DOI:
10.1039/D4DD00130C
(Perspective)
Digital Discovery, 2024,
3, 1923-1932
Digital chemistry: navigating the confluence of computation and experimentation – definition, status quo, and future perspective
Received
13th May 2024
, Accepted 15th August 2024
First published on 19th August 2024
Abstract
Digital chemistry represents a transformative approach integrating computational methods, digital data, and automation within the chemical sciences. It is defined by using digital toolkits and algorithms to simulate, predict, accelerate, and analyze chemical processes and properties, augmenting traditional experimental methods. The current status quo of digital chemistry is marked by rapid advancements in several key areas: high-throughput screening, machine learning models, quantum chemistry, and laboratory automation. These technologies have enabled unprecedented speeds in discovering and optimizing new molecules, materials, and reactions. Digital retrosynthesis and structure–active prediction tools have supported these endeavors. Furthermore, integrating large-language models and robotics in chemistry labs (e.g. demonstrated in self-driving labs) have begun to automate routine tasks and complex decision-making processes. Looking forward, the future of digital and digitalized chemistry is poised for significant growth, driven by the increasing accessibility of computational resources, the expansion of chemical databases, and the refinement of artificial intelligence algorithms. This evolution promises to accelerate innovation in drug discovery, materials science, and sustainable manufacturing, ultimately leading to more efficient, cost-effective, and environmentally friendly chemical research and production. The challenge lies in advancing the technology itself, fostering interdisciplinary collaboration, and ensuring the ethical use of digital tools in chemical research.
1. Introduction
We live in the digital age. The history of digital chemistry1 – a field of research that advances chemistry through methods from computational and data science,2 machine learning, and robotics – traces its roots back to the mid-20th century, evolving through several key stages shaped by technological and theoretical advances:
(I) Early computational models (1950s–1960s):
The inception of digital chemistry can be traced back to the development of early computational models and the application of quantum mechanics to solve chemical problems. Pioneers like Robert S. Mulliken,3 Linus Pauling,4 and Walter Kohn5 laid the groundwork with their contributions to theoretical and quantum chemistry and molecular orbital theory. The advent of computers provided the necessary tools to calculate molecular structures and properties, albeit in a very limited capacity due to the computational power available at the time.
(II) Growth of computational power (the 1970s–1980s):
As computers became more powerful and accessible (see also the Moore law),6 the 1970s and 1980s saw a significant expansion in computational (and computer) chemistry. Software packages capable of performing semi-empirical and ab initio calculations became widespread, enabling chemists to predict molecular geometries, electronic structures, and reactivity with increasing accuracy,7–10 for example, with the density functional theory (DFT).11 During this decade, E. J. Corey's digital retrosynthetic analyses,12 Ivar Ugi's chemical computer analysis (in particular for multicomponent reactions),13,14 Johann Gasteiger's neural networks,15 and the Harvard ChemDraw project emerged.16 This period also marked the beginning of molecular dynamics simulations, allowing for the exploration of the behavior of molecules in motion.
(III) Introduction of quantitative structure–activity relationships (QSAR) (1980s):
The 1980s saw the emergence of QSAR models (coined in 1967 (ref. 17)), which correlated the structure of chemical compounds with their biological activities. This was a major step forward in drug design, allowing for the prediction of the activity of new compounds before their synthesis.
(IV) Rise of high-throughput screening and robotics (1990s–2000s):
The integration of high-throughput experimentation (HTE) and screening (HTS) technologies and robotics in the 1990s and 2000s marked a shift towards automation in chemical research (Fig. 1). This period enabled the rapid screening of vast libraries of compounds for pharmaceutical research, significantly accelerating the drug discovery process.18
 |
| Fig. 1 High-throughput screening platform. © Frederique Menard-Aubin. | |
(V) Integration of machine learning and big data (2010s–present):
The most recent phase in the history of digital chemistry involves incorporating digital retrosynthesis19–21 tools for predicting reactions, machine learning (ML), and big data analytics. Data sciences include interdisciplinary questions about data integration and harmonization from different disciplines. For example, in the pharmaceutical sector, it is important to link clinical study data with drug development data. The rapid increase of chemical data available from literature, databases,22 and experimental results, combined with advanced ML algorithms, has led to unprecedented capabilities in predicting material properties, reaction outcomes, and even designing new molecules. The Human Genome Project,23 the over-exponential use of large language models,24 and the rise of chemical biology, for example, with click chemistry,25 also triggered the exploration of the chemical26 and process space, often with the aid of supercomputers.27
1.1. Prelude: future perspective
Digital chemistry is poised to become even more integrated with advancements in artificial intelligence, quantum computing, and laboratory automation technologies, such as self-driving laboratories.28,29 These developments promise to improvement the way chemical research is conducted, making it faster, more efficient, more reliable in terms of quality, more reproducible, and more sustainable.30
Throughout its history, digital chemistry has continually transformed the landscape of chemical research, moving from simple computational models to complex systems capable of simulating and predicting intricate chemical phenomena. As computational power and algorithms evolve, digital chemistry stands at the forefront of the next generation of scientific discovery and innovation.
2. Definition
Digital chemistry is the combination of chemical science, data sciences, and digital technologies, where computational tools and algorithms are employed to simulate, understand, accelerate, and predict chemical processes and molecular behaviors (Fig. 2).31 This approach harnesses the power of computers to model reactions, design new molecules, and explore the vast chemical space more efficiently than traditional experimental methods alone. Digital chemistry encompasses several key areas, including computational chemistry,32 together with molecular modeling,33 cheminformatics,34–36 virtual screening,37 machine learning,38 artificial intelligence applications,39 quantum computing, and dedicated software and devices such as electronic lab notebooks and finally robotics.40 It aims to accelerate discovery and innovation in various fields, such as drug development, materials science, and sustainable chemistry, by providing insights that are difficult, if possible, to obtain through conventional laboratory experiments. Some key elements of digital chemistry and their incorporation with related sciences, such as informatics and engineering, are sketched in Fig. 2.
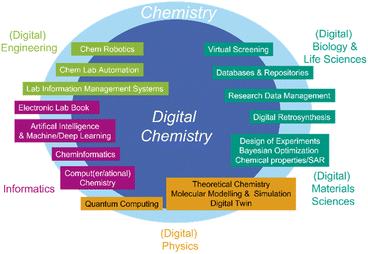 |
| Fig. 2 Some of the components of digital chemistry are embedded in chemistry and related sciences. Digital life sciences and material sciences use many of the same workflows and methods; thus, they are colored the same. | |
The brief descriptions of the seven key components of digital chemistry are:
(I) Computational chemistry (since 1975 (ref. 41)): Understand the behavior of molecules and reactions at the atomic level using quantum mechanical calculations and molecular dynamics simulations (see 4.1.).41,42 This is in combination with molecular modeling (since 1970 (ref. 43)): creating 3D models of molecules and materials to visualize their structure and properties and to optimize their design for specific applications (see 4.1.).33
(II) Cheminformatics (since 1998 (ref. 44)): Analyzing and managing large datasets of chemical compounds using computational techniques, such as data mining, to identify patterns and properties that can guide drug discovery45,46 and materials science (see 4.2.).44,47
(III) Virtual screening (since 1989 (ref. 37)), together with experimental screening: Employing computational methods to screen vast libraries of chemical compounds for potential drug candidates or materials with desired properties, thereby reducing the time and cost of experimental screening (see 4.3.).
