DOI:
10.1039/D4DD00059E
(Opinion)
Digital Discovery, 2024,
3, 1273-1279
Autonomous laboratories for accelerated materials discovery: a community survey and practical insights†
Received
27th February 2024
, Accepted 30th May 2024
First published on 31st May 2024
Abstract
What are researchers' motivations and challenges related to automation and autonomy in materials science laboratories? Our survey on this topic received 102 responses from researchers across a variety of institutions and in a variety of roles. Accelerated discovery was a clear theme in the responses, and another theme was concern about the role of human researchers. Survey respondents shared a variety of use cases targeting accelerated materials discovery, including examples where partial automation is preferred over full self-driving laboratories. Building on the observed patterns of researcher priorities and needs, we propose a framework for levels of laboratory autonomy from non-automated (L0) to fully autonomous (L5).
1 Introduction
In recent years, automation and robotics have become increasingly accessible for materials science labs, with researchers in this area motivated by the promise of experimental innovation and accelerated materials discovery. Researchers are working to implement both automation of experimental processes, and also autonomy in labs. (Lab autonomy refers to the automation and integration of experimental processes and analyses, as well as interpretation, decision-making, and planning.) To reach this point, the implementation of laboratory automation and autonomy is often a research project with significant upfront costs in terms of time and money. However, we are now entering a stage where these new capabilities are applied in experimental labs where primary research targets extend beyond optimization to scientific knowledge or materials discovery for emergent applications. As a result, different use cases and needs are emerging, which may differ from patterns seen when designing self-driving labs.1–3
At Toyota Research Institute, we work with a variety of academic and national labs, as well as other industry researchers, and we recognize the diverse set of opportunities and challenges that laboratory automation and autonomy presents to our collaborators.4 To extend our understanding beyond our consortium to the wider materials discovery community, we decided to synthesize the thoughts of researchers working in labs with varying levels of autonomy and different research priorities: their motivations, sentiments, and perceived challenges around lab automation and autonomy. Our survey in spring 2023 was advertised by email and social media to the general public. It garnered 102 responses from researchers representing a cross-section of the materials discovery community (Fig. 1). We note that this survey has provided us with wider insights than previously available, but should be considered limited scope, with results potentially biased due to the methods of participant recruitment. Details of survey design, the full list of survey questions, and anonymized responses are available in the ESI.†
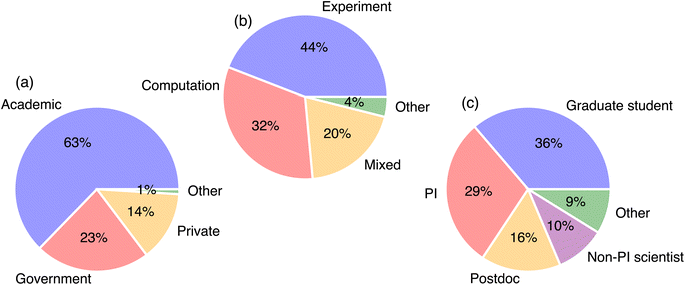 |
| Fig. 1 Demographics of 102 survey respondents, according to their (a) institution type, (b) category of research activities, and (c) role. | |
In this article, we share the outcomes of the survey as they relate to two main themes: accelerating materials discovery (Section 2) and the role of human researchers in the lab (Section 3). In each section, we provide additional context drawn from interviews with materials science, automation, and autonomy experts, which were conducted to guide the design of the survey. In Section 4, we organize researchers' stated priorities into a framework for levels of laboratory autonomy, ranging from L0 to L5. This framework, which provides finer distinctions between the extremes of not-automated and fully autonomous labs, provides a shorthand for lab capabilities that can frame future discussions of lab autonomy in the context of materials discovery. Finally, in Section 5, we recommend community-wide efforts that we believe will have a multiplicative impact on accelerating materials discovery.
