TanI as shown in Fig. 1. During the receptor–ligand pharmacophore generation, the SYK moiety was used as the receptor, and the CG9 was served as the ligand. Successively, pharmacophore model, phar-CG9 was rationally generated, which contains 2 hydrogen-bond donor, 1 hydrogen-bond acceptors, and 3 hydrophobic features (Fig. 2A and 3A).
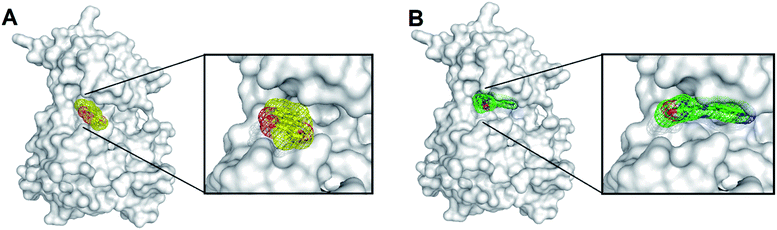 |
| Fig. 1 The complex structures of SYK-inhibitors. (A) The natural product, TanI was re-docked into the active site of SYK by using the same method describe previously.26 (B) The atomic complex structure of SYK-CG9 (PDB ID 4PUZ). (In panel (A) and (B), SYK was shown in white surface; TanI (yellow) and CG9 (green) were presented in meshes.) | |
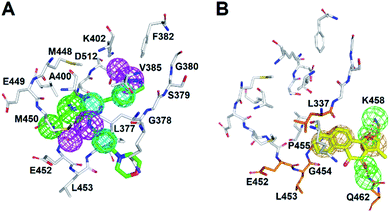 |
| Fig. 2 The pharmacophore model of SYK-CG9. (A) Receptor-ligand pharmacophore generation of phar-CG9 was based on SYK-CG9 complex structure (PDB ID 4PUZ). CG9 is shown in green stick. (B) The pharmacophore model of re-docked TanI (light-yellow) by using the same method described previously.26 (In panels (A) and (B), the mesh spheres in red, blue, magenta, green, and yellow represent positive-charged, negative-charged, hydrogen-bond donor, hydrogen-bond acceptor, and ring aromatic features, respectively. The active site residues of SYK were shown in thin sticks.) | |
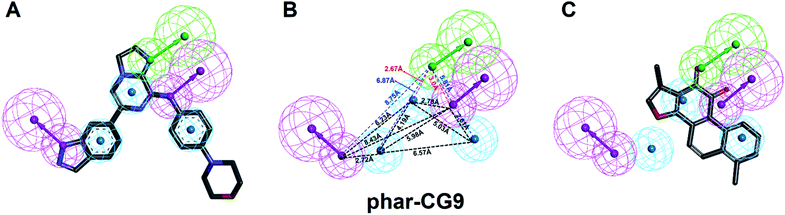 |
| Fig. 3 The pharmacophore model, phar-CG9. (A) The built pharmacophore model, phar-CG9 based the complex structure of SYK-CG9 (PDB ID 4PUZ). (B) The distances between these features of phar-CG9 were measured and labelled by dashed lines. (C) The ligand pharmacophore mapping result of TanI on to phar-CG9. (Pharmacophore features are colored as follows: hydrogen-bond acceptor, green; hydrogen-bond donor, magenta; hydrophobic group, cyan.) | |
Ligand-pharmacophore mapping
The natural product, TanI was observed exerting inhibitory activity against SYK in vitro,26 while its precise binding site and detailed mode of action was unclear. Here, we performed ligand-pharmacophore (phar-CG9) mapping to probe the possible binding site of TanI on SYK. The result showed that TanI fitted well with one hydrogen-bond donor, one hydrogen-bind receptor, and two hydrophobic features at the upper right region of phar-CG9 (fitting value = 2.21) (Fig. 3C). Notably, in this pose and orientation, TanI could bury deeply at the active site pocket of SYK. Consequently, these fitted features were grouped into a pharmacophore model, phar-TanI (Fig. 4B) and was applied for screening the potential inhibitors from natural products databases (105
911 compounds) through ligand-pharmacophore screening. The resultant 5 hits (Fig. 4), TanIIA, ST32da, ST32db, ST64, and WTS32 observed to better map with the phar-TanI (the hierarchy of fitness is TanI = TanIIA > ST32da = ST32db > ST64 = WTS32), were further subjected to functional examinations.
