DOI:
10.1039/D1RA04298J
(Paper)
RSC Adv., 2021,
11, 34503-34515
A label-free graphene-based impedimetric biosensor for real-time tracing of the cytokine storm in blood serum; suitable for screening COVID-19 patients†
Received
3rd June 2021
, Accepted 22nd August 2021
First published on 25th October 2021
Abstract
Concurrent with the pandemic announcement of SARS-CoV-2 infection by the WHO, a variety of reports were published confirming the cytokine storm as the most mortal effect of the virus on the infected patients. Hence, cytokine storm as an evidenced consequence in most of the COVID-19 patients could offer a promising opportunity to use blood as a disease progression marker. Here, we have developed a rapid electrochemical impedance spectroscopy (EIS) sensor for quantifying the overall immune activity of the patients. Since during the cytokine storm many types of cytokines are elevated in the blood, there is no need for specific detection of a single type of cytokine and the collective behavior is just measured without any electrode functionalization. The sensor includes a monolayer graphene on a copper substrate as the working electrode (WE) which is able to distinguish between the early and severe stage of the infected patients. The charge transfer resistance (RCT) in the moderate and severe cases varies about 65% and 138% compared to the normal groups, respectively and a specificity of 77% and sensitivity of 100% based on ELISA results were achieved. The outcomes demonstrate a significant correlation between the total mass of the three main hypercytokinemia associated cytokines including IL-6, TNF-α and IFN-γ in patients and the RCT values. As an extra application, the biosensor's capability for diagnosis of COVID-19 patients was tested and a sensitivity of 92% and specificity of 50% were obtained compared to the RT-PCR results.
1 Introduction
COVID-19 as the new member of fatal coronaviruses was originally discovered in China in December 2019.1 This new viral disease severely infects the lower respiratory tract and leads to the common symptoms of dry cough, fever and shortness of breath in most patients.2,3 Due to the high contagious nature of this virus even person-to-person and airborne transmission4 and the daily upsurge of the newly infected patients globally,5 the pandemic status was announced by the WHO.6
COVID-19 virus still is very weird and unknown for the scientists and many scenarios based on the case reports are raised to describe and predict its behavior.7,8 After the entry of this virus to the respiratory tract, three phases are presumed: viral replication, immune hyperactivity or cytokine storm and finally the pulmonary dysfunction.9,10 In fact, the immune system itself attacks the infected patient. When the white blood cells are faced with the virus or virus-infected cells, they become hyperactivated and a storm of the cytokines to call other immune cells are unleashed and secreted into the blood medium.11 Three cytokines named interleukin-6 (IL-6), Tumor Necrosis Factor-α (TNF-α) and interferon-γ (IFN-γ) play a more important role in cytokine storm relative to the others.12 Furthermore, the reports demonstrate that cytokine storm is the most alarming reason behind the mortality of the COVID-19 patients13 by inducing an acute respiratory distress syndrome (ARDS). On this basis, a notable fraction of clinical trials are run to suppress the hyperactivation of the immune system in response to this infection after being diagnosed.14 Hence, monitoring the acute elevation of the cytokines could be a reliable warning approach to check the severity of the disease in the infected patients.
Many methods were developed for rapid and precise tracing of this storm in blood but time-consuming and expensive protocols are the limitations of these methods and so, the conventional enzyme-linked immunosorbent assay (ELISA) method even with its limitations such as cross-reactivity of the antibodies15 is still the most applicable technique among all approved procedures.16,17 Although cytokine storm has arisen in many other types of diseases such as sepsis, flu, GvHD, etc.,18 currently the best available cohort with meaningful level of cytokine storm for developing new technologies is COVID-19 infected patients during this pandemic.
On the other hand, biosensors have been used in medicine for decades in order to diagnose many macromolecules including hormones, proteins, cancer markers, etc.19,20 Among all types of biosensors, electrochemical-based techniques could offer very promising results and are extensively utilized for many diseases.21–23 Electrochemical impedance spectroscopy is one of such methods which measure the electrical impedance of the target species in the electrolyte medium.24 Charge transfer resistance is the comparison parameter between the studied groups and is always affected by the presence of dielectric materials in the media.25 Hence, impact of the glycoprotein cytokines on the RCT elevation due to their weight could be expected especially for the serum of COVID-19 patients with the cytokine storm indication.
Here, we have proposed a new biosensing method for non-direct detection of cytokine storm in SARS-CoV-2 patients and their screening according to the severity of the overall immune activity, based on the EIS of the total cytokine mass in unprocessed blood serum. To improve electrochemical interface between biological macromolecules (such as cytokines) and electrodes, nanomaterials would be the best choice.26 Among famous mostly used biosensing electrodes including MWCNT,27 SiNWs28 and graphene29,30 we used graphene sheets deposited on a copper substrate due to their better charge mobility31 and electrical conductivity.32 Moreover, graphene showed much better site binding with polarizable bio-agents as we investigated IL-6 cytokine using MD simulation. The device showed meaningful correlation between the immune hyperactivation in COVID-19 patients (induced by acute elevation of the total mass of cytokines in the blood serum) and the increased RCT. In addition, the EIS results obtained by our method were compared with ELISA analysis. Moreover, we used this sensor for indirect detection of the patients suffering from the COVID-19 disease and validated the results by conventional RT-PCR and CT techniques in normal and infected cases.
2 Materials and methods
2.1 Sensor fabrication and characterization
In the case of the sensor fabrication, at first a bilayer of Au/Ti (30/5 nm) is deposited on top of a glass slide as the substrate using a sputtering system (Veeco, USA). Then, the circular pattern of the biosensor was designed using CorelDRAW® Suite X6 (Corel Corporation, Canada). The biosensor is an integrated three-electrode system containing a circular working electrode with a diameter of 5 mm and a counter and reference electrode. The distance between the working and reference and counter electrode is 1 mm. After preparation of the mask, the designed pattern of the three-electrode system was transferred to the glass substrate using the standard photolithography method. At the end, the graphene/Cu wafer was cut using a laser machine with a diameter of 5 mm (equal size to working electrode diameter) and placed and adhered on top of the working electrode (Fig. S1†) and sealed. The material of the reference and counter electrodes is gold and the working electrode is a graphene monolayer on top of the copper substrate (Graphenea Inc., Spain).
To investigate and determine the structure and composition of the working electrode, some characterization methods were employed. Fig. S2-A† displays the scanning electron microscope (Hitachi-S4160, Japan) images of graphene on Cu. The white and gray regions represent the Cu grains and dark areas reveal the existence of graphene on Cu. As can be seen in Fig. S2-B,† the energy dispersive X-ray (EDX) analysis verified that there are only two elements in the sample: Cu and small amount of C, estimated to be near 2.6 wt%.33 Fig. S2-C† demonstrates the Raman spectrum of graphene/Cu. The Raman spectrum (Teksan-Apus, Iran) shows two considerable peaks at 1562 cm−1 and 2712 cm−1 related to the G-band (in-plane vibration mode) and 2D-band (double resonance scattering of inter-valley) of the graphene layer, respectively. These peaks confirm the presence of the graphene layer on Cu. As observed in the Raman spectrum, there is no significant D-band peak in the diagram, which illustrates approximately no defects on graphene.34 The formation of graphene/Cu was investigated by the X-ray diffraction (XRD) technique (Fig. S2D†). The sharp diffraction peak at 59.2° is related to Cu with the plane of (200). Also, the tiny peak at 42.4° corresponds to the graphene layer.