2 Accelerating discovery
Within our group and across the community, accelerated discovery or accelerated research is often cited as a primary motivation for lab automation and autonomy. In the survey, we examined this premise by asking respondents to rank their motivations to automate. Our survey provided options of efficiency, creation of new capabilities, dataset generation, reproducibility, researcher happiness, and researcher safety. The overwhelming top-ranked motivation was efficiency (Fig. 2), which is most directly linked to research acceleration. On the other hand, researcher happiness and safety were the least important motivators to automate, and also have the least direct connection with research acceleration. We note that respondents were given the option to list motivations beyond those provided above, but very few did. In addition, the ranking of motivation remained fairly consistent across levels of experience, with the exception being that those most experienced in working with automation and autonomy were more interested in the creation of new capabilities over efficiency.
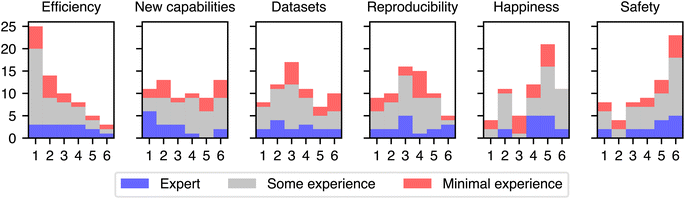 |
| Fig. 2 Experimentalists' motivations to automate (65 total responses). Histograms show the number of respondents who chose each rank for each motivation, with 1 being the most important and 6 being the least. Colors indicate the respondents' self-reported level of experience with lab automation. | |
Separate survey questions addressed acceleration by asking experimental researchers about their in-lab rate-limiting steps (RLS) for their research, and whether they would like them automated. The diversity of responses (Fig. 3) shows how the automation needed to accelerate research depends on each lab's unique workflows. Our survey respondents were interested in accelerating activities such as battery cell fabrication, polymer synthesis, thin film measurements, instrument setup, and more. A majority of researchers wanted to automate the RLS in their current lab workflow (64%). Those that did not want to automate their RLS cited reasons around domain expertise and human factors (the focus of the following section) or significant research bottlenecks outside of the lab. A third category of reasons to not automate the RLS centered around the challenge of automation. For instance, tasks straightforward for humans, like material preparation, could be challenging for robots.
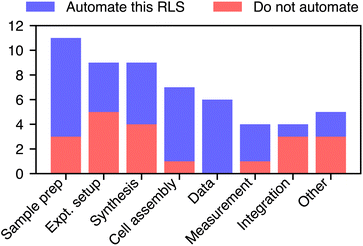 |
| Fig. 3 Number of respondents reporting rate-limiting steps (RLS) in each category, and whether they want their RLS automated (55 total responses). RLS categories include “Sample prep” for processing of materials for experiment, “Expt. setup” for instrument setup, material transfers, and preparing stock reagents, “Synthesis” for synthesis of a variety of materials, “Cell assembly” for assembly and troubleshooting of devices, “Data” for data analysis, interpretation, and management, “Measurement” for characterization and property measurements, and “Integration” for the development of software and hardware frameworks and interfaces. | |
These survey responses, our interviews with lab automation experts, and interactions with our research partners have surfaced the following common concerns of project leaders planning to automate their labs to accelerate discovery.
Efficacy vs. cost
To implement the right type of automation to accelerate discovery, project leaders must consider the technical challenge and maintenance costs of automation. Sometimes convenient but non-critical steps may be automated, but this may not provide the desired research acceleration. For example, materials from high-throughput synthesis might be bottlenecked by low-throughput characterization steps before a discovery is confirmed or an optimization plan can be created. On the other hand, automating and accelerating the RLS of workflows may require instrumentation development with careful calibration against existing equipment standards and compatibility with materials and workflows in the lab, sometimes becoming an insurmountable time sink.
Flexibility vs. robustness
From expert interviews, we learned that individual laboratory operations, such as heating and mixing, are often not complicated. However, linking separate automated steps together is challenging. Commercial workstations, such as those from Chemspeed Technologies, provide integrated automation platforms to bridge the gaps between multiple experimental steps.5,6 While standardization and robustness are enhanced by highly integrated systems, they may restrict agility necessary for scientific workflows. Therefore, flexible and modular automation has gained popularity in labs developing customized workflows. These setups often involve a robotic arm with a set of loosely integrated formulation and characterization units that can be removed or added depending on project needs, as exemplified by the Universal cobots and Opentrons modules. The choice of automation platform is influenced by the roles that researchers envision robots play in accelerating their workflows.
