DOI:
10.1039/D3NH00570D
(Communication)
Nanoscale Horiz., 2024,
9, 863-872
Graphene oxide–DNA/graphene oxide–PDDA sandwiched membranes with neuromorphic function†
Received
18th December 2023
, Accepted 15th March 2024
First published on 27th March 2024
Abstract
The behavior of polyelectrolytes in confined spaces has direct relevance to the protein mediated ion transport in living organisms. In this paper, we govern lithium chloride transport by the interface provided by polyelectrolytes, polycation, poly(diallyldimethylammonium chloride) (PDDA) and, polyanion, double stranded deoxyribonucleic acid (dsDNA), in confined graphene oxide (GO) membranes. Polyelectrolyte–GO interfaces demonstrate neuromorphic functions that were successfully applied with nanochannel ion interactions contributed, resulting in ion memory effects. Excitatory and inhibitory post-synaptic currents were tuned continuously as the number of pulses applied increased accordingly, increasing decay times. Furthermore, we demonstrated the short-term memory of a trained vs untrained device in computation. On account of its simple and safe production along with its robustness and stability, we anticipate our device to be a low dimensional building block for arrays to embed artificial neural networks in hardware for neuromorphic computing. Additionally, incorporating such devices with sensing and actuating parts for a complete feedback loop produces robotics with its own ability to learn by modifying actuation based on sensing data.
New concepts
The growing interest in information storage devices with high speed, high stability, low power consumption, and large capacity establishes a rigid foundation for the revolutionary rise of chemical memristive technologies. The polyelectrolyte 2D material inhomogeneous interface could serve as the low-dimensional building block for arrays to embed artificial neural networks. The new concept assumes the use of the interaction of 2D materials with synthetic polyelectrolytes and biopolyelectrolytes to achieve asymmetric distribution of polymer chains inside 2D material assembled layers. The proposed concept was demonstrated with the successful work of the two synchronized ionic synapses. The key advantage of the 2D material–polyelectrolyte interface is the stable and reproducible data production at high ionic forces inside the device. The strategy offers a more appropriate way to verify complex membrane materials and polymer composites for application in information storage devices.
|
Introduction
Classical von Neumann computing architecture is coming close to reaching a limit in data transfer between the physically separate processing unit and memory storage, creating a bottleneck for big data calculations.1 In contrast, the human brain uses a massively parallel and fault-tolerant technique, by combining memory and computation.2 Most of the neuromorphic functions accomplished thus far are based on the simulation of the electric pulse pattern using organic polymer devices.3–5 However, an analog of biological synapses, and especially the modulation of a chemical synapse in a water-based medium, remains very demanding with these organic polymer-based devices. Traditional complementary metal oxide semiconductor (CMOS) technology has been successful in mimicking synaptic behavior but requires energy-intensive processes.6–8 This is due to the fundamental difference between electronics and iontronics2 – that is, the nature of charge carriers. Ions as charge carriers have slow mobility and long decay time to ‘memorize’ a recent spike.9 Recently, most artificial synapses either make use of conjugated polymers,10,11 metal nanoparticles,12 doped semiconductors13,14 with low charge mobility and environmental issue or ionic gels, which are highly susceptible to atmospheric humidity, resulting in the instability of device operation.15–18 Among polyelectrolytes, the conjugated types are widely spread as the platforms of the polymer-based neuromorphic devices.19 Water soluble polyelectrolytes usually use as a countercharge for the conjugated polyelectrolytes.20–22 Natural polyelectrolyte coacervates23 also tend to serve in neuromorphic devices owing to the dielectric layer formation.3,24
The common synaptic behavior includes the ion's movement through the membrane's protein channel that initiates synaptic polarization and depolarization. During the polarization/depolarization, the membrane potential changes and initiates the release of ions. The fast ion transportation through biological ion channels is crucial for various life processes such as physiological environment pH stabilization, charge homeostasis, and adenosine triphosphate synthesis. Nano- and sub-nanochannels in proteins exist in the confined state that, together with binding sites, provide an orderly arrangement of ions.25,26 This protein confinement between two lipid bilayer membranes leads to facilitating fast ion transportation. The synthetic polyelectrolytes and natural proteins combine high affinity to particular ions with the ability to exclude other undesired permeants. Natural protein channel dimension is just in the nanoscale or even below it.27 Such distinctive ion transport features and related ones have stimulated the design of biomimetic nanochannels-based devices. Synthetic polyelectrolytes also could be present in the confined structure.28–30 Two-dimensional materials demonstrate the ability to provide nanoscale confinement for synthetic polyelectrolytes.31,32
