DOI:
10.1039/D2RA04228B
(Paper)
RSC Adv., 2022,
12, 22650-22661
Alpha glucosidase inhibitory properties of a few bioactive compounds isolated from black rice bran: combined in vitro and in silico evidence supporting the antidiabetic effect of black rice
Received
8th July 2022
, Accepted 30th July 2022
First published on 12th August 2022
Abstract
In view of the recent reports of the antidiabetic effect of the black rice bran extract, an attempt has been made in the present work to evaluate the potential α-glucosidase inhibitory activity of a few selected bioactive compounds present in the pericarp of the black rice. Out of the six bioactive compounds from black rice bran selected for the study, two compounds viz. cyanidin-3-glucoside and 6′-O-feruloylsucrose were identified as novel and highly potent α-glucosidase inhibitors via their in vitro and in silico screenings. The enzyme inhibition assay was corroborated by molecular docking and molecular dynamics simulation studies. Molecular docking studies suggested high binding energies and good binding interactions of these compounds with the active site residues of the receptor protein. A good agreement was found between the results of both modes of evaluation. The experimental results proved that the black rice bran extract can show 62% of alpha glucosidase inhibiting enzyme activity as compared to that of the popular drug Acarbose. While both the docking scores and binding affinity values indicate the formation of a ligand–enzyme complex by the major components of the extract, the molecular dynamics study further indicates the stability of the complex. The pharmacokinetic (ADMET properties) studies of these active compounds also support their use as safe oral anti-diabetic drugs. Thus, the results obtained from these studies of alpha glucosidase inhibition by bioactive compounds present in black rice bran indicate that these bioactive compounds can produce significant antidiabetic activity by inhibiting the active site of the target enzyme and hence these compounds can be used as leads for the synthesis of new antidiabetic drugs.
Introduction
Diabetes mellitus is a chronic metabolic disease which is characterized by an increase in blood glucose level.1,2 Diabetes occurs either when the pancreas does not produce enough insulin or when the body cannot effectively use the insulin it produces. Insulin-dependent diabetes mellitus (IDDM) or type 1 diabetes and non-insulin-dependent diabetes mellitus (NIDDM) or type 2 diabetes are the two major types of diabetes mellitus which share the same characteristics i.e., postprandial hyperglycemia (PPHG). Chronic hyperglycemia or raised blood sugar resulting from uncontrolled diabetes causes production of free radicals and reactive oxygen species, leading to oxidative tissue damage and complications such as nephropathy, neuropathy, retinopathy, and memory impairment.3 Diabetes also affects the metabolism of lipids and proteins in advanced stages leading to increased risk of stroke, cardiovascular diseases, renal failure, blindness and amputation.4,5 The regulation or control of PPHG is one of the most common strategy for management of diabetes. The reduction of PPHG is achieved by preventing the absorption of carbohydrates after food intake. Complex starch, oligosaccharides and disaccharides present in food are absorbed in the duodenum and upper jejunum in the form of their respective monosaccharides. The digestion of these carbohydrates is facilitated by pancreatic α-amylase and α-glucosidase enzymes that are attached to the brush border of the intestinal cells.6 Inhibition of α-glucosidases reduces and delays the digestive absorption of glucose and decreases PPHG. Therefore, oral α-glucosidase inhibitors (AGI) such as acarbose, miglitol, voglibose, etc. are widely used in the treatment of patients with type 2 diabetes.5 They decrease PPHG without inducing hypoglycemia and have a good safety profile. However, gastrointestinal adverse effects may limit long-term compliance to therapy.7 For example, a common AGI, Acarbose, can elicit unpleasant side effects including abdominal pain, diarrhea and flatulence and is not well accepted by both patients and physicians.8,9
The antidiabetic activity of bioactive substances present in many food and traditional medicines have been noticed and reported.10,11 For the treatment of diabetes, natural resources might provide a large pool of potential therapeutic agents.12 Natural α-glucosidase and α-amylase inhibitors obtained from plant resources give an attractive strategy for the control of PPHG in diabetic patients.13
A number of recent studies revealed that black rice (Oryza sativa L.) has promising anti-diabetic activity due to presence of diverse bioactive compounds. Black rice is a type of the rice species mainly cultivated in Asia and is packed with high level of nutrients.14,15 Black rice bran extract reduces inflammation of adipose and liver steatosis in rats and thereby decreases hyperglycemia in diabetic mice.16 Recently, researchers revealed that the pigmented brans have the ability to inhibit α-glucosidase activity as well as cancer.17 Wahyuni et al. reported that the ethanol extract of black rice bran can decrease blood glucose level on alloxan-induced diabetic rats.16 Administration of phenolic acids present in black rice bran was reported to decrease blood glucose levels significantly with increase in plasma insulin levels. Moreover, the ethyl acetate extract of the rice bran can significantly elevate hepatic glycogen synthesis and glucokinase activity compared with the control group.18
Several attempts have been made to identify the bioactive compounds from different varieties of pigmented rice which might be responsible for the anti-diabetic activity of black rice bran. The pericarp (outer part) of kernel of this rice is black in color due to the pigment known as anthocyanin, a class of water-soluble flavonoids. The structural identification and quantification of the black rice anthocyanins performed by high performance liquid chromatography coupled with electrospray ionization and tandem mass spectrometry found cyanidin-3-O-glucoside and peonidin-3-O-glucoside as the major anthocyanins in the black rice bran comprising of 93% of the total anthocyanins in black rice.19 The natural anthocyanin COG is known to have strong anti-oxidative and radical scavenging activities against hydroxyl and superoxide radicals. In a reported in vivo study, anti-hypoglycemic and anti-osteoporosis effects of purified cyanidin-3-glucoside have been demonstrated in the kidneys; the effects are produced by reducing blood glucose and by suppressing oxidative stress and inflammation.20
Ghasemzadeh et al. detected five phenolic compounds (viz. protocatechuic acid, syringic acid, ferulic acid, cinnamic acid, and p-coumaric acid) and five flavonoid compounds (quercetin, apigenin, catechin, luteolin, and myrecitin) in the brans of brown and black rice.21 Ferulic acid and p-coumaric acid were reported to be the most abundant flavonoid compounds in black rice bran extract. The presence of cyanadin 3-O-glucoside (COG), 6′-O-feruloylsucrose (FLS) and sinapic acid (SPA) was reported by Shao Y et al., Tian S. et al. and Pang Y et al. respectively.22–24 The authors used HPLC analysis of the black rice bran extract in this work and verified the presence of three compounds viz. syringic acid (SYA), p-coumaric acid (pCmA), and protocatechuic acid (PCA) in the extract.
