Real-time detection and classification of PFAS using dynamic behaviors at liquid–liquid interfaces†
Received
13th April 2024
, Accepted 4th May 2024
First published on 16th May 2024
Abstract
Rapid detection and classification of per- and polyfluoroalkyl substances (PFAS) are important for monitoring their concentrations at potential contamination sites due to their severe impact on environmental and health safety. Herein, we present a combination of Janus droplets and microfluidics-based sensors to measure dynamic interfacial behaviors of PFAS at liquid–liquid interfaces. The time-series data are used as chemical fingerprints to classify the identity of PFAS based on their differences in chain length and head group and quantify their concentration. We demonstrate that classification of four different PFAS is possible using the time-series data of under ten minutes. We also extend this proof-of-concept work toward complex matrices of synthetic groundwater and binary mixtures of PFAS. Our results illustrate the potential of a real-time and continuous sensing platform for on-site environmental monitoring.
Introduction
Per- and polyfluoroalkyl substances (PFAS) are amphiphilic molecules that adsorb onto surfaces or interfaces, leading to widespread and long-term contamination of water supplies.1–6 These molecules have been linked to substantial environmental impacts, and consumption of contaminated water poses adverse health effects.7–10 Such problems are especially amplified in remote communities with insufficient infrastructures and increased reliance on unregulated water sources.11–13 Thus, efforts to monitor the transport of PFAS are critically important to the maintenance of a safe living standard. While conventional laboratory-based techniques (e.g., high-performance liquid chromatography with tandem mass spectroscopy, LC-MS/MS) are highly selective and sensitive,14–16 they are cost-prohibitive as they require well-equipped laboratories and skilled personnel, unsuitable for on-site applications, and are not readily accessible.15,17,18 Furthermore, these techniques are not compatible for real-time detection to fully evaluate the presence of PFAS in water sources, or regular monitoring of water treatment plants, Hence, an alternative detection that is rapid, continuous, and portable could eliminate the need for sample transportation to centralized laboratories and provide real-time monitoring of PFAS in remote settings.19,20
In addition to rapid detection, classification of PFAS is integral to a comprehensive understanding of the transport mechanism, identification of the sources, and assessment of differences in toxicity.21,22 PFAS encompass an expansive array of compounds with different molecular structures (e.g., length of the alkyl chain, identity of the head group), which give rise to differences in physical and chemical properties (e.g., bioaccumulation, toxicity).4,23,24 For example, long-chain PFAS exhibit higher tendency to bioaccumulate, while short-chain PFAS have been shown to be more mobile and potentially volatile.25–27 Recent studies on the differences in human risk factors arising from long-chain and short-chain PFAS also underscore the importance of early identification of these contaminants.28–34 Moreover, the functional head groups (e.g., sulfonic acid, carboxylic acid, alcohol) significantly impact their environmental transports—namely, the adsorption at water–air interface, sorption in soil, and leaching into water sources.35–40
Our group and others have leveraged the differential interfacial properties of PFAS toward detection and quantification.18,37,39–42 For example, Brusseau and coworkers reported the effects of varying chemical structures on the fate and transport of PFAS in environmentally relevant media.23,39,43 Similarly, McCray and coworkers explored the interfacial behavior to evaluate the competitive adsorption of PFAS mixtures.44,45 We have demonstrated that PFAS can be distinguished from common hydrocarbon surfactants by monitoring interfacial tensions (γ) at multiple liquid–liquid interfaces.18 Specifically, the identity and concentration of PFAS dictate its interfacial behavior at multiple oil–water interfaces. A key limitation to our previously reported method is the inability to identify the type of PFAS and quantify its concentration simultaneously. Additionally, many geochemical factors (e.g., total dissolved solid, organic compounds, interaction with other surfactants) affect the interfacial partitioning, surface excess, and the effectiveness of PFAS.43,46–48 However, recent studies have demonstrated that monitoring the dynamic interfacial properties and extracting the adsorption and desorption kinetics could be used to identify the head group and chain-length of common PFAS.23,37,40,49,50 Hence, the primary goal of this study was to develop a high-throughput method to monitor changes in interfacial tension upon exposure to PFAS.
Janus droplets, comprising two distinct dispersed phases of hydrocarbon oil and fluorocarbon oil in an aqueous phase, are a class of all-liquid sensing particles whose physical and optical properties are directly dependent on the changes in interfacial tensions.51,52 Through subtle alterations in their interfacial tensions, they become sensitive probes, capable of detecting and responding to changes in their surrounding environment. Unlike conventional tensiometers that offer insights solely into equilibrium interfacial tensions at a single interface, Janus droplets are capable of monitoring dynamic interfacial tensions across multiple interfaces simultaneously and can differentiate between hydrocarbon and fluorocarbon surfactant.18 When incorporated with responsive surfactants that are selective to targeted analytes, these droplets have been tailored to detect enzymes,53,54 foodborne pathogens,55–57 dissolved metals in water,17,58,59 and trace chemicals.60 We adopted an optical detection technique known as “directional emission” that correlates interfacial tensions to the fluorescent emission of Janus droplets.17,18,55,56,59 Specifically, small changes in interfacial tensions significantly influence the Janus droplet morphology, as well as the direction and intensity of the emitted fluorescent light. This coupling of properties remains consistent in various buffer solutions, synthetic groundwaters, mixtures of surfactants, and even chicken exudate. We have also combined Janus droplets with PDMS-based microfluidics for the continuous measurement of fluorescent emission as a function of interfacial tension changes in real-time.61
In this study, we reported that real-time measurement of changes in interfacial tensions—captured from the integration of Janus droplets and a microfluidics-based sensing platform—provide sufficient distinguishing power to identify and quantify the concentration of four common PFAS (i.e., PFOS, PFOA, PFBS, and PFBA). Specifically, we used the interfacial behaviors of PFAS at hydrocarbon oil/water and fluorocarbon oil/water interfaces of Janus droplets as the real-time sensing readouts. Our approach relies on monitoring the interfacial activity, manifested through distinct morphological and optical changes in Janus droplets. We used PDMS-based microfluidics to expose Janus droplets to the four PFAS at different concentrations and measured the fluorescent emission in real-time as their “chemical fingerprints”. We then extracted key features from these time-series datasets and applied Principal Component Analysis (PCA) to perform an unsupervised classification. The results revealed that the two primary principal components described 95% of the total variances and facilitated grouping of PFAS with minimal overlaps. Additionally, we adopted Random Forest analysis, a supervised classification model, and regression modeling to predict the identity and concentration of PFAS. Lastly, we extended this proof-of-concept technique toward complex matrices of synthetic groundwater and binary mixtures of PFAS to simulate real-world conditions. Our results offer a step towards a novel approach for PFAS classification, highlighting its potential for practical applications in environmental monitoring and sensing technologies.
Experimental design
Fabrication and characterization of Janus droplets
We used Janus droplets comprising two hemispherical domains of hydrocarbon oil (H-oil) and fluorocarbon oil (F-oil) as the sensing particles (Fig. 1a). We fabricated them using a thermally induced phase separation method as previously reported in literature to produce droplets with high uniformity in both composition and size (ESI†).52,53,61 Briefly, equivalent volumes of H-oil (toluene) containing the dissolved fluorescent dye (perylene) and F-oil (9
:
1 mixture of HFE-7500 and FC-43) were emulsified within an aqueous solution containing a surfactant (Triton X-100) inside a Dolomite Microfluidic Setup. The whole process occurred at an elevated temperature above the upper critical temperature (Tc) of the two oils to produce a single miscible dispersed phase. We controlled the overall size of the droplets by adjusting the flow rates of the continuous phase (Triton solution) and the dispersed phase (miscible oil mixture) through a flow-focusing chip with a channel size of 50 μm. After emulsification and cooling to ambient temperature (T < Tc), the two oil phases separated, yielding monodispersed Janus droplets with distinct H-oil and F-oil phases. We characterized the droplets using optical and fluorescent microscopy to ensure monodispersity in size (51 ± 4 μm) and composition (ESI,† Fig. S1).