In addition, not only polyelectrolytes but chemical neuromorphic interfaces of memristors can be made of hydrogels, 2D materials, and 3D frameworks that contain nano- or sub nanopores/channels.33 The rise in attention to two-dimensional materials, such as GO, is not exaggerated. The layered structure of GO membrane serves as channels for ion conduction.34,35 Additionally, oxygenated negatively-charged groups on GO allow it to be functionalized easily with polyelectrolytes.32,36,37 Along with its mechanical toughness and flexibility, it is perfect as a scaffold for ionic gels. Previous studies38 using GO achieved a decay time of 0.1 s which is too quick for any significant computation,39 and another40 still used exposed ionic gels susceptible to leakage and humidity. Peng et al. also proposed the ion diode with horizontally aligned polyelectrolyte-modified CNTs serving as ion channels.41,42 Till now, many polyelectrolyte-solid particles composites have been proposed, including physical confinement by polymer matrix, chemical crosslinking, cationic bonding, and others.43,44 Thus, the creation of interfaces based on water-soluble polyelectrolytes in a confined medium makes it possible to obtain asymmetric ion transport in a neuromorphic device.45 In this manuscript, a new type of artificial synapse is proposed using GO–polyelectrolyte composites. To demonstrate this concept, we used the composite of GO–PDDA, stacked with another composite of GO–dsDNA. The multilayer architecture of GO tends to soak the huge amount of ions46,47 to form ionic channels. Such a phenomenon allows the making of low-resistance neuromorphic devices. The strong synthetic polyelectrolyte PDDA is used as a macromolecular confinement medium that provides uniform distribution of positively charged functional groups inside the GO nanochannels.32 The dsDNA are amphiphilic rigid polymeric molecules. The presence of DNA in GO layers leads to the induction of either negative or positive ionic current modulation.48–50
Experimental
Fabrication methods
Membranes were prepared by vacuum filtration with polyethersulfone (PES) filter (Sterlitech, PES00347100, 0.03 Micron, 47 mm) of the solution mixture consisting 5 mg of 1 mg ml−1 graphene oxide water dispersion (Graphenea, 0.4 wt% concentration), 10 mg of 1 mg ml−1 poly(diallyldimethylammonium chloride) (PDDA) (Sigma Aldrich, average Mw < 100
000, 522376) or double-stranded deoxyribonucleic acid (dsDNA) (Sigma Aldrich, deoxyribonucleic acid sodium salt from herring testes, Type XIV, D6898). After drying, 5 ml of 0.2 M lithium chloride solution (Sigma Aldrich, 310468) was added to the membranes and vacuum filtrated. The membranes were then left in the dry cabinet of 30% relative humidity (RH) for 24 hours to dry and stored in 57% RH using saturated sodium bromide (Sigma Aldrich, 310506) at room temperature. Composite stack membrane was combined by membrane fishing, which involved allowing GO–PDDA membrane to float on the surface of 0.2 M LiCl solution and using GO–dsDNA to scoop up GO–PDDA. ICP results and electrical measurements demonstrated that this did not result in an addition of LiCl ions to increase conductivity of the composite stack as compared to single membranes. Composite stack membranes were allowed to dry for 24 hours in 30% RH. The composite stack membrane was cut using scissors to 1.7 cm by 0.5 cm. Carbon tape (conductive carbon discs, Agar Scientific, AGG3111) were used as electrodes of 0.5 cm by 0.5 cm. Before conducting synaptic measurements, 0.5 V along was applied across source–drain electrodes for at least an hour until current plateaus to allow the release of trapped ions. The same was conducted afterward across the source-gate electrodes for 15 min until current plateaus. Leave the device at 57% RH for 24 hours before electrical measurements.
Electrical characterization
Electrical characterization was done using a Keithley 2401, supplemented by Tektronix Arbitrary Function generator AFG1022 and interpreted with customized LabView software. Two-terminal electrochemical impedance spectroscopy (EIS) measurements were taken using BioLogic SP-300 Potentiostat and low current probe with AC voltage ±10 mV.
Materials characterization
Scanning electron microscope (SEM) images were taken using Carl Zeiss AG, SUPRA 40. Thicknesses of membranes were measured using KLA Tencor Alpha-Step IQ Surface Profiler. Surface Zeta potential of membranes and dispersion were measured using Anton Paar SurPASS 3 and Malvern Panalytical Zetasizer Ultra, respectively. Solid samples for ICP measurement were each digested with HNO3/HCl (3
:
1) on hotplate for 2 hours and topped up to 10 ml with distilled water. PerkinElmer Avio 500 inductively coupled plasma-optical emission spectrometer (ICP-OES) was used to analyze concentration of Li+ and Na+ in extracted liquids from solid samples, additionally analysis of Li+, Na+ and Cl− were conducted using leftover solution of 0.2 M LiCl after membrane fishing. XRD was carried out using Bruker D8 ADVANCE Diffractometer with Cu Kα radiation (λ = 0.1514 nm) using LYNXEYE_XE_T detector in 1D mode.
