DOI:
10.1039/D2RA05725E
(Paper)
RSC Adv., 2023,
13, 1301-1311
Rapid detection of cancer DNA in human blood using cysteamine-capped AuNPs and a machine learning-enabled smartphone†
Received
11th September 2022
, Accepted 28th November 2022
First published on 5th January 2023
Abstract
DNA methylation occurs when a methyl group is added to a cytosine (C) residue's fifth carbon atom, forming 5-methylcytosine (5-mC). Cancer genomes have a distinct methylation landscape (Methylscape), which could be used as a universal cancer biomarker. This study developed a simple, low-cost, and straightforward Methylscape sensing platform using cysteamine-decorated gold nanoparticles (Cyst/AuNPs), in which the sensing principle is based on methylation-dependent DNA solvation. Normal and cancer DNAs have distinct methylation profiles; thus, they can be distinguished by observing the dispersion of Cyst/AuNPs adsorbed on these DNA aggregates in MgCl2 solution. After optimising the MgCl2, Cyst/AuNPs, DNA concentration, and incubation time, the optimised conditions were used for leukemia screening, by comparing the relative absorbance (ΔA650/525). Following the DNA extraction from actual blood samples, this sensor demonstrated effective leukemia screening in 15 minutes with high sensitivity, achieving 95.3% accuracy based on the measurement by an optical spectrophotometer. To further develop for practical realisation, a smartphone assisted by machine learning was used to screen cancer patients, achieving 90.0% accuracy in leukemia screening. This sensing platform can be applied not only for leukemia screening but also for other cancers associated with epigenetic modification.
1 Introduction
DNA methylation is an epigenetic modification involving the addition of a methyl group to the fifth carbon atom of the cytosine (C) residue to form 5-methylcytosine (5-mC) at CpG sites, the position where the cytosine residue is preceded by guanine (G) bases. This biological process plays a significant role in genomic imprinting and gene silencing, which are associated with the regulation of gene expression and hereditary characteristics.1,2 The pattern and level of the cytosine methylation that occurs across the entire genome define the epigenetic state of the cell, developing to certain genetic diseases and tumours, that have been broadly reported in various cancers, including leukemia, stomach, breast, lung, and colorectal cancer.2–6 The epigenetic reprogramming in these cancer genomes exhibits a distinct methylation landscape (Methylscape), a unique footprint for cancer genomes, in specific regions such as the promoter site, particularly in the CpG island. This Methylscape comprises clustered methylation at the regulatory regions, which has been found to be a common feature of most cancer types, implying that it could be employed as a universal cancer biomarker.7–9 The emergence of a platform to identify the Methylscape biomarker has the potential to vastly improve existing multi-cancer early detection (MCED) at its most treatable stages.
Various approaches to quantifying the level of DNA methylation have been extensively developed. The bisulfite conversion method, for example, has been frequently employed in the analysis of 5-mC.10–18 However, because of DNA degradation during bisulfite conversion, most bisulfite conversion-based techniques require high sample volumes and are frequently hampered by low amplification efficiency and polymerase chain reaction (PCR) bias. Enzyme-linked immunosorbent assay (ELISA)-based methylation assays were proposed to circumvent the use of bisulfite conversion and PCR amplification.19,20 These assays were reasonably straightforward, but they had low sensitivity and necessitated the use of many external controls for quantitative analysis. To address these concerns, methyl binding domain (MBD)-based assays were developed, in which MBD protein or another particular antibody was employed to detect methylated CpG sites within the target DNA sequence.15 In addition to bisulfite conversion and MBD-based approaches, high performance liquid chromatography (HPLC) and mass spectrometry (MS) have been utilised for direct DNA methylation measurement.21–23 Although HPLC and MS-based technologies directly detect DNA methylation, they also require a considerable volume of input DNA, limiting their applicability in typical clinical applications. A number of fluorescence readout-based approaches have been effectively employed to assess DNA methylation in recent years.24–27 Because of its greater sensitivity, fluorescence resonance energy transfer (FRET) is the most extensively used optical readout method in DNA methylation study.28 Semiconductor quantum dots (QDs) have also been used in fluorescence-based methylation assays to increase assay performance since they have broader absorption spectra and higher detection efficiency, compared to traditional fluorophores.26 Other optical readout methods for assessing DNA methylation include Raman spectroscopy,27 surface plasmon resonance (SPR),29 electrochemiluminescence (ECL),30 and colorimetry.31 However, further study is needed to establish the rapid Methylscape detection that is suitable for cancer screening in large patient cohorts for early treatment.
Colorimetric assays for DNA methylation detection have recently acquired interest since they are simple, reliable, cost effective, portable, and targets can be observed with the naked eye.32–36 As native nucleic acids do not absorb in the visible region, colorimetric biosensors have been developed using nanoscale organic dyes, conjugated polymers, enzymes connected to a chromogen, and metallic nanoparticles. Gold nanoparticles (AuNPs) have been widely used in the development of colorimetric biosensors,37,38 in which the target molecules can be detected as a colour change in the colloidal solution. Typical AuNP-based biosensor is replied on the salt-induced aggregation of AuNPs. However, the development of these nanoparticles to detect the Methylscape biomarker has received little attention.
Analysing the physicochemical properties alone could serve as a straightforward and effective method of determining the Methylscape biomarker for MCED. Normal DNA contains considerable amounts of dispersed 5-mC throughout the genome, but cancer cells' DNA is hypermethylated at the methylation landscape and tends to form clusters of 5-mC in the CpG rich regulatory regions. As a result of the heterogeneous methylation landscape, distinct solvations in aqueous solutions occur. Apart from the entire genome, small fragments of DNA circulate freely in the peripheral blood of healthy and diseased individuals. These cell-free DNA (cfDNA) molecules have been reported to originate from dying cells, reflecting the body's ongoing cell death.2,39,40 Circulating cfDNA methylation patterns contain important information about recent cell death events in the body, and they are a fundamental marker of cell identity. This Methylscape biomarker, which is typical of cancer DNAs and not present in DNAs from healthy individuals, would drive a unique self-assembly process, and regardless of their global methylation content, creating a distinctive assembly footprint that can be used to infer their clinicopathological state. Recently, we reported the insightful discussion of the self-assembly of the cfDNA in aqueous solution using molecular dynamics (MD) simulation.41 Our in silico study demonstrated that cfDNA clusters in aqueous solution in a methylation-dependent manner, which is due primarily to the higher hydrophobic force caused by the methyl group addition. Due to its physicochemical characteristics, the methylation-dependent assembly of DNA in solution can be used for early cancer diagnosis.
