Evaluating emissions and meteorological contributions to air quality trends in northern China based on measurements at a regional background station†
Received
28th May 2024
, Accepted 25th September 2024
First published on 8th October 2024
Abstract
The contributions of meteorology and emissions to air pollutant trends are critical for air quality management, but they have not been fully analyzed, especially in the background area of northern China. Here, we used a machine learning technique to quantify the impacts of meteorological conditions and emissions on PM2.5, NO2, SO2, O3, and CO pollution during 2013–2021 and evaluated their contributions to Clean Air Action policies. The annual effect of the meteorology on PM2.5, NO2, SO2, and CO levels was dominated by the meteorological conditions during the cold season, while that of the O3 level largely depended on the meteorological conditions during the warm season. Meteorology-driven anomalies contributed −14.8 to 10.3%, −8.5 to 7.3%, −11 to 7.1%, −7.9 to 6.0%, and −7.4 to 7.3% to the annual mean concentrations of PM2.5, NO2, SO2, O3, and CO during the study period, respectively. The Clean Air Actions have led to a major improvement in the air quality at regional scale, with the reduction of 1.7 μg m−3 year−1, 0.2 μg m−3 year−1, 1.5 μg m−3 year−1, 0.7 μg m−3 year−1, and 0.03 mg m−3 year−1 for PM2.5, NO2, SO2, O3, and CO at background area, respectively, after meteorological correction. Although emissions dominated the long-term variations in pollutants, the meteorological conditions obviously played a positive role during the action periods for pollutants except for O3. Considering the notable effects of the meteorological conditions on air pollution and the interreaction between pollutants, a more comprehensive control strategy should be considered on a broader regional scale.
Environmental significance
The contribution of meteorology and emissions to long-term trends of air pollutants is critical for air quality management. During the past decade, China has implemented two national clean air actions. In order to examine the effectiveness of such emission reductions and assess the resulting changes in air quality, we applied the random forest machine learning technique to decouple meteorological influences from emissions changes during 2013–2021. The deweathered trends of air pollutants at a regional background station in Northern China were examined. Our results emphasize that a more comprehensive control strategy is needed in this region.
|
1 Introduction
Air pollution has aroused much public concern due to its adverse impacts on the environment, human health, and climate.1,2 PM2.5, PM10, NO2, SO2, O3, and CO are defined as criteria pollutants worldwide for quantifying air pollution levels.3 In recent decades, along with rapid industrialization and urbanization, China has experienced severe air pollution problems, especially in the Beijing–Tianjin–Hebei (BTH) region. In January 2013, the BTH region suffered severe and persistent haze pollution, with the monthly average concentration of PM2.5 reaching almost 160 μg m−3, affecting approximately 1.3 million km2 and 800 million people in northern China.4
In recent years, China has experienced growing public awareness of air pollution. To alleviate air pollution, the State Council of China launched the “Air Pollution Prevention and Control Action Plan” (2013–2017) on September 10, 2013, also known as the first Clean Air Action. Although the stringent control measures were implemented, heavy pollution episodes still frequently occurred in the winter.5 To fulfill the action target, the “Action Plan for Comprehensive Control of Atmospheric Pollution in Autumn and Winter in the BTH region from 2017 to 2018” (the Comprehensive Action) was subsequently implemented in autumn 2017. To continue to reduce the heavy air pollution, the State Council issued the “Blue Sky Protection Campaign” (2018–2020) on July 3, 2018, which is also known as the second Clean Air Action. In addition to these air pollution control actions, the 13th (2016–2020) and 14th (2021–2025) Five-Year Plans (FYPs) outlined goals for reductions in air pollutants. After these actions and FYPs were implemented, the air quality in BTH was obviously improved. However, the air quality in this region still does not meet the guidelines of the WMO (AQG, 2021), highlighting the urgent need for a better understanding of how pollution could be addressed effectively.
Anthropogenic emissions have been widely accepted as the dominant factor of air pollution.6–10 However, meteorological conditions also exert a notable influence on pollutant variations, not only through transport but also by affecting natural emissions and chemical rates.11–13 For example, the probability of severe O3 pollution when there are heat waves could be up to seven times of the average probability during summertime, while temperature inversions in wintertime could enhance the probability of severe particulate matter pollution by more than a factor.14 Yousefian et al.15 pointed out that temperature was the key influencing factor for PM2.5 and PM10 concentrations, while nebulosity and solar radiation exerted major influences on ambient SO2 and O3 concentrations. Additionally, there is a moderate coupling between wind speed and NO2 and CO concentrations. Therefore, distinguishing the contributions of emissions and meteorology is essential for the evaluation of clean air policies, projection of future air quality, and formulation of control air pollution methods.
Various studies have been conducted to separate the contributions of emissions and meteorology. Chemical transport models are widely used to evaluate the response of the air quality to emission control measures.5,16 Nevertheless, due to the uncertainties in emission inventories and models, simulations may not fully reflect real-world conditions. Statistical analysis is another common method to assess meteorology-associated changes and the contribution of emissions, such as the Kolmogorov–Zurbenko filter model, multiple linear regression method, difference-in-difference approach, and deep neural networks.17–21
Among these models, machine learning models based on regression decision trees always achieve a better performance than chemical transport models and traditional statistical methods by reducing the variance, bias, and error in high-dimension datasets.22 Decision trees make no assumptions on the input data structure, allow for interaction and collinearity among variables, and will ignore variables that are irrelevant to the dependent variable.23 However, decision trees could suffer heavily from overfitting, which would result in the model being contaminated with noise, and unreliable predictions would impede analyses. Random forest (RF) is an algorithm that controls for the tendency of decision trees to overfit and has the advantage of not being a “black box” method where the learning process can be explained, investigated, and interpreted. Recently, RF has been widely adopted to identify air quality improvements in response to control strategies.20,24–29
Previous studies have mainly focused on assessing the changes in air quality using data of one or serval city observation sites; however, background measurements that could represent regional air pollution trends in key regions remain relatively limited for investigation. Thus, to improve the understanding of the contributions of meteorology and emissions to air quality trends at the regional scale in northern China, RF machine learning techniques and long-term background measurements were applied in this study. After decoupling the effects of meteorological conditions on the observed concentrations, trend analysis of the contributions of meteorology and emissions could be achieved. Our results could be useful for supporting air quality management strategies in the future.
2 Data and methods
2.1 Station and data for modeling
The background station, Shangdianzi (SDZ; 40°39′N, 117°07′E; 293.9 m a.s.l), is situated in the transitional region between the NCP and the Yanshan Mountain area and serves as one of the regional background stations in China as well as a regional Global Atmosphere Watch station (Fig. 1). It is approximately 100 and 55 km northeast of the urban area and the Miyun Township of Beijing, respectively. There are only small villages within 30 km of the station in the mountainous area; these villages have sparse populations and thus represent insignificant anthropogenic emission sources. Therefore, the atmospheric pollution level at SDZ was used to represent the background concentrations of atmospheric pollutants in the economically developed regions of North China.