Results and discussion
The neuromorphic device is prepared by stacking of GO–PDDA and GO–dsDNA composites (Fig. 1a and b). First, the GO–PDDA and GO–dsDNA composites were prepared by vacuum filtration. During vacuum filtration, the polyelectrolyte–GO confined medium has been assembled. Both PDDA and dsDNA associate with GO in water as fewer oxygenated groups are exposed51 due to hydrophobic attraction between sp2 groups on GO and diallyldimethyl groups in PDDA52 and exposed nucleobases from breathing fluctuations of dsDNA.53,54 Atomic force microscopy (AFM) images demonstrated uniform distribution of the polyelectrolytes on GO flakes (Fig. 1a and Fig. S2, ESI†). Due to the electrostatic binding between GO and polyelectrolytes,55,56 a stable multilayered composite is formed. The microroughness of the material surfaces is indicative of the homogeneity of the composites. High contrast in the phase image obtained at higher resolution (Fig. S2, ESI†) showed the difference in surface morphology of polyelectrolytes and GO sheets induced by their different stiffness and surface functionalities. Second, both composites (GO–PDDA and GO–dsDNA) were sandwiched and doped with 0.001 M − 0.2 M LiCl salt to introduce mobile charges. Such a strategy allows the preparation of self-assembled heterostructures with a network of ionic channels.32
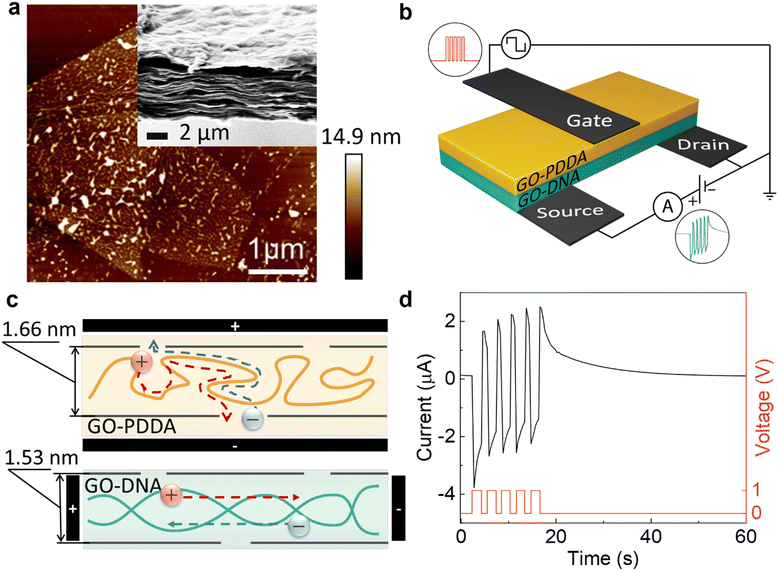 |
| Fig. 1 Structure and function of sandwiched membrane. (a) Atomic force microscopy (AFM) image of GO–dsDNA composite, demonstrating uniformity of polymer distribution. Onset: Scanning electron microscopy (SEM) image of the sandwiched composites of the GO–dsDNA (top) and GO–PDDA (bottom) indicating lamellar morphology and seamless connection between the two composites. (b) Schematic of artificial synapse with carbon tape electrodes (in black), where +1 V (or −1 V) pulses were applied via the presynaptic (gate) electrode. Source–drain current was measured using the post-synaptic (source) electrode with a constant voltage of +0.5 V (grounded). (c) Sketch of an ionic channel in GO–PDDA composite (top) and an ionic channel in GO–dsDNA composite (bottom) with Li+ (red spheres) and Cl− (blue spheres). Arrows indicate the preferred direction of ionic flow in the presence of applied potential difference due to the macromolecules’ alignment. Interlayer distances of the layers are measured using X-ray diffraction (XRD) (Fig. S5, ESI†). (d) Paired-pulse facilitation (PPF) of the synapse. The plot of source–drain current exhibited the excitatory post-synaptic current (EPSC) as current increased after each pulse and slowly decayed over time. Five +1 V pulses were applied with pulse duration of 2 s and rest duration of 1 s. | |
SEM image (Fig. 1a and Fig. S1, ESI†) demonstrates that both composites GO–dsDNA and GO–PDDA have lamellar cross-section morphology. Two composites are seamlessly assembled into a sandwich. Interlayer distances of the layers measured using X-ray diffraction (XRD) (Fig. S5, ESI†) are 1.66 nm in GO–PDDA and 1.53 nm in GO–dsDNA. Due to a multiple cross-linking between GO and polyelectrolytes, the layers are stabilized to prevent the well-known swelling issues (i.e., expansion of interlayer spacing or even resuspension) that is typical for the materials with ionic channels in the aqueous solutions. Thus, the devices are capable of producing a stable output signal for at least a few months in atmospheric conditions and more than 6 months at 59% relative humidity (Fig. S9, ESI†).
