DOI:
10.1039/D1MA01180D
(Review Article)
Mater. Adv., 2022,
3, 4088-4102
Polydiacetylene-based sensors for food applications
Received
14th December 2021
, Accepted 14th April 2022
First published on 18th April 2022
Abstract
Polydiacetylene (PDA) sensors have been developed as food management tools due to their convenient colorimetric signal, low cost, versatility, and ability to be incorporated into food packaging materials and devices. In this review, we discuss methods of PDA sensor fabrication, their use as food quality and food contaminant sensors, and their incorporation into materials and devices. We highlight PDA quality monitoring sensors for meat, milk, fruits, and beverages, and contaminant detection systems for pathogens, antibiotics, pesticides, and heavy metals. We evaluate different PDA sensor formats for food applications including solution-based tests and substrate-based sensors, such as hydrogels and films. Finally, we discuss our perspective on the challenges and opportunities for PDA food sensors to provide inspiration and direction for future research.
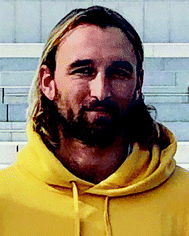
Max Weston
| Max Weston received his Bachelor of Engineering in Chemical Engineering from The University of Sydney, Australia in 2016, and PhD in Chemical Engineering from The University of New South Wales (UNSW Sydney), Australia in 2020. His research focuses on developing polydiacetylene-based sensors to monitor food quality and food contaminants. |
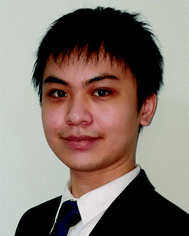
Andy-Hoai Pham
| Andy-Hoai Pham obtained his Bachelor of Engineering in Chemical Engineering from The University of New South Wales (UNSW Sydney), Australia in 2022. His research focuses on developing polydiacetylene-based sensors for the detection of disease biomarkers. |
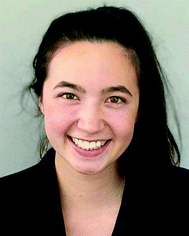
Jennessa Tubman
| Jennessa Tubman obtained her Bachelor of Science (BSc Honours) in Food Science and Technology from The University of New South Wales (UNSW Sydney), Australia in 2022. Her research focuses on colorimetric polydiacetylene-based sensors for food quality and safety. |
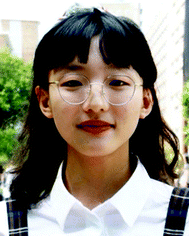
Yuan Gao
| Yuan Gao received her Bachelor of Biomedical Engineering from The University of Sydney, Australia in 2017. Yuan is now a PhD candidate at The University of New South Wales (UNSW Sydney), Australia. Her research focuses on colorimetric sensors for disease diagnosis and food monitoring. |
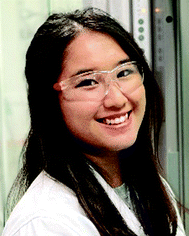
Angie Davina Tjandra
| Angie Davina Tjandra obtained her Bachelor of Engineering in Chemical and Biomolecular Engineering from The University of Sydney, Australia in 2018. She is now a Scientia PhD scholar at The University of New South Wales (UNSW Sydney), Australia. Her research focuses on developing polydiacetylene-based sensors for early-stage disease detection. |
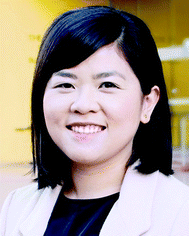
Rona Chandrawati
| Rona Chandrawati is a Scientia Associate Professor and National Health and Medical Research Council (NHMRC) Emerging Leadership Fellow at The University of New South Wales (UNSW Sydney), Australia. She obtained her PhD from the Department of Chemical and Biomolecular Engineering at The University of Melbourne in 2012. She was then a Marie Curie Fellow at Imperial College London before returning to Australia in 2015 to establish her research group. Her research interests include bioinspired nanomaterials for sensing and drug delivery. |
1. Introduction
Food waste has been identified as a major economic, environmental, and public health concern.1–3 Monitoring tools are required to mitigate food loss under increasing pressure from climate change, increasing food demand, and globalization of the food system. Sensors have been identified as useful tools to reduce waste throughout food production, distribution, and consumption.4,5 Polydiacetylenes (PDAs) are a class of conjugated polymers with unique optical properties that can be used to build colorimetric and fluorescent sensors.6–9 They exhibit a strong blue to red color change and a “turn on” fluorescence signal in response to stimuli such as heat, pressure, light, chemical and biological recognition events.9,10 The color change can be read by the naked eye, and the sensors are versatile, low in cost, and biocompatible. They can also be cast into a variety of materials including films, gels, fibers, inks, and sampling devices.11 This combination of attributes makes PDAs ideal for building sensors to detect food contaminants and quality markers.
PDA is formed by the self-assembly of amphiphilic diacetylene (DA) monomers into vesicles, films, and other organized architectures suitable for polymerization. Exposing the DA structures to 254 nm UV light drives a 1,4-addition reaction producing an alternating ene–yne polymer chain. The PDA product has a conjugated backbone with a delocalized network of π electrons which imparts the polymer with unique optical properties. Typically photopolymerization yields non-fluorescent, blue PDAs with a characteristic absorption peak at 640 nm. Stimulation of blue phase PDA with heat, mechanical stress, chemical recognition events or changes in its chemical environment can induce perturbations and conformational rotation of the PDA's ene–yne backbone (Fig. 1). This changes the degree of conjugation in the polymer and results in a characteristic blue (640 nm) to red (540 nm) color change and fluorescent signal. PDAs can be functionalized to detect specific biomarkers (e.g. pathogens, pollutants, quality markers) by the conjugation or insertion of recognition elements (e.g. aptamers, antibodies) into the polymer network during synthesis. A broad and maturing body of research on PDA sensors is developing for medical,12,13 environmental,14 and food15,16 related applications.
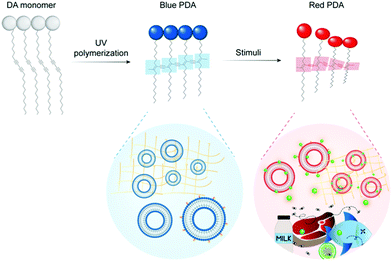 |
| Fig. 1 Schematic diagram of PDA synthesis including the self-assembly of diacetylene monomers, photopolymerization to form blue phase PDA, and the red-to-blue color change due to conformational twisting in the conjugated polymer backbone in response to stimuli, e.g. the presence of food contaminants. | |
Some excellent reviews have been conducted to summarize and discuss the state-of-the-art in PDA sensors research, and they serve as excellent guides for sensor optimization. Wen et al. provide a review on PDA chemical modification and typical research methodologies to characterize PDA.7 Recently, we discuss strategies to tune the chromatic response, sensitivity, and specificity of PDA sensors.17 In a complimentary review, Kim et al. elaborate design strategies that can be used to enhance the sensitivity and specificity of PDA sensors.18 Qian et al. reviewed recent developments of PDA-based sensors and categorized them by the structure of their DA monomer.9 Recent reviews have also discussed the use of PDA sensors in medical,12,13 environmental,14 and food15,16 related applications. A comprehensive review of the use of PDA for the detection of contaminants and quality markers in food has not been conducted. Herein, we aim to review the literature relating to PDA sensors for contaminant and quality detection in food focusing on literature produced since 2015. We will first review processes for PDA manufacturing, PDA sensors for different food products, and progress in the incorporation of PDA into packaging materials and sampling devices. Finally, we will discuss challenges and opportunities for PDA food sensors in a hope to guide and inspire future research.
2. Fabrication of polydiacetylenes
The fabrication of PDA sensors is a flexible process and a variety of methods have been developed.11 Typically, these methods will aim to: (1) arrange DA monomers into a structure suitable for polymerization, (2) facilitate the incorporation of recognition elements, (3) provide the opportunity for secondary processing into a range of materials. Many methods exploit the amphiphilic properties of DA monomers to drive self-assembly processes. Common carboxy-terminated DAs are illustrated in Fig. 2, highlighting typical variations in the DA's alkyl chain and positioning of the diacetylene functional group that can be used to control sensitivity and specificity.17,19 The most common PDA architectures are vesicles,13,20 Langmuir films,21 coatings,22 and fibers.23
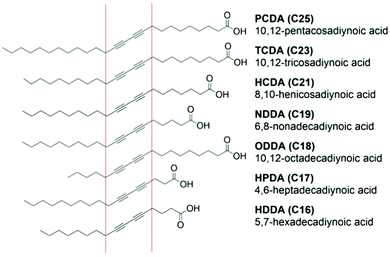 |
| Fig. 2 Commonly investigated diacetylene monomers in food applications that vary in alkyl chain length and positioning of the diacetylene functional group. Reproduced from ref. 24 with permission from Elsevier, copyright 2021. | |
The characteristics of the DA monomers play a significant role in their sensing properties and self-assembly behavior and should be considered during the fabrication of PDA-based sensors. Typical DA monomers can vary in the head group, the length of the alkyl tail, and the positioning of the diacetylene group (Fig. 2). These features dictate the intermolecular forces between neighboring DA monomers which govern self-assembly and the phase transition temperature.25 They also determine the degree and ease of distortion of PDA conjugation in response to stimuli, which determines their sensitivity as a PDA sensor.17 In general, PDAs constructed from short chain DA monomers offer higher sensitivity than longer monomers. Shorter chain monomers have lower phase transition temperatures and will self-assemble at lower temperatures, reducing the energy intensity of fabrication. However, if the carbon chain is of insufficient length, they can lack the amphiphilicity required to drive self-assembly into vesicles of regular morphology. A compromise between the ability to self-assemble and sensitivity must therefore be considered during fabrication. Short chain DAs that fail to assemble into vesicles are better suited to films and fibers.