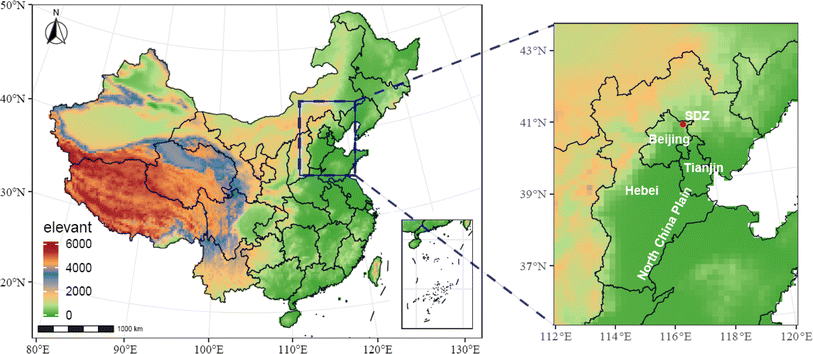 |
| Fig. 1 Location of the SDZ background station (red dot). | |
PM2.5, NO2, SO2, O3, and CO concentrations have been continuously measured at SDZ since 2005. To evaluate the effectiveness of emission reduction and the contribution of meteorological conditions to the air quality during clean air actions, background concentrations of the above pollutants from 2013 to 2021 were considered in this study. A tapered element oscillating microbalance (TEOM) (R&P Model 1400a) was employed for the PM2.5 measurements. ThermoFisher 42ITL, 43ITL, 49I, and 48I analyzers were utilized for the NO2, SO2, O3, and CO observations, respectively. The measurements, calibration methods, and quality assurance/quality control procedures have been described by Pu et al.30
Hourly meteorological data, including wind speed (WS), wind direction (WD), temperature (T), relative humidity (RH), and atmospheric pressure (P), were obtained from the China Meteorological Data Service Center (https://data.cma.cn/en). Planetary boundary layer height (PBLH) data were obtained from the ERA5 reanalysis dataset. Hourly O3 and CO data for Beijing (BJ), Tianjin (TJ), and Shijiazhuang (SJZ) were downloaded from https://quotsoft.net/air/ and https://www.mee.gov.cn and then averaged into yearly mean values. Annual average PM2.5, NO2, and SO2 data were obtained from the Ecology and Environment Bureaus of BJ, TJ, and SJZ.
2.2 Weather normalization using the RF model
A machine-learning-based RF model was applied to decouple the effects of meteorological conditions. The RF algorithm-based weather normalization technique was first proposed by Grange et al.22 A RF model was built for each pollutant independently. The original datasets include hourly concentrations of air pollutants and their predictor variables, including time variables such as Unix time, Julian day, day of the week, and hour of the day, and meteorological parameters (WS, WD, P, T, RH, and PBLH). The variables were chosen based on Grange et al.24 and Guo et al.20 The Unix time represents the emission trend term of a given pollutant, while the Julian day is a seasonal term that represents emissions or atmospheric chemistry, which varies seasonally. The weekday term represents the difference in pollution between weekdays and weekend days. The hour of the day (hour) explains emissions with a diurnal cycle. These processes are generally strong drivers of the concentrations of most atmospheric pollutants.31 70% of the original datasets were randomly inputted as training datasets to construct the RF model using the “rmweather” package in R, available at https://cran.r-project.org/web/packages/rmweather/index.html. The remaining original data were adopted as a test dataset to validate the performance of the constructed model. To eliminate the impacts of meteorological factors on air pollutants while preserving their seasonal and diurnal variations, the algorithm of Vu et al.26 was applied. The meteorological dataset from 2013 to 2021 was randomly resampled and then fed into the RF model to predict the atmospheric concentrations of the above pollutants. The resampling and calculation processes were repeated 1000 times, and the results were averaged to obtain the final weather-normalized concentration. Vu et al.26 compared RF modelling results with those from the CMAQ-WRF model.32 Their results were similar; however, the correlation coefficients between observation and prediction of pollutants by the RF model were higher than those of the CMAQ-WRF model. In addition, the concentration of pollutants after decoupling meteorology effects matched the energy consumption trend and the emission inventory, indicating the results obtained by the RF model were reliable.
3 Results and discussion
3.1 Evaluation of the RF model
The RF model achieved favorable performance based on the correlation coefficient (R2) between the hourly predicted and observed data on both the testing and training datasets (Fig. 2 and S1†). Before employing the model, statistical metrics were calculated to evaluate the model performance (Table S1†), including fraction of predictions with a factor of two (FAC2), mean bias (MB), mean gross error (MGE), normalized mean bias (NMB), normalized mean gross error (NMGE), root-mean-square error (RMSE), Pearson correlation coefficient (r), COE (coefficient of efficiency), and index of agreement (IOA). The results confirmed that the model performs well and can be employed for weather normalization analysis. The relative importance of the predictor variables in the final random forest model is shown in Fig. 3. The seasonal variable (Julian day) is the most important variable controlling the pollutant concentration, except for O3, and T mainly controls the O3 predicted values. The trend variable (Unix time) plays a very important role in the SO2 and CO models, suggesting that emission control shows effectiveness on the variation of both of them. Regarding the meteorological variables, RH and T also play more important roles in the models.
