Exploring conformational landscapes and binding mechanisms of convergent evolution for the SARS-CoV-2 spike Omicron variant complexes with the ACE2 receptor using AlphaFold2-based structural ensembles and molecular dynamics simulations†
Received
3rd April 2024
, Accepted 9th June 2024
First published on 10th June 2024
Abstract
In this study, we combined AlphaFold-based approaches for atomistic modeling of multiple protein states and microsecond molecular simulations to accurately characterize conformational ensembles evolution and binding mechanisms of convergent evolution for the SARS-CoV-2 spike Omicron variants BA.1, BA.2, BA.2.75, BA.3, BA.4/BA.5 and BQ.1.1. We employed and validated several different adaptations of the AlphaFold methodology for modeling of conformational ensembles including the introduced randomized full sequence scanning for manipulation of sequence variations to systematically explore conformational dynamics of Omicron spike protein complexes with the ACE2 receptor. Microsecond atomistic molecular dynamics (MD) simulations provide a detailed characterization of the conformational landscapes and thermodynamic stability of the Omicron variant complexes. By integrating the predictions of conformational ensembles from different AlphaFold adaptations and applying statistical confidence metrics we can expand characterization of the conformational ensembles and identify functional protein conformations that determine the equilibrium dynamics for the Omicron spike complexes with the ACE2. Conformational ensembles of the Omicron RBD–ACE2 complexes obtained using AlphaFold-based approaches for modeling protein states and MD simulations are employed for accurate comparative prediction of the binding energetics revealing an excellent agreement with the experimental data. In particular, the results demonstrated that AlphaFold-generated extended conformational ensembles can produce accurate binding energies for the Omicron RBD–ACE2 complexes. The results of this study suggested complementarities and potential synergies between AlphaFold predictions of protein conformational ensembles and MD simulations showing that integrating information from both methods can potentially yield a more adequate characterization of the conformational landscapes for the Omicron RBD–ACE2 complexes. This study provides insights in the interplay between conformational dynamics and binding, showing that evolution of Omicron variants through acquisition of convergent mutational sites may leverage conformational adaptability and dynamic couplings between key binding energy hotspots to optimize ACE2 binding affinity and enable immune evasion.
Introduction
The comprehensive array of structural and biochemical investigations focused on the spike (S) glycoprotein of the SARS-CoV-2 virus has provided crucial understanding of the mechanisms dictating virus transmission and evasion of the immune system. This glycoprotein serves as the gateway for viral entry into host cells and undergoes significant conformational alterations, transitioning between closed and open states. These transitions are orchestrated by the flexible amino (N)-terminal S1 subunit, which encompasses the N-terminal domain (NTD), the receptor-binding domain (RBD), and two structurally conserved subdomains—SD1 and SD2.1–9 The dynamic interplay among these structural domains is crucial for regulating conformational transitions within the S protein, facilitating shifts between the RBD-down closed state and the RBD-up open state, consequently enabling a variety of functional responses. Additionally, the functional motions of the NTD, RBD, SD1, and SD2 subdomains are synchronized as the S1 subunit undergoes global movements. These coordinated movements are facilitated through long-range communications with the structurally rigid S2 subunit.10–15 The synergistic interplay of functional motions within both S1 and S2 is paramount in mediating crucial interactions between the S protein and the host cell receptor ACE2. Moreover, it governs a wide array of interactions between the S protein and various classes of antibodies, thereby impacting the host immune responses triggered by the virus. The ability of the S protein to stochastically sample distinct structural states is fundamental for its effectiveness and specificity in recognizing host cell receptors and evading immune detection. The abundance of data and insights gained from biophysical studies has enriched our understanding of the S protein trimer, illuminating the complex interplay between thermodynamics and kinetics that govern spike mechanisms. These studies have revealed how mutations and long-range interactions between the dynamic S1 subunit and the more rigid S2 subunit orchestrate coordinated structural alterations within the S protein trimer and control population shifts between the open and closed RBD states, thus modulating interactions with various binding partners and shaping immune responses.16–18
The growing accessibility of cryo-electron microscopy (cryo-EM) and X-ray structures for the S protein variants of concern (VOCs) has significantly broadened our understanding of the evolutionary adaptability of the S protein and the diverse array of molecular mechanisms involved. These structural studies have revealed a multitude of functional states of the S protein and its interactions with antibodies, contributing to a complex and adaptable dynamic landscape with a diverse range of binding epitopes.19–28 The cryo-EM structures and biochemical analyses of the S trimers of different subvariants emerged during Omicron evolution including BA.1, BA.2, BA.3, and BA.4/BA.5, have revealed significant similarities and subtle differences in binding energetics with the host receptor. The binding affinities of Omicron BA.2 with ACE2 were observed to be stronger than those of BA.3 and BA.1, as indicated by the structures of the RBD–ACE2 complexes for the BA.1.1, BA.2, and BA.3 variants.29 Additionally, the Omicron BA.2 trimer exhibited a higher ACE2 binding affinity compared to both the S Wu-Hu-1 trimer and the S Omicron BA.1 trimer.30 The surface plasmon resonance (SPR) results showed that the Omicron BA.4/5 RBD had only a slightly higher binding affinity for ACE2 than the ancestral Wu-Hu-1 strain and the BA.1 variants.31 The biochemical analyses of BA.1, BA.2, BA.3, and BA.4/BA.5 variants demonstrated higher binding affinities for BA.2 compared to other Omicron variants.32,33 Biophysical studies of the Omicron BA.2.75 subvariant demonstrated a remarkable 9-fold enhancement in the binding affinity with ACE2 compared to its parental BA.2 variant, establishing it as having the strongest ACE2 binding among all S variants.34 Additionally, cryo-EM conformations of the BA.2.75 S trimer and structures of the open BA.2.75 S trimer complexes with ACE2 revealed that the BA.2.75 S-trimer exhibited the highest stability, followed by BA.1, BA.5, and BA.2 variants.35 The SPR experiments confirmed these findings, showing that the BA.2.75 subvariant exhibited a notably higher ACE2 binding affinity which is approximately 4–6 times greater than the other Omicron variants.34–36 Structural-functional studies of the Omicron BA.1, BA.2, BA.2.12.1, BA.4, and BA.5 subvariants provided further support to the mechanism in which the combined effect of the enhanced ACE2 receptor binding and stronger immune evasion may have contributed to the rapid spread of Omicron sublineages.37 Structural and virological analysis of the BA.4/BA.5 variant showed that dissociation constants and the binding affinity of the S RBDs of BA.2 and BA.4/5 are similar where F486V in the BA.4/5 S RBD may decrease the hydrophobic interaction with ACE2, while Q493 of the BA.4/5 S RBD by forming a hydrogen bond with the ACE2 residue H34 can compensate for the loss of binding.38,39
BQ.1.1 S protein harbors five convergent substitutions R346T, K444T, L452R, N460K, and F486V. Yeast surface display assays showed that BQ.1.1 can display the increased binding affinity to human ACE2 compared to BA.5 variant.39 Biophysical studies also revealed that BQ.1.1 exhibited stronger antibody evasion owing to convergent mutations R346T and N460K.40 Another study indicated that N460K-bearing subvariants BQ.1 and BQ.1.1 exhibit the strongest neutralization resistance compared with BA.4/5 variants suggesting that N460K mutation, and to a lesser extent, R346T, K444T are critical for the enhanced resistance of the BQ.1 and BQ.1.1 subvariants. Structural modeling of BQ.1.1 variant showed the important role of convergent mutational site F486V that can impact binding affinity with both ACE2 and antibodies, whereas the R346T and K444T mutations are likely responsible for evasion of specific class 3 antibody recognition.41 X-ray crystallographic analysis of the BQ.1.1 RBD-human ACE2 complex showed that R346T and K444T are not directly involved in the interaction with ACE2 but compared to the BA.5-human ACE2 complex structure may induce a different pattern of the RBD dynamics in the flexible regions.42 Functional studies confirmed that emergence of BQ.1.1 bearing R346T along with K444T and N460K substitutions are primarily associated with escape from monoclonal antibodies and vaccine-induced antibodies.43,44 Convergently evolved mutations cluster in specific locations of the S protein and concentrate in the NTD, RBD and the Furin cleavage site regions, forming co-localized patches45 and creating room for synergistic epistatic interactions, particularly evident through couplings with affinity-enhancing mutational hotspots Q498R/N501Y.46,47 Convergent evolution of mutations was also seen in the immune-evasive XBB lineages that harbor F496P and acquired additional mutations including R403K, V445S, L455F, F456L, and K478R allowing for escape from neutralizing antibodies generated through both repeated vaccination and natural infection.48–50 The latest structures and SPR-measured binding of S-RBD with human ACE2 for BA.4/BA.5, BQ.1, BQ.1.1, XBB and XBB.1.5 variants showed that BQ.1.1 affinity is comparable to that of BA.4/5 highlighting the key role of F486V as binding hotspot and also suggesting that R346T substitution can enhance ACE2 binding using long-range couplings with Q493 position.51 These studies echoed recent deep mutational scanning (DMS) experiments of BQ.1.1 and XBB.1.5 RBDs binding with ACE2 showing epistatic couplings between R493Q and mutations at positions Y453, L455, and F456.52 Convergent evolution patterns of S mutations showed that coordination of evolutionary paths at different sites may be due to epistatic rather than random selection of mutations.53,54
Computer simulation studies provided important atomistic insights into understanding the dynamics of the SARS-CoV-2 S protein and binding with diverse binding partners.55–60 Conformational dynamics and allosteric modulation of SARS-CoV-2 S in the absence or presence of ligands was studied using smFRET imaging assay, showing presence of long-range allosteric control of the RBD equilibrium, which in turn regulates the exposure of the binding site and antibody binding.61 Integrative computational modeling approaches revealed that the S protein can function as an allosteric regulatory machinery that undergoes large conformational changes and is tightly controlled by several allosteric hotspots of spike dynamics and binding.62–67 By combining atomistic simulations and a community-based network model of epistatic couplings we found that convergent Omicron mutations can display epistatic relationships with the major stability and binding affinity hotspots which may allow for the observed broad antibody resistance.65 Analysis of conformational dynamics and allosteric communications in the Omicron BA.1, BA.2, BA.3 and BA.4/BA.5 complexes with the ACE2 host receptor characterized regions of epistatic couplings that are centered at the binding affinity hotspots N501Y and Q498R enabling accumulation of multiple Omicron immune escape mutations at other sites.66 MD simulations and Markov state models systematically characterized conformational landscapes and identify specific dynamic signatures of Omicron variants and their complexes showing that convergent mutation sites could control evolution allosteric pockets through modulation of conformational plasticity in the flexible adaptable regions.67 Recent computational studies suggested that Omicron mutations have variant-specific effect on conformational dynamics changes in the S protein including allosterically induced plasticity at the remote regions leading to the formation and evolution of druggable cryptic pockets.68,69
Accurate characterization of the structural ensembles is paramount for robust predictions of the S protein activity and binding with the ACE2 and antibodies. The emergence of AlphaFold2 (AF2) technology has revolutionized protein structure modeling in structural biology, representing a significant leap forward.70,71 While AF2-based methods have made impressive strides in predicting static protein structures, they encounter hurdles in precisely capturing conformational dynamics, functional protein ensembles, conformational changes, and allosteric states.72 Recent studies underscore that while AF2 methods demonstrate proficiency in predicting individual protein structures, expanding this capability to accurately forecast conformational ensembles and map allosteric landscapes still poses a substantial challenge.73–77 The limitations of AF2 methods in predicting multiple protein conformations may be linked to the intrinsic training bias towards experimentally determined, thermodynamically stable structures, and MSAs containing evolutionary information used to infer the ground protein states. Efforts to optimize the AF2 methodology for predicting alternative conformational states were primarily focused on manipulating the MSA information to encode coevolutionary signals, aiming to capture not only the most thermodynamically probable protein state but also other functional conformational states of a protein.73,74 One approach involves subsampling of MSAs to reduce depth, resulting in shallow MSAs.73 This strategy aims to enhance diversity and generate a broader range of AF2 output models, thereby improving the ability to capture experimentally validated alternative conformational states of proteins. Another AF2-based approach, SPEACH_AF (Sampling Protein Ensembles and Conformational Heterogeneity with AlphaFold2) can model protein conformational ensembles by replacing specific residues within the MSA to manipulate the distance matrices leading to alternative conformations.74 Recent studies have investigated the combination of shallow MSA with state-annotated templates, which integrate functional or structural properties of GPCRs and protein kinases.78 AF-Cluster employs a simple method of subsampling MSAs followed by clustering of related or functionally similar sequences which enables generation of alternative protein states.79 This method showed promise in predicting previously unknown fold-switched protein states that were independently validated by NMR analysis.79
In this study, we employed an AI-enabled integrative simulation approach for probing conformational ensembles and binding energetics of the Omicron RBD–ACE2 complexes. Several different adaptations of the AF2 methodology along with MD simulations were used for a comparative characterization of structures, conformational ensembles and subsequent computations of binding affinities of the Omicron RBD–ACE2 complexes including BA.1, BA.2, BA.2.75, BA.3, BA.4/BA.5 and BQ.1.1 variants (Fig. 1). Structural organization of the RBD–ACE2 complexes for all Omicron variants is remarkably similar and the composition of the binding epitopes across Omicron variants is preserved (Fig. 1). Using a combination of the AF2-based adaptations we can efficiently expand characterization of the conformational ensembles for the RBD–ACE2 complexes capturing conformational details of the RBD fold and variant-specific functional adjustments of the RBM loop for binding. We demonstrate that AF2-based statistical assessment of generated models can be used to accurately predict the mutation-induced dynamic changes and determine functional protein ensembles.
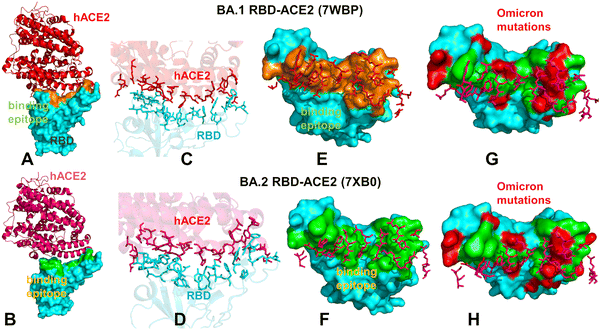 |
| Fig. 1 Structural organization and binding epitopes of the SARS-CoV-2-RBD Omicron complexes with human ACE enzyme. The cryo-EM structure of the Omicron RBD BA.1-ACE2 complex (pdb id 7WBP) (A). The RBD is in cyan surface and ACE2 is in red ribbons. (B) The cryo-EM structure of the Omicron RBD BA.2-ACE2 complex (pdb id 7XB0). The RBD is in cyan surface and the ACE2 is in pink ribbons. (C) The binding interface for the BA.1 RBD–ACE2 complex. The RBD binding residues are in cyan sticks, ACE2 binding residues are in red sticks. (D) The biding interface for the BA.2 RBD–ACE2 complex. The RBD binding residues are in cyan sticks, ACE2 binding residues are in pink sticks. (E) The binding epitope for the BA.1 RBD–ACE2 complex. The RBD-BA.1 binding epitope is in orange surface. The ACE2 binding residues are shown in pink sticks. (F) The binding epitope for the BA.2 RBD–ACE2 complex. The RBD-BA.1 binding epitope is in green surface. The ACE2 binding residues are shown in pink sticks. (G) The binding epitope residues (in green surface) and BA.1 RBD Omicron mutations (in red surface). The RBD is shown in cyan surface. (H) The map of the binding epitope residues (in green surface) and BA.2 RBD Omicron mutations (in red surface). | |
Microsecond atomistic simulations are also conducted to provide a detailed characterization of the conformational landscapes and thermodynamic stability of the Omicron variant complexes. We find that AF2 predictions of structural ensembles are consistent with the conformational flexibility patterns revealed in atomistic MD simulations. We leverage AF2-based structural ensembles and MD-generated equilibrium ensembles for a comparative prediction of the binding energetics and affinities for the Omicron RBD–ACE2 complexes, resulting in remarkably similar trends and strong correlation with the experimental data. This integrative analysis shows that combining MD simulations together with AF2-based prediction of conformational ensembles could provide more comprehensive view of the conformational landscapes and binding mechanisms for Omicron variants. We also examine how evolution of Omicron variants through acquisition of convergent mutational sites may leverage conformational adaptability and dynamic couplings between these hotspots to optimize balance between immune evasion and ACE2 affinity.
Materials and methods
Protein structure modeling using AF2 shallow subsampling adaptations
To understand the advantages and limitations of AF2 methods for predicting structural ensembles, we employ (a) MSA subsampling with shallow MSA depth and (b) random alanine scanning algorithm, which iterates through each amino acid in the native sequence to simulate random alanine substitution mutations. The structural prediction of the Omicron RBD–ACE2 complexes was initially conducted using the AF2 framework within the ColabFold implementation.80 This process involved utilizing a range of MSA depths and MSA subsampling techniques. Specifically, we utilized the max_msa field to set two AF2 parameters in the format of max_seqs:extra_seqs. These parameters dictate the number of sequences subsampled from the MSA, with max_seqs determining the number of sequences passed to the row/column attention track, and extra_seqs determining the number of sequences additionally processed by the main evoformer stack. Lower values in these parameters promote more diverse predictions but may lead to an increased number of misfolded models. The default MSAs are subsampled randomly to obtain shallow MSAs. We set the max_msa parameter to 16
:
32. This parameter is in the format of max_seqs:extra_seqs which decides the number of sequences subsampled from the MSA. Max_seq determines the number of sequences passed to the row/column attention matrix at the front end of the AF2 architecture, and extra_seqs sets the number of extra sequences processed by the Evoformer stack after the attention mechanism. We additionally manipulated the num_recycles parameters to produce more diverse outputs. We use num_recycles: 12. AF2 makes predictions using 5 models pretrained with different parameters, and consequently with different weights. To generate more data, we set the number of recycles to 12, which produces 14 structures for each model starting from recycle 0 to recycle 12 and generating a final refined structure. Recycling is an iterative refinement process, with each recycled structure getting more precise. Each of the AF2 models generates 14 structures, amounting to 70 structures in total. The MSAs were generated using the MMSeqs2 library81 using the S sequence as input. We then set the num_seed parameter to 1. This parameter quantifies the number of random seeds to iterate through, ranging from random_seed to random_seed + num_seed. Increasing the num_seeds samples predictions from the uncertainty of the model. Additionally, the dropout parameter was enabled, activating dropout layers in the model during predictions, which further increases variability within the predictions. We also predicted the structure using AF2 with the default and ‘auto’ parameters serving as a baseline structure for prediction analysis.
