DOI:
10.1039/D1MO00298H
(Research Article)
Mol. Omics, 2022,
18, 71-82
Identification of novel biomarkers for intracerebral hemorrhage via long noncoding RNA-associated competing endogenous RNA network†
Received
6th August 2021
, Accepted 16th November 2021
First published on 17th November 2021
Abstract
Intracerebral hemorrhage (ICH) is a leading cause of death and disability worldwide. This study aimed to examine the involvement of long non-coding RNAs (lncRNAs), a group of non-coding transcripts, in ICH as potential biomarkers. An expression profile of patients with ICH using four contralateral grey matter controls (GM) and four contralateral white matter controls (WM) was downloaded from the Gene Expression Omnibus (GEO) database. Co-expressed lncRNAs and mRNAs were selected to create competing endogenous RNA (ceRNA) networks. Key lncRNAs were identified in ceRNA networks, which were validated through Real-time qPCR (RT-qPCR) with peripheral blood samples from patients with ICH. A total of 49 differentially expressed lncRNAs were discovered in different brain regions. The ceRNA network in GM included 9 lncRNAs, 40 mRNAs, and 20 microRNAs (miRNAs), while the one in WM covered 6 lncRNAs, 25 mRNAs, and 14 miRNAs. Six hub lncRNAs were observed and RT-qPCR results showed that LY86-AS1, DLX6-AS1, RRN3P2, and CRNDE were down-regulated, while HCP5 and MIAT were up-regulated in patients with ICH. Receiver Operating Characteristic (ROC) assessments demonstrated the diagnostic value of these lncRNAs. Our findings highlight the potential roles of lncRNA in ICH pathogenesis. Moreover, the hub lncRNAs discovered here might become novel biomarkers and promising targets for ICH drug development.
Introduction
Intracerebral hemorrhage (ICH) is the second most predominant subtype of stroke, which is a major threat to public health worldwide due to high morbidity and mortality.1 Bleeding in the brain can lead to serious damage, affecting several biological processes such as inflammation, apoptosis, and autophagy.2 The etiology of ICH is complicated and has not been fully elucidated. The ideal therapeutic strategy for ICH remains challenging.3 Patients with ICH or survivors frequently suffer from catastrophic disabilities due to disease and a heavy economic burden is imposed on families or society. Hence, the identification of novel biomarkers as diagnostic indicators and therapeutic targets is urgent for ICH management.
Long non-coding RNAs (LncRNAs) refer to a class of non-coding (ncRNA) transcripts with a size of more than 200 nucleotides. As an essential regulator, lncRNA enables the promotion or suppression of gene expression via methods including direct coupling with the target genes or recruiting transcription factors.4,5 Moreover, based on the competing endogenous RNA (ceRNA) hypothesis, lncRNA can serve as a “microRNA (miRNA) sponge” for modulating mRNA levels.6 Currently, it is garnering significant interest because of its indispensable role in various physiological and pathological processes. The brain exhibits an abundance of lncRNA, implying its potential ability to govern molecular cascades in the neurological system. Dysfunctional lncRNAs are involved in brain disorders, ranging from brain tumors to neurodegenerative disorders.7,8 Abnormal expression of lncRNAs has been demonstrated in two ICH rodent models,9,10 suggesting lncRNA's participation in ICH onset and development. Latest research reported that lncRNA Blnc1 knock-out could relieved nerve injury caused by ICH.11 Silencing lncRNA H19 would suppress ferroptosis observed in brain microvascular endothelial cells from ICH experimental model.12 LncRNAs MEG3 and Mtss1 were both linked to the inflammatory response after ICH occurrence.13,14 However, so far, the evidence as to the lncRNA-associated mechinsm is still limited and the comprehensive understanding of lncRNA's function in ICH remains elusive.
Here, with a combination of bioinformatics analysis and experimental validation, we aimed to prospectively provide a systematic dissection of the molecular mechanisms of ICH with respect to the role of lncRNA. We also intended to examine the feasibility of hub lncRNAs as biomarkers for diagnosis, and as promising drug targets of ICH.
Materials and methods
Raw data pre-processing and probe reannotation
Primitive data of GSE242653 were downloaded from the Gene Expression Omnibus (GEO) database (https://www.ncbi.nlm.nih.gov/gds/). It contains tissue samples corresponding to four perihematomal brain tissues (PH), four contralateral grey matter controls (GM), and four contralateral white matter controls (WM) obtained from patients with ICH. The platform of the dataset was “GPL570, [HG-U133_Plus_2] Affymetrix Human Genome U133 Plus 2.0 Array”. The mRNA and lncRNA were re-annotated using the Encyclopedia of DNA Elements (ENCODE) with ENSEMBL.15,16
Identification of and differentially expressed (DE) lncRNAs and DEmRNAs
After data normalization and pre-processing with the “Impute” package in “R”, “Limma” package of “R” language (Version X64 3.6.1) was used to detect DEmRNAs and DElncRNAs between PH and GM and PH and WM groups. The software calculated P-value and false discovery rate (FDR) by the student's t-test and the Benjamini–Hochberg method, respectively. |Log2FoldChange (FC)| > 1.0 and P-value < 0.05 were finally set as the cut-off criteria. Probes with no exact corresponding gene symbol were filtered. The results of DElncRNAs were visualized through the “Pheatmap” package.
LncRNA–mRNA co-expression network (CEN) construction
The Pearson correlation coefficient (PCC) was calculated to identify co-expression relationships between DElncRNAs and DEmRNAs. The lncRNA–mRNA pairs showing absolute values of more than 0.95 for PCC and those with P < 0.05, were selected to construct the CENs, which were visualized using the Cytoscape software (Version 3.7.1).
Functional assessments of mRNAs in the CENs
Genome Ontology (GO) enrichment and Kyoto Encyclopedia of Genes and Genomes (KEGG) pathway analyses were implemented using an online tool: Database for Annotation, Visualization and Integration Discovery (DAVID, https://david.ncifcrf.gov). The species was limited to Homo sapiens, and values with P < 0.05 were considered significant. The results were visualized using the “GGplot2” package of R.
Construction of LncRNA-associated ceRNA network
To further probe the regulatory role of lncRNAs, the interactions between DElncRNAs and miRNAs were predicted. The DElncRNAs in PH vs. GM and PH vs. WM groups were characterized for predicting targeted miRNAs using the mircode database (http://www.mircode.org/, approximately 770 lncRNA–miRNA pairs). The putative miRNA–mRNA interactions were identified using miRTarBase (Version 8.0, http://miRTarBase.cuhk.edu.cn/, approximately 322135 miRNA–mRNA pairs), miRDB (Version 6.0, http://www.mirdb.org/, approximately 938564 miRNA–mRNA pairs) and TargetScan (Version 7.2, http://www.targetscan.org/, approximately 1048576 miRNA–mRNA pairs). Genes identified in all three databases were intersected with the DEmRNAs positively correlated with DElncRNAs to recognize the final mRNA targets. The lncRNA–miRNA–mRNA triplet consisted of DElncRNA, predicted miRNA and final mRNA target. A regulatory network was then established and visualized via Cytoscape (Version 3.7.1).