Another important consideration in the fabrication process is the functionalization of the PDAs with recognition elements or receptors that are specific to target analytes. Functionalization can be conducted before or after self-assembly and typically prior to photopolymerization to preserve the integrity of the PDA conjugation. This can be achieved using a variety of approaches which have been broadly categorized into two main methods in an excellent review by Chen et al.10 In the first and most common approach, a DA monomer is modified by covalently linking a recognition element to the DA head group via a chemical reaction. Changing the chemistry of the head group can impact the intermolecular forces and inhibit self-assembly. For this reason, modified DAs are commonly mixed with unmodified DAs in a ratio that will maximize the density of functional groups while still enabling self-assembly. Alternatively, in a similar approach, DA monomers can first be self-assembled into desired supramolecular structures and then covalently modified with functional groups to avoid limitations related to self-assembly. This can be achieved by directly modifying the head group of homogeneous structures of carboxy-terminated DAs. A heterogeneous mixture of DAs can also be used where a specific ratio of the monomers will contain binding sites for the desired recognition element. DAs can also be included that have binding sites selective for the surface of a substrate which can facilitate the binding of PDA particles to materials and surfaces. In contrast to the covalent approaches discussed above, PDAs can be functionalized by the hydrophobic insertion of recognition elements. In this approach, the recognition element must contain a hydrophobic moiety that is driven into the diacetylene bilayer in an aqueous solution.
2.1 Vesicles
Vesicles are bilayer, spherical colloids that consist of a hydrophobic membrane encapsulating a hydrophilic moiety. Amphiphilic DA monomers with suitable properties self-assemble into vesicular structures in aqueous media. Vesicles are categorized by size and lamellarity. This includes small unilamellar vesicles (SUVs), large unilamellar vesicles (LUVs), large multilamellar vesicles (LMVs), and multivesicular vesicles.26 SUVs are ideal architectures for PDA sensors as they provide a spherical single-layer membrane that can be decorated with recognition elements by insertion or conjugation.10 PDA vesicles are commonly utilized in solution-based assays or can be incorporated into gels and films or cast onto substrates. The thin film hydration method and the solvent injection method are the typical processes used to construct PDA vesicles.
2.1.1 Thin film hydration method.
The thin film hydration method (Bangham method)27 is a versatile micro-batch approach. It is a simple procedure and requires few resources making it a common choice for PDA researchers.13,20 DA monomers are dissolved in a polar solvent and filtered to remove polymer contaminants. The solvent is evaporated in a container with an inert gas such as nitrogen to leave a thin DA film coating the vessel. Hydration of the film above the DA phase transition temperature results in the spontaneous formation of multilamellar vesicles. The method is easily adapted to incorporate hydrophobic and hydrophilic compounds. Hydrophobic compounds are included in the DA and polar solvent solution and are ultimately encapsulated in the PDA vesicle membrane. Hydrophilic compounds can be included in the solution used to hydrate the DA film and can be encapsulated within the vesicle's hydrophilic moiety or occupy the surrounding solution. To produce unilamellar vesicles of homogenous size, secondary processing such as sonication or extrusion is necessary. These techniques are energy intensive and risk the integrity of incorporated biomolecules as they must be conducted above the relatively high phase transition temperature of the DA monomer (50–80 °C).
2.1.2 Solvent injection method.
The solvent injection method was first developed for the construction of unilamellar phospholipid vesicles without the need for secondary processing to control particle distribution and morphology.28 Our laboratory and other research groups have applied this method for the production of PDA vesicles as an alternative to the thin film hydration method.24,29–31 The DA monomers are dissolved in a polar solvent and slowly injected into an aqueous solution that is above the phase transition temperature of the monomer. The DA monomers self-assemble into vesicles and the solvent is evaporated from the solution. Control of the process parameters, such as injection rate and solvent type, can be used to control the particle's morphology and SUVs can be formed without the need for secondary processing such as extrusion or sonication. In contrast to the thin film hydration method, the solvent injection method has the potential to be adapted into a continuous flow process ideal for industrial fabrication. Further work is required to demonstrate the method's efficacy and versatility by process adaption to include recognition elements, cargo, and materials to form nanocomposites. Traiphol et al. have recently developed an adaption of this method called the solution-mixing method where they combined a mixture of water and DA monomers dissolved in an organic solvent before incubating at room temperature for at least two hours.32 By controlling the ratio and type of organic solvent, they were able to control the particle morphology.33 They have also demonstrated the use of this method to form PDA/ZnO nanoparticles to develop acid and base and reversible thermochromic sensors.32
2.2 Thin films
Thin polymer films are continuous solid materials with thicknesses ranging from several nanomaterials to micrometers. They are used to contain volumes of materials, act as a membrane or coating, or serve as a surface for a sensor. The multiple functions of thin films and their ability to be incorporated into food packaging have made them a point of interest for researchers. Thin films can be categorized by their method of manufacture or their physical properties such as the number of polymer layers and the degree of molecular order in these layers. The film's physical properties are generally dictated by the method of manufacture. Langmuir, deposition, and electrospinning methods are commonly used to construct single and multilayer PDA films.
2.2.1 Langmuir films.
As amphiphilic molecules, DA monomers readily form Langmuir films on the surface of aqueous media.21 These films are one molecule thick and organized with the hydrophilic moiety of the monomer oriented towards the solution and the hydrophobic moiety away from the solution. This organizes the films for polymerization and facilitates transfer to a variety of substrates using the Langmuir Schaffer or Langmuir Blodgett method. This is an effective way to functionalize the surface of materials with PDA sensors with a high degree of control of the molecular structure. Recognition elements can be included in the films. However, thin films are single layer and suffer from reduced color intensity making them harder to read by eye.
2.2.2 Deposited films.
DA monomers can be dissolved in a polar solvent and be directly deposited onto substrates such as glass, paper, or silica plates.22,34 These films are less organized and therefore polymerization efficiency is less than other methods. However, the process can be repeated to build multiple layers of PDA to increase the color intensity of the sensor. Although the method is inefficient, its simplicity is attractive, and it enables the use of small chain or non-linear DAs that do not self-assemble into vesicles. The DA monomers can be combined with matrix polymers by their inclusion in the polar solvent mixture that is cast onto the substrate. This can be used to improve mechanical properties, assist in binding to the substrate, and functionalize the film to analytes like volatile organic compounds (VOCs) that interact with the matrix polymer.8
2.3 Fibers
Electrospinning is an effective way to produce long PDA polymer fibers with diameters ranging from nanometres to micrometers.35,36 DA monomers are mixed with a matrix polymer and dissolved in a polar solvent. A voltage is applied to a capillary on a reservoir containing the polymer solution which ejects a controlled stream from the container. The solvent evaporates from the stream and a composite fiber is formed. The DA monomers can then be polymerized within the matrix polymer to form PDA. This method does not allow easy incorporation of biological recognition elements in contrast to vesicles and is better suited for thermochromic, mechanical, or gas sensing applications.
3. Polydiacetylene-based sensors for food applications
PDA sensors are excellent for food-related applications because they are cheap, biocompatible, versatile, and can be combined with a range of materials and devices that have promise for inclusion in food packaging, processing, and production. Furthermore, the pronounced colorimetric signal of the sensor can be easily read by consumers and workers without technical training. PDA food sensors can be broadly categorized as quality sensors and contaminant detection sensors. Quality sensors monitor the chemical composition of food and detect changes in its endogenous chemical constitution to infer quality. Typical quality sensors monitor the products of lipolysis or proteolysis such as free fatty acids and VOCs. These have great potential to be included into food packaging to complement traditional use-by dates which have been identified as a cause of food waste. In contrast, contaminant detection systems target exogenous analytes such as pathogens or pesticides. Here we review PDA quality sensors for different food products (Table 1), including meat, milk, fruits, and beverages before discussing contaminant detection systems that could be applied across a variety of products.
Table 1 Polydiacetylene-based sensors for food applications discussed in this review
Food product |
Target analyte |
Sensor format |
Limit of detection |
Temperature tested |
Ref. |
Beef |
Ammonia |
PDA/PVA film |
650 ppm |
25 °C |
40
|
Beef |
Ammonia |
PDA/chitosan film |
100 ppm |
−20 °C, 4 °C, 25 °C |
41
|
Fish |
Escherichia coli
|
PDA microneedle |
8 × 107 cells per mL |
25 °C |
50
|
Fish |
Histamine |
PDA/cellulose membrane |
70 ppm |
−20 °C, 4 °C, 25 °C |
42
|
Fish and chicken |
Propylamine |
PDA fiber |
7 ppm |
25 °C |
43
|
Chicken |
Salmonella choleraesuis
|
PDA solution |
1 S. choleraesuis strain |
25 °C |
51
|
Milk |
Lactic acid |
PDA hydrogel |
N/A |
4 °C, 25 °C |
45
|
Milk |
Free fatty acid |
PDA hydrogel |
N/A |
4 °C, 25 °C |
47
|
Milk |
Free fatty acid |
PDA solution |
10 μg mL−1 |
4 °C, 25 °C |
46
|
Milk |
Staphylococcus aureus alpha-hemolysin |
PDA solution |
6.62 μg mL−1 |
25 °C |
19
|
Fruit |
Ethylene |
PDA/chitosan film |
600 ppm |
25 °C |
48
|
Fruit |
Xylella fastidiosa
|
PDA/PVDF strip |
8 × 107 cells per mL |
25 °C |
22
|
Beer |
4-Vinyl guaiacol |
PDA solution |
0.19 mM |
25 °C |
49
|
Beer |
Diacetyl |
PDA solution |
0.15 mM |
25 °C |
49
|
3.1 Polydiacetylene food quality sensors
3.1.1 Meat.
Meat is composed of water, protein, fat, carbohydrates, and nitrogen compounds. Proteolysis during the decomposition or spoilage of meat results in a characteristic increase in ammonia and biogenic amines (BAs) such as cadaverine, putrescine, and histamine.37–39 Ammonia and BA concentration is therefore a useful indicator of meat quality and its suitability for consumption. Typical PDAs have a carboxylic acid head group that undergoes acid-base reactions with ammonia. This reaction converts the neutrally charged carboxylic acid head group of PDA to a negatively charged carboxylate. Repulsive charges between neighboring carboxylate head groups lead to strain and rotation in the PDA conjugated backbone resulting in a colorimetric and fluorescence signal. This phenomenon has been exploited to construct a variety of PDA-based sensors to monitor meat quality.
