DOI:
10.1039/D3MA00866E
(Paper)
Mater. Adv., 2024,
5, 2510-2525
A high-performance humidity sensor based on 3D porous SnO2-encapsulated MCM-48 for real-time breath monitoring and contactless gesture detection
Received
16th October 2023
, Accepted 12th January 2024
First published on 7th February 2024
Abstract
Ordered porous SnO2/MCM-48 was used as a basis for developing a resistive-type humidity sensor fabricated by drop-casting on a Ag–Pd substrate and its use was demonstrated for real-time respiration monitoring and no-contact sensing for the first time. Using a hydrothermal method, a series of SnO2/MCM-48 humidity sensors were prepared and comprehensively compared. The humidity sensor was tested in a variety of humidity levels from 11 to 98% RH for humidity detection, and various flexible humidity testing experiments were conducted and the results were recorded. The results showed a significant enhancement in the humidity-detecting capabilities of the sensor, which was ascribed to the combined outcomes of the use of SnO2 and the material's structure characterized by the pores. The sensor demonstrated fast response and recovery times of 9 s and 12 s with high sensitivity and minimal hysteresis. Further, the stability over time of the SnO2/MCM-48 sensor was confirmed, indicating its potential for commercial applications. The prepared sensor was used to test human breathing patterns under various tangible circumstances, and its successful performance demonstrated its potential for use in medical applications. In a broader context, the SnO2/MCM-48-based humidity sensor proposed in this study can detect patterns in human health without physical contact and is very stable, easy to synthesize, and environment friendly.
1. Introduction
The rise in various respiratory illnesses such bronchitis, asthma, and tuberculosis in recent years has had a significant impact on people's health. Consequently, real-time respiratory illness monitoring is becoming more and more popular.1 Researchers have created many electronic and pressure sensors to suit the demands for the real-time monitoring of respiratory disorders. However, these sensors no longer satisfy the requirements of high-performance breathing sensors because the dependability of electronic sensors is constrained by external conditions and wearers may experience pain from pressure sensors.2 It is well known that a notable portion of water molecules in human breath make up more than 90% of its relative humidity (RH), and monitoring respiratory disorders will soon involve the use of humidity sensors to keep track of variations in RH caused by breathing.3 To address the demands of respiratory monitoring, researchers need to create humidity sensors with minimal hysteresis, high responsiveness, and a capability for long-term operation. Furthermore, the application of humidity sensors for measuring human respiration for monitoring various diseases will also need to be able to achieve non-contact sensing to avoid contact among people.
The capacity to gather data without coming into close contact with a subject is known as non-contact sensing. Non-contact technology allows tracking the human body without having to make physical contact with the subject. When treating a condition as contagious as COVID-19, non-contact methods are thought to be quite beneficial because touch may aid in the disease propagation.4 This also allows patients to be monitored without having to come into contact with healthcare professionals. As wearable devices require direct physical contact with patients for them to be attached, using them can put healthcare professionals at danger. Even though safety measures, like using gloves and face masks, the risk would be reduced even further if contact with patients could be successfully cut off entirely. In light of this, the sensor is made to detect humidity without requiring touch.
The sensing materials have a direct impact on how well humidity sensors operate. Numerous types of materials for humidity sensing have been taken into account thus far, including polymers,5 carbon materials,6 semiconductor metal oxides (SMOs),7 innovative 2D materials,8 and their composites.9 Because of their superior stability and enhanced sensitivity, SMOs are frequently utilized in humidity sensors. Because of its broad band gap, uncomplicated structure, remarkable selectivity, and inexpensiveness, SnO2 has recently garnered attention as a feasible humidity-sensitive material, serving as a representative metal oxide semiconductor.10 The electrochemical stability, specific surface area,11 and SnO2's oxygen vacancies are also acceptable.12 However, the performance of pure SnO2 humidity sensors is influenced by various factors, such as the surface area and the presence of oxygen vacancies, and these sensors to date have demonstrated limited responsiveness, subpar linearity, and extended response and recovery times.13
Meanwhile, 3D hollow porous mesostructures with the ability to manage the morphology are being developed progressively to manufacture many materials due to their huge surface areas, which can be created to enable water molecules easy access to interior and exterior surfaces, unobstructed channels, and short diffusion routes.14 Recently, there have been significant advancements in the evolution of highly structured mesoporous silica materials (MSMs), which have led to their extensive research in various domains, including sensors, optical devices, drug-delivery systems, and catalysis.15 MSMs have numerous Si–OH active bonds located on the inner pore walls, uniform channels, high porosity, tuneable pore size, and thermal stability.16 They also have great mechanical strength and thermal stability. MCM-48, in particular, is a member of the M41S family and can be utilized as a template to create 3D porous SnO2 cubic structures with a large number of pores.17 Kunchakara et al.18 synthesized a RH sensor based on Ag-doped MCM-41 and obtained response and recovery times of 24 s and 32 s, respectively. Similarly, Solanki et al.19 synthesized porous SnO2 with a sensitivity of 348 and response/recovery of 200/8 s. The modification of mesoporous silica with nanometallic particles, such as Li,20 Al,21 MgO,22 and Co3O4,1 has been studied for sensing applications. By utilizing a mesoporous hybrid nanocomposite based on SnO2/MCM-48 we aimed to develop a sensor with better sensitivity, low hysteresis, quick response/recovery times, and a wide detection range.
In this study, a template called MCM-48 was used to create 3D hollow mesoporous SnO2. Modifications of MCM-48 by SnO2 with varying concentrations promoted the appearance of more tin defects along with large adsorption sites provided by the open channels and large specific surface area of MCM-48, which greatly improved the responsivity of the sensor. The structural and morphological traits of SnO2/MCM-48 were studied and the sensor was further examined for multifunctional applications. The SnO2 humidity sensor was fabricated to test its capabilities for sensing humidity at ambient temperature.
Using saturated salt solutions, the key features of humidity sensing, such as response/recovery times, hysteresis, sensitivity, and repeatability were measured. As anticipated, the SnO2 humidity sensor displayed outstanding humidity sensing capabilities, characterized by a rapid response time and wide-humidity-detection range.23 Additionally, the SnO2 humidity sensor demonstrated exceptional performance in a variety of applications related to the well-being of individuals, such as the real-time tracking of respiration and non-contact detection. Moreover, the compact and the portable nature of the sensor made it suitable for use in wearable devices. The sensor developed in this work has potential in various applications like humidity sensing, breath monitoring, and non-contact sensing.
2. Experimental
2.1. Materials
TEOS [(C2H5O)4Si, Sigma Aldrich], CTAB [cetyltrimethyl ammonium bromide, Mw = 364.45, SRL], tin(II) chloride dehydrate [(SnCl2·2H2O), Mw = 225.65, Merck], ethanol [Fisher Scientific], and ammonia solution [NH4OH, Mw = 35.05] were used as the main reagents. Double-distilled water was employed throughout the experiments.
2.2. Material preparation
2.2.1. Preparation of MCM-48.
MCM-48 was made following a previously established procedure.24 Specifically, 2.4 g CTAB was dissolved in a mixture of 100 g distilled water, 55 mL ethanol, and 14 mL ammonia solution at ambient temperature. After continuous stirring for 1 h, resulting in a clear solution, 3.4 g TEOS was introduced. The solution thus obtained was stirred at ambient temperature for 6 h. The resulting dried white powder was subjected to hydrothermal treatment in an autoclave maintained at 90 °C for 1 day. Then, after cooling to ambient temperature of 25 °C, the resultant solids were retrieved, filtered, and washed. The powder, which was mesoporous silica MCM-48, was obtained by calcinating the products in the air at 500 °C (with a heating rate of 1 °C min−1) for 7 h.
2.2.2. Synthesis of SnO2/MCM-48.
Regarding the encapsulation of different wt% SnO2 in MCM-48 via an in situ method, the salt (5 wt%, 7 wt%, 10 wt%) was added in to a watery micellar solution of CTAB. The blend was stirred for 2 h. Subsequently, TEOS was added dropwise, and the mixture was stirred for a further 6 h, following an identical process employed for pure MCM-48. The different wt% were the synthesis step by step process is depicted in Fig. 1.