In the light of this, we present a simple and straightforward sensing platform for Methylscape detection based on Cyst/AuNP assemblies, which could be completed in two simple processes, as depicted in Fig. 1. To begin with, aqueous solution is used to dissolve the DNA fragments. Cyst/AuNPs are then added to the mixed solution. The Methylscape of normal and malignant DNAs differs, resulting in distinct self-assembly patterns for the DNA fragments. Normal DNA aggregates loosely, culminating in the aggregation of Cyst/AuNPs. This results in the red-shifting property of Cyst/AuNPs, which may be observed by the naked eye as a red-to-purple colour change. In contrast, the distinctive methylation profile of cancer DNA results in the creation of larger clusters of DNA fragments. As a result, the solution's colour does not change as Cyst/AuNPs are adsorbed and disseminated throughout the aggregate. This straightforward sensing technique was computationally investigated and recently published elsewhere.41 In this study, we proved the practicality of this sensing platform and demonstrated its capability for cancer screening. Additionally, a smartphone-based biosensor has been developed to facilitate and expedite cancer screening. Machine learning-enabled smartphone system without using any optical hardware smartphone attachment has been developed for the simple detection. Our work can explore a rapid multi-cancer early detection strategy that could pave the way for the development of a universal cancer screening tool.
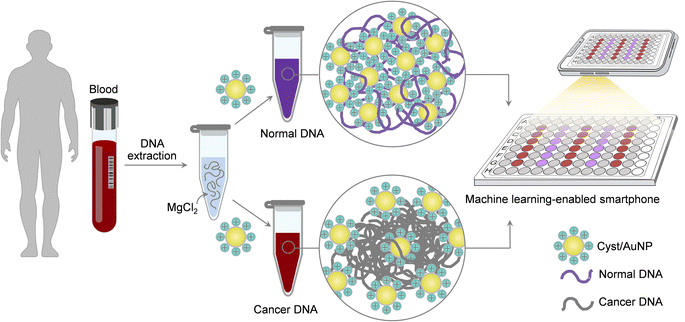 |
| Fig. 1 A simple and rapid sensing platform for cancer screening via assemblies of Cyst/AuNPs could be completed in two simple steps. Firstly, the DNA fragments are dissolved in MgCl2 solution. Subsequently, Cyst/AuNPs are added into the mixed solution. The difference in the Methylscape biomarker of cancer and normal DNA causes different self-assembly patterns, resulting in the red-shift phenomenon of Cyst/AuNPs, which can be precisely identified by the machine learning-assisted mobile phone application. | |
2 Experimental
2.1 Materials
Methylated DNA (mDNA) and unmethylated DNA (uDNA) were purchased from Ward Medic. Adenomatous polyposis coli (APC) is classified as one of the tumor suppressor genes42 linked to a variety of cancers, for example, leukemia, esophageal, stomach, colon, liver, and lung cancer.28,43–45 It has been reported that the APC gene has been found in both serum and plasma.46 Therefore, the APC gene is a remarkable candidate as a universal cancer biomarker. A fragment of the APC gene, which is frequently found in the blood of cancer patients, was used as a model for cancer DNA in this study. The sequence of the promoter region of the APC gene is 5′-CAC TGC GGA GTG CGG GTC GGG AAG CGG AGA GAG AAG CAG CTG TGT AAT CCG CTG GAT GCG GAC CAG GGC GCT CCC CAT TCC CGT CGG GAG CCC GCC GAT TGG CTG GGT GTG GGC GCA CGT GAC CGA CAT-3′, a total of 129 nucleotides with 14 CpG sites. Sodium borohydride (NaBH4, >98%) was purchased from Merck Chemicals. Gold(III) chloride trihydrate (HAuCl4, 99%), sodium phosphate dibasic (Na2HPO4), sodium phosphate monobasic monohydrate (NaH2PO4) and magnesium chloride anhydrous (MgCl2) were purchased from Sigma-Aldrich. Phosphoric acid (H3PO4) was purchased from Univar USA Inc. Leukemia cancer patient blood samples were obtained from Srinagarind Hospital, Khon Kaen, Thailand. The relevant ethical approval was obtained from Khon Kaen University, Thailand, for blood patient samples analysis presented in this study.
2.2 Apparatus
An absorbance measurement was recorded on a fluorescence microplate reader (SpectraMax M5). Transmission electron microscopy (TEM) measurements were performed on a Tecnai (FEI 5022/22 Tecnai G2 20 S Twin, CR). Dynamic light scattering (DLS) and zeta potential measurement were measured using the Malvern Zetasizer. The pH of the solution was measured using a mettler Toledo LE438.
2.3 Synthesis of Cyst/AuNPs
Cyst/AuNPs were synthesized by a reduction method.47 Cyst/AuNPs were prepared by reducing HAuCl4 with NaBH4. Briefly, 213 mM cysteamine hydrochloride and 0.1 M HAuCl4 were mixed in a glass vial. The mixture was stirred for 20 min at room temperature in the dark. Freshly prepared 10 mM NaBH4 was then added into the mixed solution under vigorous stirring, and the mixture was further stirred overnight in the dark. The resulting wine-red solution was filtered by 0.22 (or 0.45) μm filter and stored in the refrigerator (4 °C) until use. The as-prepared Cyst/AuNPs were characterised with UV-Vis absorption spectra, transmission electron microscopy (TEM) and dynamic light scattering. The concentration of the Cyst/AuNPs solution was estimated by the original concentration of the gold solution. The as-prepared Cyst/AuNPs were wine-red and showed a characteristic absorption peak at 525 nm, which was ascribed to the surface plasmon resonance of the Cyst/AuNPs. The Cyst/AuNPs solution can be highly stabilised against aggregation due to the positive capping agent's electrostatic repulsion between Cyst/AuNPs.
2.4 DNA sample preparation
The blood samples were derived from the patients in Srinagarind Hospital, Khon Kaen, Thailand, and stored in K2-EDTA tubes. The blood samples used in this study were left-over from the laboratory, of which its protocols were approved by the Ethics Committee of Khon Kaen University, Faculty of Medicine, Khon Kaen, Thailand (HE654010). 4 ml of lysis solution (1% Triton x-100, 0.32 M Saccharose, 5 mM MgCl2, 10 mM Tris–HCl) were added to the blood sample in 2 ml volumes, mixed by vortexing and inverting thoroughly for 5 min. Subsequently, the mixture was centrifuged at 10
000 g for 5 min. The supernatant was discarded. For the pellet, 1 ml of lysis solution was added and the vortexing, inverting, and centrifuging steps were repeated. Then, 200 μl of enzyme reaction (1% SDS, 5 mM EDTA–Na2, 10 mM Tris–HCl) and 8 μl of 1 mg ml−1 RNase A were added to the pellet and mixed gently, and it was incubated at room temperature (25–30 °C) for 5 min. After that, 10 μl of 17 mg ml−1 proteinase K was added to the pellet solution and mixed gently. The mixture was then incubated for 1 h at 60 °C in heating block before 300 μl of 7.6 M NaI solution (7.6 M NaI, 20 mM EDTA–Na2, 40 mM Tris–HCl) was subsequently added to the tube and mixed gently a few times by inversion. Then, 500 μl of 100% isopropanol was added and mixed well by inverting for 1 min. The mixture was then centrifuged at 10
000g for 10 min. The supernatant was then transferred to a fresh tube, and 1 ml of 40% isopropanol was added. The tube was mixed by inverting for 1 min, and was centrifuged for 5 min at 10
000g. The supernatant was discarded, and 1 ml 70% ethanol was added. The pellet was tapped gently, followed by centrifuging at 13
000g for 5 minutes and then removed the supernatant. The pellet was air-dried in a laminar air flow, and the dried pellet was resuspended in 20–40 μl in DI water and frozen at −20 °C or −80 °C until use.