Park et al. fabricated PDA/poly(vinyl alcohol) (PVA) hybrid films using a drop-casting method to detect ammonia released from beef spoilage.40 The films exhibit a blue to red color change upon exposure to ammonia, which is attributed to an acid–base reaction between the carboxylic acid head groups of PDA and ammonia gas. This was verified with Fourier-Transform Infrared (FTIR) spectroscopy analysis which confirmed the transition of carboxyl groups to carboxylate groups after exposure to ammonia gas. The study investigated the impact of DA monomer length on sensor performance. PDA/PVA films synthesized using 6,8-heneicosadiynoic acid (HCDA, 21 carbon atoms) were more sensitive than the films using 10,12-pentacosadiynoic acid (PCDA, 25 carbon atoms). These results are consistent with the literature, as shorter chain length DA monomers produce PDA with weaker intermolecular forces and higher sensitivity.17 Poly(HCDA)/PVA films exhibited a chromatic shift visible to the naked eye under the presence of 650 ppm ammonia after 2.5 h incubation. The sensor response was concentration-time-dependent, wherein red PDA formed within 1 h when exposed to 1000 ppm ammonia. They demonstrated the effectiveness of the system to monitor beef spoilage at 25 °C.
In our own work, we fabricated PDA sensor films by mixing PDA vesicles with cellulose nanocrystals stabilized in a chitosan film to produce a highly sensitive ammonia sensor suitable for incorporation in food packaging.41 The sensors were designed to monitor ammonia released by spoiling meat at temperatures between −20 °C and room temperature. Chitosan was chosen as it is biodegradable, non-toxic, and has been used in food packaging, and cellulose nanocrystals were added to improve the film's mechanical properties and to stabilize the PDA vesicle suspension. The film underwent visible chromatic shifts in the presence of 500 ppm ammonia in sub-zero conditions and can detect ammonia at 100 ppm at room temperature. We tested the film's efficacy at different temperatures demonstrating it could detect spoiled meat in a temperature range relevant to meat cold chain supply. An example of sensor attachment on food packaging is shown in Fig. 3.
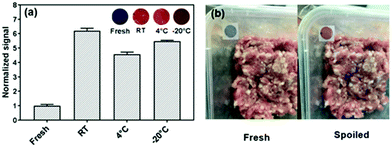 |
| Fig. 3 (a) The colorimetric response of PDA/cellulose nanocrystal/chitosan films to fresh and spoiled meat at different temperatures (RT – room temperature, 4 °C and −20 °C) (inset presents the digital images of the sensors). (b) Photographs of PDA/cellulose nanocrystal/chitosan sensors applied on food packaging as an indicator for meat spoilage. Reproduced from ref. 41 with permission from The Royal Society of Chemistry, copyright 2019. | |
Li et al. also demonstrated an example of a PDA-based sensor to detect fish spoilage at temperatures ranging between −20 °C and room temperature (Fig. 4).42 They developed PDA colorimetric strips to detect histamine as its level typically rises in fish as it ages. The strips were constructed by simply depositing PDA/DMPC vesicles onto cellulose nitrate membranes. The sensors were stable over two-week storage at room temperature and exhibited a linear response to histamine within the range from 70 to 2240 ppm. The PDA showed little response to nitrogen, oxygen, carbon dioxide, argon, and hydrogen sulfide, indicating that the sensor signal was specific to histamine. The authors demonstrated the effectiveness of the sensor by inclusion on the inside of the lid of the canned fish products.
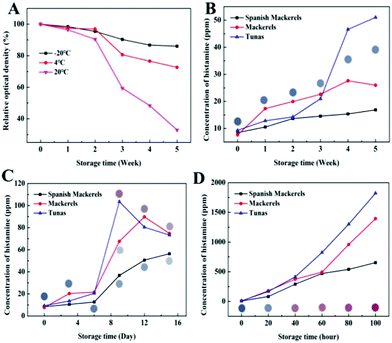 |
| Fig. 4 (A) Storage stability of PDA sensor strips at different temperatures. Histamine content in fish at (B) −20 °C, (C) 4 °C, and (D) 20 °C, and the images of the PDA sensor strips after exposure. Reproduced from ref. 42 with permission from the American Chemical Society, copyright 2020. | |
Using a different fabrication process, Valdez et al. synthesized a silica-reinforced PDA nanofiber mat using a novel Forcespinning® technique that demonstrated optical sensing of BAs.43 They synthesized the mats from two different DA monomers and found mats constructed from 5,7-eicosadienoic acid (ECDA, 20 carbon atoms) exhibited greater sensitivity to BAs than PCDA. The typical response time of the sensor to n-propylamine was 10 minutes. Fish and chicken induced a greater colorimetric response than pork or beef in the PDA-ECDA mat, whereas the PDA-PCDA mat showed very little colorimetric response to any of the spoiling meat or fish. These results highlight the significance of choice in DA monomer and the necessity to consider the natural variation in BA profile released from different meat products. These results support concerns raised in our recent review that discusses challenges in designing food sensors due to high variability in the chemical composition of food products and the chemicals they release during spoilage.4
The literature suggests PDA is well suited for monitoring meat spoilage as ammonia and BAs can be detected by reaction with PDA's carboxy moiety. This eliminates the need for further functionalization of the sensor with recognition elements. Mixed PDA/polymer films and vesicles cast in films have been demonstrated as effective architectures. PDA films allow the use of a broader range of DAs including shorter monomers that are more sensitive.40,43 This promises a high degree of control in the detection range by simple manipulation of the DA monomer chain length. However, shortening of the DA monomer to control sensitivity also increases the sensitivity to temperature, mechanical stress, light, and other VOCs.17 Considering the broad range of conditions packaged food is subject to, these films could be susceptible to sensor drift and false positive signals. The development of materials that can selectively absorb ammonia and/or BAs, be readily combined with PDA, and stabilize PDA in the face of environmental sources of color change would greatly improve the performance of PDA meat quality sensors.
3.1.2 Milk.
Milk is composed of water, lactose, protein, and fat. The decomposition of these constituents during spoilage provides a variety of chemical markers that can be targeted by PDA sensors to infer quality. Oliveira et al. studied the stability of PCDA/DMPC vesicle suspension in the presence of chemicals that simulate the components of milk as a feasibility study for use as a sensor in milk products.44 It was found that CaCl2, CaHPO4, MgCl2, and MgHPO4 did not induce colorimetric changes but caused precipitation of the PCDA/DMPC vesicles after 4 days of storage. Whey proteins, β-lactoglobulin, and α-lactalbumin induced a color change of the PCDA/DMPC vesicles after 12 days of storage. In the absence of proteins, the vesicles were stable with no aggregation over 60 days when stored at temperatures below 20 °C.
We developed a PDA/ZnO nanocomposite for the colorimetric detection of dairy milk quality.45 As milk spoils, bacteria convert lactose to lactic acid, and the pH decreases from 6.8 to 4.0. ZnO nanoparticles were encapsulated by PDA vesicles using the thin film hydration method. The vesicles were then suspended in a hydrogel to form discs suitable for submersion in milk samples. The increase in acidity in spoiling milk leads to the dissociation of the ZnO core particle which disrupts intermolecular forces between ZnO particle surface and PDA headgroups. This perturbs the conjugation in the PDA vesicle and induces a visible blue to red color change. We demonstrated that the pH at which the system produces a colorimetric signal could be tuned by the appropriate selection of DA monomer and lipid doping. The inclusion of lipids and the selection of short chain DA monomers increased sensitivity to lactic acid. We found that a system constructed from 5,7-hexadecadiynoic acid (HDDA)/ZnO exhibited a colorimetric response in a suitable range to detect spoiled milk (Fig. 5). The ability to tune the system suggests it could be adapted to monitor lactic acid concentration in other foods.
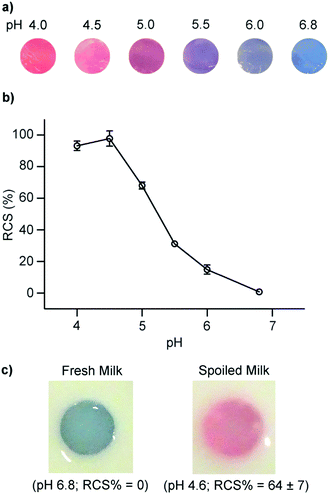 |
| Fig. 5 (a) Photographs and (b) red chromatic shift (RCS) of PDA/ZnO/hydrogels after exposure to spiked milk samples at different pH levels. Fresh milk was acidified by the addition of lactic acid to mimic microbial spoilage via lactic acid bacteria. (c) PDA/ZnO/hydrogels in fresh refrigerated milk (pH 6.8) and spoiled milk (pH 4.6). Reproduced from ref. 45 with permission from Elsevier, copyright 2020. | |
More recently, we constructed a PDA/DMPC sensor to detect free fatty acids (FFAs) to indicate milk quality.46,47 As milk spoils triglycerides are converted to short chain FFAs via bacterial lipolysis. At concentrations relevant in spoiled milk, we found the sensor exhibited a blue to red colorimetric response of short chain FFAs including octanoic acid, oleic acid, and linoleic acid. FFAs are surfactants which are known to disrupt PDA membranes by insertion of the alkyl tail into the hydrophilic moiety of the membrane. This disrupts the conjugated backbone in the PDA and results in the visible blue to red color change. We investigated the specificity of the sensor by incubation with common constituents in fresh and spoiled milk including sugar, acetic acid, ethanol, and triglycerides, which did not induce a colorimetric response in the sensor. We have demonstrated the utility and efficacy of these sensors to discriminate between fresh and spoiled almond milk46 and dairy milk.47 This highlights the sensor's potential to track fat rancidification to infer food quality in a range of fat-containing products.