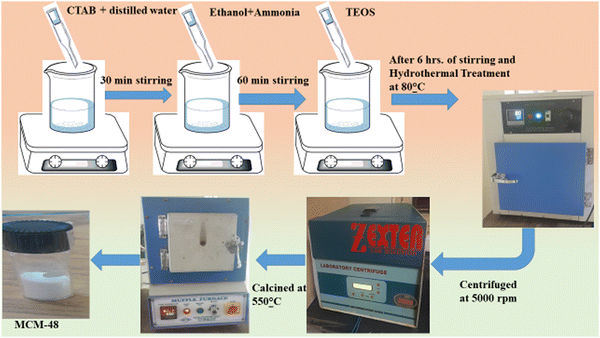 |
| Fig. 1 Schematic representation of MCM-48's synthesis route. | |
2.3 Characterization
The structural analysis of the prepared composites was characterized by small-angle X-ray scattering (SAXS) at low angles (0.8°–2.5°) and XRD utilizing CuKα radiation at a constant wavelength (λ = 1.54 Å). The surface morphology and the mesostructure were analyzed by FE-SEM and HRTEM using a TECHNAI microscope at 200 kV. The elemental composition was analyzed by EDX. A specific surface and pore-size analyzer was also used to analyze the BET surface area and various other surface-related characteristics of SnO2.
2.4 Fabrication and functionality evaluation of the SnO2/MCM-48 humidity sensor
For the fabrication of the sensors, the synthesized materials were coated onto a Ag–Pd interdigitated electrode via a 10 μL pipette, as demonstrated in Fig. 2(a). Prior to the coating, the electrode was cleaned using acetone. Then 0.3 g of the substance was combined with 2 mL of ethanol and thoroughly homogenized into a consistent paste using a mortar and pestle for use as the coating. Finally, the prepared SnO2/MCM-48 sensor was desiccated at 70 °C for 3 h to eliminate excess ethanol.
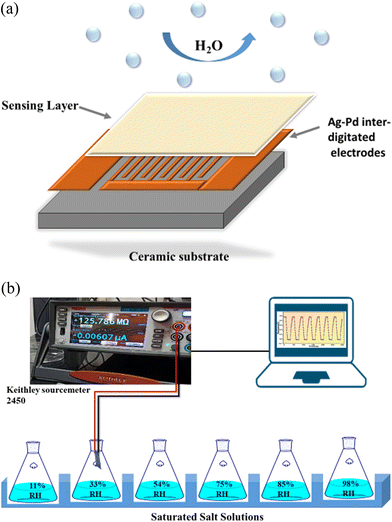 |
| Fig. 2 (a) Fabrication of the humidity sensor. (b) Schematic illustrating the configuration of the humidity sensing set-up. | |
An illustration of the dynamic humidity sensing testing set-up is displayed in Fig. 2(b). For different humidity levels corresponding to 11%, 33%, 54%, 75%, 85%, and 98% RH, distinctive salt solutions were assigned, namely LiCl, (MgCl2·6H2O), (MgNO3)2·4H2O, NaCl, KCl, and K2SO4, respectively.25 These solutions were kept at 25 °C since humidity is dependent on temperature. In order to capture any variation in resistance because of the adsorption of H2O, the electrode was directly put into a closed beaker with the different humidity levels. A constant source voltage was applied using a Keithley-2450 sourcemeter and the electrical properties were observed in accordance with Ohm's Law. The variations in resistance were recorded and subjected to thorough analysis.
3. Results and discussions
3.1 Small-angle X-ray scattering (SAXS)
The arrangement of the cubic template provided by the calcined MCM-48 materials was confirmed using SAXS. Fig. 3 presents the SAXS plots obtained for both pure MCM-48 and SnO2-encapsulated MCM-48 with varying concentrations. The obtained plots show the mesoporous integrity in all the samples, which is clearly evident through the diffraction peak corresponding to the (211) plane for 2Θ smaller than 3°, which directly indicates the presence of the cubic pore arrangement (Ia3D symmetry) of the mesoporous nanostructures.26 This type of ordered arrangement plays a significantly crucial role in the sensor performance in sensing applications. The intensity of the (211) reflection was identical in all the nanostructures, confirming that the cubic arrangement of pores had not been disturbed when SnO2 was infused. The SAXS patterns verified that the fast and facile approach for producing siliceous MCM-48 could be expanded to create SnO2/MCM-48 and that the integration of SnO2 as the guest species within the silica matrix did not disrupt the regular structure of MCM-48.
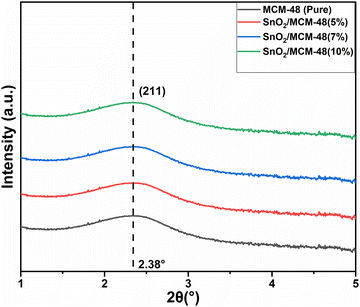 |
| Fig. 3 Small-angle X-ray plots for MCM-48 and SnO2/MCM-48. | |
3.2 Wide-angle X-ray diffraction (WA-XRD)
As displayed in Fig. 4, the wide-angle XRD spectra ranging from 10° to 80° for pure and SnO2-incorporated MCM-48 revealed the development of the tetragonal rutile SnO2 (JCPDS #41-1445) with the increase in the concentration of SnO2. The broad hump at 2Θ = 22° is characteristic of the amorphous nature of mesoporous MCM-48.27 As the concentration of the encapsulated SnO2 increased, the peaks became noticeable in the XRD patterns; whereas at low encapsulation concentrations those peaks remained obscure as the amorphous nature of the mesoporous MCM-48 was dominant. At higher encapsulation percentages, the peaks located at 2Θ = 27.81°, 34.56°, 52.78° corresponding to (110), (101), and (121) planes confirmed the introduction of a polycrystalline nature.28 Moreover, the development and resilience of the (110) plane enabled the achievement of elevated oxygen vacancy levels at reduced temperatures. The XRD patterns demonstrated that the crystalline nature of the specimen was altered as the doping concentration of SnO2 increased, leading to disruptions in the lattice structure.
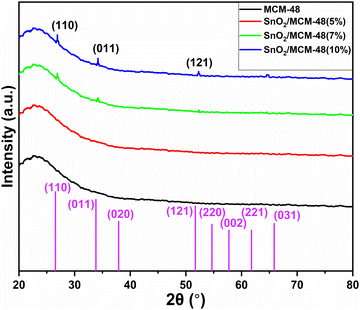 |
| Fig. 4 WA-XRD plots for MCM-48 and SnO2/MCM-48. | |
The crystallite dimensions were calculated for all the samples, specifically with respect to the (110) plane, using the Scherrer formula as defined in eqn (1).28
|  | (1) |
where
D is the crystallite size;
k is a constant value (0.95);
λ is 1.54 Å, which corresponds to the wavelength of the X-rays; and
β corresponds to the broadening factor. The interplanar spacing (
dhkl) and lattice parameter (
a) for the structural analysis were determined using
eqn (2).
28 The alterations in the lattice parameter were attributed to the presence of micro strain (
δ), as described by
eqn (3) (Table 1).
28 | 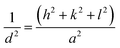 | (2) |
|  | (3) |
Table 1 XRD analysis of all the nanocomposites
Sample name |
FWHM (β) |
Crystallite size, D (Å) |
d
hkl
|
a (Å) |
δ
|
SnO2/MCM-48(10%) |
7.72526 |
0.223471 |
3.387941393 |
5.298728 |
2.115387 |
SnO2/MCM-48(7%) |
6.88335 |
0.283862 |
3.347819301 |
4.734531 |
1.876922 |
SnO2/MCM-48(5%) |
3.22213 |
1.013745 |
3.257601983 |
4.606945 |
0.993198 |
MCM-48 |
0.24117 |
6.023316 |
3.518825088 |
5.619326 |
0.407457 |
The findings indicate that introducing the tin precursor ahead of the silica precursor or mixing the tin precursor into ethanol resulted in a greater FWHM and a broader distribution of sizes among the cubic unit cell, which is an indication of the introduced crystallinity in the synthesized material.