2.2 Experimental setup
At first the patients suspected to be infected by SARS-CoV-2 virus were selected based on the severity of the disease. The presence and severity of the disease were reported based on the symptoms and clinical exams of the patients presented in latest guidelines for clinical management of COVID-19 patients published by the WHO.35 The serum samples were prepared from the residues of patients' and candidates' blood who did serological tests to check themselves. People were informed about this research and all agreed to donate their blood residue instead of wasting it out. When the blood is sampled and coagulated, the serum is isolated from the whole blood by centrifugation (10 min at 3000 rpm). For EIS analysis, the serum is mixed in a ratio of 3
:
1 with electrolyte solution (0.1 M KCl, 5 mM K3[Fe(CN)6] and 5 mM K4[Fe(CN)6]) and then is added (200 μL) to the three-electrode sensor. EIS was performed using a potentiostat (Ivium, Netherlands) for a potential amplitude of 10 mV and in the frequency range of 10 mHz to 100 kHz. Charge transfer resistance was calculated based on the Nyquist diagram for each sample.
2.3 Molecular dynamics simulation
In this subsection, more details about the system description and equilibrium simulations are presented. The crystal structure of human IL-6 which has been reported by solution NMR spectroscopy (PDB code 1IL6)36 was selected as the initial structure of the protein. The structure of IL-6 preserves many of the structural specifications assigned to this category of proteins that differentiate them from the other similar but short-chain cytokines (e.g. IL-3, IL-4, IL-5, and M-CSF).37 IL-6 monomer is composed of five alpha-barrel structures (H1 to H5, Fig. S3-A†), scattered among loops with variable lengths, including two malformed helices (H1A and H1B, Fig. S3-B†) encompassing the interior pore of the protein. The first helix (residues T21 to K47) exhibits a remarkable kink located in the surrounding domain of residue 42. The kink exists due to the fact that residues A39 and L40 possess some missing hydrogen bonds. The C-terminal segment of connecting loops for helices 1 and 2 consists of two successive turn structures (residues A69 to C74 and residues Q76 to F79). According to Fig. S3-C and -D,† the connecting loop of helices 3 and 4 is a three-residue turn at its N-terminus (A131 to L134) extending into a distinct helix (helix 4, residues T143 to A154).
In order to investigate the interaction of protein with surfaces, MD simulations are carried out for the protein solvated in a box of water on graphene and gold substrates separately. The solvent atoms within 1.5 Å of the solute were taken out from the system. Transferable intermolecular potential with 3 points (TIP3P) was used as the model of water for all simulations.38,39 The solvated structure of the protein was placed on two substrates separately. In the first case we put it on a single-layer of graphene sheet with armchair configuration and in the second case it was placed on a single layer of gold (100).
In both systems, the approximate dimension of the substrate was set to (88 × 67) Å2, which is large enough to neglect the size effects.40 Ultimately, the final configuration of the system involved ∼24
400 atoms, including 6992 water molecules with an approximate density of 0.998 g cm−3. The protein was placed on the center of each substrate at an initial normal distance of about 10 Å from the surface. Atoms of the surfaces were supposed to be fixed during the simulation, and the temperature was applied to the box of water and protein with an NVT ensemble. The final substrate-protein structure along with the solvent was considered as the input of the simulation. A Langevin thermostat with a damping coefficient of 5 ps−1 was used to control the system temperature at a constant value of 300 K. Periodic cell dimensions for both of the systems were adjusted to (89 × 68 × 47) Å3 in x, y and z directions respectively. Full long-range electrostatic interactions are calculated by the Particle Mesh Ewald (PME) method.41 The PME grid spacing was considered to be 1 Å. Bonds to all hydrogen atoms are supposed to be rigid using the SHAKE algorithm.42
Simulations are carried out for each system individually using the molecular dynamics program NAMD43 with a nonbonded cutoff of 12 Å and 1 fs timestep. The derived structures were visualized using the VMD package.44 The MD simulation procedure was performed by minimization and equilibration of the solvated protein on the substrate for 20 ns at 300 K. Moreover, a modified CHARMM22 parameter file for proteins45,46 was utilized as the system force field to consider the intermolecular and intramolecular interactions of IL-6. In order to consider non-bonded interaction between protein-solvate and the gold surface, modifications are applied to the force field. In this case, the force field parameters for Au–amino acid interactions, along with partial charges, were extracted from the GolP-CHARMM force field.47
2.4 Blood serum isolation and assays
As reported previously,60 human blood samples from normal and suspected patients were collected and then divided into ethylenediaminetetraacetic acid (EDTA) or clot activator tubes for the CBC (Complete Blood Count) or biochemical analysis, respectively. In the case of serum isolation, the clot activator tubes were maintained at 37 °C for 15 minutes and then centrifuged for 10 min at 3000 rpm. The serum as the top part of the liquid was extracted for the EIS measurements.
2.5 Enzyme-linked immunosorbent assay
The amount of IL-6 (Cat number: D6050, R&D Systems, United States), TNF-α (Cat number: QK210, R&D Systems, United States), and INF-γ (Cat number: DIF50, R&D Systems, United States) present in the serum of patients with COVID-19 was measured with an enzyme-linked immunosorbent assay as previously described. In brief, known concentrations of recombinant human IL-6, TNF-α or INF-γ and the experimental samples were added and incubated in polystyrene microtiter plates coated with an antibody against the selected cytokine, followed by incubation with an enzyme-linked polyclonal antibody directed to the cytokine. Next, a substrate solution for the enzyme was added, and the color development was stopped by adding 2N H2SO4. The substrate solution contains two parts of color reagent A: stabilized hydrogen peroxide and color reagent B: stabilized chromogen (tetramethylbenzidine). The absorbance was measured with a microtiter plate spectrophotometer. The amount of IL-6, TNF-α, or INF-γ present in each sample was determined from a standard curve generated in each assay and expressed as picograms per milliliter. The sensitivity of the enzyme-linked immunosorbent assay for IL-6, TNF-α and INF-γ is 0.70 pg mL−1, 1.88 pg mL−1, and 8 pg mL−1, respectively. The reproducibility of all measurements was within 10% in our laboratory.
2.6 Mass spectroscopy
Matrix-assisted laser desorption/ionization mass spectrometry (Applied Biosystems 4800 MALDI TOF/TOF, Nd:YAG 200-Hz laser) was used for MS experiments. For MS analysis, after diluting the sample up to 5 times with deionized water, the sample was spotted on a MALDI plate mixed with alphacyano-4-hydroxy-cinnamic acid (CHCA) in 50% CAN containing 0.1% TFA (with 1
:
2 ratio) as a matrix solution, air dried, and analyzed in linear positive mode. The data were interpreted and processed using Data Explorer software version 4.0 (Applied Biosystems).
