DOI:
10.1039/D2SD00073C
(Paper)
Sens. Diagn., 2022,
1, 851-866
SERS-based assay for multiplexed detection of cross-reactivity and persistence of antibodies against the spike of the native, P.1 and B.1.617.2 SARS-CoV-2 in non-hospitalised adults†
Received
26th April 2022
, Accepted 14th June 2022
First published on 23rd June 2022
Abstract
Monitoring antibody response to SARS-CoV-2 is critical for assessing the humoral response, especially important considering the emergence of multiple SARS-CoV-2 variants of concern (VOCs). Herein, we developed rapid and highly sensitive microfluidics-integrated multiplexed SERS to simultaneously screen multiple anti-spike immunoglobulin isotypes (IgG, IgA and IgM) to establish the level of cross-reactivity and the persistence of anti-spike immunoglobulins in immune patient sera for the native, P.1 and B.1.617.2 strains of SARS-CoV-2 virus. The study was performed on 24 non-hospitalised adults with laboratory diagnosed COVID-19 and had fully recovered before the emergence of the P.1 and B.1.617.2 mutants. We report seroconversion and cross-protection of IgG, IgA and IgM antibodies against the spike proteins of the native SARS-CoV-2, and the P.1 and B.1.617.2 VOCs in sera collected longitudinally at 3 weeks and 8 weeks following a PCR-positive test. Although high levels of IgG, IgA and IgM were detected against the native strain, immune responses of cross-reactive binding antibodies against the spike protein of the VOCs decreased significantly. Our study revealed that in addition to exhibiting the highest seropositivity rates (>97%), IgG responses were maintained up to 8 weeks post-diagnosis, irrespective of the tested spike protein. In contrast, the relatively high seropositivity rates of IgA and IgM (>86% and >80%, respectively) detected at 3 weeks post diagnosis decayed rapidly, approaching baseline by week 8 post-diagnosis, and this observation was congruent with binding affinities of IgA and IgM. We also demonstrate that the levels of anti-spike antibodies correlated with patient age, with the oldest individuals (>70 years) displaying highest antibody binding responses across the spike antigens. Collectively, our results illustrate the potential applicability of multiplexed SERS assays to screen past COVID-19 and to assess cross-protective humoral immunity against VOCs.
Introduction
The global pandemic caused by the severe acute respiratory syndrome coronavirus 2 (SARS-CoV-2), the causative agent of the 2019 novel coronavirus disease (COVID-19), has been exacerbated by the emergence of viral mutations that drove rapid upsurges in infection transmission rates and case counts in subsequent waves. As of February 1st 2022, SARS-CoV-2 virus had infected about 379 million people, leading to the death of over 5.6 million people globally since its origin in Wuhan.1 Detection of the genetic material of SARS-CoV-2 virus using polymerase chain reaction (PCR), alongside massive vaccination campaigns have proven the most effective means of managing the COVID-19 pandemic. Due to its high sensitivity and specificity, PCR molecular testing has been adopted as the gold standard tool for detecting active cases, identifying the viral strain and isolating contagious people. However, as the pandemic evolved, large populations recovered from COVID-19 and/or have been vaccinated, thereby resulting in a complex immune landscape. This raises important questions concerning cross-protection of convalescent serum against re-infection with the native strain and/or circulating variants.
In light of this situation, the focus of current epidemiological assessments has shifted towards understanding the presence and durability of COVID-19-specific functional protective immunity over time. In general, patients infected with SARS-CoV-2 develop IgA and IgM antibodies against viral antigens within 7–14 days after viral infection, which wane over time.2 By contrast, IgG antibodies are produced 10–25 days post infection. The peak titres for IgG are usually sustained for several months after viral clearance, linking IgG with potential long-term immunity.3 Understanding the dynamics in antibody levels and persistence offers vital insights into the scale of protection that antibodies provide against secondary infection(s) with SARS-CoV-2.
The humoral response of variant-naïve sera to the P.1 and B.1.617.2 variants of concern (VOCs) has been extensively studied within the course of the COVID-19 pandemic. Interestingly, a recent study illustrated that sera of non-hospitalised adults who recovered from an infection with the native SARS-CoV-2 retained neutralisation activity against tested VOCs, and that neutralisation capacity increased significantly after the subjects were vaccinated.4 This finding re-emphasised the need to track the state of immunity against SARS-CoV-2 in order to assess the probability of re-infection with the native strain or emerging VOCs. PCR testing is not suited to the detection of immunity, leading to an urgent demand for alternative assays that probe seroconversion.5,6 Serology is well-suited for clinical studies aimed at monitoring past infections, precise rate of infection circulation and durability of protective antibodies;7,8 and these factors remain vital priorities in the post-vaccination era.9,10
Various serological assays such as chemiluminescence and surface plasmon resonance (SPR) that probe the host humoral immunity against SARS-CoV-2 and VOCs have been widely reported.11–13 Amongst these immunoassays, enzyme-linked immunosorbent assay (ELISA) is the gold standard for measuring virus-specific IgG, IgA and IgM titres in blood plasma.14–16 Though ELISA enables accurate identification of patients who have developed COVID-19-specific antibodies, there is a need to apply complementary biochemical assays that allow for rapid and accurate simultaneous analysis of multiple antibody–antigen interactions in serum to enable timely intervention of appropriate healthcare.17,18
Surface-enhanced Raman scattering (SERS)-based immunoassays have gained a great deal of attention for simultaneous evaluation of multiple analytes,19,20 including protective antibodies against SARS-CoV-2 virus.21,22 What distinguishes SERS from other assays for multianalyte detection is its intrinsic molecular specificity, well-resolved spectral peaks and excellent analyte detection sensitivity,23 making SERS attractive for biochemical sensing in complex biofluids.24–26 However, the application of multiplexed SERS for serology is often held back by poor analyte resolution due to chemical complexity of clinical samples. Combining SERS with microfluidics27 or lateral flow immunoassay (LFIA)21,28 has shown potential for rapid and robust simultaneous multiplexing of clinical biomarkers at high resolution directly in serum or plasma without the need for prior analyte treatment or separation steps.29,30
Currently, few studies have reported multiplexed SERS for COVID-19 serology.21,22,28 Liu et al. employed SERS-based LFIA to identify anti-spike IgG and IgM simultaneously with >90% clinical sensitivity and specificity.21 More recently, Srivastav and co-workers accurately detected anti-RBD IgG and IgM antibodies by utilising the SERS-LFIA approach, which outperformed a conventional LFIA.28 However, most of these SERS studies focused on duplex biosensing of only IgG and IgM targeting the spike/RBD of the native SARS-CoV-2, which may not be fully representative of the humoral immunity. Importantly, current studies have not demonstrated the ability of SERS to screen the potential transfer of immunity to circulating VOCs such as the P.1 and B.1.617.2, which exacerbated the pandemic.
In this study, we employed microfluidics-integrated multiplexed SERS for screening a panel of three antibody isotypes (IgG, IgA and IgM) against spike proteins in non-hospitalised adults with mild COVID-19 induced by the native SARS-CoV-2, a group of patients that is understudied to date. Most research reports on COVID-19 immunity focused on vulnerable populations (i.e. children, healthcare workers, elders) and when adults were studied, it was mainly for more severe infection cases requiring hospitalisation. Our SERS format combined the plasmonic effects SPR on gold-coated chip and cross-section of a Raman reporter located in the vicinity of localised surface plasmon resonance (LSPR) field of AuNPs. Coupled plasmonic effects increased the sensitivity and reproducibility of measurements, as shown with the detection of antibodies in non-hospitalised adults following a PCR-positive COVID-19 diagnostics, yielding rapid multiplex results with turnaround time of ∼35 min. The resulting multivariate data enabled by the Raman reporter allowed assessment and validation of clinical results via univariate and multivariate analyses, as opposed to SPR readout that is univariate in nature, and ELISA that often takes more than 4 h to yield clinical results.
This is the first SERS study to simultaneously examine multiple cross-reactivity and longevity of three isotypes (IgG, IgA and IgM) directed against the spike of the native SARS-CoV-2, and P.1 and B.1.617.2 VOCs in a single serum sample. To accomplish simultaneous multiplexed analysis, the native and variant spike antigens were immobilised within four spatially separated channels on the sensor chip surface using the microfluidic cell of a portable SPR device. Detection of anti-spike antibodies was performed in distinct microfluidic cell chambers using three types of SERS-active nanotags (i.e., AuNPs functionalised with the same Raman reporter molecule and either IgG, IgA or IgM capture antibody) prior to SERS analysis. Our detection assay format generated SERS spectral datasets that identified seropositive patients with high clinical sensitivity and specificity. We provide evidence and validation of cross-reaction of sera elicited by the native SARS-CoV-2 against the P.1 and B.1.617.2 VOCs we have shown for IgG using ELISA and SPR.4 Here, we also highlight the role of IgA and IgM antibodies in humoral immune response, as well as correlation between antibody responses and patient age. Our study underscores the ability of SERS to rapidly probe waning cross-reactive antibody isotypes against circulating VOCs, stressing the need for vaccination and/or boosters in mildly infected adults.
Experimental methods
Materials
Gold(III) chloride trihydrate, 5,5′-dithio-bis(2-nitrobenzoic acid), poly(ethylene glycol) (2 kDa), N-ethyl-N′-(3-dimethylaminopropyl)carbodiimide hydrochloride, Tween20, trisodium citrate, bovine serum albumin, N-hydroxysuccinimide, human AB serum (cat. no. H4522) and phosphate-buffered saline and ethanolamine hydrochloride were obtained from Sigma Aldrich. Goat anti-human IgG, IgA and IgM antibodies were purchased from the Jackson Immunoresearch laboratory. The native his-tagged spike protein was produced and purified as described before.31 The his-tagged B.1.617.2 variant spike protein was expressed by transient gene expression in CHO cells32 and purified by affinity chromatography on NGL COVID-19 Spike Protein Affinity Resin (Repligen, Waltham, MA, USA). Finally, the tagless P.1 spike protein was expressed from a stable CHO pool and then purified using a proprietary 3-step chromatographic step. All proteins were >98% pure as assessed by SDS-PAGE and Coomassie staining and showed >98% trimers (native and P.1 spikes) or a mixture of 26% hexamers and 71% trimers (B.1.617.2 spike) by SEC-UPLC analysis on a BEH450 column.