3.3 Field emission scanning electron microscopy-energy dispersive X-ray (FESEM-EDX)
The surface morphologies of MCM-48 and SnO2/MCM-48(5%) were investigated by FESEM analysis. The scanning electron microscopy (SEM) images revealed that the resulting particles were spherical in shape, as shown in Fig. 5(a) and (b). The consistent spherical shape could likely be attributed to the incorporation of ammonia, which is recognized as a catalyst for shaping the morphology.17 It could also be seen that introduction of guest species in the frameworks of the pristine MCM-48 caused no damage to the framework's morphology. The grains existed within the range of 350–500 nm, and no agglomeration was visible.
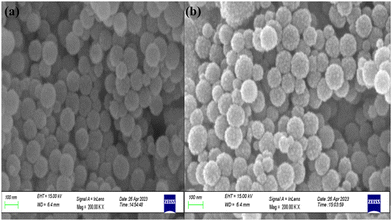 |
| Fig. 5 FESEM images illustrating the morphologies of (a) MCM-48 and (b) SnO2/MCM-48(5%) at a scale of 100 nm. | |
Energy dispersive X-ray (EDX) analysis was done to verify the composition of the elements present in SnO2/MCM-48. The EDX spectrum of pure MCM-48 along with the elemental composition are displayed in Fig. 6(a). The existence of only Si and O peaks indicated the lack of impurity particles in the prepared MCM-48. Concerning SnO2/MCM-48(5%), the occurrence of tin peaks along with Si and O indicated the successful encapsulation of tin in the siliceous matrix of MCM-48.
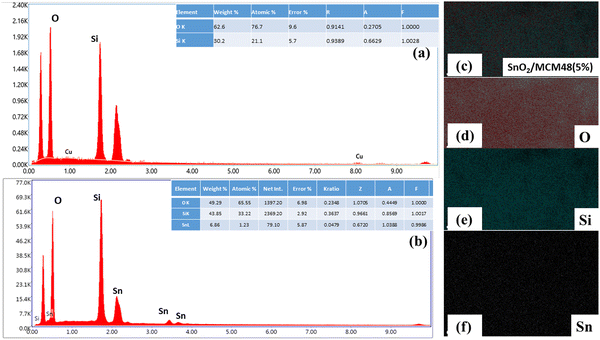 |
| Fig. 6 EDX analysis spectra of (a) MCM-48 and (b) SnO2/MCM-48(5%). (c)–(f) EDX elemental mapping. | |
Fig. 6(c–f) present the elemental mapping results, revealing the uniform distribution of all the elements within the siliceous framework.
3.4 Fourier transform infrared spectroscopy (FTIR)
The FTIR spectra of MCM-48 and SnO2/MCM-48(5%, 7%, 10%) are depicted in Fig. 7 in the wavelength range of 400–4000 cm−1. The framework bands at 1092, 805, and 463 cm−1 corresponded to asymmetric stretching, symmetric stretching, and bending vibrations of Si–O–Si bands, respectively. The wide signal at 3430 cm−1 indicated the presence of surface silanols and adsorbed moisture. The stretching band made by the vibration of Sn–O–Sn bonds of SnO2 at 571 cm−1 implied the encapsulation of SnO2. The bands centred at 1635 and 960 cm−1 corresponded to the stretching vibrations of the Si–OH group.
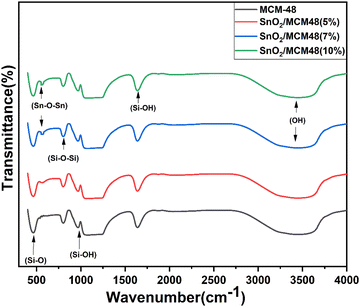 |
| Fig. 7 FTIR spectra of MCM-48 and SnO2/MCM-48. | |
3.5 X-Ray photoelectron spectroscopy (XPS)
The valence states of Si, O, and Sn in the surface regions of MCM-48 and SnO2/MCM-48(5%) were studied by XPS spectroscopy. Fig. 8(a) indicates an asymmetric O 1s profile, suggesting the presence of physically adsorbed oxygen, while the peak position corresponding to 101.4 eV indicated the presence of the Si 2p3/2 state. Fig. 8(b) shows the peaks corresponding to the Si 2p3/2 and O 1s states along with an illustration of the spin–orbital splitting of the Sn4+ 3d3/2 and Sn4+ 3d5/2 core level states of tin centred at 493.3 and 484.9 eV, respectively, corresponding to the lattice of tin oxide. Also, the distinction in the energy levels of Sn4+ 3d3/2 and Sn4+ 3d5/2 at 8.4 eV aligned with the standard spectrum of Sn, as documented in the Handbook of X-ray Photoelectron Spectroscopy.
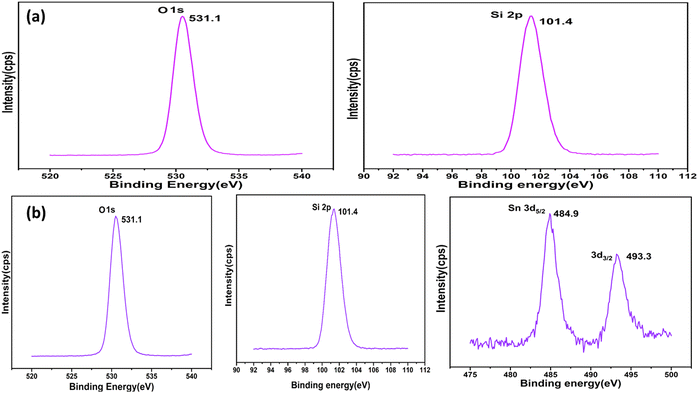 |
| Fig. 8 XPS analysis of (a) MCM-48 and (b) SnO2/MCM-48(5%). | |
3.6 Brunauer–Emmett–Teller (BET)
The nitrogen adsorption and desorption isotherms were obtained to assess the behaviours of the pure MCM-48 and Sn-encapsulated MCM-48 composites with varying compositions. As illustrated in Fig. 9(a), each of the samples revealed a type IV isotherm characterized by an H1-type hysteresis loop,29 giving a clear indication of the ordered mesoporous structures of the materials possessing cubic pores with the Ia3D symmetry.30 This confirmed the substantial integrity of the mesoporous framework attained by the sample even after the encapsulation of Sn. The pure siliceous demonstrated an exceptionally high specific surface area of 1226.8 m2 g−1. However, as the encapsulation concentration increased, a subsequent declination was seen in both the specific surface area and size of the distributed pores across the composites. The gradual declination in the BET-specific area may be due to pore filling due to the presence of tin in the pores, which also resulted in the shrinkage of the pores. The pore-size distribution curve supported this trend, making the encapsulation more obvious in the mesoporous matrix of MCM-48, as displayed in Fig. 9(b).
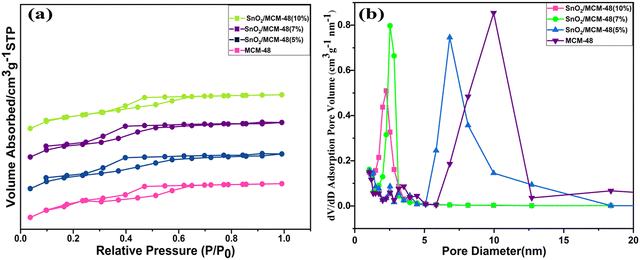 |
| Fig. 9 (a) Nitrogen hysteresis adsorption and desorption isotherms. (b) Pore analysis of all the synthesized samples. | |
Table 2 illustrates an overview of various BET characteristics of all the as-prepared composites. The average pore diameter calculated from the data provided in Table 2 was 5.392 nm. These characteristics have great importance in making a material's surface porous, thus influencing the adsorption and diffusion processes. The introduction of a catalytically active dopant, i.e. Sn components, was attributed to the declination in the specific surface area, but being a metal oxide it also facilitated an augmentation of the humidity sensing properties. The presence of a huge surface area provided good active adsorption sites for water molecules, which affected the surface ionic conduction, resulting in enhanced humidity sensing properties, showing it could serve as an efficient humidity sensor.