(IV) Machine learning (since 1990 (ref. 48)) and artificial intelligence (since 1972 (ref. 49)) in Chemistry: Utilizing machine learning algorithms, deep learning,50 and artificial intelligence techniques to predict molecular properties, optimize chemical reactions, and assist in designing new molecules and materials (see 4.4.).39,51
(V) Dedicated software tools52,53 and toolkits: Streamline and enhance the documentation, management, and analysis of chemical experiments, data, and workflows, ensuring efficiency, compliance, and collaboration in scientific research (see 4.6).
(VI) Quantum computing (since 1989 (ref. 54)): Exploring the potential of quantum computing to solve complex chemical problems more efficiently than classical computers, particularly for simulating quantum systems and optimizing molecular structures (see 4.5.).46,55
(VII) Chemical lab robotics,40,56,57 automatization, and self-driving chemical labs:12 Streamline experimental processes by employing robotic systems and advanced algorithms to perform tasks such as sample preparation, analysis, and data interpretation with minimal human intervention (see 4.7.).
3. “Digital chemistry,” “digitalized chemistry,” or “digitized chemistry”?
The terms Digital chemistry, digitalized chemistry, and digitized chemistry often overlap in usage but can imply slightly different nuances in the context of integrating chemistry with digital technology – to make chemistry digital.58
(I) Digital chemistry typically refers to the broad application of digital technologies and computational methods to solve chemical problems, encompassing everything from quantum chemistry simulations to artificial intelligence for predicting chemical reactions. It implies an inherent integration of digital tools into the chemical research and development fabric.59–62
(II) Digitalized chemistry suggests transforming traditional chemistry practices and workflows into digital formats, emphasizing changing or transitioning towards digital methods. It focuses on adopting and integrating digital tools and technologies to enhance the efficiency, accessibility, and effectiveness of chemical research and industrial processes.63,64
(III) Digitized chemistry often converts analog chemical data and information (e.g., paper lab notebooks, printed spectra) and seamless digital workflows into digital formats. It focuses on the initial step of making chemical information more accessible and manageable through digital means, which is a foundational aspect of enabling further digital analysis and computational work.65
While these terms are closely related and sometimes used interchangeably, the subtle differences lie in their emphasis: “Digital Chemistry” on the broad use of digital approaches, “Digitalized Chemistry” on the process of adopting these digital methods, and “Digitized Chemistry” on the initial step of converting analog information into digital form.
4. Elaboration, explanations, and examples of key components in digital chemistry
Expanding on the concept of digital chemistry involves delving deeper into its components, applications, and transformative impact on research, development, and industry.
4.1. Computational chemistry66 and molecular modeling
4.1.1 Quantum mechanical calculations.
At the heart of computational chemistry are quantum mechanical (QM) calculations, which predict the electronic structure, energy levels, and molecular geometries based on the principles of quantum mechanics. Techniques like density functional theory (DFT) and ab initio methods allow scientists to explore the fundamental properties of molecules with high precision.
4.1.2 Molecular dynamics (MD) simulations.
MD simulations provide dynamic insights into molecular systems, showing how atoms and molecules move and interact over time, nowadays with machine learning models. This is crucial for understanding processes like protein folding, chemical reactions in solutions, and material behaviours under different conditions.33
4.1.3 3D visualization.
Tools for 3D visualization of molecules and materials enable researchers to explore structural features, simulate docking (how two molecules fit together), and design molecules with tailored properties.
4.1.4 Design and optimization.
Advanced software packages allow for manipulating molecular structures to optimize binding affinity, stability, or other desired characteristics, facilitating the design of better drugs and materials.67,68
4.2. Cheminformatics
4.2.1 Molecular descriptors and fingerprints.
Cheminformatics involves the calculation of molecular descriptors and fingerprints, which are numerical or graphical representations of molecular structures and properties. These are used in machine learning models to predict chemical compounds' activities, properties, or toxicities.69
4.2.2 Database mining and virtual libraries.
With the proliferation of chemical databases (e.g., PubChem, ChEMBL, Scifinder (see also 4.5.), scientists can mine vast amounts of data to identify compounds with desirable properties or structural motifs, significantly speeding up the discovery process.70,71 However, measures and open-access properties annotated to structures are lacking and holding everyone back.
4.2.3 Toolkits in chemistry.
Numerous toolkits have emerged, such as OpenBabel,72,73 RDkit,74 CDK,75 Molfiles,76,77 Cinfony,78 and the Indigo toolkit.79
Cheminformatics will also deal with patents, standard operation procedures, and GHS/EHS Management.
4.3. Virtual (and experimental) screening of libraries
4.3.1 High-throughput screening (HTS).
Digital chemistry complements experimental HTS (or recently HPS – high power screening with billions of entities,80 originally invented by the late Richard Lerner)81 by predicting which compounds are most likely to be active against a target, thus prioritizing those for laboratory synthesis and testing, saving resources. The rise of combinatorial chemistry and a (massive)82–84 parallelization of virtual or real chemical experiments led to his.
4.3.2 Structure-based drug design (SBDD).
Virtual screening is integral to SBDD, where the 3D structure of a target (often a protein) is used to screen and design compounds that can bind to it effectively, guiding the development of new drugs.85 Some notable examples are BioNeMo (NVIDIA)86 and Alphafold (Google).87
4.4. Machine learning and artificial intelligence in chemistry
4.4.1 Predictive models.
AI algorithms can learn from chemical data to predict outcomes of reactions, properties of compounds, or biological activities, making the design process faster and more efficient (Fig. 3).38
 |
| Fig. 3 Digital reticular chemistry explored by Yaghi et al. The two cycles – computation and experimental – together with a human interface interacted and built the database, from ref. 88. | |
Digital reticular chemistry – pioneered by Omar Yaghi – is a complete cycle consisting of a comprehensive database for metal–organic—frameworks (MOFs), computational and experimental discovery cycles, and a human–digital interface (Fig. 3).88–92
4.4.2 Semantic data and ontologies.
Semantic data are structured for machine understanding through metadata and standardized formats, while ontologies93,94 provide a formal framework of concepts and relationships to organize and interpret this data.95
4.4.3 Autonomous optimization and decision making; design-of-experiments in chemistry (DoE).
Conventionally design-of-experiments, Bayesian optimization,96 or recently Bandit optimization,97 systematically plans and conducts experimental investigations using statistical methods to efficiently explore chemical processes, optimize parameters, and understand the effects of multiple variables on outcomes.98