2.7 RT-PCR assay
Total viral RNA was extracted from the specimens using a High Pure Viral Nucleic Acid Kit (11858874001), according to the manufacturer's instructions. Isolated RNA samples were then used for RT-PCR. The extracted RNAs were stored at −80 °C. Primer and probe sets targeting different gene regions (RdRp/helicase [Hel], spike [S], and N) of SARS-CoV-2 were designed and tested. The probes were predicted to specifically detect SARS-CoV-2 and had no homologies with human, other human-pathogenic coronaviruses or microbial genes on BLAST (National Centre for Biotechnology Information) analysis that would potentially produce false-positive test results as previously described. Primers and probes with the best amplification performance were selected and in vitro RNA transcripts for making positive controls and standards were prepared. Real-time RT-PCR assays for SARS-CoV-2 RNA detection were performed using a One-Step Real-Time PCR Kit (Qiagen, Germany) in an Applied Biosystems 7500 Real Time PCR Systems thermocycler (Applied Biosystems, Foster City, CA, USA) in a final volume of 20 μL. Each 20 μL reaction mixture contained 10 μL master mix, 1 μL of primer–probe RdRp, 1 μL primer–probe endogenous control, 5 μL of RNA as the template, and 3 μL of RNase-free water. The thermal cycling conditions were 15 min at 50 °C for reverse transcription, 3 min at 95 °C for cDNA initial denaturation, and 45 cycles of 15 s at 95 °C and 40 s at 55 °C and 10 s at 25 °C.
2.8 Ethics statement
Clinical samples and blood serums were obtained from donors who signed the written consent. Sample collection was performed under the license of Ethics Committee with the license code of IR. TUMS.VCR.REC.1399.577.
2.9 Statistical analyses
All the collected data were analyzed using GraphPad Prism software version 8.3.0 (GraphPad Software, Inc. La Jolla, CA, USA) and each data point represents the mean value of three independent measurements.
3 Results and discussion
3.1 Cytokine analysis of the serum samples by ELISA
In order to run the experiment, in the first step the blood samples were collected from normal donors, moderately and severely infected patients (with signs of inflammatory diseases such as COVID-19) in three individual cohorts which had been selected based on the clinical judgments of the patients (for more details see methods, Experimental setup). Then, an adequate volume of blood was sampled from the patients and after serum isolation, the cytokine measurement was performed by the ELISA method.
Blood serum consists of many proteins including antibodies, cytokines, hormones, etc. with different molecular weights and dielectric properties.48 In chronic inflammatory diseases with pathogens such as SARS-CoV-2 virus, many inflammatory signals are produced and cytokine release into the blood serum becomes out of control. Such a phenomenon is the so-called cytokine storm which is the most prominent feature of the disease.12 Although in the hypercytokinemia many cytokines are secreted into the blood, three key cytokines including IL-6, TNF-α and IFN-γ show the most elevated levels in the blood serum of the patients.12,49,50
On this basis, the ELISA procedure was carried out to measure the number of the produced cytokines by the immune system of each case. As presented in Fig. 1A–C, the expressed levels of IL-6, TNF-α and IFN-γ cytokines in the infected patients were markedly higher than those of the normal group. Furthermore, analyzing the cytokines for the two groups of moderately and severely infected patients demonstrates a correlated secretion pattern of those three cytokines with the severity of the disease. Here, hypercytokinemia alone means the sum of the moderate and severe cases.
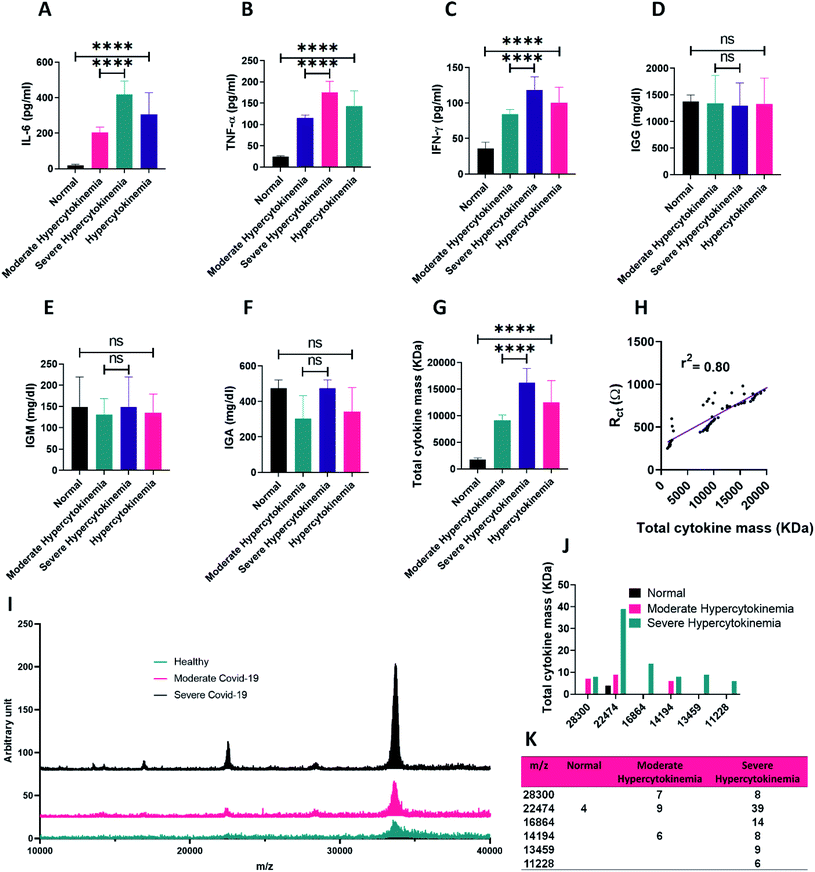 |
| Fig. 1 Comparing secreted levels of (A) IL-6, (B) TNF-α, (C) IFN-γ, (D) IGG, (E) IGM, (F) IGA and (G) total cytokine mass in the blood serum of normal donors,and patients with moderate and severe hypercytokinemia. Here, hypercytokinemia alone means the sum of the moderate ans severe cases (H) typical experimental result for the sum of the normal, moderate and severe cases, in which black dots illustrate the charge transfer resistance data, whereas the purple line denotes the corresponding data fitting results on the basis of the simple linear regression method (I) mass spectrum of the blood serum from the three groups of normal, moderate and severe cytokine strom analyzed by MALDI-TOF mass spectrometry (J) and (K) extracting the mass to charge (m/z) peaks and their intensity in the mass spectrum of the blood serums and their abundance comparison in the normal and hypercytokinemia groups. | |
Aside from the cytokine analysis, more laboratory tests including immunoglobulins G, M and A were also performed for the normal and infected groups. As demonstrated in Fig. 1D–F, no meaningful correlation in the COVID-19 infected patients compared to the normal donors could be detected in the case of the immunoglobin G (IGG), immunoglobin M (IGM) and immunoglobin A (IGA) antibodies, while a significant difference existed in the case of cytokines.
For more clarification, total molecular weight per milliliter of serum for all three types of cytokines was calculated for the normal and infected cases. As presented in Fig. 1G and S4,† the total molecular weight of the mentioned cytokines for the suspected COVID-19 patients was meaningfully increased as the stage of the disease becomes inferior. The calibration curves for the RCT vs. total cytokine mass is plotted in Fig. 1H. Based on the curves, there is a satisfactory linear relationship (r2 = 0.80) between the RCT and the total mass of the cytokines. As presented in Table 1, total cytokine mass for the patients was between 7395 and 20
770 kDa while this value for the normal group was between 1206 and 2345 kDa. Also, this value in moderate patients was about half of that in severe cases (Fig. 1G).