Table 2 Structural analysis of all the nanocomposites
Sample |
Pore diameter Dp |
Specific surface area (m2 g−1) |
Pore volume Vp (cm2 g−1) |
MCM-48 |
9.2675 |
1226.8 |
0.747 |
SnO2/MCM-48(5%) |
6.8192 |
1156.2 |
0.698 |
SnO2/MCM-48(7%) |
2.5291 |
1026.4 |
0.635 |
SnO2/MCM-48(10%) |
2.2575 |
923.7 |
0.589 |
3.7 High-resolution transmission electron microscopy (HRTEM)
Analysis of the pore morphologies of MCM-48 and SnO2/MCM-48(5%) and examination of the existence of SnO2 nanoparticles within the mesoporous silica matrix were executed using HRTEM. The TEM illustration of MCM-48 and SnO2/MCM-48(5%) in Fig. 10(a) and (b) showed well-ordered cubic arrays of 3D mesoporous channels. In Fig. 10(a–c), it can be seen that the MCM-48 sample gas 3D Ia3D had a regular cubic mesostructure. The introduction of TEOS and SnCl2·2H2O in to the reaction system and their uniform dispersion was because of the “synchronous assembly strategy”, whereas the reactant and precursor salts were blended uniformly due to the rigorous stirring for long time, making us believe that the formed SnO2 particles were uniformly dispersed in the MCM-48 matrix.27 Furthermore, when calcination was done, the SnO2 nanoparticles were dispersed uniformly inside the channels of MCM-48. In Fig. 10(d–f), the pore arrangement for SnO2/MCM-48(5%) is illustrated. Notably, numerous finely dispersed SnO2 nanoparticles could be observed within the cubic channels of MCM-48. The average thickness of the walls was approximately 2.52 nm and analysis of the pore diameter yielded a value of 5.152 nm, as encountered with the help of these images. These measurements closely aligned with the outcomes acquired by the XRD data and the BET surface analysis. The structural order thus remained unaltered, but the dimensions were reduced because of the interactions between the nanoparticles and the host. Remarkably, the cubic mesostructure was not disturbed despite the inclusion of SnO2 particles.
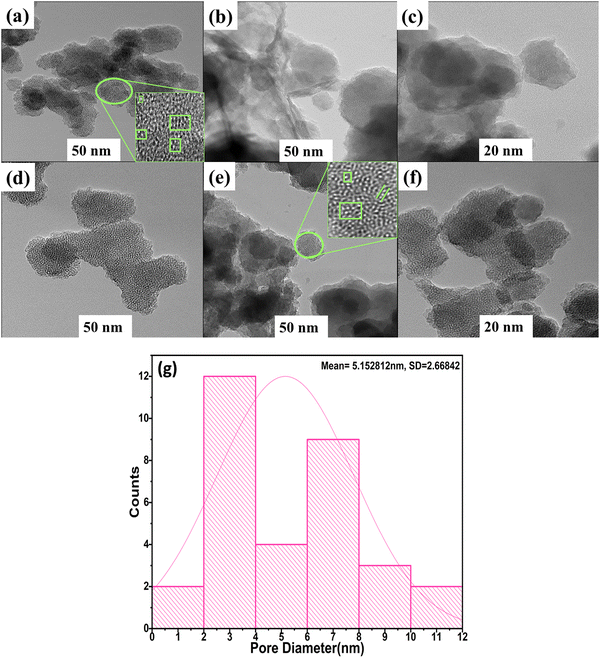 |
| Fig. 10 HRTEM images showing the uniform pore channels of (a)–(c) MCM-48 and (d)–(f) SnO2/MCM-48(5%). (g) Pore-size distribution of SnO2/MCM-48(5%). | |
3.8 Humidity sensing performance
The porous nature of the matrix plays a significant function in utilizing its potential as a humidity sensor. Fig. 11 illustrates the variations in resistance of the prepared samples over a range of humidity conditions (i.e. RH 11–98%). Fig. 11 shows the recorded resistance of the host sample MCM-48 did not change very much over the humidity extent of 11–98% RH, but when the SnO2/MCM-48 sample was subjected to the same conditions a declination in the resistance was observed. This was because of the fact that as the doping concentration increased, the metal ions could be easily transported into the matrix of MCM-48 and contributed towards the enhanced humidity sensing properties.The change in resistance of the bare MCM-48 sample was initially found to be around 2 orders of magnitude, which was increased to 5 orders after doping SnO2 in to the host siliceous MCM-48. However there was a consistent change in the resistance observed in all the prepared samples, but there is a dissimilarity in the sample with the encapsulation percentage of 7%, which may be percieved as the limitation of the siliceous matrix to transport the metal ions larger than the pore channel, leading to hinderance of the carriers and hydronium ions. Fig. 11 presents a much better visual 3D representation of the variation in resistance order concerning the humidity. As seen from Fig. 11, SnO2/MCM-48(5%) demonstrated exceptional properties as a humidity sensor, and thus was further studied for its response/recovery times, hysteresis, and stability.
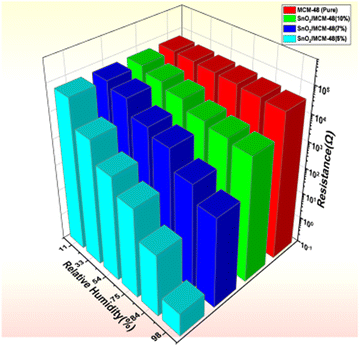 |
| Fig. 11 3D representation of the change in resistance with respect to the RH% for MCM-48 and SnO2/MCM-48 nanocomposites. | |
To create an improved humidity sensor, it is crucial to consider two vital parameters: the response time and recovery time, especially across varying humidity levels. The response time and recovery time can be defined as the timeframe to attain a change in the displayed resistance value, up to 98% relative humidity (RH), during humidification and dehumidification processes, respectively. In Fig. 12(a), the recorded response and recovery times for the processes of humidification and dehumidification can be obeserved, which were measured as 9 s and 12 s, respectively, within the extent of 11–98% RH. Furthermore, Table 3 provides a comparative analysis of the response and recovery times with reference to the existing literature. Repeating the humidification and dehumidification process for a longer interval of time gave a consistent response and recovery times, as illustrated in Fig. 12(b). Possessing fast response and recovery times at varying humidity levels established that the synthesized hybrid nanocomposite [SnO2/MCM-48] has significant promise in the future in the sensing industry as a sensing material for use in humidity-detection devices.