Synaptic characteristics of artificial synapse
The key decision-maker in the nervous system is the synapse - the connection between neurons. A synapse transmits information when a fired pre-synaptic neuron controls the flow of ions in and out of the post-synaptic neuron, modifying the potential difference across the cell membrane of the post-synaptic neuron.57 This results in an excitatory post-synaptic current (EPSC) or inhibitory post-synaptic current (IPSC), depending on the neurotransmitter used for signaling. EPSC (or IPSC) brings the current higher (or lower) with each spike given, either from the same pre-synaptic neuron or another pre-synaptic neuron. The more spikes given and in shorter intervals, the higher (or lower) the current, this is called spike-timing-dependent plasticity (STDP).58 STDP modifies the synaptic weight, which is defined as the strength or amplitude of a connection between two neurons; in other words, it modifies the likelihood of the post-synaptic neuron firing. Such experience-dependent behavior of synapses allows for short-term memory computations in the human brain.
The three-terminal device architecture (Fig. 1b) comprises of presynaptic (gate) electrode on the GO–PDDA composite where pulses were applied and modification of channel conductance along the GO–dsDNA composite measured using the post-synaptic (source and drain) electrodes contributes to its synaptic weight. Fig. 1d illustrates EPSC with increasing current after each positive pulse was applied, indicating paired-pulse facilitation (PPF) similar to a neuron. We show that this artificial synapse exhibits the synaptic functions and can be used as the building blocks of neuromorphic computing.
The advantage of our system is the achievement of regulated ionic currents that flow through the ionic channels in the device.
The changes in the conductivity of the composite stack are closely bounded with ion dynamic motion inside polyelectrolyte confined medium and GO nanochannels. The probable mechanism for the layers’ organization is attributed to the confinement effects of GO nanosheets on the alignment of macromolecules.59 The schematic in Fig. 1c illustrates the preferred direction of ionic flow in the presence of applied potential difference along rigid dsDNA chains and cross-flexible PDDA. The measure of chain rigidity can be estimated using the persistence length, which is 50 nm for DNA, and the length of the Kuhn segment, approximately 1 nm, for PDDA.60 Furthermore, both dsDNA and PDDA are strong polyelectrolytes. Their functional groups are fully charged that provide pathways for ionic flow.61 Therefore, the flexible PDDA chains tends to pack into loose coils in GO nanochannels. Such coils restrict Li+ ion tunneling along the polyelectrolyte chain. The preferable ionic current flow for the GO–PDDA composite (Fig. 1c) is across the composite. In contrast, the rigid dsDNA macromolecules form a separate confined phase for ionic flux along GO surfaces. Thus, the novelty of our devices is in the presence of two types of ionic nanochannels with cross-directional ionic flow.
To quantitatively evaluate the ionic flow in the nanochannels, we conducted dynamic monitoring of ion conductivity under different number of pulses. The overall high output current is provided by appropriate LiCl concentration (0.2 M), which was found from the IV curve with testing 0.001–0.2 M concentration range (Fig. S3, ESI†). Fig. 2 shows that the artificial synapse exhibit STDP in accordance with the number of applied pulses. As more positive pulses were applied, source–drain current took longer to decay to its original current, as seen in Fig. 2a. The curvatures of these decay curves were best fitted with the exponential decay equation, which is typical for computing human neuronal potential,62I = I0+ A1exp(−t/τ1) + A2exp(−t/τ2), where I is source–drain current and t is time taken in s. Fitted constants: I0 represented the original current as 0.5 V is applied along source and drain electrodes, A1,2 represented the curvature of the exponential function and τ1,2 represented its decay constant. As expected, the initial current remained unchanged, while curvature and decay constant continuously increased as number of pulses increased. The post-synaptic decay curves data (Fig. 2) for the EPSC and IPSC current of the composite stack shows the different current values that caused different ion redistribution in the GO–PDDA and GO–dsDNA. The different ion redistribution leads to the drop in τ = RC. Two exponents correspond to two types of ions participating in the charging effects. Despite the charge of the membranes (Fig. S7, ESI†), the ratio of Li+ and Cl− ions remained close to 1
:
1 according to ICP measurements and were also 10 to 100 times more saturated than charges present on GO or polymers (Tables S1–S3, ESI†), which means that both ions participate in charge transfer. The difference in LiCl swelling ability for the GO–PDDA and GO–dsDNA is caused by the different initial charges of the GO–polyelectrolyte composite (Fig. S7, ESI†). The decay curves (a, d) and time constants show that one type of the ions gives smaller values, so synaptic behavior and longer decay times are mostly determined by the slow kinetics of only one type of ions. It can be noticed that the relationships between curvature or decay constant and number of pulses were not linear. Exponential decay after applying pulses corresponds to the discharge of the formed double layer on the electrodes with τ ∼ RC. The double layer can be represented as a capacitor with parallel resistance (Fig. S10, ESI†). Two different capacitors and resistances can imitate the charge/discharge of anions and cations, which would give two various time decay constants. Both Ai and decay times τi eventually saturate after applying more than 200 pulses, telling us about the double layer's full charge and defining the synaptic device's working range. However, the iontronic device had a wide range of decay times as a result of slow kinetics of ions, improving device sensitivity.