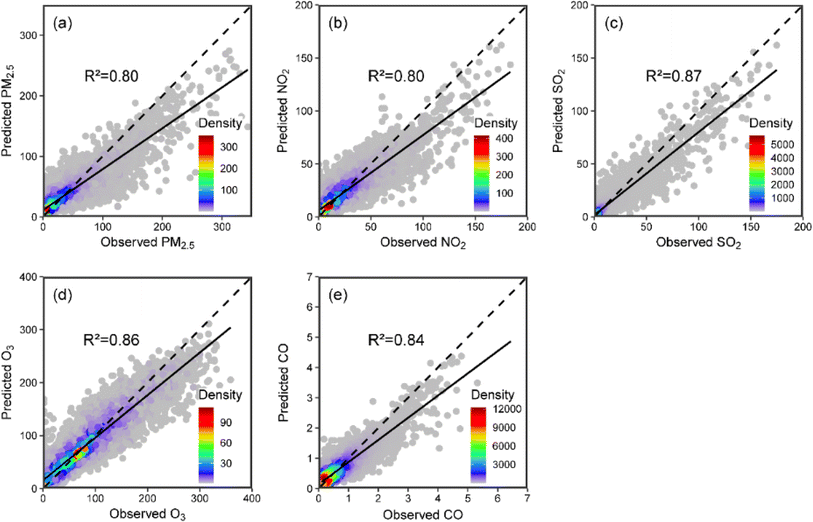 |
| Fig. 2 Correlations between the hourly observed and predicted data on the testing datasets of PM2.5 (a), NO2 (b), SO2 (c), O3 (d), and CO (e). The units of PM2.5, NO2, SO2, and O3 are μg m−3, while that of CO is mg m−3. | |
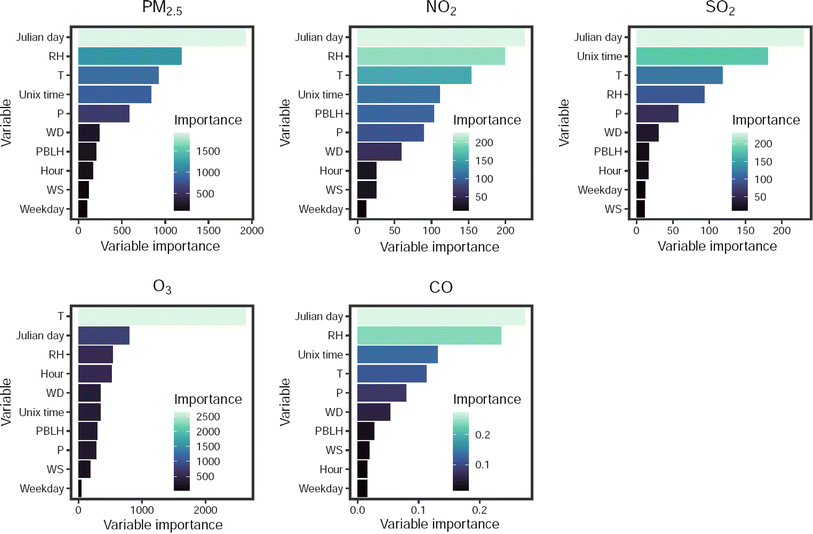 |
| Fig. 3 Relative importance of the predictor variables in the final RF model. | |
3.2 Observed levels at the background station and surrounding areas
To obtain a preliminary understanding of the characteristics of air pollutants at SDZ, the time series of the annual average concentrations of PM2.5, NO2, SO2, O3, and CO at SDZ and surrounding areas are shown in Fig. 4. During the study period, the overall air quality in northern China obviously improved, with the annual mean concentrations of air pollutants at SDZ generally declining year by year. After the first Clean Air Action was implemented in 2013, the concentrations of PM2.5, NO2, SO2, and CO at BJ, TJ, and SJZ also showed dramatic downward trends, and their concentrations in 2021 were very close to those at SDZ. These results confirm the significant improvement in the air quality in the BTH region during 2013–2021. Notably, the annual mean concentration of PM2.5 in BJ declined from 89.5 μg m−3 in 2013 to 34.4 μg m−3 in 2021, which is the first time it matched China's national ambient air quality standard (NAAQS-II) of 35 μg m−3. However, the concentrations of PM2.5 at both BJ and SDZ were still substantially higher than the WHO guideline of 5 μg m−3 (AQG, 2021). Although the NO2 concentrations at BJ, TJ, SJZ, and SDZ matched or were lower than the NAAQS-II in 2021, the concentrations also greatly deviated from the AQG standard, with an annual concentration of 10 μg m−3, which suggests that stricter policies should be continuously implemented in northern China.
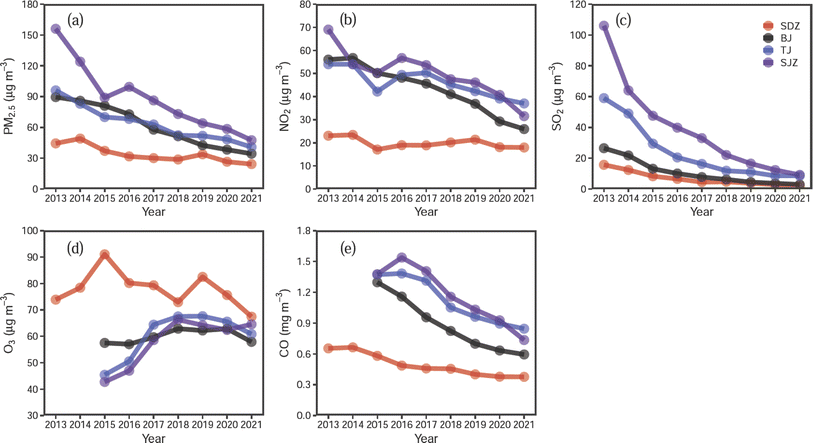 |
| Fig. 4 Annual averages of PM2.5 (a), NO2 (b), SO2 (c), O3 (d), and CO (e) at SDZ, BJ, TJ and SJZ during 2013–2021, respectively. | |
Contrary to the decreasing trend of O3 at SDZ, those at BJ, TJ, and SJZ showed the opposite variation with a lower level and a generally upward trend during 2015–2021. The lower levels of O3 in urban areas than that at the background station might be attributed to vehicle emissions. High concentrations of NO promote the reaction of O3 with NO that frequently occurs in urban areas in summer, which largely consumes O3.33 Similar to the other pollutants, the gap between the O3 concentrations at the background station and urban areas was relatively small in 2021, which might suggest that the O3 concentrations were uniform on a large regional scale. Recent research also indicated that the differences in urban and nonurban surface O3 were narrowing in the Northern Hemisphere.34,35
3.3 Meteorology-associated pollutant variabilities
Weather conditions change rapidly, resulting in variations in the concentration of air pollutants even when emissions do not change. Beneficial weather conditions with higher WS and PBLH levels may favor pollutant diffusion, whereas unfavorable weather conditions with higher RH and lower WS levels and more inversions may aggravate air pollution. After the weather normalization method was applied, the trends of monthly averaged pollutants before and after normalization (meteorology-adjusted) were achieved (Fig. S2†). Based on the observational and meteorology-adjusted data, the meteorology-associated value could be calculated. Fig. 5 shows the estimated temporal trend in the meteorology-associated pollutant levels at SDZ. The fluctuating annual variation ranged from −14.8 to 10.3%, −8.5 to 7.3%, −11 to 7.1%, −7.9 to 6.0%, and −7.4 to 7.3% for PM2.5, NO2, SO2, O3, and CO, respectively. The long-term trend of the annual meteorology-associated pollutants fluctuated at approximately 0, with decreasing trends (the meteorological conditions improved) in PM2.5, NO2, SO2, and CO and a slightly increasing (the meteorological conditions worsened) trend in O3. SO2 and CO exhibited the same interannual pattern with unfavorable meteorological conditions during 2013–2015, whereas opposite conditions occurred from 2016 to 2021. The variation patterns of PM2.5 and NO2 were slightly similar but not consistent with those of SO2 and CO. Moreover, 2018 and 2020 were two typical years during which the meteorological conditions were favorable for the air quality, with annual meteorology-associated pollutant anomalies of −10.1%, −3.8%, −5.0%, −1.1%, and −2.3% for PM2.5, NO2, SO2, O3, and CO, respectively, in 2018 and anomalies of −14.8%, −8.5%, −4.0%, −0.4%, and −5.3%, respectively, in 2020.