Throughput vs. knowledge generation
It is crucial to differentiate between data collection and knowledge generation. To maximize knowledge generation, research workflows must balance the throughput of data collection with the frequency of decision-making. This is particularly the case with “iterative learning” workflows,7–10 where batches of experiments alternate with the analyses that guide subsequent experiments. When the time and monetary cost of each experiment justifies a premium on experiment design and candidate selection, single or small batches of experiments alternating with frequent feedback would be ideal. When large amounts of data are needed to provide meaningful feedback, high throughput via parallelization would be beneficial. “One-shot” machine learning11–13 similarly often can benefit from high-throughput experiments, as all experiments are completed before advanced data evaluation. It is important to recognize that each learning mode has its own advantages and limitations relating to the cost and accuracy of decisions in the workflow, and whether these decisions are made by humans or by artificial intelligence.
3 Role of humans
In the survey, we investigated the role of human researchers in the lab by asking which tasks respondents would not want to automate. Although 26% were comfortable with automation of their full scientific workflow, the remaining respondents believed that certain tasks, such as idea generation (hypothesis generation and defining objectives), data interpretation, experimental design, and on-the-fly interactions with complex experiments require flexibility, creativity, and domain knowledge and therefore should not be automated (Fig. 4, top). Similar concerns were reflected in responses to a question about negativity around automation (Fig. 4, bottom).
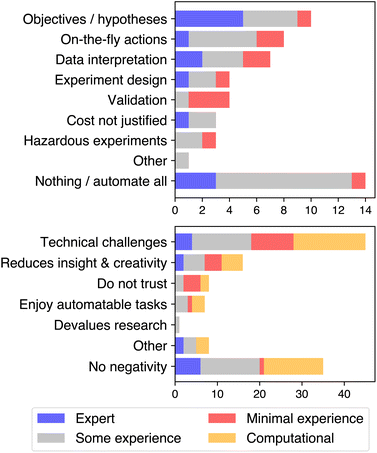 |
| Fig. 4 Categories of research tasks that experimentalists do not want to automate (top, 54 total responses), and the reasons why researchers may feel negativity around automation (bottom, 91 total responses, multiple responses could be chosen), partitioned by whether the respondent works primarily in computation or theory (“computational”), and for experimentalists, their self-reported experience with lab automation. | |
In certain areas, self-reported experts in laboratory automation had different perspectives from non-experts on how human researchers should interact with automation. Experts did not have concerns around trusting automation, in contrast to non-experts, who also found it important to have alternate non-automated avenues of validating automated workflows. In addition, some non-experts described their preference for keeping humans involved in experiment execution—whether this was to incorporate human decisions and insights during on-the-fly interactions in specific experiments, or to maintain their own enjoyment of performing automatable tasks.
The survey responses and interviews revealed that, in the age of AI and robotics, how scientists spend their time for laboratory research will be revised, but we are still at an early stage of this paradigm shift. The primary concerns of researchers in working with autonomous labs include:
Encoding human expertise and intuition
While autonomous systems can excel in optimization tasks, optimization alone does not always lead to new scientific discoveries. Breakthroughs often arise from creative approaches, intuition, observation and action on unexpected phenomena, or thinking outside the box, all which are built on human expertise and experience, and are difficult to automate. There's a growing emphasis on algorithms that enable a transfer of human knowledge to robots, prompting many scientists to learn computer science and build systems with high automation levels.14 We expect combining human and artificial intelligence to enable robotics to execute and test new scientific hypotheses to be a central focus moving forward.
Lack of trust in full autonomy
Our survey responses indicated a preference for retaining the human element in ideation, hypothesis generation, as well as some on-the-fly experiment observations, decision-making, and adjustments. At present, scientists remain responsible for scientific conclusions, even when experiments are performed by robots. There are concerns about AI drawing inaccurate scientific conclusions or failing to identify and act on novel phenomena, a concern that is addressed in designs with humans “on-the-loop”, with humans acting as supervisors overseeing the automated workflow.