Table 1 EIS, CT, RT-PCR and total cytokine mass results of the normal and COVID-19 patients
Mass spectroscopy analysis of plasma blood samples revealed a total increase in intensity of mass peaks for the patients with severe symptoms and cytokine storm (Fig. 1I and J). Moreover, in some m/z such as 22
474 (Fig. 1K) which is close to the molecular weight of the three mentioned cytokines, a meaningful increase could be observed in the mass peaks from normal samples (4) to severe patients (39). This might be in good correlation with cytokine storm based tracing of people suspected to have COVID-19.
3.2 EIS analysis of the cytokine storm in the patient samples
3.2.1 Device characterization and molecular dynamics simulation. In order to test the blood serum, three prevalent types of electrode materials51–55 including gold (Au), carbon and graphene were candidates for EIS analysis. Adhesion and spread of the serum on the selected substrates were evaluated by the contact angle method. As the most part of the serum's volume is made up of the water,48 it has more tendency to the hydrophilic substrates. The maximum contact angle was attributed to the carbon surface with ∼118° and the minimum was for the graphene electrode with an angle of ∼69° (Fig. 2A). The results demonstrate that the blood serum is mostly attracted to the graphene-coated electrode rather than the Au and carbon.
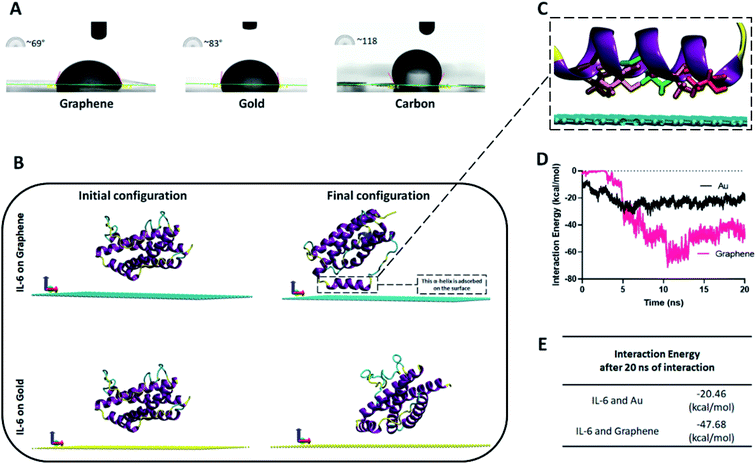 |
| Fig. 2 (A) Contact angle comparison of a normal serum over the graphene, gold and carbon substrates (B) initial and final configuration of the IL-6 on graphene and gold, after 20 ns of molecular dynamics simulation (C) protein orientation close to the graphene surface. The closest amino acids in the vicinity of graphene sheets including THR143 (red), ALA146 (orange), LEU149 (green), THR150 (pink), and GLN153 (ochre) (D) interaction energy of IL-6 on Au and graphene electrodes derived by MD simulation over the timespan of 20 ns and at the end (E). | |
In addition to the wettability experiment, molecular dynamics (MD) simulation was performed in order to choose the better material for cytokine adsorption on the electrode surface. For this purpose, the IL-6 as the most important and secreted protein in the hypercytokinemia12 was selected as the key sample in the cytokine storm to be interacted with the electrode surface. In fact, IL-6 is the representative of the interaction of other cytokines with the graphene material. As presented in Fig. 2B and Movie S1,† the IL-6 shows more tendency towards the surface of the graphene compared to the gold substrate. As can be seen in Fig. 2B, one of the 6 composing alfa helices of the IL-6 is visibly attracted toward the graphene by attractive van der Waals forces between the graphene substrate and THR143, ALA146, LEU149, THR150 and GLN153 amino acids of the IL-6 protein (Fig. 2C). For better clarification, the interaction energy between the IL-6 and substrates (gold and graphene surfaces) was plotted (Fig. 2D) for 20 nanoseconds of atomic interaction. After 5 ns of the interaction, the cytokine showed better adsorption to the graphene sheet due to its smaller amount of interaction energy (−47.68 kcal mol−1 vs. −20.46 kcal mol−1 for gold) (Fig. 2E). The attractive interaction between the IL-6 protein and the graphene substrate will be in a range that it stabilizes the position of helix 4 at a minimum distance of 3 Å of the surface. However, the minimum distance between the structural components of the protein and the gold surface will be about 6 Å.
3.2.2 Derivation of RCT in the blood serum of the infected patients. As presented in Fig. 3, after sampling blood from the donors, the blood serums were isolated and mixed with the charge carrier (KCl, K3[Fe(CN)6] and K4[Fe(CN)6]) (see methods). Charge carriers are used to better record the frequency dependent resistance of the target.25,56 Next, 200 μL of serum–electrolyte mixture was dropped on the surface of the biosensor. EIS of the solutions was scanned in the frequency range between 10 mHz and 100 kHz in a voltage amplitude of 10 mV. The Nyquist response was then plotted and RCT was derived for each of the serum samples. Finally, the RCT was compared between the cases without sign of cytokine storm and those who have hypercytokinemia based on the results of the ELISA.
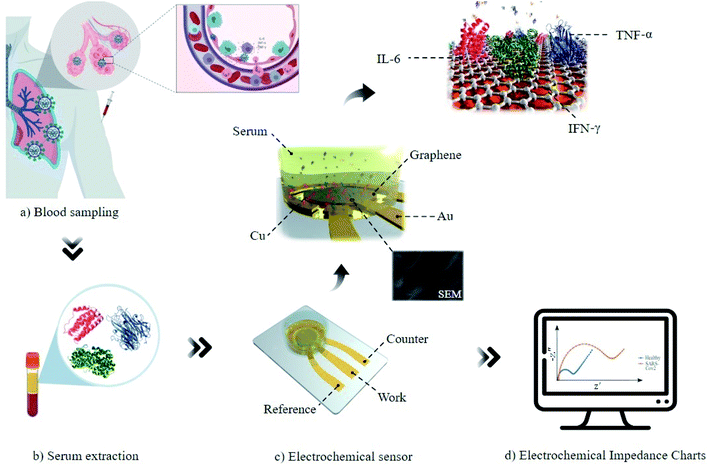 |
| Fig. 3 Schematic diagram of the procedure of cytokine storm diagnosis in patients suspected to have COVID-19 by the EIS method. Blood is sampled from the normal donors and different stages of patients and then the serum is isolated and mixed with the electrolyte. The mixed solution is then added to the three-electrode system with graphene/Cu WE and at the end EIS is performed and RCT is extracted for each sample. | |
Fig. 4A demonstrates the obtained Nyquist plot for the serums of normal people without any symptoms and patients suspected to have the COVID-19 disease. Three levels of responses with distinct curves could be observed in this figure. The smallest curves were recorded for normal donors and the largest curves were observed in the EIS responses of the patients with severe infection. As can be deduced from Fig. 4B, the semicircle diameter as the indicator of the RCT for normal groups and different stages of the patients showed meaningful ranges. RCT for all of the normal serum samples is less than 347 Ω while for the moderate cases it stands in the range of 441 Ω to 610 Ω and that of severe ones is more than 715 Ω (Fig. 4C). The results of the impedimetry analysis showed increasing correlation between RCT (dielectric properties of blood serum) and the disease from infection to progression in stage. The lowest RCT was recorded from the samples of normal people while the highest RCT was recorded from the samples of severely infected patients. An average increase of 101% was seen for the infected patients compared to the normal donors. The values of % ΔRCT/RCT for moderate and severe cases were about 65% and 138%, respectively (Fig. 4D). For an illustration of the EIS sensor detection limit, a blood serum with a specified amount of cytokine mass was serially diluted, and RCT was calculated. The detection limit for the sensor was calculated to be 175 kDa total cytokine mass, which is considerably lower than the amount in patients with cytokine storm (8000–20
000 kDa) (Fig. S5†).