2.5 Methylscape detection
According to our recent study based on MD simulations,41 the adsorption of Cyst/AuNPs onto DNA clusters in the presence of MgCl2 can distinguish between methylated and unmethylated DNA aggregates, suggesting a simple approach for cancer screening. In the light of this, we investigated the effect of magnesium ions on the increased stability of commercially available methylated and unmethylated DNAs in the sensor system, with the aim of optimising the differentiation of these two DNA variants. To begin with, MgCl2 (10 mM, 15 μl) was added to the DNA solution (30 nM, 15 μl) and the mixed solution was thoroughly mixed and left at room temperature for 15 minutes. Then, 10 nM Cyst/AuNPs (15 μl) were added to the mixed solution and the volume was adjusted by the addition of 105 μl phosphate buffer solution at a pH of 7.0 (0.1 mM). Subsequently, the absorbance of the mixture was measured at 525 and 650 nm. In the absence of MgCl2, a 15 μl phosphate buffer solution was added to the DNA solution and incubated for 15 minutes at room temperature. Then, 15 μl Cyst/AuNPs (10 nM) were added to the mixed solution and 105 μl of phosphate buffer solution was then added to reach the same volume as the system with MgCl2. Then, the mixture was measured for the absorbance at 525 and 650 nm. The Methylscape detection is summarised schematically in Fig. S1.† ΔA650/525 is utilised to determine the methylation-dependent dispersion of Cyst/AuNPs in the solution in order to discriminate cancerous and normal DNAs, where |
ΔA650/525 = (AAuNPs+DNA650/AAuNPs+DNA525) − (AAuNPs650/AAuNPs525)
| (1) |
2.6 Machine learning-based biosensing
In the present work, we investigated the possibility of using machine learning to convert the colour of Cyst/AuNPs solutions into either normal or cancer DNA using an image of a 96-well plate taken with a smartphone camera. The determination of cancer DNA by a smartphone equipped with machine learning was compared to an approach based on spectroscopy. Following the process described in Section 2.5, these were carried out on the DNA extracted from real blood samples. We compared two well-known machine learning models: Random Forests (RF) and Support Vector Machine (SVM) through the binary classification task, negative (normal DNA) and positive (cancer DNA). Images of 15 96-well plates containing normal DNA, cancer DNA, and the reference Cyst/AuNPs solution were captured for data preparation using a customized iOS application on an iPhone 12. Each plate contained five unknown columns, each of which could be populated with either normal or cancer DNA. We photographed each plate twice and indicated the actual chemical class of the substance contained in each well. This step resulted in the collection of 30 images, each of which contained 150 unknown columns. We constructed a feature vector for each data point by extracting the colour intensity of the red channel from the centre region of each well; six wells in the centre column were designated as unknown substances to be predicted, while 18 wells surrounding the centre column were designated as wells filled with the reference substance. As a result, we concatenated 24 colour intensity values from six unknown wells and 18 reference wells to create a 24-dimension feature vector for each unknown substance. We ended up collecting 150 data points, 100 of which were positive and 50 of which were negative. The data was then divided into 100 training samples and 50 test samples based on the plate; samples from the same plate will be included in either the training or test samples. The machine learning models were implemented using the Scikit-Learn package,48 with the best parameters chosen using a 5-fold cross-validation procedure on the training set. The optimal parameters for random forests were 800 trees with a maximum depth of 5 and a minimum sample split of 2. The best parameters for support vector machine were Radial Basis Function Kernel (RBF kernel) with C = 1 and γ = 0.001.
3 Results and discussion
3.1 Nanoprobe characterisation
To evaluate the efficacy of our approach in detecting the Methylscape biomarker, we used positively charged AuNPs to perform a simple cancer detection based on the different physicochemical properties of DNA. The abnormal 5-mC's physicochemical properties are primarily due to its hydrophobic nature and larger size than an ordinary cytosine.2,49 Due to their distinct hydrophobic properties, cancer and healthy DNA agglomerate differently in aqueous solutions. The difference in the Methylscape of the DNA results in distinct patterns of self-assembly for the DNA fragments. The adsorption of positively charged AuNPs onto DNA aggregates may indicate distinct self-assembly patterns in normal and cancer genomes due to the presence of different Methylscapes. In this study, Cyst/AuNPs were used as a nanoprobe for the detection of Methylscape. Cyst/AuNPs were prepared according to the reported literature.47 The UV-Vis spectrum of the Cyst/AuNPs exhibited an absorption peak at a wavelength of 525 nm (Fig. 2a), which is due primarily to the localised surface plasmon resonance (LSPR) phenomenon found in Cyst/AuNPs. Based upon the absorption of the solution, the concentration of as-prepared Cyst/AuNPs was approximated to be 10 nM using UV-Vis spectroscopy with an extinction coefficient of 3.67 × 108 M−1 cm−1 at the wavelength of 525 nm. The transmission electron microscopy image revealed that the nanoparticles were sphere-shaped nanostructure with a diameter of 33.5 ± 4.8 nm as shown in the ESI (Fig. S2†). The zeta potential of the Cyst/AuNPs was 31.7 ± 0.3 mV, indicating that the positively charged cysteamine stabilised the AuNP surfaces. Due to the electrostatic repulsion force, the Cyst/AuNPs exhibit excellent dispersion and resistance to aggregation. The Cyst/AuNPs was shown to be extremely stable, with the Cyst/AuNPs solution remaining stable for up to nine weeks without aggregation (Fig. S3†), indicating that it could be a promising candidate for a nanoprobe in a practical application.
 |
| Fig. 2 (a) Cyst/AuNPs absorption spectra under various conditions. A TEM image of Cyst/AuNPs is shown in (b). TEM images of Cyst/AuNPs with mDNA and uDNA are shown in (c) and (d), respectively. TEM images of Cyst/AuNPs with mDNA and uDNA in the presence of 1 mM MgCl2 are shown in (e) and (f), respectively. Each TEM image includes a scale bar. | |
3.2 Development and evaluation of the nanoparticle-based Methylscape detection
To apply Cyst/AuNPs in the Methylscape detection of cancer DNA in human blood, a fragment of the APC gene, fund in both serum and plasma46 of leukemia, esophageal, stomach, colon, liver, and lung cancer patients,28,44,45 is chosen as an initial model for the development of a Methylscape sensing platform. The sequence of the APC gene is provided in the Materials section. A fragment of the APC gene with and without methylation was used as models for DNA with varying Methylscapes. As previously reported by our computational study, distinct Methylscapes of these DNAs result in different agglomeration sizes. Different adsorption patterns of positively charged AuNPs onto negatively charged aggregates result in different dispersions of these nanoparticles.