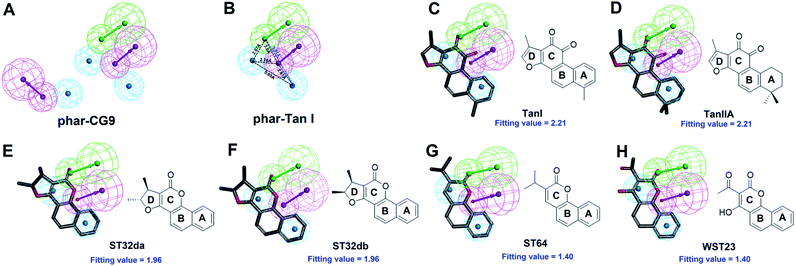 |
| Fig. 4 Pharmacophore-based inhibitor screening. (A) The built pharmacophore model, phar-CG9. (B) The pharmacophore scaffold of phar-TanI. (C)–(H) The ligand pharmacophore (phar-TanI) mapping results of TanI, TanIIA, ST32da, ST32db, ST64, and WST23. (Pharmacophore features are colored as follows: hydrogen-bond acceptor, green; hydrogen-bond donor, magenta; hydrophobic group, cyan.) | |
Inhibitory ability against SYK
We accessed the abilities of TanI along with the 5 identified hits in inhibiting SYK activity. In the presents of inhibitors (5 μM), TanI, TanIIA, ST32db, ST32da, WTS23 and ST64, the remained SYK activities were 37.0 ± 2.3, 44.7 ± 3.3, 72.0 ± 2.0, 67.0 ± 2.7, 72.9 ± 2.7 and 86.0 ± 3.7%, respectively (Fig. 5A). Subsequently, we examined the inhibitory capabilities of these compounds as a function of concentrations. The result demonstrated that all the tested compounds inhibited SYK in the dose-dependent manner, and the determined IC50 values were 1.72 ± 0.6, 3.2 ± 0.8, 46 ± 1.2, 51 ± 3.4 μM for TanI, TanIIA, ST32db, and ST32da. Whereas the IC50 for WTS23 and ST64 are over 100 μM (Fig. 5B).
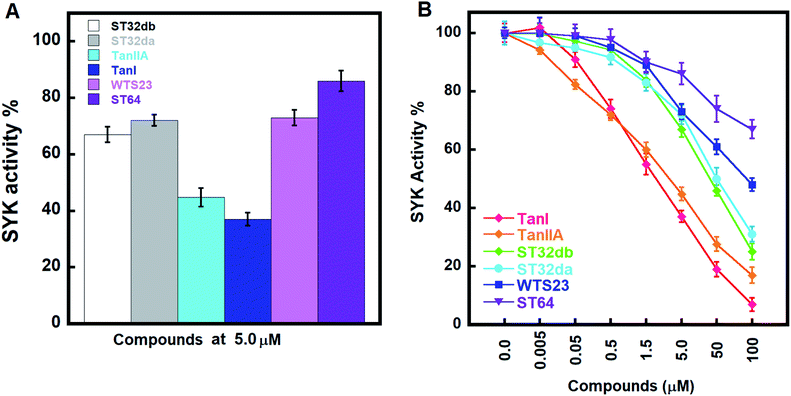 |
| Fig. 5 The inhibitory activities of the identified inhibitors against SYK. (A) The inhibitory potencies of the screened compounds at 5 μM. (B) The dose-dependent inhibitions of TanI, TanIIA, ST32da, ST32db, ST64, and WST23 against SYK. | |
Analyses of molecular interactions by Ligplot
To better understand the structure–activity relationships of the identified inhibitors towards SYK, we performed Ligplot analyses to disclose their detailed molecular interactions. The results showed that TanI and TanIIA interacted to SYK with more hydrogen bonds and additional hydrophobic interactions, compared to those of ST32db, ST32da, WTS23 and ST64 (Fig. 6). Meanwhile, these protein–ligand (SYK-inhibitors) interactions were also confirmed by analyses of intermolecular interactions module of Discovery Studio 3.5, and the results were consistent with that of Ligplot analyses.