We also utilized another approach for prediction of multiple conformational states that combined sequence clustering with AF2 and clustered the MSA by sequence distance using DBSCAN method (density-based spatial clustering of applications with noise).79 The AF2-cluster approach then runs AF2 predictions using these clusters as input. We employed this approach where MSAs that contained greater than 25% gaps were removed. The rest were clustered using the DBSCAN method, clustering the MSAs by density, and labeling those that were not included as noise and excluding them. This technique generated 13 MSA clusters, which were then used as input for AF2 models using 3 recycles for refinement. As a result, 13 additional structures were predicted using this method. Together, shallow MSA depth and AF2-cluster adaptations generated a total of 83 conformations.
We developed another AF2 adaptation which involves randomized alanine sequence scanning and MSA shallow subsampling. The initial input for the full sequence randomized alanine scanning is the original full native sequence.82 This technique utilizes an algorithm that iterates through each amino acid in the native sequence and randomly substitutes 5–15% of the residues with alanine, to simulate random alanine substitution mutations. The algorithm substitutes residue ai with alanine at each position i with a probability pi randomly generated between 0.05 and 0.15 for each sequence position. We ran this algorithm nine times on the full native sequence, resulting in nine distinct sequences, each with different frequency and position of alanine mutations. MSAs were constructed for each of these mutated sequences using the alanine-scanned full-length sequences as input for the MMSeqs2 program.81 The AF2 shallow MSA methodology is then employed on these MSAs to predict protein structures as described previously. A total of 70 predicted structures were generated from 12 recycles per model.
Statistical and structural assessment of AF2-generated models
AF2 models underwent ranking based on local distance difference test (pLDDT) scores, offering a per-residue estimation of prediction confidence ranging from 0 to 100. These scores are calculated by determining the fraction of predicted Cα distances that align with their anticipated intervals. The scores represent the model's predictions according to the lDDT-Cα metric, which assesses atomic displacements within the predicted model without requiring local superposition.70,71 The accuracy of the predicted models was evaluated against the experimental structure using the structural alignment tool TM-align.83 This algorithm, specifically developed for sequence-independent protein structure comparison, was utilized to assess and compare the accuracy of protein structure predictions. TM-align iteratively refines the alignment of residues through dynamic programming, aligns and superimposes the structures based on this alignment, and computes the TM-score as a quantitative measure of the overall accuracy of the predicted models. An optimal superposition of the two structures is then built and TM-score is reported as the measure of overall accuracy of prediction for the models. TM-score ranges from 0 to 1, where a value of 1 indicates a perfect match between the predicted model and the reference structure. When TM-score > 0.5 implies that the structures share the same fold. TM-score > 0.5 is often used as a threshold to determine if the predicted model has a fold similar to the reference structure. Several other structural alignment metrics were used including the root mean square deviation (RMSD) superposition of backbone atoms (C, Cα, O, and N) calculated using ProFit (https://www.bioinf.org.uk/software/profit/). This systematic approach provides a quantitative means to assess and rank the structural accuracy of predicted models relative to experimentally determined reference structures.
All-atom molecular dynamics simulations
The structures of the Omicron RBD–ACE2 complexes for BA.1 (pdb id 7WBP), BA.2 (pdb id 7XB0), BA.2.75 (pdb id 8ASY), BA.3 (pdb id 7XB1), BA.4/BA.5(pdb id 8AQS) and BQ.1.1 (pdb id 8IF2) are obtained from the Protein Data Bank. In addition, the best AF2 models obtained for each of the Omicron variants were selected as starting structure for MD simulations of RBD–ACE2 complexes. For simulated structures, hydrogen atoms and missing residues were initially added and assigned according to the WHATIF program web interface.84 The missing regions are reconstructed and optimized using template-based loop prediction approach ArchPRED.85 The side chain rotamers were refined and optimized by SCWRL4 tool.86 The protonation states for all the titratable residues of the ACE2 and RBD proteins were predicted at pH 7.0 using Propka 3.1 software and web server.87 The protein structures were then optimized using atomic-level energy minimization with composite physics and knowledge-based force fields implemented in the 3Drefine method.88,89 We considered glycans that were resolved in the structures. NAMD 2.13-multicore-CUDA package90 with CHARMM36 force field91 was employed to perform 1 μs all-atom MD simulations for the Omicron RBD–ACE2 complexes. The structures of the SARS-CoV-2 S-RBD complexes were prepared in Visual Molecular Dynamics (VMD 1.9.3)92 and with the CHARMM-GUI web server93,94 using the Solutions Builder tool. Hydrogen atoms were modeled onto the structures prior to solvation with TIP3P water molecules95 in a periodic box that extended 10 Å beyond any protein atom in the system. To neutralize the biological system before the simulation, Na+ and Cl− ions were added in physiological concentrations to achieve charge neutrality, and a salt concentration of 150 mM of NaCl was used to mimic a physiological concentration. All Na+ and Cl− ions were placed at least 8 Å away from any protein atoms and from each other. MD simulations are typically performed in an aqueous environment in which the number of ions remains fixed for the duration of the simulation, with a minimally neutralizing ion environment or salt pairs to match the macroscopic salt concentration.96
All systems underwent a two-stage minimization protocol. In the first stage, minimization was conducted for 100
000 steps with all hydrogen-containing bonds constrained and the protein atoms fixed. Subsequently, in the second stage, minimization was conducted for 50
000 steps with all protein backbone atoms fixed, followed by an additional 10
000 steps with no fixed atoms. Following minimization, the protein systems underwent equilibration steps by gradually increasing the system temperature in increments of 20 K, ranging from 10 K to 310 K. At each temperature step, a 1 ns equilibration was performed while maintaining a restraint of 10 kcal mol−1 Å−2 on the protein Cα atoms. After removing restraints on the protein atoms, the system was equilibrated for an additional 10 ns. Long-range, non-bonded van der Waals interactions were computed using an atom-based cutoff of 12 Å, with the switching function beginning at 10 Å and reaching zero at 14 Å. The SHAKE method was used to constrain all the bonds associated with hydrogen atoms. The simulations were run using a leap-frog integrator with a 2 fs integration time step. The ShakeH algorithm in NAMD was applied for the water molecule constraints. The long-range electrostatic interactions were calculated using the particle mesh Ewald method97 with a cut-off of 1.0 nm and a fourth-order (cubic) interpolation. The simulations were performed under an NPT ensemble with a Langevin thermostat and a Nosé–Hoover Langevin piston at 310 K and 1 atm. The damping coefficient (gamma) of the Langevin thermostat was 1 ps−1. In NAMD, the Nosé–Hoover Langevin piston method is a combination of the Nosé–Hoover constant pressure method98 and piston fluctuation control implemented using Langevin dynamics.99,100 An NPT production simulation was run on equilibrated structures for 1 μs keeping the temperature at 310 K and a constant pressure (1 atm).
Binding free energy computations
We compute the ensemble-averaged binding free energy changes using both conformational ensembles obtained from AF2 predictions and equilibrium samples from simulation trajectories. The binding free energy changes were computed by averaging the results over 1000 equilibrium samples from MD simulations and 100 AF2-predicted conformations from the obtained conformational clusters. The binding free energies were computed for the Omicron RBD–ACE2 complexes using the molecular mechanics/generalized Born surface area (MM-GBSA) approach.101,102 We also evaluated the decomposition energy to assess the energy contribution of each amino acid during the binding of RBD to ACE2.103,104 The binding free energy for the each RBD–ACE2 complex was obtained using: | ΔGbind = GRBD–ACE2 − GRBD − GACE2 | (1) |
| ΔGbind,MMGBSA = ΔEMM + ΔGsol − TΔS | (2) |
where ΔEMM is total gas phase energy (sum of ΔEinternal, ΔEelectrostatic, and ΔEvdW); ΔGsol is sum of polar (ΔGGB) and non-polar (ΔGSA) contributions to solvation.
Here, GRBD–ACE2 represent the average over the snapshots of a single trajectory of the MD RBD–ACE2complex (or 100 AF2-predicted conformations), GRBD and GACE2 corresponds to the free energy of RBD and ACE2 protein, respectively. MM-GBSA is employed to predict the binding free energy and decompose the free energy contributions to the binding free energy.105 The entropy contribution was not included in the calculations due to the difficulty of accurately calculating entropy for a large protein–protein complex but equally importantly because the entropic differences between variants for estimates of binding affinities are exceedingly small owing to small mutational changes and preservation of the conformational dynamics.
Results
Evolutionary and phylogenetic analysis of the SARS-CoV-2 Omicron lineages
We began with a brief analysis of evolutionary differences and divergence among the Omicron variants which are illustrated by the phylogenetic analysis (Fig. 2, ESI,† Fig. S1) using the corresponding clades nomenclature from Nextstrain an open-source project for real time tracking of evolving pathogen populations (https://nextstrain.org/).106 Nextstrain provides dynamic and interactive visualizations of the phylogenetic tree of SARS-CoV-2, allowing users to explore the evolutionary relationships between different lineages and variants. Omicron viruses can be divided into two major groups, referred to as PANGO lineages BA.1 and BA.2 or clades 21K and 21L (Fig. 2A, B and ESI,† Fig. S1).
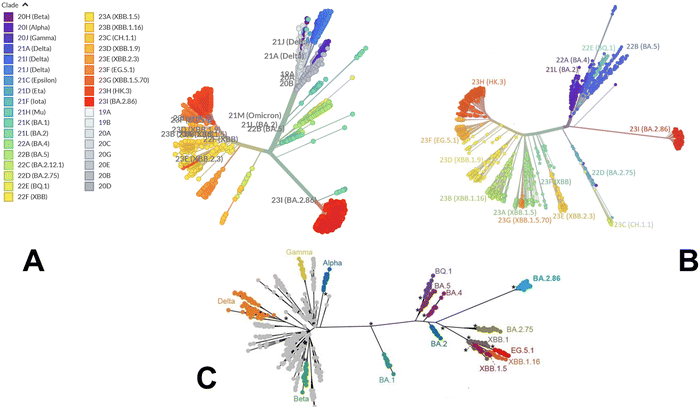 |
| Fig. 2 An overview of the phylogenetic analysis and divergence of Omicron variants. The graphs are generated using Nextstrain, an open-source project for real time tracking of evolving pathogen populations (https://nextstrain.org/). (A) The phylogenetic tree of Omicron variants BA.2 (clade 21L), BA.2.75 (clade 22), BA.4 (clade 22A), BA.5 (clade 22B), BA.2.75 (clade 22D), BQ.1 (clade 22E) and XBB lineages including XBB (22F clade) and XBB.1.5 (23A clade). (B) A radial-based phylogenetic tree of Omicron variants highlights evolutionary proximity of BA.2, BA.4, BA.5 and BQ.1.1 variants and more significant evolutionary divergence of BA.2.86 and XBB lineages. (C) A phylogenetic tree of Omicron variants relative to alpha, beta, gamma and delta variants. The evolutionary distance of BA.1, BA.2, BA.4/BA.5 and BQ.1.1 from alpha, beta, gamma and delta variants is highlighted. | |
Omicron clades 21K and 21L differ at ∼40 amino acid sites, which is substantial in the context of SARS-CoV-2 evolution while Alpha, Beta and Gamma are as divergent from each other in terms of amino acid changes across the genome as Omicron 21K and 21L are from each other (Fig. 2C). BA.3 is not officially recognized by Nextstrain since it is very rare and is labelled as 21M Omicron. In BA.3 21 mutations are shared with all Omicron sub-lineages, Of 21 common mutations, BA.3 shares ten mutations (A67V, H69del, V70del, T95I, V143del, Y144del, Y145del, N211I, L212del, and G446S) from BA.1 and two (S371F and D405N) mutations from BA.2. In other words, of the 33 mutations in the BA.3 lineage spike protein, 31 mutations are common to BA.1. There are no specific mutations for the BA.3 lineage in spike protein. Instead, it is a combination of mutations in BA.1 and BA.2 spike proteins. BA.4 and BA.5 lineages (clades 22A and 22B respectively) have emerged with amino-acid substitutions L452R, F486V, and R493Q reversed in S-protein RBD compared to BA.2 variant (Table 1 and Fig. 2). Phylogenomic reconstruction indicates that the genomes of BQ.1 (clade 22E) are clustered within the not-monophyletic GSAID Clade 21L, with a close relationship with BA.5 Omicron subvariant (Fig. 2B and C). BQ.1 which is a direct descendant of BA.5 has additional spike mutations in some key antigenic sites (K444T and N460K). Its first descendant, BQ.1.1 carries a further additional mutation R346T. In this work, we focus on the analysis of a particular branch of the Omicron tree that includes BA.2, BA.3, BA.4, BA.5 and BQ.1.1 variants (Fig. 2 and Table 1).
Table 1 Mutational landscape of the Omicron variants
Omicron variant |
Mutational landscape |
BA.1 |
T95I, G339D, S371L, S373P, S375F, K417N, N440K, G446S, S477N, T478K, E484A, Q493R, G496S, Q498R, N501Y, Y505H, T547K, D614G, H655Y, N679K, P681H, N764K, D796Y, N856K, Q954H, N969K, L981F |
BA.2 |
T19I, G142D, V213G, G339D, S371F, S373P, S375F, T376A, D405N, R408S, K417N, N440K, S477N, T478K, E484A, Q493R, Q498R, N501Y, Y505H, D614G, H655Y, N679K, P681H, N764K, D796Y, Q954H, N969K |
BA.2.75 |
T19I, G142D, K147E, W152R, F157L, I210V, V213G, G257S, G339H, S371F, S373P, S375F, T376A, D405N, R408S, K417N, N440K, G446N, N460K, S477N, T478K, E484A, Q498R, N501Y, Y505H, D614G, H655Y, N679K, P681H, N764K, D796Y, Q954H, N969K |
BA.3 |
G142D, G339D, S371F,S373P, S375F, D405N, K417N, N440K, G446, S477N, T478K, E484A, Q493R, Q498R, N501Y, Y505H, D614G, H655Y, N679K, P681H, N764K, D796Y, Q954H, N969K |
BA.4 |
T19I, G142D, V213G, G339D, S371F, S373P, S375F, T376A, D405N, R408S, K417N, N440K, L452R, S477N, T478K, E484A, F486V, R493Q reversal, Q498R, N501Y, Y505H, D614G, H655Y, N679K, P681H, N764K, D796Y, Q954H, N969K |
BA.5 |
T19I, LPPA24-27S, Del 69-70, G142D, V213G, G339D, S371F, S373P, S375F, T376A, D405N, R408S, K417N, N440K, L452R, S477N, T478K, E484A, F486V, R493Q reversal, Q498R, N501Y, Y505H, D614G, H655Y, N679K, P681H, N764K, D796Y, Q954H, N969K |
BQ.1.1 |
T19I, LPPA24-27S, H69del, V70del, V213G, G142D, G339D, R346T, S371F, S373P, S375F, T376A, D405N, R408S, K417N, N440K, K444T, L452R, N460K, S477N, T478K, E484A, F486V, R493Q reversal, Q498R, N501Y, Y505H, D614G, H655Y, N679K, P681H, N764K, D796Y, Q954H, N969K |
AF2 atomistic modeling and structural prediction of the Omicron RBD–ACE2 complexes
Despite considerable mutational differences between newly emerged Omicron variants, structural analysis of the RBD complexes with ACE2 for these variants revealed similar RBD conformations and the same binding mode of interactions. We embarked on a systematic comparative analysis of conformational dynamics and energetics of the Omicron RBD–ACE2 complexes using a panel of evolutionary proximal BA.1, BA.2, BA.2.75, BA.3, BA.4/BA.5 and BQ.1.1 variants. To facilitate this comparative analysis, we explored both AF2-based adaptations for modeling of structural ensembles and MD simulations to characterize conformational landscapes and functional conformational states of the Omicron spike proteins. We began with baseline AF2 structural predictions of the Omicron RBD–ACE2 complexes using AF2 within the ColabFold80 and best five models for each system (Fig. 3). The confidence of the AF2 predictions for RBD–ACE2 complexes is analyzed using residue-based pLDDT scores (Fig. 3). The pLDDT values between 70 and 90 indicate a high accuracy, where the prediction of the main chain of the protein is reliable. pLDDT values above 90 indicate extremely high accuracy, equivalent to structures determined by experiments. pLDDT values between 50 to 70 indicate a lower accuracy, but it is likely that the predictions of individual secondary structures are correct.