3.1.3 Fruits.
Fruits are composed of water, carbohydrates, and typically a small amount of protein. Few consumer-oriented PDA sensors have been constructed for these food products as they are low in cost, pose lesser human health risks, and typically exhibit naturally occurring signals of quality such as color change and smell. However, some sensors have been designed to aid food management through distribution and processing. We developed thiol-functionalized PDA vesicles embedded into a chitosan film to detect ethylene released from kiwi fruit to monitor ripeness (Fig. 6).48 The thiol head group of the PDA reacts with ethylene via the Michael addition pathway which causes a perturbation in the PDA conjugation leading to a visible blue to red color change. The sensor's specificity was demonstrated by incubation with nitrogen, water vapor, ethanol, benzaldehyde, and carbon dioxide, which did not induce a color change. At concentrations equal or higher than 600 ppm ethylene, a color change was visible to the naked eye. Using digital image processing, the limit of detection was 3 times lower at 200 ppm ethylene. This is a promising low-cost device that could be used to determine fruit maturity during agricultural processing and control ambient ethylene concentration during fruit storage.
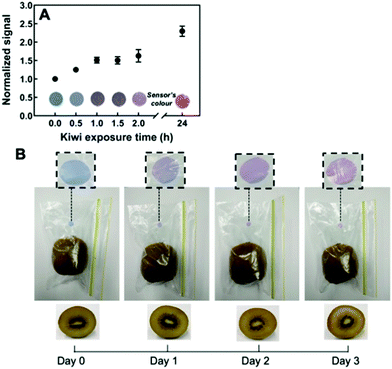 |
| Fig. 6 Monitoring ethylene gas in the headspace of ripening kiwi using thiol-functionalized PDA sensor films. (A) The sensor response is normalized to 1 kg of ripe kiwi. (B) Color transition of the sensor embedded inside a package containing one ripe kiwi over time. Reproduced from ref. 48 with permission from the American Chemical Society, copyright 2020. | |
3.1.4 Beverages.
Simple and reliable tools that can rapidly discriminate complex mixtures, e.g. alcoholic/non-alcoholic beverages, are of interest for product development and quality control in the food industry. Qian et al. designed and synthesized a sensor array comprised of five types of PDA vesicles for the discrimination of beer products.49 Each of the PDA vesicle sensors was functionalized by head group modification of the diacetylenes to detect ethanol and two specific flavoring molecules, 4-vinyl guaiacol and diacetyl (Fig. 7). The PDA sensors had a detection limit of 0.19 mM of 4-vinyl guaiacol (using the PEGylated thiol PDA) and 0.15 mM of diacetyl (using the PEGylated arginine PDA). Using spectroscopy and statistical analysis of the multiplex assay response, the authors were able to differentiate between 4 different commercial beers with ethanol concentrations ranging between 0.09 to 10 vol%. However, the ability of the sensors to discriminate between beers with similar ethanol concentrations was limited.
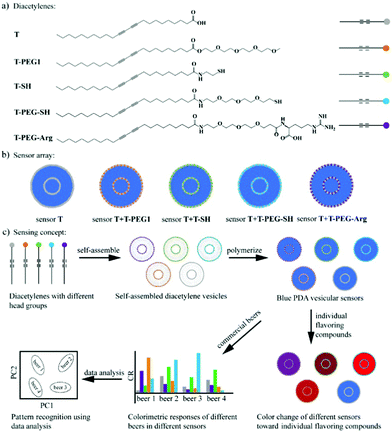 |
| Fig. 7 A PDA sensor array to discriminate beers. (a) Five DA monomers with different head groups used to assemble the PDA vesicles, including a carboxylic acid group (T), a polyethylene glycol chain (T-PEG1), a thiol group (T-SH), a PEGylated thiol group (T-PEG-SH), and a PEGylated arginine (T-PEG-Arg). (b) Five vesicular PDA sensors contained in the sensor array. (c) Schematic illustration of the discrimination of beers using the solution-based PDA sensor array: grouping of the beers using principal component analysis (PCA) on the basis of the different colorimetric responses of the sensors to different beers. Reproduced from ref. 49 with permission from the American Chemical Society, copyright 2020. | |
3.2 Polydiacetylene contaminant sensors
Contaminant detection systems target exogenous chemicals that threaten the quality of food and the safety of consumers. These systems generally employ recognition elements with high specificity for the analyte and could be applied across a range of different food products.
3.2.1 Pathogens.
Foodborne pathogens are a major public health concern and can lead to serious illness. Historic methods for pathogen detection are laboratory-based, time consuming, and expensive. Colorimetric PDA-based sensors offer a cheaper, rapid alternative that is better suited for food applications. Many PDA sensors have been designed for the detection of pathogens which are well discussed in a recent review by Lebegue et al.20 Due to the depth in literature on PDA-based systems for pathogen detection, we will limit our discussion to recent examples whose efficacy has been demonstrated in food samples. We highlight the different detection strategies including the direct and indirect detection of pathogenic contaminants.
To directly detect pathogens, PDA can be functionalized with recognition elements such as aptamers and antibodies that exhibit specific interactions with the target. Wen et al. recently developed a PDA-coated sensor strip for the detection of Xylella fastidiosa which causes Pierce's disease in grapevines.22 They conjugated an anti-Xylella fastidiosa antibody to the PDA headgroups, which upon biological interaction with Xylella fastidiosa exhibited a blue to red color change. The sensor has a 8 × 107 cells per mL limit of detection, which corresponds to the bacterial load in grapevines 18 days following bacterial inoculation. Similarly, Kim et al. functionalized PDA/DMPC vesicles with an E. coli-targeting antibody to detect the pathogen in fish samples.50 The sensors were incorporated into a silk-based microneedle sampling device for inclusion in food packaging, which will be discussed in more detail in Section 4 of this review.
Indirect detection methods target membrane-active compounds excreted by pathogens that are known to interact with biological cell membranes and they serve as excellent biomarkers. Recent works have demonstrated the biomimetic design of PDA vesicles mixed with lipids and compounds commonly found in biological cells to detect pathogens indirectly. Oliveira et al. developed a mixed PDA vesicle containing PCDA, sphingomyelin, cholesterol, and lysine to detect Salmonella in chicken samples.51 Chicken inoculated with Salmonella choleraesuis induced a strong blue to red color change in the vesicle, which the researchers attributed to chemical interactions between bacterial exotoxins and the lysine moiety of the sensors. More recently, we developed mixed vesicles composed of PDA, DMPC, and cholesterol to detect alpha-hemolysin, a pore-forming toxin produced by Staphylococcus aureus in milk, to aid in the diagnosis of bovine mastitis.19 We demonstrated cholesterol-dependent specific detection of alpha-hemolysin with a visible blue to red color change (Fig. 8). The toxins formed pores in the lipid and cholesterol moiety of the vesicles, which led to structural perturbation in the PDA sensor membrane. To investigate the sensor's selectivity, PDA/DMPC/cholesterol vesicles were incubated with lipopolysaccharide excreted by Escherichia coli and Listeriolysin O produced by Listeria monocytogenes, and no color change in the sensor was detected. The sensor was able to detect alpha-hemolysin in milk with a limit of detection of 6.62 μg mL−1, which is below the concentrations reported for bacteria isolated from mastitic cow infected with Staphylococcus aureus, indicating the sensor could be a useful contaminant detection system.
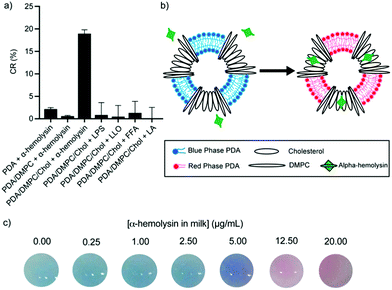 |
| Fig. 8 (a) Colorimetric response of PDA vesicles with varied constitution in response to Staphylococcus aureus α-hemolysin, Escherichia coli lipopolysaccharide (LPS), Listeria monocytogenes Listeriolysin O (LLO), free fatty acid (FFA), and lactic acid (LA). (b) Schematic illustration of the colorimetric change of a PDA vesicle caused via disruption of the phospholipid/cholesterol moiety from the pore-forming mechanism of α-hemolysin. (c) Photographs of PDA vesicles in milk spiked with different concentrations of α-hemolysin. Reproduced from ref. 19 with permission from the American Chemical Society, copyright 2020. | |
3.2.2 Antibiotics.
Due to an increase in agricultural intensity, antibiotics have become ubiquitous in animal farming to fight disease. Antibiotics in the food chain can pose human health risks and contribute to the development of antibiotic-resistant pathogens. As a result, the detection and control of antibiotics in food products is important. Recent work has developed PDA sensors as a cheap and rapid tool for the detection of antibiotic residues. Antibiotics typically inhibit bacterial proliferation by interaction with the pathogen's cells by disrupting the membrane or blocking important cell signaling pathways. PDA vesicles and membrane sensors have been designed to mimic this phenomenon to detect antibiotics. Kang et al. produced mixed PDA and phosphatidylinositol-4,5-bisphosphate (PIP2) vesicles capable of detecting aminoglycosidic antibiotics such as neomycin with a detection limit of 61 ppb. Neomycin was shown to bind to the PIP2 moiety of the mixed vesicles, which caused twisting of the PDA conjugated backbone and a visible blue to red color change.52 The sensor was shown to be specific as it did not react with non-aminoglycosidic antibiotics such as penicillin, oxytetracycline, and sulfamethazine. In a later iteration of their work, they successfully immobilized the sensor onto a simple paper-based colorimetric format.53 They mixed the PDA/PIP2 vesicles with a PVA polymer matrix before printing on a paper substrate to detect neomycin with a limit of detection of 1 ppm.