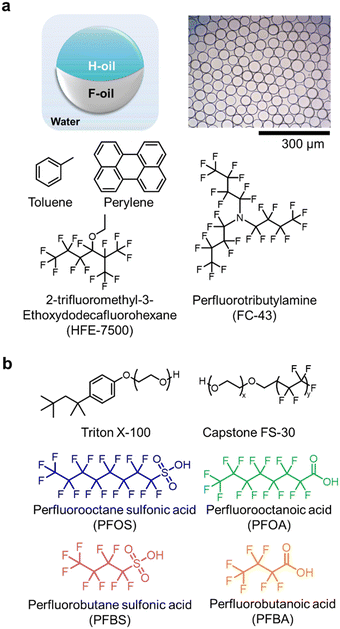 |
| Fig. 1 (a) Side-view schematic, top-view optical micrograph of Janus droplets, and chemical structures of oils and fluorescent dye. (b) Chemical structures of the control surfactants (Triton X-100 and Capstone FS-30) and the four selected PFAS: PFOS, PFOA, PFBS, and PFBA. | |
Preparation of analyte solutions
We selected four molecules of PFAS—perfluorooctane sulfonic acid (PFOS), perfluorooctanoic acid (PFOA), perfluorobutane sulfonic acid (PFBS), and perfluorobutanoic acid (PFBA)—to test the hypothesis that the variation in chemical structures (i.e., chain length, head group) impact the dynamic interfacial behaviors at the interfaces of Janus droplets (Fig. 1b). Specifically, we sought to leverage the differential adsorption and desorption kinetics at the liquid–liquid interfaces for classification.25,44,62,63 We chose two non-ionic surfactants, Triton X-100 and Capstone FS-30 at a mass ratio of 2
:
1, to stabilize the Janus droplets as they have minimal interaction with anionic surfactants (i.e., PFAS).43,47,64,65 The stock solutions of the analytes were first prepared in either Milli-Q water or synthetic groundwater (SGW). The full list of chemical compounds in SGW was adapted from Smith et al.66 and is provided in the ESI,† Table S1. The subsequent dilution series were prepared using these stock solutions in HDPE vials prior to each experiment.
Sensing mechanism of Janus droplets
We measured the real-time changes in interfacial tensions, induced by exposure to PFAS solutions, by measuring the changes in the fluorescent emission intensity from a single-layer array of Janus droplets. This coupled relationship among the chemical properties (interfacial tensions), the physical properties (droplet morphology), and optical properties (fluorescent emission) was first reported by Swager and coworkers56 and has been used by our group17,18,61 and others55,57–60,67–69 as the sensing mechanism for a variety of analytes. Briefly, the direction (and measured intensity) of the emissive light of a fluorophore (perylene) is affected by the internal morphology of Janus droplets. This internal morphology is controlled by the balance between interfacial tensions at the two interfaces: H-oil/water (γH) and F-oil/water (γF). As a result, the reduction in γF upon the exposure to fluorinated surfactant molecules (e.g., PFAS) alters the morphologies in a way that increases the emission intensity (Fig. 2a). Alternatively, the removal of PFAS reverses the morphology and decreases the emission intensity. An in-depth explanation of this sensing mechanism (previously described as “directional emission”) is provided in the ESI,† Figure S2.18,55,56 Because both the physical and optical transformations occur reversibly and dynamically, we have demonstrated previously that real-time chemical information can be extracted rapidly and continuously by combining Janus droplets with PDMS-based microfluidics platform (described in the next section).61,70,71
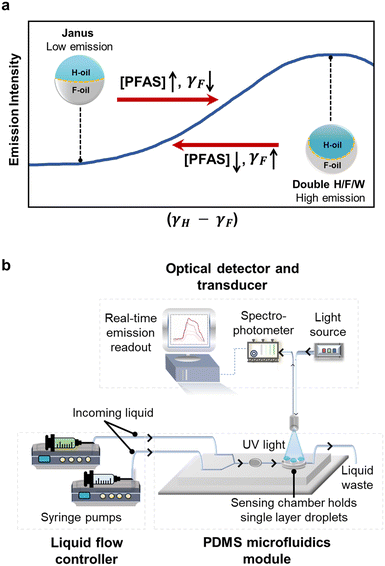 |
| Fig. 2 (a) Schematic representation of the emission intensity as a function droplet morphology and the differences between γH and γF. (b) Schematic diagram of the PDMS-based microfluidics sensing setup for real-time and continuous emission measurement of Janus droplets. | |
Fabrication of microfluidics setup for real-time detection
Similar to our previous report, we combined Janus droplets with PDMS-based microfluidics to measure real-time and continuous changes in interfacial tensions (Fig. 2b).61 Specifically, we fabricated a PDMS reservoir to host a single layer of monodispersed Janus droplets. This reservoir allows the Janus droplets to remain static and gravity-aligned vertically without agitation from the flow of analytes. The fabrication procedures were adapted from established protocols and are provided in the ESI,† Fig. S3 and S4.†72–74 We then controlled the ratio of the flow rates from the two syringe pumps—filled with the control solution (Triton and Capstone) and the PFAS solution (PFAS, Triton, and Capstone)—to expose Janus droplets to the targeted concentration of PFAS in a square-wave pattern. That is, we first established the baseline emission intensity using the control solution, then started the exposure to the analyte solution for three minutes, and finally cycled back to the control solution. Directly above the reservoir containing Janus droplets, a bifurcated optical fiber transmits UV light (λ = 405 nm) to excite the embedded fluorophore and collects real-time emission spectra via a spectrophotometer. We recorded the emission intensity as a function of time at one of the characteristic emission wavelengths of perylene (λ = 475 nm). This process was repeated for all the selected PFAS at varying concentrations.
Analysis and classification
To leverage the fluorescent emission intensity over time as a chemical fingerprint, we extracted four key features to serve as critical indicators. Specifically, for each time-series curve, we extracted (1) the magnitude of increase in emission intensity upon the adsorption of PFAS (Iads or F1), (2) the magnitude of decrease in intensity upon the desorption of PFAS (Ides or F2), (3) the response time during adsorption (tads or F3), and (4) the recovery time during desorption (tdes or F4). We chose these features because F1 and F2 represent the equilibrium values of interfacial tensions, which can be related to PFAS affinity and reversibility at the droplet interfaces. The time features (F3 and F4) represent the kinetic behaviors and the competitions between PFAS and the control surfactants.
We then employed Principal Component Analysis (PCA) as an unsupervised classification to distinguish the four selected PFAS. PCA has been widely used for the discrimination of toxic gases,75 various insecticides in different food matrices,76 protein biomarkers, and cancer cells.77 This approach produces a transformation of a data set to a new coordinate system such that each coordinate (or principal component) accounts for the greatest variance in the data set. As a result, the sensing responses are grouped based on similarity of the key features without any supervision. Additionally, we conducted a feature-by-feature importance analysis to comprehend the contributions of extracted features to the classification process.77–79
Next, we adopted Random Forest (RF) classification, a supervised classification algorithm that constructs multiple decision trees based on feature information for building the random forest and then combine the outcome of each tree to predict analyte class.78–80 RF is an effective classification model even with limited feature information and a small dataset size.81 It has been effectively used for practical applications, such as speciation and classification of volatile organic compounds in groundwater,82 identification of redox-sensitive contaminants in groundwater,83 and prediction of protein-peptide binding regions for drug discovery.84 We designed the algorithm to randomly select training and test datasets and evaluate the prediction accuracy of PFAS class. Subsequently, we performed linear regression analysis to develop a probabilistic statistical model for determining the PFAS concentration quantitatively. The analysis was conducted utilizing the scikit-learn package of Python programming (ESI†), wherein a tabular data structure was formulated such that each row of the dataset represented the concentration of a PFAS compound, while each column denoted a relevant feature extracted for analysis.