Topological analysis of ceRNA network
The degree of each node in the ceRNA network was assessed using the “Centiscape2.2” plug-in of Cytoscape. The top 10 nodes with the highest node degree were extracted to create sub-networks and visualized using the “cytoHubba” plug-in. The lncRNA with a node degree > 5 was considered as a hub lncRNA. The information regarding the relationships of hub lncRNA–miRNAs–mRNAs was then collected to generate hub lncRNA-associated sub-networks.
Patients and blood samples
Six hub lncRNAs in the two ceRNA networks were further validated using Real-time qPCR (RT-qPCR). A total of 33 patients with ICH from the Department of Neurology at the First Affiliated Hospital of China Medical University and 15 control subjects were enrolled for this study. The inclusion criteria are described in our previous manuscript.17 The peripheral blood was collected for RNA isolation and subsequent analysis. This study was approved by the Ethics Committee of the First Affiliated Hospital of China Medical University, Shenyang, Liaoning Province, China (no. [2012]38-1). All participants provided written informed consent according to the Declaration of Helsinki (as revised in 2013).
RT-qPCR
Total RNA was extracted from the blood samples using TRIzol reagent (Thermo Fisher Scientific, USA, 15596026) according to the manufacturer's instructions. First-strand cDNA was synthesized using a Revert Aid First Strand cDNA Synthesis Kit (Jizhen biology, China, AORT-0060). Resultant cDNA was added as a template, and the amplification reactions were performed using AceQ qPCR SYBR Green Master Mix (Jizhen Biology, China, Q121-02) on Bio-Rad CXF96 PCR detection system (Bio-Rad, Hercules, CA, USA). GAPDH was employed as an endogenous control. The relative gene expression levels were calculated using the comparative cycle threshold (2−ΔΔCt) method. The primer sequences are listed in Table S1 (ESI†). Area under the curve (AUC) of the Receiver Operating Characteristic (ROC) was calculated via GraphPad Prism 7.0. P < 0.05 with the AUC > 0.80 were set as the cutoff standards.
Statistical analysis
For RT-qPCR, all values are presented as mean ± SEM. The student's t-test was used to evaluate the differences between the ICH and the control groups, and statistical significance was set at P < 0.05. All statistical analyses were conducted using SPSS for Windows version 16.0 and GraphPad Prism 7.0.
Results
Differentially expressed lncRNAs
Since the Affymetrix HG-U133 Plus 2.0 array consists of probe set-targeted lncRNA genes,15 we acquired DElncRNAs in patients with ICH via probe reannotation. Based on our cut-off criteria, a volcano diagram shown in Fig. 1a was constructed. There are a total of 1316 DEgenes in the PH compared to those in the GM, in which 477 up-regulated genes, including 11 lncRNAs, and 839 down-regulated genes, including 15 lncRNAs, were observed. Fig. 1b shows a total of 790 DE genes discovered in the PH compared to those in the WM, in which 391 up-regulated genes, including 4 lncRNAs, and 399 down-regulated genes, including 22 lncRNAs, were observed. The heatmaps shown in Fig. 1c and d display all DElncRNAs in PH vs. GM and PH vs. WM groups.
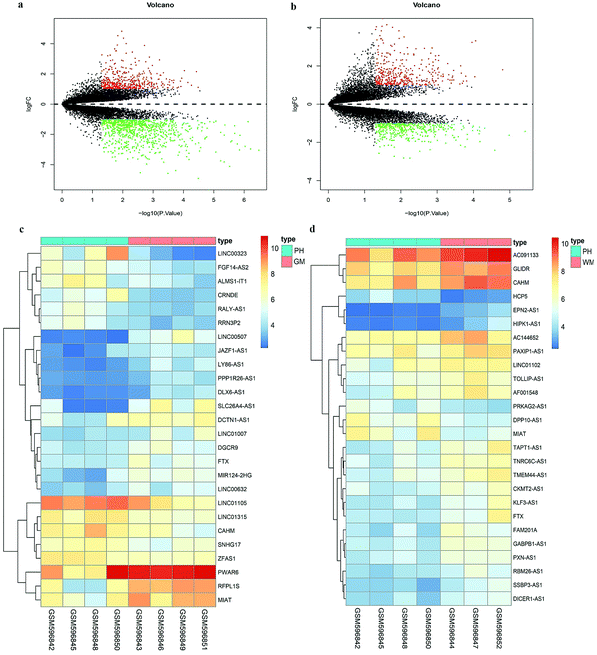 |
| Fig. 1 Differentially expressed genes in ICH. (a) The volcano plot of the differently expressed genes in PH vs. GM of GSE24265. (b) The volcano plot of the differently expressed genes in PH vs. WM of GSE24265. Black dots represent genes which are not altered. The red dots represent upregulated genes while the green dots represent downregulated ones. (c) Heatmap of differently expressed lncRNAs in PH vs. GM of GSE24265. (d) Heatmap of differently expressed lncRNAs in PH vs. WM of GSE24265. | |
Co-expression of DElncRNAs and DEmRNAs
To further explore the potential role of the DElncRNAs in ICH development, DElncRNA–DEmRNA networks were constructed based on correlation analysis.18,19 Only strong correlations with |PCC| with a value more than 0.95 along with a P-value of less than 0.05, were selected to construct the network. The GM-associated CEN included 24 lncRNAs and 635 mRNAs, exhibiting 1230 co-expression relationships, whereas the WM-associated CEN included 26 lncRNAs and 353 mRNAs, exhibiting 619 co-expression relationships. Fig. 2a and b. demonstrate the CENs in the GM and the WM, respectively.
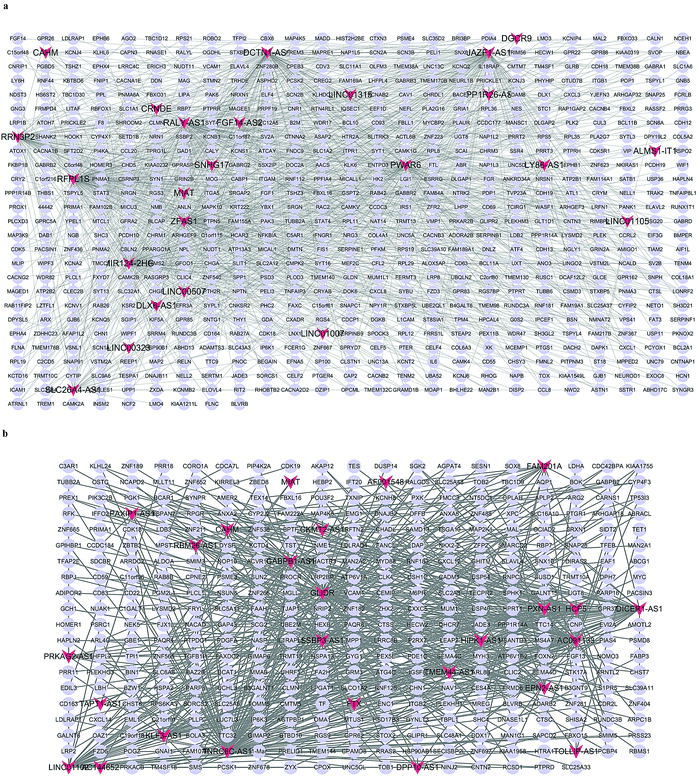 |
| Fig. 2 The co-expression networks. (a) Co-expression relationship between DElncRNAs and DEmRNAs in PH vs. GM. (b) Co-expression relationship between DElncRNAs and DEmRNAs in PH vs. WM. Red V shapes indicate lncRNAs and blue ellipses indicate mRNAs. Gray edges indicate lncRNA–mRNA interactions. | |
Functional annotation of mRNAs in CENs
To elucidate the molecular functions of the lncRNAs, GO enrichment and KEGG pathway analyses focusing on mRNA in CENs were employed. Complete lists of the outcomes are presented in Tables S2–S5 (ESI†). The bar plot shown in Fig. 3a and Table 1 demonstrates that the mRNAs in the GM-related CEN were associated with “chemical synaptic transmission”, “cell junction”, and “syntaxin-1 binding”. The KEGG results shown in Fig. 3b demonstrate the enrichment of these mRNAs primarily in “Axon guidance”. As shown in Fig. 3c and Table 2, “positive regulation of cell migration”, “microvillus”, and “cytoskeletal protein binding” were all involved in biological processes associated with the WM-related CEN mRNAs. Fig. 3d shows the enrichment of these mRNAs in the KEGG pathways, including “metabolic pathways”.