3.2.3 Pesticides.
Pesticides are toxic to humans and their residue on food is regulated. PDA sensors have been developed as cheaper and more rapid alternatives to traditional pesticide residue laboratory testing methods that are expensive and time consuming. Pimsen et al. created a PDA sensor to detect dichlorvos (an organophosphate pesticide) using an enzyme inhibition detection strategy (Fig. 9).54 The presence of dichlorvos inhibited the enzymatic activity of acetylcholinesterase towards choline ester. Negatively charged carboxylate groups of PDA interacted with the positively charged ammonium groups of the choline ester, and this reaction induced a blue-to-red color change of the PDA. The assay has a linear range of 0–200 ppb, with a limit of detection of 6.7 ppb using spectrometry and 50 ppb by naked eye observation. The system is highly selective when evaluated against other thio-organophosphates.
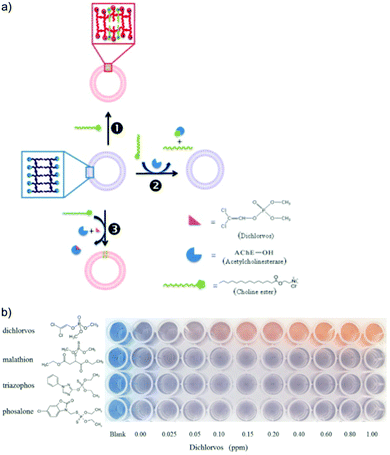 |
| Fig. 9 (a) Schematic illustration of dichlorvos detection based on PDA/acetylcholinesterase/choline ester system. (b) Colorimetric response of PDA/acetylcholinesterase/choline ester system to different types of organophosphate pesticides, highlighting its selectivity to dichlorvos with a low detection limit of 50 ppb. Reproduced from ref. 54 with permission from Elsevier, copyright 2014. | |
Alternatively, the head groups of PDA can be modified to react with organophosphate functional groups, which induce twisting in the PDA conjugated backbone and result in a visible blue to red color change. Alam et al. used an electrospinning technique to fabricate a PDA/cellulose composite fiber capable of detecting organophosphate pesticides.55 The PDA head groups were aldehyde modified to form a PDA ester (PCDA-HBA). The authors demonstrated the chemical recognition of diisopropyl fluorophosphate via a chemical reaction with the hydroxyl groups of PCDA-HBA resulting in a visible blue to red color change. They proposed the fibers could be used to manufacture smart fabrics for use in agricultural settings to detect glyphosates. Zhang et al. functionalized PDA vesicles to detect malathion by the conjugation of pyridine-2-aldoxime (PAM) to the vesicle head groups.56 The sensor was able to detect malathion in the millimolar range in a few seconds via electrostatic interaction with the positively charged PAM head groups. They attributed the blue to red color change to twisting of the PDA conjugated backbone, which they modeled with Density Functional Theory (DFT) calculations to support their hypothesis.
3.2.4 Heavy metals.
Heavy metals such as cadmium (Cd), lead (Pb), mercury (Hg), and arsenic (As) can accumulate in the food chain at various stages along the food production line, e.g. through farming, irrigation, or contamination during food processing and storage.57 These metals are highly toxic and carcinogenic even at a trace level and pose a significant threat to human health. It is therefore essential to detect and assess heavy metal residues in food products. Much of the research in PDA-based sensors to detect heavy metals is primarily in the application of environmental and water quality monitoring. It may be possible to repurpose these PDA-based sensor systems to perform heavy metal measurements in food.
In general, PDA-based sensors for heavy metal detection principally involve the modification of PDA head groups with moieties that form complexes with heavy metals. For example, for the detection of Cd2+ ions, Lee and co-workers synthesized PCDA monomers with chelidamic acid-picolylamine head groups (PCDA-CP), which selectively bind with Cd2+via Schiff base metal complexes.58 PDA vesicles composed of a mixture of PCDA and PCDA-CP monomers were formed and they exhibited colorimetric changes from blue to purple when exposed to Cd2+ in buffered solutions pH 7.4. The PDA-CP system detected Cd2+ with a limit of detection (LOD) of 18.5 μM and showed no reaction to other metal ions including Li+, Na+, K+, Cs+, Mg2+, Ca2+, Cr3+, Mn2+, Fe2+, Fe3+, Ni2+, Cu2+, Ag+, and Hg2+. However, a minor chromatic shift was observed in response to Zn2+. The authors later improved the selectivity of their PDA-based sensors by using PCDA with 5-hydroxy-N1,N3-bis(pyridin-2-ylmethyl)isophthalamide head groups (PCDA-HP) instead of PCDA-CP, which did not have any reaction to Zn2+.59 The sensitivity of the PDA-HP sensors to Cd2+ was slightly improved with a LOD of 16.5 μM in buffered solutions pH 7.4. To further increase the sensitivity, the authors designed the PDA-based sensor with different DA monomer compositions by using mono 2-picolylamine (MP) as a head group modification on PCDA (PCDA-MP).60 This led to an increase in Cd2+ sensitivity with a LOD of 2.1 μM while retaining the high selectivity of the PDA sensors to other metal ions. For the detection of Pb2+, recognition moieties such as dopamine,61 galloyl,62 1-aza-18-crown-6-ether,63 and glycine64 have been incorporated into PDA-based sensors for selective and sensitive detection of Pb2+ at as low as 0.24 μM. The maximum permissible concentration of Cd and Pb in food is 2 ppm (17.8 μM) and 3 ppm (14.5 μM), respectively,65 therefore these PDA-based sensors could potentially be used to detect Cd and Pb content in food. However, further investigation will be required to ensure that the PDA sensors with modified head groups do not inadvertently react to the myriad of chemicals found in food.
4. Polydiacetylene sensor formats
PDA has been combined into materials and testing devices suitable for use by producers, distributors, and consumers of food products. Developments have focused on providing a convenient way to interface with food samples, facilitate signal interpretation, and encapsulate or immobilize PDA sensors into or onto materials for storage and mobility. Here we discuss typical PDA strategies for food applications including solution-based tests, hydrogels, and films, before discussing some more innovative developments including inks, sampling devices, and other notable inventions.
4.1 Solution-based tests
Solution-based tests are the most prevalent form of PDA assay. In these tests, PDA vesicles are suspended in an aqueous solution, and a liquid or solid sample is added under controlled experimental conditions. The tests are typically conducted in 96 well plates for easy colorimetric analysis with UV-vis spectroscopy. Recent food-related examples include tests for pathogens,51 antibiotics,52 pesticides,54 and free fatty acids.46 PDA vesicle solutions are the product of the thin film hydration method or the solvent injection method and require no secondary processing in contrast to films and gels. Furthermore, solution-based tests facilitate fast mixing with samples and suitable conditions for detection mechanisms that rely on aqueous chemistry. However, solution-based tests can be impractical for out-of-laboratory applications. Suspensions of carboxy-terminated PDA vesicles are stabilized by steric forces from the partial disassociation of the carboxyl group. The introduction of recognition elements by insertion or conjugation into the PDA membrane can change the steric forces on the vesicle surface and threaten the suspension stability. Kang et al. investigated the impact of the insertion of charged phospholipids on PDA vesicle stability using dynamic light scattering, electron microscopy, and zeta potential measurements at different times during 7 days of storage.66 The insertion of phospholipids with strong head group charges improved the suspension stability, while neutrally charged phospholipids led to vesicle aggregation after just 4 hours. Nelson et al. optimized the surface charge of PDA vesicles by tuning the ratio of PCDA and amine-functionalized PCDA to control their stability at pH 5–9.67 This further demonstrates the impact of additives on surface charge and the ability to control and tune this phenomenon. The depth of literature relating to solution-based tests highlights their utility and convenience. Aqueous PDA vesicles are ideal transducers for systems in which the recognition mechanism relies on aqueous chemistry. The literature highlights the need to consider the impact of recognition elements on surface charge and how that may affect suspension stability and its interaction with the food sample.
4.2 Hydrogels
To overcome challenges related to solution-based tests, PDA vesicles have been combined with polymers to form hydrogels. Fabrication processes are versatile, typically involving the mixing of PDA vesicles with polymers before gelation under refrigeration or a crosslinking agent. Gels can be conveniently submerged directly into food samples or incorporated into devices suitable for out-of-laboratory applications. Gels encapsulate and immobilize the vesicles preventing suspension aggregation while maintaining a pseudo-aqueous environment necessary for many detection mechanisms. Gels can also be used to filter components of food mixtures that may inhibit the function of the sensors. To prevent vesicle aggregation, Wang et al. recently developed PDA vesicles that were encapsulated in alginate hydrogel beads using a microfluidic droplet-based method.61 The gel microbeads successfully prevented vesicle aggregation and increased the storage time to two months at 4 °C. In a different strategy, Meir et al. coated a glass-supported Langmuir–Schaeffer PDA/phospholipid film with an agarose hydrogel containing Luria-Bertani growth medium.68 The hydrogel filters constituents of blood that induce false positives in the sensor and provide nutrients to promote bacterial growth to facilitate pathogen detection. Using the device, the authors demonstrated the detection of Klebsiella pneumoniae in blood and meat samples. Hydrogel-based tests typically take longer than solution-based tests as sufficient time is required for the sample to diffuse through the hydrogel materials. In our recent work to monitor milk freshness via lactic acid detection with PDA/ZnO vesicles embedded within a hydrogel, 2 hours was required for the milk to completely diffuse through the gel and give a homogenous colorimetric signal.45 In comparison, the colorimetric response of the PDA/ZnO vesicles to lactic acid was instantaneous in solution. However, the hydrogel test speed could be improved by reducing the gel size and increasing the porosity. The inherent turbidity of many hydrogels can also limit the use of spectroscopy for quantification of PDA colorimetric signals. However, alternative techniques for colorimetric signal analysis are emerging. We recently developed a PDA-based hydrogel that could not be analyzed with spectroscopy due to its opacity. To describe the color change of the gels, we developed custom digital colorimetric analysis software to quantify the extent of color change by image processing and RGB analysis of photographs of the gel.47 Similarly, Kolusheva et al. encapsulated a variety of PDA/lipid vesicles in silica gels to make an arrayed test for disease diagnostics in blood plasma.69 They applied image analysis techniques in combination with machine learning algorithms to successfully discriminate between healthy and diseased samples by the colorimetric fingerprint of the assay.