Though the antioxidant activity of these compounds were studied by various researchers but the exact mechanism of the antidiabetic effect shown by these phytochemicals is yet to be established.16,24,25 To elucidate the mechanism of antidiabetic effect of BRBE, the present work was designed by combining in vitro and in silico studies. The percentage inhibition of alpha glucosidase enzyme was studied with fresh BRBE through in vitro experiment while molecular interactions existing between the major compounds in the rice bran extract with the enzyme was studied using in silico approaches. For the in silico studies, six bioactive compounds extracted from black rice viz. COG, FLS, pCmA, SYA, SPA, and PCA were selected. The GFAT enzyme inhibitory ability of these six bioactive components has been reported in our previous in silico study.26
The stability of the complexes formed by these compounds with alpha-glucosidase was estimated by Molecular Mechanics Generalized Born Surface Area (MMGBSA) determination followed by Molecular Dynamics (MD) simulation.27 Acarbose, a clinically accepted drug for diabetes, which is an established α-glucosidase inhibitor, was taken as the standard for comparison in the in vitro study. The in silico screening for anti-diabetic activity of naturally occurring substances by measuring inhibition of α-glucosidase enzymes through molecular docking studies has also been reported by other workers.28 In this work, the stability of the complex formed by the inhibitor and the enzyme has also been studied by employing molecular dynamics simulations.
The drug-like properties of the selected bioactive compounds present in black rice bran is studied by applying Lipinski's rule. The Lipinski's rule is one of the most popular rules to predict whether a molecule or compound can be used as an orally active drug or not.29 The criteria of these Lipinski's rule states that (i) molecular weight of the molecule should be less than 500 unified atomic mass units, (ii) the number of hydrogen bond donors should be less than 5 (iii) the number of hydrogen bond acceptors should be less than 10 and (iv) the octanol–water partition coefficient or logP value should not exceed 5.30
To know about the relationship between the activity and the potential of these bioactive compounds as an oral anti-diabetic drug, the in silico ADMET (Absorption Distribution Metabolism Excretion and toxicity) prediction study31 was also carried out.
Materials and methods
Materials
The black rice was collected in January, 2021 from Golaghat district of Assam, India. p-Nitrophenyl-α-D-glucopyranoside (PNPG) and α-glucosidase derived from baker's yeast were purchased from Sigma, USA. Buffer capsules (pH 4, 7, 9) were purchased from MERCK. All other chemicals and solvents used such as ethanol, methanol, hydrochloric acid and acetic acid were of analytical grade. The alpha-glucosidase inhibitory activity was spectrophotometrically measured by UV-vis spectrophotometer (UV-2600 SHIMADZU).
Preparation of the black rice bran extract
The crude methanolic extract was obtained by extracting 200 grams of dried black rice bran powder with 1000 mL of methanol for 72 hours using a Soxhlet apparatus. The extract was concentrated using rotary vacuum evaporator at 45–50 °C and stored at 0–4 °C for further experiment. The ethyl acetate and hexane extract was obtained by fractionation from the crude methanolic extract.
In vitro alpha-glucosidase inhibition assay
The α-glucosidase inhibitory activities of the three extracts viz. ethyl acetate extract, methanolic extract and hexane extract were determined spectrophotometrically by monitoring the release of p-nitrophenol from the PNPG.32 A mixture of α-glucosidase enzyme (2 U mL−1), 20 μL of the extract at a concentration of 100 mg mL−1 incubated for 5 min at 37 °C. Then 1 mM PNPG (20 μL) in 50 mM of phosphate buffer (pH 6.8) was added to initiate the reaction. The mixture was incubated again for 30 min at 37 °C, and then the reaction was terminated by the addition of 3 mL of NH4OH solution (0.05 M). The same volume of distilled water and Acarbose (100 mg mL−1) were used as negative and positive controls respectively. The α-glucosidase activity was determined by measuring the absorbance at 405 nm using UV-vis spectrophotometer (UV-2600 SHIMADZU).
The inhibitory activity was calculated using following formula33,34
Each test was performed 3 times and to indicate the inhibitory activity of the rice extract the results were expressed as mean value ± SD.
Determination of IC50
The half maximal inhibitory concentration (IC50) i.e., the concentrations of Acarbose and the BRBE at which 50% of the enzyme activity was inhibited was calculated by using logarithmic regression analysis.35,36
Chromatographic detection of phytochemicals
The HPLC system (agilent/1260 infinity) used for the detection of phytochemicals was fitted with a diode array detector (DAD). A binary reverse phase mobile phase system with a flow rate of 0.8 mL min−1 through a C18 column was used to perform the chromatographic separation. The mobile phase system contained aqueous acetic acid (A, v/v) and methanol (B) with 0.5% concentrations. The wavelength for p-coumaric acid, syringic acid and protocatechuic acid was set at 280 nm. The column compartment was kept at an ambient temperature throughout the analysis.37,38 By comparing the retention time and peak area with the corresponding standards peaks in the extracts were identified.