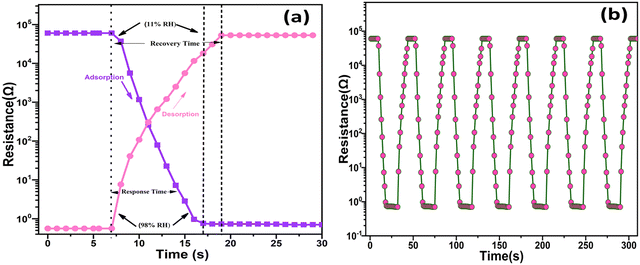 |
| Fig. 12 (a) Response/recovery times for SnO2/MCM-48(5%); (b) response/recovery times for repeated cycles. | |
Table 3 Comparative study of the humidity sensing properties for distinguished materials from previously documented literature
S. No. |
Materials |
Method of synthesis |
Response time(s) |
Recovery time(s) |
Order of change in resistance |
Ref. |
1. |
MCM-48 fibre |
Electrospinning |
15 |
28 |
2 |
31
|
2. |
Li/SBA-15 |
|
60 |
180 |
3.5 |
|
3. |
α-Fe2O3 nanorods |
Hydrothermal |
60 |
33 |
3.5 |
32
|
4. |
Nanocrystalline α-Fe2O3 |
Microwave-assisted hydrothermal route |
<60 |
350 |
— |
33
|
5. |
Li doped γ- Fe2O3 nanopower |
Simple method using a precipitating agent |
150 |
180 |
3 |
34
|
6. |
Mesoporous Fe2O3/Silica |
Hydrothermal |
20 |
40 |
4 |
35
|
7. |
Quasi Cubic Fe2O3 |
Hydrothermal |
,2 |
1568 |
— |
36
|
8. |
LiCl/KIT-6 |
Hydrothermal |
15 |
26 |
3 |
37
|
9. |
Ag/MCM-41 |
Magnetron sputtering |
24 |
32 |
5 |
18
|
10. |
Fe/SBA-15 |
Hydrothermal |
20 |
50 |
4 |
35
|
11. |
SnO2 |
Microwave irradiaton method |
32 |
25 |
2 |
10
|
12. |
SnO2/SiO2 |
Sol–Gel |
14 |
16 |
2 |
12
|
13. |
Fe/SnO2 |
Precipitation technique |
— |
— |
0.8 |
38
|
14. |
NiO/SnO2 |
Electrospinning method |
18.4 |
37.2 |
1 |
39
|
15. |
SnO2/MCM-48 |
Hydrothermal |
9 |
12 |
5 |
This work |
Fig. 13(a) illustrates the response and recovery times with variation of the RH% for the SnO2/MCM-48(5%) sensor. The resistance profiles acquired from the humidity-increasing experiment and the humidity-decreasing experiment appeared to be substantially symmetrical, indicating outstanding humidification and dehumidification performance. Another important humidity sensor examining property is the “repeatability”, which is crucial for evaluating a sensor's performance, and it can be described as “the closeness of output values with the same repeated input values under the same conditions of measurements”. The repeated measurements of the sensor are illustrated in Fig. 13(b). At 33 RH%, 54 RH%, 75 RH%, 85 RH%, 98 RH%, the measurements were examined repeatedly ensuring the resistance was the same in all three measurements, thus indicating the outstanding repeatability of the SnO2/MCM-48(5%) sensor.
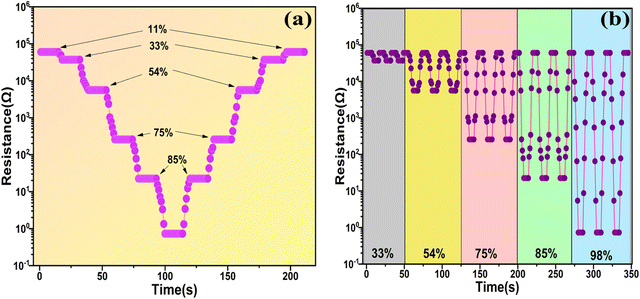 |
| Fig. 13 (a) Staircase plot representation of the variation of resistance with time for the SnO2/MCM-48(5%) sensor. (b) Repeatability performance of the SnO2/MCM-48(5%) sensor exposed to 33% RH, 54% RH, 75% RH, 85% RH, 98% RH. | |
Fig. 14(a) demonstrates the variation in resistance because of the adsorption (11–98 RH%) and desorption (98–11 RH%) of the water molecules. A hysteresis curve can also indicate a sample's credibility during humidification and dehumidification processes. Using the results, we generated a hysteresis curve, and from this it was clear that the hysteresis impact of the SnO2/MCM-48(5%) sensor was negligible (1.1%) and it can be deployed as a reliable humidity sensor.
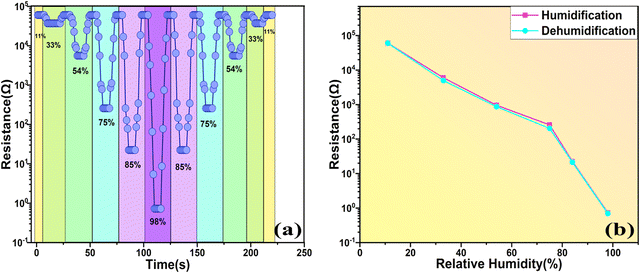 |
| Fig. 14 (a) Uplink and downlink testing of the SnO2/MCM-48(5%) sensor. (b) Hysteresis characteristics of the SnO2/MCM-48(5%) sensor at varying RH (11–98%). | |
The hysteresis error is determined with the help of the following expression (4):22
|  | (4) |
where Δ
Hmax is the variation in output for adsorption and desorption processes and
Ffs represents the full-scale output.
Furthermore, durability and reliability analysis of the sensor was performed over a 30-day period, as displayed in Fig. 15(a). Remarkably, the sensor showed negligible shift in resistance, confirming the exceptional stability of the sensor. We next examined the functional connection between the resistance changes in diverse relative humidity environments during both humidification and dehumidification processes. As depicted in Fig. 15(b), the sensor's response was nearly linear in the mid-range of humidity, spanning from 33% RH to 75% RH. However, a more precise representation of the response behaviour across the entire humidity extent could be achieved using a polynomial fitting equation, i.e.eqn (5), yielding a regression coefficient (R2 = 0.99):
| y = 2.43x3 − 3.63x2 + 926.43x + 47294.3 | (5) |
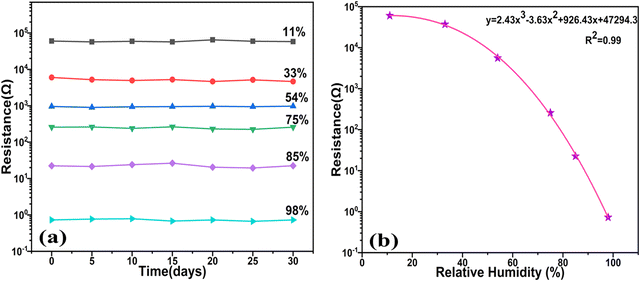 |
| Fig. 15 (a) Stability plot of the sensor over a duration of 30 days. (b) Cubic polynomial fitting of the resistance with the RH%. | |
This reveals that the sensor exhibited greater sensitivity within the middle range of humidity, as opposed to at lower and higher RH levels. The sensitivity of the suggested sensor was assessed by calculating the fraction of the resistance variation to the variation in RH% value,40 as illustrated in eqn (6):41
|  | (6) |
The plot provided an average sensitivity value of 693.1180 Ω/RH%, while the sensor's highest sensitivity, within the suggested linear range of 33–75 RH%, was recorded at 879.206 Ω/RH%. This heightened sensitivity might be attributed to the surface irregularities, which augment the number of effective sites available for water adsorption.
3.9 Breath monitoring
We were inspired to investigate the applicability of the SnO2/MCM-48-based humidity sensor device in monitoring human breath by the aforementioned exceptional results of its performance. The difference in the relative humidity of the air that is breathed in and out was the cause of this.42 A humidity sensor application that has become increasingly relevant in recent years is the analysis of human breath to determine levels of dehydration.42 The monitoring of oxygen levels and breath patterns in secluded patients who were infected amidst the highly infectious COVID-19 crisis were the critical markers for giving them necessary medical support.43 Remote monitoring of a patient is possible in these circumstances if the intelligent humidity sensor devices are put in a wearable mask, which would also ease the load of providing acute patient care. The creation of inexpensive sensors that do not need an external power source has recently been the subject of research. A self-powered humidity sensor that can measure both skin moisture and ambient humidity was proposed by Wang et al.44 and was based on a nanofibers film. Similar to this, Zhang et al.45 created flexible humidity sensors with tin disulfide nanoflowers and reduced graphene oxide nanohybrids that were powered by triboelectric nanogenerators made of poly(tetrafluoroethylene). For multiple purposes, the SnO2 breath sensor demonstrated the ability to measure human breath using a varied rate and finger technique.41
When employed to analyze human breath patterns, the sensor demonstrates notable fluctuations in the resistance that correspond to the inhalation and exhalation processes. Siliceous SnO2/MCM-48 is non-toxic, making it suitable for integration into a mask for monitoring human breath patterns. Fig. 16 depicts the breath sensing set-up for the in vitro study. The individual's consent was obtained for inclusion of the photograph depicting the experimental set-up.