4.3 Free-standing films
PDA polymer films are a common architecture for vesicular and non-vesicular PDA sensors.21,36 Vesicular PDA solutions can be combined with soluble polymers to fabricate films by a solution casting method.40,41,48,70 Alternatively, non-vesicular PDA films can be fabricated using forcespinning43 or electrospinning35,71 techniques. Polymer films have superior mechanical properties than gels for inclusion in food packaging. Due to the low water capacity of many polymer films, the efficacy of recognition elements that rely on aqueous chemistry can be limited. As a result, gaseous analytes are most commonly targeted. For example, PDA/chitosan films have been developed for the detection of ammonia gas and ethylene gas to monitor meat spoilage41 and fruit ripening,48 respectively. These materials successfully formed a mechanically strong, yet sensitive and flexible film stable between room temperature and freezer temperatures (−20 °C). Similarly, Park et al. developed a PDA/PVA hybrid film to detect ammonia gas released from beef spoilage via the interaction with PDA's carboxylic acid head groups.40
4.4 Other materials & notable developments
A range of other PDA materials and devices have been developed and here we discuss some of the most innovative and useful for food-oriented applications. Inkjet printing provides an easy, fast, and cost-effective way to fabricate substrate-based sensors using PDA ink. Park et al. printed hydrochromic images on paper using PDA inks via inkjet printing.72 To create an ink solution that is compatible with an inkjet printer and to avoid clogging of the nozzle vicinity of the inkjet head, imidazolium-containing DA monomers were dissolved in water and further diluted with 20% (v/v) ethanol. The printed images were polymerized with UV irradiation (254 nm) producing blue PDA that turned red with water contact at temperatures above 20 °C. Wu et al. described the fabrication of a reversible thermochromic sensor via inkjet printing using PDA/ZnO composites.73,74 They created a PDA-based QR code sensor with the PDA/ZnO ink, which could be used to store information and at the same time to monitor temperature. Due to its versatility, PDA ink can also be readily inkjet printed on other flexible substrates, such as photopaper and polyethylene terephthalate (PET) plastic film (Fig. 10).75
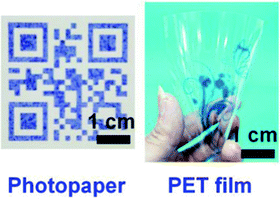 |
| Fig. 10 PDA materials produced via inkjet printing using PDA ink on photopaper (left) and polyethylene terephthalate (PET) plastic film (right). Reproduced from ref. 75 with permission from John Wiley & Sons, copyright 2019. | |
In an interesting application, Seo et al. brushed imidazole-modified PCDA (PCDA-Im) dissolved in chloroform directly onto plant leaves to study stomata.76 Stomata are the pores in plant leaves that release water in the process of perspiration and play a fundamental role in plant ecology. After evaporation of the chloroform, the PCDA-Im was photopolymerized and acted as a fluorescent probe capable of detecting the release of water vapor from individual stomata (Fig. 11). This allowed monitoring the activity of stomata to gain insight into the heterogeneity of their activity and their response to environmental stimuli such as light, humidity, wind, and temperature. The authors have also developed a variation of this sensor for human sweat pore mapping.77
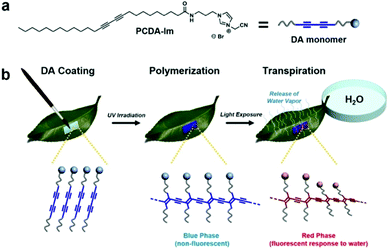 |
| Fig. 11 (a) Structure of PCDA-Im monomer used for coating. (b) Procedure for coating PCDA-Im monomers onto the under-side (abaxial surface) of a leaf followed by UV polymerization to afford the non-fluorescent blue-phase PDA and subsequent exposure of the petiole to water to permit transpiration. Conductance of water vapor at the site of active stomata causes a localized red-phase response in the PDA sensor coating. Reproduced from ref. 76 with permission from Springer Nature, copyright 2016. | |
Kim et al. recently developed an innovative device that can non-destructively sample packaged food and deliver it to a PDA-based sensor to monitor food quality.50 The device incorporates silk-based porous microneedles to transport fluid from the food product to a printed PDA bioink sensor via capillary action. The PDA bioink was prepared from PCDA/DMPC vesicles conjugated with E. coli targeting antibody. Silk fibroin was used to stabilize and preserve the PDA-antibody vesicles. The microneedle block was designed to be strong enough to penetrate commercial polyvinylidene chloride (PVDC) films as well as the food product (fish) (Fig. 12). The sensors were applied to spoiled fish fillets wrapped in the food film, showing a color change within 4 hours (the time it took for the internal fluid of the fish to be transported from the flesh and across the packaging to the microneedle/PDA sensors). For the detection of other pathogens, e.g. Salmonella, another PDA-antibody bioink may be designed by changing the antibody used for detection.
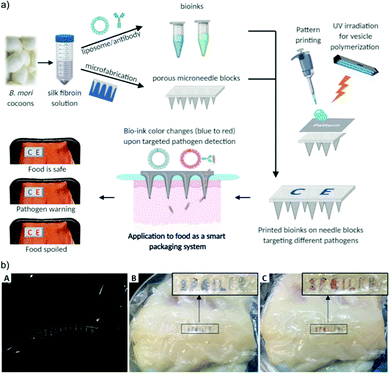 |
| Fig. 12 (a) Schematic illustration of the colorimetric food quality monitoring system using silk microneedle arrays with PDA-antibody vesicle bioinks. (b) A silk microneedle array penetrating a commercial food wrap (A) and its application to detect spoiled fish fillet at (B) 0 h and (C) 4 h. Reproduced from ref. 50 with permission from John Wiley & Sons, copyright 2021. | |
We recently developed a hydrogel encapsulating milk and PDA/DMPC vesicles to make a quality monitoring tag for dairy products.47 Instead of sampling the milk or submerging the sensor, encapsulation of milk in the gel removes the need for a direct interface with the product. This protects the integrity of the product while allowing the tag to be conveniently placed on the outside of the packaging for signal reading. We used PDA/DMPC vesicles to monitor the milk quality by the detection of FFAs produced from the rancidification of triglycerides. We demonstrated that milk encapsulated in the hydrogel spoiled with similar kinetics to bottled milk. Upon spoilage of the encapsulated milk, FFAs inserted into the membrane of the PDA vesicle inducing a blue to red color change to indicate milk quality. We demonstrated a method of “switching” the quality tags on and off by dehydration (off) and rehydration (on). Similar to dried food, dehydration of the tag prevents spoilage prohibiting bacterial proliferation and rehydration allows spoilage to continue. This removes the need for in situ manufacturing and means the tags could be stored and switched on when appropriate.
5. Challenges and opportunities
The application of PDA sensors as food management tools is a promising yet challenging prospect. The depth and variety of academic research highlights the versatility and utility of PDA for a variety of food products across production, distribution, and consumption. Despite this body of work, few developments have been successfully translated into commercial food management tools. Drawing insights from our review of the literature, we will discuss some of the limitations and challenges of PDA sensors in food applications before highlighting opportunities and promising areas of research.
Traditional micro-batch fabrication of PDA vesicles via the thin film hydration method has been excellent for laboratory research, however it is limited in scale and susceptible to batch variation, making it inappropriate for commercial applications. The solvent injection method can be more easily scaled and could be adapted for continuous flow production which exhibits better product consistency and easier incorporation into product assembly processes.78 Recent work has demonstrated control of PDA particle size and morphology by tuning solvent injection process variables, which eliminates the need for further particle processing to control sizes such as sonication and extrusion.24,29 Considering the relatively high phase transition temperature25 of common carboxy-terminated DAs, this greatly reduces the process complexity, energy intensity, and cost. Furthermore, concerns over vesicle suspension stability can be avoided by immediate secondary processing into materials. To further address challenges related to PDA vesicle suspension stability, the solvent injection method could be applied as a distributed manufacturing method79 to produce vesicles in situ or on devices just prior to use to avoid time dependent aggregation. Technology for on-demand PDA sensor production could feasibly be incorporated into robotics or low-resource settings where suitable storage conditions for vesicle solutions are not available. Due to the utility of PDA and the ease of chemical functionalization and conjugation of different recognition elements, tests could be designed specifically to criteria of the immediate demand. These technologies would help to improve the flexibility of biotechnology supply chains and contribute to more effective and efficient use of resources. However, further work is required to develop standards and methods to verify the quality and safety of distributed biotechnology.