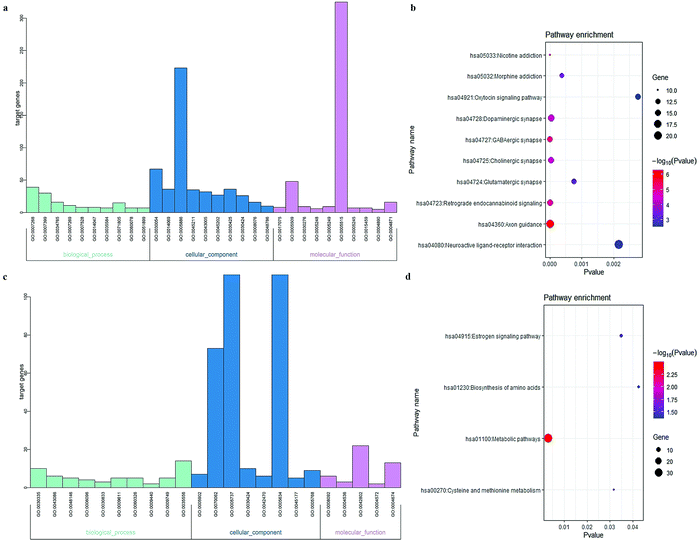 |
| Fig. 3 Functional analyses of the mRNA in CENs. Each category only presented top 10 terms (P < 0.05). (a) GO enrichment analysis of mRNA in GM-associated CEN. (b) KEGG pathway analysis of mRNA in GM-associated CEN. (c) GO enrichment analysis of mRNA in WM-associated CEN. (d) KEGG pathway analysis of mRNA in WM-associated CEN. | |
Table 1 Functional enrichments analysis of mRNAs in GM-related CEN
Functional enrichments |
Terms |
Description |
P-value |
GO_BP |
GO:0007268 |
Chemical synaptic transmission |
8.25 × 10−16 |
|
GO:0007399 |
Nervous system development |
1.03 × 10−7 |
|
GO:0034765 |
Regulation of ion transmembrane transport |
3.87 × 10−6 |
|
GO:0007269 |
Neurotransmitter secretion |
5.58 × 10−6 |
|
GO:0007528 |
Neuromuscular junction development |
2.75 × 10−5 |
|
GO:0014047 |
Glutamate secretion |
2.75 × 10−5 |
|
GO:0035584 |
Calcium-mediated signaling using intracellular calcium source |
3.38 × 10−5 |
|
GO:0071805 |
Potassium ion transmembrane transport |
4.82 × 10−5 |
|
GO:0060078 |
Regulation of postsynaptic membrane potential |
6.15 × 10−5 |
|
GO:0051899 |
Membrane depolarization |
1.34 × 10−4 |
GO_CC |
GO:0030054 |
Cell junction |
2.37 × 10−24 |
|
GO:0014069 |
Postsynaptic density |
2.87 × 10−17 |
|
GO:0005886 |
Plasma membrane |
7.11 × 10−16 |
|
GO:0045211 |
Postsynaptic membrane |
1.69 × 10−14 |
|
GO:0043005 |
Neuron projection |
6.59 × 10−11 |
|
GO:0045202 |
Synapse |
2.94 × 10−10 |
|
GO:0030425 |
Dendrite |
1.98 × 10−09 |
|
GO:0030424 |
Axon |
9.67 × 10−08 |
|
GO:0008076 |
Voltage-gated potassium channel complex |
1.78 × 10−07 |
|
GO:0048786 |
Presynaptic active zone |
2.48 × 10−7 |
GO_MF |
GO:0017075 |
Syntaxin-1 binding |
9.28 × 10−7 |
|
GO:0005509 |
Calcium ion binding |
5.42 × 10−6 |
|
GO:0030276 |
Clathrin binding |
2.73 × 10−4 |
|
GO:0005248 |
Voltage-gated sodium channel activity |
3.88 × 10−4 |
|
GO:0005249 |
Voltage-gated potassium channel activity |
5.85 × 10−4 |
|
GO:0005515 |
Protein binding |
0.001334181 |
|
GO:0005245 |
Voltage-gated calcium channel activity |
0.001822166 |
|
GO:0015459 |
Potassium channel regulator activity |
0.001822166 |
|
GO:0004890 |
GABA-A receptor activity |
0.003022365 |
|
GO:0004871 |
Signal transducer activity |
0.003121369 |
KEGG |
hsa04360 |
Axon guidance |
5.20 × 10−7 |
|
hsa05033 |
Nicotine addiction |
8.84 × 10−6 |
|
hsa04727 |
GABAergic synapse |
8.86 × 10−6 |
|
hsa04723 |
Retrograde endocannabinoid signaling |
1.29 × 10−5 |
|
hsa04725 |
Cholinergic synapse |
3.84 × 10−5 |
|
hsa04728 |
Dopaminergic synapse |
4.87 × 10−5 |
|
hsa05032 |
Morphine addiction |
3.81 × 10−4 |
|
hsa04724 |
Glutamatergic synapse |
7.51 × 10−4 |
|
hsa04080 |
Neuroactive ligand-receptor interaction |
0.002170521 |
|
hsa04921 |
Oxytocin signaling pathway |
0.002762355 |
Table 2 Functional enrichments analysis of mRNAs in WM-related CEN
Functional enrichments |
Terms |
Description |
P value |
GO_BP |
GO:0030335 |
Positive regulation of cell migration |
0.007315187 |
|
GO:0043086 |
Negative regulation of catalytic activity |
0.012517416 |
|
GO:0048146 |
Positive regulation of fibroblast proliferation |
0.017480309 |
|
GO:0006096 |
Glycolytic process |
0.024449498 |
|
GO:0030833 |
Regulation of actin filament polymerization |
0.026705051 |
|
GO:0009611 |
Response to wounding |
0.028977114 |
|
GO:0060326 |
Cell chemotaxis |
0.032017746 |
|
GO:0009440 |
Cyanate catabolic process |
0.036582608 |
|
GO:0009749 |
Response to glucose |
0.036916882 |
|
GO:0035556 |
Intracellular signal transduction |
0.037199119 |
GO_CC |
GO:0005902 |
Microvillus |
5.10 × 10−4 |
|
GO:0070062 |
Extracellular exosome |
7.01 × 10−4 |
|
GO:0005737 |
Cytoplasm |
0.015363395 |
|
GO:0030424 |
Axon |
0.018197632 |
|
GO:0042470 |
Melanosome |