Alleviating dull, dirty, and dangerous tasks
In conversations with industry researchers, automation has been seen as an avenue to improve safety in workflows. To our surprise, our survey revealed the wider materials science community's preference for not automating tasks that are perceived as unsafe. One key concern expressed was that in most laboratory settings, humans and robots share the same workspace. In the case of hazard exposure, a robot may not have been trained to recognize or mitigate the issue, posing a risk to humans nearby. Creating a safe work environment that accounts for the intricacies of the workflows becomes a crucial design consideration for automated and autonomous labs. In addition, although the goal of automation is to eliminate tedious or boring tasks traditionally carried out by humans (often talented graduate students), many students may also perceive the monitoring and maintenance robots to be as dull, or even more so, compared to bench chemistry tasks.
4 Levels of laboratory autonomy
The above survey responses highlight that researchers' desired level of laboratory autonomy is not all-or-nothing. Depending on the research task, as well as the researcher performing the task, the desired level of autonomy will lie along a spectrum. We partition automatable activities into five broad categories: (1) process execution, (2) data analysis, (3) data interpretation, (4) decision making, and (5) communication in workflows, as defined below.
Process execution is the physical performance of experiments in a lab, including synthesis processes, characterization processes, and sample transfers. Automation here can be provided by custom design and manufacturing, instrument vendors, or implemented using external equipment such as robot arms.
Data analysis, data interpretation, and decision-making all occur in software. For our purposes, we consider data analysis to be the execution of context-agnostic algorithms. This contrasts with data interpretation, which incorporates domain-specific or context-specific insights which may not be well-defined a priori. Tasks such as denoising, background subtraction, extraction of figures of merit, and visualization may be categorized as either data analysis or interpretation depending on the specific research project. Decision-making draws on the information from data analysis and interpretation, so that a recommendation or decision about the next step in an experiment can be provided.
Finally, to integrate the workflow, information must be communicated at each step, tying together the hardware and software, and physical and digital infrastructure.9,15–18 Communication in workflows can be automated with fixed or hard-coded linkages, or with application programming interfaces (APIs) that allow better modularity. With a sufficiently expressive and unified level of communication, multiple autonomous workflows could be orchestrated and interleaved ad hoc.
These categories are used in our framework for levels of laboratory autonomy in Fig. 5. This framework takes inspiration from the Society of Automotive Engineers (SAE) vehicle autonomy levels, which describes six levels of driving autonomy ranging from no driving automation (Level 0) to full driving automation (Level 5).19 The table entries show the minimal amount of automation needed in each category to achieve a given level of laboratory autonomy. These levels of autonomy span the range in desired automation expressed by survey respondents. Automation plays an assistive role in L0 to L2 (Levels 0 to 2), and autonomy has a central role in driving scientific research in L3 to L5.
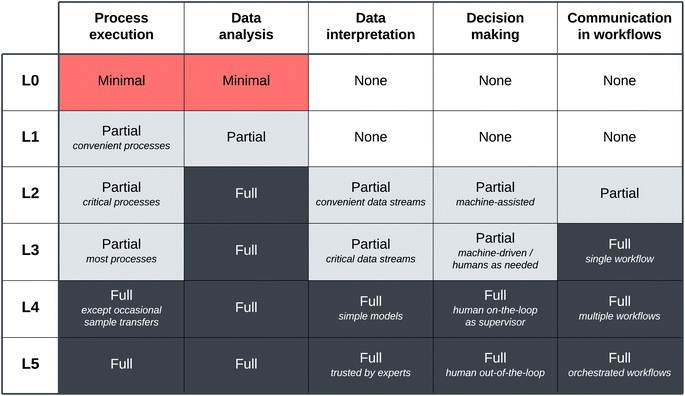 |
| Fig. 5 Levels of autonomy for laboratory research. The table defines the minimum automation needed in each category to achieve a given overall level of autonomy. | |
This framework intentionally projects diverse aspects of automation in both software and hardware onto the single axis of laboratory autonomy. We note that levels of laboratory (hardware) automation could also be defined by referring only to the process execution column. However, a six-level framework did not seem to provide additional clarity when describing software automation (i.e., data analysis, data interpretation, decision making, or communications).