To develop the sensor for Methylscape detection, the interaction between Cyst/AuNPs and DNA with different methylation landscapes (with and without methylation) has been observed. As shown in Fig. 2a, the AuNP solution shows a characteristic absorption peak at 525 nm, which is due primarily to the LSPR phenomenon found in spherical Cyst/AuNPs. This corresponds to the dispersion of Cyst/AuNPs in the solution, as illustrated in Fig. 2b. The color of the solution appears in red-wine. In the presence of methylated DNA (mDNA), the absorption spectrum was obviously changed owing to the electrostatic attractions between Cyst/AuNPs and the mDNA aggregate. This resulted in the aggregation of the Cyst/AuNPs, as confirmed by the TEM image shown in Fig. 2c. In the case of unmethylated DNA (uDNA), the mixture of Cyst/AuNPs and uDNA led to a dramatic aggregation of Cyst/AuNPs in a similar manner to that observed in mDNA (Fig. 2d). These findings show that the hydrophobic force that maintains mDNA aggregation is weaker than the electrostatic interactions that cause the DNA assembly to collapse. As a result, the Cyst/AuNPs in both mDNA and uDNA are equally aggregated in the solution. This suggests that detecting Methylscape based upon this approach in aqueous solution is impossible.
Electrostatic screening was employed to decrease the attractive interactions between the nanoparticles and DNA aggregates while retaining the assembly of the Cyst/AuNPs used for Methylscape indication. To preserve DNA aggregation and enhance DNA stability, 1 mM MgCl2 was added to the solution. In the case of the mDNA aggregate, the addition of MgCl2 results in the electrostatic screening. The hydrophobic force contributed by the methyl group and the electrostatic attraction between the Cyst/AuNPs and DNA backbone are balanced, maintaining the aggregation of the methylated DNA in the presence of the Cyst/AuNPs. This suggests that the densely mDNA aggregate will influence the dispersion of the Cyst/AuNPs, adsorbed on the DNA assemblage, in a different manner from the loosened uDNA aggregates. The absorption spectra of both cases revealed different absorption behaviours of both cases. This was confirmed by the TEM image, as illustrated in Fig. 2e and f for mDNA and uDNA, respectively. In a nutshell, Methylscape detection could be accomplished through the dispersion of Cyst/AuNPs adsorbed on DNA agglomerate while being electrostatically screened by MgCl2.
To further investigate the role of electrostatic screening in the Methylscape detection, the hydrodynamic size and zeta potential of DNA clusters under different conditions were determined, as summarised in Table 1. In the aqueous solution, the hydrodynamic size (dH) of the mDNA aggregate was much smaller than that of the uDNA aggregate (ΔdH = 91.1 ± 70.6 nm) due to the increased hydrophobic force of the methyl group in mDNA. In the presence of MgCl2, the difference of the hydrodynamic size between mDNA and uDNA (ΔdH = 44.0 ± 47.4 nm) is smaller than that in aqueous solution. However, the addition of Cyst/AuNPs into the DNA solution resulted in an electrostatic attraction between these nanoparticles and the DNA aggregate. In aqueous solution, in the presence of Cyst/AuNPs, the uDNA aggregate was disintegrated in a similar manner to mDNA due to the strong electrostatic attraction between the Cyst/AuNPs and DNA. However, in the presence of MgCl2, once Cyst/AuNPs were added into the DNA solution, the hydrophobic force contributed from the methyl group and the electrostatic attraction between the Cyst/AuNPs and DNA backbone are balanced, maintaining the aggregation of the mDNA and uDNA in the presence of the Cyst/AuNPs. This indicates that the densely mDNA aggregate will influence the dispersion of the Cyst/AuNPs, adsorbed on the DNA assemblage, in a different manner from the loosened uDNA aggregates. This implies that Methylscape detection could be accomplished through the dispersion of Cyst/AuNPs adsorbed on DNA agglomerate while being electrostatically screened by MgCl2.
Table 1 Hydrodynamic size and zeta potential of DNA aggregates with (+) and without (−) MgCl2 determined by DLS technique. This experiment was performed under the following conditions: MgCl2 concentration, 1 mM; DNA concentration, 3 nM; incubation time, 15 min
Sample |
MgCl2 |
Size (nm) |
Zeta potential (mV) |
mDNA |
− |
632.4 ± 45.4 |
−27.2 ± 4.7 |
uDNA |
− |
723.5 ± 54.1 |
−23.6 ± 1.0 |
mDNA |
+ |
717.1 ± 37.6 |
−16.7 ± 2.0 |
uDNA |
+ |
761.1 ± 28.8 |
−11.5 ± 0.2 |
3.3 Sensing performance optimisation
Based on the detection principle shown in Fig. 1, the developed biosensor shows a characteristic peak of the UV-Vis spectrum at λ = 525 nm. The absorption of the sensor at this signature wavelength decreases once Cyst/AuNPs interact with the DNA aggregate. This is due to the adsorption of the positively charged AuNPs on the negatively charged DNA, resulting in the red shift of the absorption spectrum. The colour of the AuNP solution therefore changes from red to purple-blue. To optimise parameters affecting the analytical figure of merit of the sensor, ΔA650/525 was optimised for all related parameters including (i) the concentrations of MgCl2; (ii) Cyst/AuNPs concentration; (iii) DNA concentration; and (iv) incubation time. The strong electrostatic interactions between Cyst/AuNPs and DNA aggregate result in the disintegration of the agglomerated DNA, as discussed in the previous section. Electrostatic screening in the presence of MgCl2 can reduce the strong attractive interactions between Cyst/AuNPs and DNA, allowing normal and cancer DNA to be distinguished in a divalent electrolyte. The concentration of MgCl2 was initially varied to optimise the analytical figure of merit of the sensor. MgCl2, however, may cause nanoparticle aggregation. The absorbance of Cyst/AuNPs solutions with different MgCl2 concentrations (0–100 mM) was measured to determine the optimal condition, the MgCl2 concentration that Cyst/AuNPs remain dispersed in the solution. The absorbance of Cyst/AuNPs at 525 nm decreased with increasing MgCl2 concentrations, as shown in Fig. S4.† The solution colour appeared red at low MgCl2 concentrations (1–10 mM), while increasing MgCl2 concentrations resulted in a dramatic decrease in absorbance at λ = 525 nm, indicating that the nanoparticles were aggregated provided that the MgCl2 concentrations were greater than 10 mM, as shown in Fig. 3a. Thus, 1 mM MgCl2 was used for further studies to reduce strong electrostatic attraction between DNA and the nanoparticles while maintaining their dispersion in solution.