ADMET prediction and Lipinki's rule
The ADMET (Absorption, Distribution, Metabolism, Excretion and Toxicity) properties, as well as the drug likeness of the six bioactive compounds isolated from black rice bran were studied with the help of Protox-II virtual lab (https://www.tox-new.charite.de/protox_II/), pkCSM (http://www.biosig.unimelb.edu.au/pkcsm/) preADMET (https://www.preadmet.qsarhub.com/) and Drug Likeness Tool (DruLiTo, http://www.niper.gov.in/pi_dev_tools/DruLiToWeb/DruLiTo_index.html).29,39,40
Molecular docking study
The molecular docking study reported in the work involved the following components:
Preparation of protein and ligands
The crystal structure of alpha-glucosidase enzyme (PDBs: 3A4A) protein was downloaded from Protein Data Bank (PDB, http://www.rcsb.org/) at a resolution of 1.9 Å and refined by removing water molecules followed by the addition of hydrogen bond by utilizing the protein preparation wizard of the Glide (Schrodinger suite 2018-2; Schrodinger, LLC), module and the Prime 3.0 module. Geometry optimization by the OPLS_2005 force field to a maximum root-mean-square deviation (rmsd) of 0.30 Å was performed.
The 3D ligand structures of the six phytochemicals present in BRBE which were selected for the present study were all downloaded from PubChem (https://www.pubchem.ncbi.nlm.nih.gov/) using the PubChem IDs of all the ligands. The two -dimensional structures of the six ligands are shown in Fig. 1. Maestro 11.6 software (Maestro, version 11.6, Schrodinger, LLC) was used for drawing of ligand structures and utilizing LigPrep module software (Schrodinger 2018: LigPrep, version 3.1, Schrodinger, LLC) preparation of ligands was done. OPLS-2005 force field software was used for generation of least energy conformations as well as to obtain correct molecular geometries and ionization at pH 7.0 ± 2.0.
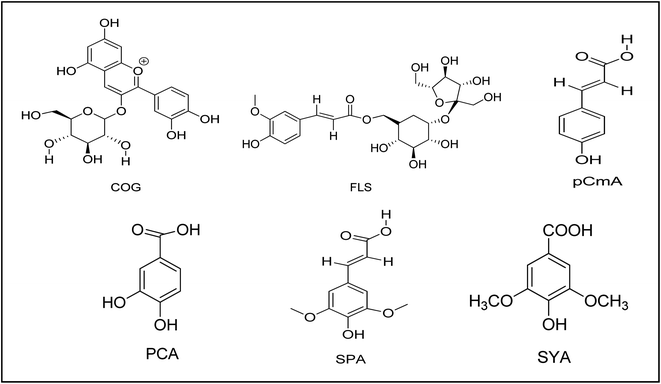 |
| Fig. 1 Structures of the six phytochemicals present in BRBE chosen for the study viz. cyanadin 3-O-glucoside (COG), 6′-O-feruloylsucrose (FLS), protocatechuic acid (PCA), sinapic acid (SPA), syringic acid (SYA), and p-coumaric acid (pCmA). | |
Molecular docking
Induced Fit Docking (IFD) module with Prime program of Schrodinger software package was used for docking analyses of the six ligands of BRBEs, COG, FLS, PCA, SYA, SPA, and pCmA with the target enzyme. In the Induced Fit protocol various ligand poses were generated temporarily eliminating highly flexible side chains during the docking step. These were subjected to energy minimization and finally, each ligand is re-docked into its corresponding low energy protein structures and the resulting complexes are positioned according to Glide Score.
Calculation of binding energy
The binding affinity calculation was done to evaluate ligand–alpha glucosidase complex formation by utilizing the Prime module of Schrodinger 2018-2 with Molecular Mechanics Generalized Born Surface area (MMGB-SA).
Molecular dynamics simulations
MD simulations were carried out to evaluate how tightly the compounds can bind to the active sites of alpha-glucosidase. For this, the docked conformation of COG molecule with human alpha glucosidase enzyme (PDB id: 3A4A) which showed promising result was selected. The docked structure was used to run the MD simulations at 300 K for 35 ns. The molecular dynamics simulation was carried out by using the Amber18 software package.41 GAFF (general amber force field) and ff14SB were the force field parameters used for the ligand COG and the enzyme respectively.42 The Antechamber module of Amber was used to generate the topology file and other force field parameters. For solvation of the enzyme 38058 TIP3P water molecules were used in a cubic box. 28 Na+ ions were required to neutralize the system.
Results and discussions
Alpha-glucosidase inhibition assay
The results obtained from the enzyme inhibition assay of the black rice bran indicate that out of the three extracts viz. ethyl acetate (EA) extract, methanolic extract and hexane extract, the α-glucosidase inhibitory effect of the EA extract and the methanolic extract are more or less comparable while that of the hexane extract of the BRBE is slightly less. The α-glucosidase inhibitory effect of the EA extract is comparable to that produced by the clinically established drug at similar concentration. For example, at a concentration of 100 μg mL−1, the EA extract showed 66.7% inhibitory effect on α -glucosidase while Acarbose showed about 75.4% inhibitory activity (Fig. 2).