0.034243687 |
|
GO:0005634 |
Nucleus |
0.041779378 |
|
GO:0045177 |
Apical part of cell |
0.04458957 |
|
GO:0005768 |
Endosome |
0.048244172 |
GO_MF |
GO:0008092 |
Cytoskeletal protein binding |
0.001713596 |
|
GO:0004536 |
Deoxyribonuclease activity |
0.013387921 |
|
GO:0042802 |
Identical protein binding |
0.032099848 |
|
GO:0004572 |
Mannosyl-oligosaccharide 1,3-1,6-alpha-mannosidase activity |
0.035926247 |
|
GO:0004674 |
Protein serine/threonine kinase activity |
0.042230075 |
KEGG |
hsa01100 |
Metabolic pathways |
0.003198261 |
|
hsa00270 |
Cysteine and methionine metabolism |
0.031772014 |
|
hsa04915 |
Estrogen signaling pathway |
0.034758077 |
|
hsa01230 |
Biosynthesis of amino acids |
0.042510774 |
Construction and topological analysis of ceRNA network
We next attempted to elucidate the potential regulatory mechanism of these lncRNAs in ICH We selected DEmRNAs that were positively correlated with DElncRNAs and constructed the lncRNA-associated ceRNA network. The Venn diagrams shown in Fig. 4a and d indicate the final number of mRNA targets for the networks. For “PH vs. GM”, the ceRNA network was composed of 9 lncRNAs, 40 mRNAs, 20 microRNAs (miRNAs), and 128 edges, shown in Fig. 4b and Table 1. For in “PH vs. WM”, the ceRNA network was composed of 6 lncRNAs, 25 mRNAs, 14 miRNAs, and 71 edges, shown in Fig. 4e and Table 3. We further assessed the topological features of each gene in the ceRNA network. As shown in Table 4, in the GM-related ceRNA network, the degree scores of five lncRNAs were over five, while WM-related ceRNA network showed two lncRNAs with degree scores of over five. Fig. 4c and f show the top 10 nodes with high degree scores in the two ceRNA networks. Interestingly, MIAT appeared in both lists of key nodes, implying its significance. Finally, Fig. 5a–g. display the hub lncRNA-associated subnetworks in GM and WM.
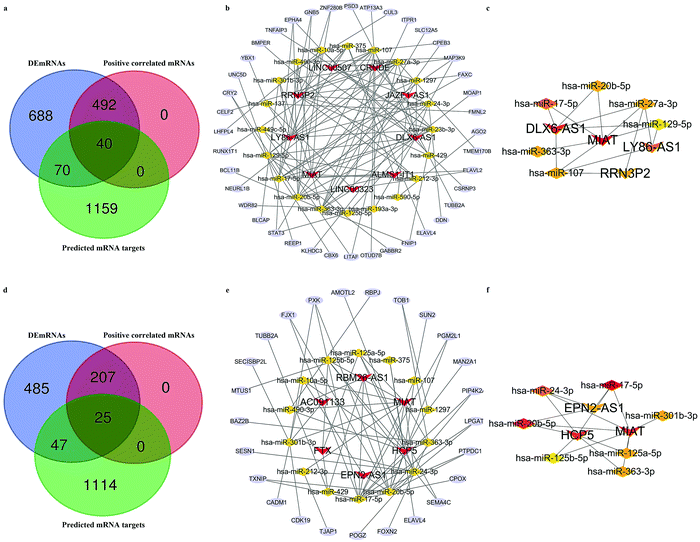 |
| Fig. 4 The lncRNA-associated ceRNA network. (a) Venn diagram of mRNA targets in GM-related ceRNA network. (b) GM-related ceRNA network. (c) Network of the top 10 hub nodes in GM. (d) Venn diagram of mRNA targets in WM-related ceRNA network. (e) WM-related ceRNA network. (f) Network of the top 10 hub nodes in WM; Red V shapes indicate lncRNAs. Yellow triangles indicate miRNAs. Blue ellipses indicate mRNAs. Gray edges indicate lncRNA–miRNA–mRNA interactions. Different colors represent the different degrees of nodes. | |
Table 3 The details of the ceRNA networks
|
GM-related ceRNA |
WM-related ceRNA |
lncRNAs |
RRN3P2, LY86-AS1, MIAT, LINC00323, ALMS1-IT1, DLX6-AS1, JAZF1-AS1, CRNDE, LINC00507 |
HCP5, MIAT, RBM26-AS1, AC091133, FTX, EPN2-AS1 |
miRNAs |
hsa-miR-10a-5p, hsa-miR-17-5p, hsa-miR-27a-3p, hsa-miR-590-5p, hsa-miR-137, hsa-miR-363-3p, hsa-miR-301b-3p, hsa-miR-107, hsa-miR-429, hsa-miR-20b-5p, hsa-miR-23b-3p, hsa-miR-212-3p, hsa-miR-129-5p, hsa-miR-490-3p, hsa-miR-193a-3p, hsa-miR-24-3p, hsa-miR-1297, hsa-miR-449c-5p, hsa-miR-125b-5p, hsa-miR-375 |
hsa-miR-375, hsa-miR-17-5p, hsa-miR-20b-5p, hsa-miR-22-3p, hsa-miR-23b-3p, hsa-miR-125b-5p, hsa-miR-107, hsa-miR-125a-5p, hsa-miR-27a-3p, hsa-miR-490-3p, hsa-miR-425-5p, hsa-miR-217, hsa-miR-301b-3p, hsa-miR-363-3p, hsa-miR-24-3p, hsa-miR-129-5p, hsa-miR-216b-5p, hsa-miR-507, hsa-miR-212-3p, hsa-miR-140-5p, hsa-miR-1297, hsa-miR-429, hsa-miR-590-5p, hsa-miR-876-3p, hsa-miR-10a-5p |
mRNAs |
CSRNP3, ELAVL2,TMEM170B, AGO2, FMNL2, MOAP1, FAXC, MAP3K9, CPEB3, SLC12A5, ITPR1, CUL3, ATP13A3, PSD3, ZNF280B, GNB5, EPHA4, TNFAIP3, BMPER, YBX1, UNC5D, CRY2, CELF2, LHFPL4, RUNX1T1, BCL11B, NEURL1B, WDR82, BLCAP, STAT3, REEP1, KLHDC3, CBX6, LITAF, OTUD7B, GABBR2, FNIP1, ELAVL4, DDN, TUBB2A |