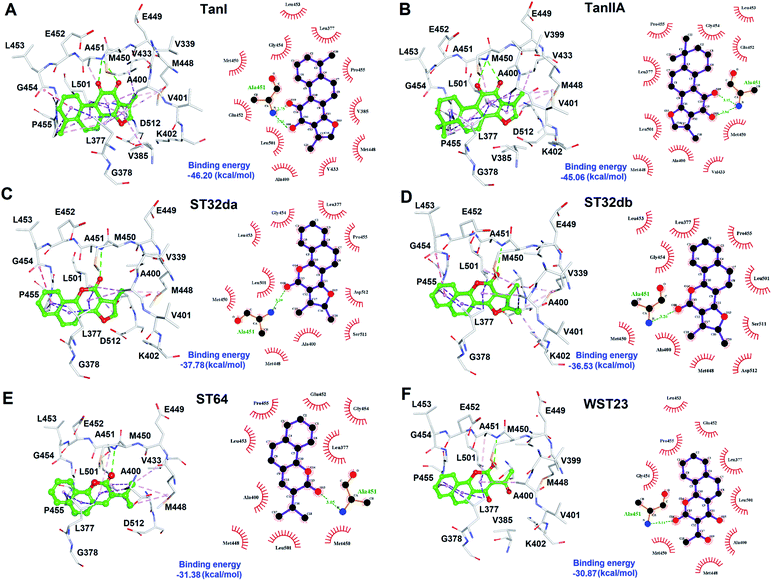 |
| Fig. 6 The molecular interactions of SYK-inhibitors. (A)–(F) The results of Ligplot analyses revealed the interactive networks of SYK-TanI, SYK-TanIIA, SYK-ST32da, SYK-ST32db, SYK-ST64, and SYK-WST23 complex structures, respectively. In panels (A)–(F), inhibitors were shown in ball-and-sticks (green); the active site residues were presented as white sticks. The dash lines indicate hydrogen-bond (green) and hydrophobic interactions (light-purple). | |
Discussion
The cytoplasmic non-receptor protein-tyrosine kinase, SYK (72 kDa) is essential in signal transduction of distinct cell types.29 SYK was firstly discovered in 1990s, and several evidence suggest that SYK was highly associated with various autoimmune disease and allergic disorders.30 SYK is widely express in macrophages, dendritic cells, mast cells, neutrophils, B and T lymphocytes.31–33 The activations of SYK in immune cells prompts B-cell are involved inflammation, proliferation, cytokine release, and cytoskeletal rearrangements.6,34 Importantly, inhibition of SYK causes apoptosis in several types of cancers, such as lung cancer, small cell lung cancer, pancreatic cancer, breast cancer, and B-Bell lymphocytic leukemia, indicating the potential of SYK as an anticancer target.35–41 Fostamatinib,42,43 BAY61-3606,44 cerdulatinib,45 MK-8457,46 and Entospletinib28 are SYK inhibitors and have been assessed in clinical trials to treat cancer and autoimmune disease. Nevertheless, unacceptable physicochemical properties and poor oral bioavailability were observed in these developed SYK inhibitors.47 Thus, developing new/novel SYK inhibitors are urgently needed for the treatments of anti-cancer and autoimmune diseases.
Wang et al., conducted a virtual screening (molecular docking) approach to identify a natural product, tanshinone I (TanI) inhibiting the activity of SYK in vitro.26 In their study, a SYK-TanI docking model was conducted to reveal the molecular mechanism of TanI binding to SYK. They found that TanI may interact with residues P455, Q462, L377, and K458 of SYK to attenuate its activity. However, these residues are actually far from the active site; the binding mode that they report may not be correct. Here, we built a SYK-TanI complex model by the same method that Wang et al., reported. The result showed that indeed TanI bound outside the active site, compared to that of inhibitor, CG9 (Fig. 1A and B). To precisely identify the possible binding site of TanI, we employed receptor–ligand pharmacophore generation to build a bioactive pharmacophore model on the basis of SYK-CG9 complex structure. Our pharmacophore modeling reveals that the inhibitor, CG9 binds deep inside the active site of SYK exerting strong inhibition; hence is of great potential containing the key functional groups (features) interacting with SYK. The built pharmacophore model, phar-CG9, composed of 1 hydrogen-bond acceptors, 2 hydrogen-bond donors, and 3 hydrophobic features (Fig. 2A), was then probed by TanI. Consequently, one hydrogen-bond donor, one hydrogen-bond receptor, and two hydrophobic features fitted well with TanI were gathered and names as pharmacophore, phar-TanI (Fig. 4B). This demonstrates that TanI is most likely bind deep into the active site of SYK through contacting with residues, L377, V385, A400, V433, M448, M450, E452, L453, G454, P455, and L501 (Fig. 6A).