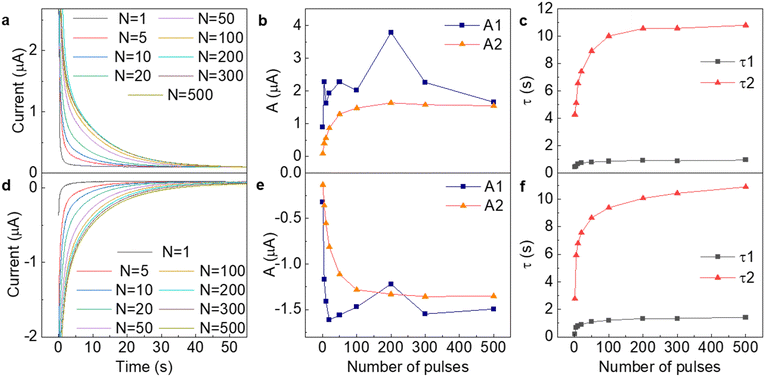 |
| Fig. 2 Synaptic behavior. (a)–(c) Results of excitatory post-synaptic decay curves from 1 to 500 pulses of +1 V with pulse duration of 0.2 s and rest duration of 0.1 s. (a) Results of EPSC (in solid lines) which are fitted with quite high accuracy with decay equation: I = I0+ A1exp(−t/τ1) + A2exp(−t/τ2), where A1,2 are plotted in (b) and τ1,2 are plotted in (c). Fitted curves completely coincide with experimental curves. A1,2 showed a continuously increasing amplitude of the decay curve, while τ1,2 demonstrated a continuously increasing time constant of decay as the number of pulses increased. (d)–(f), Results of inhibitory post-synaptic decay curves from 1 to 500 pulses of negative voltage of −1 V with the same input parameters and fitting, as plotted in (e) and (f). | |
Mechanisms of synaptic behavior
To elucidate the mechanism of synaptic behavior in our devices, two-terminal measurements were taken in various configurations and combinations of the composites along (Fig. 3a) and across (d) the composite. The combinations with two layers of GO–dsDNA or GO–PDDA can be found in SI (Fig. S12–S16, ESI†). Electrochemical impedance spectroscopy (EIS) measures AC impedance determined frequency-independent resistance of each configuration. Nyquist plots for along (Fig. 3c) and across (f) the composites were fitted to either first- or second-order RC model (Fig. S14, ESI†), depending on the presence of two separate layers in the composite. GO–dsDNA showed low resistance along the composite while high resistance across, and the reverse is true for the GO–PDDA composite. This observation can be intuitively understood from the differing structure of these membranes (Fig. 1c). Rigid chains of dsDNA support ionic transport along the membrane. Flexible PDDA, on the other hand, with exposed hydrophobic main chain interacts well with hydrophobic pristine graphene on GO and intersperse between GO layers. Oxygenated groups on GO form clasters63 creating ionic channels along the GO surface as the preferred direction of ionic movement in GO–PDDA. The GO–PDDA and GO–dsDNA composite stack combines with these advantages and showed to have decreased resistance in both the along and across directions, that is not observed in the combination of two GO–dsDNA membranes or two GO–PDDA composites (Fig. S13 and S14, ESI†).
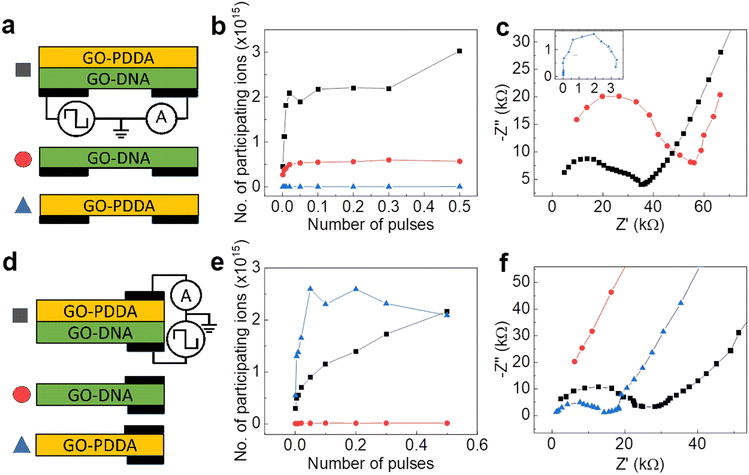 |
| Fig. 3 Working principle of synaptic behavior. (a) Schematic of electrical circuit used for (b) and (c) along the GO–polyelectrolyte composites. (d) Schematic of electrical circuit used for (e) and (f) for across the GO–polyelectrolyte composites. (b) and (e) Number of ions participating in charge transfer against number of 1 V pulses applied on the source electrode with pulse duration of 0.2 s and rest duration of 0.1 s. EPSC Decay curves were fitted with the exponential decay equation: I = I0+ A1exp(−t/τ1) + A2exp(−t/τ2), where τ2 was the higher time decay constant and major contribution on synaptic behaviour. Capacitance was calculated from τ2= RC, where resistance R was taken from fitted Nyquist plots (c) and (f), the larger resistance chosen as major contribution on synaptic behaviour. Charge transferred, Q = CV then determines the number of Li+ and Cl− ions participating in charge transfer. (c) and (f) Nyquist plot of AC impedance measurements. The inset in (c) shows high impedance region. Single membranes GO–DNA (along) and GO–PDDA were fitted to first-order RC model, while the combined membrane and GO–DNA (across) were fitted to second-order RC model, due to separate layers of GO–DNA and GO–PDDA or GO and DNA in the GO–DNA membrane. | |