ELAVL4, CPOX, ANKIB1, PTPDC1, ZNF532, LPGAT1, RUFY2, PIP4K2A, SP1, BRWD1, TMEM136, PGM2L1, SUN2, KMT2C, DDX5, RBPJ, BCOR, ERBB3, FOXO3, UBN2, KIAA1109, AMOTL2, DPYSL2, EXPH5, CEP97, ZNF566, GAB1, BACH2, FXR1, SMAD5, TRIM23, SEMA6A, PXK, NLN, SLC12A5, VEZF1, RNF11, AMOT, KCTD10, ATG14, HIP1, CKS2, TET2, H2AFX, KAT6B, TUBB2A, MBTD1, CBX4, N4BP1, PSPC1, MAP3K3, RBBP6, SECISBP2L, MTUS1, BAZ2B, PIM2, CNOT4, KLF13, AKAP1, CADM1, MAP3K12, MARCKSL1, CDK19, RBL2, DUSP10, TJAP1, GPATCH8, UBXN2A, POGZ, HOXA10, FOXN2 |
Table 4 The key genes in ceRNA networks (Degree > 5)
Network |
Gene name |
Gene type |
Degree |
GM-related ceRNA |
MIAT |
lncRNA |
14 |
|
DLX6-AS1 |
lncRNA |
13 |
|
LY86-AS1 |
lncRNA |
12 |
|
hsa-miR-17-5p |
miRNA |
12 |
|
RRN3P2 |
lncRNA |
10 |
|
hsa-miR-27a-3p |
miRNA |
10 |
|
hsa-miR-107 |
miRNA |
10 |
|
hsa-miR-20b-5p |
miRNA |
10 |
|
hsa-miR-363-3p |
miRNA |
10 |
|
hsa-miR-129-5p |
miRNA |
9 |
|
hsa-miR-23b-3p |
miRNA |
8 |
|
hsa-miR-24-3p |
miRNA |
8 |
|
hsa-miR-10a-5p |
miRNA |
7 |
|
hsa-miR-1297 |
miRNA |
6 |
|
CRNDE |
lncRNA |
6 |
WM-related ceRNA |
hsa-miR-17-5p |
miRNA |
12 |
|
HCP5 |
lncRNA |
11 |
|
hsa-miR-20b-5p |
miRNA |
11 |
|
MIAT |
lncRNA |
11 |
|
hsa-miR-24-3p |
miRNA |
7 |
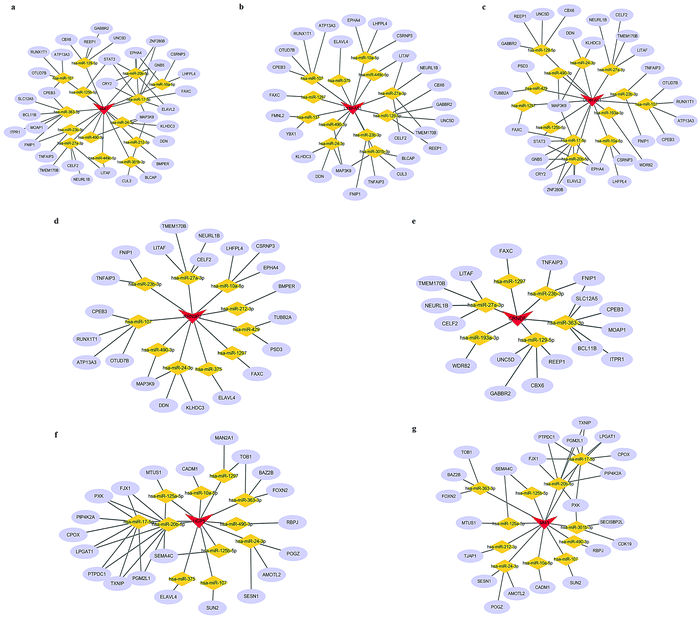 |
| Fig. 5 Sub-networks of hub lncRNA candidates. (a) MIAT-associated ceRNA network (in GM). (b) LY86-AS1-associated ceRNA network. (c) DLX6-AS1-associated ceRNA network. (d) RRN3P2-associated ceRNA network. (e) CRNDE-associated ceRNA network. (f) HCP5-associated ceRNA network. (g) MIAT-associated ceRNA network (in WM). Blue ellipses indicate mRNAs. Gray edges indicate lncRNA–miRNA–mRNA interactions. | |
Experimental validation of hub lncRNAs
Since one of our objectives was to determine key lncRNAs with diagnostic value, we further performed RT-qPCR for the aforementioned hub lncRNAs. Considering that MIAT appeared in two networks, six final candidates were considered. The results shown in Fig. 6. indicate that the expressions of LY86-AS1, DLX6-AS1, RRN3P2, and CRNDE were reduced remarkably in the ICH group, while the expressions of MIAT and HCP5 were enhanced. ROC results shown in Fig. 6. demonstrate that based on our criteria, MIAT (AUC = 0.9293, P < 0.0001), LY86-AS1 (AUC = 0.9010, P < 0.0001), DLX6-AS1 (AUC = 0.8162, P = 0.0005), RRN3P2 (AUC = 0.8505, P = 0.0001), CRNDE (AUC = 0.8909, P < 0.0001), and HCP5 (AUC = 0.8020, P = 0.0008) might serve as potential markers for discriminating patients with ICH from the healthy controls.
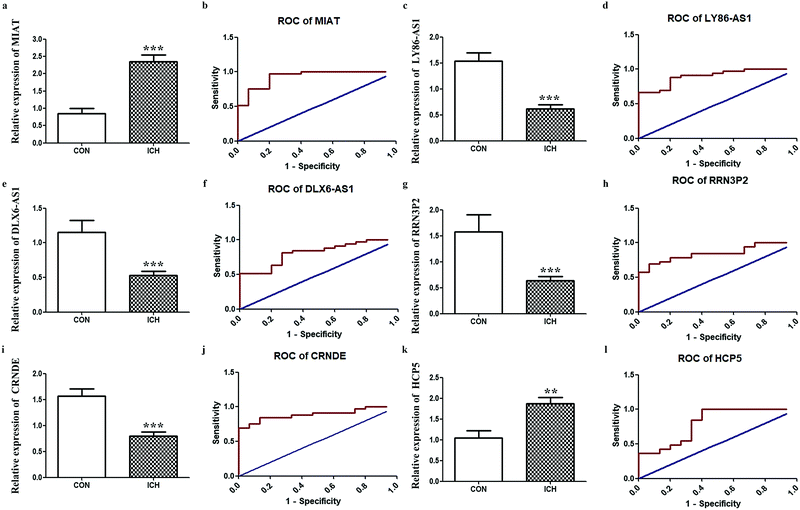 |
| Fig. 6 Validations of hub lncRNAs. RT-qPCR results of key lncRNA candidates in peripheral blood from patients with ICH compared to healthy people. (a) Relative expression of MIAT. (b) The ROC of MIAT. (c) Relative expression of LY86-AS1. (d) The ROC of LY86-AS1. (e) Relative expression of DLX6-AS. (f) The ROC of DLX6-AS1. (g) Relative expression of RRN3P2. (h) The ROC of RRN3P2. (i) Relative expression of CRNDE. (j) The ROC of CRNDE. (k) Relative expression of HCP5. (l) The ROC of HCP5 ** P < 0.01, *** P < 0.001. | |
Discussion
A set of lncRNAs could modulate brain development, and the perturbation of these molecules disturbs the cross-talk of miRNA with their mRNA targets, thereby leading to brain disorders, including Huntington's disease, Alzheimer's diseases, and ischemic stroke.20–23 Here, we primarily concentrated on lncRNAs in ICH and examined the following: (1) lncRNA-associated molecular cascades involved in ICH; (2) potential lncRNA–miRNA–mRNA interactions, and (3) hub lncRNAs that could be considered as biomarkers. We mined lncRNA data by re-annotating probes in a microarray expression profile, filtered DElncRNAs, and established ceRNA networks. To the best of our knowledge, this is the first report focusing on the lncRNA-associated ceRNA network in ICH.