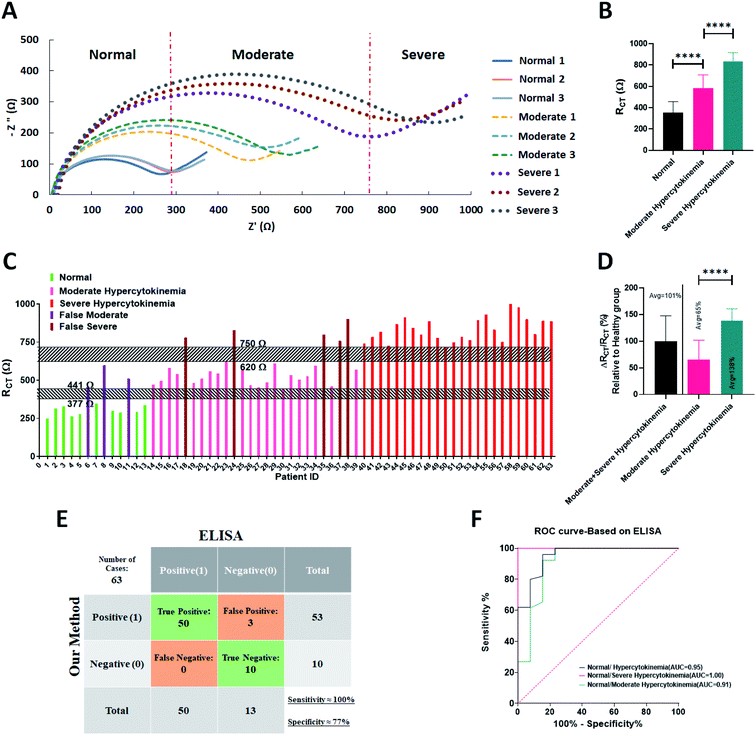 |
| Fig. 4 (A) Nyquist plot for 9 samples of blood serum for each group of normal, moderate and severely infected patients (B) comparing the average value of the RCT for the normal and SARS-CoV-2 patients (C) representation of the RCT range for the individual normal and infected patients. Ranges 377 Ω to 441 Ω and 620 Ω to 750 Ω are the free bands between normal-moderate and moderate-severe groups, respectively (D) increased percentage of RCT for COVID-19 patients in different progression stages, relative to the average value of the normal group (E) confusion matrix and (F) ROC curves for comparing our method with the ELISA technique on detection of cytokine storm, respectively. | |
During the EIS recording of a solution, site binding phenomena would happen25 after which a film of polarized dielectric materials such as cytokines would be formed in the interface between the electrode and electrolyte. This dielectric layer would increase the RCT in the EIS response. These findings prove a strong correlation between the hyper-secretion of the cytokines and elevated RCT. To prove this, we have added different amounts of IL-6 protein to the sensor and the Nyquist diagram was plotted. As can be seen from Fig. S6,† the semicircle diameter was increased in harmony with an increase in the IL-6 concentration. This observation corroborates the theory that any increase in serum cytokines or hypercytokinemia has a direct impact on the elevation of the RCT. For more clarification, the results of two consecutive EIS of a sensor from a specific serum were plotted. As can be seen in Fig. S7-A,† the semicircle diameter increases and it is due to the binding of the serum proteins to the graphene which increases the charge transfer resistance for the next experiment. On this basis, a new graphene electrode was utilized for any of the tests to exclude the effect of any other disturbing parameters. In addition, Fig. S7-B† demonstrates the EIS result of the three different sensors with one serum. The results are the same, and this means that the sensors are reliable and have the same result.
Cytokine analysis by the ELISA method represented no hypersecretion of the key cytokines in the normal group, while RCT for three of the cases (ID#6, ID#8 and ID#11) tested using the EIS sensor relied on the range of the moderate cohort. Furthermore, for five cases (ID#18, ID#24, ID#35, ID#37 and ID#38) in which the ELISA test revealed a moderate secretion of the cytokines, the RCT was obtained in the range of the severely infected patients. These inconsistencies might happen due to the non-specific binding of other agents existing in the blood serum to the graphene electrode.
To assess the correlation of the hypercytokinemia with the increased charge transfer resistance obtained by the EIS, Receiver-Operating Characteristic (ROC) analysis was performed (Fig. 4E and F). Our gold standard for positively scoring a patient with cytokine storm was ELISA tests from the three cytokines of IL-6, TNF-α and IFN-γ and evaluating the total cytokine mass. The statistical analysis shows a sensitivity of 100% and specificity of 77% (Fig. 4E) with an AUC of 0.95 (Fig. 4F) for the EIS biosensor with respect to the ELISA as the gold standard for cytokine measurements. It is worth noting that the sum of the moderate and severe cases was studied for the ROC analysis.
3.3 Evaluating the capability of the biosensor for COVID-19 detection
Since the cytokine storm is an evidenced consequence in most of the COVID-19 patients,49,57–59 the capability of the EIS biosensor to detect the patients with COVID-19 disease was tested.
Our gold standard for positively scoring a COVID-19 infected patient was either RT-PCR or CT-scan. As an example (Fig. 5A), the chest CT image of a normal lung (ID#4) shows no abnormal opacification while for a moderate case the glass-ground opacification (GGO) is seen in the both lobes of the lung (ID#29). Such a bilateral GGO pattern in the severe cases is more intensive with more involved area of both lung lobes accompanied by consolidation (ID#55).