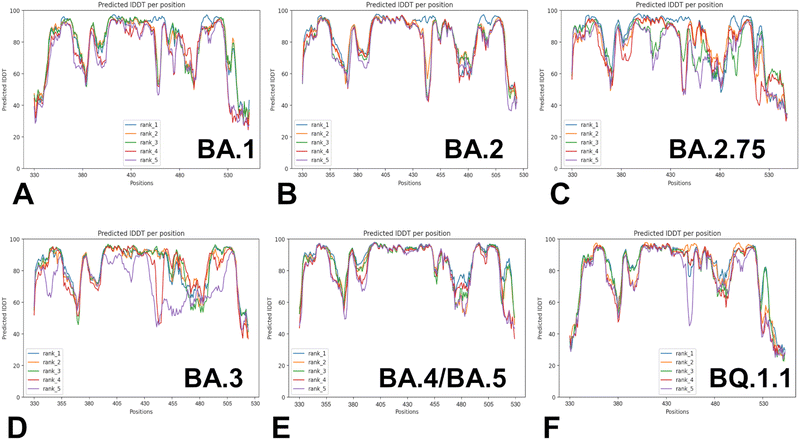 |
| Fig. 3 The AF2 analysis of predictions for the Omicron RBD–ACE2 The pLDDT per residue for the top five models obtained from AF2 predictions of the BA.1 RBD–ACE2 complex (A), BA.2 RBD–ACE2 complex (B), BA.2.75 RBD–ACE2 complex (C), BA.3 RBD–ACE2 complex (D), BA.4/BA.5 RBD–ACE2 complex (E) and BQ.1.1 RBD–ACE2 complex (F). | |
The results showed a significant convergence among five independent AF2 runs for each complex. However, this pattern is particularly evident for BA.2 (Fig. 3B), BA.4/B.5 (Fig. 3E) and BQ.1.1 complexes (Fig. 3F). We noticed also appreciable divergence between the pLDDT values of the top five models for these regions. These preliminary results suggested that AF2-generated top structural models can accurately reproduce the RBD fold and stability of the RBD core regions. Moreover, we argue that the lower pLDDT values obtained for the RBD loops and particularly RBM resides reflected the intrinsically flexible and adaptable nature of these regions rather than a reduced prediction quality of the AF2 pipeline.
Structural alignment of the best five AF2 models with the experimental structures for the Omicron RBD–ACE2 complexes yielded the RMSD values of ∼0.5–0.8 Å (Fig. 4), suggesting high prediction accuracy for all systems. In addition, we highlighted the pLDDT confidence values and RMSDs for the best model, showing that pLDDT scores were above 80.0–82.0 and the RMSD values were below 0.5 Å (Fig. 4). A comparison of the top predicted models with the experimental structures demonstrated the high accuracy of predictions and highlighted only small deviations in the intrinsically flexible RBM loop (residues 475–487) and in peripheral flexible region (residues 520–527) (Fig. 4). Importantly, the AF2 results for all Omicron RBD–ACE2 complexes showed that selection of best models based on the highest pLDDT scores can yield functionally relevant mobility in the RBD loop 444–452 and RBM tip 475–487 that harbor important mutational sites across all Omicron variants. Although there are some minor differences in the amplitude of the RBM tip fluctuations among variants, the predicted spectrum of RBM conformations displayed an ordered “hook-like” folded RBM tip which is a preferable state in the experimental structures. Previous structural and computational studies suggested that a hook-like folded RBD tip (the “Hook” state) may interconvert during equilibrium with less dominant and highly dynamic “disordered” state in which the RBD tip cycles between a variety of conformations.67 Importantly, the best AF2 models preserved the hook-like folded RBM conformations only revealing modest lateral displacements (Fig. 4).
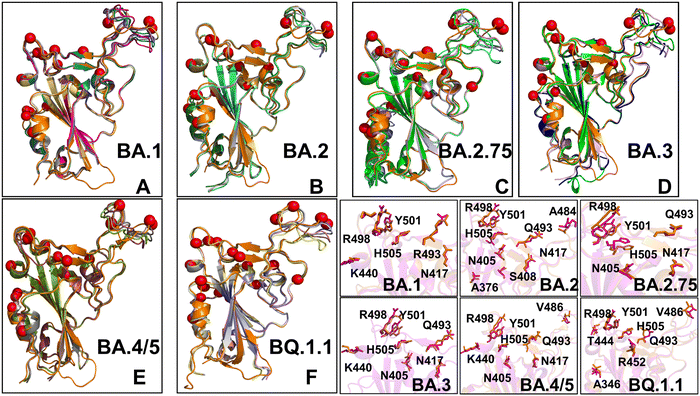 |
| Fig. 4 Structural alignment of the AF2-predicted top five models with the experimental structure for the Omicron RBD–ACE2 complexes. (A) Structural alignment of the AF2-predicted top five BA.1 conformations with highest pLDDT values and the experimental structure of the BA.1 RBD–ACE2 complex (in orange ribbons), pdb id 7WBP. (B) Structural alignment of the AF2-predicted top five BA.2 conformations and the experimental structure of the BA.2 RBD–ACE2 complex (in orange ribbons), pdb id 7XB0. (C) Structural alignment of the AF2-predicted top five BA.2.75 conformations and the experimental structure of the BA.2.75 RBD–ACE2 complex (in orange ribbons), pdb id 8ASY. (D) Structural alignment of the AF2-predicted top five BA.3 conformations and the experimental structure of the BA.3 RBD–ACE2 complex (in orange ribbons), pdb id 7XB1. (E) Structural alignment of the AF2-predicted top five BA.4/BA.5 conformations and the experimental structure of the BA.4/5 RBD–ACE2 complex (in orange ribbons), pdb id 8AQS. (F) Structural alignment of the AF2-predicted top five BQ.1.1 conformations with high pLDDT values and the experimental structure of the BQ.1.1 RBD–ACE2 complex (in orange ribbons), pdb id 8IF2. The RBD conformations are shown in ribbons and the positions of the Omicron RBD mutational sites for each of the respective variants are shown in red spheres. (bottom right panel) The closeups of the predicted side-chains (shown in magenta sticks) and the experimental conformations (in orange sticks) for the Omicron mutational sites in BA.1, BA.2, BA.2.75, BA.3, BA.4/BA.5 and BQ.1.1 variants. | |
It is worth noting that dynamic changes in the RBM tip may affect conformations and interacting positions for key mutational sites for making favorable interactions with ACE2 for mutational sites E484A and F486 (or F486V in BA.4/BA.5 and BQ.1.1). In the structural context of the full length trimer, conformational mobility of the RBM tip may promote the increased population of the RBD-up states and modulate binding interactions with ACE2 and antibodies. Similar functional variations of the RBM residues were also observed in MD simulations showing that the RBM loop has an inherent conformational flexibility that is not observed in the static structures and that ACE2 and antibody binding to this region may elicit specific distribution of conformations as compared to the unbound RBD form.107 The predicted plasticity of the RBM tip in the top AF2 models is functionally relevant and consistent with the hydrogen/deuterium-exchange mass spectrometry (HDX-MS) studies of spike flexibility108–110 particularly showing bimodal isotopic distributions between two distinct interconverting populations of ACE2-bound structurally stable and more flexible RBM conformations.109
AF2 adaptations with shallow MSA and randomized sequence scanning enable predictions of protein conformational ensembles for the Omicron RBD–ACE2 complexes
We used AF2 adaptation with varied MSA depth to predict structural ensembles of the RBD–ACE2 complexes. In this analysis, it is assumed that the experimental structure would be the best (or among best) ranked models within the ensemble based on the pLDDT metric assessment. The density distribution of the pLDDT scores for the ensemble of the AF2 models revealed pronounced cluster peaks at pLDDT ∼ 80–90 for BA.2 (Fig. 5B) and BA.4/BA.5 (Fig. 5E) corresponding to the native state. Interestingly, for BA.1 (Fig. 5A), BA.2.75 (Fig. 5C), BA.3 (Fig. 5D) and BQ.1.1 variants (Fig. 5F). The distributions are somewhat broader featuring also several minor peaks at pLDDT ∼65–75 indicative of moderate conformational variability in the flexible RBD regions. Notably, for all distributions, there is a sharp decay of the density at the lower pLDDT values ∼50–70 reflecting minor populations of highly flexible RBD conformations (Fig. 5). AF2 predictions with pLDDT values ∼70–90 are typically associated with high confidence, while the regions with pLDDT values ∼50–70 have lower confidence. Hence, the vast majority of the AF2-predicted conformations using shallow MSA subsampling are characterized by appreciable level of confidence, reflecting strong bias towards the native structures (Fig. 5).
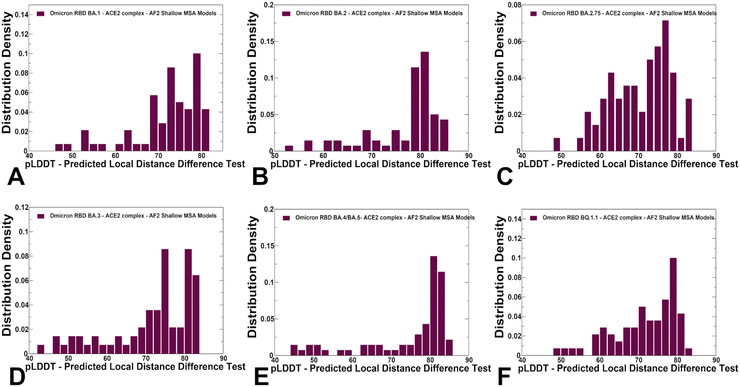 |
| Fig. 5 The distributions of the pLDDT metric for the RBD–ACE2 conformational ensembles obtained from AF2-MSA shallow depth predictions. The density distribution of the pLDDT values for structural ensembles are shown for BA.1 (A) BA.2 (B), BA.2.75 (C), BA.3 (D), BA.4/BA.5 (E) and BQ.1.1 (F). The density distributions are depicted as maroon-colored filled bars. | |
Using structural similarity metrics TM-score and RMSD we evaluated the prediction accuracy of AF2-MSA depth models. The density distributions of TM-scores showed a considerable similarity between the predicted conformations and the experimental structures with the major peaks corresponding to TM-score ∼0.9 (ESI,† Fig. S2). For BA.1, BA.2 and BA.4/BA.5 variants the TM score distribution revealed clusters at TM score ∼0.8–0.95 thereby showing a strong preference for the native structure. A revealing picture emerged from the density distribution of the RMSDs for the predicted conformations with respect to the experimental structures (Fig. 6), showing dominant cluster peaks at RMSD∼ 0.6–0.8 Å and markedly reduced density at RMSDs ∼1.0–1.2 Å. Hence, for all variants, the AF2 conformations sampled the native RBD conformations in the close proximity of the experimental structures.
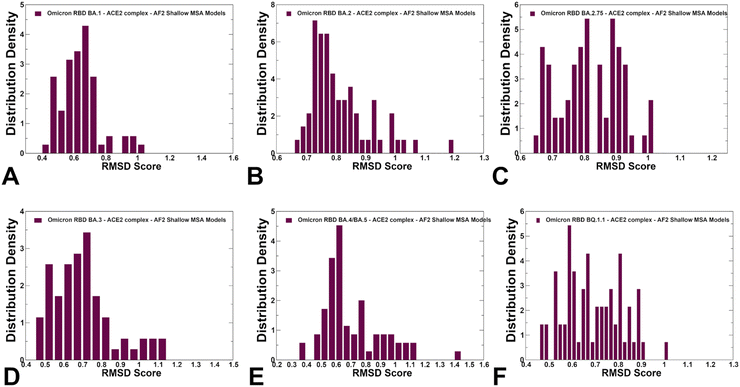 |
| Fig. 6 The densities of the RMSD scores for predicted ensembles with respect to the experimental structures are shown for BA.1 (A) BA.2 (B), BA.2.75 (C), BA.3 (D), BA.4/BA.5 (E) and BQ.1.1 (F). The density distributions are depicted as maroon-colored filled bars. | |
We also examined the relationship between pLDDT estimates and RMSD values of the predicted conformations from the experimental structures (Fig. 7). A strong correlation was obtained for all systems showing that the higher pLDDT value of the predicted conformation the lower the RMSD value for this model from the experimental structure (Fig. 7). The Pearson correlation coefficients were significant for all variants. Another interesting feature of the scatter profiles is a clear evidence of highly populated clusters of native conformations with pLDDT values ∼75–85 and RMSD < 0.7 Å (Fig. 7). Hence, AF2-generated ensemble is strongly biased towards the experimental structures with only limited variability in the flexible RBD loops.
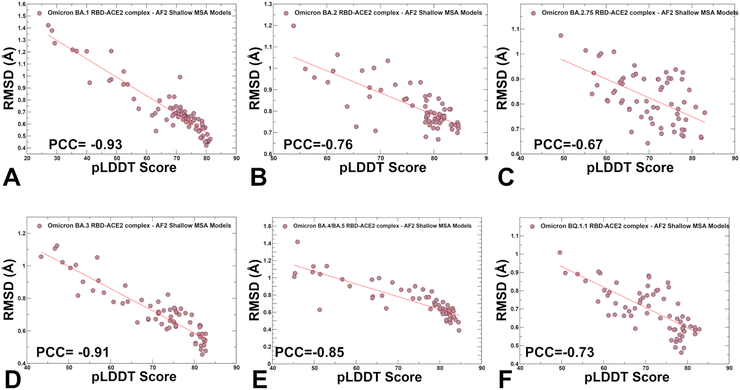 |
| Fig. 7 The scatter plots between the pLDDT scores and RMSD scores of the predicted conformational ensembles with respect to the experimental structures. The scatter plots are shown for BA.1 (A) BA.2 (B), BA.2.75 (C), BA.3 (D), BA.4/BA.5 (E) and BQ.1.1 (F). The density distributions are depicted as brown-colored filled circles. The Pearson correlation coefficients (PCC) values between the pLDDT and RMSD values are shown in respective panels. | |
Principal component analysis (PCA) of the AF2-predicted ensembles provided a more detailed analysis of the S-RBD conformational landscapes reconstructed by shallow MSA subsampling approach (ESI,† Fig. S3). The generated models from all methods were processed to exclude models with a pLDDT < 60. MDAnalysis library for PCA of the structural ensembles created the trajectories of all the generated structures and then PCA algorithm was employed to project the respective conformational ensembles on two principal components (ESI,† Fig. S3). Consistent with our analysis, the PCA of the AF2-generated ensemble revealed concentrated localized clusters of the RBD conformations and fairly limited conformational heterogeneity which is particularly evident for BA.1, BA.3 and BA.4/BA.5 variants. The PCA graphs also highlighted a greater sampling of the conformational space for BA.2, BA.2.75 and BQ.1.1 variants.
We compared the performance of different AF2 methods for predicting conformational ensembles of the Omicron RBD–ACE2 complexes. One of the main objectives of this analysis was to compare the ability of AF2 adaptations to reproduce key functional features of the RBD heterogeneity that is primarily associated with modulation of the flexible RBM loops involved in the interactions with the ACE2 receptor. In particular, we employed the AF2-cluster approach that was designed to capture conformational variability by clustering MSA sequences and performing AF2 predictions for each cluster.79 Our results indicated the increased diversity of the predicted conformations and we examined whether the generated ensemble of the RBD conformations can reproduce differences in the dynamics of the RBD–ACE2 complexes for different Omicron variants ESI,† Fig. S4. In particular, we analyzed the pLDDT, TM-score, and RMSDs of the generated conformational clusters for BA.2 and BQ.1.1 variants that are known to exhibit higher degree of conformational plasticity.39,40 The results revealed a broad range of pLDDT values indicating the reduced confidence level due to elevated flexibility of the RBD conformations. Similarly, while most AF2-cluster conformations yielded reasonable TM-score values >0.65–0.7 for the native-like RBD states, several predicted conformations with TM-scores ∼−0.4 to 0.6 highlighted the elevated variability across all RBD regions (ESI,† Fig. S4). The RMSD values for the cluster conformations reflected the increased variability consistent with the pLDDT analysis (ESI,† Fig. S4). Overall, we found that in addition to functionally relevant RBD cluster conformations, this approach tends to produce RBD conformations with elevated flexibility and even generate partially disordered states.
To characterize functionally relevant conformational states and structural ensembles using the AF2 framework, we proposed randomized alanine scanning adaptation of the AF2 methodology in which the algorithm operates on sequences and iterates through each amino acid in the native sequence to randomly substitute 5–15% of the residues with alanine, thus emulating random alanine mutagenesis.82 Using a combination of randomized alanine sequence scanning and shallow MSA subsampling we predicted structures and conformational ensembles of the Omicron RBD–ACE2 complexes. A comparison of the pLDDT profiles highlighted a shift and broadening of the distribution when using alanine scanning AF2 adaptation, yet still yielding high confidence pLDDT values ∼65–85 for the majority of generated conformations (Fig. 8). The shallow peaks of the pLDDT distribution obtained with this approach suggested that the increased structural heterogeneity of the RBD conformations can reflect the functionally relevant fluctuations of the reliably predicted native RBD fold.