Bleeding within the brain parenchyma will harm both GM and the proximal or distal WM. Owing to the distinct cellular architecture, the blood supply pattern and corresponding function of WM and its response to ICH may vary from that of GM.24,25 The discrepancy associated with DElncRNAs in the PH versus the GM and the WM observed here could be related to this phenomenon. According to our GO and KEGG analyses, the mRNAs in GM-related CEN were enriched in biological terms, including “chemical synaptic transmission”, “cell junction”, and “syntaxin-1 binding”. The pooled erythrocytes caused by bleeding in hemorrhagic stroke would lyse to produce hemoglobin, contributing a dose dependent suppression of synaptic transmission in hippocampal CA1 neurons.26 Interestingly, syntaxin-1 is a key component of the synaptic vesicle docking/fusion machinery.27 Given that vascular disruption is the underlying reason of ICH, the role of endothelial cell-to-cell junction on maintaining vascular homeostasis might be associated with ICH occurrence.28,29 However, the mRNAs in WM-related CEN were associated with different cellular processes, including “positive regulation of cell migration”, “microvillus”, and “cytoskeletal protein binding”. When ICH occurred, the neurogenesis in the subventricular zone and hippocampal subgranular zone would be triggered to generate new neuronal cells and migrate to damaged brain regions. This neurogenetic activity is usually accompanied with cytoskeletal protein remodeling and assembling.30,31 The occurrence of endothelial microvilli could be viewed as an indicator to assess capillary injury in ischemic stroke.32 KEGG analysis revealed that the mRNA in the GM-related CEN were enriched in biological categories regarding neuronal synapses involved in primary disease processes, including “Axon guidance” and “Dopaminergic synapse”, whereas the mRNAs in WM-related CEN were linked to the metabolic processes, including “metabolic pathways” and “cysteine and methionine metabolism”. As mentioned above, the different structures of the two regions of the brain might explain these results. The abundance of neuronal bodies and fibers, glial cells, and blood vessels in the GM possibly activated a series of molecular cascades, such as axon loss or repair, directly in the initial phase of ICH. The myelin sheaths are later destroyed due to blood volume, which leads to the development of WM lesions, affecting other cellular processes indirectly, such as usual metabolic and energy pathways.33 Accordingly, it is reasonable to speculate that the lncRNAs may be involved in many molecular cascades during different stages of ICH.
According to the ceRNA theory, lncRNA and mRNA could competitively interact with mutual miRNA, constituting a lncRNA–miRNA–mRNA association. Compared to the limited reports on lncRNA, many studies have demonstrated the pathophysiological role of miRNA in ICH. Based on our findings, 20 and 25 miRNAs were predicted to build the ceRNA network for the GM and the WM, respectively. Our previous work demonstrated that miR-27a-3p could protect against post-ICH complications in the capillary endothelial cells of the brain.34 Similarly, as regulators of inflammatory responses observed in the aftermath of ICH, miR-140-5p and miR-590-5p might relieve ICH-induced brain injury.35,36 An increase in miR-129-5p levels inhibited the HMGB1-RAGE axis, affecting revascularization in the rat models of ICH.37 Nevertheless, whether lncRNA participates in the aforementioned molecular processes and if yes, what its exact role definitely require further examination.
MIAT was highlighted in our topological estimation of both ceRNA networks. MIAT, namely myocardial infarction-associated transcript, was initially reported to be associated with myocardial infarction in the Japanese population.38 The main function of this lncRNA involves gene regulation at the post-transcriptional level, which is implemented via the ceRNA machinery.39 It is a critical regulator in neurological disorders, especially neurovascular illnesses. Accumulating evidence has illustrated its pathophysiological role in ischemic stroke. In line with our findings in ICH patients, enhanced MIAT expression was observed in peripheral blood leukocytes of ischemic stroke patients, which was positively correlated with infarct volume. Hence, patients with higher MIAT expression might have a relatively poor prognosis.40 In contrast, the neuroprotective ability of this lncRNA was confirmed by another group; overexpressed MIAT dampened neuronal apoptosis via miR-211/GDNF in a neonatal rat model of hypoxic-ischemic injury.41 The controversial conclusion reflects its complicated role under different stroke-like pathological conditions. Therefore, we hypothesize that MIAT might participate in ICH progression in various ways, and the ceRNA networks would be conducive to the advancement of our understanding of the underlying mechanisms.
The function of hub lncRNAs discovered here in ICH remains unclear, demonstrating a huge knowledge gap. However, several disorders are associated with these genes. DLX6-AS1 could serve as an oncogene and facilitates tumorigenesis by binding to miRNA targeting sites for competing endogenous RNAs, especially in neuroblastoma.42,43 Sponge miR-149-30 promotes apoptotic activity, contributing to impairments of neurons of cerebral ischemia/reperfusion mice.44 Similarly, accumulating evidence has concentrated on the role of HCP5 in oncogenesis, including glioma, gastric cancer, and ovarian cancer.45,46 In terms of neurological disorders, enhanced HCP5 expression was associated with the aggravation of cerebral ischemic reperfusion injury by sponging miR-652-3p.47CRNDE is located in specific brain regions of humans and mice that are tightly linked to gliomagenesis.48,49 Knocking out LncRNA CRNDE alleviates brain damage, resulting from hypoxic-ischemic or traumatic brain injury by promoting autophagy or nerve repair.50,51 Besides, as immune-related lncRNAs, LY86-AS1 variants are involved in the onset of autoimmune blistering disease of the skin and type 2 diabetes mellitus.52,53 The expressions of gender-associated LY86-AS1 are negatively correlated with the Braak stage of Alzheimer's disease.54 In accordance with these reports, our hub lncRNAs might manage neuron survival via couples of pathways in the scenario of ICH, such as promoting cell apoptosis or regulating inflammatory response. Accordingly, it is greatly worthy to arrange more researches on this topic. In addition, as a quick and cheap sampling method, blood can be routinely acquired in clinical tests, which was employed for the validation of hub lncRNA candidates here. We observed altered expressions of MIAT, LY86-AS1, DLX6-AS1, RRN3P2, CRNDE, and HCP5 in the blood of patients with ICH, implying their involvement in ICH. ROC curves, together with the AUCs, are useful tools for evaluating the test accuracy and for selecting potential biomarkers.55,56 Based on our ROC results, these key lncRNAs may be employed as molecular indicators for early screening and monitoring of hemorrhage stroke.
There are several limitations of the current study. Firstly, the sample size of GSE24265 for analysis is relatively small, thereby, more clinical samples are necessary to confirm the expression pattern of hub lncRNAs in the future. Moreover, the relationship between lncRNA and other factors should be investigated for further elucidating the ICH mechanism, such as vascular risk factors. Finally, although we have utilized three databases together with our co-expressed network analysis to acquire mRNA targets for the interested lncRNAs, the incomplete coverage of various interactions will probably produce some biased prediction for lncRNA–miRNA or miRNA–mRNA pairs, particularly those unknown interactions.57 On the one hand, using diverse computational models, including machine learning-based models and models with no requirement of uncovering ncRNA pairs, might be a promising approach for lncRNA–miRNA–mRNA selection.57,58 On the other hand, to probe the relationship between hub lncRNAs or miRNAs and ICH using different computational tools, such as Laplacian Regularized Least Squares for LncRNA–Disease Association (LRLSLDA),59 Inductive Matrix Completion for MiRNA–Disease Association prediction (IMCMDA)60 or Matrix Decomposition and Heterogeneous Graph Inference for miRNA–disease association prediction (MDHGI),61 would become our task in the future as well. Additionally, capitalizing on hypergeometric distribution models may be also helpful for evaluating the mRNA–lncRNA interaction simultaneously instead of detecting lncRNA–mRNA interaction only.