PDA sensors have been developed to detect pathogens, toxins, biogenic amines, gases, heavy metals, antibiotics, and a range of other chemical and biological analytes. In conjunction with this, PDA is chromatically sensitive to environmental factors such as temperature, pressure, pH, and light. This means PDA is an incredibly versatile transducer, however as many analytes interact directly with the PDA transducer, the impact on sensor specificity must be considered. For example, PDA/phospholipid vesicles have been developed for bacteria, toxins, FFAs, and enzymes. Phospholipids are also included in PDA vesicles as a route to conjugate recognition elements such as antibodies and aptamers. Although many sensors rely on specific mixtures of PDA/phospholipids, many of these sensors would be receptive to multiple analytes. Without careful consideration of the food samples in which the sensor is being deployed, the opportunity for false positive signals is significant. PDA sensors deployed in food packaging materials are also likely to encounter sensor drift as a result of light, temperature, and exposure to mechanical forces. Further developments in materials and sampling devices could help to alleviate these concerns. Recent developments have demonstrated control of thermochromism by material additives. Materials have also been used for in situ sample processing by only allowing specific constituents of food samples to interact with the sensor.50 However, due to the complexity of food samples and variation in chemical decomposition under different conditions, the multi receptive nature of some PDA sensors could be leveraged to detect a variety of chemical changes in food.
The biocompatibility of PDA has been investigated and verified due to interest in its use as a drug delivery platform and use in tissue scaffolding.12,13 Recent work has demonstrated that PDAs with a higher degree of polymerization exhibit lower toxicity than partially polymerized PDA due to higher toxicity of DA monomers.80,81 However, there have been no specific studies on the impact of contamination or addition of PDA in food. Recent investigations have focused on the toxicology of non-organic nanoparticles. Further studies are necessary to verify the safety of PDA in food products and fully elucidate its toxicity.
Food production is heavily subsidized and as a result, the price of many food products do not represent their cost relative to their environmental impact and resource intensity. This is a contributing factor to poor consumer attitudes toward food waste and limits the incorporation of sensors into food products. As low-cost sensors, PDAs are well positioned to lead the incorporation of sensors into food products. To encourage their adoption, targeting products of high value and resource intensity such as meat is a sensible strategy. Highly processed products that do not exhibit natural and intuitive indicators of spoilage is also a promising area of application. PDA sensors could also play a role in verifying premium quality products and anti-counterfeiting applications. We hope that the introduction of PDA sensors into food products will help to heighten awareness of the significance of food waste and help to positively influence consumer attitudes towards food management. Convincing government and industry of the potential impact of PDA sensors to reduce waste could be key to their widespread adoption.
6. Conclusions
In conclusion, we have provided a structured review of PDA sensors for food applications, including fabrication techniques, applications by food products, materials and sampling devices, and challenges and opportunities for the translation of these sensors into food management tools. A review of fabrication methods illustrates a diverse collection of laboratory scale micro-batch fabrication strategies for vesicles, films, and fibers. The need for scalable techniques suitable for industrial application is evident and has become a focus of recent investigations. We found a variety of PDA sensors have been developed for quality monitoring of meat, milk, fruits, and beverages. These sensors are designed by considering changes in the endogenous chemistry of the food products. Sensors typically target the by-products of prominent constituents that are characteristic of the food, such as proteins (e.g. meat) and sugars (e.g. milk). Contaminant detection systems require greater specificity and as a result typically include recognition elements such as aptamers and antibodies. These have been combined with PDAs to detect pathogens, antibiotics, and pesticides. Recent PDA materials and food sensing devices include solution-based tests, hydrogels, films, and others. Solution-based tests can be limited by vesicle suspension stability and lack of mobility which has led to their incorporation into other materials such as hydrogels, films, fibers, and devices. Recent works of note have demonstrated the use of materials and devices to overcome inherent challenges in operating sensors in complex and heterogenous food samples. To realize the potential of PDA sensors for food applications, further research should focus on fabrication methods, sensor specificity, PDA toxicity, and targeting food products of high value.
Conflicts of interest
There are no conflicts to declare.
Acknowledgements
Y. G. acknowledges the support from the Australian Government Research Training Program Scholarship. A. D. T acknowledges the support from the University of New South Wales Scientia PhD Scholarship. R. C. acknowledges support from the National Health and Medical Research Council Emerging Leadership Investigator Grant (NHMRC APP1173428) and the UNSW Scientia Fellowship.
Notes and references
-
J. Gustavsson, C. Cederberg, U. Sonesson, R. Otterdijk and A. McYbeck, Global Food Losses and Food Waste: Extent, Causes and Prevention, FAO, Rome, 2011 Search PubMed.
- P. Alexander, C. Brown, A. Arneth, J. Finnigan, D. Moran and M. D. A. Rounsevell, Agric. Syst., 2017, 153, 190–200 CrossRef.
- Food and Agriculture Organization of the United Nations, The State of Food and Agriculture, 2019 Search PubMed.
- M. Weston, S. Geng and R. Chandrawati, Adv. Mater. Technol., 2021, 6, 2001242 CrossRef CAS.
- H. Yousefi, H.-M. Su, S. M. Imani, K. Alkhaldi, C. D. M. Filipe and T. F. Didar, ACS Sens., 2019, 4, 808–821 CrossRef CAS.
- S. Okada, S. Peng, W. Spevak and D. Charych, Acc. Chem. Res., 1998, 31, 229–239 CrossRef CAS.
- J. T. Wen, J. M. Roper and H. Tsutsui, Ind. Eng. Chem. Res., 2018, 57, 9037–9053 CrossRef CAS.
- A. D. Tjandra, A.-H. Pham and R. Chandrawati, Chem. Mater., 2022, 34, 2853–2876 CrossRef CAS.
- X. Qian and B. Städler, Chem. Mater., 2019, 31, 1196–1222 CrossRef CAS.
- X. Chen, G. Zhou, X. Peng and J. Yoon, Chem. Soc. Rev., 2012, 41, 4610–4630 RSC.
- M. A. Reppy and B. A. Pindzola, Chem. Commun., 2007, 4317–4338 RSC.
- F. Fang, F. Meng and L. Luo, Mater. Chem. Front., 2020, 4, 1089–1104 RSC.
- Y.-J. Hao and G.-M. Zhu, Chin. J. Anal. Chem., 2020, 48, 164–173 CAS.
- E. Cho and S. Jung, Molecules, 2018, 23, 107 CrossRef.
-
M. Weston, F. Mazur and R. Chandrawati, in Smart Sensors for Environmental and Medical Applications, John Wiley and Sons, Hoboken, NJ, 2020, pp. 81–102 Search PubMed.
- H. Wang, L. Han, W. Gong and R. Chu, E3S Web Conf., 2021, 261, 02090 CrossRef CAS.
- M. Weston, A. D. Tjandra and R. Chandrawati, Polym. Chem., 2020, 11, 166–183 RSC.
- C. Kim, C. Hong and K. Lee, Biosens. Bioelectron., 2021, 181, 113120 CrossRef CAS.
- M. Weston, M. Ciftci, R. P. Kuchel, C. Boyer and R. Chandrawati, ACS Appl. Polym. Mater., 2020, 2, 5238–5248 CrossRef CAS.
- E. Lebegue, C. Farre, C. Jose, J. Saulnier, F. Lagarde, Y. Chevalier, C. Chaix and N. Jaffrezic-Renault, Sensors, 2018, 18, 599 CrossRef.
- R. Carpick, D. Sasaki, M. Marcus, M. Eriksson and A. Burns, J. Phys.: Condens. Matter, 2004, 16, R679 CrossRef CAS.
- J. T. Wen, P. Viravathana, B. Ingel, C. Roper and H. Tsutsui, SLAS Technol., 2017, 22, 406–412 CrossRef.
- J. P. Yapor, A. Alharby, C. Gentry-Weeks, M. M. Reynolds, A. K. M. M. Alam and Y. V. Li, ACS Omega, 2017, 2, 7334–7342 CrossRef CAS.
- A. Tjandra, M. Weston, J. Tang, R. Kuchel and R. Chandrawati, Colloids Surf., A, 2021, 619, 126497 CrossRef CAS.
- C. Khanantong, N. Charoenthai, T. Phuangkaew, F. Kielar, N. Traiphol and R. Traiphol, Colloids Surf., A, 2018, 553, 337–348 CrossRef CAS.
- E. Elizondo, E. Moreno, I. Cabrera, A. Cordoba, S. Sala, J. Veciana and N. Ventosa, Prog. Mol. Biol. Transl. Sci., 2011, 104, 1–52 CrossRef CAS.
- A. D. Bangham, M. M. Standish and J. C. Watkins, J. Mol. Biol., 1965, 13, 238–252 CrossRef CAS PubMed.
- S. Batzri and E. D. Korn, Biochim. Biophys. Acta, Biomembr., 1973, 298, 1015–1019 CrossRef CAS.
- J. Tang, M. Weston, R. P. Kuchel, F. Lisi, K. Liang and R. Chandrawati, Mater. Adv., 2020, 1, 1745–1752 RSC.
- S. Jeon, B. Yoon and J.-M. Kim, Bull. Korean Chem. Soc., 2015, 36, 1949–1950 CrossRef CAS.
- J. H. Kwon, J. E. Song, B. Yoon, J. M. Kim and E. C. Cho, Bull. Korean Chem. Soc., 2014, 35, 1809–1816 CrossRef CAS.
- A. Chanakul, R. Saymung, S. Seetha, R. Traiphol and N. Traiphol, Colloids Surf., A, 2021, 615, 126241 CrossRef CAS.
- R. Saymung, N. Traiphol and R. Traiphol, Colloids Surf., A, 2021, 626, 127046 CrossRef CAS.
- J. H. Park, H. Choi, C. Cui and D. J. Ahn, ACS Omega, 2017, 2, 7444–7450 CrossRef CAS.