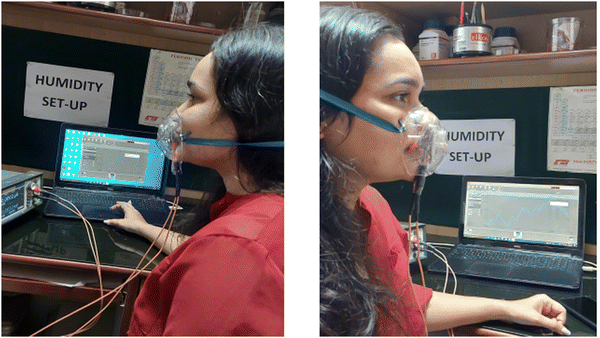 |
| Fig. 16 Photograph of the combination of the humidity sensor and a wearable mask. | |
The analysis of the breathing configuration was conducted at various rates, while concurrently, the electrical characteristics of the SnO2/MCM-48 sensor were assessed using a Keithley 2450 sourcemeter with a voltage of 1 V applied. The sensor's reactions to different human breathing rates, specifically fast, normal, and slow, were examined, and the resulting alterations in the device resistance were recorded, and are presented in Fig. 17(a, b, and c), respectively.
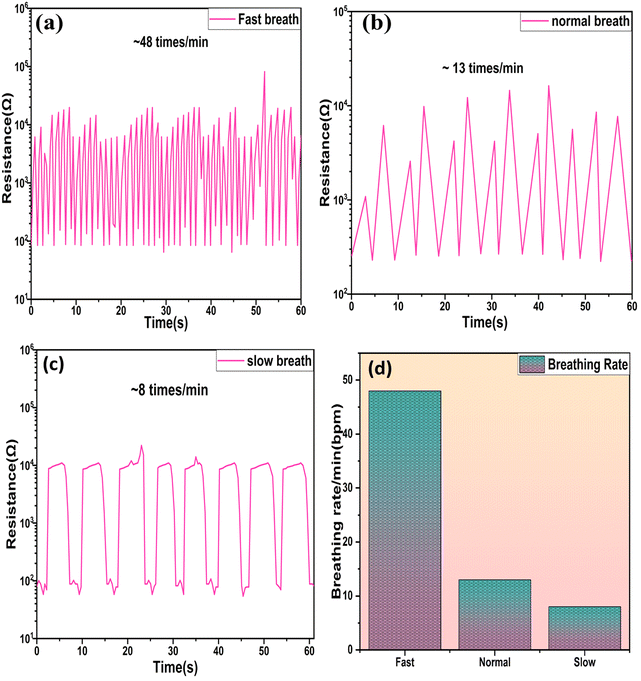 |
| Fig. 17 Sensor's response to various breathing rates: (a) fast breathing, (b) normal breathing, (c) slow breathing. (d) Breathing rate per min for a 26-year-old individual with various breath patterns. | |
The peaks in the graph indicate the number of breaths/minute (bpm) for an individual, amounting to 8, 13, and 48 bpm in cases of slow, normal, and rapid breathing, respectively, as depicted in Fig. 17(d). Additionally, the sensor displayed the capability to analyze the durations of inhalation and exhalation intervals in various breath rhythms. Typically, in fit individuals, inhalation exhibits shorter time intervals compared to exhalation,46 as illustrated in Fig. 18(a–c) for distinct breathing patterns. The electric signal patterns displayed varying amplitudes at different breathing rhythms, enabling the sensor to recognize various physical conditions possessed by the individual. During slow breathing, the signal magnitude was notably more stable, followed by the normal and fast respiration rate. This amplitude fluctuation was because of the fact that varying quantity of H2O molecules engaged with the sensor during the various breathing rhythms. During slow breathing, the extended time intervals facilitated a large amount of H2O molecules to be resent to the sensor surface, resulting in a relatively smaller resistance.
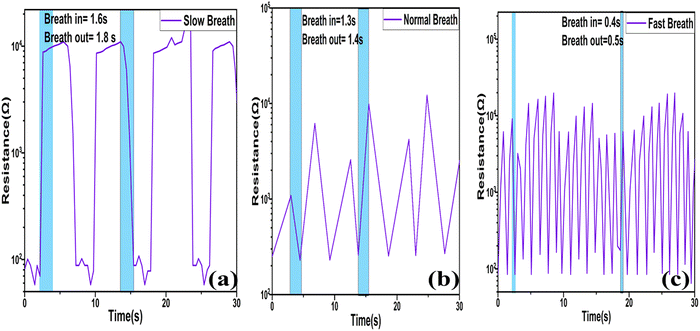 |
| Fig. 18 Breath inhale and breath exhale timings at distinct breath rates: (a) slow, (b) normal, (c) fast. | |
3.10 Non-contact skin sensing
Sweat glands, which are distributed across most of the human body's skin, consistently release sweat. Moreover, we observed that the SnO2/MCM-48(5%) humidity sensor was sufficiently sensitive to detect variations in humidity caused by the proximity of human fingers. In Fig. 19(a), the resistance change resulting from swiping a finger over the sensor five times demonstrated the SnO2/MCM-48(5%) humidity sensor's capability to effectively detect finger movement. Fig. 19(b) depicts the resistance variation plot while the finger was in near vicinity (∼6 mm) to the sensor and when it was moved away. While the finger was in near vicinity to the sensor, the sensor's resistance significantly decreased due to water evaporation from the finger's surface. Upon lifting the finger, the resistance rapidly increased, showcasing a robust non-contact switching characteristic.28 The SnO2/MCM-48(5%) humidity sensor consistently exhibited similar resistance variations when the finger was brought near to or moved distant from the sensor. These findings in Fig. 19 demonstrate that the sensor could accurately and consistently detect various gestures. These applications underscore the significant potential of the proposed SnO2/MCM-48(5%) humidity sensor for tracking human activity and different relevant domains.
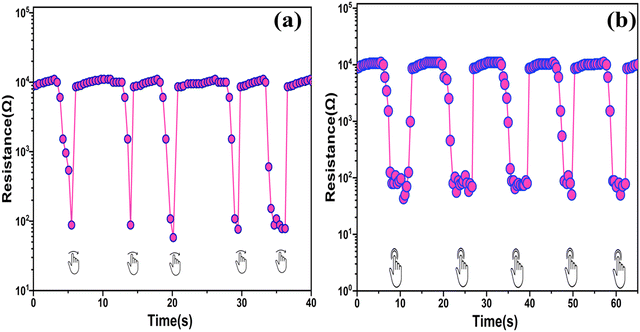 |
| Fig. 19 Response of the SnO2/MCM-48(5%) humidity sensor to a (a) finger sliding over and (b) finger moving in an upward and downward motion at a distance of ∼6 mm. | |
3.11 Grotthuss mechanism
The fundamental idea behind humidity sensing is connected to how semiconducting materials react differently in a variety of humidity conditions. The variation in resistance of these semiconductor materials at varied RH stages can be ascribed to the humidification process of water onto the oxide surface.47 The engagement of water molecules with the prepared material, particularly at the surface in a humid atmosphere, results in changing the material's electrical resistance. Electronic conduction takes place because of the increase in charge-carrier concentration and lower electrical resistance caused by the adsorbed H2O molecules at the interior surfaces of the composites.48 However, depending on the level of humidity in the air, the resistivity may rise or fall. Water must be adsorbed through the internal surfaces of materials in order for conduction to occur. Elevated water adsorption onto a sample's surface amplifies protonic conduction within the water layers, and this phenomenon was concisely elucidated through the Grotthuss mechanism (as depicted in Fig. 20(a)), which was initially introduced approximately two centuries ago. Protonic conduction is initiated in the layers of adsorbed H2O at a sensor's surface, and this mechanism allows protons to travel from one H2O molecule to another by means of H bonds. During the study of TiO2 and Fe2O3, the procedure underlying the protonic conduction process on the sensing material surfaces was identified.49,50 Hair and Hertl reported an analogous technique of hydroxylation and subsequent multiple layer synthesis on the siliceous surface in 1969.51 In the initial phase of adsorption (chemisorption process), (i) a H2O molecule adheres to an active site; (ii) it then proceeds to create an adsorption complex, which then results in the development of surface OH groups during the (iii) step (physisorption); In step (iv), different water molecules approach the surface and attach themselves through two adjacent OH groups, forming a double H bond. In Fig. 20(b), the four steps of adsorption are depicted. Additionally, the double H bonding (iv) prevents the top water molecule from moving around easily. Since no H bonds were established within H2O in this first physically adsorbed layer, there is no proton conduction at this stage (eqn (7) and (8)). As a result, this layer is static. The chemisorption mechanism, however, happens only at lower humidity levels; when the humidity levels rise, H2O molecules physisorb onto the OH layer. The charge complexes of cations originating from either the base material or impurities, as well as the water molecules in the vicinity at its surface, i.e. the hydroxyl ions, are what actually drive the physisorption process.52 | H2O → H+ + OH− (chemisorption) | (7) |
| 2H2O → H3O+ + OH− (physisorption) | (8) |
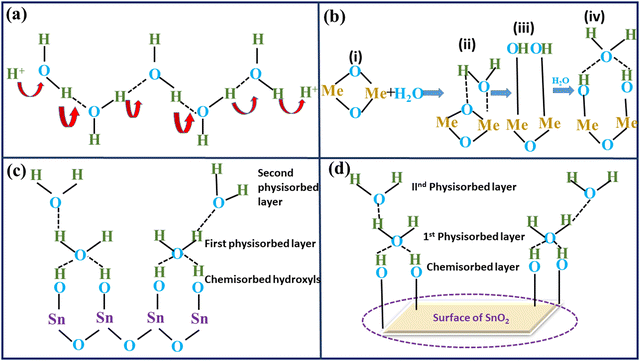 |
| Fig. 20 (a) Grotthuss mechanism; (b) processes of water retention; (c) illustration of multiple layers of water in the condensed state; (d) representation of the Grotthuss chain reaction based on the SnO2/MCM-48 humidity sensor. | |