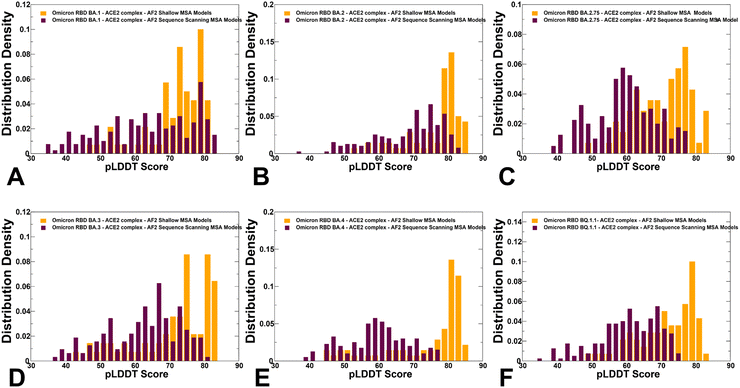 |
| Fig. 8 The distributions of the pLDDT metric for the RBD–ACE2 conformational ensembles obtained from AF2-MSA shallow depth predictions and AF2 with randomized sequence scanning. The density distribution of the pLDDT values for structural ensembles are shown for BA.1 (A) BA.2 (B), BA.2.75 (C), BA.3 (D), BA.4/BA.5 (E) and BQ.1.1 (F). The pLDDT density distribution for conformations obtained using AF2-MSA shallow depth are shown in orange-colored filled bars and the pLDDT distribution for conformations using AF2 with randomized sequence scanning are in maroon filled bars. | |
The RMSD distributions of protein conformational ensembles generated by alanine scanning AF2 adaptation quantified the extent of heterogeneity for the predicted states (Fig. 9). The shallow peaks of RMSDs ∼ 0.8–1.7 Å from the experimental structures were seen for all RBD–ACE2 complexes, reflecting moderate conformational motions that are typically observed in MD simulations of the Omicron RBD–ACE2 complexes.67
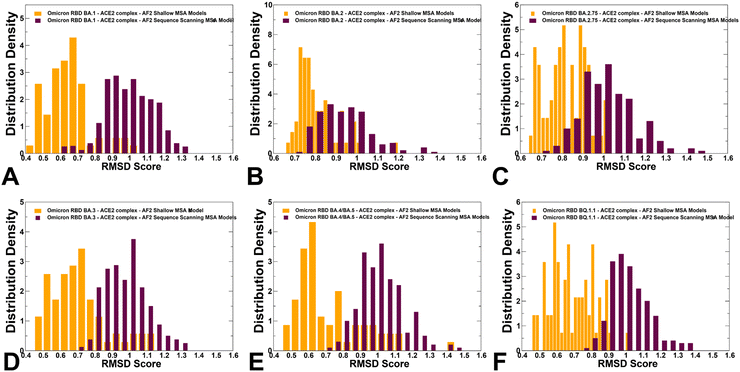 |
| Fig. 9 The distributions of the RMSD values from the experimental structures for the RBD–ACE2 conformational ensembles obtained from AF2-MSA shallow depth predictions and AF2 with randomized sequence scanning. The density distribution of the RMSD values for structural ensembles are shown for BA.1 (A) BA.2 (B), BA.2.75 (C), BA.3 (D), BA.4/BA.5 (E) and BQ.1.1 (F). The RMSD density distribution for conformations obtained using AF2-MSA shallow depth are shown in orange-colored filled bars and the RMSD distribution for conformations using AF2 with randomized sequence scanning are in maroon filled bars. | |
The scatter plots between pLDDT and RMSD values for protein conformations produced by AF2 with alanine sequence scanning showed a statistically significant correlation (ESI,† Fig. S5), also revealing several clusters of conformations associated with the higher RMSD ∼ 1.0–1.7 Å and respectively lower pLDDT values ∼60–70. Instructively, the scatter plots displayed a greater dispersion of the distribution with a significant fraction of generated conformations yielding pLDDT values ∼60–70 and RMSDs < 1.0 Å from the native structure (ESI,† Fig. S5). As a result, the generated ensembles can describe moderate functionally relevant displacements of the RBD conformations around the experimentally determined structures of the RBD–ACE2 complexes. In particular, and importantly, randomized alanine scanning AF2 experiments showed the ability to generate functional ensemble of the RBD conformations with a variability for the RBM loop. Hence, by combining the AF2-genersted ensembles can expand the scope of sampled conformational states. Moreover, the results showed that the inverse correlation between pLDDT and RMSD values can be preserved in the generated ensemble (ESI,† Fig. S5), supporting the notion that the pLDDT metric can serve as a robust indicator of the functional conformational ensembles. Based on the results of this analysis we argue that by selecting AF2-generated conformations produced by both AF2 schemes with high confidence pLDDT values ∼70–90 the predicted pool of functional RBD conformations can be judiciously expanded.
Of particular interest is a comparison of PCA plots for conformational ensembles produced by shallow MSA subsampling (ESI,† Fig. S3) and randomized alanine scanning of the full sequence (Fig. 10). The PCA projection enables characterization of the underlying conformational landscapes for the RBD–ACE2 complexes by mapping densities of states from the high-dimensional to low-dimensional space. The conformational densities are generally dominated by clusters of states near the native structures of the RBD–ACE2 complexes, while differences in minor density clusters between variants can reflect the extent and nature of conformational flexibility in the RBD loops (Fig. 10). Furthermore, the PCA plots also revealed a significantly greater heterogeneity of the generated RBD conformations (Fig. 10) as compared to more limited variability of the RBD states obtained with shallow MSA subsampling. Interestingly, this analysis showed that conformational plasticity around the native RBD structure is present for all variants, particularly highlighting the increased heterogeneity of BA.2 (Fig. 10B) and BQ.1.1 variants (Fig. 10F) which is consistent with biophysical studies.39,40
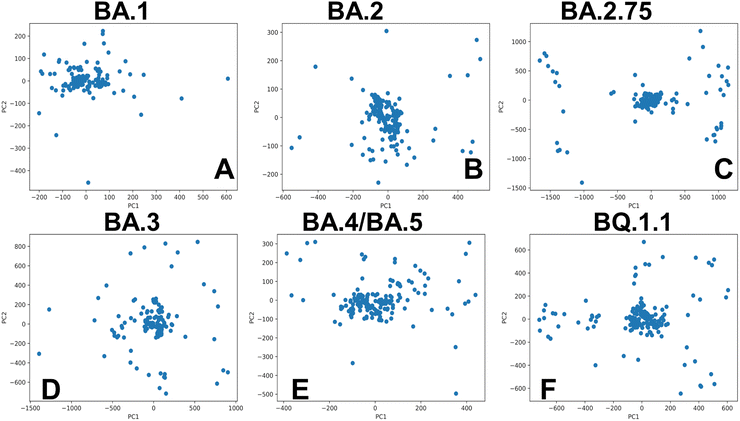 |
| Fig. 10 PCA of the AF2-generated ensembles for the Omicron RBD–ACE2 complexes using randomized alanine sequence scanning adaptation of AF2. PCA graphs are shown for the BA.1 RBD–ACE2 complex (A), BA.2 RBD–ACE2 complex (B), BA.2.75 RBD–ACE2 complex (C), BA.3 RBD–ACE2 complex (D), BA.4/BA.5 RBD–ACE2 complex (E), and BQ.1.1 RBD–ACE2 complex (F). | |
Structural alignment of the AF2 conformational ensembles illustrated the predicted patterns of RBD mobility in which the RBD core and most of the loops largely remain in their native positions, while most of variability is observed in the RBD loop 457–475, RBM loop 475–487 and peripheral flexible region (residues 520–527) (Fig. 11). This flexible region is immediately next to the C-terminal domain CTD1 (residues 529–591) in the full length S trimer that functions as a structural relay between RBD and S2 regions that can arguably sense the functional movements in the S1 and S2 subunits. The flexible region 520–527 in the trimer is a part of the RBD-CTD1 hinge connecting the C-terminal of the RBD with CTD1 (C-connector). Together with the N-connector located near the N-terminal of the RBD, these flexible regions can modulate RBD openings during functional changes between the closed and open RBD states. Instructively, we found that the conformational ensembles for BA.2 (Fig. 11B), BA./BA.5 (Fig. 11E) and BQ.1.1 complexes (Fig. 11F) displayed moderate variations of the RBD loops and were mostly confined to the native state. At the same time, more heterogeneous conformational ensembles were obtained for BA.1 (Fig. 11A) and BA.3 variants (Fig. 11D). Interestingly, one of the most dynamic regions of the RBD (loop 457–475) is immediately adjacent to residues L455 and F456 that emerged among convergent mutational hotspots in the XBB variants.111–113 The predicted RBD conformations also yielded accurate side-chain conformations (Fig. 11G and H). This becomes particularly relevant and important to accurately evaluate the strength of RBD–ACE2 interactions and binding affinities of the RBD–ACE2 complexes. Based on these findings, we argue that the AF2-predicted conformational plasticity in the RBM region may be functionally significant. Importantly, pLDDT assessment of the AF2 models can be used to quantify the variability and extent of dynamic changes in the flexible RBD regions. Although AF2 predictions do not necessarily represent the equilibrium ensemble of conformations, our results suggested that the generated conformational states may represent functionally significant representatives of the equilibrium ensemble of RBD conformations. In general, these results showed that AF2-generated conformational ensembles can accurately reproduce the experimental structures and capture conformational details of the RBD fold and variant-specific functional adjustments of the RBM loop impacting the exposure for mutational positions in this region.
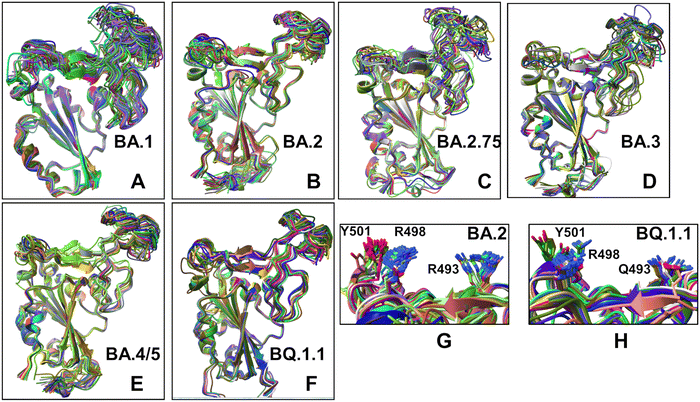 |
| Fig. 11 Structural alignment of the AF2-generated conformational ensembles obtained by AF2 shallow MSA approach and AF2 adaptation with randomized alanine sequence scanning. Structural alignment of the AF2-predicted ensemble of RBD conformations with the respective experimental structures (shown in orange ribbons) for the BA.1 RBD–ACE2 complex (A), BA.2 RBD–ACE2 complex (B), BA.2.75 RBD–ACE2 complex (C), BA.3 RBD–ACE2 complex (D), BA.4/BA.5 RBD–ACE2 complex (E), and BQ.1.1 RBD–ACE2 complex (F). The RBD conformations are shown in ribbons. (G) A closeup of the side-chain conformations in the predicted ensemble of BA.2 RBD–ACE2 complex for the key Omicron mutational sites R493, R498 and Y501. The residue conformations are shown in sticks. (H) A closeup of the side-chain conformations in the predicted ensemble of BQ.1.1 RBD–ACE2 complex for the key Omicron mutational sites R493Q, R498 and Y501. The residue conformations are shown in sticks. | |
Atomistic MD simulations of the XBB RBD–ACE2 ACE2 complexes
To characterize conformational landscapes and dynamic signatures of the Omicron RBD–ACE2 variants we also conducted microsecond MD simulations (Fig. 10). Conformational dynamics profiles obtained from MD simulations were similar and revealed several important trends. The RMSF profiles showed local minima regions corresponding to the structured five-stranded antiparallel β-sheet core region that functions as a stable core scaffold (residues 350–360, 375–380, 394–403) and the interfacial RBD positions involved in the contacts with the ACE2 receptor (Fig. 12A and B). The RMSF profiles also displayed common RMSF peaks corresponding to the flexible RBD regions including residues 360–373, residues 380–396 as well as RBM tip residues 475–487. As expected, the conformational dynamics profiles also showed the increased RMSF values in the flexible N-terminal and C-terminal ends of the RBD structure, but fluctuations in these regions were moderate as compared to more elevated mobility of C-terminal RBD region (residues 515–527) in the AF2 predictions (Fig. 12A and B). The RBD core regions (residues 390–420, 430–450) exhibited small fluctuations, particularly in the BA.2 and BA.2.75 variants (Fig. 12A) suggesting the increased RBD stability for these variants which may be a relevant contributing factor to the experimentally observed stronger ACE2 binding. In addition, the RMSF profiles are characterized by several local minima corresponding to the ACE2 interfacial sites (residues 485–505). Consistent with AF2 predictions, the MD profiles displayed larger displacements in the flexible RBD regions (residues 355–375, 381–394, 444–452, 455–471, 475–487, 515–527) for BA.3 and BA.4/BA.5 complexes (Fig. 12A and B). Despite significant similarities of the RMSF profiles, we found several notable differences that are particularly important in the context of the AF2 predictions.
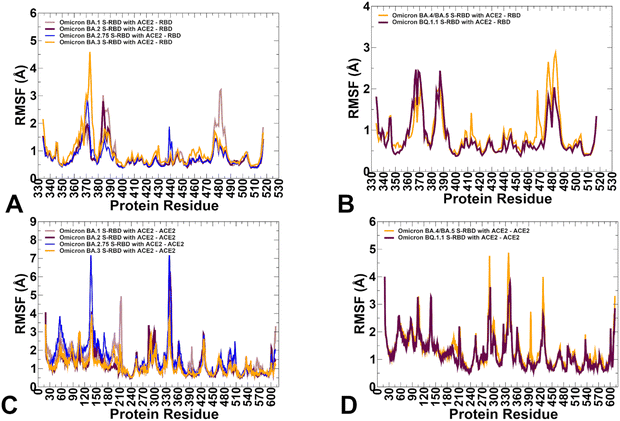 |
| Fig. 12 Conformational dynamics profiles obtained from all-atom MD simulations of the Omicron RBD complexes with ACE2. (A) The RMSF profiles for the RBD residues obtained from MD simulations of the BA.1 RBD–ACE2 complex, pdb id 7WBP (in light brown lines), the BA.2 RBD–ACE2 complex, pdb id 7XB0 (in maroon lines), BA.2.75 RBD–ACE2 complex, pdb id 8ASY (in blue lines) and BA.3 RB-ACE2 complex, pdb id 7XB1 (in orange lines). (B) The RMSF profiles for the RBD residues obtained from MD simulations of the BA.4/BA.5 RBD–ACE2 complex, pdb id 8AQS (in orange lines) and the BQ.1.1 RBD–ACE2 complex, pdb id 8IF2 (in maroon lines). (C) The RMSF profiles for the ACE2 residues obtained from MD simulations of the BA.1 RBD–ACE2 complex (in light brown lines), the BA.2 RBD–ACE2 complex (in maroon lines), BA.2.75 RBD–ACE2 complex (in blue lines) and BA.3 RB-ACE2 complex (in orange lines). (D) The RMSF profiles for the ACE2 residues obtained from MD simulations of the BA.4/BA.5 RBD–ACE2 complex, pdb id 8AQS (in orange lines) and the BQ.1.1 RBD–ACE2 complex, pdb id 8IF2 (in maroon lines). | |
The dynamics profiles showed the markedly larger fluctuations of the RBM tip region (residues 475–487) in the BA.1 variant and smallest fluctuations in this region for BA.2.75 variant (Fig. 12A). We also observed smaller fluctuations in BA.2.75 for the hairpin loop (residues 373–380). These findings are consistent with the experimental studies showing that the reversion of R493Q and local conformational alterations in the hairpin loop 373–380 can result in the overall improved stability for the RBD and the RBM region.35 It is worth noting that the AF2-generated ensembles for BA.2.75 variant showed more conformational plasticity as compared to MD simulations and produced high confidence RBD states with lateral changes in the RBB tip. MD simulations also highlighted the moderately increased flexibility of the BA.4/BA.5 RBD as compared to more stable BQ.1.1 variant (Fig. 12B). These observations are in line with structural analysis showing that F486V in the BA.4/5 S RBD may decrease the hydrophobic interaction with ACE2 and promote greater mobility of the RBM region, while Q493 forms a hydrogen bond with the ACE2 residue H34 can compensate for the loss of binding.38,39 Consistent with this analysis, conformational dynamics profile of the BA.4/BA.5 RBD–ACE2 complex revealed markedly increased flexibility of the RBM region where the RBM tip residues, including F486V position can undergo RMSF changes ∼2.5–3.0 Å (Fig. 12B). The conformational dynamics profile of the ACE2 receptor showed a similar and strong stabilization of the interfacial helices on ACE2, indicating that dynamics signatures of ACE2 are conserved across all Omicron RBD complexes (Fig. 12C and D). While the stability of the ACE2 interfacial regions (residues 350–395) is stronger in the BA.2 and BA.2.75 complexes, MD simulations revealed larger fluctuations in the flexible ACE2 regions for these variants which may reflect long-range communications between the binding interface and peripheral regions in the ACE2.
In general, a comparison of AF2 ensemble predictions and MD simulations suggested that MD profiles can be more sensitive to mutational changes and depict subtle changes in the conformational stability between variants. The range of thermal fluctuations in the MD-generated conformational ensembles is more limited than in the AF2 ensembles and confined to the close proximity of the native state. In some contrast, AF2-generated conformational ensembles may produce a broader pool of the energetically favorable and diverse RBD conformations, enabling a better representation of functional RBM displacements and movements of the flexible RBD loops during binding with the ACE2 receptor. Despite the increased mobility, the RBM tip maintains a stable folded “hook” conformation that is similar to the cryo-EM conformations.
To illustrate similarities and differences between conformational landscapes produced by AF2 adaptations and MD simulations, we constructed PCA graphs using MD trajectories of BA.1 and BA.2 Omicron RBD–ACE2 (ESI,† Fig. S6). Despite some differences, conformational densities of states obtained with the sequence scanning AF2 adaptation and MD simulations displayed greater heterogeneity of the BA.2 variant and emergence of several conformational clusters for this Omicron variant, while conformational landscape for the BA.1 RBD–ACE2 complex is largely confined to the native structure. These results suggested complementarities and potential synergies between AF2 predictions of protein conformational ensembles and MD simulations showing that integrating information from both methods can potentially yield a more adequate characterization of the conformational landscapes for the Omicron RBD–ACE2 complexes. Noteworthy, the nature of the AF2 adaptations for probing structural ensembles rather than predicting a single structure is based on different manipulations of the MSAs which drive diversity of conformational predictions without necessarily rigorously considering the underlying thermodynamic distributions. Nonetheless, by evaluating the quality of the generated conformations using pLDDT metric and considering conformations with high confidence pLDDT ∼70–90 these tools can greatly expand coverage of the accessible conformational landscape and identify functionally relevant clusters of states. Our results suggested that the AF2 predicted ensemble of RBD conformations can capture important dynamics signatures of the RBD–ACE2 complexes obtained from microscond MD simulations. However, using AF2 predictions to directly map the conformational dynamics landscape might be challenging as conformational flexibility and structural changes are intimately connected with physical models of protein energetics. Ideally, by launching MD runs from a diverse set of the AF2-predicted conformational pool, simulations can reweight structural distributions and provide a physically rigorous assessment of most probable functional conformations. Hence, combining AF2 predictions with MD simulations may enable a more robust and accurate characterization of dynamic binding mechanisms.