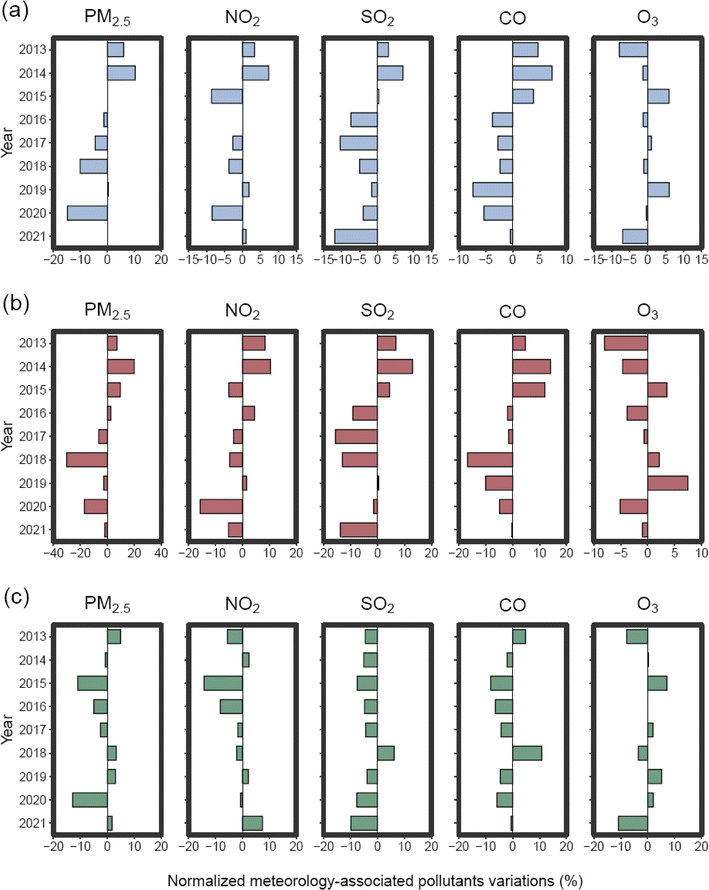 |
| Fig. 5 Relative impact of the meteorology on the annual average concentration of pollutants (a), the relative impact of meteorology on the average concentration of pollutants during the cold season (b), and the relative impact of meteorology on the average concentration of pollutants during the warm season (c). The meteorology-associated pollutants lower than 0 represent the meteorological conditions favorable to the pollutants reduction, and vice versa. | |
The effects of the meteorological conditions during the warm and cold seasons were also analyzed. Here, the warm season included March to August, while the cold season comprised January, February, and September to December for each year. As shown in Fig. 5, the annual effect of the meteorology on PM2.5, NO2, SO2, and CO was mainly dominated by the meteorological conditions during the cold season, when regional haze events frequently occurred. However, the effect on O3 largely depended on the meteorological conditions during the warm season, when photochemical pollution was common. Typical beneficial meteorological conditions during the cold season occurred in 2020, with effects on all pollutants less than 0. In a certain year (i.e., PM2.5 in 2016), the weather conditions during the cold season were unfavorable for pollution management, but because the weather conditions during the warm season were beneficial, the overall weather effect was still favorable. Similarly, the meteorological conditions during the warm season were unfavorable for pollution control, but since the meteorological conditions during the cold season were favorable, the annual meteorological effect was favorable for pollution control (i.e., PM2.5, SO2, and CO in 2018).
During the cold season, compared to those at the stage of the first Clean Air Action, the effects of the meteorological conditions were favorable for PM2.5 control at the stage of the second Clean Air Action. We calculated the East Asian winter monsoon (EAWM) index values from 2013 to 2021 (Fig. S3†). A positive/negative index value indicates a strong/weak EAWM. A strong EAWM could significantly control air pollution levels to benefit the air quality since pollutants are transported southward from north to south.36 The EAWM was weak from 2013 to 2016 and relatively strong from 2017 to 2020, which included the period of the second Clean Air Action. The highest index value appeared in 2018, especially in January and December, corresponding to the best weather conditions during the cold period from 2013 to 2021, leading to a 7.0 μg m−3 decrease in the meteorology-associated PM2.5 during the cold season of 2018. Additionally, the EAWM was strong in December 2020 with a relatively high index value, resulting in a 6.0 μg m−3 decrease relative to the model. On the other hand, large-scale atmospheric circulations could also influence pollutant concentration variations. The fall–winter meteorology in the northern part of eastern China was significantly unfavorable for pollution control in 2015 and 2016 with a strong El Niño phenomenon,21 and the meteorological effects were unfavorable for concentration decline until 2017. In the winter of 2017, the last implementation year of the first Clean Air Action, cold air activities were more frequent than before, which was conducive to pollutant diffusion,37 thereby contributing to the fulfillment of the Air Action targets in BTH.
Regarding O3 during the warm season, the meteorological conditions in 2013 and 2021 were beneficial for pollution control. Although many meteorological variables could influence the photochemical formation of O3, the prevailing variable should be T. This variable is highly related to the intensity of solar radiation, which plays a critical role in the photochemical formation of O3. The daily maximum T was selected and averaged to calculate the annual mean (Fig. S4†). T was lower in 2013 and 2021, with 1.3 °C and 1 °C lower values, respectively, than the average level during the study period, which is unfavorable for O3 formation. Overall, the favorable meteorological conditions during the warm seasons of 2013 and 2021 contributed 7.5 and 8.7 μg m−3 to the reductions during these periods, respectively. Apart from T, the meteorological factors such as WD, WS, and transport from the nocturnal residual layer could also affect O3 levels.
The different variations or trends of pollutants were influenced by meteorological factors; however, the different meteorological conditions could not completely explain the difference in the interannual and seasonal patterns of each pollutant since the interactions between pollutants were very complex. Considering only one or two meteorological parameters could hardly explain the fluctuations in all the pollutants at the different time scales. Further studies are needed to improve our knowledge of the mechanisms of the interannual and seasonal differences in the meteorology–pollution relationships.
3.4 Pollutant trends after adjusting the meteorological effects
The annual average meteorology-adjusted concentrations of pollutants and emission data from Multi-resolution Emission Inventory model for Climate and air pollution research (MEIC; https://meicmodel.org.cn) for the BTH region were normalized to 2013, as shown in Fig. 6. The MEIC is a modeling platform of atmospheric emissions from anthropogenic sources, developed from the Multi-resolution Emission Inventory for China.38 As shown in Fig. 6 and S5,† the meteorology-adjusted SO2 concentration was dramatically reduced and continued its downward trend from 2013, with an annual decline rate of −9.6% per year. The meteorology-adjusted SO2 clearly showed that the peak monthly concentration in winter decreased from 30.4 to 2.2 μg m−3 in December 2021, while the minimum monthly concentration in summer also declined during the study period. After adjusting for meteorological effects, the temporal variations in SO2 were very consistent with those in the pollutant emissions retrieved from the MEIC data. SO2 is mainly emitted by coal-fired sources. The remarkable decrease in SO2 was attributed to the employment of clean coal technologies enforced by the “Action Plan for Transformation and Upgrading of Coal Energy Conservation and Emission Reduction (2014–2020)”, in which tighter environmental standards targeting the emission concentrations at power plants prompted substantial SO2 reductions,39 and the replacement of residential coal heating with natural gas was promoted. Similar to SO2, CO continued its downward trend since 2013, leading to a −5.1% per year decrease. The declining pattern of the adjusted concentrations of CO was similar to that of emissions; however, the decrease rate was lower than the emission reduction rate. CO and CO2 are both emitted by combustion sources and could be regarded as trace products of inefficient and efficient combustion processes, respectively. By comparing the opposite long-term trends in CO and CO2 at SDZ, Li et al.40 suggested that improvements in the energy use efficiency and emission control led to the downward trend in CO.