The operational principle of SnO2/MCM-48 humidity sensors relies on water-phase protonic conduction, as elucidated earlier. This occurs within the adsorbed water layers on the surface of the sensing material. The electrical resistance of the sensor decreases due to the chemisorbed hydroxyl ions, facilitating proton hopping between adjacent hydroxyl groups when an electric field is applied. The first physically adsorbed layer was followed by an additional layer as water persisted to physically re-enter the SnO2/MCM-48 sensor's surface, transforming the single-layer physisorption process into a multiple layer one (Fig. 20(c)). The H2O molecules may be singly bound to H molecules, operating just like bulk liquid water. The multiple layers are less organized than the first physically adsorbed layer. In this fashion, each H2O molecule joins with each OH− ion to create H3O+, which then separates into H2O and H+ ions (eqn (9)), in accordance with Grotthuss's chain reaction theory. The outcome is a dramatic increase in the number of H+ ions and a fall in resistance. As the water is adsorbed on the oxide surface in molecular and hydroxyl forms, a SnO2–H2O interaction model was constructed (Fig. 20(d)). On the surface of SnO2, the water molecule acts as a donor. Consequently, the SnO2/MCM-48's surface water-phase conduction mechanism is responsible for the variation in resistance with humidity adsorption.
The sensor uses a similar method for monitoring breath. The resistance, and consequently the resistance of SnO2/MCM-48(5%), is influenced by the amount of adsorbed H2O molecules.28 Since the expelled air has a high humidity level, water molecules adhere to the sensor surface and reduce the resistance being measured. The resistance starts to rise when the H2O molecules begin to desorb from the sensor's surface during breathing. The sensor's reaction is quick enough to quickly discern between the two settings with different relative humidities. We can determine a person's breath rate by evaluating the graph. The developed sensor holds significant promise due to the informative nature of the breath and its rate concerning human health. Consequently, this sensor has the potential to be highly beneficial in hospital settings, aiding in the early identification of critical health conditions in patients. Practical implementation is feasible through the creation of a compact, battery-operated device that can be easily integrated into masks. Notably, our SnO2/MCM-48(5%) resistance humidity sensor operates at 1 V during breath monitoring readings.
Moreover, the sensor exhibited excellent responses across varying breathing patterns, encompassing fast, slow, and deep breathing. This suggests that with the application of AI-based algorithms for the interpretation of the sensor yielded motif, remote data translation and warning signals could be efficiently generated. These advancements could lead to the integration of this technology in applications pertaining to both relative humidity and tracking respiration.
The uncomplicated, environmentally friendly synthesis process and favourable physio-chemical properties of SnO2/MCM-48(5%) underscore its potential for use in creating affordable and eco-friendly utilizable sensors.
4. Conclusion
A resistive SnO2/MCM-48-type humidity sensor was fabricated on a Ag–Pd substrate via a drop-casting technique. SAXS plots confirmed the mesoporous nature of SnO2/MCM-48 due to the presence of diffraction peak corresponding to the (211) plane. With the help of XRD, SnO2 was confirmed to be present in the siliceous matrix as the doping concentration increased. Through meticulous FESEM-EDX and HRTEM examinations, we confirmed the surface's morphology and uniform dispersion of tin particles, seamlessly aligned within the cubic mesopores. The research next investigated the feasibility of utilizing SnO2/MCM-48 for humidity sensing applications. The humidity sensing properties revealed a 5 orders of magnitude change in resistance when varying the RH from 11% to 98%. The sensor displayed a dynamic response of 9 s and a rapid recovery time of 12 s, which were much better than many other sensors. The sensor was found to be highly sensitive towards humidity sensing, along with demonstrating an average sensitivity value of 693.1180 Ω/RH% and minimal hysteresis of 1%. The sensor's ability to preserve extraordinary stability over a 30-day period suggested that it has long-lasting performance potential, paving the way for continued use. The sensor, in its original state, was capable of the ongoing monitoring of human respiratory activity, discerning both the rate and depth of breathing (for slow: 8 breaths per min; for normal: 13 breaths per min; for fast: 48 breaths per min), which suggests it can play a marvellous role in future diagnostic breath analysis. Furthermore, facial non-contact skin breath sensing was effectively achieved by the SnO2/MCM-48 sensor, which is useful for gesture recognition. Hence, this pioneering work presents a viable approach for crafting a high-performance humidity sensor using SnO2/MCM-48 hybrid nanocomposite and opens the door to create real-time humidity tracking and contactless sensing devices.
Author contributions
Priya: methodology, formal analysis, investigation, data curation, conceptualization, writing – original draft. Surender Duhan: writing – review & editing, supervision. Rakesh Malik: writing – review & editing, supervision.
Data availability statement
The data will be accessible on demand.
Conflicts of interest
The author declare that they do not have any recognized competing factors that might have impacted the research presented in this paper.
Acknowledgements
Authors are grateful to the DST Delhi, for giving experimental support facilities via FIST Research Grant (Sanction Order No. & Date: SR/FST/PS-1/2018/32).
References
- Y. Zhang, Y. Wu, Z. Duan, B. Liu, Q. Zhao, Z. Yuan, S. Li, J. Liang, Y. Jiang and H. Tai, Appl. Surf. Sci., 2022, 585, 152698 CrossRef CAS.
- S. Kano, K. Kim and M. Fujii, RSC Adv., 2020, 10, 8910–8916 RSC.
- L. Gong, X. Wang, D. Zhang, X. Ma and S. Yu, J. Mater. Chem. A, 2021, 9, 5619–5629 RSC.
- W. Taylor, Q. H. Abbasi, K. Dashtipour, S. Ansari, S. A. Shah, A. Khalid and M. A. Imran, Sensors, 2020, 20, 1–19 Search PubMed.
- J. Yu, H. Byun, M. So and J. Huh, Sens. Actuators, B, 2005, 108, 305–308 CrossRef CAS.
- Y. Pang, J. Jian, T. Tu, Z. Yang, H. Tian, Y. Yang and T. Ren, Biosens. Bioelectron., 2018, 116, 123–129 CrossRef CAS PubMed.
- M. Lei, M. Gao, X. Yang, Y. Zou, A. Alghamdi, Y. Ren and Y. Deng, ACS Appl. Mater. Interfaces, 2021, 13, 51933–51944 CrossRef CAS PubMed.