Synthesis of AuNPs
All glassware used in the synthesis of AuNPs were pre-cleaned using aqua regia (3
:
1 HCl
:
HNO3) and washed thoroughly with deionised water. The glassware was left to dry at room temperature before use. AuNPs were synthesised using the kinetically controlled seed-mediated growth strategy involving step-wise increase of pre-synthesised citrate-reduced AuNP seeds.33 Briefly, 150 mL of 2.2 mM trisodium citrate aqueous solution was transferred into a 2-necked round bottomed flask and heated to boiling on an oil bath with vigorous stirring using a magnetic stirrer bar. A Liebig condenser was used to prevent evaporation of the citrate solution. Next, 1 mL of 25 mM HAuCl4 aqueous solution was injected into the boiling citrate solution, which resulted in colour changes from clear citrate solution to blue tint and finally to light pink, indicating the formation of Au seeds. The suspension was left boiling for 10 min before the temperature was adjusted to 90 °C while keeping the solution stirring. The reaction temperature was reduced to 90 °C to slow down the reaction and to minimise the formation of secondary Au nuclei during seed growth steps, which led to the production of nanoparticles of high stability and morphology.33 Afterwards, colloidal seeds were heated to boiling for optimal reaction after which 1 mL of 25 mM HAuCl4 was injected and temperature adjusted as discussed above. Stepwise injection of HAuCl4 and temperature adjustments were repeated five times to achieve suitable AuNP size. The final burgundy colloid was cooled to room temperature and kept at 4 °C before subsequent functionalisation.
Preparation and functionalisation of SERS-active nanotags
Three types of SERS nanotags, each functionalised with IgG, IgA or IgM secondary antibodies targeting human anti-spike antibodies against the native SARS-CoV-2 strain, and the P.1 and B.1.617.2 VOCs, were prepared in this study. Firstly, colloidal AuNPs were washed once by centrifugation at 4100 rpm for 15 min. The supernatant was removed and the pellet resuspended in 30 mL of Milli-Q water (18.2 MΩ cm−1 at 25 °C). Then, 5,5′-dithio-bis(2-nitrobenzoic acid) (DTNB) Raman reporter was added to 30 mL of 0.5 nM AuNPs to a final concentration of 10 μM and left on an orbital shaker (Forma Scientific Inc., USA) shaking at 150 rpm for 30 min. The suspension was removed from the shaker and then centrifuged at 4100 rpm for 15 min. After discarding the supernatant, the pellet was resuspended in Milli-Q water. At this stage, the AuNP–DTNB conjugates were divided into three equal volumes of 10 mL each and transferred into three separate 15 mL Falcon tubes (Fisher Scientific, USA), one for each of the IgG, IgA and IgM nanotags. For antibody binding, 25 μg mL−1 of each of the AffiniPure goat anti-human IgG (H + L), IgA or IgM antibodies was incubated with the AuNP–DTNB conjugates in the appropriate tubes and kept on a shaker overnight at room temperature under the shaking set up explained above. The following morning, 100 μL of thiolated poly(ethylene glycol) (2 kDa) (PEG2000, 1 mM) was added to AuNPs–DTNB-antibody mixtures as backfill for the remaining bare NP surfaces, vortexed and shaken further for 1 h before being centrifuged at 2000 rpm for 10 min. The pellets were kept and the supernatant centrifuged again at 4500 rpm for 15 min. The supernatant was discarded whilst the pellets from the first and second centrifugation were combined and resuspended in 10 mL of phosphate-buffered saline (PBS, 10 mM, pH 7.4) containing 0.1% bovine serum albumin (BSA). The nanotags were washed twice to remove unreacted chemicals and resuspended in PBS–BSA (10 mM, pH 7.4) ready for use. It should be noted that each of the three synthesised nanotag was specific for the anti-spike IgG, IgA or IgM target infection biomarkers.
Characterisation of SERS nanotags
UV-visible extinction spectra of bare AuNPs and SERS nanotags were acquired using a Cary Bio 100 spectrophotometer equipped with a 350–900 nm wavelength spectral range. The hydrodynamic diameter was measured using a Zetasizer nano ZS dynamic light scattering (DLS) instrument equipped with 633 nm excitation whilst zeta potential was examined with Malvern ZS Nano Analyser (Malvern Instruments). Morphology of nanoparticles was probed with a Phillips Technai 12 transmission electron microscopy operating at an accelerating voltage of 120 kV.
Clinical samples and ethics approval
Adult volunteers were recruited by the Centre Hospitalier Universitaire de Québec – Université Laval (CHUL) in Quebec City, Canada following ethical approval reported recently.34 The study was approved by the “Comité d'éthique de la recherche du CHU de Québec – Université Laval” (registration number 2021-5241). Patients were included after written informed consent, and patient sera used in this study were sampled from the same cohort of patients as those investigated in our recent study.34 All the volunteers were 18 years or older and had received a PCR-positive diagnostic for COVID-19 between 2 and 3 weeks prior to serum collection. The volunteers did not show symptoms of fever or dyspnea for at least 48 h prior to sampling, with little or no cough. The 24 volunteers included in this study were stratified by age (18–49, 50–59, 60–69 and 70+ years old); each group comprised six randomly selected participants. All the patients had mild COVID-19 and were not hospitalised at the time of sera sampling. Negative controls were collected from individuals who never received a COVID-positive test. Blood samples were anonymised and collected in 6 mL tubes (BD Vacutainer 367
815). The tubes were gently inverted, held at room temperature for 15–30 min and spun at 1600 g for 15 min. Serum (1 mL aliquots) was transferred into cryovials (Sarstedt Inc., product 72.694.006) and frozen in an upright position at −20 °C until the batch was sent to the Montreal laboratories on dry ice. All serum samples were stored at −80 °C until use. During experimental analysis, serum samples were kept on ice.
Immobilisation of the native, P.1 and B.1.617.2 spike proteins for multiplexed analysis
All experiments on patient serum samples were conducted in a laminar flow biological safety cabinet in biosafety level 2 laboratory (BSL 2) following standard biosafety protocols and guidelines.
The native, P.1 and B.1.617.2 spike antigens were conjugated onto a commercially available gold-coated sensor chip via cross-coupling reactions between spike and 3-mercaptopropionic-Leu-His-Asp-Leu-His-Asp-COOH peptide (3-MPA-LHDLHD-COOH, Afficoat, Affinité Instruments, Canada). The solid-phase sensor was constructed in a microfluidic cell consisting of a four-independent channel SPR device (Affinité Instruments, Canada) to allow for sensing of multiple and distinct antibody–spike binding interactions in a single sample within four spatially resolved fluidic channels.34 In all cases chemicals and serum samples were injected into fluidic channels using sterile syringes. Briefly for the cross-linking reaction, a 1
:
1 aqueous solution of N-hydroxysuccinimide (NHS, 100 mM) and N-ethyl-N′-(3-dimethylaminopropyl)carbodiimide hydrochloride (EDC, 400 mM) was reacted with the peptide-coated sensor before 200 μL of each of the spike for the native strain, and the P.1 and B.1.617.2 mutants were immobilised at a concentration of 20 μg mL−1 in the acetate buffer (10 mM, pH 4.5). The sensor surface was washed sequentially with 300 μL of ethanolamine (1 M, pH 8.5) and the running buffer comprising PBS (10 mM, pH 7.4) supplemented with 0.1% BSA and 0.005% Tween20 before it was equilibrated with 200 μL of commercial serum diluted 5× in running buffer. Clinical sera (n = 32) were diluted 5× in the running buffer and randomised prior to injection onto the sensor surface as we recently reported.34 In a single injection run, the same serum sample (200 μL) was simultaneously injected into three separate sample microfluidic channels to induce distinct antibody–spike interactions. Secondary detection was performed with AuNPs conjugated with 200 μL of the goat anti-human IgG (H + L), IgA or IgM antibodies (so-called nanotags). After a quick wash with 300 μL of running buffer, the chip was carefully removed from the SPR device cavity and placed on a motorised sample stage under the Raman microscope for SERS spectral acquisition. It should be emphasised that an SPR instrument used in this study consisted of four independent channels on a microfluidic cell. So, the spike proteins of the native strain, and the P.1 and B.1.617.2 variants were immobilised in distinct spatially resolved channels of a fluidic cell to allow simultaneous triplex detection of antibody–spike cross-reactions in the same sample. Note that the running buffer was injected in the fourth channel to act as a blank control.
SERS spectral analysis of the detection assay
The same Raman label molecule 5,5′-dithio-bis(2-nitrobenzoic acid) (DTNB) was employed in all SERS-active nanotags, which were specific for anti-spike IgG, IgA and IgM antibodies targeting native and variant spike proteins. After a secondary detection step for the antibody–nanotag conjugates, SERS spectra were immediately recorded using an alpha300 Raman microscope (WITec, Germany) equipped with 633 nm HeNe excitation laser, grating of 300 grooves per cm and a CCD camera. For all spectral analyses, laser power on the sample was adjusted to ∼2 mW with an exposure time of 3 s for five accumulations per signal. A 20× LWD (long working distance) microscope objective (NA = 0.30) was used to view samples on the sensor surface and collect back-scattered photons. A static scan mode was used to acquire four spectral replicates from different positions of the same clinical sample, each analysed in triplicates. Instrument control and data capture were achieved using the Control 5 software.
Data processing and chemometrics
Statistical analyses were conducted in Matlab software 2021b (The MathWorks Inc., Natwick, US) and GraphPad Prism (version 9.3.0). Univariate analysis was performed through the regression of a single SERS vibrational mode for DTNB reporter detected at 1329 cm−1 in all the samples. Statistical significance tests for univariate datasets were carried out using parametric two-tailed t-tests at the 95% confidence intervals (CI), with all results presented as averages of spectral data for individual sample classes, and error bars represented one standard deviation. To assess diagnostic accuracy of SERS assay in clinical sera and to investigate the degree of separability between sample classes, receiver operating characteristic (ROC) curves with corresponding area under the curve (AUC) were plotted and p-values computed at 95% CI. For multivariate analysis, SERS data were subjected to baseline correction using the asymmetric least squares,35 before non-parametric tests based on principal component analysis (PCA) was applied. PCA is an unsupervised mathematical algorithm that is extensively used to reduce dimensionality of spectral data, identify outliers, and importantly, to explore natural clustering trends arising from dominant variables.36 PCA plots were constructed from each multivariate spectral dataset (32 samples in triplicates), with each data point constituting full spectral range from 400 to 1800 cm−1.
Results and discussion
Synthesis and characterisation of AuNPs nanotags
The SERS-based nanotags employed in this study were developed via stepwise conjugation of Raman reporter and capture secondary antibodies onto AuNP surfaces followed by secondary detection assay format as summarised in Fig. 1.