Results and discussion
Generation of real-time responses
The central hypothesis of this work was that the dynamic interfacial behaviors of PFAS are directly related to their chemical structure and concentrations. Specifically, the overall magnitudes and rates of change in interfacial tensions during adsorption and desorption from the liquid–liquid interface of Janus droplets could provide sufficient discriminating power to classify PFAS of different functional head group and alkyl-chain length. To test our hypothesis, we began by generating characteristic time-series responses to the exposure of the four individual PFAS at different concentrations. We leveraged a sensing platform comprising Janus droplets embedded in a PDMS-based microfluidic device.61,70 This sensing platform provides a rapid and continuous measurement of fluorescent emission intensity from Janus droplets, which is directly correlated to the interfacial tension and the concentration of the targeted analyte.17,18,56,57,60,67,68 For each experiment, we collected the emission intensity as a function of time at a characteristic wavelength of perylene (λ = 475 nm) as the Janus droplets were exposed to PFAS solutions. The duration and concentration of PFAS exposure were adjusted by tuning the ratio of the flowrates of the two syringe pumps upstream from the Janus droplets, containing the control solution and PFAS solution. Furthermore, we note that PFAS preferentially adsorbs at the F-oil/water interface rather than going into the oil phase, thus the influence of PFAS on the fluorescent dye molecules dissolved within the hydrocarbon oil phase is minimal. This phenomenon arises due to the affinity of PFAS compounds towards the oil/water interface, where they preferentially accumulate. This selective accumulation ensures that the dye molecules remain significantly unaffected by the presence of PFAS, thereby preserving the sensitivity and accuracy of the sensing mechanism based on directional emission.
Fig. 3a shows the design of our experiment, expected droplet morphologies, and a representative real-time emission data for a cycle of exposure. We designed a three-step process that started with (1) a flow of the control solution, (2) followed by a flow of PFAS solution, and (3) reverted to the control solution. Each step was kept consistent at a three-minute interval. We maintained a constant total flow rate (200 μL min−1) to ensure minimal mechanical agitation to the array of Janus droplets.61 While changing solutions from control to PFAS, we observed consistent time delay (td ≈ 25 ± 3 seconds), corresponding to the time it takes for the solution to travel from the syringe pumps to the sensing chamber (ESI†). In step 1, the values of γF and γH are similar in the control solution with both Triton and Capstone, resulting in a Janus morphology with dispersed, low emission intensity.56 Upon the exposure to PFAS solution in step 2, the value of γF reduced more significantly than γH, transforming Janus droplets into double (H/F/W) configuration and produced higher emission intensity. And in step 3, the PFAS solution was replaced with the control solution, which causes the two interfacial tensions to converge back to a similar value and reducing the emission intensity.
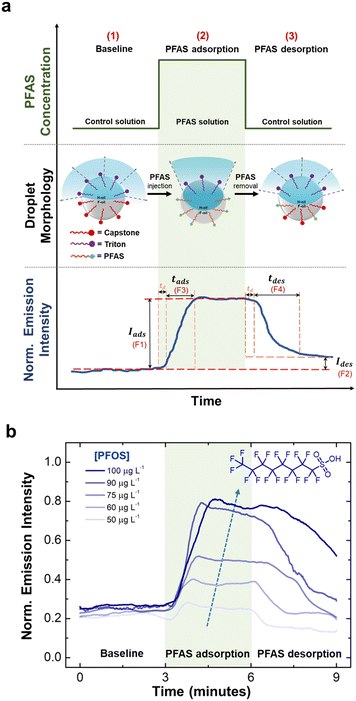 |
| Fig. 3 (a) Graphical representation of real-time emission response of PFAS generated by applying three-step square wave input flow. Shaded area signifies the injection of PFAS solutions from t = 3 min to t = 6 min. (b) Real-time emission responses to different concentrations of PFOS in Milli-Q water. Each response is an averaged response from separate experiments (N ≥ 5). | |
Fig. 3b demonstrates the real-time emission responses to PFOS with the concentration range of 50 to 100 μg L−1. We observed a direct relationship between change in emission intensity (F1) and concentration of PFOS. Specifically, high concentration of PFOS caused larger reduction in γF,18 which ultimately transformed Janus droplets into double (H/F/W) configuration with higher emission intensity.17,18,56,61 This observation agreed well with our group's discrete (ex situ) measurements of droplet morphology18 and previously reported measurement of interfacial tensions as a function of PFOS concentration.36,39,43,46 We note here that the sensitivity range can be adjusted by tuning the overall concentration of the control solution. By reducing the concentration of control surfactants (Triton and Capstone) used to stabilize the Janus droplets, we are able to lower the experimental limit of detection by 10 to 100 folds, as demonstrated previously18 (ESI,† Fig. S5). We also observed the dependency on concentration for the change in emission intensity during desorption (F2): the higher the concentration, the higher the F2 value. This observation suggests that Capstone (in the control solution) is less effective at replacing PFOS molecules from the F-oil/water interface as the concentration of PFAS increases. Moreover, it indicates that the desorption of PFOS was notably slower compared to the adsorption process which hindered the complete replacement of PFOS by the control solution within the designated three-minute time interval. We note here that increasing the flushing duration beyond three-minute would allow the droplets to revert to their initial morphological state. This, in turn, would have facilitated the reusability of the Janus droplet layer for subsequent PFOS detection purposes. We then looked at the dynamic response (F3 and F4), which revealed that both are similarly dependent on concentration. Specifically, both F3 and F4 values indicated that longer time is necessary for the droplets to attain equilibrium state at higher PFOS concentration.85–87
Characterization of PFAS using real-time responses
After obtaining the real-time characteristic curve for PFOS at varying concentrations, we repeated the same experimental procedures for PFOA, PFBS, and PFBA. The emission intensity vs. time for these PFAS are provided in ESI,† Fig. S6. Similar to PFOS, we observed a direct relationship between larger changes to emission intensities (F1 and F2) and higher concentration (Fig. 4a and b). Again, this observation agrees well with previous literature values that all the four PFAS reduce surface and interfacial tensions more readily at higher concentration.39 However, we clearly observed the differences in values of F1 among PFAS at a constant concentration. This observation is attributed to the variation in the surfactant effectiveness. Long-chain PFAS exhibited greater interfacial activity compared to short-chain PFAS, arising from the stronger hydrophobicity of long-chain PFAS.23,39 We observed minimal differences between F1 values for sulfonic acid and carboxylic acid head group of same chain length and same concentration, which were consistent with previous studies.23,36,39,43,46
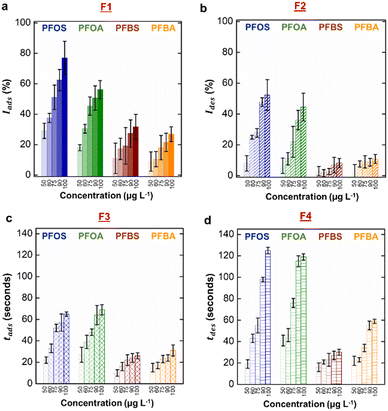 |
| Fig. 4 Average values and standard deviations (N ≥ 3) of the four extracted key features (a) Iads (F1), (b) Ides (F2), (c) tads (F3), and (d) tdes (F4) for the four individual PFAS in the concentration range from 50 to100 μg L−1 in Milli-Q water. | |
We note here that our measurement of F1 exemplified the limitation in simply using the equilibrium interfacial tensions for classification, as it is impossible to deconvolute two unknowns (identity and concentration) from just one measurement. We observed that the changes in emission intensity during desorption (F2) were more sensitive to varying concentrations for long-chain PFAS (Fig. 4b). We attributed this observation to their strong affinity toward the F-oil/water interface and increased resistance to desorption from the interface.88–91 Low values of F2 for short-chain PFAS also indicated significant removal from the interface due to their high mobility and reversible interfacial adsorption.91,92 For this same reason, we observed a weak dependency of response time during adsorption (F3) and recovery time during desorption (F4) on concentration for short-chain PFAS (Fig. 4c and d). In contrast, long-chain PFAS exhibited longer response and recovery times, which are strong functions of concentration. We also noted that their recovery times (F4) were significantly longer than their response times (F3).