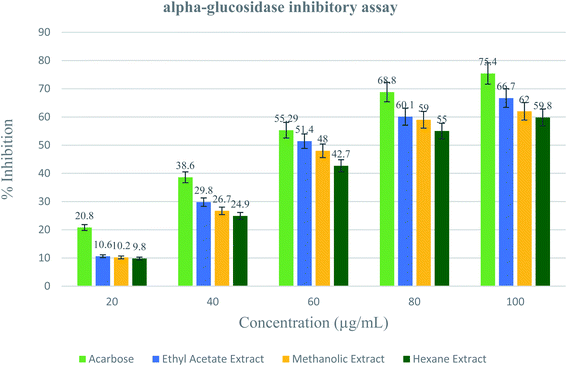 |
| Fig. 2 Inhibitory activity of Acarbose and black rice bran extract in ethyl acetate, methanol and hexane against alpha glucosidase enzyme. | |
Determination of half-maximal inhibitory concentration (IC50)
To determine the half maximal inhibitory concentration (IC50) of the methanolic extract of black rice bran logarithmic regression analysis method was followed. The amount of BRBE required per mL for the inhibition of the alpha-glucosidase enzyme activity by 50% was expressed in μg mL−1. All the three extracts of black rice exhibited almost comparable IC50 values ranging from 47 to 52 μg mL−1 (Table 1).
Table 1 Half-maximal inhibitory concentration (IC50) value of BRBE for inhibition of alpha-glucosidase enzyme activity
|
% inhibition |
IC50 (μg mL−1) |
Concentrations (μg mL−1) |
20 |
40 |
60 |
80 |
100 |
Acarbose |
20.8 ± 0.75 |
38.6 ± 0.26 |
55.29 ± 0.70 |
68.8 ± 1.21 |
75.4 ± 0.91 |
56.42 ± 4.17 |
Ethyl acetate |
10.6 ± 0.72 |
29.8 ± 0.26 |
51.4 ± 0.50 |
60.1 ± 1.41 |
66.7 ± 0.52 |
47.79 ± 2.28 |
Methanolic extract |
10.2 ± 0.96 |
26.7 ± 1.50 |
48 ± 1.51 |
59 ± 4.46 |
62 ± 2.19 |
48.50 ± 0.83 |
Hexane extract |
9.8 ± 0.36 |
24.9 ± 0.69 |
42.7 ± 0.65 |
55 ± 0.52 |
59.8 ± 1.05 |
52.80 ± 1.65 |
Detection of major phytochemicals in the extract
The methanol extract was subjected to HPLC in order to detect the major phenolic components. The HPLC chromatogram of the black rice bran extract and the standards are shown in Fig. 3. The peak at retention time 5.080 min of the chromatogram of the extract corresponds to the standard protocatachuic acid. At retention time 8.500 min, the peak resembles with the standard syringic acid while the peak at 13.507 min showed a significant match with the chromatograms of p-coumaric acid. Therefore, it may be inferred from the analysis that the extract of black rice bran contains these three phytochemicals along with other compounds.
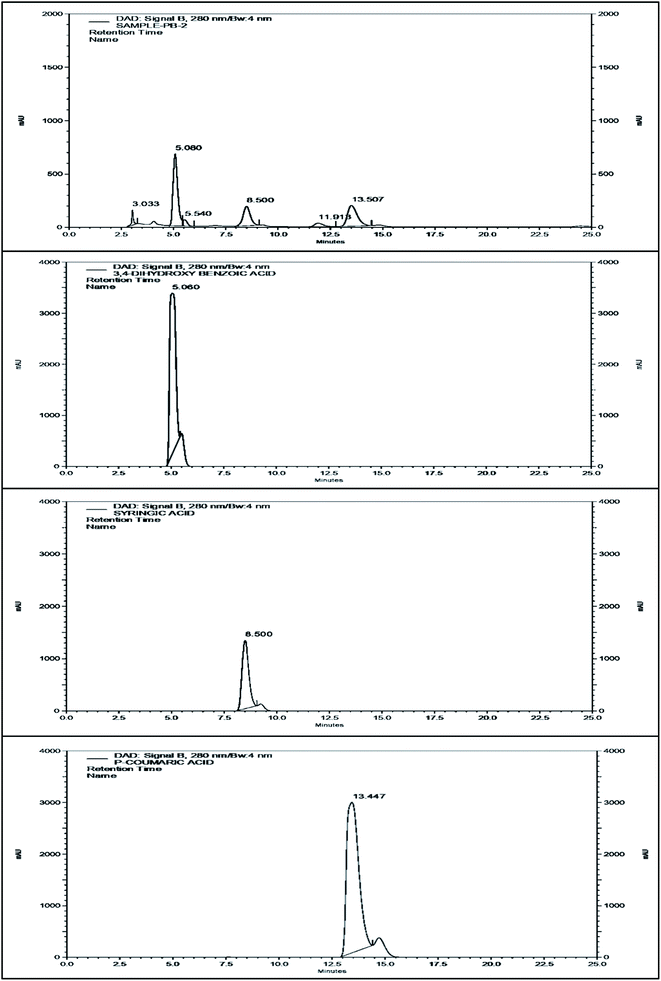 |
| Fig. 3 The HPLC chromatogram of the BRBE, protocatachuic acid, syringic acid and p-coumaric acid respectively. | |
In silico studies
ADMET prediction and Lipinski's rule of five. The analysis of absorption, distribution, metabolism, excretion and toxicity prediction results showed that almost all the six ligands isolated from black rice bran possess ideal drug-like characteristics (Table 2). For the study of absorption, the water-solubility, Caco-2 permeability and intestinal absorption values were evaluated. The volume of distribution (VDss), blood–brain barrier (BBB) permeability and CNS permeability values were considered to measure the distribution of the compounds. Similarly, for metabolism cytochrome P450 inhibition using CYP2D6/CYP3A4 substrate and for excretion renal OCT2 substrate and total clearance were studied. For the determination of toxicity in rat, LD50, maximum tolerated dose and hepatotoxicity were studied. The intestinal absorption of all the ligands was found to be in the range of 30–93% which indicates good absorption in the small intestine. The lower values of BBB permeability implies that these ligands would not affect the brain and would not produce any side effect within the brain which is also supported by the CNS permeability values of all the ligands having values <−3. All other values of toxicity, excretion and metabolism support the potential of the ligands to be used as leads for the development of antidiabetic drug.