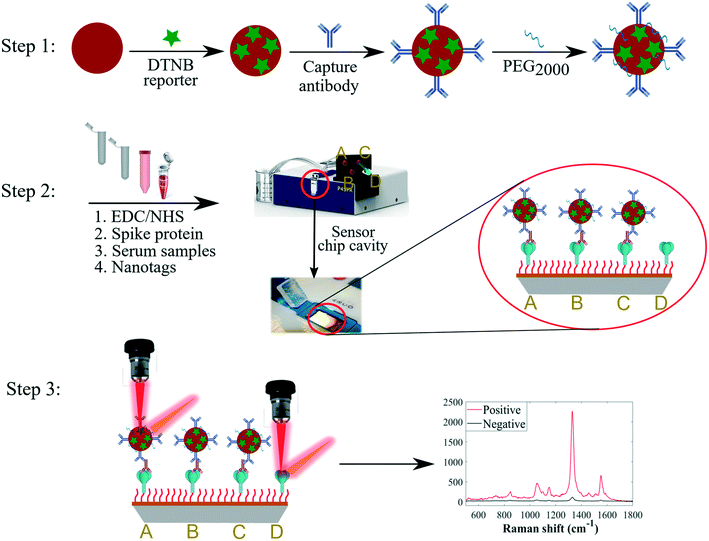 |
| Fig. 1 Schematic diagram of multiplexed SERS detection strategy. Step 1: Synthesis of SERS-active nanotags. Step 2: Immobilisation of the different types of spike proteins on gold-coated sensor surface modified with a self-assembled monolayer of 3-MPA-LHDLHD-COOH linker via EDC/NHS chemistry in spatially separated fluidic channels (A) native, (B) P.1, (C) B.1.617.2, and (D) native or variant-spike, followed by secondary detection of anti-spike antibodies with AuNP-conjugated capture antibodies. Sterile syringes were used to inject samples into channels. Step 3: Detection of immunocomplexes using SERS; strong DTNB signal (positive, red trace) was detected when target antibodies were present in patient serum in A, B and C channels whilst weak signal (negative, black trace) was measured when target antibodies were absent from sera in D channel. Note that the scheme above represents detection format for anti-spike IgG using IgG nanotags for illustrative purposes. Same synthetic routes and detection steps were employed for IgA and IgM antibodies and the respective nanotags. Nanoparticles and molecules not drawn to scale. | |
Kinetically-controlled seeded-growth method was used to synthesise plasmonic nanoparticles via sequential reduction of HAuCl4 by trisodium citrate aqueous solutions as previously reported by Bastús et al.33 According to TEM image and size distribution of nanoparticles, the synthesised AuNPs exhibited monodispersed spherical shapes with an average diameter of 40 nm as shown in Fig. 2a. Since the three different sets of nanotags (IgG, IgA and IgM) were optimised and characterised using same procedures, and that they generated comparable spectral outputs and surface properties, only the data for IgG nanotags were included in Fig. 1 for illustrative purposes. The spectral data acquired for the IgA and IgM nanotags characterisation steps are detailed in Fig. S1 (ESI†).
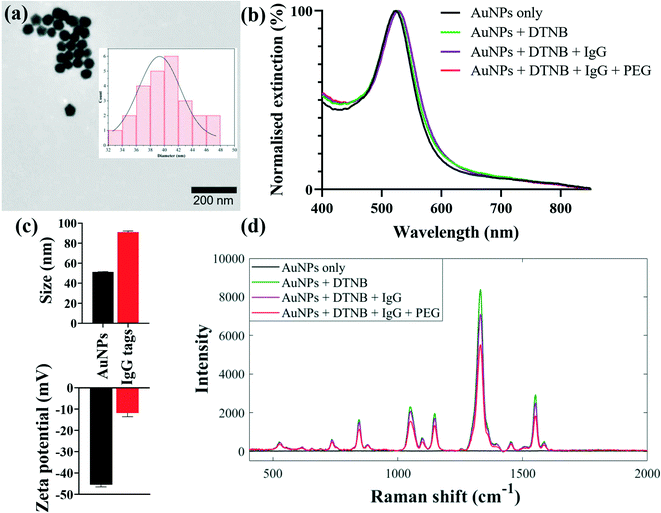 |
| Fig. 2 Characterisation of SERS nanotags for detecting multiple IgG antibody responses to spike proteins: (a) TEM image of and size distribution (insert) of 40 nm AuNPs, (b) UV-vis extinction spectra, (c) dynamic light scattering and zeta potential, and (d) representative SERS spectra, measured before and after functionalisation steps of AuNPs with DTNB Raman label and capture secondary IgG. All measurements were performed in triplicate. Error bars in (c) represent 1 × standard deviation. | |
SERS-active nanotags were developed by coating nanoparticle surfaces with DTNB via Au–S coordinate bonds followed by the immobilisation of IgG, IgA or IgM secondary capture antibodies onto DTNB-coated AuNPs (Fig. 1, step 1). DTNB reporter was employed to create strong AuNP–DTNB tags enabled by covalent binding of thiol groups to Au surfaces to avoid leaching of the Raman label in subsequent conjugation steps. This resulted in strong and stable spectra needed for multiplex detection. Secondary antibodies were conjugated directly onto AuNPs by the standard passive binding mechanism mediated by particle–antibody non-covalent interactions.37,38 This conjugation procedure reduces the number and duration of surface functionalisation steps in comparison with the traditional covalent binding approaches,21 paving the way for rapid production of stable SERS nanotags. Thiolated PEG2000 and BSA were added to AuNP–DTNB-antibody mixtures to block any bare nanoparticle surfaces and to prevent opsonisation and non-specific binding of biochemical constituents from serum samples. It is worth noting that three sets of antibody-coated nanotags each specific for anti-spike IgG, IgA or IgM were designed and each conjugation step was characterised using extinction spectroscopy, zeta potential and dynamic light scattering. Extinction spectra in Fig. 2b show plasmonic bands of AuNPs before and after surface conjugation. Compared to the λmax of plain AuNPs detected at 530 nm, the λmax of IgG-functionalised nanotags displayed a clear redshift of Δ6 nm due to change in refractive index of colloids on successful molecular adsorption onto AuNPs. Using the Bradford protein assay, the loading of IgG linked with the redshift was estimated to be ∼60 IgGs/AuNP. Details of the Bradford assay are included in Fig. S2.† Given the footprint of a typical IgG is ∼81.3 nm2,39 and an average surface area of 5.03 × 103 nm2 for 40 nm nanospheres,40 our results suggest a monolayer coverage of nanotags. The nanotags retained a single symmetrical LSPR peak in the 350–800 nm wavelength range, suggesting isotropic, monodispersed and stable nanotags devoid of any noticeable particle aggregation. Antibody conjugation was further confirmed by an increase in hydrodynamic diameter from 51 ± 0.3 nm to 91 ± 1.3 nm and corresponding decrease in zeta potential from −45 ± 1.1 mV to −12 ± 1.8 mV, as a function of changes in particle density, size and surface environment after attaching DTNB, IgG and PEG onto AuNPs (Fig. 2c). In addition, SERS data were also utilised to assess the sensitivity and stability of nanotags. As shown in Fig. 2d, SERS spectra changed at each conjugation stage presumably due to molecular attachment to AuNPs. Fig. S3† shows that the synthesised nanotags were stable at different time points and detection sensitivity was reproducible up to one month after production. Combined together, this multimodal evidence confirmed the successful adsorption of molecules onto AuNPs, and that nanotags were stable and fit for the purpose of detecting immunoreactions and antibody levels in serum samples.
Immobilisation of the native, P.1 and B.1.617.2 spike proteins for multiplexed analysis
Key to our multiplex analysis of the cross-reactivity of IgG, IgA and IgM binding to the spike proteins in a single sample was the development of a reproducible peptide-modified SPR sensor. Spike ectodomain of the native, P.1 and B.1.617.2 SARS-CoV-2 viruses were conjugated onto the gold-coated chip surface through the carbodiimide cross-linking chemistry, where an amide bond was formed between the carboxylic moiety of the 3-MPA-LHDLHD-COOH peptide and the primary amine group of spike proteins. Coupling reactions were performed in acetate buffer (10 mM, pH 4.5) to allow ideal covalent bioconjugation of spike proteins onto the self-assembled monolayer (SAM; not to be mistaken with the standard addition method that is also abbreviated with the same acronym) of the zwitterionic peptide on the sensor chip surface. Several alternative linker molecules, e.g., alkanethiols and PEG, are readily available for cross-coupling of biomolecules onto the sensor surface. However, in our bespoke study design,34 3-MPA-LHDLHD-COOH linker demonstrates superior ability to minimise non-specific interactions and surface fouling in chemically dense human fluids, whilst upholding excellent detection sensitivity in parallel. Effective coupling of spike proteins to the sensor surface was confirmed through optimally intense SPR sensorgrams measured during the coupling process, as we recently reported for SPR sensing of COVID-19-specific antibodies under similar experimental conditions.34 The sensor was ultimately washed with a running buffer to remove weakly bound capture nanotags and other residual matrices prior to SERS spectral measurements, resulting in reduced background SERS spectral signals. The total assay time from secondary detection step in a microfluidics cell to SERS signals was ∼35 min.