4.4.4 Large-language models.
Large-language models for chemistry utilize vast amounts of text data and advanced natural language processing techniques (Fig. 4) to generate insights, predict properties, and assist in drug discovery (Fig. 5)100 and materials design101,102 within chemistry.103,104
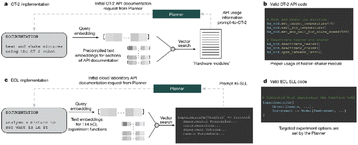 |
| Fig. 4 Large language model in chemistry by Gomes et al.99 | |
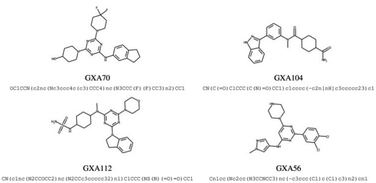 |
| Fig. 5 Selected compounds from a large library identified for COVID. | |
Gabe Gomes recently showed that a large-language model could be integrated into a chemical workflow (Fig. 4).99
In a consortial effort, researchers from IBM, Oxford University, and Enamine drug target inhibitor discovery with a deep generative foundation model. They target a protein sequence-conditioned sampling on the generative foundation model to design small-molecule inhibitors for two dissimilar targets: the spike protein receptor-binding domain (RBD) and the main protease from SARS-CoV-2 (Fig. 5).105
4.4.5 Analyze analytical data with artificial intelligence.
Artificial intelligence analyzes analytical data by employing advanced algorithms and computational techniques to extract insights, patterns, and correlations, enhancing decision-making and predictive modeling capabilities. In particular, generative models like Foundation Models such as LLMs and Artificial General Intelligence (AGI) will play a role in the future. Digital retrosynthesis is an active application of these techniques.
4.4.6 Accelerated simulations.
Machine-learned potentials have emerged over the past 20 years as a new paradigm in atomistic simulations106 Starting in 2007,107 neural network-based potentials became an integral part in the acceleration of ab initio molecular dynamics simulations, reaching new records in data efficiency simulations106,107 and applicability to complex systems.108
4.4.7 Inverse design.
An active field of development is generative machine learning models that solve inverse design challenges, i.e., the prediction of molecules given desired target properties.109,110 Initial efforts of inverse design made use of variational autoencoders,111 later replaced by generative adversarial networks (GANs)112 and reinforcement learning.113,114 Currently, the main research focus lies on diffusion models.
4.5. Dedicated software tools and RDM
4.5.1 Research data management (RDM), the FAIR principles, and open digital chemistry.
RDM involves the organized and systematic handling of data throughout its lifecycle to adhere to the FAIR principles (findable, accessible, interoperable, and reusable (Fig. 6))115,116 and facilitates Open Digital Chemistry, promoting transparent and collaborative research practices in the field.117,118 Cheminformatics will also deal with patents, standard operation procedures, and GHS/EHS Management.
 |
| Fig. 6 Infrastructure and the workflow provided with NFD4Chem. | |
The NFD4Chem119 is the national research data infrastructure for chemistry in Germany. This research data infrastructure initiative enables innovative services for research data to collect, store, process, analyze, publish, and re-use research data dedicated to chemistry. It provides a platform for RDM and FAIR principles (Fig. 6).
4.5.2 Laboratory information management systems (LIMS).
These components are specialized software solutions that organize, track, and manage chemical samples, data, and workflows, facilitating streamlined operations and data integrity in chemical laboratories. They support quality control, regulatory compliance, and efficient research and development processes by providing a centralized digital platform for managing laboratory information.72,120–124
4.5.3 Electronic laboratory notebooks (ELN).
Electronic lab notebooks (or journals) for chemistry (Fig. 7) are digital platforms that enable researchers to systematically document experiments, observations, and results in a searchable, secure, and shareable format, streamlining the research process and enhancing collaboration.73
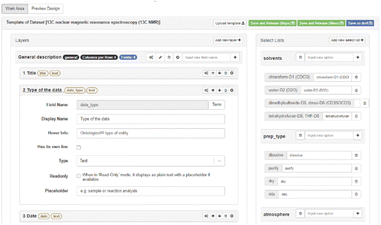 |
| Fig. 7 Screenshot of the Chemotion ELN.74 | |
The open-source Electronic Lab Notebook Chemotion ELN includes tools for molecular chemistry and supports the connection of devices (Fig. 7).74 It uses chemical ontologies to semantically annotate,75,94 incorporates SMILES,76 uses Ketcher,77 supports automated curation of analytical data,78 and has a generic module called LabIMotion.
4.5.4 Repositories.
The chemical community has relied on general and abreacting databases (e.g., CAS, Beilstein/Reaxys, see 4.2.) or specific (e.g., CCDC) for decades – but seemingly not error-free.22 Nowadays, community-based repositories, including raw data, play a major role (e.g., NIST Materials Data Repository),125 preferentially in a curated manner,74 and increase transparency and reproducibility.
4.6. Quantum computing in chemistry125,126
4.6.1 Solving complex problems.
Quantum computers offer the potential to perform intractable calculations for classical computers, such as accurately simulating large quantum systems, which could revolutionize how we design materials and understand chemical reactions.
4.6.2 Optimization.
Quantum algorithms are being developed for optimizing molecular structures, reaction paths, and even experimental parameters, promising to unlock new frontiers in chemistry and materials science.
Quantum computing could support solutions, for example, molecular dynamics (MD) simulation, which often fails in practice on large problems due to lack of computing power; QM calculations are only accurate to a limited extent; DFT is dependent on functionals and basis sets, and only a rough approximation; more accurate ab initio calculations such as CCSD(T), full CI or quantum Monte Carlo methods already fail at benzene with high accuracy.127
Quantum computing will make large database searches significantly better/more efficient, and molecular modeling – a 3D structure of large molecules (protein folding, conformer calculations128) or active side modeling/docking is raised to the next level. The data sets required for machine learning – with quantum machine learning – to train powerful models can be drastically reduced (i.e., less experimental data is needed for the same result, or better predictions can be made with the same number of data). Automation/robotics benefits from “path optimization” and scheduling calculations (i.e., the same robot has a higher throughput).
4.7. Automation and robotics129,130
Integration with automated laboratory systems and robotics131,132 for high-throughput experimentation enables a seamless loop of design, synthesis, testing, and feedback, accelerating the pace of discovery (Fig. 8 and 9).86,133,135 Liquid handling is a must, but the progress of solid dosing was remarkable.136
 |
| Fig. 8 A so-called Chemputer, designed by the Cronin lab.133 | |
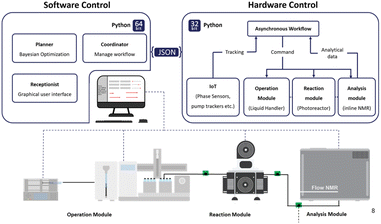 |
| Fig. 9 The Robochem from the Noël group features continuous flow.134 | |
Lee Cronin and his team created a so-called Chemputer,133,137 which refers to a concept that combines chemistry with automated processes, specifically using automated systems and software using a chemical programming language to synthesize chemicals and materials (Fig. 8). A chemputer essentially automates the chemical synthesis process, enabling researchers to produce compounds quickly and precisely by following digital protocols.