Table 2 Pharmacokinetic properties of the bioactive compounds isolated from BRBE
Ligands |
Absorption |
Distribution |
Metabolism |
Excretion |
Toxicity |
Caco-2 permeability |
Intestinal absorption |
Water solubility |
VDss |
BBB permeability |
CNS permeability |
Cytochrome P450 inhibitors |
CYP2D6/CYP3A4 substrate |
Renal OCT2 substrate |
Total clearance |
Rat LD50 |
Max. tolerated dose |
Hepatotoxicity |
COG |
0.058 |
45.392 |
−2.929 |
1.464 |
−1.713 |
−3.813 |
No |
No |
No |
0.522 |
2.549 |
0.562 |
No |
FLS |
−0.478 |
29.911 |
−1.863 |
−0.186 |
−1.358 |
−4.977 |
No |
No |
No |
0.715 |
2.505 |
0.174 |
No |
PCA |
0.49 |
71.174 |
−2.069 |
−1.298 |
−0.683 |
−3.305 |
No |
No |
No |
0.551 |
2.423 |
0.814 |
No |
SPA |
0.272 |
93.064 |
−2.869 |
−1.11 |
−0.247 |
−2.663 |
No |
No |
No |
0.718 |
2.24 |
1.193 |
No |
SYA |
0.495 |
73.076 |
−2.223 |
−1.443 |
−0.191 |
−2.701 |
No |
No |
No |
0.646 |
2.157 |
1.374 |
No |
pCmA |
1.21 |
93.494 |
−2.378 |
−1.151 |
−0.225 |
−2.418 |
No |
No |
No |
0.662 |
2.155 |
1.111 |
No |
Table 3 shows the molecular weight, log
P, number of H bond donor and number of H bond acceptors present in the bioactive compounds. From the observed values it is clear that almost all the ligands obey Lipinski's rule suggesting that these ligands can be used as an oral drug or can be used as a lead for the synthesis of new antidiabetic drug (Table 4).
Table 3 Molecular properties of the bioactive ligands from BRBE (Lipinki's rule)
Ligands |
Molecular weight |
Log P |
No. of atoms |
H-bond acceptor |
H-bond donor |
Topological polar surface area |
Molecular refractivity |
Predicted toxicity class |
COG |
449.388 |
0.382 |
53 |
10 |
8 |
193.44 |
108.29 |
5 |
FLS |
518.468 |
−3.4171 |
66 |
14 |
8 |
225.06 |
116.02 |
5 |
PCA |
154.121 |
0.796 |
17 |
3 |
3 |
77.76 |
37.45 |
4 |
SPA |
224.212 |
1.5072 |
28 |
4 |
2 |
75.99 |
58.12 |
4 |
SYA |
198.174 |
1.1076 |
24 |
4 |
2 |
75.99 |
48.41 |
4 |
pCmA |
164.16 |
1.49 |
20 |
2 |
2 |
57.13 |
45.13 |
5 |
Table 4 Molecular docking score, binding affinity values, no. of interacting residues, common interacting residues, and no. of hydrogen bonds for the binding of bioactive compounds of black rice bran with α-glucosidase enzyme
Ligands |
Docking score (kcal mol−1) |
Binding affinity (kcal mol−1) |
No. of interacting residues |
No. of common interacting residues |
No of H-bonds |
COG |
−12.872 |
−92.47 |
23 |
12 |
5 |
FLS |
−11.925 |
−58.91 |
24 |
13 |
10 |
α-D-Glucopyranose |
−9.304 |
−26.22 |
16 |
16 |
7 |
SPA |
−5.858 |
−30.86 |
16 |
8 |
3 |
PCmA |
−4.382 |
−15.97 |
9 |
0 |
2 |
SYA |
−4.315 |
−23.29 |
16 |
13 |
2 |
PCA |
−3.773 |
−8.65 |
10 |
0 |
3 |
Molecular docking analysis. Molecular docking study was performed with six important compounds to study the mode of their interaction with the active site of the enzymeα-glucosidase from Saccharomyces cerevisiae (PDB id: 3A4A) which bears 84% similarity to human α-glucosidase. The selected ligands showed a comparable docking scores and binding affinity values (Table 4).
Molecular docking analysis of the compounds with α-glucosidase. The analysis revealed that the native ligand α-D-glucopyranose can bind to the active site of the enzyme forming seven H bonding interactions with six amino acid residues (Hie112, Asp215, Arg213, Asp352, Arg442, Asp69) of the target enzyme (Fig. 4(a)) (Table 5).