By measuring EPSC decay curves in accordance with the circuits shown in Fig. 3a and d, τ2 was similarly extracted as the higher time decay constant and major contribution on synaptic behavior (Fig. S11 and S12, ESI†). From Nyquist plots the higher resistance value, R, was also extracted as the major contribution on synaptic behavior. Capacitances, C, for each configuration were calculated, using relation τ2= RC (Fig. S15, ESI†). Since C is highly frequency-dependent owing to the amount of time needed for charge/discharge, it cannot be directly extracted from AC impedance measurements as τ2 values are quite high, but only from EPSC. Therefore, using Q = CV, where V is 1 V applied pulses, the charge, Q, and therefore number of Li+ and Cl− participating in charge transfer could be calculated (Fig. 3b and e and Fig. S16, ESI†). All configurations indicated a greater number of participating ions as the number of pulses increased. This was particularly observed for pulses applied across the composite stack. As pulses to the synapse were applied across the composite stack while source–drain current is measured along the GO–dsDNA, it is likely that GO–PDDA acted as an ion source to insert ions into GO–dsDNA and increased its conductance for it to display synaptic behavior. Number of ions transferred was also higher across the GO–PDDA membrane and along the GO–dsDNA membrane, supporting this view.
Although all configurations of the membranes displayed EPSC/IPSC behavior (Fig. S11 and S12, ESI†), only the composite stack in three-terminal electrode configuration demonstrated long decay times with smooth relation according to number of pulses (Fig. 2 and Fig. S8, ESI†). In such device the GO–dsDNA serves as the source–drain part and provides ionic conductivity along the GO–dsDNA composite, while GO–PDDA composite serves as a gate electrode. The applied constant voltage results in Li ions flux to the drain. The Li+ moves along the channels and generates two output currents—the drain current and an additional gate current, effectively doubling the number of reservoir states. The gate current is caused by applying the pulse voltage to the GO–PDDA composite, which leads to the Li+ flux across the GO–PDDA composite and the opening of the nanochannels that are initially blocked by dsDNA. The different rates of ionic transport in the GO–PDDA and the GO–dsDNA delay the drain current's response compared to the gate current. This delayed response enables short-term memory possibilities within the device, endorsing the reservoir to retain and utilize information from past inputs.
Demonstration of artificial synapse in computation
To demonstrate the use of the suggested synapse in computation, a binary logic was conducted on two synapses (Fig. 4 and Fig. S17, ESI†). One of the synapses was trained with 300 pulses, while the other one was left untrained. Afterwards, while the synapse still remembers its state, test pulses were applied. As expected, only trained synapses would continuously display a higher current for a short-term duration, even when certain test pulses were applied to the trained and/or untrained synapses. The synapse then achieved a form of short-term memory. Once this synapse ‘forgot’ its training as current returned to normal, the other synapse was trained instead. Implementing linear model for our case
, which relates the output ym to the input xm through the weights wn,m. By utilizing synaptic devices with conductance Gn,m, we can represent this model as
, where Im denotes the total signal (current) for a given synapse. In our case, considering two synapses, the total current I can be expressed as I = I0 + G1V1 + G2V2, where I0 represents a baseline current. Setting the activation function to be at specific value which can be achieved only when two synapses are trained, logical AND operator can be realized, demonstrating a binary logic. This perceptron for the inverse AND operator is demonstrated in Fig. 4c, where pulses were automatically applied when the current drops below a setpoint value. Fig. 4c shows three cases of different synapse weights for two samples. In cases 1 and 2, synapse 1 (G1 = 57 and 37 Ω−1 for case 1 and 2, respectively) has weight higher than the second synapse (G1 = 14 Ω−1), which is mostly untrained and is close to its base current. In case 3, both synapses have same setpoint current of I = 36 nA, thus, having similar weights (G1 = G2 = 51 Ω−1). Eventually, a grid of such synapses could be manufactured for more complex training and memory functions by increasing the number of nodes or layers similar to an artificial neural network. For example, such memristive device could be implemented for developing alternative computational platforms,67,68 for emulation skin receptors,69,70 and for the restoration of the communication between brain circuitry.71