Robochem from the Noël group went in another direction (Fig. 9). This is combined with flow chemistry and photochemistry with integrated analytics.134
5. Embedding digital chemistry in traditional chemistry and science
All branches of chemistry benefit from digital chemistry, including analytical, bio-, food, inorganic, material,138–140 medicinal,141,142 organic, pharmaceutical, physical, polymer, quantum,143 radio-, technical, and theoretical chemistry. Furthermore, the industrial impact of digital chemistry revolutionizes manufacturing processes, product development, and quality control by leveraging computational methods, automation, and data analytics to streamline operations,144 optimize formulations, and accelerate innovation.145 Digital chemistry also requires dedicated teaching on all levels.146–150 and specific notations of entities151 while setting new benchmarks.
Digital chemistry, when integrated with digital biology, creates a synergistic platform for simulating complex biological systems and chemical interactions at the molecular level, enhancing drug discovery and biomaterials development. Coupling this with digital materials science, for example, with nano and micro 3D prints of materials that require digital blueprints,152 further expands the potential for designing novel materials with tailored properties, revolutionizing fields from sustainable energy to advanced manufacturing through predictive modeling and high-throughput computational experiments. Data harmonization and integration of semantic web technologies will allow mapping and overarching data analysis across disciplines.
Branching out digitalization – computer-aided chemistry153 and synthesis planning (CASP),154 pattern recognition in chemistry,155 virtual chemistry labs,72 digital sustainable chemistry,156 digital synthetic electrochemistry,157 digital twins34,158–160 curiosity-driven discovery,161 iterative chemical synthesizers,162 data-driven discovery,163 digitization of synthesis and chemical programming,164 natural language processing (NLP),165 chemical graph theory,166 computer-based automatic indexing,167 Neural Networks in Chemistry and Drug Design,15,168 chemometrics,169 chemical ‘Oracles’170 and reinforcement learning,171 semantic in chemistry/chemical semantic,172,173 knowledge graphs174 are other terms relevant to digital chemistry.
6. Conclusions: impact of digital chemistry and future directions
Digital chemistry transforms chemical research and enables sustainable practices, such as more efficient catalysts, renewable energy materials, and reduced need for hazardous chemicals in drug synthesis. As computational power grows and algorithms become more sophisticated, integrating digital tools in chemistry promises to deepen our understanding of the molecular world and accelerate the development of innovative solutions to global challenges in health, energy, and materials science.
Data availability
As this is a Perspective article, no primary research results, data, software or code have been included.
Conflicts of interest
There are no conflicts to declare.
Acknowledgements
We acknowledge the valuable input from our team at KIT and colleagues in the last few years. Very valuable input came from Alexander Böser (5-HT), Daniel Volz (Kipu Quantum), Georg Wuitschik (Roche), Pascal Friederich, Wolfgang Wenzel (both KIT), Christoph Steinbeck (Jena), Oliver Koepler (TIB – Leibniz Information Centre for Science and Technology, Hannover), and Zhengtao Xu (Singapore). Our research is funded by Helmholtz Information, the KIT (KMNFi), and the DFG (Excellence Cluster 3DMM2O EXC-2082/1–390761711, NFDI4Chem). Note: this article used, in smaller parts, large-language models (ChatGPT175) in addition to databases (SciFinder, Scopus) to search for openly available data, keywords, and definitions. The author paraphrased, edited, and polished the text.
Notes and references
- We prefer “Digital Chemistry” over “Digitalized Chemistry” or “Digitized Chemistry”: see Section 3..
-
T. Hey, S. Tansley, K. Tolle and J. Gray, The Fourth Paradigm: Data-Intensive Scientific Discovery, Microsoft Research, 2009 Search PubMed
.
- R. S. Mulliken, J. Chem. Phys., 1955, 23, 1833 Search PubMed
.
- L. Pauling, R. B. Corey and H. R. Branson, Proc. Natl. Acad. Sci. U. S. A., 1951, 37, 205 Search PubMed
.
- W. Kohn, A. D. Becke and R. G. Parr, J. Phys. Chem., 1996, 100, 12974–12980 Search PubMed
.
-
R. Chau, B. Doyle, M. Doczy, S. Datta, S. Hareland, B. Jin, J. Kavalieros and M. Metz, 61st Device Research Conference. Conference Digest (Cat. No. 03TH8663), 2003 Search PubMed.
- R. Ahlrichs, M. Baer, M. Haeser, H. Horn and C. Koelmel, Chem. Phys. Lett., 1989, 162, 165 Search PubMed
.
- H. Sun, J. Phys. Chem. B, 1998, 102, 7338–7364 Search PubMed
.
- V. Hornak, R. Abel, A. Okur, B. Strockbine, A. Roitberg and C. Simmerling, Proteins: Struct., Funct., Bioinf., 2006, 65, 712–725 Search PubMed
.
- J. Hafner, J. Comput. Chem., 2008, 29, 2044–2078 Search PubMed
.
- P. Hohenberg and W. Kohn, Phys. Rev., 1964, 136, B864–B871 Search PubMed
.
- E. J. Corey, Chem. Soc. Rev., 1988, 17, 111–133 Search PubMed
.
- I. Ugi and G. Kaufhold, Adv. Cycloaddit., 1967, 709, 11–28 Search PubMed
.
- I. Ugi, A. Domling and W. Horl, Endeavour, 1994, 18, 115 Search PubMed
.
-
J. Zupan and J. Gasteiger, Neural Networks for Chemists: Textbook, VCH Verlagsgesellschaft, Germany, 1993 Search PubMed
.
- D. A. Evans, Angew. Chem., Int. Ed., 2014, 53, 11140–11145 Search PubMed
.
- J. A. Singer and W. P. Purcell, J. Med. Chem., 1967, 10, 1000–1002 Search PubMed
.
- C. A. Lipinski, F. Lombardo, B. W. Dominy and P. J. Feeney, Adv. Drug Delivery Rev., 2001, 46, 3–26 Search PubMed
.
- C. D. Christ, M. Zentgraf and J. M. Kriegl, J. Chem. Inf. Model., 2012, 52, 1745–1756 Search PubMed
.
- I. V. Tetko, P. Karpov, R. Van Deursen and G. Godin, Nat. Commun., 2020, 11, 5575 Search PubMed
.
- M. H. Todd, Chem. Soc. Rev., 2005, 34, 247–266 Search PubMed
.
- A. J. Williams, S. Ekins and V. Tkachenko, Drug Discovery Today, 2012, 17, 685–701 Search PubMed
.