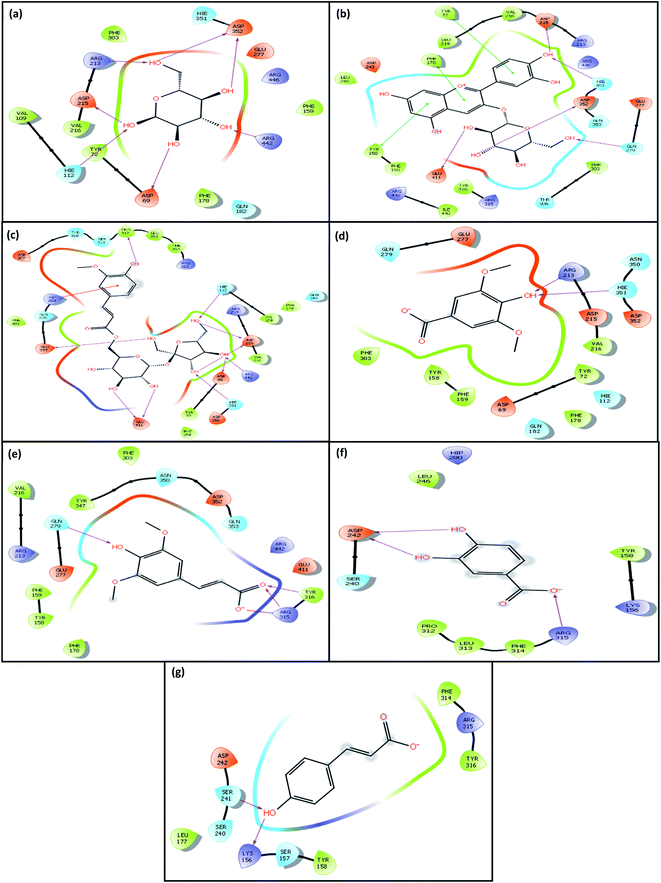 |
| Fig. 4 Amino-acid residues in the binding pocket of α-glucosidase enzyme involved in the interactions of with (a) native ligand α-D-glucopyranose (b) COG (c) FLS (d) SYA (e) SPA (f) PCA (g) p-CmA respectively. | |
Table 5 H-bond forming residues, π–π stacking interaction, and interacting residues along with their bond distances in the binding of bioactive compounds of black rice bran with α-glucosidase enzyme
Compound |
H-bond |
π–π interactions |
Amino acid residues involved in interaction |
Residues |
Bond distance (Å) |
Residues |
Bond distance (Å) |
COG |
Glu411 |
2.04 |
Tyr158, Tyr72, Phe178 |
3.94, 4.19, 5.24 |
Leu246, asp242, phe178, leu219, tyr72, val216, asp215, arg213, arg446, hie351, asp352, gln353, gln279, glu277, phe303, thr306, arg315, tyr316, ile440, arg442, glu411, tyr158, phe159 |
Asp352 |
1.97 |
Gln279 |
1.73 |
Hie351 |
1.87 |
Asp215 |
1.82 |
FLS |
Glu277 |
1.77 |
Hip280 |
4.51 |
Phe303, glu277, gln279, hip280, asp307, thr310, ser311, pro312, leu313, phe314, arg315, hie112, val101, phe178, gln182, val109, arg213, asp215, val216, arg442, asp69, tyr72, phe159, glu411 |
Pro312 |
1.94 |
Hie122 |
2.22 |
Asp215 |
1.81 |
Asp215 |
1.79 |
Hie351 |
1.88 |
Asp69 |
1.85 |
Arg442 |
1.88 |
Glu411 |
2.56 |
Glu411 |
1.97 |
PCA |
Asp242 |
1.89 |
|
|
Ser240, asp242, pro312, leu313, phe314, arg315, lys156, tyr158, leu246, hip280 |
Asp242 |
1.93 |
Arg315 |
2.24 |
SYA |
Arg213 |
2.05 |
|
|
Phe303, tyr158, phe159, asp69, tyr72, gln182, phe178, hie112, val216, asp215, arg213, asp352, hie351, asn350, gln279, glu277 |
Hie315 |
2.34 |
SPA |
Gln279 |
1.93 |
|
|
Phe178, tyr158, phe159, arg213, val216, glu277, gln279, tyr347, asn350, asp352, gln353, phe303, arg442, glu411, tyr316, arg315 |
Tyr316 |
1.85 |
Arg315 |
1.90 |
pCmA |
Ser241 |
1.93 |
|
|
Leu177, ser240, asp242, lys156, ser157, tyr158, tyr316, arg315, phe314 |
Lys156 |
1.69 |
α-D-Gluco pyranose |
Hie112 |
2.31 |
|
|
Val109, hie112, asp69, tyr72, val216, asp215, arg213, phe303, hie351, asp352, glu277, arg446, phe159, arg442, phe178, gln182 |
Asp215 |
1.66 |
Arg213 |
1.97 |
Asp352 |
1.85 |
Asp352 |
1.86 |
Arg442 |
1.72 |
Asp69 |
1.80 |
The docking analysis of COG with α-glucosidase enzyme revealed that the COG ligand can bind to the active site of the enzyme through formation of H-bonding as well as π–π stacking interactions in addition to the electrostatic interactions as shown in the figures (Fig. 4(b)). The ligand COG is involved in H bonding interactions with five residues (Glu411, Asp352, Gln279, Hie351 and Asp215) and π–π stacking interactions with three residues (Tyr158, Tyr72 and Phe178) of the target enzyme (Table 5). The docking score and binding affinity values are higher than the native ligand (Table 3). This indicates that COG can competitively inhibit the binding site of the enzyme by interacting with majority of the amino acids in the binding pocket. This is evident from the finding that out of the 23 amino acids interacting with COG, 12 amino acids are also found to be present at the binding site of the native ligand (Table 3).