- M. Sajid, Z. J. Khattak, K. Rahman, G. Hassan and K. H. Choi, Bull. Mater. Sci., 2022, 47 DOI:10.1007/s12034-022-02799-x.
- B. Li, Q. Tian, H. Su, X. Wang, T. Wang and D. Zhang, Sens. Actuators, B, 2019, 299, 126973 CrossRef CAS.
- M. Parthibavarman, V. Hariharan and C. Sekar, Mater. Sci. Eng., C, 2011, 31, 840–844 CrossRef CAS.
- R. Rajalakshmi, V. V. Srinivasan, M. P. Pachamuthu and R. Maheswari, Mater. Chem. Phys., 2015, 154, 164–169 CrossRef CAS.
- Y. Zhu, J. Chen, H. Li, Y. Zhu and J. Xu, Sens. Actuators, B, 2014, 193, 320–325 CrossRef CAS.
- F. Li, P. Li and H. Zhang, Sensors, 2022, 22, 1–13 Search PubMed.
- C. Anand, P. Srinivasu, G. P. Mane, S. N. Talapaneni, M. R. Benzigar, S. Vishnu Priya, S. S. Al-Deyab, Y. Sugi and A. Vinu, Microporous Mesoporous Mater., 2013, 167, 146–154 CrossRef CAS.
- Q. Meng, A. Duan, C. Xu, Z. Zhao, J. Li, B. Wang, C. Liu, D. Hu, H. Li and Y. Li, Catal. Sci. Technol., 2018, 8, 5062–5072 RSC.
- P. Malik, S. Sehrawat, A. Boora, B. Anisha, S. Kumari, Y. Ahlawat and S. Duhan, Mater. Prot., 2023, 64, 512–518 Search PubMed.
- K. Schumacher, P. I. Ravikovitch, A. Du Chesne, A. V. Neimark and K. K. Unger, Langmuir, 2000, 16, 4648–4654 CrossRef CAS.
- S. Kunchakara, A. Ratan, M. Dutt, J. Shah, R. K. Kotnala and V. Singh, J. Phys. Chem. Solids, 2020, 145, 109531 CrossRef CAS.
- V. Solanki, S. B. Krupanidhi and K. K. Nanda, ACS Appl. Mater. Interfaces, 2017, 9, 41428–41434 CrossRef CAS PubMed.
- T. Zhang, R. Wang, W. Geng, X. Li, Q. Qi, Y. He and S. Wang, Sens. Actuators, B, 2008, 128, 482–487 CrossRef CAS.
- Y. Li, W. Zhang, L. Zhang, Q. Yang, Z. Wei, Z. Feng and C. Li, J. Phys. Chem. B, 2004, 108, 9739–9744 CrossRef CAS.
- S. Sehrawat, S. P. Nehra and S. Duhan, Mater. Res. Innovations, 2023, 00, 1–12 Search PubMed.
- D. Zhang, D. Wang, X. Zong, G. Dong and Y. Zhang, Sens. Actuators, B, 2018, 262, 531–541 CrossRef CAS.
- L. A. Solovyov, O. V. Belousov, R. E. Dinnebier, A. N. Shmakov and S. D. Kirik, J. Phys. Chem. B, 2005, 109, 3233–3237 CrossRef CAS PubMed.
- B. J. F. Young, J. Appl. Chem., 1967, 17, 241–245 CrossRef.
- B. Echchahed, A. Moen, D. Nicholson and L. Bonneviot, Chem. Mater., 1997, 9, 1716–1719 CrossRef CAS.
- R. Schmidt, M. Stöcker, D. Akporiaye, E. Heggelund Tørstad and A. Olsen, Microporous Mater., 1995, 5, 1–7 CrossRef CAS.
- M. Panday, G. K. Upadhyay and L. P. Purohit, J. Alloys Compd., 2022, 904, 164053 CrossRef CAS.
- S. Gao, P. Zhang, Z. Wang, G. Cui, J. Qiu and J. Wang, ACS Sustainable Chem. Eng., 2020, 8, 586–593 CrossRef CAS.
- D. Zhao, S. Budhi, A. Rodriguez and R. T. Koodali, Int. J. Hydrogen Energy, 2010, 35, 5276–5283 CrossRef CAS.
- Y. Xia, H. Zhao, S. Liu and T. Zhang, RSC Adv., 2014, 4, 2807–2812 RSC.
- P. V. Adhyapak, U. P. Mulik, D. P. Amalnerkar and I. S. Mulla, J. Am. Ceram. Soc., 2013, 96, 731–735 CrossRef CAS.
- R. G. Deshmukh, S. S. Badadhe and I. S. Mulla, Mater. Res. Bull., 2009, 44, 1179–1182 CrossRef CAS.
- P. V. Adhyapak, V. Kadam, U. Mahadik, D. P. Amalnerkar and I. S. Mulla, Ceram. Int., 2013, 39, 8153–8158 CrossRef CAS.
- Q. Qi, T. Zhang, X. Zheng and L. Wan, Sens. Actuators, B, 2008, 135, 255–261 CrossRef CAS.
- H. Yu, S. Gao, X. Cheng, P. Wang, X. Zhang, Y. Xu, H. Zhao and L. Huo, Sens. Actuators, B, 2019, 297, 126744 CrossRef CAS.
- H. Zhao, S. Liu, R. Wang and T. Zhang, Mater. Lett., 2015, 147, 54–57 CrossRef CAS.
- D. Toloman, A. Popa, M. Stan, C. Socaci, A. R. Biris, G. Katona, F. Tudorache, I. Petrila and F. Iacomi, Appl. Surf. Sci., 2017, 402, 410–417 CrossRef CAS.
- P. Pascariu, A. Airinei, N. Olaru, I. Petrila, V. Nica, L. Sacarescu and F. Tudorache, Sens. Actuators, B, 2016, 222, 1024–1031 CrossRef CAS.
- Q. Kuang, C. Lao, Z. L. Wang, Z. Xie and L. Zheng, J. Am. Chem. Soc., 2007, 129, 6070–6071 CrossRef CAS PubMed.
- A. Kumar, P. Kumari, M. S. Kumar, G. Gupta, D. D. Shivagan and K. Bapna, Ceram. Int., 2023, 49, 24911–24921 CrossRef CAS.
- M. Velumani, A. Prasanth, S. Narasimman, A. Chandrasekhar, A. Sampson, S. R. Meher, S. Rajalingam, E. Rufus and Z. C. Alex, Coatings, 2022, 12, 1989 CrossRef CAS.
- N. Shehada, S. Christiansen, M. Leja, H. Haick and K. Funka, Nano Lett., 2015, 15, 1288–1295 CrossRef CAS PubMed.
- D. Wang, D. Zhang, P. Li, Z. Yang, Q. Mi and L. Yu, Nano-Micro Lett., 2021, 13, 1–13 CrossRef CAS PubMed.
- D. Zhang, Z. Xu, Z. Yang and X. Song, Nano Energy, 2020, 67, 104251 CrossRef CAS.
- H. Zhang, W. Gu and C. Chen, Appl. Surf. Sci., 2022, 599, 154031 CrossRef CAS.
- P. M. Faia, E. L. Jesus and C. S. Louro, Sens. Actuators, B, 2014, 203, 340–348 CrossRef CAS.
- B. Yao, H. Shi, H. Bi and L. Zhang, J. Phys.: Condens. Matter, 2000, 12, 6265 CrossRef CAS.
- T. Morimoto, M. Nagao and F. Tokuda, J. Phys. Chem., 1969, 73, 243–248 CrossRef CAS.
- E. McCafferty and A. C. Zettlemoyer, Discuss. Faraday Soc., 1971, 52, 239–254 RSC.
- M.L. Hair and W. Hertl, Adsorpt. J. Int. Adsorpt. Soc, 1969, 73, 4269–4276 CAS.
- E. Poonia, V. Kiran, J. Sangwan, S. Duhan and K. Kumar, Sens. Lett., 2019, 17, 213–218 CrossRef.
|
This journal is © The Royal Society of Chemistry 2024 |
Click here to see how this site uses Cookies. View our privacy policy here.