To further test the precision of phar-TanI in identifying SYK inhibitors, we performed ligand-pharmacophore mapping to screen potential candidates from natural products database. About 5 hits were selected fitting well with phar-TanI, and showed conspicuous inhibitory efficiency against SYK. The compounds TanI (IC50 = 1.72 ± 0.6 μM) and TanIIA (IC50 = 3.2 ± 0.8 μM) displayed strongest inhibitory ability; ST32da (IC50 = 46 ± 1.2 μM) and ST32db (IC50 = 51 ± 3.4 μM) showed moderate inhibiting potency, while ST64 (IC50 > 100 μM) and WTS23 (IC50 > μM) are less potent in SYK inhibition (Fig. 5B). These results corroborate with the fitness (Fig. 4) of compounds towards pharmacophore, phar-TanI, demonstrating the reliability and precise of phar-TanI in screening the inhibitors against SYK. These observations also support the accuracy of our identified TanI binding site on SYK. Moreover, the SYK-inhibitor complexes obtained from ligand-pharmacophore mapping were subjected to MD simulations for 10 ns. The resultant total and potential energies as a function of time were shown in Fig. S1–S6.† The SYK-inhibitor complexes with lowest total and/or potential energies were further analyzed by Ligplot to investigate the molecular interactions. The results illustrated that TanI and TanIIA both bind into the active site of SYK in the same manner – 2 hydrogen bonds interacting with residue A451 and 10 hydrophobic interactions contacting with residues L377, A400, V433, M448, M450, E452, L453, G454, P455, and L501 (Fig. 6A and B). Similar binding mode was observed between the interactions of ST32da and ST32db with SYK, while only one hydrogen bond was formed to interact with A451 and 10 hydrophobic interactions with residues L377, A400, M448, M450, L453, G454, P455, L501, S511, and D512 (Fig. 6C and D). In contrast, ST64 and WTS23 bind to SYK by forming only one hydrogen bond to A451 and 9 hydrophobic interactions to residues L377, A400, M448, M450, E452, L453, G454, P455, and L501 (Fig. 6E and F). The chemical structures of TanI, TanIIA, ST32da, and ST32db are all composed of four rings – tetrahydronaphthalene rings A and B; a para- or ortho-quinone or lactone ring C; a dihydro- or furan ring D (Fig. 4). However, ST64 and WST23 lack of ring D, leading to less hydrophobic interactions with SYK, compared to those of TanI, TanIIA, ST32da, and ST32db. Structurally, TanI and TanIIA both are characterized by an ortho-quinone ring C which forms 2 hydrogen bonds with residue A451 strengthening the binding with SYK. While ST32da, ST32db, ST64 and WTS23 contain a lactone rather than the ortho-quinone ring C, resulting in only one hydrogen bond interacting with A451. These together with the reduced hydrophobic interactions cause the decreases of their binding affinities and inhibitory abilities to SYK (Fig. 5 and 6).