Classification and quantification analysis using real-time responses
After extracting the four key features (F1–F4), we performed statistical analysis in two steps. The first step is classification analysis to identify and categorize PFAS and the second step is regression modeling that focuses on quantifying the concentration of the identified PFAS within the samples. We pursued two classification approaches: dimension reduction through Principal Component Analysis (PCA) and Random Forest (RF) supervised classification analysis. First, we subjected the four key features to PCA and transformed the original data into a new coordinate system, where each coordinate (principal component) represents the variations in the data set while preserving as much information as possible.93,94 This transformation is achieved by calculating eigenvectors of the covariance matrix of the data. Fig. 5a demonstrates the class separability of the four PFAS. We observed that the first two principal components captured the majority of the variance in the data set (ESI,† Fig. S7). We also observed a clear distinction between the two short-chain PFAS (PFBS and PFBA), indicating notable differences in their interfacial behavior. However, the long-chain PFAS (PFOS and PFOA) exhibited some degrees of overlap. We suspected that the dominance of hydrophobic tail diminishes the impact of head group variation for the long-chain PFAS. Interestingly, we observed a minor degree of overlap between PFOS and PFBA. This unexpected discrepancy can be attributed to the potential misinterpretation of data between low concentration of PFOS and high concentration of PFBA. Furthermore, we conducted a feature-by-feature importance analysis to elucidate the relative contribution of the four features to the classification process (Fig. 5b). We found that Ides (F2) emerged as the most important distinguishing factor across all PFAS classes, which suggests PFAS desorption can be further investigated for better evaluation of dynamic interfacial properties. However, we note here that all four features are necessary to perform this analysis.
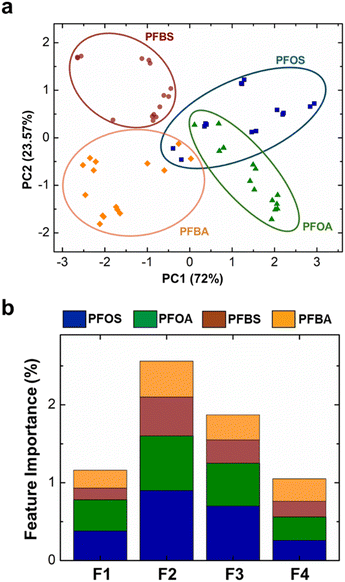 |
| Fig. 5 (a) Principal component analysis (PCA) of Janus droplet responses to PFAS in Milli-Q water for varying concentrations. (b) Significance of four extracted features on the classification of PFAS analyzed by feature-by-feature importance analysis. | |
Next, we employed the RF classification model to identify PFAS class. Our algorithm involved a 3-fold cross-validation across the data set, employing a 67/33 training-test data split ratio. To validate the outcomes, we generated a confusion matrix to compare the actual and predicted classes of PFAS (Table 1). Similar to PCA, we observed some degree of misclassification between PFOS and PFBA using RF, with the overall accuracy of 77%.
Table 1 Random Forest (RF) classification matrix based on the time-series responses to identify the PFAS class
Actual |
Predicted |
Accuracy |
PFOS |
PFOA |
PFBS |
PFBA |
PFOS |
6 |
0 |
0 |
9 |
40% |
PFOA |
0 |
15 |
0 |
0 |
100% |
PFBS |
0 |
0 |
15 |
0 |
100% |
PFBA |
4 |
1 |
0 |
10 |
67% |
|
Average |
77% |
We attributed this attained accuracy to the limited size of dataset and inherent similarities between data obtained from various PFAS. Despite these challenges, the classification model demonstrates its capability to differentiate PFAS identity utilizing extracted features. Broadening the data range and employing pattern recognition techniques can improve the classification model accuracy. Furthermore, we conducted the RF model excluding PFOS and PFBA from the dataset respectively and demonstrated that the overall accuracy approaches 100% (ESI,† Fig. S8 and S9). These classification results are summarized in Tables S3–S5.†
Following the identification of the type of PFAS, we performed a linear regression analysis to quantify the concentration. This model was preferred due to its simplicity and the provision of an easily interpretable mathematical formula for quantification. For each PFAS, a regression line was generated based on the input variables (F1–F4), and the mathematical formulation is expressed as:
yconc = β0 + βF1xF1 + βF2xF2 + βF3xF3 + βF4xF4 + ∈ |
The regression models were fitted to ascertain the intercept (β0) and coefficients (βF1, βF2, βF3, βF4). The fitted regression models were then employed to predict concentration, and the resulting errors (∈) represented the deviation of predicted values from actual values. The mean absolute error for the models across four different PFAS ranged from 1.2 to 2.5 μg L−1. Additionally, the coefficient of determination values, indicative of goodness of fit, varied from 0.88 to 0.96 (ESI,† Fig. S10 and S11). These values indicate the appropriateness of the linear regression model for the dataset, suggesting a high level of accuracy in predicting concentration (ESI,† Table S6).
Case studies 1: binary mixtures of PFAS
Next, we sought to test the interfacial behavior of binary mixtures of PFAS. We prepared four combinations: (1) PFOS-PFBS, (2) PFOA-PFBA, (3) PFOS-PFOA, and (4) PFBS-PFBA in Milli-Q water, at various ratios but constant total molar concentration. We observed that long-chain PFAS dominated interfacial responses, which is consistent with previous reports (ESI,† Fig. S12).95,96 From PCA results, we noticed decent separations between pure PFAS and 1
:
1 binary mixtures of PFAS. However, increased overlaps were observed when the dataset included additional molar ratios (ESI,† Fig. S13).
Case studies 2: effects of dissolved ions
After the proof-of-concept in Milli-Q water, we extended our study to account for potential interference from dissolved ions.43,48 Thus, we repeated the experiments using synthetic groundwater (SGW) as the matrix. We observed that the magnitude of emission changes (F1) is greater in SGW compared to the Milli-Q water for all PFAS (ESI,† Fig. S14a). The electrostatic interaction between cations and PFAS improves their effectivity as surfactants,48 which caused greater reductions in γF and resulted in higher emission intensity. We observed, from PCA outcomes using the same extracted key features, that near perfect separation between PFAS were obtained when the concentration was kept constant (ESI,† Fig. S15a). However, poor separation was obtained when the dataset included the whole range of concentrations (ESI,† Fig. S15b). This result suggested that more information is needed to deconvolute PFAS in SGW. We also note here that other geochemical factors (e.g., pH, competing surfactants) can significantly impact interfacial properties of PFAS, which could necessitate the implementation of multiplexed sensors61 to generate additional characteristic features.
Conclusion
In conclusion, we report a Janus droplet and microfluidics-based sensing platform to detect and classify PFAS in aqueous samples. We measured the changes in interfacial tension due to the exposure of PFAS in real-time through optical emissions. The collected emission responses were then analyzed using Principal Component Analysis and Random Forest analysis to develop a PFAS classification model. We achieved sufficient separation from PCA and an overall accuracy of 77% from RF analysis. Furthermore, we explored the utility of Janus droplets for detecting PFAS in complex aqueous systems, such as synthetic groundwater and PFAS mixtures. Our study represents a step toward developing a multiplexed sensing device capable of selectively identifying analytes in real-world environmental samples.
Conflicts of interest
The authors declare no conflict of interest.
Acknowledgements
The research reported here was funded by the Army Research Office via grant # W911-NF-2110310 to the University of Arizona. Any opinions or errors are not those of the Army Research Office or Department of Defense and are attributed solely to the authors. This work was also supported by startup funds from the University of Arizona through the Department of Chemical and Environmental Engineering, the BIO5 Institute, and Research Innovation, and Impact (RII). This work was performed in part at the Micro/Nano Fabrication Center at the University of Arizona.
References
- Y. Zhao, L. Shi, Y. Tian and L. Zhang, Self-assembly at Liquid-Liquid Interface A New SERS Substrate for Analytical, Chin. J. Chem., 2023, 41, 569–580 CrossRef CAS.