Principle of the multiplexed SERS detection assay
A sensitive secondary detection scheme involving binding interactions between anti-spike isotypes and SERS-active antibody-functionalised AuNPs was developed and applied for simultaneous detection of multiple antibody–spike interactions as depicted in Fig. 1. Immunoreactions occurred on the surface of a commercial sensor chip when serum was injected by lateral flow into the four inlets of microfluidic channels, each containing a different type of spike protein. It is important to note that the same Raman label DTNB was conjugated to each of the three sets of the IgG, IgA and IgM antibody-functionalised nanotags, since simultaneous detection was done in distinct parallel fluidic chambers. Previous studies have demonstrated that microfluidics cell-assisted multi-component analysis allows more rapid and reproducible multiplexing strategy than the ‘one pot’ kind of workflow, where multiple spectrally resolved Raman labels are used.41 For the latter workflow, different molecular recognition nanosensors are used to unravel differential spectral features by superimposing signals measured from multi-analyte mixture on signals acquired for corresponding individual pure analytes,42 a scheme which regularly requires computationally intensive deconvolution and multi-curve resolution algorithms.43
In our work, DTNB reporter was present only on capture antibody-conjugated nanotags, and not on the sensor chip surface, which consisted only of the spike–antibody complexes prior to secondary detection with SERS-active nanotags. For this reason, SERS spectra were detected only when anti-spike antibodies reacted with biorecognition nanotags, which were antibody specific (Fig. 1, step 2). In contrast, when target antibodies were absent in sera, capture nanotags were washed away with running buffer in the rinsing step, since AuNP-conjugated secondary antibodies did not react directly with spike proteins (Fig. 1, step 2). This left only spike-peptide conjugates on the sensor surface that were not SERS-active, and thus only generated weak background signals upon laser illumination (Fig. 1, step 3). Our DTNB-based extrinsic SERS assays exhibited highly intense and sensitive signals (Fig. 3a) since DTNB reporter possesses a large Raman scattering cross-section, making it attractive for clinical biosensing in clinical sera where biomarkers are often present at low concentrations. Fig. 3 depicts typical averaged SERS spectra of DTNB-encoded antibody–spike immunocomplexes measured from seropositive and negative control sera for anti-spike IgG target biomarker investigation using 633 nm excitation laser and 3 s signal acquisition. The asterisk (*) on the SERS spectrum indicates the major DTNB band detected at 1329 cm−1 (assigned to –NO2 stretching modes) that was applied to measure multiple cross-reactions and seroconversion. SERS data profiles detected for IgA and IgM antibodies were collected under the same experimental conditions are shown in Fig. S4.†
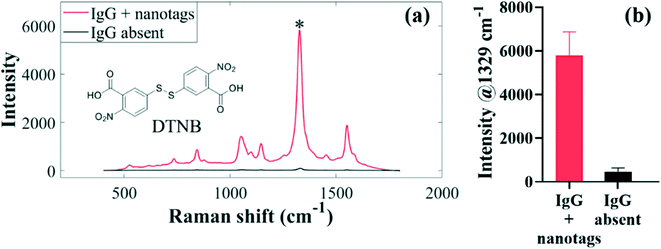 |
| Fig. 3 Illustration of the detection of antibody–spike immunocomplexes in serum using SERS assays: (a) SERS spectra of DTNB observed when IgG is present (red trace) and when IgG is absent (black trace). The chemical structure for DTNB is inserted in (a). (b) Corresponding bar graphs plotted from spectra in (a) when IgG is detected (red) and when IgG is absent in serum samples (black). An asterisk (*) in (a) represents the dominant SERS band used to compute peak intensities, and thus to identify seropositive and seronegative clinical serum samples in spatially separated microfluidic channels. | |
By visual inspection of Fig. 3a, strong SERS signals are generated when anti-spike IgG, IgA and IgM antibodies and biorecognition nanotags bind to each other to form immunocomplexes, leading to successful identification of seropositive patients based on intensity differences of the 1329 cm−1 band for DTNB, which is present on the nanotags. By comparison, since anti-spike antibodies were absent in the negative control subjects, only very weak spectra are detected. The observed weak DTNB-specific background signals arise from residual SERS-active nanotags that are not completely washed away from the sensor surface. Nevertheless, residual signals from negative control sera were negligible when compared to strong SERS enhancement conferred by antibody–nanotag complexes observed in positive sera. Bar charts in Fig. 3b, which were computed from the characteristic spectral intensity, confirmed clear-cut qualitative and semi-quantitative differences between seropositive and negative control sera.
Multiplex detection of cross-reactivity of antibodies against the native, P.1 and B.1.617.2 spike
An important aspect of this study was the rapid multiplexed detection of cross-reactivity and persistence of native SARS-CoV-2-elicited humoral immunity against the native, P.1 and B.1.617.2 spike proteins in the same sample. Microfluidics design and detection format reported by Djaileb et al.,34 was adopted in the present study. Spike glycoproteins were immobilised in four spatially separated microfluidic chambers for simultaneous parallel detection of IgG, IgA and IgM. Three different types of recognition nanotags, each functionalised with DTNB and either IgG, IgA or IgM anti-human capture antibody, were employed to identify specific target anti-spike antibodies in clinical samples. SERS data were acquired right after the secondary interaction step. The persistence of antibodies in the recovering individuals was investigated at two time intervals that were 3 weeks and 8 weeks post-diagnosis. Thirty-two (32) serum samples were probed of which 24 subjects tested positive to native SARS-CoV-2 and recovered without needing hospitalisation prior to enrolment to this study. The time scale between the detection of the PCR positive COVID-19 test and the first sampling time point ranged from 2 to 3 weeks after the resolution of symptoms. Considering that the convalescent subjects were infected early in the pandemic before the emergence of SARS-CoV-2 mutants, antibody responses expressed by the subjects under investigation were induced by infection with a point-mutated variant of SARS-CoV-2 virus that originated from Wuhan (Wuhan-1D614G, also known as HK-95), which we refer to as the native strain. Hence, the study subjects were variant-naïve at the time convalescent sera were longitudinally collected at 3 weeks and 8 weeks post-diagnosis.4 On the other hand, the patients used as negative control samples (n = 8) never received a positive COVID-19 test and never exhibited any symptoms related to COVID-19 at the time of enrolment. The age ranges for the seropositive and negative control subjects were 18–80 and 20–55 years, respectively.
Fig. 4 shows averaged results for SERS multiplex analyses of anti-spike IgG, IgA and IgM antibodies binding to three different classes of spike antigens using three different types of SERS-active nanotags. The performance of the SERS immunoassay was evaluated by plotting ROC curves profiling the sensitivity and specificity associated with the cross-reactive IgG, IgA and IgM binding to the spike proteins of the native and VOCs (Fig. S5 and S6†). The decision threshold criterion for discriminating positive from negative patients via ROC curves was calculated from the SERS characteristic spectral band as: mean intensity + 3 standard deviations of the negative control samples.44 Thus, peak intensities detected above the cut-off value were classified as seropositive while the intensities below the cut-off were considered seronegative. For significance tests, parametric independent samples t-test was used to probe dynamics in binding antibody responses across time point samples by comparing mean intensities of the dominant SERS peak, as summarised in Fig. 4.
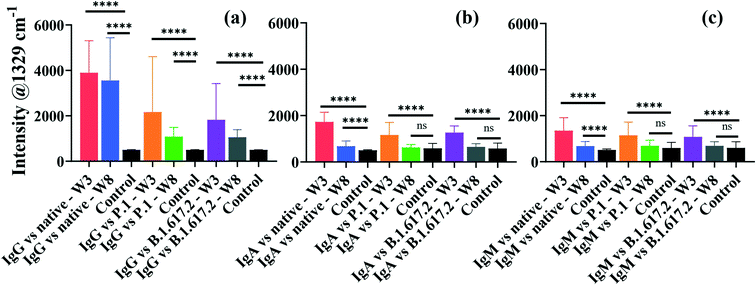 |
| Fig. 4 Averaged bar graphs plotted from the characteristic SERS peak intensity showing dynamics in multiplexed antibody responses and cross-reactivities in patient sera collected after 3 weeks and 8 weeks post-diagnosis for detecting spike-specific IgG (a), IgA (b) and IgM (c). Asterisks indicate statistical significance 95% CI between the seropositive and negative control subjects as determined by the independent sample t-test p-values: ****p < 0.0001 and ns: not significant. Error bars represent 1 × standard deviation. Abbreviations; W3: 3 weeks, and W8: 8 weeks sampling time points post-infection. | |
The averaged SERS intensities reveal that sera from 24 PCR-confirmed COVID-19-positive subjects tested positive for IgG against the native, P.1 and B.1.617.2 spike proteins (sensitivity = 100%) at week 3 and all corresponding negative control sera were correctly identified (specificity = 100%), as indicated by ROC curves in Fig. S5a–c.† Notably, much higher and robust levels of IgG were detected against the native spike glycoprotein than those for IgA and IgM (Fig. 4). Conversely, all recovered patients presented significantly lower cross-reactive IgA and IgM binding responses than IgG responses against all three spike antigens (Fig. 4b and c). Qualitative examination of cross-reactive IgA and IgM antibody levels to the native spike led to high seropositivity rates of 100% and >99% for IgA and IgM, respectively (Fig. S5d and g†). Anti-spike IgA and IgM exhibited comparable antibody response profiles against the native strain and VOCs (Fig. 4b and c and S5d–i†).
When simultaneously cross-reacted against the P.1 and B.1.617.2 viral spike, IgG, IgA and IgM antibody responses diminished significantly in comparison to those for the ancestral spike, although the decrease in IgG response to VOCs did not compromise the classification accuracy of seropositive and negative controls against VOCs (AUC: 1.000) as illustrated in Fig. S5b and c.† Patients were identified at >86% and 100% seropositivity rates for IgA targeting the P.1 and B.1.617.2 VOCs, respectively (Fig. S5e and f†). On the other hand, cross-reactive serum IgM were detected at lower sensitivities at >83% against the P.1 spike and >80% against the B.1.617.2 spike (Fig. S5h and i†). The decrease in the native SARS-CoV-2-acquired antibody response against the VOCs highlights the influence of the E484K and T478K spike mutations,45 which induce immune escape against anti-spike antibodies acquired from the native SARS-CoV-2 viral infection. However, despite losing part of immune potency, antibodies arising from infection with ancestral viral strain significantly cross-reacted against variants, which is consistent with a recent report.46 Based on results presented here, an infection with the native SARS-CoV-2 induces production of binding antibodies that most strongly recognise the infecting native strain than the VOCs. Similar to these data highlighting considerable loss of cross-reactive anti-spike antibody titres to VOCs, increasing evidence shows that spike-specific antibodies whose production was stimulated by the native strain had reduced cross-reactivity against variants, by a factor of up to 4-fold.45,47,48 Whereas the extent of immune resistance of VOCs to binding antibodies varies considerably, the lower cross-reactivity of antibodies against VOCs has been widely reported in previous studies. For example, a prior study by Dupont et al. detected remarkably high sera IgG and IgM neutralisation escape of the B.1.617.2 spike in the variant-naïve convalescent sera,46 and the results not only aligned with more recent findings,4 but also supported the reduced antibody-VOCs responses observed in this study.