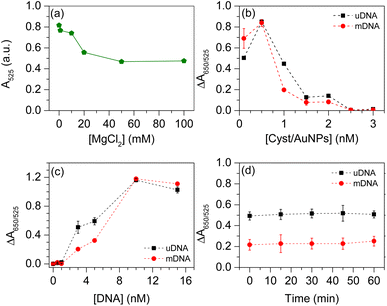 |
| Fig. 3 (a) AuNP absorption at 525 nm (A525) in the presence of MgCl2 with different concentrations ranging from 0–100 mM. (b) ΔA650/625 of AuNP solution in the presence of either mDNA or uDNA with varying Cyst/AuNP concentrations. (c) ΔA650/625 of AuNP solution in the presence of either mDNA or uDNA with different DNA concentrations. (d) ΔA650/625 of AuNP solution as a function of incubation time. | |
To further optimise the conditions, the effect of Cyst/AuNP concentration on sensing performance was evaluated by varying nanoparticle concentrations from 0.1–3 nM. It was discovered that different concentrations of Cyst/AuNPs interact with both mDNA and uDNA aggregates in distinct manners, as shown in Fig. 3b. The ΔA650/525 of uDNA (0.450) was much higher than that of mDNA (0.190) at a concentration of 1 nM Cyst/AuNPs. This suggests that in the MgCl2 solution, uDNAs were loosely agglomerated, causing Cyst/AuNPs to be closer together than those adsorbed on the mDNA aggregate. For further optimisation, Cyst/AuNPs at a concentration of 1 nM were used.
Another crucial factor for Methylscape detection is DNA concentration. The DNA concentration optimisation in the present study was performed in the range of 0.5–15 nM, in which the concentrations of MgCl2 and Cyst/AuNPs were fixed at 1 mM and 1 nM, respectively. As depicted in Fig. 3c, at low (0.5–1 nM) and high (10–15 nM) DNA concentrations, the ΔA650/525 of uDNA and mDNA was not significantly different. These DNA concentrations cannot be used to distinguish between the two forms of DNA. When the concentration of DNA is between 3–5 nM, the ΔA650/525 of Cyst/AuNPs treated with both uDNA and mDNA shows a significant difference. This DNA concentration range provides the most distinction between uDNA and mDNA. Thus, the concentration of DNA at 3 nM was used to further optimise the sensing performance.
Finally, the incubation time between the DNA and Cyst/AuNPs was observed. This was investigated in the time range of 0–60 min. As shown in Fig. 3d, the ΔA650/525 of Cyst/AuNPs with uDNA and mDNA remained relatively constant as the reaction time increased. This suggests that the reaction time between the nanoparticles and DNA was rapid. Therefore, to ensure the complete interactions, the incubation time at 15 minutes was selected to develop the sensor for cancer screening based upon the Methylscape detection.
After optimising the conditions, we obtained the optimal parameters as follows: MgCl2 concentration, 1 mM; Cyst/AuNPs concentration, 1 nM; DNA concentration, 3 nM. The incubation time of 15 minutes was selected. To demonstrate that these conditions can be used for Methylscape detection, the ΔA650/525 of Cyst/AuNPs solution in the presence of either mDNA or uDNA under the optimised conditions was observed. It was revealed that the developed sensor can obviously distinguish DNA with a distinct methylation profile, as shown in Fig. 4a. The averages of ΔA650/525 of Cyst/AuNPs solution (n = 4) in the presence of uDNA and mDNA were 0.5581 ± 0.0572 and 0.2275 ± 0.0542, respectively. This is in good agreement with our previously reported MD simulation.41 Our simulations revealed that the solvation of DNA is methylation-dependent as the addition of the methyl group to cytosine residues results in an increased hydrophobic force. Our MD simulation demonstrated that the nanoparticles are adsorbed on the methylated DNA aggregate in a different manner from the unmethylated one in the presence of divalent electrolyte. The backbone of the oligonucleotide plays a significant role in the adsorption mechanism onto the gold surface. The methyl group increases the π electron density in the pyrimidine ring due to the electron donating mechanism as revealed by the density functional theory (DFT) study. The adsorption energy of 5-mC onto the AuNP surface is lower than that of C, resulting in increased binding affinity onto the gold surface. Due to the enhanced hydrophobic force, the dense aggregation of the mDNA can prevent the aggregation of the Cyst/AuNPs as these nanoparticles are dispersed exclusively on the outer layer of the mDNA aggregate, as schematically illustrated in Fig. 4b. This results in different dispersions of the Cyst/AuNPs adsorbed on the methylated and unmethylated DNA aggregates.
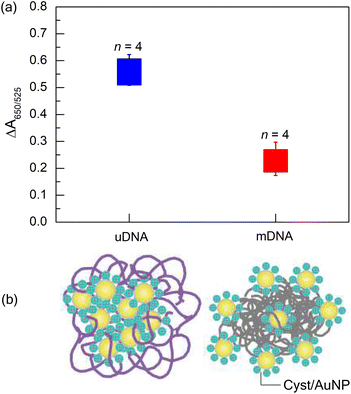 |
| Fig. 4 (a) Box plot showing the relative absorbance values ΔA650/525 of Cyst/AuNPs solutions in the presence of uDNA (blue) and mDNA (red) under the optimised conditions: MgCl2 concentration, 1 mM; Cyst/AuNPs concentration, 1 nM; DNA concentration, 3 nM; incubation time; 15 min (n = 4). (b) Schematic illustration of Cyst/AuNPs absorbed on (left) unmethylated and (right) methylated DNA agglomerates. | |
3.4 Cancer screening in real blood samples
To extend the sensing platform's practicality, we applied a sensor that is successful in distinguishing mDNA and uDNA in real blood samples. Typically, epigenetic changes like DNA methylation have been extensively reported. The methylation pattern of CpG sites regulates gene expression epigenetically. In almost all cancer types, extensive changes in DNA methylation have been observed, resulting in an alternation in gene expression that promotes oncogenesis. Particular changes in methylation profiles are reproducibly found in specific cancer types such as leukemia, stomach, breast, lung, and colorectal cancer.2–6,50–55 As a model for other cancers associated with DNA methylation, we used blood samples from leukemia patients and healthy controls to investigate the utility of the methylation landscape (Methylscape) in differentiating leukemia cancers. This Methylscape biomarker not only outperformed current methods for rapid leukemia screening, but it also demonstrated the feasibility of extending this approach to multi-cancer early detection using a simple and straightforward assay. To assess the clinical utility of the assay, we analysed DNA derived from the blood samples of 31 leukemia patients and 12 healthy individuals under the optimised conditions obtained from the previous section. We extracted the DNA from 12 healthy individuals and 31 leukemia patients. Clinical information of representative blood samples is summarised in Table S1.† As shown in the box plot in Fig. 5a, cancer samples provided a lower relative absorbance (ΔA650/525) than that of DNA derived from normal samples. This is due primarily to the enhanced hydrophobic force, which led to the dense aggregation of the cancer DNA that prevented the aggregation of Cyst/AuNPs. As a result, these nanoparticles are dispersed exclusively on the outer layer of the cancer aggregate, as discussed in the previous section. This results in different dispersions of the Cyst/AuNPs adsorbed on the normal and cancer DNA aggregates. The ROC curve was then generated by varying the threshold from the minimum of ΔA650/525 to its maximum. As shown in Fig. 5b, the area under the ROC curve (AUC = 0.9274) derived from our developed sensor demonstrates a high level of sensitivity and specificity. According to the box plot, the whisker representing the lowest point of the normal DNA group and the whisker representing the highest point of the cancer DNA group are not well separated; therefore, logistic regression is used to distinguish between the normal and cancer groups. On the basis of a given set of independent variables, it estimates the probability of an event, such as cancer or a normal group, occurring. The dependent variable is bounded between 0 and 1; thus, the outcome is a probability. We determined the probability of cancer identification (P) in term of ΔA650/525 as follows, |
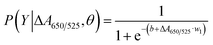 | (2) |
where b = 5.3533 and w1 = −6.965. For sample classification, a probability less than 0.5 indicates a normal sample, whereas a probability of 0.5 or greater implies a cancer sample. Fig. 5c depicts the confusion matrix generated by the leave-one-out cross validation used to validate the robustness of our classification method. This approach's diagnostic test evaluation, including sensitivity, specificity, positive predictive value (PPV), and negative predictive value (NPV), is summarised in Fig. 5d. The calculations for these values are explained in detail in the ESI.† It was revealed that the statistical diagnostic efficacy test provides an accuracy of 95.34%. These results demonstrate that an AuNP-based assay could potentially detect the presence of a small amount of cancer DNA (3 nM) in a rapid and cost-effective manner.