- D. Schrenk, M. Bignami, L. Bodin, J. K. Chipman, J. del Mazo, B. Grasl-Kraupp, C. Hogstrand, L. Hoogenboom, J. C. Leblanc and C. S. Nebbia,
et al., Risk to Human Health Related to the Presence of Perfluoroalkyl Substances in Food, EFSA J., 2020, 18(9), 6223, DOI:10.2903/j.efsa.2020.6223.
- C. E. Schaefer, D. Nguyen, E. Christie, S. Shea, C. P. Higgins and J. Field, Desorption Isotherms for Poly- and Perfluoroalkyl Substances in Soil Collected from an Aqueous Film-Forming Foam Source Area, J. Environ. Eng., 2022, 148(1), 04021074, DOI:10.1061/(asce)ee.1943-7870.0001952.
- J. L. Guelfo, S. Korzeniowski, M. A. Mills, J. Anderson, R. H. Anderson, J. A. Arblaster, J. M. Conder, I. T. Cousins, K. Dasu and B. J. Henry,
et al., Environmental Sources, Chemistry, Fate, and Transport of Per- and Polyfluoroalkyl Substances: State of the Science, Key Knowledge Gaps, and Recommendations Presented at the August 2019 SETAC Focus Topic Meeting, Environ. Toxicol. Chem., 2021, 3234–3260, DOI:10.1002/etc.5182.
- S. E. Fenton, A. Ducatman, A. Boobis, J. C. DeWitt, C. Lau, C. Ng, J. S. Smith and S. M. Roberts, Per- and Polyfluoroalkyl Substance Toxicity and Human Health Review: Current State of Knowledge and Strategies for Informing Future Research, Environ. Toxicol. Chem., 2021, 606–630, DOI:10.1002/etc.4890.
- L. Ahrens, Polyfluoroalkyl Compounds in the Aquatic Environment: A Review of Their Occurrence and Fate, J. Environ. Monit., 2011, 13(1), 20–31, 10.1039/c0em00373e.
- C. S. Law, J. Wang, S. Gunenthiran, S. Y. Lim, A. D. Abell, L. Ahrens, T. Kumeria, A. Santos and N. H. Voelcker, Real-Time Detection of per-Fluoroalkyl Substance (PFAS) Self-Assembled Monolayers in Nanoporous Interferometers, Sens. Actuators, B, 2022, 355, 131340, DOI:10.1016/j.snb.2021.131340.
- R. Ranaweera, S. An, Y. Cao and L. Luo, Highly Efficient Preconcentration Using Anodically Generated Shrinking Gas Bubbles for Per- and Polyfluoroalkyl Substances (PFAS) Detection, Anal. Bioanal. Chem., 2023, 415(18), 4153–4162, DOI:10.1007/s00216-022-04175-4.
- J. Park, K.-A. Yang, Y. Choi and J. K. Choe, Novel SsDNA Aptamer-Based Fluorescence Sensor for Perfluorooctanoic Acid Detection in Water, Environ. Int., 2022, 158, 107000, DOI:10.1016/j.envint.2021.107000.
- E. E. Harrison and M. L. Waters, Detection and Differentiation of Per- and Polyfluoroalkyl Substances (PFAS) in Water Using a Fluorescent Imprint-and-Report Sensor Array, Chem. Sci., 2022, 14(4), 928–936, 10.1039/d2sc05685b.
- L. Jones, J. Credo, R. Parnell and J. C. Ingram, Dissolved Uranium and Arsenic in Unregulated Groundwater Sources – Western Navajo Nation, J. Contemp. Wat. Res. Ed., 2020, 169(1), 27–43, DOI:10.1111/j.1936-704x.2020.03330.x.
- T. Rock and J. C. Ingram, Traditional Ecological Knowledge Policy Considerations for Abandoned Uranium Mines on Navajo Nation, Hum. Biol., 2020, 92(1), 19, DOI:10.13110/humanbiology.92.1.01.
- J. Credo, J. Torkelson, T. Rock and J. C. Ingram, Quantification of Elemental Contaminants in Unregulated Water across Western Navajo Nation, Int. J. Environ. Res. Public Health, 2019, 16(15), 2727, DOI:10.3390/ijerph16152727.
- X. Theurillat, C. Mujahid, B. Eriksen, A. Griffin, A. Savage, T. Delatour and P. Mottier, An LC-MS/MS Method for the Quantitative Determination of 57 per- and Polyfluoroalkyl Substances at Ng/Kg Levels in Different Food Matrices, Food Addit. Contam.: Part A, 2023, 40(7), 862–877, DOI:10.1080/19440049.2023.2226771.
- M. Al Amin, Z. Sobhani, Y. Liu, R. Dharmaraja, S. Chadalavada, R. Naidu, J. M. Chalker and C. Fang, Recent Advances in the Analysis of Per- and Polyfluoroalkyl Substances (PFAS)—A Review, Environ. Technol. Innovation, 2020, 19, 100879, DOI:10.1016/j.eti.2020.100879.
- W. Nassazzi, F. Y. Lai and L. Ahrens, A Novel Method for Extraction, Clean-up and Analysis of per- and Polyfluoroalkyl Substances (PFAS) in Different Plant Matrices Using LC-MS/MS, J. Chromatogr. B: Anal. Technol. Biomed. Life Sci., 2022, 1212, 123514, DOI:10.1016/j.jchromb.2022.123514.
- T. J. Durkin, B. Barua, J. J. Holmstrom, V. Karanikola and S. Savagatrup, Functionalized Amphiphilic Block Copolymers and Complex Emulsions for Selective Sensing of Dissolved Metals at Liquid-Liquid Interfaces, Langmuir, 2023, 39(36), 12845–12854, DOI:10.1021/acs.langmuir.3c01761.
- V. Trinh, C. S. Malloy, T. J. Durkin, A. Gadh and S. Savagatrup, Detection of PFAS and Fluorinated Surfactants Using Differential Behaviors at Interfaces of Complex Droplets, ACS Sens., 2022, 7(5), 1514–1523, DOI:10.1021/acssensors.2c00257.
- M. Hemida, A. Ghiasvand, V. Gupta, L. J. Coates, A. A. Gooley, H. J. Wirth, P. R. Haddad and B. Paull, Small-Footprint, Field-Deployable LC/MS System for On-Site Analysis of Per- And Polyfluoroalkyl Substances in Soil, Anal. Chem., 2021, 93(35), 12032–12040, DOI:10.1021/acs.analchem.1c02193.
- S. Garg, P. Kumar, G. W. Greene, V. Mishra, D. Avisar, R. S. Sharma and L. F. Dumée, Nano-Enabled Sensing of per-/Poly-Fluoroalkyl Substances (PFAS) from Aqueous Systems – A Review, J. Environ. Manage., 2022, 308, 114655, DOI:10.1016/j.jenvman.2022.114655.
- R. C. Buck, S. H. Korzeniowski, E. Laganis and F. Adamsky, Identification and Classification of Commercially Relevant Per- and Poly-Fluoroalkyl Substances (PFAS), Integr. Environ. Assess. Manage., 2021, 17(5), 1045–1055, DOI:10.1002/ieam.4450.
- D. J. Burns, P. Stevenson and P. J. C. Murphy, PFAS Removal from Groundwaters Using Surface-Active Foam Fractionation, Remediation, 2021, 31(4), 19–33, DOI:10.1002/rem.21694.
- B. Guo, H. Saleem and M. L. Brusseau, Predicting Interfacial Tension and Adsorption at Fluid-Fluid Interfaces for Mixtures of PFAS and/or Hydrocarbon Surfactants, Environ. Sci. Technol., 2023, 57(21), 8044–8052, DOI:10.1021/acs.est.2c08601.