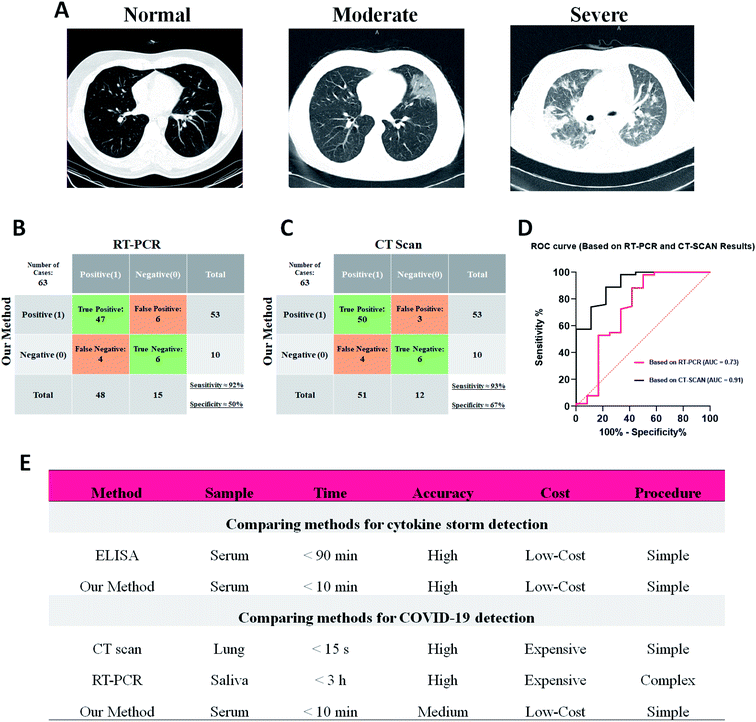 |
| Fig. 5 (A) CT images of healthy chest and patients with moderate and severe COVID-19 infection. (B) and (C) Confusion matrix and (D) ROC curves for comparing our method with the RT-PCR and CT scan techniques, respectively (E) descriptive comparison on the performance of the cytokine storm and COVID-19 detection between the proposed and other methods. | |
Table 1 compares the detailed data of the patients analyzed using the EIS sensor, RT-PCR, CT-scan and cytokine analyses. As presented, three of the patients (ID#23, ID#59 and ID#60) with clinical symptoms of infection were also analyzed in the case of cytokine storm as well as their CT/PCR results. Interestingly, their RCT value was placed in the range of the severely infected patients with CT involvement but the RT-PCR showed no signs of COVID-19 infection and diagnosis of acute flu was more probable. On the other hand, RCT for three cases (ID#6, ID#8 and ID#11) was in the range of moderately infected patients, while CT/PCR was negative and no cytokine storm was detected by the ELISA results. As mentioned in the previous section, these false signals of EIS for such cases might be due to the unknown non-specific binding of other elevated reagents in the blood serum of the studied patients. Moreover, four false negatives were recorded by EIS analysis with normal RT-PCR and chest CT results (ID#3, ID#7, ID#9 and ID#13). The blood analysis of these patients revealed non-elevated IL-6, TNF-α and IFN-γ cytokines which indicated that the immune system did not react to the virus yet and just the lungs were involved in the infection. Hence, no cytokine storm happened and neither ELISA nor EIS diagnosed the COVID-19 disease. In the case of COVID-19 detection, about 92% sensitivity with respect to both CT/PCR, 67% and 50% specificity and AUC of 0.91 and 0.73 were reached based on CT and RT-PCR methods, respectively (Fig. 5B–D).
These outcomes mean that the EIS method and our sensor showed strong responses to the patients with cytokine storm activity in their blood serum irrespective of the type of disease. So, due to the remarkable features of the EIS method it could compete with the current ELISA method on detection of the cytokine storm in the serum samples (Fig. 5E). In addition, since most of the patients with COVID-19 disease suffer from the cytokine storm, the results of the EIS method on diagnosis of the infected patients by SARS-CoV-2 virus seem acceptable and could be compared with the current methods of diagnosis such as RT-PCR and CT scan (Fig. 5E).
3.4 Follow-up of the patients with cytokine storm by the EIS method
In order to examine the sensitivity of the biosensor to the cytokine production, five patients with SARS-CoV-2 infection suffering from the hypercytokinemia were monitored for two weeks. All patients were hospitalized under standard treatment protocols and recovered with improved symptoms.
The EIS measurements were applied for the blood serum of the candidates after two weeks and as depicted in Fig. 6A, the RCT after treatment was reduced in all patients (Fig. 6B).
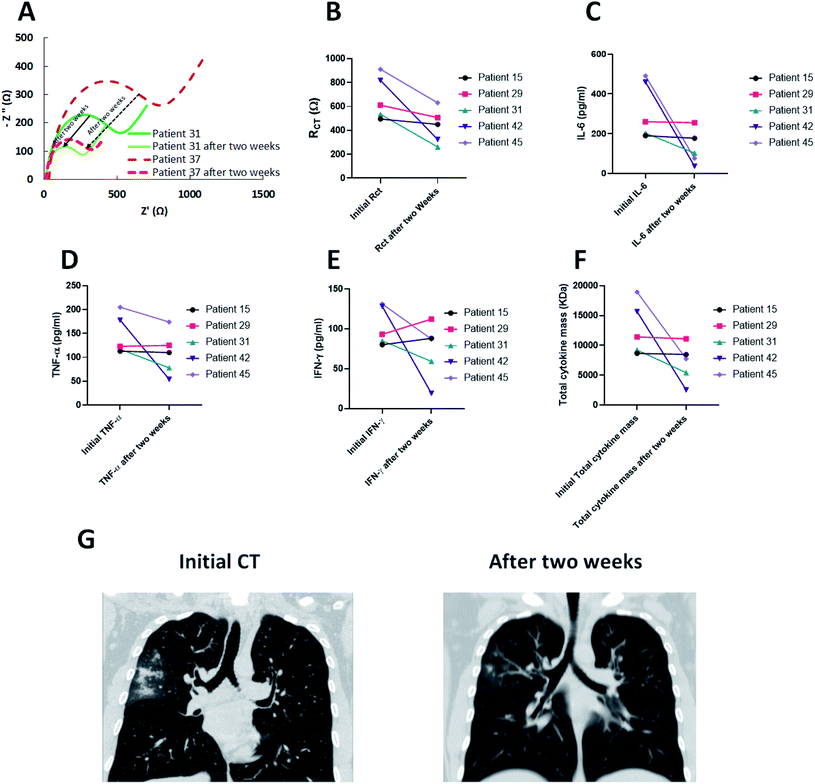 |
| Fig. 6 (A) Nyquist plot of two patients after two weeks of treatment (B) the corresponding RCT, (C) IL-6, (D) TNF-α, (E) IFN-γ and (F) total cytokine mass in kDa for the patients recovered after two weeks (G) recovered lung CT images of patient ID#31 after two weeks. | |
Moreover, ELISA results demonstrated that the cytokine secretions were in accordance with the EIS outcomes. While IFN-γ levels increased in two patients (ID#15 and ID#29), the overall levels of three cytokines (IL-6 + IFN-γ + TNF-α) exhibited a reduction for all five patients after two weeks of treatment (Fig. 6C–E). In fact, the RCT acts in harmony with the results of the total cytokine mass and whenever it is increased, the RCT also rises. The reduction in total cytokine masses showed a better insight into the correlation of EIS results and the cytokine changes of the blood serum (Fig. 6F). In addition, chest CT (Fig. 6G) was performed for the infected patients and showed a significant decrease in GGO area after two weeks of treatment compared to the initial CT (ID#31).
4 Conclusion
In summary we designed an electrochemical impedimetric biosensor based on graphene electrodes to measure the collective levels of cytokine storm in patients infected by chronic inflammatory diseases. No bioreceptor on the working electrode was utilized due to the fact that many types of cytokines are increased during the cytokine storm and measuring the collective presence of the cytokines seems more rational than specific detection of a single type. This sensor could be helpful as a complementary diagnostic tool to warn about the presence of cytokine storm in patients who are suspected to have COVID-19 during this pandemic. The sensor shows diagnostic responses from serum of the blood in less than 10 minutes. A complete correlation was observed between the total cytokine levels and the EIS responses which reveals that the biosensor is able to detect the hypercytokinemia in the COVID-19 patients. It is worth noting that the sensor works when a cytokine storm exists, no matter the cause of the hypercytokinemia is COVID-19 or other diseases such as sepsis, flu, etc. Furthermore, the graphene-based biosensor was applied for indirect detection of the COVID-19 disease based on the serum cytokine levels. A sensitivity of 92% was achieved with respect to the RT-PCR results which shows that this biosensor could also be applied for COVID-19 diagnosis. The results (Fig. 5E) prove that the proposed EIS biosensor based on the advantage of easy fabrication, fast detection and high accuracy could also be used for evaluating the new drugs and vaccines developed for the treatment of cytokine storm in COVID-19 patients.