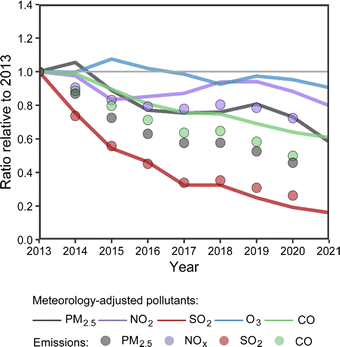 |
| Fig. 6 Normalized trend of the meteorology-adjusted air pollutant concentrations at SDZ and emissions of PM2.5, NOx, SO2 and CO. The emission data during 2013–2021 were obtained from https://meicmodel.org/. | |
In contrast to SO2 and CO, the meteorology-adjusted PM2.5, NO2, and O3 levels did not always maintain a downward trend, with average decrease rates of −4.7% per year, −1.3% per year, and −1.4% per year, respectively. As shown in Fig. 6, the fluctuation tendency of NO2 also differed from that of NOx emissions, and the ratio difference has remained larger since 2016. Compared to sulfur emission control, which drastically reduced the ambient SO2 concentration, NO2 still lacked effective emission control. Coal combustion is not only a major source of SO2 but also an important source of NOx. The different trends between these compounds indicate that other sources, such as traffic emissions or atmospheric processes, impose a greater effect on NO2 than coal combustion. For example, chemical depletion of nocturnal O3 mainly occurs through titration by NO–NO2, causing nonlinearity of the NO2–NOx relationships.41,42 Regarding vehicle emissions, the number of private gasoline-fueled cars will continue to increase in BTH, and the fuel quality standard and vehicle emission standard will be tightened approximately every 3–5 years. However, these two standards are not always upgraded simultaneously, which could increase NOx and other pollutants in this region.43
Similar to NO2, the variation in PM2.5 was not consistent with that in the emissions, with a peak in 2014, decreasing until 2018, rising in 2019, and declining again. The gap in the ratio difference between the emissions and meteorology-adjusted values of PM2.5 has also increased since 2016. Compared to the variations in the meteorology-adjusted concentrations with emissions, PM2.5 was more likely driven by NOx emission reduction after 2016. Due to emission control, the SO2 concentration decreased significantly, while the NO2 concentration far exceeded that of SO2 and continued to increase in Beijing in winter.44 On the other hand, the ammonia concentrations were much higher in the NCP.45–47 Due to effective sulfur emission control and abundant ammonia in the atmosphere, which provide a favorable environment for ammonium nitrate formation, the effectiveness of particle pollution control achieved through SO2 and NOx emission reduction was limited.48 Therefore, the PM2.5 concentrations did not show the same continued downward trend in this region as the MEIC emissions.
Regarding O3, the highest meteorology-adjusted O3 occurred in 2015. In the lower atmosphere, O3 is a secondary pollutant formed by the photochemical reaction of its precursors, which are organics such as volatile organic compounds (VOCs), CO, and NOx. In 2016, the 13th FYP for ecological and environmental protection and the policy on VOCs control technology issued by the government demanded 15% and 10% decreases in the NOx and VOCs emission amounts, respectively, in key regions of China. Corresponding to both policies, O3 decreased relative to 2013 after 2015. However, the O3 level in 2021 did not show an obvious decrease relative to that in 2013. Compared to NOx, VOCs control is difficult since non-organizational emissions are involved. Moreover, the relationships between O3 and its precursors are nonlinear, and aerosols could also impact tropospheric O3 through changes in atmospheric dynamics and photolysis rates.49 These factors could make O3 control more challenging.
3.5 Evaluating the effectiveness of the Clean Air Action policies
At the first Clean Air Action stage, a number of policies, control measures, and action plans were proposed, with a specific focus on the BTH, Yangtze River Delta, and Pearl River Delta areas, to reduce PM2.5 by 25%, 20%, and 15%, respectively. The details of the main mitigation measures implemented in China and key regions during this period are summarized in Zhang et al.7 and Zheng et al.50 The first Clean Air Action period showed significant reductions in PM2.5, NO2, SO2, O3, and CO, with observed concentrations of SDZ reducing by 24.6%, 13.0%, 67.8%, 1.5, and 24.2%, respectively, after weather normalization (Tables 1 and S2†). The reductions of NO2, SO2, and CO at SDZ were higher than those of average in 12 megacities in China.20 To further reduce air pollution, the second Clean Air Action implemented measures to strengthen pollutant emission reductions, such as promoting clean heating in winter in northern China, replacing coal with electricity and gas in more than 3 million households, eliminating all small coal-fired appliances, and controlling vehicle exhaust gas emissions. At the end of the second Clean Air Action stage, the observed concentrations of PM2.5, NO2, SO2, and CO were dramatically lower than those in 2013, with decreases of 27%, 11.7%, 80.9%, 4.8%, and 35.5% in PM2.5, NO2, SO2, O3, and CO, respectively, after meteorology correction (Table S2†).
Table 1 Comparison of the concentrations of pollutants before and after weather normalizationa
Year |
Observed concentration |
Meteorology-adjusted concentration |
PM2.5 |
NO2 |
SO2 |
O3 |
CO |
PM2.5 |
NO2 |
SO2 |
O3 |
CO |
Unit: μg m−3 for all pollutants, except CO (mg m−3).