- S. K. Chae, H. Park, J. Yoon, C. H. Lee, D. J. Ahn and J. M. Kim, Adv. Mater., 2007, 19, 521–524 CrossRef CAS.
- B. Yoon, S. Lee and J. M. Kim, Chem. Soc. Rev., 2009, 38, 1958–1968 RSC.
- A. I. Danchuk, N. S. Komova, S. N. Mobarez, S. Y. Doronin, N. A. Burmistrova, A. V. Markin and A. Duerkop, Anal. Bioanal. Chem., 2020, 412, 4023–4036 CrossRef CAS PubMed.
- D. Doeun, M. Davaatseren and M.-S. Chung, Food Sci. Biotechnol., 2017, 26, 1463–1474 CrossRef CAS PubMed.
- G. Spano, P. Russo, A. Lonvaud-Funel, P. Lucas, H. Alexandre, C. Grandvalet, E. Coton, M. Coton, L. Barnavon, B. Bach, F. Rattray, A. Bunte, C. Magni, V. Ladero, M. Alvarez, M. Fernández, P. Lopez, P. F. de Palencia, A. Corbi, H. Trip and J. S. Lolkema, Eur. J. Clin. Nutr., 2010, 64, S95–S100 CrossRef CAS.
- S. Park, G. S. Lee, C. Cui and D. J. Ahn, Macromol. Res., 2016, 24, 380–384 CrossRef CAS.
- L. H. Nguyen, S. Naficy, R. McConchie, F. Dehghani and R. Chandrawati, J. Mater. Chem. C, 2019, 7, 1919–1926 RSC.
- Q. Li, S. Ren, Y. Peng, Y. Lv, W. Wang, Z. Wang and Z. Gao, Anal. Chem., 2020, 92, 1611–1617 CrossRef CAS PubMed.
- M. Valdez, S. K. Gupta, K. Lozano and Y. Mao, Sens. Actuators, B, 2019, 297, 126734 CrossRef CAS.
- C. P. d. Oliveira, N. d. F. F. Soares, E. A. F. Fontes, T. V. d. Oliveira and A. M. M. Filho, Food Chem., 2012, 135, 1052–1056 CrossRef PubMed.
- M. Weston, R. P. Kuchel, M. Ciftci, C. Boyer and R. Chandrawati, J. Colloid Interface Sci., 2020, 572, 31–38 CrossRef CAS.
- M. Weston, R. P. Kuchel and R. Chandrawati, Macromol. Rapid Commun., 2020, 41, 2000172 CrossRef CAS.
- M. Weston, R. P. Kuchel and R. Chandrawati, Anal. Chim. Acta, 2021, 1148, 238190 CrossRef CAS PubMed.
- L. H. Nguyen, F. Oveissi, R. Chandrawati, F. Dehghani and S. Naficy, ACS Sens., 2020, 5, 1921–1928 CrossRef CAS PubMed.
- X. Qian, C. L. Gargalo, K. V. Gernaey and B. Städler, ACS Appl. Nano Mater., 2020, 3, 3439–3448 CrossRef CAS.
- D. Kim, Y. Cao, D. Mariappan, M. S. Bono Jr., A. J. Hart and B. Marelli, Adv. Funct. Mater., 2021, 31, 2005370 CrossRef CAS.
- T. V. de Oliveira, N. d. F. F. Soares, N. J. de Andrade, D. J. Silva, E. A. A. Medeiros and A. T. Badaró, Food Chem., 2015, 172, 428–432 CrossRef CAS.
- D. H. Kang, H.-S. Jung, N. Ahn, J. Lee, S. Seo, K.-Y. Suh, J. Kim and K. Kim, Chem. Commun., 2012, 48, 5313–5315 RSC.
- D. H. Kang, K. Kim, Y. Son, P.-S. Chang, J. Kim and H.-S. Jung, Analyst, 2018, 143, 4623–4629 RSC.
- R. Pimsen, A. Khumsri, S. Wacharasindhu, G. Tumcharern and M. Sukwattanasinitt, Biosens. Bioelectron., 2014, 62, 8–12 CrossRef CAS PubMed.
- A. Alam, D. Jenks, G. A. Kraus and C. Xiang, Nanomaterials, 2021, 11, 1869 CrossRef CAS.
- Y. Zhang, L. Bromberg, Z. Lin, P. Brown, T. Van Voorhis and T. A. Hatton, J. Colloid Interface Sci., 2018, 528, 27–35 CrossRef CAS PubMed.
- S. Mukherjee, S. Bhattacharyya, K. Ghosh, S. Pal, A. Halder, M. Naseri, M. Mohammadniaei, S. Sarkar, A. Ghosh, Y. Sun and N. Bhattacharyya, Trends Food Sci. Technol., 2021, 109, 674–689 CrossRef CAS.
- T. C. Pham, Y. K. Kim, J. B. Park, S. Jeon, J. Ahn, Y. Yim, J. Yoon and S. Lee, ChemPhotoChem, 2019, 3, 1133–1137 CrossRef CAS.
- T. C. Pham, S. Lee, D. Kim, O.-S. Jung, M. W. Lee and S. Lee, ACS Omega, 2020, 5, 31254–31261 CrossRef CAS.
- Y. K. Kim, T. C. Pham, J. Kim, C. Bae, Y. Choi, M. H. Jo and S. Lee, Bull. Korean Chem. Soc., 2021, 42, 265–269 CrossRef CAS.
- D.-E. Wang, Y. Wang, C. Tian, L. Zhang, X. Han, Q. Tu, M. Yuan, S. Chen and J. Wang, J. Mater. Chem. A, 2015, 3, 21690–21698 RSC.
- D. H. Kang, H.-S. Jung, N. Ahn, S. M. Yang, S. Seo, K.-Y. Suh, P.-S. Chang, N. L. Jeon, J. Kim and K. Kim, ACS Appl. Mater. Interfaces, 2014, 6, 10631–10637 CrossRef CAS PubMed.
- M. Wang, F. Wang, Y. Wang, W. Zhang and X. Chen, Dyes Pigm., 2015, 120, 307–313 CrossRef CAS.
- Y. Li, L. Wang, Y. Wen, B. Ding, G. Sun, T. Ke, J. Chen and J. Yu, J. Mater. Chem. A, 2015, 3, 9722–9730 RSC.
- Codex Alimentarius. Codex general standard for contaminants and toxins in food and feed – CODEX STAN 193-1995. Joint FAO/WHO, 2015, p. 59. Available: DOI: 10.13140/RG.2.1.4910.2560.
- D. H. Kang, H.-S. Jung, J. Lee, S. Seo, J. Kim, K. Kim and K.-Y. Suh, Langmuir, 2012, 28, 7551–7556 CrossRef CAS PubMed.
- A. David Nelson, P. Shiveshwarkar, B. Lim, G. Rojas, I. Abure, A. Shrestha and J. Jaworski, Biosensors, 2020, 10, 132 CrossRef PubMed.
- D. Meir, L. Silbert, R. Volinsky, S. Kolusheva, I. Weiser and R. Jelinek, J. Appl. Microbiol., 2008, 104, 787–795 CrossRef CAS PubMed.
- S. Kolusheva, R. Yossef, A. Kugel, M. Katz, R. Volinsky, M. Welt, U. Hadad, V. Drory, M. Kliger, E. Rubin, A. Porgador and R. Jelinek, Anal. Chem., 2012, 84, 5925–5931 CrossRef CAS PubMed.
- P. F. R. Ortega, B. R. L. Galvão, P. S. C. de Oliveira, G. A. A. Bastos, M. R. F. Bernardes, R. L. Lavall and J. P. C. Trigueiro, Ind. Eng. Chem. Res., 2021, 60, 13243–13252 CrossRef CAS.
- N. Moazeni, M. Latifi, A. A. Merati and S. Rouhani, Soft Matter, 2017, 13, 8178–8187 RSC.
- D.-H. Park, W. Jeong, M. Seo, B. J. Park and J.-M. Kim, Adv. Funct. Mater., 2016, 26, 631 CrossRef.
- A. Wu, Y. Gu, C. Beck, Z. Iqbal and J. F. Federici, Sens. Actuators, B, 2014, 193, 10–18 CrossRef CAS.
- A. Wu, Y. Gu, C. Stavrou, H. Kazerani, J. F. Federici and Z. Iqbal, Sens. Actuators, B, 2014, 203, 320–326 CrossRef CAS.
- B. Hu, S. Sun, B. Wu and P. Wu, Small, 2019, 15, 1804975 CrossRef PubMed.
- M. Seo, D.-H. Park, C. W. Lee, J. Jaworski and J.-M. Kim, Sci. Rep., 2016, 6, 32394 CrossRef CAS PubMed.
- M. Seo, D.-H. Park, B. J. Park and J.-M. Kim, J. Appl. Polym. Sci., 2017, 134 Search PubMed.
- G. Jas and A. Kirschning, Chem. – Eur. J., 2003, 9, 5708–5723 CrossRef CAS.
- J. S. Srai, M. Kumar, G. Graham, W. Phillips, J. Tooze, S. Ford, P. Beecher, B. Raj, M. Gregory, M. K. Tiwari, B. Ravi, A. Neely, R. Shankar, F. Charnley and A. Tiwari, Int. J. Prod. Res., 2016, 54, 6917–6935 CrossRef.
- F. Costamagna, H. Hillaireau, J. Vergnaud, D. Clarisse, L. Jamgotchian, O. Loreau, S. Denis, E. Gravel, E. Doris and E. Fattal, Nanoscale, 2020, 12, 2452–2463 RSC.
- K. H. Park, M. Ku, N. Yoon, D. Y. Hwang, J. Lee, J. Yang and S. Seo, Nanotechnology, 2019, 30, 245101 CrossRef CAS PubMed.
|
This journal is © The Royal Society of Chemistry 2022 |