We would also like to emphasize that this framework does not assume or define the scope of the research being categorized. The framework can be equally applied to describe the level of autonomy for comprehensive discovery workflows or for smaller processes that feed into a larger materials discovery research pipeline. The following examples illustrate some research areas where the framework may be applied.
We have observed that labs with a primary focus on accelerating discovery currently exist mostly between L0 to L2, with a few labs entering L3 autonomy. For example, we would consider the experimental work to synthesize and characterize an AI-predicted novel ternary oxide to be L1;20 high-throughput automation is leveraged on the computational side, automation of experiment is minimal apart from a specific instrument with automated varying-temperature XRD measurements. An example of L2 research is reflected in research optimizing battery cycling protocols.21 While process execution is mostly automated, and data analysis, interpretation, and decision making are fully automated—all aligning with L3—the communication between steps are not, keeping the laboratory research at L2. Humans must place cells into the cycler, initiate and terminate cycling processes, and decide the experiment is complete. An example of L3 research is the automated test stand.22 Here humans need to replenish electrolytes in the test stand, but an entire single workflow is automated.
From L0–L2 autonomy, automation increases the efficiency of experimental workflows and may augment the reproducibility of results by eliminating dull and repetitive tasks. In general, parts of process execution are automated and data analysis streamlined, but humans are ultimately responsible for data interpretation and decision making—tasks that require scientific intuition and iterative thinking. From L3–L6, these higher level scientific tasks (data interpretation and decision making) become automated and machine-driven. At these levels, human researchers only step in as necessary. Finally, labs that achieve L4 and especially L5 autonomy must include researchers and engineers with a strong interest in laboratory autonomy, and/or have applications where automated data interpretation and decision making is sufficiently mature and trusted.
5 Outlook
The content of this survey centered around laboratory automation and autonomy from the viewpoint of a single researcher or a specific lab; the community's response has provided visibility into the diverse perspectives that materials researchers hold on these topics. However, to further accelerate the impact of automated labs, the community needs to align its activities through shared visions and strategies. We recommend two community initiatives that could alleviate pain points and concerns reported in the survey, and amplify the impact of lab automation.
Open source or widely-accessible software and hardware
One research bottleneck cited by multiple survey respondents is the development of software and hardware that unifies automated or autonomous workflows (“Integration” in Fig. 3). Respondents listed bottlenecks from their own research, including “hardware–software integration”, “integrating new instrumentation”, “compatibility between different instruments”, and “defining error-free, robust, and reliable protocols”. The need to design, prototype, troubleshoot, and refine these tools can be a significant barrier to entry. Therefore, the availability of open source or widely-accessible software and hardware becomes essential for the rapid bootstrapping of new labs at all levels of autonomy, and also addresses the “Technical challenges” concern shown in Fig. 4. Open source software development is already a cornerstone of automated materials science research. Community norms around reproducibility (as well as publication and funding requirements) have prompted the growth of an increasing catalog of open source tools, and efforts such as MaRDA23 take input from across the community. Open source hardware and more accessible frugal twins aim to similarly “democratize” physical experiments.24 We note that while open source is the gold standard and should remain a priority to the community—especially for academic and prototyping research—we expect that well-documented and well-supported proprietary tools will become a practical alternative in many labs.
Close integration of theory and experiment
The biggest concerns that researchers have about fully autonomous research lie in the ability of autonomous labs to properly interpret data, produce hypotheses, and set objectives (Fig. 4). Some specific survey feedback around why respondents would avoid automation includes, “sometimes by processing data step by step you can notice trends/irregularities that do not appear in the fully processed dataset”, “this needs domain knowledge and I do not think robots can do this”, “we need to learn insights”, and “that's a trust/QX issue.” An important pathway toward alleviating these concerns would be by integrating large-scale theory predictions along with experimental testing and validation, likely in a lab equipped with L3 or more automation. Iterating using such workflows improves confidence in experimental data interpretation, and also aids the development of more accurate theory predictions (hypotheses). This type of tighter integration is already being demonstrated in predictive synthesis.25–30 There is still a critical gap between simulation and experiment, largely due to differences in materials representation,31 but automated labs that integrate theory and experiment enable the creation of new multimodal datasets and models that can aid in the construction of a large experimental knowledge graph32 and ultimately improve our fundamental understanding of materials.