|
2013 |
44.4 |
23.1 |
15.7 |
73.9 |
0.65 |
41.8 |
22.3 |
15.2 |
79.7 |
0.62 |
2014 |
49.1 |
23.4 |
12.4 |
78.4 |
0.66 |
44.0 |
21.7 |
11.5 |
79.5 |
0.62 |
2015 |
37.2 |
17.1 |
8.31 |
91.0 |
0.58 |
37.1 |
18.6 |
8.3 |
85.6 |
0.56 |
2016 |
31.8 |
19.0 |
6.56 |
80.2 |
0.49 |
32.2 |
19.0 |
7.0 |
81.2 |
0.5 |
2017 |
30.1 |
18.9 |
4.48 |
79.3 |
0.46 |
31.5 |
19.4 |
4.9 |
78.5 |
0.47 |
2018 |
28.8 |
20.2 |
4.71 |
72.9 |
0.46 |
31.7 |
20.9 |
4.9 |
73.7 |
0.47 |
2019 |
33.8 |
21.4 |
3.74 |
82.5 |
0.4 |
33.7 |
21 |
3.8 |
77.6 |
0.43 |
2020 |
26.5 |
18.1 |
2.81 |
75.6 |
0.38 |
30.5 |
19.7 |
2.9 |
75.9 |
0.40 |
In order to further evaluate the effect of actions, the Theil–Sen regression technique was performed on the observed and normalized data in this study. The advantage of using the Theil–Sen estimator is that it tends to yield accurate confidence intervals even with non-normal data and heteroscedasticity (non-constant error variance). The Theil–Sen function is provided by “openair” R packages. The normalized annual levels of air pollutants decreased obviously with median slopes of 1.7 μg m−3 year−1, 0.2 μg m−3 year−1, 1.5 μg m−3 year−1, 0.7 μg m−3 year−1, and 0.03 mg m−3 year−1 for PM2.5, NO2, SO2, O3, and CO, respectively (Fig. S6†). It is worth noting that the decreasing trends were significant except for NO2, indicating more attention should be paid to the emission reduction of it. Additionally, the annual observed concentrations of PM2.5, NO2, SO2, and CO decreased by 2.7 μg m−3 year−1, 0.3 μg m−3 year−1, 1.7 μg m−3 year−1, and 0.04 mg m−3 year−1, respectively, whereas the concentration of O3 increased by 0.03 μg m−3 year−1 (Fig. S6†). The decreasing trends of the meteorology-adjusted pollutant concentrations were weaker than those of the observed concentrations, suggesting that the meteorological conditions were favorable for pollution control, except for O3. That is to say, the contribution of meteorological conditions to the improvement of PM2.5, NO2, SO2, and CO were approximately 1.0 μg m−3 year−1, 0.1 μg m−3 year−1, 0.2 μg m−3 year−1, and 0.01 mg m−3 year−1. In contrast, the meteorological conditions negatively contributed to O3 reductions, as the magnitudes of the reductions decreased after meteorology adjustment, with 0.76 μg m−3 year−1. Li et al.51 fitted O3 to meteorological variables with a multiple linear regression model showed that meteorology played a significant role in the 2013–2019 O3 trend, contributing approximately 2.7 μg m−3 year−1 over NCP, which was much higher than that at SDZ. These results suggested that the impact of meteorological conditions should be considered in the control of pollutant level. In addition, previous studies demonstrated that the concentrations of pollutants at SDZ were not only affected by the emissions in BTH but also by those of south of the NCP.30,45 Therefore, the emission control strategies should be considered on a broader regional scale.
4 Conclusions and implications
In this study, we applied a machine-learning-based model to analyze the meteorology- and emission-driven variations in pollutant concentrations based on long-term data of a background station in northern China. The overall air quality significantly improved in BTH, with the annual mean concentrations of air pollutants at SDZ generally descending year by year during the study period.
The annual variations in the effects of the meteorological conditions on the annual mean concentrations of PM2.5, NO2, SO2, O3, and CO ranged from −14.8 to 10.3%, −8.5 to 7.3%, −11 to 7.1%, −7.9 to 6.0%, and −7.4 to 7.3%, respectively, during 2013–2021. The meteorology-adjusted SO2 and CO levels slashed dramatically and continued their downward trend from 2013, with annual decline rates of −9.6% per year and −5.1% per year during 2013–2021, respectively. Different from SO2 and CO, the meteorology-adjusted PM2.5, NO2, and O3 levels did not always maintain a downward trend, with average decrease rates of −4.7% per year, −1.3% per year, and −1.4% per year, respectively.
We further assessed the effectiveness of the two Clean Air Actions by removing the meteorological influences. Our results confirm that actions have led to a major improvement in the air quality at regional scale, with the reduction of 1.7 μg m−3 year−1, 0.2 μg m−3 year−1, 1.5 μg m−3 year−1, 0.7 μg m−3 year−1, and 0.03 mg m−3 year−1 for PM2.5, NO2, SO2, O3, and CO, respectively. Although emissions dominated the long-term variations in pollutants, the meteorological conditions obviously played a positive role during the two action periods for pollutants except for O3. This suggested that future target setting should take meteorology conditions into account. Since the reducing variation in PM2.5 had a large gap between that in the emission and the lower decrease rate of NO2 and O3 relative to that in 2013, a more comprehensive control strategy should be considered in Northern China.
Data availability
Data available on request.
Conflicts of interest
The authors declare that they have no known competing financial interests or personal relationships that could have appeared to influence the work reported in this paper.
Acknowledgements
The authors would like to acknowledge the staff of the SDZ station for their support in the data collection. This work was supported by the National Natural Science Foundation of China (Grant No. 42275188, 42177091, 42275199, 42207115, 42005094).
References
- M. B. Hadley, R. Vedanthan and V. Fuster, Air pollution and cardiovascular disease: a window of opportunity, Nat. Rev. Cardiol., 2018, 15, 193–194 CrossRef PubMed
.
- H. Fan, C. Zhao and Y. Yang, A comprehensive analysis of the spatio-temporal variation of urban air pollution in China during 2014–2018, Atmos. Environ., 2020, 220, 117066 CrossRef CAS
.
- J. Hu, Q. Ying, Y. Wang and H. Zhang, Characterizing multi-pollutant air pollution in China: Comparison of three air quality indices, Environ. Int., 2015, 84, 17–25 CrossRef CAS PubMed
.
- R.-J. Huang, Y. Zhang, C. Bozzetti, K.-F. Ho, J.-J. Cao, Y. Han, K. R. Daellenbach, J. G. Slowik, S. M. Platt, F. Canonaco, P. Zotter, R. Wolf, S. M. Pieber, E. A. Bruns, M. Crippa, G. Ciarelli, A. Piazzalunga, M. Schwikowski, G. Abbaszade, J. Schnelle-Kreis, R. Zimmermann, Z. An, S. Szidat, U. Baltensperger, I. E. Haddad and A. S. H. Prévôt, High secondary aerosol contribution to particulate pollution during haze events in China, Nature, 2014, 514, 218–222 CrossRef CAS PubMed
.
- P. Wang, H. Guo, J. Hu, S. H. Kota, Q. Ying and H. Zhang, Responses of PM2.5 and O3 concentrations to changes of meteorology and emissions in China, Sci. Total Environ., 2019, 662, 297–306 CrossRef CAS PubMed
.
- N. Cheng, B. Cheng, S. Li and T. Ning, Effects of meteorology and emission reduction measures on air pollution in Beijing during heating seasons, Atmos. Pollut. Res., 2019, 10, 971–979 CrossRef CAS
.
- Q. Zhang, Y. Zheng, D. Tong, M. Shao, S. Wang, Y. Zhang, X. Xu, J. Wang, H. He, W. Liu, Y. Ding, Y. Lei, J. Li, Z. Wang, X. Zhang, Y. Wang, J. Cheng, Y. Liu, Q. Shi, L. Yan, G. Geng, C. Hong, M. Li, F. Liu, B. Zheng, J. Cao, A. Ding, J. Gao, Q. Fu, J. Huo, B. Liu, Z. Liu, F. Yang, K. He and J. Hao, Drivers of improved PM2.5 air quality in China from 2013 to 2017, Proc. Natl. Acad. Sci. U. S. A., 2019, 116, 24463–24469 CrossRef CAS PubMed
.