- H. Campos-Pereira, D. B. Kleja, L. Ahrens, A. Enell, J. Kikuchi, M. Pettersson and J. P. Gustafsson, Effect of PH, Surface Charge and Soil Properties on the Solid–Solution Partitioning of Perfluoroalkyl Substances (PFASs) in a Wide Range of Temperate Soils, Chemosphere, 2023, 321, 138133, DOI:10.1016/j.chemosphere.2023.138133.
- Y. Lyu, M. L. Brusseau, W. Chen, N. Yan, X. Fu and X. Lin, Adsorption of PFOA at the Air-Water Interface during Transport in Unsaturated Porous Media, Environ. Sci. Technol., 2018, 52(14), 7745–7753, DOI:10.1021/acs.est.8b02348.
- D. Zhou, M. L. Brusseau, Y. Zhang, S. Li, W. Wei, H. Sun and C. Zheng, Simulating PFAS Adsorption Kinetics, Adsorption Isotherms, and Nonideal Transport in Saturated Soil with Tempered One-Sided Stable Density (TOSD) Based Models, J. Hazard. Mater., 2021, 411, 125169, DOI:10.1016/j.jhazmat.2021.125169.
- S. van Glubt and M. L. Brusseau, Contribution of Nonaqueous-Phase Liquids to the Retention and Transport of per and Polyfluoroalkyl Substances (PFAs) in Porous Media, Environ. Sci. Technol., 2021, 55(6), 3706–3715, DOI:10.1021/acs.est.0c07355.
- X. Meng, G. Yu, T. Luo, R. Zhang, J. Zhang and Y. Liu, Transcriptomics Integrated with Metabolomics Reveals Perfluorobutane Sulfonate (PFBS) Exposure Effect during Pregnancy and Lactation on Lipid Metabolism in Rat Offspring, Chemosphere, 2023, 341, 140120, DOI:10.1016/j.chemosphere.2023.140120.
- C. E. Crute, C. D. Landon, A. Garner, S. M. Hall, J. I. Everitt, S. Zhang, B. Blake, D. Olofsson, H. Chen and H. M. Stapleton,
et al., Maternal Exposure to Perfluorobutane Sulfonate (PFBS) during Pregnancy: Evidence of Adverse Maternal and Fetoplacental Effects in New Zealand White (NZW) Rabbits, Toxicol. Sci., 2023, 191(2), 239–252, DOI:10.1093/toxsci/kfac126.
- H. Su, Y. Shi, Y. Lu, P. Wang, M. Zhang, A. Sweetman, K. Jones and A. Johnson, Home Produced Eggs: An Important Pathway of Human Exposure to Perfluorobutanoic Acid (PFBA) and Perfluorooctanoic Acid (PFOA) around a Fluorochemical Industrial Park in China, Environ. Int., 2017, 101, 1–6, DOI:10.1016/j.envint.2017.01.016.
- Y. Yue, S. Li, Z. Qian, R. F. Pereira, J. Lee, J. J. Doherty, Z. Zhang, Y. Peng, J. M. Clark and A. R. Timme-Laragy,
et al., Perfluorooctanesulfonic Acid (PFOS) and Perfluorobutanesulfonic Acid (PFBS) Impaired Reproduction and Altered Offspring Physiological Functions in Caenorhabditis Elegans, Food Chem. Toxicol., 2020, 145, 111695, DOI:10.1016/j.fct.2020.111695.
- S. Saikat, I. Kreis, B. Davies, S. Bridgman and R. Kamanyire, The Impact of PFOS on Health in the General Population: A Review, Environ. Sci.: Processes Impacts, 2013, 15(2), 329–335, 10.1039/c2em30698k.
- B. Pachkowski, G. B. Post and A. H. Stern, The Derivation of a Reference Dose (RfD) for Perfluorooctane Sulfonate (PFOS) Based on Immune Suppression, Environ. Res., 2019, 171, 452–469, DOI:10.1016/j.envres.2018.08.004.
- K. Abraham, A. H. El-Khatib, T. Schwerdtle and B. H. Monien, Perfluorobutanoic Acid (PFBA): No High-Level Accumulation in Human Lung and Kidney Tissue, Int. J. Hyg. Environ. Health, 2021, 237, 113830, DOI:10.1016/j.ijheh.2021.113830.
- J. L. Guelfo and D. T. Adamson, Evaluation of a National Data Set for Insights into Sources, Composition, and Concentrations of per- and Polyfluoroalkyl Substances (PFASs) in U.S. Drinking Water, Environ. Pollut., 2018, 236, 505–513, DOI:10.1016/j.envpol.2018.01.066.
- M. L. Brusseau, Differential Sorption of Short-Chain versus Long-Chain Anionic Per- and Poly-Fluoroalkyl Substances by Soils, Environ., 2023, 10(10), 175, DOI:10.3390/environments10100175.
- M. L. Brusseau and S. Van Glubt, The Influence of Molecular Structure on PFAS Adsorption at Air-Water Interfaces in Electrolyte Solutions, Chemosphere, 2021, 281, 130829, DOI:10.1016/j.chemosphere.2021.130829.
- M. L. Brusseau, N. Yan, S. Van Glubt, Y. Wang, W. Chen, Y. Lyu, B. Dungan, K. C. Carroll and F. O. Holguin, Comprehensive Retention Model for PFAS Transport in Subsurface Systems, Water Res., 2019, 148, 41–50, DOI:10.1016/j.watres.2018.10.035.
- M. L. Brusseau, The Influence of Molecular Structure on the Adsorption of PFAS to Fluid-Fluid Interfaces: Using QSPR to Predict Interfacial Adsorption Coefficients, Water Res., 2019, 152, 148–158, DOI:10.1016/j.watres.2018.12.057.
- M. L. Brusseau, Determining Air-Water Interfacial Areas for the Retention and Transport of PFAS and Other Interfacially Active Solutes in Unsaturated Porous Media, Sci. Total Environ., 2023, 884, 163730, DOI:10.1016/j.scitotenv.2023.163730.
- T. Buckley, T. Vuong, K. Karanam, P. H. N. Vo, P. Shukla, M. Firouzi and V. Rudolph, Using Foam Fractionation to Estimate PFAS Air-Water Interface Adsorption Behaviour at Ng/L and μg/L Concentrations, Water Res., 2023, 239, 120028, DOI:10.1016/j.watres.2023.120028.
- L. E. Breshears, S. Mata-Robles, Y. Tang, J. C. Baker, K. A. Reynolds and J.-Y. Yoon, Rapid, Sensitive Detection of PFOA with Smartphone-Based Flow Rate Analysis Utilizing Competitive Molecular Interactions during Capillary Action, J. Hazard. Mater., 2023, 446(2022), 130699, DOI:10.1016/j.jhazmat.2022.130699.
- M. L. Brusseau and S. Van Glubt, The Influence of Surfactant and Solution Composition on PFAS Adsorption at Fluid-Fluid Interfaces, Water Res., 2019, 161, 17–26, DOI:10.1016/j.watres.2019.05.095.
- J. A. K. Silva, W. A. Martin and J. E. McCray, Air-Water Interfacial Adsorption Coefficients for PFAS When Present as a Multi-Component Mixture, J. Contam. Hydrol., 2021, 236(2020), 103731, DOI:10.1016/j.jconhyd.2020.103731.
- J. A. K. Silva, W. A. Martin, J. L. Johnson and J. E. McCray, Evaluating Air-Water and NAPL-Water Interfacial Adsorption and Retention of Perfluorocarboxylic Acids within the Vadose Zone, J. Contam. Hydrol., 2019, 223(2018), 103472, DOI:10.1016/j.jconhyd.2019.03.004.
- J. Costanza, M. Arshadi, L. M. Abriola and K. D. Pennell, Accumulation of PFOA and PFOS at the Air-Water Interface, Environ. Sci. Technol. Lett., 2019, 6(8), 487–491, DOI:10.1021/acs.estlett.9b00355.
- T. Buckley, K. Karanam, H. Han, H. N. P. Vo, P. Shukla, M. Firouzi and V. Rudolph, Effect of Different Co-Foaming Agents on PFAS Removal from the Environment by Foam Fractionation, Water Res., 2023, 230, 119532, DOI:10.1016/j.watres.2022.119532.