MM-GBSA analysis of the binding affinity computations for the RBD–ACE2 complexes
Using the conformational ensembles obtained from AF2 predictions and MD simulations, we computed the binding free energies for the RBD–ACE2 complexes using the MM-GBSA method.101–104 The results of MM-GBSA computations using MD-based equilibrium ensemble showed a good agreement with the experimental SPR-measured binding affinities for the RBD–ACE2 complexes (Table 2). Interestingly, the decomposition of binding free energy terms showed relatively similar contributions of the van der Waals and electrostatic interactions in the BA.2 and BA.2.75 RBD–ACE2 complexes that yielded the best binding free energies (Table 2).
Table 2 MM-GBSA binding energies for the RBD–ACE2 complexes using MD ensembles
System |
E
vdW
|
E
elec
|
E
GB
|
E
SA
|
ΔGbind (kcal mol−1) |
Exp. binding (nM) |
BA.1 RBD–ACE2 |
−102.42 |
−1420.36 |
1453.71 |
−13.35 |
−82.42 |
19.5 |
BA.2 RBD–ACE2 |
−113.81 |
−1474.06 |
1514.1 |
−14.82 |
−88.58 |
10.0 |
BA.2.75 RBD–ACE2 |
−113.47 |
−1475.26 |
1512.98 |
−14.75 |
−90.05 |
8.21 |
BA.3 RBD–ACE2 |
−102.97 |
−1453.82 |
1492.93 |
−13.79 |
−83.94 |
22 |
BA.4/BA.5 RBD–ACE2 |
−84.23 |
−1337.37 |
1344.24 |
−10.95 |
−88.31 |
14.37 |
BQ.1.1 RBD–ACE2 |
−95.51 |
−1231.18 |
1251.38 |
−12.74 |
−88.06 |
14.70 |
These energetic contributions are also the most favorable for BA.2 and BA.2.75 complexes. The analysis revealed that for BA.1, BA.2, BA.2.75 and BA.3 variants the increased electrostatic contributions are positively correlated with the enhanced ACE2-binding affinities. At the same time, we noticed the reduced electrostatic contributions in BA.4/BA.5 which may be attributable to L452R and R493Q reversal mutations. A number of studies emphasized the role of electrostatic interactions as a dominant thermodynamic force leading at binding of the S-protein with the ACE2 receptor and antibodies.114–116
We employed the AF2-generated single structures, AF2-generated conformational ensembles and MD trajectories in the MM-GBSA computations of binding affinities. Interestingly, while AF2-predicted single structures resulted in a relatively moderate correlation with the experiments (Fig. 13A) both conformational ensembles yielded a strong correlation between the experimental dissociation constants and the predicted binding free energies (Fig. 13B and C). The correlation graph of binding energies based on the AF2 conformational ensemble showed a larger dispersion of data points, while MD-based binding energies yielded only small standard deviations indicating that data points are clustered tightly around the mean. We also performed the residue-based decomposition of the MM-GBSA energies computed with MD ensembles.
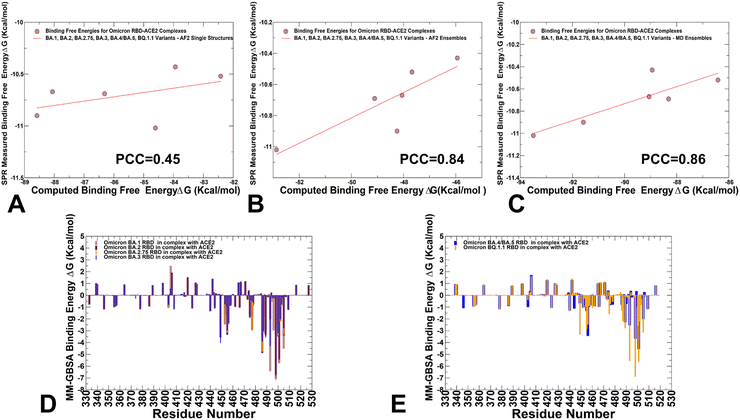 |
| Fig. 13 MM-GBSA binding energy analysis of the Omicron RBD–ACE2 complexes. The scatter correlation graph between the SPR-measured binding affinities and the computed binding free energies based on AF2-generated single structures (A), AF2 conformational ensembles using randomized alanine scanning (B), and MD-generated equilibrium conformational ensembles (C). The Pearson correlation coefficient values are indicated. (D) The residue-based decomposition of the total MM-GBSA binding energy ΔG contribution for the BA.1 RBD–ACE2 complex (light brown bars), BA.2 RBD–ACE2 complex (maroon bars), BA.2.75 RBD–ACE2 complex (orange bars) and BA.3 RBD–ACE2 complex (blue bars). (E) The residue-based decomposition of the total MM-GBSA binding energy ΔG contribution for the BA.4/BA.5 RBD–ACE2 complex (blue bars), and BQ.1.1 RBD–ACE2 complex (orange bars). The MM-GBSA contributions are evaluated using 1000 samples from MD simulations. The statistical errors for the residue-based decomposition of the total MM-GBSA binding energy ΔG contribution were estimated on the basis of the deviation between block average and are within 0.55–1.25 kcal mol−1. | |
The analysis revealed that the binding energies are determined by contributions of only several hotspot centers (Fig. 13D and E). The major contributors of binding affinity in the RBD–ACE2 complexes include RBD residues L455, F456, F486, N487, Y489, Q493, R498, T500, Y501 and G502. Among these sites, the strongest binding centers correspond to Omicron hotspots R498 and Y501 that are also known to cooperate in ACE2 binding via strong epistatic interactions.46,47 (Fig. 13D and E). A comparison of the binding contributions for BA1, BA.2, BA.2.75 and BA.3 complexes indicated that the key hotspots at positions F486, Y489, F490, R493 (Q493), R498 and Y501 provide the most favorable contributions for BA.2 and BA.2.75 variants (Fig. 13D). The second group of binding hotspots includes L455, F456 positions.
Mutational sites that contribute to the ACE2-binding affinity are also located in the flexible RBD regions, including D405N, N440K, L452R, S477N, T478K, E484A, Q493R, Q498R and N501Y, among which Q493R and N501Y are the most significant. In addition, small binding contributions provided by RBD residues K378, R403, K424, K440, K444, K460, N477, K478 are determined by strong electrostatic interactions mediated by lysine residues, which is the result of Omicron evolution leading to significant accumulation of positively charged substitutions interacting with the negatively charged ACE2 binding interface. To separately analyze differences between BA.4/BA.5 and BQ.1.1 complexes, we compared the contributions of individual RBD residues (Fig. 13E). The breakdown highlighted stronger contributions of binding hotspots V486, Q493, R498 and Y501 in BQ.1.1 variant. Indeed, in BA.4/BA.5 the binding energy contribution for Q493 is ΔG = −2.54 kcal mol−1 while in the BQ.1.1 complex this contribution ΔG = −5.54 kcal mol−1. Similarly, more favorable energy ΔG = −6.88 kcal mol−1 for Y501 in BQ.1.1 can be contrasted with less favorable ΔG = −4.54 kcal mol−1 for BA.4/BA.5 (Fig. 13E). Of particular interest is comparison of contributions for convergent mutations in BQ.1.1 R346T, K444T, L452R, N460K, and F486V. It appeared that L452R and N460K sites are characterized by favorable electrostatic contributions resulting in the net ΔG = −1.5 kcal mol−1 for L452R and ΔG = −1.06 kcal mol−1 for N460K in BQ.1.1. In BA.4/BA.5 complex, we found a similar contribution of L452R position but the total binding contribution for N460 position is only ΔG = 0.04 kcal mol−1. At the same time, the presence of R346 and K444 in BA.4/BA.5 produces electrostatically-driven favorable contributions for both sites (ΔG = −1.08 kcal mol−1 for R346 and ΔG = −1.11 kcal mol−1 for K444). On the other side, R346T an K4454T mutations result in minor unfavorable contributions (ΔG = 0.18 kcal mol−1 for R346T and ΔG = 0.19 kcal mol−1 for K444T). The decreased charge on the BQ.1.1 RBD is due to mutations of two positively charged residues, R346T and K444T that only weakly interact with ACE2 which leads to a markedly reduced electrostatic contribution. These observations are consistent with previous studies showing that both R346T and K444T residues have a destabilizing effect on the interaction between RBD and ACE2.117 The negligible contributions of R346T and K444T mutational sites in BQ.1.1 were also confirmed in DMS study of BQ.1.1 variant in which mutations in these positions produced ΔG values within 0.1 kcal mol−1 and the reversed T346R and T444K modifications become basically neutral in the BQ.1.1 background.52 Hence, the emergence of reversed mutations introducing large positive charge induced a fairly “muted” effect on binding in the BQ.1.1, suggesting that the electrostatic contribution becomes less dominant in this Omicron variant. The latest study showed that the R346T, K444T, and N460K modifications can greatly affect the immune escape potential of BQ.1.1 rather than the affinity for the receptor.42 Our results are consistent with this study showing that R346T and K444T are not involved in the interaction with ACE2, and these mutations do not perturb the loop structure compared to BA.4/BA.5. Another functional study demonstrated that R336T and K444T mutations may have emerged to reduce electrostatic interactions with antibodies and boost immune escape profile,118 suggesting that the observed reverse of the “electrostatic driving force” of ACE2 binding affinity for BQ.1.1 may be byproduct of optimized immune evasion profile. Another important finding of the binding energy analysis is that the effect of most Omicron RBD mutations on binding with ACE2 is rather small despite appreciable mutational modifications. Our results are consistent with the DMS experiments performed in different genetic backgrounds of BA,1, BA.2, BQ,1.1 and XBB.1.5 variants119–121 showing for example that many sites in the BA2 and BQ.1.1 RBDs are highly tolerant to mutations. These experimental studies showed that tolerance to mutations in many RBD positions is not a sign of insignificance as RBD residues that interact with ACE2 could also be involved in immune escape and exhibit considerable mutational plasticity.122
To summarize, the central result of this analysis is the evidence that AF2-generated extended conformational ensembles can yield fairly accurate binding energies for Omicron RBD–ACE2 complexes leading to strong correlations with the experimental dissociation constants. Moreover, the observed correlations with the experiments using MD-derived equilibrium ensemble and AF2-generated conformational ensemble are quite similar. Hence, despite the lack of physically rigorous thermodynamic distribution of the equilibrium states in the AF2 predictions, AF2-based adaptations can efficiently explore and predict pools of functionally relevant conformational states that dominate the thermodynamics of the binding reaction.
Conclusions
Atomistic level structural predictions and microsecond atomistic MD simulations provided a detailed characterization of the conformational ensembles and identified important differences in conformational landscape of the Omicron variants. Several different adaptations of the AF2 methodology along with MD simulations were used for a comparative characterization of structures, conformational ensembles and subsequent computations of binding affinities of the Omicron RBD–ACE2 complexes including BA.1, BA.2, BA.2.75, BA.3, BA.4/BA.5 and BQ.1.1 variants. By integrating predictions of the conformational states using AF2-based approaches we can efficiently expand characterization of the conformational ensembles for the RBD–ACE2 complexes capturing conformational details of the RBD fold and variant-specific functional adjustments of the RBD functional regions and mutational sites. We found that by evaluating the quality of the generated conformations using pLDDT metric and considering conformations with high confidence pLDDT values of ∼70–90 AF2 approaches can greatly expand coverage of the accessible conformational landscape and characterize functional protein ensembles. We leveraged AF2-based structural ensembles and MD-generated equilibrium ensembles for accurate comparative prediction of the binding energetics for the Omicron RBD–ACE2 complexes, which is consistent with the experimental data. The important finding of this analysis is that AF2-generated extended conformational ensembles can produce accurate binding energies for Omicron RBD–ACE2 complexes leading to strong correlations with the experimental dissociation constants. Our analysis offered support to the notion that using pLDDT assessment of structural confidence for screening of generated structures can efficiently determine the ensemble of functionally important conformations to compute binding affinities. The results suggested that combining MD simulations together with AF2-based prediction of conformational ensembles could provide more comprehensive view of the conformational landscapes and binding mechanisms for Omicron variants.
Data availability
The data underlying this study are available in the article and ESI.† Crystal structures were obtained and downloaded from the Protein Data Bank (https://www.rcsb.org). All simulations were performed using the all-atom additive CHARMM36 protein force field that can be obtained from https://mackerell.umaryland.edu/charmm_ff.shtml. The rendering of protein structures was done with ChimeraX package (https://www.rbvi.ucsf.edu/chimerax/) and Pymol (https://pymol.org/2/). The software tools used in this study are freely available at the following GitHub sites: https://github.com/deepmind/alphafold; https://github.com/sokrypton/ColabFold/; https://www.github.com/HWaymentSteele/AFCluster; https://github.com/nextstrain; https://github.com/Amber-MD/cpptraj; https://github.com/Amber-MD/cpptraj. Data for this article, including AF2-generated protein structures and conformational ensembles obtained using different approaches, simulation trajectories, topology and parameter files, the software tools, and scripts are freely available at ZENODO website https://zenodo.org/records/10904485.
Author contributions
Conceptualization, G. V.; methodology, N. R., M. A., G. C., S. X., G. V. P. T.; software, N. R., S. X., M. A., G. G., G. V. and P. T.; validation, N. R., G. V.; formal analysis, N. R., G. V., M. A., G. G., S. X., and P. T.; investigation, N. R., M. A., G. C., G. V. and P. T.; resources, N. R., G. V., M. A. S. X., and G. V.; data curation, N. R., M. A., G. C.,G. V.; writing—original draft preparation, N. R., M. A., G. V.; writing—review and editing, N. R. and G. V.; visualization, N. R., M. A., G. C., S. X., G. V. G. V.; supervision, G. V.; project administration, G. V.; funding acquisition, P. T. and G. V. All authors have read and agreed to the published version of the manuscript.
Conflicts of interest
The authors declare no conflict of interest. The funders had no role in the design of the study; in the collection, analyses, or interpretation of data; in the writing of the manuscript; or in the decision to publish the results.
Acknowledgements
This research was supported by the National Institutes of Health under Award 1R01AI181600-01 and Subaward 6069-SC24-11 to G. V. and National Institutes of Health under Award No. R15GM122013 to P. T. G. V. acknowledges support from Schmid College of Science and Technology at Chapman University for providing computing resources at the Keck Center for Science and Engineering.
References
- W. Tai, L. He, X. Zhang, J. Pu, D. Voronin, S. Jiang, Y. Zhou and L. Du, Characterization of the receptor-binding domain (RBD) of 2019 novel coronavirus: implication for development of RBD protein as a viral attachment inhibitor and vaccine, Cell. Mol. Immunol., 2020, 17, 613–620, DOI:10.1038/s41423-020-0400-4.
- Q. Wang, Y. Zhang, L. Wu, S. Niu, C. Song, Z. Zhang, G. Lu, C. Qiao, Y. Hu, K. Y. Yuen, Q. Wang, H. Zhou, J. Yan and J. Qi, Structural and functional basis of SARS-CoV-2 entry by using human ACE2, Cell, 2020, 181, 894–904.e9, DOI:10.1016/j.cell.2020.03.045.
- A. C. Walls, Y. J. Park, M. A. Tortorici, A. Wall, A. T. McGuire and D. Veesler, Structure, Function, and Antigenicity of the SARS-CoV-2 Spike Glycoprotein, Cell, 2020, 181, 281–292.e6, DOI:10.1016/j.cell.2020.02.058.
- D. Wrapp, N. Wang, K. S. Corbett, J. A. Goldsmith, C. L. Hsieh, O. Abiona, B. S. Graham and J. S. McLellan, Cryo-EM structure of the 2019-nCoV spike in the prefusion conformation, Science, 2020, 367, 1260–1263, DOI:10.1126/science.abb2507.
- Y. Cai, J. Zhang, T. Xiao, H. Peng, S. M. Sterling, R. M. Walsh Jr., S. Rawson, S. Rits-Volloch and B. Chen, Distinct conformational states of SARS-CoV-2 spike protein, Science, 2020, 369, 1586–1592, DOI:10.1126/science.abd4251.
- C. L. Hsieh, J. A. Goldsmith, J. M. Schaub, A. M. DiVenere, H. C. Kuo, K. Javanmardi, K. C. Le, D. Wrapp, A. G. Lee, Y. Liu, C. W. Chou, P. O. Byrne, C. K. Hjorth, N. V. Johnson, J. Ludes-Meyers, A. W. Nguyen, J. Park, N. Wang, D. Amengor, J. J. Lavinder, G. C. Ippolito, J. A. Maynard, I. J. Finkelstein and J. S. McLellan, Structure-based design of prefusion-stabilized SARS-CoV-2 spikes, Science, 2020, 369, 1501–1505, DOI:10.1126/science.abd0826.
- R. Henderson, R. J. Edwards, K. Mansouri, K. Janowska, V. Stalls, S. M. C. Gobeil, M. Kopp, D. Li, R. Parks, A. L. Hsu, M. J. Borgnia, B. F. Haynes and P. Acharya, Controlling the SARS-CoV-2 spike glycoprotein conformation, Nat. Struct. Mol. Biol., 2020, 27, 925–933, DOI:10.1038/s41594-020-0479-4.
- M. McCallum, A. C. Walls, J. E. Bowen, D. Corti and D. Veesler, Structure-guided covalent stabilization of coronavirus spike glycoprotein trimers in the closed conformation, Nat. Struct. Mol. Biol., 2020, 27, 942–949, DOI:10.1038/s41594-020-0483-8.