- H. Zhang, H. Yuan, X. Liu, J. Yu and Y. Jiao, Impact of synoptic weather patterns on 24 h-average PM2.5 concentrations in the North China Plain during 2013–2017, Sci. Total Environ., 2018, 627, 200–210 CrossRef CAS PubMed
.
- M. O. P. Ramacher, V. Matthias, A. Aulinger, M. Quante, J. Bieser and M. Karl, Contributions of traffic and shipping emissions to city-scale NOx and PM2.5 exposure in Hamburg, Atmos. Environ., 2020, 237, 117674 CrossRef CAS
.
- K. R. Daellenbach, G. Uzu, J. Jiang, L.-E. Cassagnes, Z. Leni, A. Vlachou, G. Stefenelli, F. Canonaco, S. Weber, A. Segers, J. J. P. Kuenen, M. Schaap, O. Favez, A. Albinet, S. Aksoyoglu, J. Dommen, U. Baltensperger, M. Geiser, I. El Haddad, J.-L. Jaffrezo and A. S. H. Prévôt, Sources of particulate-matter air pollution and its oxidative potential in Europe, Nature, 2020, 587, 414–419 CrossRef CAS PubMed
.
- Z. Chen, D. Chen, C. Zhao, M.-p. Kwan, J. Cai, Y. Zhuang, B. Zhao, X. Wang, B. Chen, J. Yang, R. Li, B. He, B. Gao, K. Wang and B. Xu, Influence of meteorological conditions on PM2.5 concentrations across China: A review of methodology and mechanism, Environ. Int., 2020, 139, 105558 CrossRef CAS PubMed
.
- Y. Pan, S. Tian, Y. Zhao, L. Zhang, X. Zhu, J. Gao, W. Huang, Y. Zhou, Y. Song, Q. Zhang and Y. Wang, Identifying Ammonia Hotspots in China Using a National Observation Network, Environ. Sci. Technol., 2018, 52, 3926–3934 CrossRef CAS PubMed
.
- D. J. Jacob and D. A. Winner, Effect of climate change on air quality, Atmos. Environ., 2009, 43, 51–63 CrossRef CAS
.
- P. Hou and S. Wu, Long-term Changes in Extreme Air Pollution Meteorology and the Implications for Air Quality, Sci. Rep., 2016, 6, 23792 CrossRef CAS PubMed
.
- F. Yousefian, S. Faridi, F. Azimi, M. Aghaei, M. Shamsipour, K. Yaghmaeian and M. S. Hassanvand, Temporal variations of ambient air pollutants and meteorological influences on their concentrations in Tehran during 2012–2017, Sci. Rep., 2020, 10, 292 CrossRef CAS PubMed
.
- J. Xu, J. Li, X. Zhao, Z. Zhang, Y. Pan and Q. Li, Effectiveness of emission control in sensitive emission regions associated with local atmospheric circulation in O3 pollution reduction: A case study in the Beijing-Tianjin-Hebei region, Atmos. Environ., 2022, 269, 118840 CrossRef CAS
.
- Z. Ma, J. Xu, W. Quan, Z. Zhang, W. Lin and X. Xu, Significant increase of surface ozone at a rural site, north of eastern China, Atmos. Chem. Phys., 2016, 16, 3969–3977 CrossRef CAS
.
- S. Zhai, D. J. Jacob, X. Wang, L. Shen, K. Li, Y. Zhang, K. Gui, T. Zhao and H. Liao, Fine particulate matter (PM2.5) trends in China, 2013–2018: separating contributions from anthropogenic emissions and meteorology, Atmos. Chem. Phys., 2019, 19, 11031–11041 CrossRef CAS
.
- G. He, Y. Pan and T. Tanaka, The short-term impacts of COVID-19 lockdown on urban air pollution in China, Nat. Sustain., 2020, 3, 1005–1011 CrossRef
.
- Y. Guo, K. Li, B. Zhao, J. Shen, W. J. Bloss, M. Azzi and Y. Zhang, Evaluating the real changes of air quality due to clean air actions using a machine learning technique: Results from 12 Chinese mega-cities during 2013–2020, Chemosphere, 2022, 300, 134608 CrossRef CAS PubMed
.
- Q. Xiao, Y. Zheng, G. Geng, C. Chen, X. Huang, H. Che, X. Zhang, K. He and Q. Zhang, Separating emission and meteorological contributions to long-term PM2.5 trends over eastern China during 2000–2018, Atmos. Chem. Phys., 2021, 21, 9475–9496 CrossRef CAS
.
- S. K. Grange, D. C. Carslaw, A. C. Lewis, E. Boleti and C. Hueglin, Random forest meteorological normalisation models for Swiss PM10 trend analysis, Atmos. Chem. Phys., 2018, 18, 6223–6239 CrossRef CAS
.
- A. Ziegler and I. R. König, Mining data with random forests: current options for real-world applications, Wiley Interdiscip. Rev.: Data Min. Knowl. Discov., 2014, 4, 55–63 Search PubMed
.
- S. K. Grange and D. C. Carslaw, Using meteorological normalisation to detect interventions in air quality time series, Sci. Total Environ., 2019, 653, 578–588 CrossRef CAS PubMed
.
- X. Chen, X. Li, J. Liang, X. Li, S. Li, G. Chen, Z. Chen, S. Dai, J. Bin and Y. Tang, Causes of the unexpected slowness in reducing winter PM(2.5) for 2014-2018 in Henan Province, Environ. Pollut., 2023, 319, 120928 CrossRef CAS PubMed
.
- T. V. Vu, Z. Shi, J. Cheng, Q. Zhang, K. He, S. Wang and R. M. Harrison, Assessing the impact of clean air action on air quality trends in Beijing using a machine learning technique, Atmos. Chem. Phys., 2019, 19, 11303–11314 CrossRef CAS
.
- Z. Shi, C. Song, B. Liu, G. Lu, J. Xu, T. Van Vu, R. J. R. Elliott, W. Li, W. J. Bloss and R. M. Harrison, Abrupt but smaller than expected changes in surface air quality attributable to COVID-19 lockdowns, Sci. Adv., 2021, 7, eabd6696 CrossRef CAS PubMed
.
- N. K. Colombi, D. J. Jacob, L. H. Yang, S. Zhai, V. Shah, S. K. Grange, R. M. Yantosca, S. Kim and H. Liao, Why is ozone in South Korea and the Seoul metropolitan area so high and increasing?, Atmos. Chem. Phys., 2023, 23, 4031–4044 CrossRef CAS
.
- M. Diaz Resquin, P. Lichtig, D. Alessandrello, M. De Oto, D. Gómez, C. Rössler, P. Castesana and L. Dawidowski, A machine learning approach to address air quality changes during the COVID-19 lockdown in Buenos Aires, Argentina, Earth Syst. Sci. Data, 2023, 15, 189–209 CrossRef
.
- W. Pu, X. Shi, L. Wang, J. Xu and Z. Ma, Potential source regions of air pollutants at a regional background station in Northern China, Environ. Technol., 2019, 40, 3412–3421 CrossRef CAS PubMed
.