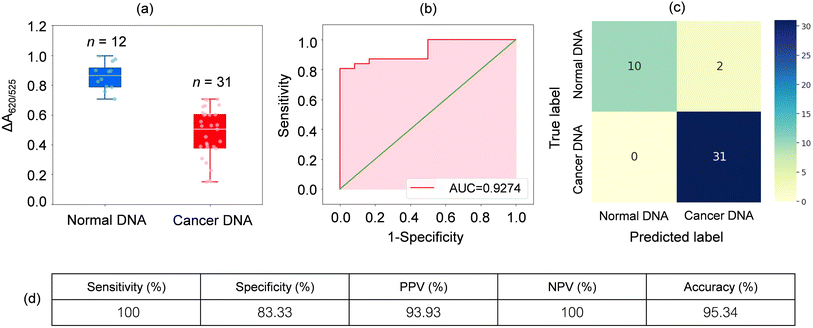 |
| Fig. 5 (a) Box plot showing the relative absorbance values (ΔA650/525) of Cyst/AuNPs solutions in the presence of normal (blue) and cancer (red) DNAs under the optimised conditions: MgCl2 concentration, 1 mM; Cyst/AuNPs concentration, 1 nM; the DNA concentration, 3 nM; the incubation time; 15 min. (b) The ROC analysis and diagnostic test evaluation. (c) The confusion matrix for diagnosing normal and cancer individuals. (d) Sensitivity, specificity, positive predictive value (PPV), negative predictive value (NPV) and the accuracy of the developed method. | |
3.5 Mobile phone-based biosensing
To further simplify sample testing without using a spectrophotometer, artificial intelligence (AI) was used for interpreting the solution colour and distinguishing normal and cancerous individuals. In the light of this, we utilised a computer vision to translate the colour of the Cyst/AuNPs solution into either normal DNA (purple) or cancer DNA (red) via the image of the 96-well plate taken by a mobile phone camera. AI was used to reduce the cost and increase the accessibility of interpreting the colour of the nanoparticle solution without using any optical hardware smartphone attachment. To analyse the DNA extracted from the blood, the mobile phone-based biosensing was set up as follows. In the image acquisition step, we prepared 15 96-well plates filled with normal DNA, cancer DNA, and the reference solution, as shown in Fig. 6a. Subsequently, we took 3–5 images of the 96-well plates along with recording the actual class of the solution in each well via our designed mobile phone application which provided 96-well plate to assist data capturing process (see Fig. S5† and 6b). With the AI, a machine learning model was developed to map feature vector (x) to the target class (y). In this step, we initially extracted a colour intensity from the red channel of the image from each well. We then constructed a feature vector (x) which consisted of colour intensities from the designated wells, eight from the unknown solution in which we wish to predict the class and 16 from the reference ones used as a reference colour, as shown in Fig. 6c. Finally, two machine learning models, including Random Forest (RF) and Support Vector Machine (SVM), were trained to build a model to map the prepared feature vector (x) to the target class (y), either normal or cancer DNA samples. As shown in Table 2, both RF and SVM models correctly classified the samples as positive and negative, demonstrating an accuracy of 90.0%. It is worth noting that the SVM model performed slightly better precision. This suggests that it is highly possible to use AI in mobile phone-based biosensing. More importantly, this mobile phone-based biosensor, which allowed us to develop a smartphone attachment-free optical sensor for Methylscape detection, can be conducted in ambient light, making it possible for its easy practical realisation.
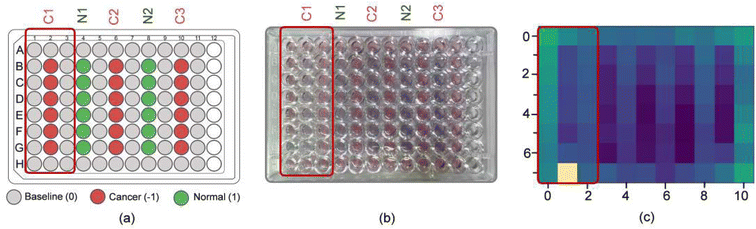 |
| Fig. 6 (a) Target classes of the solutions recorded while preparing samples for the image acquisition step. (b) An example image taken in the image acquisition step. (c) Colour intensities of the Cyst/AuNPs solution in each well of the 96-well plate extracted from the image (b). The colour intensity chosen in this study is the colour intensity of the red channel of the image. | |
Table 2 Comparing the performance of two machine learning models, including (i) random forests and (ii) support vector machine, on four performance metrics. Both models were trained to classify the input vector as either normal or cancer DNA when the input vector was composed of colour intensities extracted from 6 wells of the unknown solution and 18 wells of the reference one (24 dimensional feature vector)
Model |
Performance |
Accuracy |
Precision |
Recall |
F1-score |
Random forests |
0.90 |
0.93 |
0.90 |
0.92 |
Support vector machine |
0.90 |
0.97 |
0.90 |
0.93 |
4 Conclusions
A simple sensing platform for Methylscape detection has been successfully developed using Cyst/AuNPs. The sensing principle is based on the fact that the physicochemical properties of DNA in solution are methylation-dependent. This results in a distinct self-assembly of normal and cancer DNA, which can be distinguished by the dispersion of Cyst/AuNPs adsorbed on these DNA aggregates in MgCl2 solution. After optimising the conditions, the following parameters were determined to be optimal: 1 mM MgCl2; 1 nM Cyst/AuNPs; 3 nM DNA. A 15 minute incubation time was chosen to ensure complete interactions between Cyst/AuNPs and DNA aggregates. These conditions were initially used to distinguish methylated and unmethylated DNA, and the sensing platform was demonstrated by comparing the dispersion of Cyst/AuNPs in the MgCl2 solution in terms of the relative absorbance (ΔA650/525). To demonstrate the sensing platform's effectiveness, we used a sensor that is capable of discriminating between mDNA and uDNA in leukemia screening using real blood samples. The developed sensor demonstrates unequivocally that after the DNA extraction from real blood samples, an AuNP-based assay is capable of rapidly and cost-effectively detecting (15 minutes) the presence of a small amount of cancer DNA (3 nM) in leukemia patients, demonstrating 95.3% accuracy. To further simplify sample testing by eliminating the need for a spectrophotometer, machine learning was used to interpret the solution colour and discriminate between normal and cancerous individuals, achieving a 90.0% accuracy. This enables us to develop a smartphone-based biosensor for Methylscape detection that can be used in ambient light, enabling its practical implementation. This sensing platform demonstrated rapid early cancer screening, not just for leukemia screening, but also for other cancers associated with DNA methylation. Our study paves the way for the development of a rapid universal cancer screening method that is well-suited for screening large patient cohorts for early detection and treatment.