Conclusions
We identified DElncRNAs in various regions of the brain by analyzing a published dataset of ICH. Based on co-expression interactions and the ceRNA theory, the lncRNA-related ceRNA networks were constructed. Six lncRNAs: MIAT, LY86-AS1, DLX6-AS1, RRN3P2, CRNDE, and HCP5 may be utilized as biomarkers for ICH progression. Our work may contribute to an increased understanding of the pathogenesis of ICH and elucidate the role of novel lncRNAs as potential targets for a breakthrough in ICH diagnosis and treatment.
Data availability
This study was carried out using publicly available data from Gene Expression Omnibus (GEO) database (https://www.ncbi.nlm.nih.gov/gds/) with GSE24265. All data generated or analysed during this study are included in this published article.
Author contributions
Conceptualization: X. X. and C. Y.; formal analysis: C. Y., J. W., S. X., X. L. and X. X.; funding acquisition: X. X.; methodology: X. L. and X. X.; resources: C. Y., J. W. and X. X.; supervision: X. X.; visualization: C. Y., J. W. and X. X.; writing – original draft: C. Y., J. W., X. L., S. X. and X. X.; writing – review&editing: X. X.
Conflicts of interest
We have no conflict of interests.
Acknowledgements
This work was kindly supported by National Natural Science Foundation of China (81601129) and Science Foundation of Shenyang (F16-205-1-48).
References
- A. I. Qureshi, A. D. Mendelow and D. F. Hanley, Lancet, 2009, 373, 1632–1644 CrossRef.
- R. F. Keep, Y. Hua and G. Xi, Lancet Neurol., 2012, 11, 720–731 CrossRef CAS.
- A. Rosell, A. Vilalta, T. Garcia-Berrocoso, I. Fernandez-Cadenas, S. Domingues-Montanari, E. Cuadrado, P. Delgado, M. Ribo, E. Martinez-Saez, A. Ortega-Aznar and J. Montaner, PLoS One, 2011, 6, e16750 CrossRef CAS.
- K. T. Lee and J. W. Nam, BMB Rep., 2017, 50, 226–231 CrossRef.
- Z. Li, X. Li, C. Chen, S. Li, J. Shen, G. Tse, M. T. V. Chan and W. K. K. Wu, Cell Proliferation, 2018, 51, e12483 CrossRef PubMed.
- L. Salmena, L. Poliseno, Y. Tay, L. Kats and P. P. Pandolfi, Cell, 2011, 146, 353–358 CrossRef CAS PubMed.
- P. Wan, W. Su and Y. Zhuo, Mol. Neurobiol., 2017, 54, 2012–2021 CrossRef CAS.
- D. Liu, Z. Zou, G. Li, P. Pan and G. Liang, Cancer Control, 2020, 27, 1073274819897977 Search PubMed.
- J. M. Kim, J. Moon, J. S. Yu, D. K. Park, S. T. Lee, K. H. Jung and K. Chu, Ann. Clin. Transl. Neurol., 2019, 6, 2014–2025 CrossRef CAS PubMed.
- C. Hanjin, L. Tao, L. Pengfei, Y. Ali, Z. Huajun, L. Jiekun, W. Yang and T. Tao, Cell. Physiol. Biochem., 2018, 45, 1284–1301 CrossRef.
- L. Xie, Y. Wang and Z. Chen, Life Sci., 2021, 267, 118942 CrossRef CAS.
- B. Chen, H. Wang, C. Lv, C. Mao and Y. Cui, Bioengineered, 2021, 12, 4004–4015 CrossRef CAS.
- B. Xie, M. Qiao and J. Xuan, Med. Sci. Monit., 2021, 27, e929435 CAS.
- J. X. Chen, Y. P. Wang, X. Zhang, G. X. Li, K. Zheng and C. Z. Duan, Brain Res. Bull., 2020, 162, 20–29 CrossRef CAS.
- X. Zhang, S. Sun, J. K. Pu, A. C. Tsang, D. Lee, V. O. Man, W. M. Lui, S. T. Wong and G. K. Leung, Neurobiol. Dis., 2012, 48, 1–8 CrossRef.
- X. Xu, Y. Hao, S. Xiong and Z. He, Interdiscip. Sci., 2020, 12, 447–460 CrossRef CAS PubMed.
- X. Wang, S. Ma, M. Mao, C. Li, X. Shen, S. Xu and J. Yang, BioMed Res. Int., 2019, 2019, 7505260 Search PubMed.
- Y.-H. Shi, X.-W. He, F.-D. Liu, Y.-S. Liu, Y. Hu, L. Shu, G.-H. Cui, R. Zhao, L. Zhao, J.-J. Su and J.-R. Liu, Gene, 2019, 697, 184–193 CrossRef CAS PubMed.
- J. Wang, Y. Zhu, F. Jin, L. Tang, Z. He and Z. He, J. Int. Med. Res., 2016, 44, 419–432 CrossRef CAS PubMed.
- J. Aprea, S. Prenninger, M. Dori, T. Ghosh, L. S. Monasor, E. Wessendorf, S. Zocher, S. Massalini, D. Alexopoulou, M. Lesche, A. Dahl, M. Groszer, M. Hiller and F. Calegari, EMBO J., 2013, 32, 3145–3160 CrossRef CAS PubMed.
- J. S. Sunwoo, S. T. Lee, W. Im, M. Lee, J. I. Byun, K. H. Jung, K. I. Park, K. Y. Jung, S. K. Lee, K. Chu and M. Kim, Mol. Neurobiol., 2017, 54, 1577–1586 CrossRef CAS PubMed.
- M. A. Faghihi, F. Modarresi, A. M. Khalil, D. E. Wood, B. G. Sahagan, T. E. Morgan, C. E. Finch, G. St Laurent, 3rd, P. J. Kenny and C. Wahlestedt, Nat. Med., 2008, 14, 723–730 CrossRef CAS PubMed.
- J. Li, M. Hao, B. Yang, T. Shi, Y. Zhang, J. Feng and J. Chen, Medicine, 2020, 99, e22964 CrossRef CAS PubMed.
- C. Tao, X. Hu, H. Li and C. You, Front. Hum. Neurosci., 2017, 11, 422 CrossRef PubMed.
- Y. B. Jiang, K. Y. Wei, X. Y. Zhang, H. Feng and R. Hu, CNS Neurosci. Ther., 2019, 25, 1113–1125 CrossRef.
- S. Yip and B. R. Sastry, Brain Res., 2000, 864, 1–12 CrossRef CAS.
- G. Lao, V. Scheuss, C. M. Gerwin, Q. Su, S. Mochida, J. Rettig and Z. H. Sheng, Neuron, 2000, 25, 191–201 CrossRef CAS.