Salvia miltiorrhiza Bunge (Danshen), a famous traditional chinese herb, has been used clinically for the treatment of various diseases for centuries.48 The traditional functions of Danshen are to nourish the blood, tranquilize the mind, promote blood circulation, and end blood stagnation.48 Danshen shows several pharmacological activities including anti-fibrotic, anti-oxidative, anti-cancer, anti-inflammation and neuroprotection.49–52 TanI and TanIIA are two of the lipophilic ingredients in Salvia miltiorrhiza Bunge (Danshen).53 The tanshinoneIIA (TanIIA) is approved for the treatments of angina pectoris, myocardial infraction, and coronary heart disease by SFDA. Here, we investigated and demonstrated the compatible inhibitory abilities of TanI and TanIIA against SYK, revealing the potential of Danshen in the treatments of SYK associated diseases. Thus, TanI and TanIIA could serve as a lead compound for drug development. Interestingly, ST32da, a synthetic compound related to neo-tanshinlactone, was reported to be an ATF3 inducer.54 ST32da promotes the expression of ATF3, resulting in downregulations of adipokine genes and inducing adipocyte browning.54 Also, ST32da was observed to increase the browning of white adipose and decreased the lipogenesis of HFD-induced obese mice.54 Although ST32da has been reported as a promising therapeutic drug to treat the metabolic dysfunction and diet-induced obesity, the specific target (protein) interacting with ST32da is still unclear. Here, we demonstrated that ST32da can interact with SYK and reduced its activity (IC50 = 46 ± 1.2 μM). Importantly, SYK was found to be upregulated by β-adrenergic stimulation during brown adipocyte differentiation.55 Moreover, inhibition of SYK interfered brown and white pre-adipocyte differentiation and proliferation.55 Accordingly, all the above information imply that ST32da could serve as an inducer of ATF3 by targeting SYK, while further in-depth investigations are still needed. Nowadays, SYK was also reported to be associated with diabetes and Alzheimer's disease and could be the potential new target for treatments. Thus, traditional chinese herb, Danshen and its ingredients (tanshinone and analogues) are of great potential for drug developments to treat these SYK-associated diseases.
Conclusions
Conclusively, we performed pharmacophore-based approach coupled with biochemical assay to screen and characterize inhibitors targeting SYK. The built pharmacophore model, phar-TanI, comprehensively screened 105
911 natural products and identified compounds, TanI, TanIIA, ST32da, and ST32db which apparently disrupted the activities of SYK. We demonstrated that TanI and TanIIA interfered SYK activity through binding deeply inside the active site. The modeled complex structures revealed TanI and TanIIA mainly interact with residues L377, A400, V433, M448, M450, A451, E452, L453, G454, P455, and L501, which are key residues for structure-based lead optimization against SYK protein. Besides, our structure–activity relationships (SAR) study of the screened SYK inhibitors demonstrating the precise and reliability of pharmacophore, phar-TanI in identifying the inhibitors against SYK activity, supporting the accuracy of TanI binding site on SYK protein which we explored.
Conflicts of interest
There are no conflicts to declare.
Acknowledgements
We thank to National Center for High-performance Computing (NCHC) for providing computational and storage resources. This study was supported by the Ministry of Science and Technology, Taiwan, ROC (MOST 108-2320-B-005-007 and 109-2320-B-005-006-MY2) and Tung's Taichung Metroharbor Hospital (TTMHH-NCHULS109001).
Notes and references
- K. Sada, T. Takano, S. Yanagi and H. Yamamura, J. Biochem., 2001, 130, 177–186 CrossRef CAS.
- T. Kurosaki, A. Maeda, M. Ishiai, A. Hashimoto, K. Inabe and M. Takata, Immunol. Rev., 2000, 176, 19–29 CrossRef CAS.
- J. B. Bolen and J. S. Brugge, Annu. Rev. Immunol., 1997, 15, 371–404 CrossRef CAS.
- M. Turner, P. J. Mee, P. S. Costello, O. Williams, A. A. Price, L. P. Duddy, M. T. Furlong, R. L. Geahlen and V. L. Tybulewicz, Nature, 1995, 378, 298–302 CrossRef CAS.
- A. M. Cheng, B. Rowley, W. Pao, A. Hayday, J. B. Bolen and T. Pawson, Nature, 1995, 378, 303–306 CrossRef CAS.
- A. Mocsai, J. Ruland and V. L. Tybulewicz, Nat. Rev. Immunol., 2010, 10, 387–402 CrossRef CAS.
- S. L. Tan, C. Liao, M. C. Lucas, C. Stevenson and J. A. DeMartino, Pharmacol. Ther., 2013, 138, 294–309 CrossRef CAS.
- L. Alinari, B. Christian and R. A. Baiocchi, Oncotarget, 2012, 3, 203–211 CrossRef.
- T. Kurosaki, Curr. Opin. Immunol., 1997, 9, 309–318 CrossRef CAS.
- T. Kurosaki, Int. J. Mol. Med., 1998, 1, 515–527 CAS.
- T. Kurosaki, S. A. Johnson, L. Pao, K. Sada, H. Yamamura and J. C. Cambier, J. Exp. Med., 1995, 182, 1815–1823 CrossRef CAS.