- L. R. F. Henneman, H. A. Holmes, J. A. Mulholland and A. G. Russell, Meteorological detrending of primary and secondary pollutant concentrations: Method application and evaluation using long-term (2000–2012) data in Atlanta, Atmos. Environ., 2015, 119, 201–210 CrossRef CAS
.
- J. Cheng, J. Su, T. Cui, X. Li, X. Dong, F. Sun, Y. Yang, D. Tong, Y. Zheng, Y. Li, J. Li, Q. Zhang and K. He, Dominant role of emission reduction in PM2.5 air quality improvement in Beijing during 2013–2017: a model-based decomposition analysis, Atmos. Chem. Phys., 2019, 19, 6125–6146 CrossRef CAS
.
- W. Li, L. Shao, W. Wang, H. Li, X. Wang, Y. Li, W. Li, T. Jones and D. Zhang, Air quality improvement in response to intensified control strategies in Beijing during 2013–2019, Sci. Total Environ., 2020, 744, 140776 CrossRef CAS PubMed
.
- H. Han, L. Zhang, Z. Liu, X. Yue, L. Shu, X. Wang and Y. Zhang, Narrowing Differences in Urban and Nonurban Surface Ozone in the Northern Hemisphere Over 1990–2020, Environ. Sci. Technol. Lett., 2023, 10, 410–417 CrossRef CAS
.
- J. Massagué, M. Escudero, A. Alastuey, E. Mantilla, E. Monfort, G. Gangoiti, C. P. García-Pando and X. Querol, Spatiotemporal variations of tropospheric ozone in Spain (2008–2019), Environ. Int., 2023, 176, 107961 CrossRef PubMed
.
- J. I. Jeong and R. J. Park, Winter monsoon variability and its impact on aerosol concentrations in East Asia, Environ. Pollut., 2017, 221, 285–292 CrossRef CAS PubMed
.
- X. Zhang, X. Xu, Y. Ding, Y. Liu, H. Zhang, Y. Wang and J. Zhong, The impact of meteorological changes from 2013 to 2017 on PM2.5 mass reduction in key regions in China, Sci. China Earth Sci., 2019, 62, 1885–1902 CrossRef CAS
.
- B. Zheng, Q. Zhang, G. Geng, C. Chen, Q. Shi, M. Cui, Y. Lei and K. He, Changes in China’s anthropogenic emissions and air quality during the COVID-19 pandemic in 2020, Earth Syst. Sci. Data, 2021, 13, 2895–2907 CrossRef
.
- V. J. Karplus, S. Zhang and D. Almond, Quantifying coal power plant responses to tighter SO2 emissions standards in China, Proc. Natl. Acad. Sci., 2018, 115, 7004–7009 CrossRef CAS PubMed
.
- Y. Li, Z. Ma, T. Han, W. Quan, J. Wang, H. Zhou, D. He and F. Dong, Long-term declining in carbon monoxide (CO) at a rural site of Beijing during 2006–2018 implies the improved combustion efficiency and effective emission control, J. Environ. Sci., 2022, 115, 432–442 CrossRef PubMed
.
- X. Zhu, Z. Ma, Z. Li, J. Wu, H. Guo, X. Yin, X. Ma and L. J. A. E. Qiao, Impacts of meteorological conditions on nocturnal surface ozone enhancement during the summertime in Beijing, Atmos. Environ., 2020, 225, 117368 CrossRef CAS
.
- N. R. Awang, N. A. Ramli, A. S. Yahaya and M. Elbayoumi, High Nighttime Ground-Level Ozone Concentrations in Kemaman: NO and NO2 Concentrations Attributions, Aerosol Air Qual. Res., 2015, 15, 1357–1366 CrossRef CAS
.
- J. Wang, Q. Wu, J. Liu, H. Yang, M. Yin, S. Chen, P. Guo, J. Ren, X. Luo, W. Linghu and Q. Huang, Vehicle emission and atmospheric pollution in China: problems, progress, and prospects, PeerJ, 2019, 7, e6932 CrossRef PubMed
.
- Y. Xie, G. Wang, X. Wang, J. Chen, Y. Chen, G. Tang, L. Wang, S. Ge, G. Xue and Y. J. C. G. Wang, Nitrate-dominated PM 2.5 and elevation of particle pH observed in urban Beijing during the winter of 2017, Atmos. Chem. Phys., 2020, 5019–5033 CrossRef CAS
.
- W. Pu, Z. Ma, J. L. Collett Jr, H. Guo, W. Lin, Y. Cheng, W. Quan, Y. Li, F. Dong and D. He, Regional transport and urban emissions are important ammonia contributors in Beijing, China, Environ. Pollut., 2020, 265, 115062 CrossRef CAS PubMed
.
- M. Van Damme, L. Clarisse, S. Whitburn, J. Hadji-Lazaro, D. Hurtmans, C. Clerbaux and P.-F. Coheur, Industrial and agricultural ammonia point sources exposed, Nature, 2018, 564, 99–103 CrossRef CAS PubMed
.
- W. Pu, J. Sheng, P. Tian, M. Huang, X. Liu, J. L. Collett, Z. Li, X. Zhao, D. He, F. Dong, N. Zhang, W. Quan, Y. Qiu, Y. Song, W. Lin, Y. Pan and Z. Ma, On-road mobile mapping of spatial variations and source contributions of ammonia in Beijing, China, Sci. Total Environ., 2023, 864, 160869 CrossRef CAS PubMed
.
- X. Fu, S. Wang, J. Xing, X. Zhang, T. Wang and J. Hao, Increasing Ammonia Concentrations Reduce the Effectiveness of Particle Pollution Control Achieved via SO2 and NOX Emissions Reduction in East China, Environ. Sci. Technol. Lett., 2017, 4, 221–227 CrossRef CAS
.
- J. Xing, J. Wang, R. Mathur, S. Wang, G. Sarwar, J. Pleim, C. Hogrefe, Y. Zhang, J. Jiang, D. C. Wong and J. Hao, Impacts of aerosol direct effects on tropospheric ozone through changes in atmospheric dynamics and photolysis rates, Atmos. Chem. Phys., 2017, 17, 9869–9883 CrossRef CAS PubMed
.
- B. Zheng, D. Tong, M. Li, F. Liu, C. Hong, G. Geng, H. Li, X. Li, L. Peng, J. Qi, L. Yan, Y. Zhang, H. Zhao, Y. Zheng, K. He and Q. Zhang, Trends in China's anthropogenic emissions since 2010 as the consequence of clean air actions, Atmos. Chem. Phys., 2018, 18, 14095–14111 CrossRef CAS
.
- K. Li, D. J. Jacob, L. Shen, X. Lu, I. De Smedt and H. Liao, Increases in surface ozone pollution in China from 2013 to 2019: anthropogenic and meteorological influences, Atmos. Chem. Phys., 2020, 20, 11423–11433 CrossRef CAS
.
|
This journal is © The Royal Society of Chemistry 2024 |
Click here to see how this site uses Cookies. View our privacy policy here.