For samples collected after 8 weeks post diagnosis, high IgG detection accuracy (pooled AUC: 0.9988) was maintained (Fig. 4 and S6a–c†). The ability of anti-spike IgA and IgM to cross-protect against spike antigens plummeted appreciably at 8 weeks, resulting in much lower seropositivity rates in spite of retaining high specificities (Fig. S6d–i†). Thus, the clinical sensitivity of serological assays, such as SERS, is perhaps best harnessed with respect to the kinetics of antibody seroconversion after exposure to SARS-CoV-2. Since seropositivity rate appears to be high around 2–3 weeks post infection, serological tests are likely to return reliable results within this recovery time window.2,49
Currently, much of research on COVID-19 immunology focuses on the evolution of antibody response and how long convalescent patients can sustain functional immunity after recovering from infection, as this is crucial for controlling the pandemic. The emergence of SARS-CoV-2 mutants with reduced sensitivity to host neutralising antibodies and increased breakthrough infections50 has escalated fears for compromised and suboptimal antiviral immunity. To better understand this, we conducted a longitudinal study of antibody persistence in sera at two time intervals: 3 weeks (baseline) and 8 weeks post-diagnosis as shown in Fig. 4. The findings indicate that SERS responses for IgG targeting the native strain and cross-reactivity to VOCs that were detected at 3 weeks remained stable for at least 8 weeks. Numerous studies confirm this to be the case,51–54 where high titres of IgG remained stable for as long as 16 months post infection,55 suggesting that IgG mediate long-term immunity, though potency decreases over time.56 The level of cross-reactive IgG recognising spike of the P.1 and B.1.617.2 were immediately lower but were stable over time (Fig. 4a). By contrast IgA and IgM had a short lifespan that perhaps dominate the early host humoral immunity before B-cells undergo isotype class switching and somatic hypermutation to produce more specific and long-lasting neutralising IgG antibodies.57
Stratification of seropositivity against the native, P.1 and B.1.617.2 spike by age group
In the next stage of the analysis, we examined the association between the levels of infection-acquired anti-spike sera antibodies and patient age groups (i.e., 18–49, 50–59, 60–69 and 70+ years). As shown in Fig. 5, the levels of anti-spike IgG, IgA and IgM increased with the age of patients. A distinct drop in the levels of antibodies cross-reacting with the VOCs, compared to the native strain, was also noticeable at the age level. Generally, because IgG titres (Fig. 5a) were much higher than IgA and IgM levels (Fig. 5b and c) across spike antigens and age groups, IgG titres showed greater loss in potency against VOCs than IgA and IgM isotypes. Disproportionately low antibody responses were detected in younger patients (18–49 years) than the older group (>50 years) against all spike antigens (Fig. 5a–c). Whether this decline in titre signifies potentially sub-optimal antibody binding response remains to be confirmed through affinity or neutralisation assays that test antibody capability to inhibit spike-ACE2 interaction. However, this observation is not entirely unique to our study as researchers recently reported a decrease in IgG antibody response to the native SARS-CoV-2 and VOCs in young adults,4 whose age range was the same as that for the patients examined in this study. By contrast, sera from patients above 50 years old exhibited the highest antibody responses regardless of the type of the tested spike proteins. Overall, the detected correlation between levels of binding antibodies and patient age was consistent irrespective of the class of spike antigens as shown in Fig. 5, and this finding is in complete agreement with our recent results that highlighted similar antibody responses detected using SPR and ELISA assays.4 The differences in antibody responses between patient age groups were tested for statistical significance at 95% CI. Antibody levels tested against the native spike in week 3 were all statistically significant across age groups (p < 0.0001), apart from those between 50–59 and 70+ years for IgA and IgM isotypes. IgG responses to VOCs exhibited a similar trend (p < 0.0432), except between 50–59 and 60–69 years (P.1) and among 50–59, 60–69 and 70+ years (B.1.617.2) groups, which were not significant. For IgA response against VOCs, the binding response differences were all significant (p < 0.0323), besides those between 18–49 and 50–59 (P.1), and between 50–59 and 60–69 patient age groups (P.1 and B.1.617.2), that did not exhibit any statistical significance. In contrast, IgM responses to VOCs by patient age were only significant among the 18–49, 50–59 and 60–69 age groups for week 3 samples (p < 0.0330). At week 8, significant differences were only detected for IgG against the native spike (p < 0.0001), with the rest of the antibody titres vs. age groups being insignificant at 95% CI.
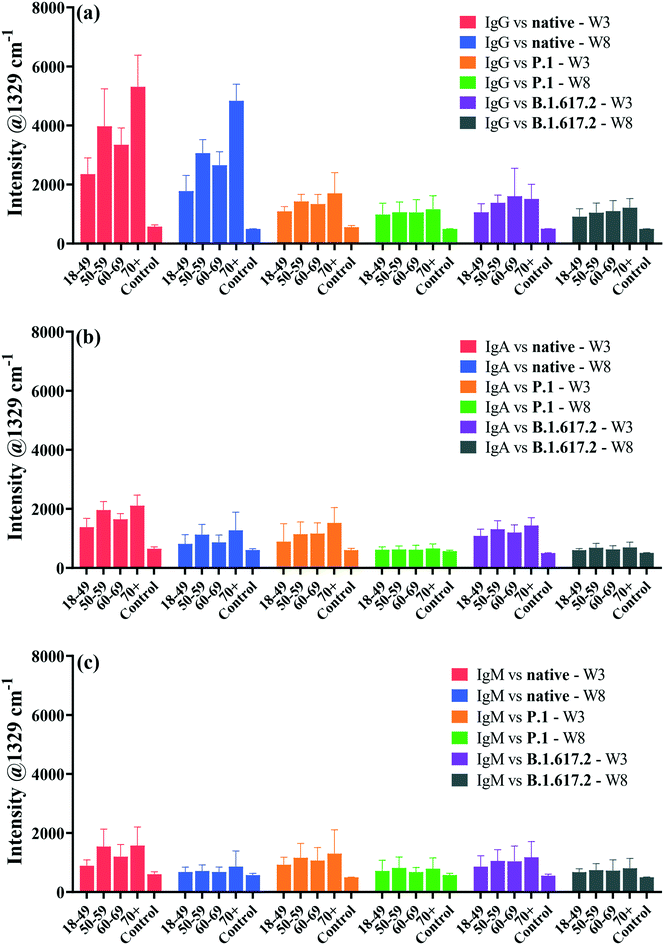 |
| Fig. 5 Comparative analysis of antibody–spike binding responses by patient age using univariate analysis: (a) anti-spike IgG targeting the native strain, and the P.1 and B.1.617.2 VOCs (a), IgA (b), and IgM (c) sampled at 3 weeks and 8 weeks post-diagnosis. Error bars represent 1 x standard deviation. For the abbreviations; WT: wild-type, and control: negative controls. Graphs were equally scaled for ease of comparison. The oldest group (>70 years) exhibited highest antibody titres whilst the youngest subjects (18–49 years) had the lowest titres of antibodies. Middle aged adults (50–59 and 60–69 years) had intermediate antibody responses. Abbreviations; W3: 3 weeks, and W8: 8 weeks sampling time points post-infection. | |
To further explore the patient age and antibody titre correlation, PCA was applied, that employs multivariate SERS data comprising the entire spectral ranges for each sample as the only input variable. The resultant PCA score biplots for all isotypes and spike proteins are shown in Fig. 6.
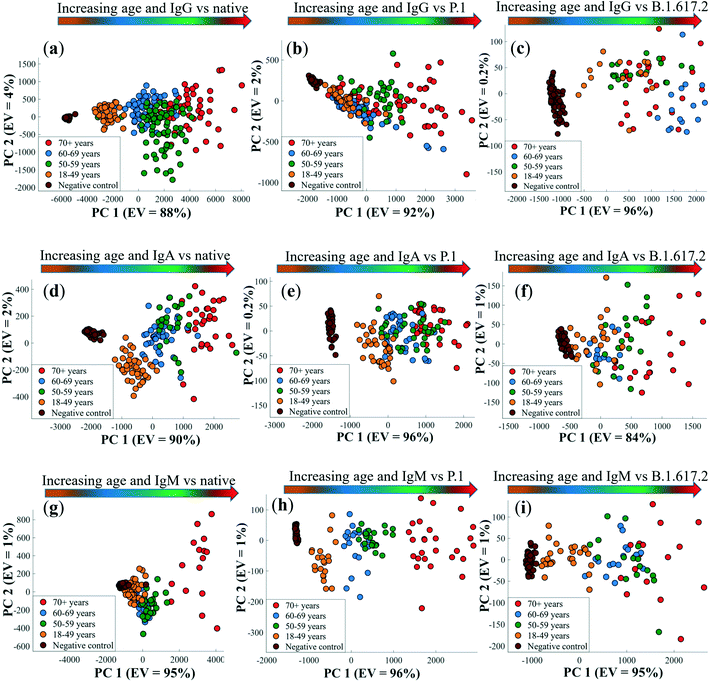 |
| Fig. 6 PCA scores plots of pre-processed DTNB-encoded multivariate SERS spectral data generated from antibody–spike immunoreactions highlighting a general increase in antibody titres with patient age for the detection of IgG (a–c), IgA (d–f), and IgM (g–i). Coloured spherical symbols represent different age categories examined in this study. The values in parentheses are the percentage of explained variance (EV) the SERS dataset. Gradient arrows indicate the direction of increasing antibody titres according to the age of patients compared to negative control samples, and these arrows have no statistical significance. Spectral data represented on PCA were measured in triplicates from each (n = 6) of the 4 patient groups and controls (n = 8). | |
The first principal component (PC1), which captures the highest variance within the datasets, discerned an increase in patient age with antibody titres for IgG, IgA and IgM (Fig. 6a–c) from left to right of in the order: 18–49 < 60–69 < 50–59 < 70+ years groups, and seropositive adults clearly differentiated from negative controls. This observation is not surprising, given that the concentration of binding antibodies dominated the SERS data. The discrepancy in antibody level-age distribution pattern seen earlier is also apparent along the PC1 axis (Fig. 6a–c), where the 60–69 years old patients again clustered before the 50–59 and 70+ years old subjects. As one may have predicted, PC1 loadings plots (Fig. S7†) confirmed the peak detected at 1329 cm−1 (–NO2 stretching vibrations) to be the most essential input variable linked with PCA scores plots presented in Fig. 6, that again corroborate the results of univariate analytical data described earlier on Fig. 5. PC2 on the other hand accounted for less variance mainly related to variations in spectral shifts of DTNB bands in biochemically dense sera, and this was also clearly reflected in PC2 loadings plots in Fig. S7.† The variation of antibody response with patient age is considered critical for assessing risk factors associated with severity and prognosis of COVID-19. Our data build upon previous evidence,4,58,59 which demonstrated that older (>70 years) individuals elicit stronger antibody responses, and are more likely than younger and middle-aged people to develop severe COVID-19.60,61 This information continues to guide appropriate targeted preventative interventions and vaccination strategies aimed to prioritise protection of the vulnerable age groups from developing severe infections and mortality due to COVID-19 infection.62
The results presented so far in this study have mainly provided a qualitative picture of antibody serology based on the semi-quantitative SERS spectral intensities measured from patient sera. SERS is best known for its inherently high quantitative detection of low levels of disease markers and one may expect to see quantitative tests of spike-specific antibodies in clinical samples. We performed SERS on serially diluted pooled sera to illustrate quantitative analytical sensitivity of the SERS assay as shown in Fig. S8.† The range of dilutions was: 1
:
2000, 1
:
1000, 1
:
500, 1
:
100, 1
:
10, 1
:
5 and undiluted pooled serum. SERS intensities of the spectral marker peak increased proportionally with the levels of serum anti-spike IgG, IgA and IgM. The SERS bands for antibodies measured in undiluted serum and the 1
:
5 dilution displayed higher spectral intensities for week 3 samples than week 8 sera. Weak SERS signals were detected from the largest dilution factors of 1
:
1000 and 1
:
2000 dilutions. No appreciable signals from the blank (running buffer only) as it was devoid of patient samples.