Conflicts of interest
There are no conflicts of interest to declare.
Acknowledgements
This project is funded by the National Research Council of Thailand (NRCT) and Khon Kaen University. This work is also supported by Innovation and Enterprise Affairs, Khon Kaen University.
References
- L. Syedmoradi, F. Esmaeili and M. L. Norton, Analyst, 2016, 141, 5922–5943 RSC.
- A. A. I. Sina, L. G. Carrascosa, Z. Liang, Y. S. Grewal, A. Wardiana, M. J. A. Shiddiky, R. A. Gardiner, H. Samaratunga, M. K. Gandhi, R. J. Scott, D. Korbie and M. Trau, Nat. Commun., 2018, 9, 4915 CrossRef.
- R. Straussman, D. Nejman, D. Roberts, I. Steinfeld, B. Blum, N. Benvenisty, I. Simon, Z. Yakhini and H. Cedar, Nat. Struct. Mol. Biol., 2009, 16, 564–571 CrossRef CAS.
- Z. D. Smith and A. Meissner, Nat. Rev. Genet., 2013, 14, 204–220 CrossRef CAS PubMed.
- D. Schübeler, Nature, 2015, 517, 321–326 CrossRef.
- M. M. Suzuki and A. Bird, Nat. Rev. Genet., 2008, 9, 465–476 CrossRef CAS.
- A. Koch, S. C. Joosten, Z. Feng, T. C. de Ruijter, M. X. Draht, V. Melotte, K. M. Smits, J. Veeck, J. G. Herman, L. Van Neste, W. Van Criekinge, T. De Meyer and M. van Engeland, Nat. Rev. Clin. Oncol, 2018, 15, 459–466 CrossRef CAS PubMed.
- C. Leygo, M. Williams, H. C. Jin, M. W. Chan, W. K. Chu, M. Grusch and Y. Y. Cheng, Dis. Markers, 2017, 2017, 1–13 CrossRef PubMed.
- S. Ø. Jensen, N. Øgaard, M.-B. W. Ørntoft, M. H. Rasmussen, J. B. Bramsen, H. Kristensen, P. Mouritzen, M. R. Madsen, A. H. Madsen, K. G. Sunesen, L. H. Iversen, S. Laurberg, I. J. Christensen, H. J. Nielsen and C. L. Andersen, Clin. Epigenet., 2019, 11, 158 CrossRef PubMed.
- M. J. Pajares, C. Palanca-Ballester, R. Urtasun, E. Alemany-Cosme, A. Lahoz and J. Sandoval, Methods, 2021, 187, 3–12 CrossRef CAS.
- E. Lianidou, Mol. Oncol., 2021, 15, 1683–1700 CrossRef CAS.
- H. Luo, W. Wei, Z. Ye, J. Zheng and R.-h. Xu, Trends Mol. Med., 2021, 27, 482–500 CrossRef CAS PubMed.
- W. Liang, Y. Zhao, W. Huang, Y. Gao, W. Xu, J. Tao, M. Yang, L. Li, W. Ping, H. Shen, X. Fu, Z. Chen, P. W. Laird, X. Cai, J.-B. Fan and J. He, Theranostics, 2019, 9, 2056–2070 CrossRef CAS PubMed.
- B. Luo, J. Zhou, Z. Li, J. Song, P. An, H. Zhang, Y. Chen, F. Lan, B. Ying and Y. Wu, Anal. Chem., 2022, 94, 6261–6270 CrossRef CAS.
- Q. Feng, M. Wang, L. Qin and P. Wang, ACS Sens., 2019, 4, 2615–2622 CrossRef CAS PubMed.
- Y. Zhang, C.-c. Li, X. Zhang, Q. Xu and C.-y. Zhang, Anal. Chem., 2020, 92, 10223–10227 CrossRef CAS.
- X. Chen, J. Huang, S. Zhang, F. Mo, S. Su, Y. Li, L. Fang, J. Deng, H. Huang, Z. Luo and J. Zheng, ACS Appl. Mater. Interfaces, 2019, 11, 3745–3752 CrossRef CAS PubMed.
- Y. Wang, C. Liu, T. Wang, T. Hong, H. Su, S. Yu, H. Song, S. Liu, X. Zhou, W. Mao and X. Zhou, Anal. Chem., 2016, 88, 3348–3353 CrossRef CAS PubMed.
- M. M. Sarabi and F. Naghibalhossaini, Cell Biochem. Funct., 2015, 33, 427–433 CrossRef CAS.
- B. Consortium, Nat. Biotechnol., 2016, 34, 726–737 CrossRef.
- H. Ushijima, R. Maekawa, E. Igarashi and S. Akashi, J. Am. Soc. Mass Spectrom., 2019, 30, 2335–2346 CrossRef CAS PubMed.
- P. Farinelli, A. Perera, B. Arango-Gonzalez, D. Trifunovic, M. Wagner, T. Carell, M. Biel, E. Zrenner, S. Michalakis, F. Paquet-Durand and P. A. R. Ekström, Cell Death Dis., 2014, 5, e1558 CrossRef CAS PubMed.
- X. Ye, L. Zhang, B. Chen, J. Li, Q. Yang, Q. Huang, J. Zhang, Y. Gao, Z. Li and C. Cai, Talanta, 2017, 169, 136–140 CrossRef CAS.
- J. A. Hambalek, J. E. Kong, C. Brown, H. E. Munoz, T. Horn, M. Bogumil, E. Quick, A. Ozcan and D. Di Carlo, ACS Sens., 2021, 6, 3242–3252 CrossRef CAS.
- Z. Taleat, K. Mathwig, E. Sudhölter and L. Rassaei, TrAC, Trends Anal. Chem., 2015, 66, 80–89 CrossRef CAS.
- Y. Ma, H. Zhang, F. Liu, Z. Wu, S. Lu, Q. Jin, J. Zhao, X. Zhong and H. Mao, Nanoscale, 2015, 7, 17547–17555 RSC.
- Y. Wang, E. Wee and M. Trau, Chem. Commun., 2016, 52, 3560–3563 RSC.
- M. Dadmehr, M. Hosseini, S. Hosseinkhani, M. Ganjali, M. Khoobi, H. Behzadi, M. Hamedani and R. Sheikhnejad, Biosens. Bioelectron., 2014, 60, 35–44 CrossRef CAS.