Data availability
De-identified responses to the survey, additional details on survey design and deployment, and figures summarizing selected responses are provided in the ESI.†
Author contributions
Linda Hung: conceptualization, investigation, methodology, data curation, visualization, writing – original draft, writing – review & editing. Joyce A. Yager: investigation, data curation, methodology, writing – review & editing. Danielle Potocek: investigation, data curation, methodology, writing – review & editing. Dave Baiocchi: investigation, methodology. Ha-Kyung Kwon: conceptualization, methodology, writing – original draft, writing – review & editing. Shijing Sun: conceptualization, methodology, writing – original draft, writing – review & editing. Santosh Suram: conceptualization, methodology, writing – original draft, writing – review & editing.
Conflicts of interest
The authors have no conflicts of interest to declare.
Acknowledgements
We appreciate the time and thought that members of the Energy & Materials Division at Toyota Research Institute spent on initial brainstorming and feedback throughout the process—especially Amalie Trewartha, Joey Montoya, and Kevin Tran. We also obtained valuable perspectives from Debasish Banerjee, Chip Roberts, and Masato Hozumi from Toyota Research Institute of North America in the brainstorming session. We thank Kate Sieck and members of the Human-Centered AI division at Toyota Research Institute for their guidance on user study and survey processes. We thank Helge Stein, Michaela Stevens and other members of the Jaramillo lab, Ben Burchfield, Sera Evcimen, Calder Phillips-Grafflin, Ian McMahon, whose interviews and discussions provided the user stories and insights that informed survey design. Finally, we thank the materials science community for their responses to the survey.
References
- P. M. Maffettone, P. Friederich, S. G. Baird, B. Blaiszik, K. A. Brown, S. I. Campbell, O. A. Cohen, R. L. Davis, I. T. Foster, N. Haghmoradi, M. Hereld, H. Joress, N. Jung, H.-K. Kwon, G. Pizzuto, J. Rintamaki, C. Steinmann, L. Torresi and S. Sun, Digital Discovery, 2023, 2, 1644–1659 RSC.
-
G. Tom, S. P. Schmid, S. G. Baird, Y. Cao, K. Darvish, H. Hao, S. Lo, S. Pablo-García, E. M. Rajaonson, M. Skreta, N. Yoshikawa, S. Corapi, G. D. Akkoc, F. Strieth-Kalthoff, M. Seifrid and A. Aspuru-Guzik, Self-Driving Laboratories for Chemistry and Materials Science, 2024, https://chemrxiv.org/engage/chemrxiv/article-details/65a887f29138d231612bf6df Search PubMed.
- A. A. Volk and M. Abolhasani, Nat. Commun., 2024, 15, 1378 CrossRef CAS PubMed.
- J. H. Montoya, M. Aykol, A. Anapolsky, C. B. Gopal, P. K. Herring, J. S. Hummelshøj, L. Hung, H.-K. Kwon, D. Schweigert, S. Sun, S. K. Suram, S. B. Torrisi, A. Trewartha and B. D. Storey, Applied Physics Reviews, 2022, 9, 011405 CrossRef CAS.
- P. Cui, D. P. McMahon, P. R. Spackman, B. M. Alston, M. A. Little, G. M. Day and A. I. Cooper, Chem. Sci., 2019, 10, 9988–9997 RSC.
- R. L. Greenaway, V. Santolini, M. J. Bennison, B. M. Alston, C. J. Pugh, M. A. Little, M. Miklitz, E. G. B. Eden-Rump, R. Clowes, A. Shakil, H. J. Cuthbertson, H. Armstrong, M. E. Briggs, K. E. Jelfs and A. I. Cooper, Nat. Commun., 2018, 9, 2849 CrossRef CAS PubMed.