Dilution series of pooled serum were used to estimate equilibrium dissociation constants (KDs) in order to provide insights into the strength of binding of patient sera antibodies to SARS-CoV-2 spike antigens. KDs were calculated by fitting the Langmuir isotherm assuming a 1
:
1 protein–protein binding site model, as described in previous literature.4 Note that KDs were expressed as dilution factors, and so the magnitude of KDs varied inversely with dilution factors. Hence, larger KDs indicate stronger binding affinity of antibodies to the spike, and smaller KDs correlate with weaker antibody–antigen interactions. Strong binding affinities to spike antigens were detected at the initial stage of recovery (3 weeks post diagnosis), which then decreased by week 8 post diagnosis (Fig. 7). In all cases, antibodies appeared to have higher binding affinity for the native spike that VOCs proteins at the week 3 than week 8 post-symptom onset. The difference in KDs for IgG (p = 0.2804 for native; p = 0.2861 for P.1 and p = 0.2241 for B.1.617.2) were not statistically significant between weeks 3 and 8 post diagnosis, a result that was also true for IgA (p = 0.2034 for native; p = 0.1467 for P.1 and p = 0.1739 for B.1.617.2) between weeks 3 and week 8 post diagnosis. KDs for IgM against spike trended higher (large KDs) by week 3, which reached statistical significance for the P.1 (p = 0.0120) and B.1.617.2 (p = 0.0177) compared to the affinity against the native (p = 0.1023) which was not statistically significant between the sampling points (Fig. 7c). These dynamics in binding affinity are consistent with KD profiles for IgG against the native and VOCs reported for weeks 4 and 16 in patients drawn from the same cohort as in the present study.4 Information on the degree of binding affinity of infection-acquired convalescent sera may be crucial for predicting the mutational effect(s) of SARS-CoV-2 on therapy and accelerated engineering of potent therapeutic antibodies.63
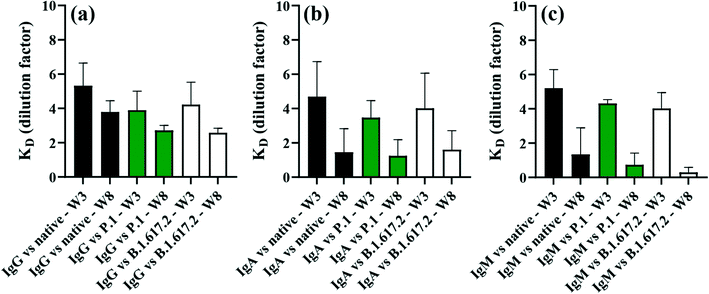 |
| Fig. 7 Dissociation constants (KDs) highlighting the strength of antibody–antigen interactions in serial dilutions of pooled immune serum samples for (a) IgG, (b) IgA and (c) IgM antibodies. Error bars represent 1 × standard deviation of the mean. | |
Given that the absolute level of SARS-CoV-2-induced antibody isotypes in the investigated patient sera was unknown, the absolute quantification of patient-specific antibodies was not possible. However, we employed known concentration of a purified primary human IgG antibody as a calibration standard to probe the performance of our SERS assay. Different concentrations of IgG from 500 ng mL−1 to 1 pg mL−1 were prepared in running buffer before being immobilised on the sensor surface and subjected to SERS spectral analysis. Fig. S9† shows a linear calibration curve resulting from the SERS analysis of various levels of IgG. The LOD of our SERS assay was calculated to be 5 pg mL−1, which is orders of magnitude better than those reported for standard assays such as ELISA (1.953 ng mL−1),64 and LFIA (1 μg mL−1).28
Conclusion
We have shown that microfluidics-integrated multiplexed SERS assays can provide valuable insights into COVID-19 serological immunity profiling of multiple antibody–spike binding interactions in a single serum sample with high sensitivity (LOD = 5 pg mL−1) and rapid turnaround time of 35 min. As high as >80% and >96% clinical sensitivity and specificity, respectively were detected when anti-spike antibodies reacted against the native and variant spike in the same serum collected from variant-naïve non-hospitalised adults with mild COVID-19, who were PCR-diagnosed with the native SARS-CoV-2 a priori. Peak antibody responses were detected at 3 weeks post-diagnosis which were sustained for IgG after 8 weeks post-diagnosis, as opposed to rapidly declining IgA and IgM responses, and this trend was similar to that for antibody–spike binding affinities. For cross-reactive anti-spike antibodies, binding responses were nearly halved on exposure of sera antibodies to the P.1 and B.1.617.2 spike compared to the native spike protein. Univariate and multivariate data revealed that older patients (>70 years) had greater antibody responses than the younger subjects (18–49 years) against the investigated spike glycoproteins. While our results provide clinically relevant evidence on cross-reactive and persistence of COVID-19-acquired humoral immunity up to 8 weeks post diagnosis, further studies with larger patient sample size, and with more regular and prolonged time intervals are needed to extrapolate our multiplexed SERS results to larger populations. There is also a need to determine the precise proportion of neutralising antibodies in sera that are correlates of protective humoral immunity. Based on the results reported in this study, SERS assay promises to be a rapid and sensitive technique for simultaneous multiplex analysis of multiple antibody responses against SARS-CoV-2 infection(s).
Author contributions
Conceptualisation: J.-F. M., J. N. P. and M. C. Methodology: M. C., J. N. P. and J.-F. M. Investigation: M. C., M. S., C. G., D. L., B. C., L. B., A. P. and S. L. D. Data acquisition and analysis: M. C., M. S., C. G., D. L., B. C., L. B., A. P. and S. L. D. Funding: S. T., D. B., J. N. P. and J.-F. M. Writing original draft: M. C. and edited by all authors. Project supervision: S. T., D. B., J. N. P. and J.-F. M. The final version of this article was read and approved by all authors.
Conflicts of interest
J.-F. M. and J. N. P. have financial interest in Affinité Instruments. Authors declare no other competing interests.
Acknowledgements
We acknowledge financial support from the Canadian Institutes of Health Research (CIHR), the Natural Sciences and Engineering Research Council of Canada (NSERC), the Pandemic Response Challenge Program of the National Research Council Canada and the Canadian Foundation for Innovation (CFI).
References
-
Johns Hopkins University and Medicine - Coronavirus Resource Center, (https://coronavirus.jhu.edu), (Accessed 1st February, 2022) Search PubMed.
- K. H. D. Crawford, A. S. Dingens, R. Eguia, C. R. Wolf, N. Wilcox, J. K. Logue, K. Shuey, A. M. Casto, B. Fiala, S. Wrenn, D. Pettie, N. P. King, A. L. Greninger, H. Y. Chu and J. D. Bloom, J. Infect. Dis., 2021, 223, 197–205 CrossRef CAS PubMed.
- S. F. Lumley, D. O'Donnell, N. E. Stoesser, P. C. Matthews, A. Howarth, S. B. Hatch, B. D. Marsden, S. Cox, T. James, F. Warren, L. J. Peck, T. G. Ritter, Z. de Toledo, L. Warren, D. Axten, R. J. Cornall, E. Y. Jones, D. I. Stuart, G. Screaton, D. Ebner, S. Hoosdally, M. Chand, D. W. Crook, A. M. O'Donnell, C. P. Conlon, K. B. Pouwels, A. S. Walker, T. E. A. Peto, S. Hopkins, T. M. Walker, K. Jeffery, D. W. Eyre and G. Oxford Univ Hosp Staff Testing, N. Engl. J. Med., 2021, 384, 533–540 CrossRef CAS PubMed.
- M. H. Jodaylami, A. Djaileb, P. Ricard, E. Lavallee, S. Cellier-Goethebeur, M. F. Parker, J. Coutu, M. Stuible, C. Gervais, Y. Durocher, F. Desautels, M. P. Cayer, M. J. de Grandmont, S. Rochette, D. Brouard, S. Trottier, D. Boudreau, J. N. Pelletier and J. F. Masson, Sci. Rep., 2021, 11, 21601–21611 CrossRef PubMed.
- D. Jacofsky, E. M. Jacofsky and M. Jacofsky, J. Arthroplasty, 2020, 35, S74–S81 CrossRef PubMed.
- L. J. Carter, L. V. Garner, J. W. Smoot, Y. Z. Li, Q. Q. Zhou, C. J. Saveson, J. M. Sasso, A. C. Gregg, D. J. Soares, T. R. Beskid, S. R. Jervey and C. Liu, ACS Cent. Sci., 2020, 6, 591–605 CrossRef CAS PubMed.
- B. Lou, T. D. Li, S. F. Zheng, Y. Y. Su, Z. Y. Li, W. Liu, F. Yu, S. X. Ge, Q. D. Zou, Q. Yuan, S. Lin, C. M. Hong, X. Y. Yao, X. J. Zhang, D. H. Wu, G. L. Zhou, W. H. Hou, T. T. Li, Y. L. Zhang, S. Y. Zhang, J. Fan, J. Zhang, N. S. Xia and Y. Chen, Eur. Respir. J., 2020, 56, 2000763–2000772 CrossRef CAS PubMed.
- A. S. Rudberg, S. Havervall, A. Manberg, A. Jernbom Falk, K. Aguilera, H. Ng, L. Gabrielsson, A. C. Salomonsson, L. Hanke, B. Murrell, G. McInerney, J. Olofsson, E. Andersson, C. Hellstrom, S. Bayati, S. Bergstrom, E. Pin, R. Sjoberg, H. Tegel, M. Hedhammar, M. Phillipson, P. Nilsson, S. Hober and C. Thalin, Nat. Commun., 2020, 11, 5064–5071 CrossRef CAS PubMed.
- J. L. Bernal, N. Andrews, C. Gower, E. Gallagher, R. Simmons, S. Thelwall, J. Stowe, E. Tessier, N. Groves, G. Dabrera, R. Myers, C. N. J. Campbell, G. Amirthalingam, M. Edmunds, M. Zambon, K. E. Brown, S. Hopkins, M. Chand and M. Ramsay, N. Engl. J. Med., 2021, 385, 585–594 CrossRef CAS PubMed.