- F. R. Toapanta, P. J. Bernal and M. B. Sztein, Front. Cell. Infect. Microbiol., 2012, 2, 128 Search PubMed.
- Y. Baba, S. Hashimoto, M. Matsushita, D. Watanabe, T. Kishimoto, T. Kurosaki and S. Tsukada, Proc. Natl. Acad. Sci. U. S. A., 2001, 98, 2582–2586 CrossRef CAS.
- K. S. Currie, J. E. Kropf, T. Lee, P. Blomgren, J. Xu, Z. Zhao, S. Gallion, J. A. Whitney, D. Maclin, E. B. Lansdon, P. Maciejewski, A. M. Rossi, H. Rong, J. Macaluso, J. Barbosa, J. A. Di Paolo and S. A. Mitchell, J. Med. Chem., 2014, 57, 3856–3873 CrossRef CAS.
- M. C. Lucas, N. Bhagirath, E. Chiao, D. M. Goldstein, J. C. Hermann, P. Y. Hsu, S. Kirchner, J. J. Kennedy-Smith, A. Kuglstatter, C. Lukacs, J. Menke, L. Niu, F. Padilla, Y. Peng, L. Polonchuk, A. Railkar, M. Slade, M. Soth, D. Xu, P. Yadava, C. Yee, M. Zhou and C. Liao, J. Med. Chem., 2014, 57, 2683–2691 CrossRef CAS.
- O. N. Pamuk and G. C. Tsokos, Arthritis Res. Ther., 2010, 12, 222 CrossRef CAS.
- C. L. Cywin, B. P. Zhao, D. W. McNeil, M. Hrapchak, A. S. Prokopowicz, D. R. Goldberg, T. M. Morwick, A. Gao, S. Jakes, M. Kashem, R. L. Magolda, R. M. Soll, M. R. Player, M. A. Bobko, J. Rinker, R. L. DesJarlais and M. P. Winters, Bioorg. Med. Chem. Lett., 2003, 13, 1415–1418 CrossRef CAS.
- F. Padilla, N. Bhagirath, S. Chen, E. Chiao, D. M. Goldstein, J. C. Hermann, J. Hsu, J. J. Kennedy-Smith, A. Kuglstatter, C. Liao, W. Liu, L. E. Lowrie Jr, K. C. Luk, S. M. Lynch, J. Menke, L. Niu, T. D. Owens, O-Yang C, A. Railkar, R. C. Schoenfeld, M. Slade, S. Steiner, Y. C. Tan, A. G. Villasenor, C. Wang, J. Wanner, W. Xie, D. Xu, X. Zhang, M. Zhou and M. C. Lucas, J. Med. Chem., 2013, 56, 1677–1692 CrossRef CAS.
- J. Liddle, F. L. Atkinson, M. D. Barker, P. S. Carter, N. R. Curtis, R. P. Davis, C. Douault, M. C. Dickson, D. Elwes, N. S. Garton, M. Gray, T. G. Hayhow, C. I. Hobbs, E. Jones, S. Leach, K. Leavens, H. D. Lewis, S. McCleary, M. Neu, V. K. Patel, A. G. Preston, C. Ramirez-Molina, T. J. Shipley, P. A. Skone, N. Smithers, D. O. Somers, A. L. Walker, R. J. Watson and G. G. Weingarten, Bioorg. Med. Chem. Lett., 2011, 21, 6188–6194 CAS.
- G. Coffey, A. Rani, A. Betz, Y. Pak, H. Haberstock-Debic, A. Pandey, S. Hollenbach, D. D. Gretler, T. Mant, S. Jurcevic and U. Sinha, J. Clin. Pharmacol., 2017, 57, 194–210 CrossRef CAS.
- B. Lam, Y. Arikawa, J. Cramlett, Q. Dong, R. de Jong, V. Feher, C. E. Grimshaw, P. J. Farrell, I. D. Hoffman, A. Jennings, B. Jones, J. Matuszkiewicz, J. Miura, H. Miyake, S. R. Natala, L. Shi, M. Takahashi, E. Taylor, C. Wyrick, J. Yano, J. Zalevsky and Z. Nie, Bioorg. Med. Chem. Lett., 2016, 26, 5947–5950 CrossRef CAS.