- T. Desautels, A. Krause and J. W. Burdick, J. Mach. Learn. Res., 2014, 15, 4053–4103 Search PubMed.
- S. Sun, A. Tiihonen, F. Oviedo, Z. Liu, J. Thapa, Y. Zhao, N. T. P. Hartono, A. Goyal, T. Heumueller, C. Batali, A. Encinas, J. J. Yoo, R. Li, Z. Ren, I. M. Peters, C. J. Brabec, M. G. Bawendi, V. Stevanovic, J. Fisher and T. Buonassisi, Matter, 2021, 4, 1305–1322 CrossRef CAS.
- F. Rahmanian, J. Flowers, D. Guevarra, M. Richter, M. Fichtner, P. Donnely, J. M. Gregoire and H. S. Stein, Adv. Mater. Interfaces, 2022, 9, 2101987 CrossRef.
- N. H. Angello, V. Rathore, W. Beker, A. Wołos, E. R. Jira, R. Roszak, T. C. Wu, C. M. Schroeder, A. Aspuru-Guzik, B. A. Grzybowski and M. D. Burke, Science, 2022, 378, 399–405 CrossRef CAS PubMed.
- L. Yang, J. A. Haber, Z. Armstrong, S. J. Yang, K. Kan, L. Zhou, M. H. Richter, C. Roat, N. Wagner, M. Coram, M. Berndl, P. Riley and J. M. Gregoire, Proc. Natl. Acad. Sci. U. S. A., 2021, 118, e2106042118 CrossRef CAS PubMed.
- S. Sun, N. T. P. Hartono, Z. D. Ren, F. Oviedo, A. M. Buscemi, M. Layurova, D. X. Chen, T. Ogunfunmi, J. Thapa, S. Ramasamy, C. Settens, B. L. DeCost, A. G. Kusne, Z. Liu, S. I. P. Tian, I. M. Peters, J.-P. Correa-Baena and T. Buonassisi, Joule, 2019, 3, 1437–1451 CrossRef CAS.
- M. Politi, F. Baum, K. Vaddi, E. Antonio, J. Vasquez, B. P. Bishop, N. Peek, V. C. Holmberg and L. D. Pozzo, Digital Discovery, 2023, 2, 1042–1057 RSC.
-
H. Hysmith, E. Foadian, S. P. Padhy, S. V. Kalinin, R. G. Moore, O. S. Ovchinnikova and M. Ahmadi, The Future of Self-Driving Laboratories: From Human in the Loop Interactive AI to Gamification, 2024, https://chemrxiv.org/engage/chemrxiv/article-details/65a052849138d23161b70212 Search PubMed.
- R. Chard, J. Pruyne, K. McKee, J. Bryan, B. Raumann, R. Ananthakrishnan, K. Chard and I. T. Foster, Future Gener. Comput. Syst., 2023, 142, 393–409 CrossRef.
-
M. Sim, M. G. Vakili, F. Strieth-Kalthoff, H. Hao, R. Hickman, S. Miret, S. Pablo-García and A. Aspuru-Guzik, ChemOS 2.0: An Orchestration Architecture for Chemical Self-Driving Laboratories, 2023, https://chemrxiv.org/engage/chemrxiv/article-details/64cbe80adfabaf06ffa61204 Search PubMed.
- M. J. Statt, B. A. Rohr, D. Guevarra, S. K. Suram and J. M. Gregoire, Digital Discovery, 2024, 3, 238–242 RSC.
- D. Guevarra, K. Kan, Y. Lai, R. J. R. Jones, L. Zhou, P. Donnelly, M. Richter, H. S. Stein and J. M. Gregoire, Digital Discovery, 2023, 2, 1806–1812 RSC.
-
J3016_202104: Taxonomy and Definitions for Terms Related to Driving Automation Systems for On-Road Motor Vehicles, SAE International, https://www.sae.org/standards/content/j3016_202104/ Search PubMed.