- C. DeLisi, Nature, 2008, 455, 876–877 Search PubMed
.
-
S. R. Bowman, arXiv, 2023, preprint, arXiv:2304.00612, DOI:10.48550/arXiv.2304.00612.
- H. C. Kolb, M. G. Finn and K. B. Sharpless, Angew. Chem., Int. Ed., 2001, 40, 2004–2021 Search PubMed
.
- J.-L. Reymond and M. Awale, ACS Chem. Neurosci., 2012, 3, 649–657 Search PubMed
.
-
https://www.helmholtz.de/en/research/research-fields/information/engineering-digital-futures/
.
- B. P. MacLeod, F. G. L. Parlane, T. D. Morrissey, F. Hase, L. M. Roch, K. E. Dettelbach, R. Moreira, L. P. E. Yunker, M. B. Rooney, J. R. Deeth, V. Lai, G. J. Ng, H. Situ, R. H. Zhang, M. S. Elliott, T. H. Haley, D. J. Dvorak, A. Aspuru-Guzik, J. E. Hein and C. P. Berlinguette, Sci. Adv., 2020, 6, eaaz8867 Search PubMed
.
- F. Hase, L. M. Roch and A. Aspuru-Guzik, Trends Chem., 2019, 1, 282–291 Search PubMed
.
- K. M. Jablonka, L. Patiny and B. Smit, Nat. Chem., 2022, 14, 365–376 Search PubMed
.
- This definition overlaps sometimes with the description of chemoinformatics..
-
P. A. Dub and M. D. Halls, ACS Fall 2023, San Francisco, CA, USA, 2023 Search PubMed.
- W. Humphrey, A. Dalke and K. Schulten, J. Mol. Graphics, 1996, 14, 33 Search PubMed
.
-
T. Gressling, ACS Spring 2020, CINF-0160, 2020 Search PubMed.
-
Sometimes, Chemoinformatics have been Used:
https://en.wikipedia.org/wiki/Cheminformatics
Search PubMed.
- T. Engel, J. Chem. Inf. Model., 2006, 46, 2267–2277 Search PubMed
.
- R. Cté and H. Haug, Phys. Rev. B: Condens. Matter Mater. Phys., 1989, 40, 3802–3811 Search PubMed
.
- K. Jorner, Chimia, 2023, 77, 22–30 Search PubMed
.
- K. T. Butler, D. W. Davies, H. Cartwright, O. Isayev and A. Walsh, Nature, 2018, 559, 547–555 Search PubMed
.
- S. Chatterjee, M. Guidi, P. H. Seeberger and K. Gilmore, Nature, 2020, 579, 379–384 Search PubMed
.
-
J. B. Collins, PhD thesis, Princeton University, 1975
.
- A. L. Robinson, Science, 1976, 193, 470–472 Search PubMed
.
- B. Ford, G. G. Hall and J. C. Packer, Int. J. Quantum Chem., 1970, 4, 533–539 Search PubMed
.
- F. K. Brown, Annu. Rep. Med. Chem., 1998, 33, 375–384 Search PubMed
.
- A. Daina, O. Michielin and V. Zoete, Sci. Rep., 2017, 7, 42717 Search PubMed
.
- R. Santagati, A. Aspuru-Guzik, R. Babbush, M. Degroote, L. González, E. Kyoseva, N. Moll, M. Oppel, R. M. Parrish, N. C. Rubin, M. Streif, C. S. Tautermann, H. Weiss, N. Wiebe and C. Utschig-Utschig, Nat. Phys., 2024, 549–557 Search PubMed
.
- This definition is similar to data sciences in chemistry..
- H. Gelernter, J. R. Rose and C. Chen, J. Chem. Inf. Comput. Sci., 1990, 30, 492–504 Search PubMed
.
- B. R. Kowalski and C. F. Bender, J. Am. Chem. Soc., 1972, 94, 5632–5639 Search PubMed
.
- A. C. Mater and M. L. Coote, J. Chem. Inf. Model., 2019, 59, 2545–2559 Search PubMed
.
- K. T. Schuett, H. E. Sauceda, P. J. Kindermans, A. Tkatchenko and K. R. Mueller, J. Chem. Phys., 2018, 148, 241722 Search PubMed
.
- N. M. O'Boyle, M. Banck, C. A. James, C. Morley, T. Vandermeersch and G. R. Hutchison, J. Cheminf., 2011, 3, 33 Search PubMed
.
- M. D. Hanwell, D. E. Curtis, D. C. Lonie, T. Vandermeersch, E. Zurek and G. R. Hutchison, J. Cheminf., 2012, 4, 17 Search PubMed
.
- M. Conrad, Biosystems, 1989, 22, 197–213 Search PubMed
.
- J. E. Moore, Nature, 2010, 464, 194–198 Search PubMed
.
- This includes microfluidics, lab-on-chip, and flow chemistry..
- A. Slattery, Z. Wen, P. Tenblad, J. Sanjose-Orduna, D. Pintossi, T. den Hartog and T. Noel, Science, 2024, 383, eadj1817 Search PubMed
.
- The term “Chemistry 4.0” reflects the digital industrial chemistry..
- P. Seavill, Nat. Synth., 2023, 2, 469–470 Search PubMed
.
- A. Cardinale, A. Castrogiovanni, T. Gaudin, J. Geluykens, T. Laino, M. Manica, D. Probst, P. Schwaller, A. Sobczyk, A. Toniato, A. C. Vaucher, H. Wolf and F. Zipoli, Chimia, 2023, 77, 484–488 Search PubMed
.
- R. L. Greenaway, K. E. Jelfs, A. C. Spivey and S. N. Yaliraki, Nat. Rev. Chem, 2023, 7, 527–528 Search PubMed
.
- Chemputation is a part of Digital Chemistry – see Section 4.8..
- C. Eitemüller, F. Trauten, M. Striewe and M. Walpuski, J. Sci. Educ. Technol., 2023, 32, 453–467 Search PubMed
.
- V. M. Petruševski, M. Stojanovska and B. Šoptrajanov, Educ. Quim., 2009, 20, 466–470 Search PubMed
.
- F. Hase, T. Tamayo-Mendoza, C. Boixo, J. Romero, L. M. Roch and A. Aspuru-Guzik, PLoS One, 2020, 15(4), 1–16 Search PubMed
.
- Some companies: Schrödinger, Quantistry, MQS, HafniumLabs..
-
P. Sanschagrin, ACS Fall 2023, San Francisco, CA, USA, 2023 Search PubMed.
-
J. G. Brandenburg, ACS Fall 2022, Chicago, IL, USA, 2022 Search PubMed.
- D. Rogers and M. Hahn, J. Chem. Inf. Model., 2010, 50, 742–754 Search PubMed
.