- A. Markham, Drugs, 2018, 78, 959–963 CrossRef CAS.
- K. Kim, Y. K. Chang, Y. Bian, O. N. Bae, K. M. Lim and J. H. Chung, J. Thromb. Haemost., 2018, 118, 1765–1775 CrossRef.
- W. Zhuang, T. Li, C. Wang, X. Shi, Y. Li, S. Zhang, Z. Zhao, H. Dong and Y. Qiao, Free Radic. Biol. Med., 2018, 121, 127–135 CrossRef CAS.
- S. Wang, Z. Wang, Q. Fan, J. Guo, G. Galli, G. Du, X. Wang and W. Xiao, Br. J. Pharmacol., 2016, 173, 2402–2418 CrossRef CAS.
- X. Wang, J. Guo, Z. Ning and X. Wu, Molecules, 2018, 23, 323–342 CrossRef.
- J. M. Burke, A. Shustov, J. Essell, D. Patel-Donnelly, J. Yang, R. Chen, W. Ye, W. Shi, S. Assouline and J. Sharman, Clin. Lymphoma Myeloma Leuk., 2018, 18, e327–e331 CrossRef.
- J. Sharman, M. Hawkins, K. Kolibaba, M. Boxer, L. Klein, M. Wu, J. Hu, S. Abella and C. Yasenchak, Blood, 2015, 125, 2336–2343 CrossRef CAS.
- M. D. Barker, J. Liddle, F. L. Atkinson, D. M. Wilson, M. C. Dickson, C. Ramirez-Molina, H. Lewis, R. P. Davis, D. O. Somers, M. Neu, E. Jones and R. Watson, Bioorg. Med. Chem. Lett., 2018, 28, 3458–3462 CrossRef CAS.
- T. K. Ma, S. P. McAdoo and F. W. Tam, Nephron, 2016, 133, 261–269 CrossRef CAS.
- C. A. Lowell, Cold Spring Harb. Perspect. Biol., 2011, 3, a002352 Search PubMed.
- G. Thoma, J. Blanz, P. Buhlmayer, P. Druckes, M. Kittelmann, A. B. Smith, M. van Eis, E. Vangrevelinghe, H. G. Zerwes, J. J. Che, X. He, Y. Jin, C. C. Lee, P. Y. Michellys, T. Uno and H. Liu, Bioorg. Med. Chem. Lett., 2014, 24, 2278–2282 CrossRef CAS.
- R. Singh, E. S. Masuda and D. G. Payan, J. Med. Chem., 2012, 55, 3614–3643 CrossRef CAS.
- Y. S. Yi, Y. J. Son, C. Ryou, G. H. Sung, J. H. Kim and J. Y. Cho, Mediators Inflamm., 2014, 2014, 270302 CrossRef.
- M. Buchner, S. Fuchs, G. Prinz, D. Pfeifer, K. Bartholome, M. Burger, N. Chevalier, L. Vallat, J. Timmer, J. G. Gribben, H. Jumaa, H. Veelken, C. Dierks and K. Zirlik, Cancer Res., 2009, 69, 5424–5432 CrossRef CAS.
- S. Cheng, G. Coffey, X. H. Zhang, R. Shaknovich, Z. Song, P. Lu, A. Pandey, A. M. Melnick, U. Sinha and Y. L. Wang, Blood, 2011, 118, 6342–6352 CrossRef CAS.
- R. L. Geahlen, Trends Pharmacol. Sci., 2014, 35, 414–422 CrossRef CAS.
- V. P. Ghotra, S. He, G. van der Horst, S. Nijhoff, H. de Bont, A. Lekkerkerker, R. Janssen, G. Jenster, G. J. van Leenders, A. M. Hoogland, E. I. Verhoef, Z. Baranski, J. Xiong, B. van de Water, G. van der Pluijm, B. E. Snaar-Jagalska and E. H. Danen, Cancer Res., 2015, 75, 230–240 CrossRef CAS.
- S. J. Lee, J. S. Choi, B. G. Han, H. S. Kim, H. J. Song, J. Lee, S. Nam, S. H. Goh, J. H. Kim, J. S. Koh and B. I. Lee, FEBS J., 2016, 283, 3613–3625 CrossRef CAS.