- T. Buckley, K. Karanam, X. Xu, P. Shukla, M. Firouzi and V. Rudolph, Effect of Mono- and Di-Valent Cations on PFAS Removal from Water Using Foam Fractionation – A Modelling and Experimental Study, Sep. Purif. Technol., 2022, 286, 120508, DOI:10.1016/j.seppur.2022.120508.
- N. J. M. Fitzgerald, A. Wargenau, C. Sorenson, J. Pedersen, N. Tufenkji, P. J. Novak and M. F. Simcik, Partitioning and Accumulation of Perfluoroalkyl Substances in Model Lipid Bilayers and Bacteria, Environ. Sci. Technol., 2018, 52(18), 10433–10440, DOI:10.1021/acs.est.8b02912.
- C. E. Schaefer, V. Culina, D. Nguyen and J. Field, Uptake of Poly-And Perfluoroalkyl Substances at the Air-Water Interface, Environ. Sci. Technol., 2019, 53(21), 12442–12448, DOI:10.1021/acs.est.9b04008.
- L. D. Zarzar, V. Sresht, E. M. Sletten, J. A. Kalow, D. Blankschtein and T. M. Swager, Dynamically Reconfigurable Complex Emulsions via Tunable Interfacial Tensions, Nature, 2015, 518(7540), 520–524, DOI:10.1038/nature14168.
- R. V. Balaj and L. D. Zarzar, Reconfigurable Complex Emulsions: Design, Properties, and Applications, Chem. Phys. Rev., 2020, 1(1), 011301, DOI:10.1063/5.0028606.
- L. D. Zarzar, J. A. Kalow, X. He, J. J. Walish and T. M. Swager, Optical Visualization and Quantification of Enzyme Activity Using Dynamic Droplet Lenses, Proc. Natl. Acad. Sci., 2017, 114(15), 3821–3825, DOI:10.1073/pnas.1618807114.
- C.-J. Lin, L. Zeininger, S. Savagatrup and T. M. Swager, Morphology-Dependent Luminescence in Complex Liquid Colloids, J. Am. Chem. Soc., 2019, 141(9), 3802–3806, DOI:10.1021/jacs.8b13215.
- L. Zeininger, E. Weyandt, S. Savagatrup, K. S. Harvey, Q. Zhang, Y. Zhao and T. M. Swager, Waveguide-Based Chemo- and Biosensors: Complex Emulsions for the Detection of Caffeine and Proteins, Lab Chip, 2019, 19(8), 1327–1331, 10.1039/c9lc00070d.
- L. Zeininger, S. Nagelberg, K. S. Harvey, S. Savagatrup, M. B. Herbert, K. Yoshinaga, J. A. Capobianco, M. Kolle and T. M. Swager, Rapid Detection of Salmonella Enterica via Directional Emission from Carbohydrate-Functionalized Dynamic Double Emulsions, ACS Cent. Sci., 2019, 5(5), 789–795, DOI:10.1021/acscentsci.9b00059.
- A. W. Baryzewska, C. Roth, P. H. Seeberger and L. Zeininger,
In Situ Tracking of Exoenzyme Activity Using Droplet Luminescence Concentrators for Ratiometric Detection of Bacteria, ACS Sens., 2023, 8(11), 4143–4151, DOI:10.1021/acssensors.3c01385.
- M. Pavlovic, H. K. Ramiya Ramesh Babu, S. Djalali, Z. Pavlovic, M. Vraneš and L. Zeininger, Dynamic In Situ Monitoring of the Salt Counter-Ion Effect on Surfactant Effectiveness Using Reconfigurable Janus Emulsions, Langmuir, 2023, 39(6), 2152–2160, DOI:10.1021/acs.langmuir.2c02346.
- M. Pavlovic, H. K. Ramiya Ramesh Babu, S. Djalali, M. Vraneš, V. Radonić and L. Zeininger, Facile Monitoring of Water Hardness Levels Using Responsive Complex Emulsions, Anal. Chem., 2021, 93(27), 9390–9396, DOI:10.1021/acs.analchem.1c00868.
- D. Fong and T. M. Swager, Trace Detection of Hydrogen Peroxide via Dynamic Double Emulsions, J. Am. Chem. Soc., 2021, 143(11), 4397–4404, DOI:10.1021/jacs.1c00683.
- B. Barua, T. J. Durkin, I. M. Beeley, A. Gadh and S. Savagatrup, Multiplexed and Continuous Microfluidic Sensors Using Dynamic Complex Droplets, Soft Matter, 2023, 19(10), 1930–1940, 10.1039/d3sm00074e.
- S. Yuan, X. Wang, Z. Jiang, H. Zhang and S. Yuan, Contribution of Air-Water Interface in Removing PFAS from Drinking Water: Adsorption, Stability, Interaction and Machine Learning Studies, Water Res., 2023, 236, 119947, DOI:10.1016/j.watres.2023.119947.
- L. Zhao, Y. Zhang, S. Fang, L. Zhu and Z. Liu, Comparative Sorption and Desorption Behaviors of PFHxS and PFOS on Sequentially Extracted Humic Substances, J. Environ. Sci., 2014, 26(12), 2517–2525, DOI:10.1016/j.jes.2014.04.009.
- M. J. Qazi, S. J. Schlegel, E. H. G. Backus, M. Bonn, D. Bonn and N. Shahidzadeh, Dynamic Surface Tension of Surfactants in the Presence of High Salt Concentrations, Langmuir, 2020, 36(27), 7956–7964, DOI:10.1021/acs.langmuir.0c01211.
- T. R. Carale, Q. T. Pham and D. Blankschtein, Salt Effects on Intramicellar Interactions and Micellization of Nonionic Surfactants in Aqueous Solutions, Langmuir, 1994, 10(1), 109–121, DOI:10.1021/la00013a016.
- E. J. Smith, W. Davison and J. Hamilton-Taylor, Methods for Preparing Synthetic Freshwaters, Water Res., 2002, 36(5), 1286–1296, DOI:10.1016/S0043-1354(01)00341-4.
- J. Li, A. Concellón, K. Yoshinaga, Z. Nelson, Q. He and T. M. Swager, Janus Emulsion Biosensors for Anti-SARS-CoV-2 Spike Antibody, ACS Cent. Sci., 2021, 7(7), 1166–1175, DOI:10.1021/acscentsci.1c00173.
- J. Li, S. Savagatrup, Z. Nelson, K. Yoshinaga and T. M. Swager, Fluorescent Janus Emulsions for Biosensing of Listeria Monocytogenes, Proc. Natl. Acad. Sci. U. S. A., 2020, 117(22), 1–8, DOI:10.1073/pnas.2002623117.
- L. Zeininger, Responsive Janus Droplets as Modular Sensory Layers for the Optical Detection of Bacteria, Anal. Bioanal. Chem., 2023, 415(21), 5205–5219, DOI:10.1007/s00216-023-04838-w.
- N. Rakesh, H. Tu, P. C. Chang, S. T. Gebreyesus and C. Lin, Innovative Real-Time Flow Sensor Using Detergent-Free Complex Emulsions with Dual-Emissive Semi-Perfluoroalkyl Substituted A-Cyanostilbene, Adv. Sci., 2023, 10(31), 1–9, DOI:10.1002/advs.202304108.
- B. D. Frank, S. Djalali, A. W. Baryzewska, P. Giusto, P. H. Seeberger and L. Zeininger, Reversible Morphology-Resolved Chemotactic Actuation and Motion of Janus Emulsion Droplets, Nat. Commun., 2022, 13(1), 1–12, DOI:10.1038/s41467-022-30229-3.
- P. Kim, K. W. Kwon, M. C. Park, S. H. Lee, S. M. Kim and K. Y. Suh, Soft Lithography for Microfluidics: A Review, BioChip J., 2008, 2(1), 1–11 Search PubMed.