- T. Barrett, T. O. Suzek, D. B. Troup, S. E. Wilhite, W.-C. Ngau, P. Ledoux, D. Rudnev, A. E. Lash, W. Fujibuchi and R. Edgar, Nucleic Acids Res., 2005, 33, D562 Search PubMed
.
- J. J. Irwin and B. K. Shoichet, J. Chem. Inf. Comput. Sci., 2005, 45, 177–182 Search PubMed
.
- I. V. Tetko, J. Gasteiger, R. Todeschini, A. Mauri, D. Livingstone, P. Ertl, V. A. Palyulin, E. V. Radchenko, N. S. Zefirov, A. S. Makarenko, V. Y. Tanchuk and V. V. Prokopenko, J. Comput.-Aided Mol. Des., 2005, 19, 453–463 Search PubMed
.
- P. Tremouilhac, A. Nguyen, Y.-C. Huang, S. Kotov, D. S. Lütjohann, F. Hübsch, N. Jung and S. Bräse, J. Cheminf., 2017, 9, 54 Search PubMed
.
- P. Tremouilhac, C.-L. Lin, P.-C. Huang, Y.-C. Huang, A. Nguyen, N. Jung, F. Bach, R. Ulrich, B. Neumair, A. Streit and S. Bräse, Angew. Chem., Int. Ed., 2020, 59, 22771–22778 Search PubMed
.
- J. Hastings, D. Magka, C. Batchelor, L. Duan, R. Stevens, M. Ennis and C. Steinbeck, J. Cheminf., 2012, 4, 8 Search PubMed
.
- D. Weininger, J. Chem. Inf. Comput. Sci., 1988, 28, 31 Search PubMed
.
- B. Karulin and M. Kozhevnikov, J. Cheminf., 2011, 3, P3 Search PubMed
.
-
Y.-C. Huang, P. Tremouilhac, S. Kuhn, P.-C. Huang, C.-L. Lin, N. Schloerer, O. Taubert, M. Goetz, N. Jung and S. Bräse, ChemRxiv, 2024, preprint, pp. 1–30, DOI:10.26434/chemrxiv-2024-1r9tb.
-
https://lifescience.opensource.epam.com/indigo/
.
- Y. K. Sunkari, V. K. Siripuram, T.-L. Nguyen and M. Flajolet, Trends Pharmacol. Sci., 2022, 43, 4–15 Search PubMed
.
- S. Brenner and R. A. Lerner, Proc. Natl. Acad. Sci. U. S. A., 1992, 89, 5381–5383 Search PubMed
.
- J. Pihl, M. Karlsson and D. T. Chiu, Drug Discovery Today, 2005, 10, 1377–1383 Search PubMed
.
- C. Holtze, J. Phys. D: Appl. Phys., 2013, 46, 114008 Search PubMed
.
- P. C. Nickels, B. Wuensch, P. Holzmeister, W. Bae, L. M. Kneer, D. Grohmann, P. Tinnefeld and T. Liedl, Science, 2016, 354, 305–307 Search PubMed
.
- S. P. Leelananda and S. Lindert, Beilstein J. Org. Chem., 2016, 12, 2694–2718 Search PubMed
.
-
https://github.com/NVIDIA/BioNeMo
.
-
https://alphafold.ebi.ac.uk/
.
- H. Lyu, Z. Ji, S. Wuttke and O. M. Yaghi, Chem, 2020, 6, 2219–2241 Search PubMed
.
- K. M. Jablonka, A. S. Rosen, A. S. Krishnapriyan and B. Smit, ACS Cent. Sci., 2023, 9, 563–581 Search PubMed
.
- Z. Zheng, Z. Rong, N. Rampal, C. Borgs, J. T. Chayes and O. M. Yaghi, Angew. Chem., Int. Ed., 2023, 62, e202311983 Search PubMed
.
- Z. Zheng, Z. He, O. Khattab, N. Rampal, M. A. Zaharia, C. Borgs, J. T. Chayes and O. M. Yaghi, Digital Discovery, 2024, 3, 491–501 Search PubMed
.
- A. A. AlGhamdi, Mol. Front. J., 2024 DOI:10.1142/s2529732524500019
.
-
C. Pachl, N. Frank, J. Breitbart and S. Bräse, arXiv, 2020, preprint, arXiv:2002.03842, DOI:10.48550/arXiv.2002.03842.
- P. Strömert, J. Hunold, A. Castro, S. Neumann and O. Koepler, Pure Appl. Chem., 2022, 94, 605–622 Search PubMed
.
- J. Hastings, L. Chepelev, E. Willighagen, N. Adams, C. Steinbeck and M. Dumontier, PLoS One, 2011, 6, e25513 Search PubMed
.
- B. J. Shields, J. Stevens, J. Li, M. Parasram, F. Damani, J. I. M. Alvarado, J. M. Janey, R. P. Adams and A. G. Doyle, Nature, 2021, 590, 89–96 Search PubMed
.
- J. Y. Wang, J. M. Stevens, S. K. Kariofillis, M.-J. Tom, D. L. Golden, J. Li, J. E. Tabora, M. Parasram, B. J. Shields, D. N. Primer, B. Hao, D. Del Valle, S. DiSomma, A. Furman, G. G. Zipp, S. Melnikov, J. Paulson and A. G. Doyle, Nature, 2024, 626, 1025–1033 Search PubMed
.
- S. A. Weissman and N. G. Anderson, Org. Process Res. Dev., 2015, 19, 1605–1633 Search PubMed
.
- D. A. Boiko, R. MacKnight, B. Kline and G. Gomes, Nature, 2023, 624, 570–578 Search PubMed
.
- T. Burki, Lancet Digital Health, 2020, 2, e226–e227 Search PubMed
.
- Y. Luo, S. Bag, O. Zaremba, A. Cierpka, J. Andreo, S. Wuttke, P. Friederich and M. Tsotsalas, Angew. Chem., Int. Ed., 2022, 61, e202200242 Search PubMed
.
- L. Pilz, C. Natzeck, J. Wohlgemuth, N. Scheuermann, S. Spiegel, S. Oßwald, A. Knebel, S. Bräse, C. Wöll, M. Tsotsalas and N. Prasetya, J. Mater. Chem. A, 2023, 11, 24724–24737 Search PubMed
.
- A. Cardinale, A. Castrogiovanni, T. Gaudin, J. Geluykens, T. Laino, M. Manica, D. Probst, P. Schwaller, A. Sobczyk, A. Toniato, A. C. Vaucher, H. Wolf and F. Zipoli, Chimia, 2023, 77, 484–488 Search PubMed
.
-
A. C. Vaucher, F. Shortt de Hernandez, S. Hausberg and T. Laino, Abstracts of Papers, ACS Fall 2022, Chicago, IL, United States, 2022, vol. 2022 Search PubMed.