-
J. Montoya, C. Grimley, M. Aykol, C. Ophus, H. Sternlicht, B. H. Savitzky, A. M. Minor, S. Torrisi, J. Goedjen, C.-C. Chung, A. Comstock and S. Sun, Computer-Assisted Discovery and Rational Synthesis of Ternary Oxides, 2023, https://chemrxiv.org/engage/chemrxiv/article-details/644194d1df78ec50151bf1e3 Search PubMed.
- P. M. Attia, A. Grover, N. Jin, K. A. Severson, T. M. Markov, Y.-H. Liao, M. H. Chen, B. Cheong, N. Perkins, Z. Yang, P. K. Herring, M. Aykol, S. J. Harris, R. D. Braatz, S. Ermon and W. C. Chueh, Nature, 2020, 578, 397–402 CrossRef CAS PubMed.
- A. Dave, J. Mitchell, S. Burke, H. Lin, J. Whitacre and V. Viswanathan, Nat. Commun., 2022, 13, 5454 CrossRef CAS PubMed.
-
Materials Research Data Alliance, https://github.com/marda-alliance Search PubMed.
- S. Lo, S. Baird, J. Schrier, B. J Blaiszik, N. Carson, I. Foster, A. Aguilar-Granda, S. V. Kalinin, B. Maruyama, M. Politi, H. Tran, T. D. Sparks and A. Aspuru-Guzik, Digital Discovery, 2024, 3, 842–868 RSC.
- T. Ha, D. Lee, Y. Kwon, M. S. Park, S. Lee, J. Jang, B. Choi, H. Jeon, J. Kim, H. Choi, H.-T. Seo, W. Choi, W. Hong, Y. J. Park, J. Jang, J. Cho, B. Kim, H. Kwon, G. Kim, W. S. Oh, J. W. Kim, J. Choi, M. Min, A. Jeon, Y. Jung, E. Kim, H. Lee and Y.-S. Choi, Sci. Adv., 2023, 9, eadj0461 CrossRef CAS PubMed.
- J. M. Gregoire, L. Zhou and J. A. Haber, Nat. Synth, 2023, 2, 493–504 CrossRef.
- N. J. Szymanski, B. Rendy, Y. Fei, R. E. Kumar, T. He, D. Milsted, M. J. McDermott, M. Gallant, E. D. Cubuk, A. Merchant, H. Kim, A. Jain, C. J. Bartel, K. Persson, Y. Zeng and G. Ceder, Nature, 2023, 624, 86–91 CrossRef CAS PubMed.
- A. M. Lunt, H. Fakhruldeen, G. Pizzuto, L. Longley, A. White, N. Rankin, R. Clowes, B. Alston, L. Gigli, G. M. Day, A. I. Cooper and S. Y. Chong, Chem. Sci., 2024, 15, 2456–2463 RSC.
-
J. Chen, S. R. Cross, L. J. Miara, J.-J. Cho, Y. Wang and W. Sun, Navigating Phase Diagram Complexity to Guide Robotic Inorganic Materials Synthesis, 2023, http://arxiv.org/abs/2304.00743 Search PubMed.
-
F. Rahmanian, S. Fuchs, B. Zhang, M. Fichtner and H. S. Stein, Autonomous Millimeter Scale High Throughput Battery Research System (Auto-MISCHBARES), 2024, https://chemrxiv.org/engage/chemrxiv/article-details/659ead759138d231619ca38c Search PubMed.
- S. B. Torrisi, M. Z. Bazant, A. E. Cohen, M. G. Cho, J. S. Hummelshøj, L. Hung, G. Kamat, A. Khajeh, A. Kolluru, X. Lei, H. Ling, J. H. Montoya, T. Mueller, A. Palizhati, B. A. Paren, B. Phan, J. Pietryga, E. Sandraz, D. Schweigert, Y. Shao-Horn, A. Trewartha, R. Zhu, D. Zhuang and S. Sun, APL Mach. Learn., 2023, 1, 020901 CrossRef.
- M. J. Statt, B. A. Rohr, D. Guevarra, J. Breeden, S. K. Suram and J. M. Gregoire, Digital Discovery, 2023, 2, 909–914 RSC.
|
This journal is © The Royal Society of Chemistry 2024 |
Click here to see how this site uses Cookies. View our privacy policy here.