Author contributions
Mohammad Ali Khayamian: conceptualization, data curation, formal analysis, investigation, methodology, validation, visualization, project administration, supervision, writing – original draft, and writing – review & editing. Mohammad Salemizadeh Parizi: data curation, formal Analysis, investigation, methodology, validation, and visualization. Mohammadreza Ghaderinia: data curation, formal analysis, investigation, methodology, validation, and visualization. Hamed Abadijoo: data curation, formal analysis, investigation, methodology, validation, and visualization. Shohreh Vanaei: data curation, formal analysis, investigation, methodology, validation, and visualization. Hossein Simaee: data curation, formal analysis, investigation, validation, visualization, and resources. Saeed Abdolhosseini: visualization. Shahriar Shalileh: investigation. Mahsa Faramarzpour: investigation. Vahid Fadaei Naeini: software. Parisa Hoseinpour: resources. Fatemeh Shojaeian: resources. Fereshteh Abbasvandi: resources. Mohammad Abdolahad: conceptualization, data curation, formal analysis, methodology, validation, funding acquisition, project administration, supervision, writing – original draft, and writing – review & editing. Conceptualization, data curation, formal analysis, funding acquisition, investigation, methodology, project administration, resources, software, supervision, validation, visualization, writing – original draft, writing – review & editing. All of the authors have contributed to the writing of the article and all of them have approved the final version of the paper.
Conflicts of interest
The authors declare that they have no conflict of interest.
References
- A. L. Phelan, R. Katz and L. O. Gostin, JAMA, 2020, 323, 709–710 CrossRef CAS PubMed.
- C. Sohrabi, Z. Alsafi, N. O'Neill, M. Khan, A. Kerwan, A. Al-Jabir, C. Iosifidis and R. Agha, Int. J. Surg., 2020, 76, 71–76 CrossRef PubMed.
- World Health Organization, Clinical Management of Severe Acute Respiratory Infection when Novel Coronavirus (nCoV) Infection Is Suspected: Interim Guidance, https://www.who.int/docs/default-source/coronaviruse/clinical-management-of-novel-cov.pdf Search PubMed.
- How COVID-19 Spreads, https://www.cdc.gov/coronavirus/2019-ncov/prevent-getting-sick/how-covid-spreads.html?CDC_AA_refVal=https%3A%2F%2Fwww.cdc.gov%2Fcoronavirus%2F2019-ncov%2Fprepare%2Ftransmission.html.
- Covid-19 coronavirus pandemic, https://www.worldometers.info/coronavirus/?utm_campaign=homeAdTOA?.
- WHO announces COVID-19 outbreak a pandemic, http://www.euro.who.int/en/health-topics/health-emergencies/coronavirus-covid-19/news/news/2020/3/who-announces-covid-19-outbreak-a-pandemic.
- I. Thevarajan, T. H. Nguyen, M. Koutsakos, J. Druce, L. Caly, C. E. van de Sandt, X. Jia, S. Nicholson, M. Catton and B. Cowie, Nat. Med., 2020, 26, 453–455 CrossRef CAS PubMed.
- S. Wang, L. Guo, L. Chen, W. Liu, Y. Cao, J. Zhang and L. Feng, Clinical infectious diseases: an official publication of the Infectious Diseases Society of America, 2020 Search PubMed.
- X. Cao, COVID-19: immunopathology and its implications for therapy, Nat. Rev. Immunol., 2020, 20(5), 269–270 CrossRef CAS PubMed.
- M. Z. Tay, C. M. Poh, L. Rénia, P. A. MacAry and L. F. Ng, Nat. Rev. Immunol., 2020, 1–12 Search PubMed.
- Q. Ye, B. Wang and J. Mao, J. Infect., 2020, 80, 607–613 CrossRef CAS PubMed.
- D. McGonagle, K. Sharif, A. O'Regan and C. Bridgewood, Autoimmun. Rev., 2020, 19, 102537 CrossRef CAS PubMed.
- D. Ragab, H. Salah Eldin, M. Taeimah, R. Khattab and R. Salem, Front. Immunol., 2020, 11, 1446 CrossRef CAS PubMed.
- T. Lammers, A. M. Sofias, R. van der Meel, R. M. Schiffelers, G. Storm, F. Tacke, S. Koschmieder, T. H. Bruemmendorf, F. Kiessling and J. M. Metselaar, Nat. Nanotechnol., 2020, 15(8), 622–624 CrossRef CAS PubMed.
- M. S. Draz, M. Venkataramani, H. Lakshminarayanan, E. Saygili, M. Moazeni, A. Vasan, Y. Li, X. Sun, S. Hua, X. G. Yu and H. Shafiee, Nanoscale, 2018, 10, 11841–11849 RSC.
- S. M. Russell, A. Alba-Patiño, E. Baron, M. Borges, M. González-Freire and R. de la Rica, ACS Sens., 2020, 5(6), 1506–1513 CrossRef CAS PubMed.
- P. Chen, N.-T. Huang, M.-T. Chung, T. T. Cornell and K. Kurabayashi, Adv. Drug Delivery Rev., 2015, 95, 90–103 CrossRef CAS PubMed.
- J. R. Tisoncik, M. J. Korth, C. P. Simmons, J. Farrar, T. R. Martin and M. G. Katze, Microbiol. Mol. Biol. Rev., 2012, 76, 16–32 CrossRef CAS PubMed.
- B. Leca-Bouvier and L. J. Blum, Anal. Lett., 2005, 38, 1491–1517 CrossRef CAS.
- I. E. Tothill, Semin Cell Dev Biol, 2009, 20, 55–62 CrossRef CAS PubMed.
- D. Sadighbayan, K. Sadighbayan, M. R. Tohid-kia, A. Y. Khosroushahi and M. Hasanzadeh, TrAC, Trends Anal. Chem., 2019, 118, 73–88 CrossRef CAS.
- X. Luo and J. J. Davis, Chem. Soc. Rev., 2013, 42, 5944–5962 RSC.
- C. Dincer, R. Bruch, E. Costa-Rama, M. T. Fernández-Abedul, A. Merkoçi, A. Manz, G. A. Urban and F. Güder, Adv. Mater., 2019, 31, 1806739 CrossRef PubMed.
- B.-Y. Chang and S.-M. Park, Annu. Rev. Anal. Chem., 2010, 3, 207–229 CrossRef CAS PubMed.
- A. Zandi, A. Gilani, F. Abbasvandi, P. Katebi, S. R. Tafti, S. Assadi, H. Moghtaderi, M. S. Parizi, M. Saghafi, M. A. Khayamian, Z. Davari sh, P. Hoseinpour, M. Gity, H. Sanati and M. Abdolahad, Biosens. Bioelectron., 2019, 142, 111566 CrossRef CAS PubMed.