- E. J. Haas, F. J. Angulo, J. M. McLaughlin, E. Anis, S. R. Singer, F. Khan, N. Brooks, M. Smaja, G. Mircus, K. J. Pan, J. Southern, D. L. Swerdlow, L. Jodar, Y. Levy and S. Alroy-Preis, Lancet, 2021, 397, 1819–1829 CrossRef CAS.
- N. Ravi, D. L. Cortade, E. Ng and S. X. Wang, Biosens. Bioelectron., 2020, 165, 112454–112466 CrossRef CAS PubMed.
- A. Yakoh, U. Pimpitak, S. Rengpipat, N. Hirankarn, O. Chailapakul and S. Chaiyo, Biosens. Bioelectron., 2021, 176, 112912–112919 CrossRef CAS PubMed.
- F. Y. Cui and H. S. S. Zhou, Biosens. Bioelectron., 2020, 165, 112349–112357 CrossRef CAS PubMed.
- C. H. GeurtsvanKessel, N. M. A. Okba, Z. Igloi, S. Bogers, C. W. E. Embregts, B. M. Laksono, L. Leijten, C. Rokx, B. Rijnders, J. Rahamat-Langendoen, J. P. C. van den Akker, J. J. A. van Kampen, A. A. van der Eijk, R. S. van Binnendijk, B. Haagmans and M. Koopmans, Nat. Commun., 2020, 11, 3436–3441 CrossRef PubMed.
- F. Amanat, D. Stadlbauer, S. Strohmeier, T. H. O. Nguyen, V. Chromikova, M. McMahon, K. J. Jiang, G. A. Arunkumar, D. Jurczyszak, J. Polanco, M. Bermudez-Gonzalez, G. Kleiner, T. Aydillo, L. Miorin, D. S. Fierer, L. A. Lugo, E. M. Kojic, J. Stoever, S. T. H. Liu, C. Cunningham-Rundles, P. L. Felgner, T. Moran, A. Garcia-Sastre, D. Caplivski, A. L. C. Cheng, K. Kedzierska, O. Vapalahti, J. M. Hepojoki, V. Simon and F. Krammer, Nat. Med., 2020, 26, 1033–1036 CrossRef CAS PubMed.
- L. H. Harritshoj, M. Gybel-Brask, S. Afzal, P. R. Kamstrup, C. S. Jorgensen, M. K. Thomsen, L. Hilsted, L. Friis-Hansen, P. B. Szecsi, L. Pedersen, L. Nielsen, C. B. Hansen, P. Garred, T. L. Korsholm, S. Mikkelsen, K. O. Nielsen, B. K. Moller, A. T. Hansen, K. K. Iversen, P. B. Nielsen, R. B. Hasselbalch, K. Fogh, J. B. Norsk, J. H. Kristensen, K. Schonning, N. S. Kirkby, A. C. Y. Nielsen, L. H. Landsy, M. Loftager, D. K. Holm, A. C. Nilsson, S. G. Saekmose, B. Grum-Schwensen, B. Aagaard, T. G. Jensen, D. M. Nielsen, H. Ullum and R. B. Dessau, J. Clin. Microbiol., 2021, 59, e02596 CrossRef CAS PubMed.
- N. C. Cady, N. Tokranova, A. Minor, N. Nikvand, K. Strle, W. T. Lee, W. Page, E. Guignon, A. Pilar and G. N. Gibson, Biosens. Bioelectron., 2021, 171, 112679–112686 CrossRef CAS PubMed.
- R. B. M. Schasfoort, J. van Weperen, M. van Amsterdam, J. Parisot, J. Hendriks, M. Koerselman, M. Karperien, A. Mentink, M. Bennink, H. Krabbe, L. Terstappen and A. H. L. Mulder, Biosens. Bioelectron., 2021, 183, 113165–1131693 CrossRef CAS PubMed.
- J. Langer, D. J. de Aberasturi, J. Aizpurua, R. A. Alvarez-Puebla, B. Auguie, J. J. Baumberg, G. C. Bazan, S. E. J. Bell, A. Boisen, A. G. Brolo, J. Choo, D. Cialla-May, V. Deckert, L. Fabris, K. Faulds, F. J. G. de Abajo, R. Goodacre, D. Graham, A. J. Haes, C. L. Haynes, C. Huck, T. Itoh, M. Ka, J. Kneipp, N. A. Kotov, H. Kuang, E. C. Le Ru, H. K. Lee, J. F. Li, X. Y. Ling, S. A. Maier, T. Mayerhofer, M. Moskovits, K. Murakoshi, J. M. Nam, S. Nie, Y. Ozaki, I. Pastoriza-Santos, J. Perez-Juste, J. Popp, A. Pucci, S. Reich, B. Ren, G. C. Schatz, T. Shegai, S. Schlucker, L. L. Tay, K. G. Thomas, Z. Q. Tian, R. P. Van Duyne, T. Vo-Dinh, Y. Wang, K. A. Willets, C. Xu, H. Xu, Y. Xu, Y. S. Yamamoto, B. Zhao and L. M. Liz-Marzan, ACS Nano, 2020, 14, 28–117 CrossRef CAS.
- H. Kearns, R. Goodacre, L. E. Jamieson, D. Graham and K. Faulds, Anal. Chem., 2017, 89, 12666–12673 CrossRef CAS.
- H. F. Liu, E. H. Dai, R. Xiao, Z. H. Zhou, M. L. Zhang, Z. K. Bai, Y. Shao, K. Z. Qi, J. Tu, C. W. Wang and S. Q. Wang, Sens. Actuators, B, 2021, 329, 129196–129205 CrossRef CAS PubMed.
- S. L. Chen, L. W. Meng, L. T. Wang, X. X. Huang, S. Ali, X. J. Chen, M. G. Yu, M. Yi, L. M. Li, X. Chen, L. M. Yuan, W. Shi and G. Z. Huang, Sens. Actuators, B, 2021, 348, 130706–130716 CrossRef CAS PubMed.
- J. Aizpurua, H. Arnolds, J. Baumberg, I. Bruzas, R. Chikkaraddy, M. Chisanga, P. Dawson, V. Deckert, I. Delfino, B. de Nijs, G. Di Martino, J. Edel, H. Fleming, S. Gawinkowski, F. Giorgis, R. Goodacre, D. Graham, M. Hardy, C. Heck, S. Heeg, K. Hewitt, L. Jamieson, A. Keeler, A. Krolikowska, C. Kuttner, N. Lidgi-Guigui, C. Lightner, J. Lombardi, S. Mahajan, N. M. Sabanes, J. F. Masson, N. S. Mueller, H. Muhamadali, K. Murakoshi, J. Popp, M. Porter, S. Reich, G. Schatz, Z. Q. Tian, A. Tripathi, R. Van Duyne, X. P. Wang, A. Wark, K. Willets and M. Willner, Faraday Discuss., 2017, 205, 291–330 RSC.
- L. Wu, Z. Y. Wang, S. F. Zong, H. Chen, C. L. Wang, S. H. Xu and Y. P. Cui, Analyst, 2013, 138, 3450–3456 RSC.
- A. Das, K. Kim, S. G. Park, N. Choi and J. Choo, Biosens. Bioelectron., 2021, 192, 113125–113532 CrossRef PubMed.
- M. Chisanga, H. Muhamadali, D. I. Ellis and R. Goodacre, Appl. Sci., 2019, 9, 1163–1186 CrossRef CAS.
- J. Jeon, N. Choi, H. Chen, J. I. Moon, L. X. Chen and J. Choo, Lab Chip, 2019, 19, 674–681 RSC.
- S. Srivastav, A. Dankov, M. Adanalic, R. Grzeschik, V. Tran, S. Pagel-Wieder, F. Gessler, I. Spreitzer, T. Scholz, B. Schnierle, O. E. Anastasiou, U. Dittmer and S. Schlucker, Anal. Chem., 2021, 93, 12391–12399 CrossRef CAS PubMed.
- R. K. Gao, Z. Y. Cheng, A. J. Demello and J. Choo, Lab Chip, 2016, 16, 1022–1029 RSC.
- A. Kapara, K. A. F. Paterson, V. G. Brunton, D. Graham, M. Zagnoni and K. Faulds, Anal. Chem., 2021, 93, 5862–5871 CrossRef CAS PubMed.
- B. Isho, K. T. Abe, M. Zuo, A. J. Jamal, B. Rathod, J. H. Wang, Z. J. Li, G. Chao, O. L. Rojas, Y. M. Bang, A. N. Pu, N. Christie-Holmes, C. Gervais, D. Ceccarelli, P. Samavarchi-Tehrani, F. Guvenc, P. Budylowski, A. G. Li, A. Paterson, F. Y. Yue, L. M. Marin, L. Caldwell, J. L. Wrana, K. Colwill, F. Sicheri, S. Mubareka, S. D. Gray-Owen, S. J. Drews, W. L. Siqueira, M. Barrios-Rodiles, M. Ostrowski, J. M. Rini, Y. Durocher, A. J. McGeer, J. L. Gommerman and A. C. Gingras, Sci. Immunol., 2020, 5, eabe5511–eabe5530 CrossRef PubMed.
- M. Stuible, C. Gervais, S. Lord-Dufour, S. Perret, D. L'Abbe, J. Schrag, G. St-Laurent and Y. Durocher, J. Biotechnol., 2021, 326, 21–27 CrossRef CAS PubMed.
- N. G. Bastús, J. Comenge and V. Puntes, Langmuir, 2011, 27, 11098–11105 CrossRef PubMed.
- A. Djaileb, M. H. Jodaylami, J. Coutu, P. Ricard, M. Lamarre, L. Rochet, S. Cellier-Goetghebeur, D. Macaulay, B. Charron, E. Lavallee, V. Thibault, K. Stevenson, S. Forest, L. S. Live, N. Abonnenc, A. Guedon, P. Quessy, J. F. Lemay, O. Farnos, A. Kamen, M. Stuible, C. Gervais, Y. Durocher, F. Cholette, C. Mesa, J. Kim, M. P. Cayer, M. J. De Grandmont, D. Brouard, S. Trottier, D. Boudreau, J. N. Pelletier and J. F. Masson, Analyst, 2021, 146, 4905–4917 RSC.
- P. H. C. Eilers, Anal. Chem., 2004, 76, 404–411 CrossRef CAS PubMed.
- M. Chisanga, D. Linton, H. Muhamadali, D. I. Ellis, R. L. Kimber, A. Mironov and R. Goodacre, Analyst, 2020, 145, 1236–1249 RSC.
- M. Sanchez-Purra, B. Roig-Solvas, C. Rodriguez-Quijada, B. M. Leonardo and K. Hamad-Schifferli, ACS Omega, 2018, 3, 10733–10742 CrossRef CAS PubMed.