- X. Xiong, K. Qu, K. A. Ciazynska, M. Hosmillo, A. P. Carter, S. Ebrahimi, Z. Ke, S. H. W. Scheres, L. Bergamaschi, G. L. Grice, Y. Zhang, CITIID-NIHR COVID-19 BioResource Collaboration, J. A. Nathan, S. Baker, L. C. James, H. E. Baxendale, I. Goodfellow, R. Doffinger and J. A. G. Briggs, A thermostable, closed SARS-CoV-2 spike protein trimer, Nat. Struct. Mol. Biol., 2020, 27, 934–941, DOI:10.1038/s41594-020-0478-5.
- S. M. Costello, S. R. Shoemaker, H. T. Hobbs, A. W. Nguyen, C. L. Hsieh, J. A. Maynard, J. S. McLellan, J. E. Pak and S. Marqusee, The SARS-CoV-2 spike reversibly samples an open-trimer conformation exposing novel epitopes, Nat. Struct. Mol. Biol., 2022, 27, 229–238, DOI:10.1038/s41594-022-00735-5.
- K. D. McCormick, J. L. Jacobs and J. W. Mellors, The emerging plasticity of SARS-CoV-2, Science, 2021, 371, 1306–1308, DOI:10.1126/science.abg4493.
- D. Ghimire, Y. Han and M. Lu, Structural Plasticity and Immune Evasion of SARS-CoV-2 Spike Variants, Viruses, 2022, 14, 1255, DOI:10.3390/v14061255.
- C. Xu, Y. Wang, C. Liu, C. Zhang, W. Han, X. Hong, Y. Wang, Q. Hong, S. Wang, Q. Zhao, Y. Wang, Y. Yang, K. Chen, W. Zheng, L. Kong, F. Wang, Q. Zuo, Z. Huang and Y. Cong, Conformational dynamics of SARS-CoV-2 trimeric spike glycoprotein in complex with receptor ACE2 revealed by cryo-EM, Sci. Adv., 2021, 7, eabe5575, DOI:10.1126/sciadv.abe5575.
- D. J. Benton, A. G. Wrobel, P. Xu, C. Roustan, S. R. Martin, P. B. Rosenthal, J. J. Skehel and S. J. Gamblin, Receptor binding and priming of the spike protein of SARS-CoV-2 for membrane fusion, Nature, 2020, 588, 327–330, DOI:10.1038/s41586-020-2772-0.
- B. Turoňová, M. Sikora, C. Schuerman, W. J. H. Hagen, S. Welsch, F. E. C. Blanc, S. von Bülow, M. Gecht, K. Bagola, C. Hörner, G. van Zandbergen, J. Landry, N. T. D. de Azevedo, S. Mosalaganti, A. Schwarz, R. Covino, M. D. Mühlebach, G. Hummer, J. Krijnse Locker and M. Beck, In situ structural analysis of SARS-CoV-2 spike reveals flexibility mediated by three hinges, Science, 2020, 370, 203–208, DOI:10.1126/science.abd5223.
- M. Lu, P. D. Uchil, W. Li, D. Zheng, D. S. Terry, J. Gorman, W. Shi, B. Zhang, T. Zhou, S. Ding, R. Gasser, J. Prevost, G. Beaudoin-Bussieres, S. P. Anand, A. Laumaea, J. R. Grover, L. Lihong, D. D. Ho, J. R. Mascola, A. Finzi, P. D. Kwong, S. C. Blanchard and W. Mothes, Real-time conformational dynamics of SARS-CoV-2 spikes on virus particles, Cell Host Microbe, 2020, 28, 880–891.e8, DOI:10.1016/j.chom.2020.11.001.
- Z. Yang, Y. Han, S. Ding, W. Shi, T. Zhou, A. Finzi, P. D. Kwong, W. Mothes and M. Lu, SARS-CoV-2 Variants Increase Kinetic Stability of Open Spike Conformations as an Evolutionary Strategy, mBio, 2022, 13, e0322721, DOI:10.1128/mbio.03227-21.
- M. A. Díaz-Salinas, Q. Li, M. Ejemel, L. Yurkovetskiy, J. Luban, K. Shen, Y. Wang and J. B. Munro, Conformational dynamics and allosteric modulation of the SARS-CoV-2 spike, eLife, 2022, 11, e75433, DOI:10.7554/eLife.75433.
- P. Han, L. Li, S. Liu, Q. Wang, D. Zhang, Z. Xu, X. Li, Q. Peng, C. Su, B. Huang, D. Li, R. Zhang, M. Tian, L. Fu, Y. Gao, X. Zhao, K. Liu, J. Qi, G. F. Gao and P. Wang, Receptor binding and complex structures of human ACE2 to spike
RBD from omicron and delta SARS-CoV-2, Cell, 2022, 185, 630–640, DOI:10.1016/j.cell.2022.01.001.
- J. W. Saville, D. Mannar, X. Zhu, S. S. Srivastava, A. M. Berezuk, J. P. Demers, S. Zhou, K. S. Tuttle, I. Sekirov, A. Kim, W. Li, D. S. Dimitrov and S. Subramaniam, Structural and biochemical rationale for enhanced spike protein fitness in delta and kappa SARS-CoV-2 variants, Nat. Commun., 2022, 13, 742, DOI:10.1038/s41467-022-28324-6.
- Y. Wang, C. Liu, C. Zhang, Y. Wang, Q. Hong, S. Xu, Z. Li, Y. Yang, Z. Huang and Y. Cong, Structural basis for SARS-CoV-2 Delta variant recognition of ACE2 receptor and broadly neutralizing antibodies, Nat. Commun., 2022, 13, 871, DOI:10.1038/s41467-022-28528-w.
- J. Zhang, T. Xiao, Y. Cai, C. L. Lavine, H. Peng, H. Zhu, K. Anand, P. Tong, A. Gautam, M. L. Mayer, R. M. Walsh Jr., S. Rits-Volloch, D. R. Wesemann, W. Yang, M. S. Seaman, J. Lu and B. Chen, Membrane fusion and immune evasion by the spike protein of SARS-CoV-2 Delta variant, Science, 2021, 374, 1353–1360, DOI:10.1126/science.abl9463.
- D. Mannar, J. W. Saville, X. Zhu, S. S. Srivastava, A. M. Berezuk, K. S. Tuttle, A. C. Marquez, I. Sekirov and S. Subramaniam, SARS-CoV-2 Omicron variant: Antibody evasion and cryo-EM structure of spike protein-ACE2 complex, Science, 2022, 375, 760–764, DOI:10.1126/science.abn7760.
- Q. Hong, W. Han, J. Li, S. Xu, Y. Wang, C. Xu, Z. Li, Y. Wang, C. Zhang, Z. Huang and Y. Cong, Molecular basis of receptor binding and antibody neutralization of Omicron, Nature, 2022, 604, 546–552, DOI:10.1038/s41586-022-04581-9.
- M. McCallum, N. Czudnochowski, L. E. Rosen, S. K. Zepeda, J. E. Bowen, A. C. Walls, K. Hauser, A. Joshi, C. Stewart, J. R. Dillen, A. E. Powell, T. I. Croll, J. Nix, H. W. Virgin, D. Corti, G. Snell and D. Veesler, Structural basis of SARS-CoV-2 Omicron immune evasion and receptor engagement, Science, 2022, 375, 864–868, DOI:10.1126/science.abn8652.
- W. Yin, Y. Xu, P. Xu, X. Cao, C. Wu, C. Gu, X. He, X. Wang, S. Huang, Q. Yuan, K. Wu, W. Hu, Z. Huang, J. Liu, Z. Wang, F. Jia, K. Xia, P. Liu, X. Wang, B. Song, J. Zheng, H. Jiang, X. Cheng, Y. Jiang, S. J. Deng and H. E. Xu, Structures of the Omicron Spike trimer with ACE2 and an anti-Omicron antibody, Science, 2022, 375, 1048–1053, DOI:10.1126/science.abn8863.
- S. M.-C. Gobeil, R. Henderson, V. Stalls, K. Janowska, X. Huang, A. May, M. Speakman, E. Beaudoin, K. Manne, D. Li, R. Parks, M. Barr, M. Deyton, M. Martin, K. Mansouri, R. J. Edwards, A. Eaton, D. C. Montefiori, G. D. Sempowski, K. O. Saunders, K. Wiehe, W. Williams, B. Korber, B. F. Haynes and P. Acharya, Structural Diversity of the SARS-CoV-2 Omicron Spike, Mol. Cell, 2022, 82, 2050–2068.e6, DOI:10.1016/j.molcel.2022.03.028.
- Z. Cui, P. Liu, N. Wang, L. Wang, K. Fan, Q. Zhu, K. Wang, R. Chen, R. Feng, Z. Jia, M. Yang, G. Xu, B. Zhu, W. Fu, T. Chu, L. Feng, Y. Wang, X. Pei, P. Yang, X. S. Xie, L. Cao, Y. Cao and X. Wang, Structural and functional characterizations of infectivity and immune evasion of SARS-CoV-2 Omicron, Cell, 2022, 185, 860–871.e13, DOI:10.1016/j.cell.2022.01.019.
- L. Li, H. Liao, Y. Meng, W. Li, P. Han, K. Liu, Q. Wang, D. Li, Y. Zhang, L. Wang, Z. Fan, Y. Zhang, Q. Wang, X. Zhao, Y. Sun, N. Huang, J. Qi and G. F. Gao, Structural basis of human ACE2 higher binding affinity to currently circulating Omicron SARS-CoV-2 sub-variants BA.2 and BA.1.1, Cell, 2022, 185, 2952–2960.e10, DOI:10.1016/j.cell.2022.06.023.
- Y. Xu, C. Wu, X. Cao, C. Gu, H. Liu, M. Jiang, X. Wang, Q. Yuan, K. Wu, J. Liu, D. Wang, X. He, X. Wang, S. J. Deng, H. E. Xu and W. Yin, Structural and biochemical mechanism for increased infectivity and immune evasion of Omicron BA.2 variant compared to BA.1 and their possible mouse origins, Cell Res., 2022, 32, 609–620, DOI:10.1038/s41422-022-00672-4.
- A. Tuekprakhon, R. Nutalai, A. Dijokaite-Guraliuc, D. Zhou, H. M. Ginn, M. Selvaraj, C. Liu, A. J. Mentzer, P. Supasa, H. M. E. Duyvesteyn, R. Das, D. Skelly, T. G. Ritter, A. Amini, S. Bibi, S. Adele, S. A. Johnson, B. Constantinides, H. Webster, N. Temperton, P. Klenerman, E. Barnes, S. J. Dunachie, D. Crook, A. J. Pollard, T. Lambe, P. Goulder, N. G. Paterson, M. A. Williams and D. R. Hall, OPTIC Consortium; ISARIC4C Consortium; Fry, E.E.; Huo, J.; Mongkolsapaya, J.; Ren, J.; Stuart, D.I.; Screaton, G.R. Antibody escape of SARS-CoV-2 Omicron BA.4 and BA.5 from vaccine and BA.1 serum, Cell, 2022, 185, 2422–2433.e13, DOI:10.1016/j.cell.2022.06.005.
- Y. Cao, A. Yisimayi, F. Jian, W. Song, T. Xiao, L. Wang, S. Du, J. Wang, Q. Li, X. Chen, Y. Yu, P. Wang, Z. Zhang, P. Liu, R. An, X. Hao, Y. Wang, J. Wang, R. Feng, H. Sun, L. Zhao, W. Zhang, D. Zhao, J. Zheng, L. Yu, C. Li, N. Zhang, R. Wang, X. Niu, S. Yang, X. Song, Y. Chai, Y. Hu, Y. Shi, L. Zheng, Z. Li, Q. Gu, F. Shao, W. Huang, R. Jin, Z. Shen, Y. Wang, X. Wang, J. Xiao and X. S. Xie, BA.2.12.1, BA.4 and BA.5 escape antibodies elicited by Omicron infection, Nature, 2022, 608, 593–602, DOI:10.1038/s41586-022-04980-y.
- J. E. Bowen, A. Addetia, H. V. Dang, C. Stewart, J. T. Brown, W. K. Sharkey, K. R. Sprouse, A. C. Walls, I. G. Mazzitelli, J. K. Logue, N. M. Franko, N. Czudnochowski, A. E. Powell, E. Dellota Jr., K. Ahmed, A. S. Ansari, E. Cameroni, A. Gori, A. Bandera, C. M. Posavad, J. M. Dan, Z. Zhang, D. Weiskopf, A. Sette, S. Crotty, N. T. Iqbal, D. Corti, J. Geffner, G. Snell, R. Grifantini, H. Y. Chu and D. Veesler, Omicron spike function and neutralizing activity elicited by a comprehensive panel of vaccines, Science, 2022, 377, 890–894, DOI:10.1126/science.abq0203.
- J. Huo, A. Dijokaite-Guraliuc, C. Liu, D. Zhou, H. M. Ginn, R. Das, P. Supasa, M. Selvaraj, R. Nutalai, A. Tuekprakhon, H. M. E. Duyvesteyn, A. J. Mentzer, D. Skelly, T. G. Ritter, A. Amini, S. Bibi, S. Adele, S. A. Johnson, N. G. Paterson, M. A. Williams, D. R. Hall, M. Plowright, T. A. H. Newman, H. Hornsby, T. I. de Silva, N. Temperton, P. Klenerman, E. Barnes, S. J. Dunachie, A. J. Pollard, T. Lambe, P. Goulder, E. E. Fry, J. Mongkolsapaya, J. Ren, D. I. Stuart and G. R. Screaton, A Delicate Balance between Antibody Evasion and ACE2 Affinity for Omicron BA.2.75, Cell Rep., 2023, 42, 111903, DOI:10.1016/j.celrep.2022.111903.
- Y. Cao, W. Song, L. Wang, P. Liu, C. Yue, F. Jian, Y. Yu, A. Yisimayi, P. Wang, Y. Wang, Q. Zhu, J. Deng, W. Fu, L. Yu, N. Zhang, J. Wang, T. Xiao, R. An, J. Wang, L. Liu, S. Yang, X. Niu, Q. Gu, F. Shao, X. Hao, B. Meng, R. K. Gupta, R. Jin, Y. Wang, X. S. Xie and X. Wang, Characterization of the Enhanced Infectivity and Antibody Evasion of Omicron BA.2.75, Cell Host Microbe, 2022, 30, 1527–1539.e5, DOI:10.1016/j.chom.2022.09.018.
- A. Saito, T. Tamura, J. Zahradnik, S. Deguchi, K. Tabata, Y. Anraku, I. Kimura, J. Ito, D. Yamasoba, H. Nasser, M. Toyoda, K. Nagata, K. Uriu, Y. Kosugi, S. Fujita, M. Shofa, M. Monira Begum, R. Shimizu, Y. Oda, R. Suzuki, H. Ito, N. Nao, L. Wang, M. Tsuda, K. Yoshimatsu, J. Kuramochi, S. Kita, K. Sasaki-Tabata, H. Fukuhara, K. Maenaka, Y. Yamamoto, T. Nagamoto, H. Asakura, M. Nagashima, K. Sadamasu, K. Yoshimura, T. Ueno, G. Schreiber, A. Takaori-Kondo, K. Shirakawa, H. Sawa, T. Irie, T. Hashiguchi, K. Takayama, K. Matsuno, S. Tanaka, T. Ikeda, T. Fukuhara and K. Sato, Virological Characteristics of the SARS-CoV-2 Omicron BA.2.75 Variant, Cell Host Microbe, 2022, 30, 1540–1555.e15, DOI:10.1016/j.chom.2022.10.003.
- J. E. Bowen, A. Addetia, H. V. Dang, C. Stewart, J. T. Brown, W. K. Sharkey, K. R. Sprouse, A. C. Walls, I. G. Mazzitelli, J. K. Logue, N. M. Franko, N. Czudnochowski, A. E. Powell, E. Dellota Jr., K. Ahmed, A. S. Ansari, E. Cameroni, A. Gori, A. Bandera, C. M. Posavad, J. M. Dan, Z. Zhang, D. Weiskopf, A. Sette, S. Crotty, N. T. Iqbal, D. Corti, J. Geffner, G. Snell, R. Grifantini, H. Y. Chu and D. Veesler, Omicron spike function and neutralizing activity elicited by a comprehensive panel of vaccines, Science, 2022, 377, 890–894, DOI:10.1126/science.abq0203.
- D. Ni, P. Turelli, B. Beckert, S. Nazarov, E. Uchikawa, A. Myasnikov, F. Pojer, D. Trono, H. Stahlberg and K. Lau, Cryo-EM Structures and Binding of Mouse and Human ACE2 to SARS-CoV-2 Variants of Concern Indicate That Mutations Enabling Immune Escape Could Expand Host Range, PLoS Pathog., 2023, 19, e1011206, DOI:10.1371/journal.ppat.1011206.
- I. Kimura, D. Yamasoba, T. Tamura, N. Nao, T. Suzuki, Y. Oda, S. Mitoma, J. Ito, H. Nasser, J. Zahradnik, K. Uriu, S. Fujita, Y. Kosugi, L. Wang, M. Tsuda, M. Kishimoto, H. Ito, R. Suzuki, R. Shimizu, M. M. Begum, K. Yoshimatsu, K. T. Kimura, J. Sasaki, K. Sasaki-Tabata, Y. Yamamoto, T. Nagamoto, J. Kanamune, K. Kobiyama, H. Asakura, M. Nagashima, K. Sadamasu, K. Yoshimura, K. Shirakawa, A. Takaori-Kondo, J. Kuramochi, G. Schreiber, K. J. Ishii, T. Hashiguchi, T. Ikeda, A. Saito, T. Fukuhara, S. Tanaka, K. Matsuno and K. Sato, Virological Characteristics of the SARS-CoV-2 Omicron BA.2 Subvariants, Including BA.4 and BA.5, Cell, 2022, 185, 3992–4007.e16, DOI:10.1016/j.cell.2022.09.018.
- Q. Wang, S. Iketani, Z. Li, L. Liu, Y. Guo, Y. Huang, A. D. Bowen, M. Liu, M. Wang, J. Yu, R. Valdez, A. S. Lauring, Z. Sheng, H. H. Wang, A. Gordon, L. Liu and D. D. Ho, Alarming Antibody Evasion Properties of Rising SARS-CoV-2 BQ and XBB Subvariants, Cell, 2023, 186, 279–286.e8, DOI:10.1016/j.cell.2022.12.018.