- A. Walcarius, S. D. Minteer, J. Wang, Y. Lin and A. Merkoçi, J. Mater. Chem. B, 2013, 1, 4878–4908 RSC.
- S. Zanganeh, F. Khodadadei, S. R. Tafti and M. Abdolahad, J. Mater. Sci. Technol., 2016, 32, 617–625 CrossRef CAS.
- M. Abdolahad, H. Taghinejad, A. Saeidi, M. Taghinejad, M. Janmaleki and S. Mohajerzadeh, RSC Adv., 2014, 4, 7425–7431 RSC.
- O. Akhavan, E. Ghaderi, R. Rahighi and M. Abdolahad, Carbon, 2014, 79, 654–663 CrossRef CAS.
- R. Hajian, S. Balderston, T. Tran, T. deBoer, J. Etienne, M. Sandhu, N. A. Wauford, J.-Y. Chung, J. Nokes, M. Athaiya, J. Paredes, R. Peytavi, B. Goldsmith, N. Murthy, I. M. Conboy and K. Aran, Nat. Biomed. Eng., 2019, 3, 427–437 CrossRef CAS PubMed.
- S. H. Chae and Y. H. Lee, Nano Convergence, 2014, 1, 15 CrossRef PubMed.
- Y. Shao, J. Wang, H. Wu, J. Liu, I. A. Aksay and Y. Lin, Electroanal., 2010, 22, 1027–1036 CrossRef CAS.
- G. Korznikova, T. Czeppe, G. Khalikova, D. Gunderov, E. Korznikova, L. Litynska-Dobrzynska and M. Szlezynger, Mater. Charact., 2020, 161, 110122 CrossRef CAS.
- H. C. Lee, W.-W. Liu, S.-P. Chai, A. R. Mohamed, A. Aziz, C.-S. Khe, N. M. Hidayah and U. Hashim, RSC Adv., 2017, 7, 15644–15693 RSC.
- Clinical management of COVID-19, https://www.who.int/publications-detail/clinical-management-of-covid-19.
- G.-Y. Xu, H.-A. Yu, J. Hong, M. Stahl, T. McDonagh, L. E. Kay and D. A. Cumming, J. Mol. Biol., 1997, 268, 468–481 CrossRef CAS PubMed.
- S. R. Sprang and J. F. Bazan, Curr. Opin. Struct. Biol., 1993, 3, 815–827 CrossRef CAS.
- E. Neria, S. Fischer and M. Karplus, J. Chem. Phys., 1996, 105, 1902–1921 CrossRef CAS.
- W. L. Jorgensen, J. Chandrasekhar, J. D. Madura, R. W. Impey and M. L. Klein, J. Chem. Phys., 1983, 79, 926–935 CrossRef CAS.
- Z. Wang and M. Devel, Phys. Rev. B: Condens. Matter Mater. Phys., 2011, 83, 125422 CrossRef.
- T. Darden, D. York and L. Pedersen, J. Chem. Phys., 1993, 98, 10089–10092 CrossRef CAS.
- J.-P. Ryckaert, G. Ciccotti and H. J. Berendsen, J. Comput. Phys., 1977, 23, 327–341 CrossRef CAS.
- J. C. Phillips, R. Braun, W. Wang, J. Gumbart, E. Tajkhorshid, E. Villa, C. Chipot, R. D. Skeel, L. Kale and K. Schulten, J. Comput. Chem., 2005, 26, 1781–1802 CrossRef CAS PubMed.
- W. Humphrey, A. Dalke and K. Schulten, J. Comput. Chem., 1996, 14, 33–38 CAS.
- A. MacKerell, FASEB J., 1992, 6, A143 Search PubMed.
- A. D. MacKerell Jr, D. Bashford, M. Bellott, R. L. Dunbrack Jr, J. D. Evanseck, M. J. Field, S. Fischer, J. Gao, H. Guo and S. Ha, J. Phys. Chem. B, 1998, 102, 3586–3616 CrossRef PubMed.
- L. B. Wright, P. M. Rodger, S. Corni and T. R. Walsh, J. Chem. Theory Comput., 2013, 9, 1616–1630 CrossRef CAS PubMed.
- J. Lazarovits, Y. Y. Chen, E. A. Sykes and W. C. Chan, Chem. Commun., 2015, 51, 2756–2767 RSC.
- P. Mehta, D. F. McAuley, M. Brown, E. Sanchez, R. S. Tattersall and J. Manson, The Lancet, 2020, 395, 1033–1034 CrossRef CAS.
- G. Chen, D. Wu, W. Guo, Y. Cao, D. Huang, H. Wang, T. Wang, X. Zhang, H. Chen and H. Yu, J. Clin. Investig., 2020, 130, 2620–2629 CrossRef CAS PubMed.
- M. A. Khayamian, S. Ansaryan, S. R. Tafti, M. S. Nikshoar, M. H. Amiri, M. Baniassadi and M. Abdolahad, Sens. Actuators, B, 2018, 255, 1–7 CrossRef CAS.
- M. A. Khayamian, M. Baniassadi and M. Abdolahad, Ultrason. Sonochem., 2018, 41, 619–625 CrossRef CAS PubMed.
- G. Jo, M. Choe, S. Lee, W. Park, Y. H. Kahng and T. Lee, Nanotechnol., 2012, 23, 112001 CrossRef PubMed.
- K. Yamanaka, M. d. C. Vestergaard and E. Tamiya, Sens. Actuators, B, 2016, 16, 1761 Search PubMed.
- M. A. Khayamian, S. Shalileh, S. Vanaei, M. Salemizadeh Parizi, S. Ansaryan, M. Saghafi, F. Abbasvandi, A. Ebadi, P. Soltan Khamsi and M. Abdolahad, Drug Delivery, 2019, 26, 928–934 CrossRef CAS PubMed.
- G. Yang, J. Cao, L. Li, R. K. Rana and J.-J. Zhu, Carbon, 2013, 51, 124–133 CrossRef CAS.
- S. M. Abdin, S. M. Elgendy, S. K. Alyammahi, D. W. Alhamad and H. A. Omar, Life Sci., 2020, 118054 CrossRef CAS PubMed.
- S.-h. Lin, Y.-s. Zhao, D.-x. Zhou, F.-c. Zhou and F. Xu, Genes Dis., 2020, 7(4), 520–527 CrossRef CAS PubMed.
- N. Vaninov, Nat. Rev. Immunol., 2020, 20(5), 277 CrossRef CAS PubMed.
- M. Ghaderinia, M. A. Khayamian, H. Abadijoo, S. Shalileh, M. Faramarzpour, A. Zandi, H. Simaee, F. Abbasvandi, M. R. Esmailinejad, S. Rafizadeh-Tafti, M. Jahangiri, Y. Kordehlachin, H. Ghaffari, E. Ansari, N. Dabbagh, M. E. Akbari, P. Hoseinpour and M. Abdolahad, Biosens. Bioelectron., 2021, 183, 113194 CrossRef CAS PubMed.
Footnote |
† Electronic supplementary information (ESI) available. See DOI: 10.1039/d1ra04298j |
|
This journal is © The Royal Society of Chemistry 2021 |
Click here to see how this site uses Cookies. View our privacy policy here.