- P. Prinos, D. Garneau, J. F. Lucier, D. Gendron, S. Couture, M. Boivin, J. P. Brosseau, E. Lapointe, P. Thibault, M. Durand, K. Tremblay, J. Gervais-Bird, H. Nwilati, R. Klinck, B. Chabot, J. P. Perreault, R. J. Wellinger and S. A. Elela, Nat. Struct. Mol. Biol., 2011, 18, 673–679 CrossRef CAS.
- A. Rinaldi, I. Kwee, M. Taborelli, C. Largo, S. Uccella, V. Martin, G. Poretti, G. Gaidano, G. Calabrese, G. Martinelli, L. Baldini, G. Pruneri, C. Capella, E. Zucca, F. E. Cotter, J. C. Cigudosa, C. V. Catapano, M. G. Tibiletti and F. Bertoni, Br. J. Haematol., 2006, 132, 303–316 CrossRef CAS.
- J. Bussel, D. M. Arnold, E. Grossbard, J. Mayer, J. Trelinski, W. Homenda, A. Hellmann, J. Windyga, L. Sivcheva, A. A. Khalafallah, F. Zaja, N. Cooper, V. Markovtsov, H. Zayed and A. M. Duliege, Am. J. Hematol., 2018, 93, 921–930 CrossRef CAS.
- N. T. Connell and N. Berliner, Blood, 2019, 133, 2027–2030 CrossRef CAS.
- N. Yamamoto, K. Takeshita, M. Shichijo, T. Kokubo, M. Sato, K. Nakashima, M. Ishimori, H. Nagai, Y. F. Li, T. Yura and K. B. Bacon, J. Pharmacol. Exp. Ther., 2003, 306, 1174–1181 CrossRef CAS.
- G. Coffey, A. Betz, F. DeGuzman, Y. Pak, M. Inagaki, D. C. Baker, S. J. Hollenbach, A. Pandey and U. Sinha, J. Pharmacol. Exp. Ther., 2014, 351, 538–548 CrossRef.
- J. Wu, Z. Zhu, Q. Yu and C. Ding, Expert Opin. Investig. Drugs, 2019, 28, 1113–1123 CrossRef CAS.
- Y. Huang, Y. Zhang, K. Fan, G. Dong, B. Li, W. Zhang, J. Li and C. Sheng, Bioorg. Med. Chem. Lett., 2017, 27, 1776–1779 CrossRef CAS.
- X. Chen, J. Yu, B. Zhong, J. Lu, J. J. Lu, S. Li and Y. Lu, Pharmacol. Res., 2019, 145, 104254 CrossRef CAS.
- X. Chen, J. Guo, J. Bao, J. Lu and Y. Wang, Med. Res. Rev., 2014, 34, 768–794 CrossRef CAS.
- C. Y. Su, Q. L. Ming, K. Rahman, T. Han and L. P. Qin, Chin. J. Nat. Med., 2015, 13, 163–182 CAS.
- Y. Guo, Y. Li, L. Xue, R. P. Severino, S. Gao, J. Niu, L. P. Qin, D. Zhang and D. Bromme, J. Ethnopharmacol., 2014, 155, 1401–1416 CrossRef.
- X. Z. Zhang, S. S. Qian, Y. J. Zhang and R. Q. Wang, Pharm. Biol., 2016, 54, 18–24 CrossRef CAS.
- Y. Dong, S. L. Morris-Natschke and K. H. Lee, Nat. Prod. Rep., 2011, 28, 529–542 RSC.
- C. F. Cheng, H. C. Ku, J. J. Cheng, S. W. Chao, H. F. Li, P. F. Lai, C. C. Chang, M. J. Don, H. H. Chen and H. Lin, Commun. Biol., 2019, 2, 389 CrossRef.
- M. Knoll, S. Winther, A. Natarajan, H. Yang, M. Jiang, P. Thiru, A. Shahsafaei, T. E. Chavarria, D. W. Lamming, L. Sun, J. B. Hansen and H. F. Lodish, Nat. Commun., 2017, 8, 2115 CrossRef.
Footnotes |
† Electronic supplementary information (ESI) available. See DOI: 10.1039/d0ra08769f |
‡ These authors contributed equally to this work. |
|
This journal is © The Royal Society of Chemistry 2021 |
Click here to see how this site uses Cookies. View our privacy policy here.