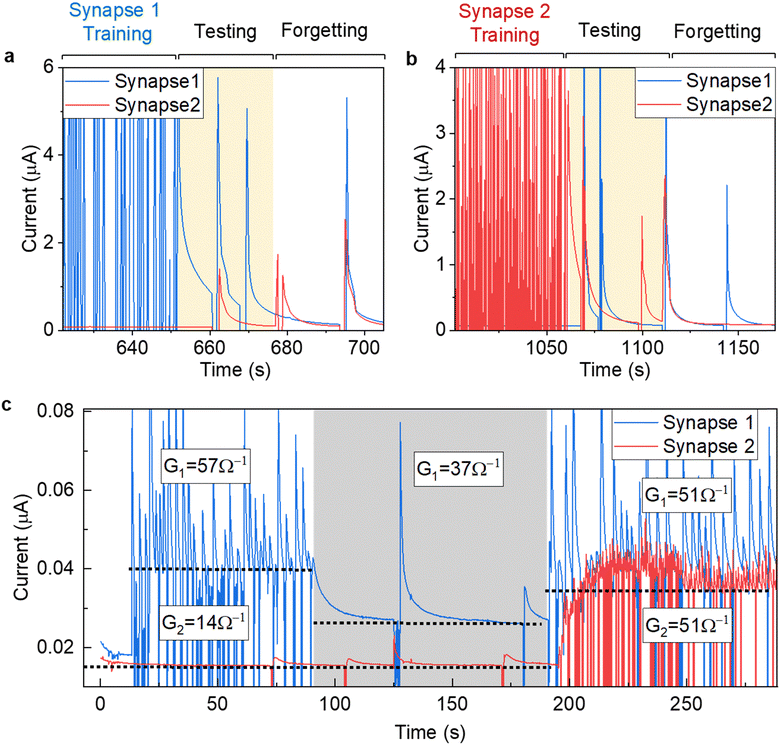 |
| Fig. 4 Binary logic functions with trained and untrained synapses. (a) and (b) EPSC of two synapses measured as one of the synapses was trained with 300 pulses of +1 V with pulse duration of 0.2 s and rest duration of 0.1 s. Either or both synapses were tested with 5 pulses of +1 V with pulse duration of 0.2 s and rest duration of 0.1 s, but trained synapse constantly remain of higher current for approximately 30 s. (a) Synapse 1 (in blue) was trained. (b) Synapse 2 (in red) was trained. (c) EPSC of two synapses computationally trained by automatically applying 10 pulses of +1 V when current drops below setpoint current, indicated by dashed lines. VSD = 0.7 V for both synapses. | |
Thus, the proposed here composite membranes with nanochannels would be best suited as an artificial synapse with high device sensitivity for neuromorphic computation, brain-computer interfaces, medical diagnostics and monitoring. Our devices demonstrate low resistance accompanied by high charge capacity, fast retention time, and high endurance. The performance of our devices is comparable with those that are based on redox memristive behavior and active layer materials.64–66 Nevertheless, the redox-based memristors have application restrictions under high electrolyte concentration. Such a disadvantage makes impossible to integrate redox-based memristors in physiological media. Furthermore, avoiding interfacial redox processes can enhance the biocompatibility of devices, especially those intended for biomedical applications. Our approach that is solely based on regulated ionic transport is an important strategy for improving the biocompatibility of biomedical devices, enhancing their performance, and ensuring their safe and effective use in clinical applications.
Conclusions
This work demonstrates a synaptic device based on the graphene oxide–polyelectrolyte composite stack that exhibits synaptic-like plasticity through the formation of ionic double layers. The structures combination of GO–PDDA and GO–dsDNA composites was essential for improving its synaptic behavior, contributing to prolonged times for decay. This was achieved by providing the polyelectrolyte confined medium in assembled GO nanochannels. The possibility to implement short-term and long-term plasticity makes it possible to realize adaptive learning and memory functions. The system also shows promise in binary logic operations, laying the groundwork for pattern recognition and neural computing applications. New ion-based neuromorphic computing systems may be developed with more study and optimization of the proposed synaptic device.
Author contributions
S. G. and D. V. A. raised the idea, and together with K. G. N and K. S. N. directed the study. J. H. B. carried out all the experimental work and analyzed the data with the help of K. Y., S. C. and C. R. W. D. B. and C. C. P. T. assisted with the collection of some experimental data. J. H. B. wrote the manuscript, S. G. and K. G. N. corrected and finalized the manuscript. All the authors reviewed the final manuscript.
Conflicts of interest
There are no conflicts to declare.
Acknowledgements
This research is supported by the Ministry of Education, Singapore, under its Research Centre of Excellence award to the Institute for Functional Intelligent Materials (I-FIM, project no. EDUNC-33-18-279-V12).
Notes and references
-
H. Thimbleby in Modes, WYSIWYG and the von Neumann bottleneck. IEEE Colloquium on Formal Methods and Human-Computer Interaction: II 1–5 (IET, 1988) Search PubMed.
- C. Mead, Neuromorphic Electronic Systems, Proc. IEEE, 1990, 78, 1629–1636 CrossRef.