- M. N. Islam, S. Yadav, M. H. Haque, A. Munaz, F. Islam, M. S. Al Hossain, V. Gopalan, A. K. Lam, N.-T. Nguyen and M. J. Shiddiky, Biosens. Bioelectron., 2017, 92, 668–678 CrossRef PubMed.
- H.-F. Zhao, R.-P. Liang, J.-W. Wang and J.-D. Qiu, Biosens. Bioelectron., 2015, 63, 458–464 CrossRef CAS PubMed.
- E. Wee, T. Ngo and M. Trau, Clin. Epigenet., 2015, 7, 65 CrossRef.
- K. Chen, M. Zhang, Y.-N. Chang, L. Xia, W. Gu, Y. Qin, J. Li, S. Cui and G. Xing, Nanoscale Res. Lett., 2016, 11, 1–10 CrossRef.
- J. Huang, Y.-L. Liou, Y.-N. Kang, Z.-R. Tan, M.-J. Peng and H.-H. Zhou, Int. J. Nanomed., 2016, 11, 5335 CrossRef CAS.
- Z.-M. Li, T. Pi, X.-L. Yan, X.-M. Tang, R.-H. Deng and X.-J. Zheng, Spectrochim. Acta, Part A, 2020, 238, 118375 CrossRef CAS.
- F. Su, L. Wang, Y. Sun, C. Liu, X. Duan and Z. Li, Chem. Commun., 2015, 51, 3371–3374 RSC.
- Y.-Z. Lin and P.-L. Chang, ACS Appl. Mater. Interfaces, 2013, 5, 12045–12051 CrossRef CAS.
- C. Choodet, P. Toomjeen, W. Phanchai, P. Matulakul, R. Thanan, C. Sakonsinsiri and T. Puangmali, RSC Adv., 2019, 9, 17592–17600 RSC.
- P. Matulakul, D. Vongpramate, S. Kulchat, A. Chompoosor, R. Thanan, P. Sithithaworn, C. Sakonsinsiri and T. Puangmali, ACS Omega, 2020, 5, 17423–17430 CrossRef CAS PubMed.
- J. Moss, J. Magenheim, D. Neiman, H. Zemmour, N. Loyfer, A. Korach, Y. Samet, M. Maoz, H. Druid, P. Arner, K.-Y. Fu, E. Kiss, K. L. Spalding, G. Landesberg, A. Zick, A. Grinshpun, A. M. J. Shapiro, M. Grompe, A. D. Wittenberg, B. Glaser, R. Shemer, T. Kaplan and Y. Dor, Nat. Commun., 2018, 9, 5068 CrossRef PubMed.
- A. J. Bronkhorst, V. Ungerer and S. Holdenrieder, Biomol. Detect. Quantif., 2019, 17, 100087 CrossRef CAS PubMed.
- W. Phanchai, U. Srikulwong, A. Chuaephon, S. Koowattanasuchat, J. Assawakhajornsak, R. Thanan, C. Sakonsinsiri and T. Puangmali, ACS Appl. Nano Mater., 2022, 5, 9042–9052 CrossRef CAS.
- K. Aoki and M. M. Taketo, J. Cell Sci., 2007, 120, 3327–3335 CrossRef CAS PubMed.
- A. Nazha, B. Przychodzen, H. Makishima, M. Narkhede, M. E. Kalaycio, D. J. Seastone, H. Carraway, A. T. Gerds, S. Mukherjee, A. S. Advani, J. Carew, M. A. Sekeres and J. P. Maciejewski, J. Clin. Oncol., 2015, 33, 11047 Search PubMed.
- R. Sheikhnejad, M. Zohri, M. Shadmehr, M. Rahmani-Khalili, N. Doozande, Z. Farsad and K. Sheikhzade, Ann. Oncol., 2013, 24, 2705–2706 CrossRef CAS PubMed.
- I. Daugaard, D. Dominguez, T. E. Kjeldsen, L. S. Kristensen, H. Hager, T. K. Wojdacz and L. L. Hansen, Sci. Rep., 2016, 6, 1–11 CrossRef.
- L. Zhu, X. Li, Y. Yuan, C. Dong and M. Yang, Front. Oncol., 2021, 11, 1291 Search PubMed.
- M. Zhang, Y.-Q. Liu and B.-C. Ye, Analyst, 2011, 136, 4558–4562 RSC.
- F. Pedregosa, G. Varoquaux, A. Gramfort, V. Michel, B. Thirion, O. Grisel, M. Blondel, P. Prettenhofer, R. Weiss, V. Dubourg, J. Vanderplas, A. Passos, D. Cournapeau, M. Brucher, M. Perrot and É. Duchesnay, J. Mach. Learn. Res., 2011, 12, 2825–2830 Search PubMed.
- U. Srikulwong, W. Phanchai, P. Srepusharawoot, C. Sakonsinsiri and T. Puangmali, J. Phys. Chem. B, 2021, 125, 6697–6708 CrossRef CAS.
- H. Jiang, Z. Ou, Y. He, M. Yu, S. Wu, G. Li, J. Zhu, R. Zhang, J. Wang, L. Zheng, X. Zhang, W. Hao, L. He, X. Gu, Q. Quan, E. Zhang, H. Luo, W. Wei, Z. Li, G. Zang, C. Zhang, T. Poon, D. Zhang, I. Ziyar, R.-z. Zhang, O. Li, L. Cheng, T. Shimizu, X. Cui, J.-k. Zhu, X. Sun and K. Zhang, Signal Transduction Targeted Ther., 2020, 5, 3 CrossRef CAS PubMed.
- V. Moisoiu, A. Stefancu, S. D. Iancu, T. Moisoiu, L. Loga, L. Dican, C. D. Alecsa, I. Boros, A. Jurj, D. Dima, C. Bagacean, R. Tetean, E. Burzo, C. Tomuleasa, F. Elec and N. Leopold, Anal. Bioanal. Chem., 2019, 411, 7907–7913 CrossRef CAS.
- M. A. Kerachian, A. Javadmanesh, M. Azghandi, A. Mojtabanezhad Shariatpanahi, M. Yassi, E. Shams Davodly, A. Talebi, F. Khadangi, G. Soltani, A. Hayatbakhsh and K. Ghaffarzadegan, Sci. Rep., 2020, 10, 2813 CrossRef CAS.
- C.-c. Li, Z.-y. Wang, L.-j. Wang and C.-y. Zhang, Biosens. Bioelectron., 2019, 144, 111695 CrossRef CAS.
- G. M. Dexheimer, J. Alves, L. Reckziegel, G. Lazzaretti and A. L. Abujamra, Dis. Markers, 2017, 2017, 5472893 Search PubMed.
- Y. Li, Q. Xu, N. Lv, L. Wang, H. Zhao, X. Wang, J. Guo, C. Chen, Y. Li and L. Yu, J. Hematol. Oncol., 2017, 10, 41 CrossRef.
Footnote |
† Electronic supplementary information (ESI) available: Description of data analysis, additional experimental method and results, and clinical information of representative DNA samples. See DOI: https://doi.org/10.1039/d2ra05725e |
|
This journal is © The Royal Society of Chemistry 2023 |
Click here to see how this site uses Cookies. View our privacy policy here.