The docking analysis of FLS with the same enzyme revealed that the ligand can bind to the active site of the enzyme through formation of H-bonding as well as π–π stacking interactions as shown in the figures (Fig. 4(c)). The ligand shows ten H bonding interactions with eight different amino acid residues (Glu277, Pro312, Hie112, Asp69, Arg442, Asp215, Hie351, and Glu411) and one π–πstacking interaction with one residue (Hip280) of the target enzyme (Table 5). The docking score and binding affinity values are higher than the native ligand. This molecule is also capable of showing competitive inhibition as 81% of the amino acid residues present in the binding site of the native ligand α-glucopyranose are found to interact with FLS (Table 3).
The ligand SYA binds to the active site of the enzyme through formation of H-bonding as well as π–π stacking interactions as shown in the figures (Fig. 4(d)). The ligand SYA involved in two H bonding interactions with two residues (Arg213, Hie315) of the target enzyme (Table 5).
The docking analysis of SPA revealed that the ligand binds to the enzyme through formation of H-bonding as well as π–π stacking interactions as shown in the figures (Fig. 4(e)). However, the binding affinity and docking score were found to be lesser than the other two (Table 3). Moreover, only eight amino acids interacting with the molecule are from the binding site of the native molecule.
The molecules PCA and pCmA can also bind to the enzyme through formation of H-bonding as well as π–π stacking interactions as shown in the figures (Fig. 4(f) and (g) respectively). However, the binding does not involve any common amino acid residues. This indicates that the molecules probably bind to allosteric sites of the enzyme.
Conclusion
The present study used combined in vitro and in silico approaches to evaluate the α-glucosidase inhibitory activity of black rice bran extract. For the in vitro study fresh extracts of black rice bran was used. On the other hand six bioactive compounds isolated from black rice bran extract were chosen to measure their interaction with a-glucosidase enzyme. Induced fit docking, molecular dynamics simulation, ADMET properties prediction were carried out as component of the in silico study. The aim of the study was to investigate any possible role of these compounds in the reported antidiabetic activity of black rice bran extract. The in vitro α-glucosidase inhibition assay showed that the polar extracts of the black rice bran possess high inhibitory effect on α-glucosidase comparable to the clinically used drug Acarbose. The results obtained from in silico studies for ADMET property predictions and drug likeness of these compounds suggested that these compounds have the ability or potential to be used as a lead molecule for the synthesis of new oral drug for diabetic patients. The results of the docking studies indicated that out of the six compounds selected for the study, two compounds showed high α-glucosidase inhibitory activity comparable to the native ligand α-D-glucopyranose used as the standard in this study. The molecular dynamics simulation study, which indicated formation of a number of hydrogen bonds between the compounds and the enzyme, further supported the formation of stable ligand–protein complex through the binding. The studies also indicated that these compounds can lead to the inhibition of the activity of the enzyme α-glucosidase which may be a possible mechanism of the antidiabetic effect exhibited by black rice bran extract. Thus, the preliminary studies identified a few phenolic acids as bioactive compounds as the probable leads for further studies.
Author contributions
Pranjal Bhuyan: conceptualization, methodology, software, writing-original draft, Mausumi Ganguly: writing-review & editing, supervision, Indrani Baruah: software, Gargi Borgohain: software, Jnyandeep Hazarika: validation, Shruti Sarma: validation.
Conflicts of interest
There are no conflicts to declare.
References
- P. Bougnères and A. J. Valleron, J. Exp. Med., 2008, 205, 2953–2957 CrossRef PubMed.
- Q. Shang, J. Xiang, H. Zhang, Q. Li and Y. Tang, PLoS One, 2013, 8, 8–12 Search PubMed.
- F. Moradi-Afrapoli, B. Asghari, S. Saeidnia, Y. Ajani, M. Mirjani, M. Malmir, R. D. Bazaz, A. Hadjiakhoondi, P. Salehi, M. Hamburger and N. Yassa, Daru, J. Pharm. Sci., 2012, 20, 2–7 CrossRef PubMed.
- N. D. T. Nguyen and L. T. Le, Adv. Nat. Sci.: Nanosci. Nanotechnol., 2012, 3, 013001 Search PubMed.
- P. J. Ochieng, T. Sumaryada and D. Okun, Asian J. Pharm. Clin. Res., 2017, 10, 392–398 CrossRef CAS.
- T. Matsui, C. Yoshimoto, K. Osajima, T. Oki and Y. Osajima, Biosci., Biotechnol., Biochem., 1996, 60, 2019–2022 CrossRef CAS.
- H. Laoufi, N. Benariba, S. Adjdir and R. Djaziri, J. Appl. Pharm. Sci., 2017, 7, 191–198 CAS.
- S. Liu, Z. Yu, H. Zhu, W. Zhang and Y. Chen, BMC Complement. Altern. Med., 2016, 16, 1–8 Search PubMed.
- G. Oboh, A. T. Isaac, A. J. Akinyemi and R. A. Ajani, Int. J. Biomed. Sci., 2014, 10, 210–218 Search PubMed.
- S. Önal, S. Timur, B. Okutucu and F. Zihnioǧlu, Prep. Biochem. Biotechnol., 2005, 35, 29–36 CrossRef.
- M. Pandithurai, S. Thennarasan and S. Thennarasan, Int. J. Adv. Pharm., 2019, 4, 2–6 Search PubMed.
- B. Elya, K. Basah, A. Mun’Im, W. Yuliastuti, A. Bangun and E. K. Septiana, J. Biomed. Biotechnol., 2012, 2012, 1–6 CrossRef.
- R. Subramanian, M. Z. Asmawi and A. Sadikun, Acta Biochim. Pol., 2008, 55, 391–398 CrossRef CAS.
- C. Chaiyasut, B. S. Sivamaruthi, N. Pengkumsri, W. Keapai, P. Kesika, M. Saelee, P. Tojing, S. Sirilun, K. Chaiyasut, S. Peerajan and N. Lailerd, Pharmaceuticals, 2017, 10, 3 CrossRef.