- V. Chenthamarakshan, S. C. Hoffman, C. D. Owen, P. Lukacik, C. Strain-Damerell, D. Fearon, T. R. Malla, A. Tumber, C. J. Schofield, H. M. E. Duyvesteyn, W. Dejnirattisai, L. Carrique, T. S. Walter, G. R. Screaton, T. Matviiuk, A. Mojsilovic, J. Crain, M. A. Walsh, D. I. Stuart and P. Das, Sci. Adv., 2023, 9, eadg7865 Search PubMed
.
- P. Friederich, F. Häse, J. Proppe and A. Aspuru-Guzik, Nat. Mater., 2021, 20, 750–761 Search PubMed
.
- J. Behler and M. Parrinello, Phys. Rev. Lett., 2007, 98, 146401 Search PubMed
.
-
I. Batatia, P. Benner, Y. Chiang, A. M. Elena, D. P. Kovács, J. Riebesell, X. R. Advincula, M. Asta, M. Avaylon, W. J. Baldwin, F. Berger, N. Bernstein, A. Bhowmik, S. M. Blau, V. Cărare, J. P. Darby, S. De, F. D. Pia, V. L. Deringer, R. Elijošius, Z. El-Machachi, F. Falcioni, E. Fako, A. C. Ferrari, A. Genreith-Schriever, J. George, R. E. A. Goodall, C. P. Grey, P. Grigorev, S. Han, W. Handley, H. H. Heenen, K. Hermansson, C. Holm, J. Jaafar, S. Hofmann, K. S. Jakob, H. Jung, V. Kapil, A. D. Kaplan, N. Karimitari, J. R. Kermode, N. Kroupa, J. Kullgren, M. C. Kuner, D. Kuryla, G. Liepuoniute, J. T. Margraf, I.-B. Magdău, A. Michaelides, J. H. Moore, A. A. Naik, S. P. Niblett, S. W. Norwood, N. O'Neill, C. Ortner, K. A. Persson, K. Reuter, A. S. Rosen, L. L. Schaaf, C. Schran, B. X. Shi, E. Sivonxay, T. K. Stenczel, V. Svahn, C. Sutton, T. D. Swinburne, J. Tilly, C. van der Oord, E. Varga-Umbrich, T. Vegge, M. Vondrák, Y. Wang, W. C. Witt, F. Zills and G. Csányi, arXiv, 2024, preprint, arXiv:2401.00096, DOI:10.48550/arXiv.2401.00096.
- B. Sanchez-Lengeling and A. Aspuru-Guzik, Science, 2018, 361, 360–365 Search PubMed
.
- D. M. Anstine and O. Isayev, J. Am. Chem. Soc., 2023, 145, 8736–8750 Search PubMed
.
- R. Gómez-Bombarelli, J. N. Wei, D. Duvenaud, J. M. Hernández-Lobato, B. Sánchez-Lengeling, D. Sheberla, J. Aguilera-Iparraguirre, T. D. Hirzel, R. P. Adams and A. Aspuru-Guzik, ACS Cent. Sci., 2018, 4, 268–276 Search PubMed
.
-
N. D. Cao and T. Kipf, arXiv, 2022, preprint, arXiv:1805.11973, DOI:10.48550/arXiv.1805.11973.
- Z. Zhou, S. Kearnes, L. Li, R. N. Zare and P. Riley, Sci. Rep., 2019, 9, 10752 Search PubMed
.
- A. Eberhard, H. Metni, G. Fahland, A. Stroh and P. Friederich, Mach. Learn.: Sci. Technol., 2024, 5, 015055 Search PubMed
.
- F. Tristram, N. Jung, P. Hodapp, R. R. Schröder, C. Wöll and S. Bräse, Adv. Funct. Mater., 2023, 34, 2303615 Search PubMed
.
- F. Tristram, N. Jung, P. Hodapp, R. R. Schröder, C. Wöll and S. Bräse, Adv. Funct. Mater., 2024, 2303615, DOI:10.1002/adfm.202303615
.
-
T. Gressling, ACS Fall 2022, Chicago, IL, United States, 2022 Search PubMed.
-
E. Davis, Abstracts of Papers, ACS Fall 2022, Chicago, IL, United States, 2022, vol. 2022 Search PubMed.
-
NFD4chem:
https://www.nfdi4chem.de
Search PubMed.
- M. C. Kolb, J. T. Maddock and B. N. Weaver, Am. Doc., 1966, 17, 180 Search PubMed
.
- A. N. Stevens, J. Chem. Doc., 1961, 1, 74 Search PubMed
.
- P. F. Cole, R. Dickinson, L. Guczi, D. Hill and H. J. V. Tyrrell, J. Sci. Instrum., 1965, 42, 650 Search PubMed
.
- P. C. Sarkar, J. Indian Counc. Chem., 1998, 15, 1–6 Search PubMed
.
- M. Thompson, S. L. R. Ellison and R. Wood, Pure Appl. Chem., 2002, 74, 835–855 Search PubMed
.
-
https://www.mckinsey.com/industries/chemicals/our-insights/the-next-big-thing-quantum-computings-potential-impact-on-chemicals
.
- F. A. Evangelista and V. S. Batista, J. Chem. Theory Comput., 2023, 19, 7435–7436 Search PubMed
.
- Y. Cao, J. Romero, J. P. Olson, M. Degroote, P. D. Johnson, M. Kieferová, I. D. Kivlichan, T. Menke, B. Peropadre, N. P. D. Sawaya, S. Sim, L. Veis and A. Aspuru-Guzik, Chem. Rev., 2019, 119, 10856–10915 Search PubMed
.
- Y. Cao, J. Romero, J. P. Olson, M. Degroote, P. D. Johnson, M. Kieferova, I. D. Kivlichan, T. Menke, B. Peropadre, N. P. D. Sawaya, S. Sim, L. Veis and A. Aspuru-Guzik, Chem. Rev. (Washington, DC, U. S.), 2019, 119, 10856–10915 Search PubMed
.
- C. W. Coley, N. S. Eyke and K. F. Jensen, Angew. Chem., Int. Ed., 2020, 59, 22858–22893 Search PubMed
.
- C. W. Coley, N. S. Eyke and K. F. Jensen, Angew. Chem., Int. Ed., 2020, 59, 23414–23436 Search PubMed
.
- For example articulated robots..
- J. Li, S. G. Ballmer, E. P. Gillis, S. Fujii, M. J. Schmidt, A. M. E. Palazzolo, J. W. Lehmann, G. F. Morehouse and M. D. Burke, Science, 2015, 347, 1221–1226 Search PubMed
.
- S. Steiner, J. Wolf, S. Glatzel, A. Andreou, J. M. Granda, G. Keenan, T. Hinkley, G. Aragon-Camarasa, P. J. Kitson, D. Angelone and L. Cronin, Science, 2019, 363, eaav2211 Search PubMed
.
- A. Slattery, Z. Wen, P. Tenblad, J. Sanjosé-Orduna, D. Pintossi, T. den Hartog and T. Noël, Science, 2024, 383, eadj1817 Search PubMed
.