- J. Neng, M. H. Harpster, W. C. Wilson and P. A. Johnson, Biosens. Bioelectron., 2013, 41, 316–321 CrossRef CAS PubMed.
- Y. H. Tan, M. Liu, B. Nolting, J. G. Go, J. Gervay-Hague and G. Y. Liu, ACS Nano, 2008, 2, 2374–2384 CrossRef CAS PubMed.
-
Gold Nanoparticles: Physical Properties – nanoComposix (Accessed 9th June, 2022), https://nanocomposix.com/pages/gold-nanoparticles-physical-properties Search PubMed.
- A. Kaminska, K. Winkler, A. Kowalska, E. Witkowska, T. Szymborski, A. Janeczek and J. Waluk, Sci. Rep., 2017, 7, 10656–10666 CrossRef PubMed.
- U. S. Dinish, G. Balasundaram, Y. T. Chang and M. Olivo, Sci. Rep., 2014, 4, 4075–4081 CrossRef CAS PubMed.
- R. Goodacre, D. Graham and K. Faulds, TrAC, Trends Anal. Chem., 2018, 102, 359–368 CrossRef CAS.
- M. Greiner, D. Pfeiffer and R. D. Smith, Prev. Vet. Med., 2000, 45, 23–41 CrossRef CAS PubMed.
- T. Pan, Z. W. Hu, F. Y. Hu, Y. W. Zhang, B. F. Liu, C. W. Ke, Q. M. She, X. He, X. P. Tang and H. Zhang, Cell. Mol. Immunol., 2021, 18, 2560–2562 CrossRef CAS PubMed.
- L. Dupont, L. B. Snell, C. Graham, J. Seow, B. Merrick, T. Lechmere, T. J. A. Maguire, S. R. Hallett, S. Pickering, T. Charalampous, A. Alcolea-Medina, I. Huettner, J. M. Jimenez-Guardeno, S. Acors, N. Almeida, D. Cox, R. E. Dickenson, R. P. Galao, N. Kouphou, M. J. Lista, A. M. Ortega-Prieto, H. Wilson, H. Winstone, C. Fairhead, J. Z. Su, G. Nebbia, R. Batra, S. Neil, M. Shankar-Hari, J. D. Edgeworth, M. H. Malim and K. J. Doores, Nat. Microbiol., 2021, 6, 1433–1442 CrossRef CAS PubMed.
- S. Changrob, Y. B. Fu, J. J. Guthmiller, P. J. Halfmann, L. Li, C. T. Stamper, H. L. Dugan, M. Accola, W. Rehrauer, N. Y. Zheng, M. Huang, J. L. Wang, S. A. Erickson, H. A. Utset, H. M. Graves, F. Amanat, D. N. Sather, F. Krammer, Y. Kawaoka and P. C. Wilson, MBio, 2021, 12, e02975 CrossRef CAS PubMed.
- D. Cromer, M. Steain, A. Reynaldi, T. E. Schlub, A. K. Wheatley, J. A. Juno, S. J. Kent, J. A. Triccas, D. S. Khoury and M. P. Davenport, Lancet Microbe, 2022, 3, e52–e60 CrossRef CAS PubMed.
- M. L. Bastos, G. Tavaziva, S. K. Abidi, J. R. Campbell, L. P. Haraoui, J. C. Johnston, Z. Y. Lan, S. Law, E. MacLean, A. Trajman, D. Menzies, A. Benedetti and F. A. Khan, BMJ [Br. Med. J.], 2020, 370, m2516–m2528 Search PubMed.
- M. Bergwerk, T. Gonen, Y. Lustig, S. Amit, M. Lipsitch, C. Cohen, M. Mandelboim, E. G. Levin, C. Rubin, V. Indenbaum, I. Tal, M. Zavitan, N. Zuckerman, A. Bar-Chaim, Y. Kreiss and G. Regev-Yochay, N. Engl. J. Med., 2021, 385, 1474–1484 CrossRef CAS PubMed.
- S. P. Anand, J. Prevost, M. Nayrac, G. Beaudoin-Bussieres, M. Benlarbi, R. Gasser, N. Brassard, A. Laumaea, S. Y. Gong, C. Bourassa, E. Brunet-Ratnasingham, H. Medjahed, G. Gendron-Lepage, G. Goyette, L. Gokool, C. Morrisseau, P. Begin, V. Martel-Laferriere, C. Tremblay, J. Richard, R. Bazin, R. Duerr, D. E. Kaufmann and A. Finzi, Cell Rep. Med., 2021, 2, 100290–100304 CrossRef PubMed.
- A. K. Wheatley, J. A. Juno, J. J. Wang, K. J. Selva, A. Reynaldi, H. X. Tan, W. S. Lee, K. M. Wragg, H. G. Kelly, R. Esterbauer, S. K. Davis, H. E. Kent, F. L. Mordant, T. E. Schlub, D. L. Gordon, D. S. Khoury, K. Subbarao, D. Cromer, T. P. Gordon, A. W. Chung, M. P. Davenport and S. J. Kent, Nat. Commun., 2021, 12, 1162–1172 CrossRef CAS PubMed.
- J. Seow, C. Graham, B. Merrick, S. Acors, S. Pickering, K. J. A. Steel, O. Hemmings, A. O'Byrne, N. Kouphou, R. P. Galao, G. Betancor, H. D. Wilson, A. W. Signell, H. Winstone, C. Kerridge, I. Huettner, J. M. Jimenez-Guardeno, M. J. Lista, N. Temperton, L. B. Snell, K. Bisnauthsing, A. Moore, A. Green, L. Martinez, B. Stokes, J. Honey, A. Izquierdo-Barras, G. Arbane, A. Patel, M. K. I. Tan, L. O'Connell, G. O'Hara, E. MacMahon, S. Douthwaite, G. Nebbia, R. Batra, R. Martinez-Nunez, M. Shankar-Hari, J. D. Edgeworth, S. J. D. Neil, M. H. Malim and K. J. Doores, Nat. Microbiol, 2020, 5, 1598–1607 CrossRef CAS PubMed.
- A. Wajnberg, F. Amanat, A. Firpo, D. R. Altman, M. J. Bailey, M. Mansour, M. McMahon, P. Meade, D. R. Mendu, K. Muellers, D. Stadlbauer, K. Stone, S. Strohmeier, V. Simon, J. Aberg, D. L. Reich, F. Krammer and C. Cordon-Cardo, Science, 2020, 370, 1227–1230 CrossRef CAS PubMed.
- Y. Yang, M. Yang, Y. Peng, Y. Liang, J. Wei, L. Xing, L. Guo, X. Li, J. Li, J. Wang, M. Li, Z. Xu, M. Zhang, F. Wang, Y. Shi, J. Yuan and Y. Liu, Nat. Microbiol, 2022, 7, 423–433 CrossRef CAS PubMed.
- G. Beaudoin-Bussieres, A. Laumaea, S. P. Anand, J. Prevost, R. Gasser, G. Goyette, H. Medjahed, J. Perreault, T. Tremblay, A. Lewin, L. Gokool, C. Morrisseau, P. Begin, C. Tremblay, V. Martel-Laferriere, D. E. Kaufmann, J. Richard, R. Bazin and A. Finzi, MBio, 2020, 11, e02590 CrossRef CAS PubMed.
- P. J. M. Brouwer, T. G. Caniels, K. van der Straten, J. L. Snitselaar, Y. Aldon, S. Bangaru, J. L. Torres, N. M. A. Okba, M. Claireaux, G. Kerster, A. E. H. Bentlage, M. M. van Haaren, D. Guerra, J. A. Burger, E. E. Schermer, K. D. Verheul, N. van der Velde, A. van der Kooi, J. van Schooten, M. J. van Breemen, T. P. L. Bijl, K. Sliepen, A. Aartse, R. Derking, I. Bontjer, N. A. Kootstra, W. J. Wiersinga, G. Vidarsson, B. L. Haagmans, A. B. Ward, G. J. de Bree, R. W. Sanders and M. J. van Gils, Science, 2020, 369, 643–650 CrossRef CAS PubMed.
- A. Remuzzi and G. Remuzzi, Lancet, 2020, 395, 1225–1228 CrossRef CAS.
- X. C. Li, S. Y. Xu, M. Q. Yu, K. Wang, Y. Tao, Y. Zhou, J. Shi, M. Zhou, B. Wu, Z. Y. Yang, C. Zhang, J. Q. Yue, Z. G. Zhang, H. Renz, X. S. Liu, J. G. Xie, M. Xie and J. P. Zhao, J. Allergy Clin. Immunol., 2020, 146, 110–118 CrossRef CAS PubMed.
- J. Y. Ko, M. L. Danielson, M. Town, G. Derado, K. J. Greenlund, P. D. Kirley, N. B. Alden, K. Yousey-Hindes, E. J. Anderson, P. A. Ryan, S. Kim, R. Lynfield, S. M. Torres, G. R. Barney, N. M. Bennett, M. Sutton, H. K. Talbot, M. Hill, A. J. Hall, A. M. Fry, S. Garg, L. Kim and C.-N. S. Team, Clin. Infect. Dis., 2021, 72, E695–E703 CrossRef CAS PubMed.
- E. J. Williamson, A. J. Walker, K. Bhaskaran, S. Bacon, C. Bates, C. E. Morton, H. J. Curtis, A. Mehrkar, D. Evans, P. Inglesby, J. Cockburn, H. I. McDonald, B. MacKenna, L. Tomlinson, I. J. Douglas, C. T. Rentsch, R. Mathur, A. Y. S. Wong, R. Grieve, D. Harrison, H. Forbes, A. Schultze, R. Croker, J. Parry, F. Hester, S. Harper, R. Perera, S. J. W. Evans, L. Smeeth and G. Ben, Nature, 2020, 584, 430–436 CrossRef CAS PubMed.
- Y. Y. Chen, S. L. Klein, B. T. Garibaldi, H. F. Li, C. J. Wu, N. M. Osevala, T. S. Li, J. B. Margolick, G. Pawelec and S. X. Leng, Ageing Res. Rev., 2021, 65, 101205–101215 CrossRef CAS PubMed.
- C. O. Barnes, C. A. Jette, M. E. Abernathy, K. M. A. Dam, S. R. Esswein, H. B. Gristick, A. G. Malyutin, N. G. Sharaf, K. E. Huey-Tubman, Y. E. Lee, D. F. Robbiani, M. C. Nussenzweig, A. P. West and P. J. Bjorkman, Nature, 2020, 588, 682–687 CrossRef CAS PubMed.
- R. Vernet, E. Charrier, J. Grogg and N. Mach, Vaccines, 2021, 9, 770–778 CrossRef CAS PubMed.
|
This journal is © The Royal Society of Chemistry 2022 |