- A. L. D. S. Dias, B. Pachikian, Y. Larondelle and J. Quetin-Leclercq, Curr. Opin. Clin. Nutr. Metab. Care, 2017, 20, 470–476 CrossRef CAS.
- A. S. Wahyuni, R. Munawaroh and M. Da'i, Natl. J. Physiol., Pharm. Pharmacol., 2016, 6, 106–110 CrossRef CAS.
- S. M. Boue, K. W. Daigle, M. H. Chen, H. Cao and M. L. Heiman, J. Agric. Food Chem., 2016, 64, 5345–5353 CrossRef CAS PubMed.
- H. J. Eun, R. K. Sung, K. H. In and Y. H. Tae, J. Agric. Food Chem., 2007, 55, 9800–9804 CrossRef.
- K. Pitija, M. Nakornriab, T. Sriseadka, A. Vanavichit and S. Wongpornchai, Int. J. Food Sci. Technol., 2013, 48, 300–308 CrossRef CAS.
- H. X. Zheng, S. S. Qi, J. He, C. Y. Hu, H. Han, H. Jiang and X. S. Li, J. Agric. Food Chem., 2020, 68, 4399–4410 CrossRef CAS PubMed.
- A. Ghasemzadeh, M. T. Karbalaii, H. Z. E. Jaafar and A. Rahmat, Chem. Cent. J., 2018, 12, 1–13 CrossRef.
- Y. Shao, F. Xu, X. Sun, J. Bao and T. Beta, J. Cereal Sci., 2014, 59, 211–218 CrossRef CAS.
- A. H. K. Su Tian and K. O. Z. O. Nakamura, J. Agric. Food Chem., 2004, 52, 4808 CrossRef.
- Y. Pang, S. Ahmed, Y. Xu, T. Beta, Z. Zhu, Y. Shao and J. Bao, Food Chem., 2018, 240, 212–221 CrossRef CAS PubMed.
- Y. Nurnaistia, S. Aisyah, H. S. H. Munawaroh and Zackiyah, J. Phys.: Conf. Ser., 2018, 1013, 012204 CrossRef.
- P. Bhuyan, S. Sarma, M. Ganguly, J. Hazarika and R. Mahanta, J. Mol. Struct., 2020, 1222 Search PubMed.
- X. Li, Z. R. Lü, W. Wang, X. P. Han, J. M. Yang, Y. D. Park, H. M. Zhou, Q. Sheng and J. Lee, Process Biochem., 2015, 50, 582–588 CrossRef CAS.
- M. Taha, S. A. A. Shah, M. Afifi, S. Imran, S. Sultan, F. Rahim and K. M. Khan, Bioorg. Chem., 2018, 77, 586–592 CrossRef CAS PubMed.
- M. Rashid, Bioorg. Chem., 2020, 96, 103576 CrossRef CAS.
- C. A. Lipinski, Drug Discovery Today: Technol., 2004, 1, 337–341 CrossRef CAS.
- A. M. Udrea, G. Gradisteanu Pircalabioru, A. A. Boboc, C. Mares, A. Dinache, M. Mernea and S. Avram, Biomolecules, 2021, 11, 1–31 CrossRef.
- Y. M. Kim, Y. K. Jeong, M. H. Wang, W. Y. Lee and H. I. Rhee, Nutrition, 2005, 21, 756–761 CrossRef CAS.
- M. R. Bhandari, N. Jong-Anurakkun, G. Hong and J. Kawabata, Food Chem., 2008, 106, 247–252 CrossRef CAS.
- A. Gholamhoseinian, H. Fallah and F. Sharifi far, Phytomedicine, 2009, 16, 935–941 CrossRef CAS PubMed.
- A. N. Spiess and N. Neumeyer, BMC Pharmacol., 2010, 10, 1–11 Search PubMed.
- J. L. Sebaugh, Pharm. Stat., 2011, 10, 128–134 CrossRef CAS PubMed.
- M. Bordoloi, P. K. Bordoloi, P. P. Dutta, V. Singh, S. Nath, B. Narzary, P. D. Bhuyan, P. G. Rao and I. C. Barua, J. Funct. Foods, 2016, 23, 220–229 CrossRef CAS.
- S. Nath, K. J. Tamuli, B. Gogoi, M. Bordoloi, A. Das, C. C. Barua and I. C. Barua, Eur. J. Integr. Med., 2020, 40, 101247 CrossRef.
- J. Wairata, E. R. Sukandar, A. Fadlan, A. S. Purnomo, M. Taher and T. Ersam, Biomedicines, 2021, 9, 3390 CrossRef.
- D. E. V. Pires, T. L. Blundell and D. B. Ascher, J. Med. Chem., 2015, 58, 4066–4072 CrossRef CAS.
- D. A. Case, R. C. Walker, T. E. Cheatham, C. Simmerling, A. Roitberg, K. M. Merz, R. Luo and T. Darden, Amber 2018, Univ. California, San Fr., 2018, pp. 1–923 Search PubMed.
- J. Wang, R. M. Wolf, J. W. Caldwell, P. A. Kollman and D. A. Case, J. Comput. Chem., 2004, 56531, 1157–1174 CrossRef.
- W. Wang, N. Gan, Q. Sun, D. Wu, R. Gan, M. Zhang, P. Tang and H. Li, Spectrochim. Acta, Part A, 2019, 219, 83–90 CrossRef CAS PubMed.
|
This journal is © The Royal Society of Chemistry 2022 |
Click here to see how this site uses Cookies. View our privacy policy here.