-
Y. Nishimura, K. Yano, M. Itoh and M. Ito, Photolithography, in Flat Panel Display Manufacturing, Wiley, 2018, pp. 287–310, DOI:10.1002/9781119161387.ch13.
- D. Qin, Y. Xia and G. M. Whitesides, Soft Lithography for Micro- and Nanoscale Patterning, Nat. Protoc., 2010, 5(3), 491–502, DOI:10.1038/nprot.2009.234.
- M. A. H. Khan, B. Thomson, R. Debnath, A. Motayed and M. V. Rao, Nanowire-Based Sensor Array for Detection of Cross-Sensitive Gases Using PCA and Machine Learning Algorithms, IEEE Sens. J., 2020, 20(11), 6020–6028, DOI:10.1109/JSEN.2020.2972542.
- Y. Zhang, D. Zhang, Y. Zhao, X. Yuan, H. Liu, J. Wang and B. Sun, An Ionic Liquid-Assisted Quantum Dot-Grafted Covalent Organic Framework-Based Multi-Dimensional Sensing Array for Discrimination of Insecticides Using Principal Component Analysis and Clustered Heat Map, Microchim. Acta, 2021, 188(9), 298, DOI:10.1007/s00604-021-04936-5.
- L. Shi, Q. Tang, B. Yang, W. Liu, B. Li, C. Yang and Y. Jin, Portable and Label-Free Sensor Array for Discriminating Multiple Analytes via a Handheld Gas Pressure Meter, Anal. Chem., 2022, 94(41), 14453–14459, DOI:10.1021/acs.analchem.2c03497.
- F. Cui, Y. Yue, Y. Zhang, Z. Zhang and H. S. Zhou, Advancing Biosensors with Machine Learning, ACS Sens., 2020, 5(11), 3346–3364, DOI:10.1021/acssensors.0c01424.
- S. Zhong, K. Zhang, M. Bagheri, J. G. Burken, A. Gu, B. Li, X. Ma, B. L. Marrone, Z. J. Ren and J. Schrier,
et al., Machine Learning: New Ideas and Tools in Environmental Science and Engineering, Environ. Sci. Technol., 2021, 55(19), 12741–12754, DOI:10.1021/acs.est.1c01339.
- Z. Jin, J. Shang, Q. Zhu, C. Ling, W. Xie and B. Qiang, Employee Turnover Prediction Based on Random Forests and Survival Analysis, Lect. Notes Comput. Sci., 2020, 12343 LNCS, 503–515, DOI:10.1007/978-3-030-62008-0_35.
- S. Han, B. D. Williamson and Y. Fong, Improving Random Forest Predictions in Small Datasets from Two-Phase Sampling Designs, BMC Med. Inf. Decis. Making, 2021, 21(1), 1–9, DOI:10.1186/s12911-021-01688-3.
- J. S. Cooper, H. Kiiveri, E. Chow, L. J. Hubble, M. S. Webster, K. H. Müller, B. Raguse and L. Wieczorek, Quantifying Mixtures of Hydrocarbons Dissolved in Water with a Partially Selective Sensor Array Using Random Forests Analysis, Sens. Actuators, B, 2014, 202, 279–285, DOI:10.1016/j.snb.2014.05.094.
- A. J. Tesoriero, J. A. Gronberg, P. F. Juckem, M. P. Miller and B. P. Austin, Predicting Redox-sensitive Contaminant Concentrations in Groundwater Using Random Forest Classification, Water Resour. Res., 2017, 53(8), 7316–7331, DOI:10.1002/2016WR020197.
- G. Taherzadeh, Y. Zhou, A. W. C. Liew and Y. Yang, Structure-Based Prediction of Protein-Peptide Binding Regions Using Random Forest, Bioinformatics, 2018, 34(3), 477–484, DOI:10.1093/bioinformatics/btx614.
- M. L. Brusseau, B. Guo, D. Huang, N. Yan and Y. Lyu, Ideal versus Nonideal Transport of PFAS in Unsaturated Porous Media, Water Res., 2021, 202, 117405, DOI:10.1016/j.watres.2021.117405.
- J. E. F. Abraham, K. G. Mumford, D. J. Patch and K. P. Weber, Retention of PFOS and PFOA Mixtures by Trapped Gas Bubbles in Porous Media, Environ. Sci. Technol., 2022, 56(22), 15489–15498, DOI:10.1021/acs.est.2c00882.
- M. L. Brusseau, N. Yan, S. Van Glubt, Y. Wang, W. Chen, Y. Lyu, B. Dungan, K. C. Carroll and F. O. Holguin, Comprehensive Retention Model for PFAS Transport in Subsurface Systems, Water Res., 2019, 148, 41–50, DOI:10.1016/j.watres.2018.10.035.
- P. Zareitalabad, J. Siemens, M. Hamer and W. Amelung, Perfluorooctanoic Acid (PFOA) and Perfluorooctanesulfonic Acid (PFOS) in Surface Waters, Sediments, Soils and Wastewater - A Review on Concentrations and Distribution Coefficients, Chemosphere, 2013, 725–732, DOI:10.1016/j.chemosphere.2013.02.024.
- C. You, C. Jia and G. Pan, Effect of Salinity and Sediment Characteristics on the Sorption and Desorption of Perfluorooctane Sulfonate at Sediment-Water Interface, Environ. Pollut., 2010, 158(5), 1343–1347, DOI:10.1016/j.envpol.2010.01.009.
- Y. Deng, Z. Liang, X. Lu, D. Chen, Z. Li and F. Wang, The Degradation Mechanisms of Perfluorooctanoic Acid (PFOA) and Perfluorooctane Sulfonic Acid (PFOS) by Different Chemical Methods: A Critical Review, Chemosphere, 2021, 283, 131168, DOI:10.1016/j.chemosphere.2021.131168.
- L. Ahrens, N. Yamashita, L. W. Y. Yeung, S. Taniyasu, Y. Horii, P. K. S. Lam and R. Ebinghaus, Partitioning Behavior of Per- and Polyfluoroalkyl Compounds between Pore Water and Sediment in Two Sediment Cores from Tokyo Bay, Japan, Environ. Sci. Technol., 2009, 43(18), 6969–6975, DOI:10.1021/es901213s.
- S. E. Hale, H. P. H. Arp, I. Schliebner and M. Neumann, Persistent, Mobile and Toxic (PMT) and Very Persistent and Very Mobile (VPvM) Substances Pose an Equivalent Level of Concern to Persistent, Bioaccumulative and Toxic (PBT) and Very Persistent and Very Bioaccumulative (VPvB) Substances under REACH, Environ. Sci. Eur., 2020, 32(1), 155, DOI:10.1186/s12302-020-00440-4.
- H. Singh, G. Singh, N. Kaur and N. Singh, Pattern-Based Colorimetric Sensor Array to Monitor Food Spoilage Using Automated High-Throughput Analysis, Biosens. Bioelectron., 2022, 196, 113687, DOI:10.1016/j.bios.2021.113687.
-
T. Archana, Dimensionality Reduction and Classification through PCA and LDA, 2015, vol. 122 Search PubMed.
- Y. Zhang, A. Thomas, O. Apul and A. K. Venkatesan, Coexisting Ions and Long-Chain per- and Polyfluoroalkyl Substances (PFAS) Inhibit the Adsorption of Short-Chain PFAS by Granular Activated Carbon, J. Hazard. Mater., 2023, 460, 132378, DOI:10.1016/j.jhazmat.2023.132378.
- J. A. K. Silva, W. A. Martin and J. E. McCray, Air-Water Interfacial Adsorption Coefficients for PFAS When Present as a Multi-Component Mixture, J. Contam. Hydrol., 2021, 236, 103731, DOI:10.1016/j.jconhyd.2020.103731.
Footnote |
† Electronic supplementary information (ESI) available: Experimental details, fabrication and characterization of Janus droplets, control experiments, and data analysis. See DOI: https://doi.org/10.1039/d4lf00128a |
|
This journal is © The Royal Society of Chemistry 2024 |
Click here to see how this site uses Cookies. View our privacy policy here.