- R. F. Keep, A. V. Andjelkovic, J. Xiang, S. M. Stamatovic, D. A. Antonetti, Y. Hua and G. Xi, J. Cereb. Blood Flow Metab., 2018, 38, 1255–1275 CrossRef CAS PubMed.
- G. Bazzoni and E. Dejana, Physiol. Rev., 2004, 84, 869–901 CrossRef CAS.
- X. Xu, L. E. Wicki-Stordeur, J. C. Sanchez-Arias, M. Liu, M. S. Weaver, C. S. W. Choi and L. A. Swayne, Front. Cell. Neurosci., 2018, 12, 124 CrossRef.
- C. Lei, S. Lin, C. Zhang, W. Tao, W. Dong, Z. Hao, M. Liu and B. Wu, Neuroscience, 2013, 230, 86–93 CrossRef CAS PubMed.
- Z. Süle, E. Mracskó, E. Bereczki, M. Sántha, T. Csont, P. Ferdinandy, F. Bari and E. Farkas, Life Sci., 2009, 84, 935–939 CrossRef.
- P. J. Derry, A. T. T. Vo, A. Gnanansekaran, J. Mitra, A. V. Liopo, M. L. Hegde, A. L. Tsai, J. M. Tour and T. A. Kent, Front. Cell. Neurosci., 2020, 14, 603043 CrossRef CAS.
- T. Xi, F. Jin, Y. Zhu, J. Wang, L. Tang, Y. Wang, D. S. Liebeskind, F. Scalzo and Z. He, J. Biol. Chem., 2018, 293, 20041–20050 CrossRef CAS.
- S. Wang, Y. Cui, J. Xu and H. Gao, Inflammation, 2019, 42, 1869–1877 CrossRef CAS PubMed.
- Q. Guo, H. Su, J. B. He, H. Q. Li and J. J. Sha, Biochem. Biophys. Res. Commun., 2018, 504, 61–67 CrossRef CAS.
- X. L. Ma, S. Y. Li and F. Shang, Biomed. Pharmacother., 2017, 93, 238–244 CrossRef CAS PubMed.
- Y. Ohnishi, T. Tanaka, R. Yamada, K. Suematsu, M. Minami, K. Fujii, N. Hoki, K. Kodama, S. Nagata, T. Hayashi, N. Kinoshita, H. Sato, H. Sato, T. Kuzuya, H. Takeda, M. Hori and Y. Nakamura, Hum. Genet., 2000, 106, 288–292 CrossRef CAS.
- C. M. Da, C. Y. Gong, W. Nan, K. S. Zhou, Z. L. Wu and H. H. Zhang, Biomed. Pharmacother., 2020, 129, 110359 CrossRef CAS PubMed.
- M. Zhu, N. Li, P. Luo, W. Jing, X. Wen, C. Liang and J. Tu, J. Stroke Cerebrovasc. Dis., 2018, 27, 326–337 CrossRef PubMed.
- E. Y. Li, P. J. Zhao, J. Jian, B. Q. Yin, Z. Y. Sun, C. X. Xu, Y. C. Tang and H. Wu, Cell Cycle, 2019, 18, 156–166 CrossRef CAS PubMed.
- H. Y. Zhang, M. Q. Xing, J. Guo, J. C. Zhao, X. Chen, Z. Jiang, H. Zhang and Q. Dong, Cancer Cell Int., 2019, 19, 313 CrossRef CAS.
- Q. Zheng, X. Gu, Q. Yang, Q. Chu, Y. Dai and Z. Chen, Clin. Chim. Acta, 2021, 517, 1–8 CrossRef CAS PubMed.
- X. Hu, Z. Xiang, W. Zhang, Z. Yu, X. Xin, R. Zhang, Y. Deng and Q. Yuan, Aging, 2020, 12, 23096–23113 CAS.
- J. Zhao, X. Song, T. Xu, Q. Yang, J. Liu, B. Jiang and J. Wu, Front. Genet., 2020, 11, 607722 CrossRef CAS.
- W. Chen, K. Zhang, Y. Yang, Z. Guo, X. Wang, B. Teng, Q. Zhao, C. Huang and Z. Qiu, Int. J. Biol. Sci., 2021, 17, 623–634 CrossRef CAS.
- J. D. Gao, R. J. Li, P. L. Ma, L. L. Yu, J. T. Li and H. T. Tian, J. Biol. Regul. Homeostatic Agents, 2020, 34, 893–900 CAS.
- B. C. Ellis, P. L. Molloy and L. D. Graham, Front. Genet., 2012, 3, 270 CAS.
- A. Zottel, N. Šamec, A. Videtič Paska and I. Jovčevska, Cancers, 2020, 12, 1842 CrossRef CAS PubMed.
- C. H. Fu, F. F. Lai, S. Chen, C. X. Yan, B. H. Zhang, C. Z. Fang and G. H. Wang, Mol. Cell. Biochem., 2020, 472, 1–8 CrossRef CAS PubMed.
- M. Yi, X. Dai, Q. Li, X. Xu, Y. Chen and D. Wang, Cell Cycle, 2019, 18, 2332–2343 CrossRef CAS PubMed.
- A. Salviano-Silva, T. D. J. Farias, V. Bumiller-Bini, M. S. Castro, S. C. Lobo-Alves, H. Busch, C. Pföhler, M. Worm, M. Goebeler, N. van Beek, A. Franke, M. Wittig, D. Zillikens, R. C. de Almeida, J. E. Hundt, A. B. W. Boldt, S. Ibrahim, D. G. Augusto, M. L. Petzl-Erler, E. Schmidt and D. Malheiros, Exp. Dermatol., 2021, 30, 831–840 CrossRef CAS.
- L. Saeidi, H. Ghaedi, M. Sadatamini, R. Vahabpour, A. Rahimipour, M. Shanaki, Z. Mansoori and F. Kazerouni, Mol. Biol. Rep., 2018, 45, 2601–2608 CrossRef CAS PubMed.
- M. Cao, H. Li, J. Zhao, J. Cui and G. Hu, Neurobiol. Aging, 2019, 81, 116–126 CrossRef CAS PubMed.
- G. Heller, V. E. Seshan, C. S. Moskowitz and M. Gonen, Biostatistics, 2017, 18, 260–274 Search PubMed.
- K. Soreide, H. Korner and J. A. Soreide, Ann. Surg., 2011, 253, 27–34 CrossRef.
- X. Chen, C. C. Yan, X. Zhang and Z. H. You, Briefings Bioinf., 2017, 18, 558–576 CAS.
- X. Chen, D. Xie, Q. Zhao and Z. H. You, Briefings Bioinf., 2019, 20, 515–539 CrossRef CAS PubMed.
- X. Chen and G. Y. Yan, Bioinformatics, 2013, 29, 2617–2624 CrossRef CAS PubMed.
- X. Chen, L. Wang, J. Qu, N. N. Guan and J. Q. Li, Bioinformatics, 2018, 34, 4256–4265 CAS.
- X. Chen, J. Yin, J. Qu and L. Huang, PLoS Comput. Biol., 2018, 14, e1006418 CrossRef PubMed.
Footnote |
† Electronic supplementary information (ESI) available. See DOI: 10.1039/d1mo00298h |
|
This journal is © The Royal Society of Chemistry 2022 |
Click here to see how this site uses Cookies. View our privacy policy here.