-
Other concepts include ChemASAP:
https://www.chemasap.kit.edu/
Search PubMed.
- M. Abolhasani and E. Kumacheva, Nat. Synth., 2023, 2, 483–492 Search PubMed
.
- Cronin also coined chemputation..
- S. Okur, T. Hashem, E. Bogdanova, P. Hodapp, L. Heinke, S. Bräse and C. Wöll, ACS Sens., 2024, 9, 622–630 Search PubMed
.
- P. Kalhor, N. Jung, S. Bräse, C. Wöll, M. Tsotsalas and P. Friederich, Adv. Funct. Mater., 2024, 34, 2302630 Search PubMed
.
- I. Boldog, G. J. Reiss, K. V. Domasevitch, T. Base and S. Bräse, Cryst. Growth Des., 2019, 19, 5218–5227 Search PubMed
.
- R. Macarron, M. N. Banks, D. Bojanic, D. J. Burns, D. A. Cirovic, T. Garyantes, D. V. S. Green, R. P. Hertzberg, W. P. Janzen, J. W. Paslay, U. Schopfer and G. S. Sittampalam, Nat. Rev. Drug Discovery, 2011, 10, 188–195 Search PubMed
.
- M. K. Gilson, G. Georg and S. Wang, J. Med. Chem., 2014, 57, 1137 Search PubMed
.
- F. Neese, Wiley Interdiscip. Rev.: Comput. Mol. Sci., 2012, 2, 73–78 Search PubMed
.
- A. J. Williams, L. Harland, P. Groth, S. Pettifer, C. Chichester, E. L. Willighagen, C. T. Evelo, N. Blomberg, G. Ecker, C. Goble and B. Mons, Drug Discovery Today, 2012, 17, 1188–1198 Search PubMed
.
- Most of the larger chemical companies have a dedicated Chief Digital Officer..
-
https://www.imperial.ac.uk/study/courses/postgraduate-taught/digital-chemistry/
.
-
M.-T. Kuo, P. Lekhavat and L. L. Jones, Book of Abstracts, 211th ACS National Meeting, New Orleans, LA, 1996 Search PubMed.
- For example, Chemwiki, http://chemwiki.scc.kit.edu/main/mediawiki/Main_Page.
-
C. Neidiger, et al., ChemRxiv, 2024, preprint, DOI:10.26434/chemrxiv-2024-5dkhm.
-
https://knowledgebase.nfdi4chem.de/knowledge_base/?userLocale=true
.
- E. A. Smolenskii, I. V. Chuvaeva and A. L. Lapidus, Dokl. Chem., 2011, 437, 116–119 Search PubMed
.
- J. Fischer and M. Wegener, Laser Photonics Rev., 2013, 7, 22–44 Search PubMed
.
- D. A. Burns, ACS Symp. Ser., 1984, 261, 93–107 Search PubMed
.
- C. W. Coley, W. H. Green and K. F. Jensen, Acc. Chem. Res., 2018, 51, 1281–1289 Search PubMed
.
- D. Garfinkel, W. Polk, J. J. Higgins and R. T. Oscher, Commun. Assoc. Comput. Mach., 1962, 5, 115 Search PubMed
.
- This is perspective – the phrase has not been coined so far..
-
For example
https://en.etos.uni-mainz.de/
Search PubMed.
- J. N. Acosta, G. J. Falcone, P. Rajpurkar and E. J. Topol, Nat. Med., 2022, 28, 1773–1784 Search PubMed
.
- B. Björnsson, C. Borrebaeck, N. Elander, T. Gasslander, D. R. Gawel, M. Gustafsson, R. Jörnsten, E. J. Lee, X. Li, S. Lilja, D. Martínez-Enguita, A. Matussek, P. Sandström, S. Schäfer, M. Stenmarker, X. F. Sun, O. Sysoev, H. Zhang and M. Benson, Genome Med., 2019, 12, 4 Search PubMed
.
- A. C. Ngandjong, T. Lombardo, E. N. Primo, M. Chouchane, A. Shodiev, O. Arcelus and A. A. Franco, J. Power Sources, 2021, 485, 229320 Search PubMed
.
- L. Bustillo, T. Laino and T. Rodrigues, Chem. Sci., 2023, 14, 10378–10384 Search PubMed
.
- A. I. Leonov, A. J. S. Hammer, S. Lach, S. H. M. Mehr, D. Caramelli, D. Angelone, A. Khan, S. O'Sullivan, M. Craven, L. Wilbraham and L. Cronin, Nat. Commun., 2024, 15, 1240 Search PubMed
.
- R. Pollice, G. dos Passos Gomes, M. Aldeghi, R. J. Hickman, M. Krenn, C. Lavigne, M. Lindner-D'Addario, A. Nigam, C. T. Ser, Z. Yao and A. Aspuru-Guzik, Acc. Chem. Res., 2021, 54, 849–860 Search PubMed
.
- L. Wilbraham, S. H. M. Mehr and L. Cronin, Acc. Chem. Res., 2021, 54, 253–262 Search PubMed
.
- K. Choudhary and M. L. Kelley, J. Phys. Chem. C, 2023, 127, 17545–17555 Search PubMed
.
- A. T. Balaban, J. Chem. Inf. Comput. Sci., 1985, 25, 334–343 Search PubMed
.
-
P. A. D. deMaine and T. Rotwitt, presented in part at the Proceedings of the 1971 ACM SIGFIDET (now SIGMOD) Workshop on Data Description, Access and Control, San Diego, California, 1971 Search PubMed.
- J. Gasteiger and J. Zupan, Angew. Chem., Int. Ed., 1993, 32, 503–527 Search PubMed
.
-
M. Otto, Chemometrics: Statistics and Computer Application in Analytical Chemistry, John Wiley & Sons, 2023 Search PubMed
.
- D. Reker, E. A. Hoyt, G. J. L. Bernardes and T. Rodrigues, Cell Rep. Phys. Sci., 2020, 1, 100247 Search PubMed
.
- C. Beeler, S. G. Subramanian, K. Sprague, M. Baula, N. Chatti, A. Dawit, X. Li, N. Paquin, M. Shahen, Z. Yang, C. Bellinger, M. Crowley and I. Tamblyn, Digital Discovery, 2024, 3, 742–758 Search PubMed
.
-
https://www.semanticuniverse.com/articles-semantic-chemistry/
.
- J. G. Frey and C. L. Bird, Wiley Interdiscip. Rev. Comput. Mol. Sci., 2013, 3, 465–481 Search PubMed
.
- M. H. S. Segler and M. P. Waller, Chem.–Eur. J., 2017, 23, 6118–6128 Search PubMed
.
- G. E. Cacciamani, I. S. Gill and G. S. Collins, Nature, 2023, 618, 238 Search PubMed
.
|
This journal is © The Royal Society of Chemistry 2024 |
Click here to see how this site uses Cookies. View our privacy policy here.