- P. Qu, J. P. Evans, J. N. Faraone, Y.-M. Zheng, C. Carlin, M. Anghelina, P. Stevens, S. Fernandez, D. Jones, G. Lozanski, A. Panchal, L. J. Saif, E. M. Oltz, K. Xu, R. J. Gumina and S.-L. Liu, Enhanced Neutralization Resistance of SARS-CoV-2 Omicron Subvariants BQ.1, BQ.1.1, BA.4.6, BF.7, and BA.2.75.2, Cell Host Microbe, 2023, 31, 9–17.e3, DOI:10.1016/j.chom.2022.11.012.
- J. Ito, R. Suzuki, K. Uriu, Y. Itakura, J. Zahradnik, K. T. Kimura, S. Deguchi, L. Wang, S. Lytras, T. Tamura, I. Kida, H. Nasser, M. Shofa, M. M. Begum, M. Tsuda, Y. Oda, T. Suzuki, J. Sasaki, K. Sasaki-Tabata, S. Fujita, K. Yoshimatsu, H. Ito, N. Nao, H. Asakura, M. Nagashima, K. Sadamasu, K. Yoshimura, Y. Yamamoto, T. Nagamoto, J. Kuramochi, G. Schreiber, S. Suzuki, M. Kato, Z. Ferdous, H. Mouri, K. Shishido, N. Misawa, I. Kimura, Y. Kosugi, P. Lin, M. Suganami, M. Chiba, R. Yoshimura, K. Yasuda, K. Iida, N. Ohsumi, A. P. Strange, D. Sauter, S. Nakagawa, J. Wu, Y. Watanabe, A. Sakamoto, N. Yasuhara, Y. Nakajima, H. Yajima, K. Shirakawa, A. Takaori-Kondo, K. Nagata, Y. Kazuma, R. Nomura, Y. Horisawa, Y. Tashiro, Y. Kawa, T. Irie, R. Kawabata, R. Shimizu, O. Takahashi, K. Ichihara, C. Motozono, M. Toyoda, T. Ueno, Y. Shibatani, T. Nishiuchi, A. Saito, K. Matsuno, K. Takayama, T. Hashiguchi, S. Tanaka, T. Fukuhara, T. Ikeda and K. Sato, Convergent Evolution of SARS-CoV-2 Omicron Subvariants Leading to the Emergence of BQ.1.1 Variant, Nat. Commun., 2023, 14, 2671, DOI:10.1038/s41467-023-38188-z.
- A. Zhu, P. Wei, M. Man, X. Liu, T. Ji, J. Chen, C. Chen, J. Huo, Y. Wang and J. Zhao, Antigenic Characterization of SARS-CoV-2 Omicron Subvariants XBB.1.5, BQ.1, BQ.1.1, BF.7 and BA.2.75.2, Signal Transduction Targeted Ther., 2023, 8, 125, DOI:10.1038/s41392-023-01391-x.
- D. Planas, T. Bruel, I. Staropoli, F. Guivel-Benhassine, F. Porrot, P. Maes, L. Grzelak, M. Prot, S. Mougari, C. Planchais, J. Puech, M. Saliba, R. Sahraoui, F. Fémy, N. Morel, J. Dufloo, R. Sanjuán, H. Mouquet, E. André, L. Hocqueloux, E. Simon-Loriere, D. Veyer, T. Prazuck, H. Péré and O. Schwartz, Resistance of Omicron Subvariants BA.2.75.2, BA.4.6, and BQ.1.1 to Neutralizing Antibodies, Nat. Commun., 2023, 14, 824, DOI:10.1038/s41467-023-36561-6.
- J. Zahradník, J. Nunvar and G. Schreiber, Perspectives: SARS-CoV-2 Spike Convergent Evolution as a Guide to Explore Adaptive Advantage, Front. Cell Infect. Microbiol., 2022, 12, 748948, DOI:10.3389/fcimb.2022.748948.
- T. N. Starr, A. J. Greaney, W. W. Hannon, A. N. Loes, K. Hauser, J. R. Dillen, E. Ferri, A. G. Farrell, B. Dadonaite, M. McCallum, K. A. Matreyek, D. Corti, D. Veesler, G. Snell and J. D. Bloom, Shifting mutational constraints in the SARS-CoV-2 receptor-binding domain during viral evolution, Science, 2022, 377, 420–424, DOI:10.1126/science.abo7896.
- A. Moulana, T. Dupic, A. M. Phillips, J. Chang, S. Nieves, A. A. Roffler, A. J. Greaney, T. N. Starr, J. D. Bloom and M. M. Desai, Compensatory Epistasis Maintains ACE2 Affinity in SARS-CoV-2 Omicron BA.1, Nat. Commun., 2022, 13, 7011, DOI:10.1038/s41467-022-34506-z.
- C. Yue, W. Song, L. Wang, F. Jian, X. Chen, F. Gao, Z. Shen, Y. Wang, X. Wang and Y. Cao, ACE2 Binding and Antibody Evasion in Enhanced Transmissibility of XBB.1.5, Lancet Infect. Dis., 2023, 23, 278–280, DOI:10.1016/s1473-3099(23)00010-5.
- M. Hoffmann, P. Arora, I. Nehlmeier, A. Kempf, A. Cossmann, S. R. Schulz, G. Morillas Ramos, L. A. Manthey, H.-M. Jäck, G. M. N. Behrens and S. Pöhlmann, Profound Neutralization Evasion and Augmented Host Cell Entry Are Hallmarks of the Fast-Spreading SARS-CoV-2 Lineage XBB.1.5, Cell. Mol. Immunol., 2023, 1–4, DOI:10.1038/s41423-023-00988-0.
- T. Tamura, J. Ito, K. Uriu, J. Zahradnik, I. Kida, Y. Anraku, H. Nasser, M. Shofa, Y. Oda, S. Lytras, N. Nao, Y. Itakura, S. Deguchi, R. Suzuki, L. Wang, M. M. Begum, S. Kita, H. Yajima, J. Sasaki, K. Sasaki-Tabata, R. Shimizu, M. Tsuda, Y. Kosugi, S. Fujita, L. Pan, D. Sauter, K. Yoshimatsu, S. Suzuki, H. Asakura, M. Nagashima, K. Sadamasu, K. Yoshimura, Y. Yamamoto, T. Nagamoto, G. Schreiber, K. Maenaka, H. Ito, N. Misawa, I. Kimura, M. Suganami, M. Chiba, R. Yoshimura, K. Yasuda, K. Iida, N. Ohsumi, A. P. Strange, O. Takahashi, K. Ichihara, Y. Shibatani, T. Nishiuchi, M. Kato, Z. Ferdous, H. Mouri, K. Shishido, H. Sawa, R. Hashimoto, Y. Watanabe, A. Sakamoto, N. Yasuhara, T. Suzuki, K. Kimura, Y. Nakajima, S. Nakagawa, J. Wu, K. Shirakawa, A. Takaori-Kondo, K. Nagata, Y. Kazuma, R. Nomura, Y. Horisawa, Y. Tashiro, Y. Kawai, T. Irie, R. Kawabata, C. Motozono, M. Toyoda, T. Ueno, T. Hashiguchi, T. Ikeda, T. Fukuhara, A. Saito, S. Tanaka, K. Matsuno, K. Takayama and K. Sato, Virological Characteristics of the SARS-CoV-2 XBB Variant Derived from Recombination of Two Omicron Subvariants, Nat. Commun., 2023, 14, 2800, DOI:10.1038/s41467-023-38435-3.
- W. Li, Z. Xu, T. Niu, Y. Xie, Z. Zhao, D. Li, Q. He, W. Sun, K. Shi, W. Guo, Z. Chang, K. Liu, Z. Fan, J. Qi and G. F. Gao, Key Mechanistic Features of the Trade-off between Antibody Escape and Host Cell Binding in the SARS-CoV-2 Omicron Variant Spike Proteins, EMBO J., 2024, 43, 1484–1498, DOI:10.1038/s44318-024-00062-z.
- A. L. Taylor and T. N. Starr, Deep Mutational Scans of XBB.1.5 and BQ.1.1 Reveal Ongoing Epistatic Drift during SARS-CoV-2 Evolution, PLoS Pathog., 2023, 19, e1011901, DOI:10.1371/journal.ppat.1011901.
- A. D. Neverov, G. Fedonin, A. Popova, D. Bykova and G. Bazykin, Coordinated Evolution at Amino Acid Sites of SARS-CoV-2 Spike, eLife, 2023, 12, e82516, DOI:10.7554/eLife.82516.
- G. A. Bazykin, F. A. Kondrashov, A. Y. Ogurtsov, S. Sunyaev and A. S. Kondrashov, Positive Selection at Sites of Multiple Amino Acid Replacements since Rat–Mouse Divergence, Nature, 2004, 429, 558–562, DOI:10.1038/nature02601.
- L. Casalino, Z. Gaieb, J. A. Goldsmith, C. K. Hjorth, A. C. Dommer, A. M. Harbison, C. A. Fogarty, E. P. Barros, B. C. Taylor and J. S. McLellan,
et al., Beyond shielding: The roles of glycans in the SARS-CoV-2 spike potein, ACS Cent. Sci., 2020, 6, 1722–1734 CrossRef CAS PubMed.
- T. Sztain, S. H. Ahn, A. T. Bogetti, L. Casalino, J. A. Goldsmith, E. Seitz, R. S. McCool, F. L. Kearns, F. Acosta-Reyes, S. Maji, G. Mashayekhi, J. A. McCammon, A. Ourmazd, J. Frank, J. S. McLellan, L. T. Chong and R. E. Amaro, A glycan gate controls the opening of the SARS-CoV-2 spike protein, Nat. Chem., 2021, 13, 963–968, DOI:10.1038/s41557-021-00758-3.
- Y. T. Pang, A. Acharya, D. L. Lynch, A. Pavlova and J. C. Gumbart, SARS-CoV-2 Spike Opening Dynamics and Energetics Reveal the Individual Roles of Glycans and Their Collective Impact, Commun. Biol., 2022, 5, 1170, DOI:10.1038/s42003-022-04138-6.
- M. I. Zimmerman, J. R. Porter, M. D. Ward, S. Singh, N. Vithani, A. Meller, U. L. Mallimadugula, C. E. Kuhn, J. H. Borowsky, R. P. Wiewiora, M. F. D. Hurley, A. M. Harbison, C. A. Fogarty, J. E. Coffland, E. Fadda, V. A. Voelz, J. D. Chodera and G. R. Bowman, SARS-CoV-2 simulations go exascale to predict dramatic spike opening and cryptic pockets across the proteome, Nat. Chem., 2021, 13, 651–659, DOI:10.1038/s41557-021-00707-0.
- H. M. Dokainish, S. Re, T. Mori, C. Kobayashi, J. Jung and Y. Sugita, The inherent flexibility of receptor binding domains in SARS-CoV-2 spike protein, eLife, 2022, 11, e75720, DOI:10.7554/eLife.75720.
- J. Singh, S. Vashishtha and B. Kundu, Spike Protein Mutation-Induced Changes in the Kinetic and Thermodynamic Behavior of Its Receptor Binding Domains Explain Their Higher Propensity to Attain Open States in SARS-CoV-2 Variants of Concern, ACS Cent. Sci., 2023, 9(10), 1894–1904, DOI:10.1021/acscentsci.3c00810.
- M. A. Díaz-Salinas, Q. Li, M. Ejemel, L. Yurkovetskiy, J. Luban, K. Shen, Y. Wang and J. B. Munro, Conformational dynamics and allosteric modulation of the SARS-CoV-2 spike, eLife, 2022, 11, e75433, DOI:10.7554/eLife.75433.
- G. M. Verkhivker and L. Di Paola, Integrated Biophysical Modeling of the SARS-CoV-2 Spike Protein Binding and Allosteric Interactions with Antibodies, J. Phys. Chem. B, 2021, 125, 4596–4619, DOI:10.1021/acs.jpcb.1c00395.
- G. M. Verkhivker, S. Agajanian, D. Y. Oztas and G. Gupta, Comparative Perturbation-Based Modeling of the SARS-CoV-2 Spike Protein Binding with Host Receptor and Neutralizing Antibodies: Structurally Adaptable Allosteric Communication Hotspots Define Spike Sites Targeted by Global Circulating Mutations, Biochemistry, 2021, 60, 1459–1484, DOI:10.1021/acs.biochem.1c00139.
- G. M. Verkhivker, S. Agajanian, D. Y. Oztas and G. Gupta, Dynamic Profiling of Binding and Allosteric Propensities of the SARS-CoV-2 Spike Protein with Different Classes of Antibodies: Mutational and Perturbation-Based Scanning Reveals the Allosteric Duality of Functionally Adaptable Hotspots, J. Chem. Theory Comput., 2021, 17, 4578–4598, DOI:10.1021/acs.jctc.1c00372.
- G. Verkhivker, M. Alshahrani and G. Gupta, Balancing Functional Tradeoffs between Protein Stability and ACE2 Binding in the SARS-CoV-2 Omicron BA.2, BA.2.75 and XBB Lineages: Dynamics-Based Network Models Reveal Epistatic Effects Modulating Compensatory Dynamic and Energetic Changes, Viruses, 2023, 15, 1143, DOI:10.3390/v15051143.
- G. Verkhivker, M. Alshahrani, G. Gupta, S. Xiao and P. Tao, Probing Conformational Landscapes of Binding and Allostery in the SARS-CoV-2 Omicron Variant Complexes Using Microsecond Atomistic Simulations and Perturbation-Based Profiling Approaches: Hidden Role of Omicron Mutations as Modulators of Allosteric Signaling and Epistatic Relationships, Phys. Chem. Chem. Phys., 2023, 25, 21245–21266, 10.1039/d3cp02042h.
- S. Xiao, M. Alshahrani, G. Gupta, P. Tao and G. Verkhivker, Markov State Models and Perturbation-Based Approaches Reveal Distinct Dynamic Signatures and Hidden Allosteric Pockets in the Emerging SARS-Cov-2 Spike Omicron Variant Complexes with the Host Receptor: The Interplay of Dynamics and Convergent Evolution Modulates Allostery and Functional Mechanisms, J. Chem. Inf. Model., 2023, 63, 5272–5296, DOI:10.1021/acs.jcim.3c00778.
- G. Verkhivker, M. Alshahrani and G. Gupta, Exploring Conformational Landscapes and Cryptic Binding Pockets in Distinct Functional States of the SARS-CoV-2 Omicron BA.1 and BA.2 Trimers: Mutation-Induced Modulation of Protein Dynamics and Network-Guided Prediction of Variant-Specific Allosteric Binding Sites, Viruses, 2023, 15, 2009, DOI:10.3390/v15102009.
- M. Alshahrani, G. Gupta, S. Xiao, P. Tao and G. Verkhivker, Comparative Analysis of Conformational Dynamics and Systematic Characterization of Cryptic Pockets in the SARS-CoV-2 Omicron BA.2, BA.2.75 and XBB.1 Spike Complexes with the ACE2 Host Receptor: Confluence of Binding and Structural Plasticity in Mediating Networks of Conserved Allosteric Sites, Viruses, 2023, 15, 2073, DOI:10.3390/v15102073.
- J. Jumper, R. Evans, A. Pritzel, T. Green, M. Figurnov, O. Ronneberger, K. Tunyasuvunakool, R. Bates, A. Žídek, A. Potapenko, A. Bridgland, C. Meyer, S. A. A. Kohl, A. J. Ballard, A. Cowie, B. Romera-Paredes, S. Nikolov, R. Jain, J. Adler, T. Back, S. Petersen, D. Reiman, E. Clancy, M. Zielinski, M. Steinegger, M. Pacholska, T. Berghammer, S. Bodenstein, D. Silver, O. Vinyals, A. W. Senior, K. Kavukcuoglu, P. Kohli and D. Hassabis, Highly Accurate Protein Structure Prediction with AlphaFold, Nature, 2021, 596, 583–589, DOI:10.1038/s41586-021-03819-2.
- K. Tunyasuvunakool, J. Adler, Z. Wu, T. Green, M. Zielinski, A. Žídek, A. Bridgland, A. Cowie, C. Meyer, A. Laydon, S. Velankar, G. J. Kleywegt, A. Bateman, R. Evans, A. Pritzel, M. Figurnov, O. Ronneberger, R. Bates, S. A. A. Kohl, A. Potapenko, A. J. Ballard, B. Romera-Paredes, S. Nikolov, R. Jain, E. Clancy, D. Reiman, S. Petersen, A. W. Senior, K. Kavukcuoglu, E. Birney, P. Kohli, J. Jumper and D. Hassabis, Highly Accurate Protein Structure Prediction for the Human Proteome, Nature, 2021, 596, 590–596, DOI:10.1038/s41586-021-03828-1.
- T. C. Terwilliger, D. Liebschner, T. I. Croll, C. J. Williams, A. J. McCoy, B. K. Poon, P. V. Afonine, R. D. Oeffner, J. S. Richardson, R. J. Read and P. D. Adams, AlphaFold Predictions Are Valuable Hypotheses and Accelerate but Do Not Replace Experimental Structure Determination, Nat. Methods, 2023, 21, 110–116, DOI:10.1038/s41592-023-02087-4.
- D. Del Alamo, D. Sala, H. S. Mchaourab and J. Meiler, Sampling alternative conformational states of transporters and receptors with AlphaFold2, eLife, 2022, 11, e75751, DOI:10.7554/eLife.75751.
- R. A. Stein and H. S. Mchaourab, SPEACH_AF: Sampling Protein Ensembles and Conformational Heterogeneity with Alphafold2, PLoS Comput. Biol., 2022, 18, e1010483, DOI:10.1371/journal.pcbi.1010483.
- T. Saldaño, N. Escobedo, J. Marchetti, D. J. Zea, J. Mac Donagh, A. J. Velez Rueda, E. Gonik, A. García Melani, J. Novomisky Nechcoff, M. N. Salas, T. Peters, N. Demitroff, S. Fernandez Alberti, N. Palopoli, M. S. Fornasari and G. Parisi, Impact of Protein Conformational Diversity on AlphaFold Predictions, Bioinformatics, 2022, 38, 2742–2748, DOI:10.1093/bioinformatics/btac202.
- D. Chakravarty and L. L. Porter, AlphaFold2 fails to predict protein fold switching, Protein Sci., 2022, 31, e4353, DOI:10.1002/pro.4353.
-
D. Chakravarty, J. W. Schafer, E. A. Chen, J. R. Thole and L. L. Porter, AlphaFold2 Has More to Learn about Protein Energy Landscapes, bioRxiv, 2023, preprint DOI:10.1101/2023.12.12.571380.
- D. Sala, P. W. Hildebrand and J. Meiler, Biasing AlphaFold2 to Predict GPCRs and Kinases with User-Defined Functional or Structural Properties, Front. Mol. Biosci., 2023, 10, 1121962, DOI:10.3389/fmolb.2023.1121962.
- H. K. Wayment-Steele, S. Ovchinnikov, L. Colwell and D. Kern, Predicting multiple conformations via sequence clustering and AlphaFold2, Nature, 2024, 625, 832–839, DOI:10.1038/s41586-023-06832-9.
- M. Mirdita, K. Schütze, Y. Moriwaki, L. Heo, S. Ovchinnikov and M. Steinegger, ColabFold: Making Protein Folding Accessible to All, Nat. Methods, 2022, 19, 679–682, DOI:10.1038/s41592-022-01488-1.
- M. Steinegger and J. Söding, MMseqs2 Enables Sensitive Protein Sequence Searching for the Analysis of Massive Data Sets, Nat. Biotechnol., 2017, 35, 1026–1028, DOI:10.1038/nbt.3988.
-
N. Raisinghani, M. Alshahrani, G. Gupta, H. Tian, S. Xiao, P. Tao and G. Verkhivker, Interpretable Atomistic Prediction and Functional Analysis of Conformational Ensembles and Allosteric States in Protein Kinases Using AlphaFold2 Adaptation with Randomized Sequence Scanning and Local Frustration Profiling, bioRxiv, 2024, preprint DOI:10.1101/2024.02.15.580591.
- Y. Zhang, TM-Align: A Protein Structure Alignment Algorithm Based on the TM-Score, Nucleic Acids Res., 2005, 33, 2302–2309, DOI:10.1093/nar/gki524.
- M. L. Hekkelman, T. A. Te Beek, S. R. Pettifer, D. Thorne, T. K. Attwood and G. Vriend, WIWS: A protein structure bioinformatics web service collection, Nucleic Acids Res., 2010, 38, W719–W723, DOI:10.1093/nar/gkq453.
- N. Fernandez-Fuentes, J. Zhai and A. Fiser, ArchPRED: A template based loop structure prediction server, Nucleic Acids Res., 2006, 34, W173–W176, DOI:10.1093/nar/gkl113.
- G. G. Krivov, M. V. Shapovalov and R. L. Dunbrack Jr., Improved prediction of protein side-chain conformations with SCWRL4, Proteins, 2009, 77, 778–795, DOI:10.1002/prot.22488.
- M. H. Olsson, C. R. Søndergaard, M. Rostkowski and J. H. Jensen, PROPKA3: consistent treatment of internal and surface residues in empirical pKa predictions, J. Chem. Theory Comput., 2011, 7, 525–537, DOI:10.1021/ct100578z.
- D. Bhattacharya and J. Cheng, 3Drefine: Consistent Protein Structure Refinement by Optimizing Hydrogen Bonding Network and Atomic-Level Energy Minimization, Proteins, 2013, 81, 119–131, DOI:10.1002/prot.24167.
- D. Bhattacharya, J. Nowotny, R. Cao and J. Cheng, 3Drefine: An Interactive Web Server for Efficient Protein Structure Refinement, Nucleic Acids Res., 2016, 44, W406–W409, DOI:10.1093/nar/gkw336.
- J. C. Phillips, D. J. Hardy, J. D. C. Maia, J. E. Stone, J. V. Ribeiro, R. C. Bernardi, R. Buch, G. Fiorin, J. Hénin and W. Jiang,
et al., Scalable Molecular Dynamics on CPU and GPU Architectures with NAMD, J. Chem. Phys., 2020, 153, 044130, DOI:10.1063/5.0014475.
- J. Huang, S. Rauscher, G. Nawrocki, T. Ran, M. Feig, B. L. de Groot, H. Grubmüller and A. D. MacKerell Jr., CHARMM36m: An improved force field for folded and intrinsically disordered proteins, Nat. Methods, 2017, 14, 71–73, DOI:10.1038/nmeth.4067.
- H. S. Fernandes, S. F. Sousa and N. M. F. S. A. Cerqueira, VMD Store-A VMD Plugin to Browse, Discover, and Install VMD Extensions, J. Chem. Inf. Model., 2019, 59, 4519–4523, DOI:10.1021/acs.jcim.9b00739.
- S. Jo, T. Kim, V. G. Iyer and W. Im, CHARMM-GUI: A Web-based Graphical User Interface for CHARMM, J. Comput. Chem., 2008, 29, 1859–1865, DOI:10.1002/jcc.20945.
- J. Lee, X. Cheng, J. M. Swails, M. S. Yeom, P. K. Eastman, J. A. Lemkul, S. Wei, J. Buckner, J. C. Jeong, Y. Qi, S. Jo, V. S. Pande, D. A. Case, C. L. Brooks III, A. D. MacKerell Jr., J. B. Klauda and W. Im, CHARMM-GUI Input Generator for NAMD, GROMACS, AMBER, OpenMM, and CHARMM/OpenMM Simulations Using the CHARMM36 Additive Force Field, J. Chem. Theory Comput., 2016, 12, 405–413, DOI:10.1021/acs.jctc.5b00935.
- W. L. Jorgensen, J. Chandrasekhar, J. D. Madura, R. W. Impey and M. L. Klein, Comparison of Simple Potential Functions for Simulating Liquid Water, J. Chem. Phys., 1983, 79, 926–935, DOI:10.1063/1.445869.
- G. A. Ross, A. S. Rustenburg, P. B. Grinaway, J. Fass and J. D. Chodera, Biomolecular Simulations under Realistic Macroscopic Salt Conditions, J. Phys. Chem. B, 2018, 122, 5466–5486, DOI:10.1021/acs.jpcb.7b11734.
- M. Di Pierro, R. Elber and B. Leimkuhler, A Stochastic Algorithm for the Isobaric-Isothermal Ensemble with Ewald Summations for All Long Range Forces, J. Chem. Theory Comput., 2015, 11, 5624–5637, DOI:10.1021/acs.jctc.5b00648.
- G. J. Martyna, D. J. Tobias and M. L. Klein, Constant pressure molecular dynamics algorithms, J. Chem. Phys., 1994, 101, 4177–4189, DOI:10.1063/1.467468.
- S. E. Feller, Y. Zhang, R. W. Pastor and B. R. Brooks, Constant pressure molecular dynamics simulation: The Langevin piston method, J. Chem. Phys., 1995, 103, 4613–4621, DOI:10.1063/1.470648.
- R. L. Davidchack, R. Handel and M. V. Tretyakov, Langevin thermostat for rigid body dynamics, J. Chem. Phys., 2009, 130, 234101, DOI:10.1063/1.3149788.
- J. Srinivasan, T. E. Cheatham, P. Cieplak, P. A. Kollman and D. A. Case, Continuum Solvent Studies of the Stability of DNA, RNA, and Phosphoramidate−DNA Helices, J. Am. Chem. Soc., 1998, 120, 9401–9409, DOI:10.1021/ja981844.
- P. A. Kollman, I. Massova, C. Reyes, B. Kuhn, S. Huo, L. Chong, M. Lee, T. Lee, Y. Duan, W. Wang, O. Donini, P. Cieplak, J. Srinivasan, D. A. Case and T. E. Cheatham, Calculating Structures and Free Energies of Complex Molecules:
Combining Molecular Mechanics and Continuum Models, Acc. Chem. Res., 2000, 33, 889–897, DOI:10.1021/ar000033j.
- T. Hou, J. Wang, Y. Li and W. Wang, Assessing the Performance of the MM/PBSA and MM/GBSA Methods. 1. The Accuracy of Binding Free Energy Calculations Based on Molecular Dynamics Simulations, J. Chem. Inf. Model., 2011, 51, 69–82, DOI:10.1021/ci100275a.
- H. Sun, Y. Li, S. Tian, L. Xu and T. Hou, Assessing the Performance of MM/PBSA and MM/GBSA Methods. 4. Accuracies of MM/PBSA and MM/GBSA Methodologies Evaluated by Various Simulation Protocols Using PDBbind Data Set, Phys. Chem. Chem. Phys., 2014, 16, 16719–16729, 10.1039/c4cp01388c.
- G. Weng, E. Wang, Z. Wang, H. Liu, F. Zhu, D. Li and T. Hou, HawkDock: A Web Server to Predict and Analyze the Protein–Protein Complex Based on Computational Docking and MM/GBSA, Nucleic Acids Res., 2019, 47, W322–W330, DOI:10.1093/nar/gkz397.
- J. Hadfield, C. Megill, S. M. Bell, J. Huddleston, B. Potter, C. Callender, P. Sagulenko, T. Bedford and R. A. Neher, Nextstrain: Real-Time Tracking of Pathogen Evolution, Bioinformatics, 2018, 34, 4121–4123, DOI:10.1093/bioinformatics/bty407.
- J. K. Williams, B. Wang, A. Sam, C. L. Hoop, D. A. Case and J. Baum, Molecular Dynamics Analysis of a Flexible Loop at the Binding Interface of the SARS-CoV-2 spike protein receptor-binding domain, Proteins, 2022, 90, 1044–1053, DOI:10.1002/prot.26208.
- S. M. Costello, S. R. Shoemaker, H. T. Hobbs, A. W. Nguyen, C.-L. Hsieh, J. A. Maynard, J. S. McLellan, J. E. Pak and S. Marqusee, The SARS-CoV-2 Spike Reversibly Samples an Open-Trimer Conformation Exposing Novel Epitopes, Nat. Struct. Mol. Biol., 2022, 29, 229–238, DOI:10.1038/s41594-022-00735-5.
- V. Calvaresi, A. G. Wrobel, J. Toporowska, D. Hammerschmid, K. J. Doores, R. T. Bradshaw, R. B. Parsons, D. J. Benton, C. Roustan, E. Reading, M. H. Malim, S. J. Gamblin and A. Politis, Structural Dynamics in the Evolution of SARS-CoV-2 Spike Glycoprotein, Nat. Commun., 2023, 14, 1421, DOI:10.1038/s41467-023-36745-0.
- S. M. Braet, T. S. Buckley, V. Venkatakrishnan, K.-M. A. Dam, P. J. Bjorkman and G. S. Anand, Timeline of Changes in Spike Conformational Dynamics in Emergent SARS-CoV-2 Variants Reveal Progressive Stabilization of Trimer Stalk with Altered NTD Dynamics, eLife, 2023, 12, e82584, DOI:10.7554/eLife.82584.
- F. Jian, L. Feng, S. Yang, Y. Yu, L. Wang, W. Song, A. Yisimayi, X. Chen, Y. Xu, P. Wang, L. Yu, J. Wang, L. Liu, X. Niu, J. Wang, T. Xiao, R. An, Y. Wang, Q. Gu, F. Shao, R. Jin, Z. Shen, Y. Wang, X. Wang and Y. Cao, Convergent Evolution of SARS-CoV-2 XBB Lineages on Receptor-Binding Domain 455-456 Synergistically Enhances Antibody Evasion and ACE2 Binding, PLoS Pathog., 2023, 19, e1011868, DOI:10.1371/journal.ppat.1011868.
- Y. Cao, F. Jian, J. Wang, Y. Yu, W. Song, A. Yisimayi, J. Wang, R. An, X. Chen and N. Zhang,
et al., Imprinted SARS-CoV-2 Humoral Immunity Induces Convergent Omicron RBD Evolution, Nature, 2023, 614, 521–529, DOI:10.1038/s41586-022-05644-7.
- D. Focosi, R. Quiroga, S. McConnell, M. C. Johnson and A. Casadevall, Convergent Evolution in SARS-CoV-2 Spike Creates a Variant Soup from Which New COVID-19 Waves Emerge, Int. J. Mol. Sci., 2023, 24, 2264, DOI:10.3390/ijms24032264.
- H. H. Gan, A. Twaddle, B. Marchand and K. C. Gunsalus, Structural Modeling of the SARS-CoV-2 Spike/Human ACE2 Complex Interface can Identify High-Affinity Variants Associated with Increased Transmissibility, J. Mol. Biol., 2021, 433, 167051, DOI:10.1016/j.jmb.2021.167051.
- H. H. Gan, J. Zinno, F. Piano and K. C. Gunsalus, Omicron Spike Protein Has a Positive Electrostatic Surface That Promotes ACE2 Recognition and Antibody Escape, Front. Virol., 2022, 2, DOI:10.3389/fviro.2022.894531.
- F. L. Barroso da Silva, C. C. Giron and A. Laaksonen, Electrostatic Features for the Receptor Binding Domain of SARS-COV-2 Wildtype and Its Variants. Compass to the Severity of the Future Variants with the Charge-Rule, J. Phys. Chem. B, 2022, 126, 6835–6852, DOI:10.1021/acs.jpcb.2c04225; S. H. Hristova and A. M. Zhivkov, Omicron Coronavirus: pH-Dependent Electrostatic Potential and Energy of Association of Spike Protein to ACE2 Receptor, Viruses, 2023, 15, 1752, DOI:10.3390/v15081752.
- F. Scarpa, D. Sanna, D. Benvenuto, A. Borsetti, I. Azzena, M. Casu, P. L. Fiori, M. Giovanetti, A. Maruotti, G. Ceccarelli, A. Caruso, F. Caccuri, R. Cauda, A. Cassone, S. Pascarella and M. Ciccozzi, Genetic and Structural Data on the SARS-CoV-2 Omicron BQ.1 Variant Reveal Its Low Potential for Epidemiological Expansion, Int. J. Mol. Sci., 2022, 23, 15264, DOI:10.3390/ijms232315264.
- A. Addetia, L. Piccoli, J. B. Case, Y.-J. Park, M. Beltramello, B. Guarino, H. Dang, G. D. de Melo, D. Pinto, K. Sprouse, S. M. Scheaffer, J. Bassi, C. Silacci-Fregni, F. Muoio, M. Dini, L. Vincenzetti, R. Acosta, D. Johnson, S. Subramanian, C. Saliba, M. Giurdanella, G. Lombardo, G. Leoni, K. Culap, C. McAlister, A. Rajesh, E. Dellota Jr., J. Zhou, N. Farhat, D. Bohan, J. Noack, A. Chen, F. A. Lempp, J. Quispe, L. Kergoat, F. Larrous, E. Cameroni, B. Whitener, O. Giannini, P. Cippà, A. Ceschi, P. Ferrari, A. Franzetti-Pellanda, M. Biggiogero, C. Garzoni, S. Zappi, L. Bernasconi, M. J. Kim, L. E. Rosen, G. Schnell, N. Czudnochowski, F. Benigni, N. Franko, J. K. Logue, C. Yoshiyama, C. Stewart, H. Chu, H. Bourhy, M. A. Schmid, L. A. Purcell, G. Snell, A. Lanzavecchia, M. S. Diamond, D. Corti and D. Veesler, Neutralization, Effector Function and Immune Imprinting of Omicron Variants, Nature, 2023, 621, 592–601, DOI:10.1038/s41586-023-06487-6.
- S. M.-C. Gobeil, K. Janowska, S. McDowell, K. Mansouri, R. Parks, V. Stalls, M. F. Kopp, K. Manne, D. Li, K. Wiehe, K. O. Saunders, R. J. Edwards, B. Korber, B. F. Haynes, R. Henderson and P. Acharya, Effect of Natural Mutations of SARS-CoV-2 on Spike Structure, Conformation, and Antigenicity, Science, 2021, 373, eabi6226, DOI:10.1126/science.abi6226.
- T. N. Starr, A. J. Greaney, S. K. Hilton, D. Ellis, K. H. D. Crawford, A. S. Dingens, M. J. Navarro, J. E. Bowen, M. A. Tortorici, A. C. Walls, N. P. King, D. Veesler and J. D. Bloom, Deep Mutational Scanning of SARS-CoV-2 Receptor Binding Domain Reveals Constraints on Folding and ACE2 Binding, Cell, 2020, 182, 1295–1310.e20, DOI:10.1016/j.cell.2020.08.012.
- T. N. Starr, A. J. Greaney, C. M. Stewart, A. C. Walls, W. W. Hannon, D. Veesler and J. D. Bloom, Deep Mutational Scans for ACE2 Binding, RBD Expression, and Antibody Escape in the SARS-CoV-2 Omicron BA.1 and BA.2 Receptor-Binding Domains, PLoS Pathog., 2022, 18, e1010951, DOI:10.1371/journal.ppat.1010951.
- B. Dadonaite, K. H. D. Crawford, C. E. Radford, A. G. Farrell, T. C. Yu, W. W. Hannon, P. Zhou, R. Andrabi, D. R. Burton, L. Liu, D. D. Ho, H. Y. Chu, R. A. Neher and J. D. Bloom, A Pseudovirus System Enables Deep Mutational Scanning of the Full SARS-CoV-2 Spike, Cell, 2023, 186, 1263–1278.e20, DOI:10.1016/j.cell.2023.02.001.
|
This journal is © the Owner Societies 2024 |
Click here to see how this site uses Cookies. View our privacy policy here.