- T. Xiong,
et al., Neuromorphic functions with a polyelectrolyte-confined fluidic memristor, Science, 1979, 379, 156–161 CrossRef PubMed.
- K. K. Kim,
et al., A substrate-less nanomesh receptor with meta-learning for rapid hand task recognition, Nat. Electron., 2023, 6, 64–75 Search PubMed.
- Y. van de Burgt, F. Santoro, B. Tee and F. Alibart, Editorial: Focus on organic materials, bio-interfacing and processing in neuromorphic computing and artificial sensory applications, Neuromorphic Comput. Eng., 2023, 3, 040202 CrossRef.
- G. Indiveri, E. Chicca and R. Douglas, A VLSI array of low-power spiking neurons and bistable synapses with spike-timing dependent plasticity, IEEE Trans. Neural Networks, 2006, 17, 211–221 CrossRef PubMed.
- E. Chicca,
et al., A VLSI Recurrent Network of Integrate-and-Fire Neurons Connected by Plastic Synapses With Long-Term Memory, IEEE Trans. Neural Networks, 2003, 14, 1297–1307 CrossRef CAS PubMed.
- J. V. Arthur and K. A. Boahen, Silicon-Neuron Design: A Dynamical Systems Approach, IEEE Trans. Circuits Syst., 2011, 58, 1034–1043 Search PubMed.
- H. Chun and T. D. Chung, Iontronics, Annu. Rev. Anal. Chem., 2015, 8, 441–462 CrossRef CAS PubMed.
- L. Shao, Y. Zhao and Y. Liu, Organic Synaptic Transistors: The Evolutionary Path from Memory Cells to the Application of Artificial Neural Networks, Adv. Funct. Mater., 2021, 31, 1–16 Search PubMed.
- W. Xu, S. Y. Min, H. Hwang and T. W. Lee, Organic core-sheath nanowire artificial synapses with femtojoule energy consumption, Sci. Adv., 2016, 2, 1–8 Search PubMed.
- C. Novembre, D. Guérin, K. Lmimouni, C. Gamrat and D. Vuillaume, Gold nanoparticle-pentacene memory transistors, Appl. Phys. Lett., 2008, 92, 90–93 CrossRef.
- T. F. Yu,
et al., Solution-Processable Anion-doped Conjugated Polymer for Nonvolatile Organic Transistor Memory with Synaptic Behaviors, ACS Appl. Mater. Interfaces, 2020, 12, 33968–33978 CrossRef CAS PubMed.
- C. Qian,
et al., Artificial Synapses Based on in-Plane Gate Organic Electrochemical Transistors, ACS Appl. Mater. Interfaces, 2016, 8, 26169–26175 CrossRef CAS PubMed.
- Y. Van De Burgt,
et al., A non-volatile organic electrochemical device as a low-voltage artificial synapse for neuromorphic computing, Nat. Mater., 2017, 16, 414–418 CrossRef CAS PubMed.
- Y. Kim,
et al., A bioinspired flexible organic artificial afferent nerve, Science, 1979, 360, 998–1003 CrossRef PubMed.
- Y. Lee,
et al., Stretchable organic optoelectronic sensorimotor synapse, Sci. Adv., 2018, 4, 1–10 Search PubMed.
- F. Torricelli,
et al., Electrolyte-gated transistors for enhanced performance bioelectronics, Nat. Rev. Methods Primers, 2021, 1, 66 CrossRef CAS PubMed.
- R. He,
et al., Organic Electrochemical Transistor Based on Hydrophobic Polymer Tuned by Ionic Gels, Angew. Chem., Int. Ed., 2023, 62, e202304549 CrossRef CAS PubMed.
- F. Mariani,
et al., High-Endurance Long-Term Potentiation in Neuromorphic Organic Electrochemical Transistors by PEDOT:PSS Electrochemical Polymerization on the Gate Electrode, ACS Appl. Mater. Interfaces, 2023 DOI:10.1021/acsami.3c10576.
- P. Gkoupidenis, N. Schaefer, B. Garlan and G. G. Malliaras, Neuromorphic Functions in PEDOT:PSS Organic Electrochemical Transistors, Adv. Mater., 2015, 27, 7176–7180 CrossRef CAS PubMed.
- Y. van de Burgt, F. Santoro, B. Tee and F. Alibart, Editorial: Focus on organic materials, bio-interfacing and processing in neuromorphic computing and artificial sensory applications, Neuromorphic Comput. Eng., 2023, 3, 040202 CrossRef.
- C. Zhang,
et al., Natural polyelectrolyte-based ultraflexible photoelectric synaptic transistors for hemispherical high-sensitive neuromorphic imaging system, Nano Energy, 2022, 95, 107001 CrossRef CAS.
- L. Yu, X. Li, C. Luo, Z. Lei, Y. Wang, Y. Hou, M. Wang and X. Hou, Nano Res., 2024, 17, 503–514 CrossRef.
- B. Lu, T. Xiao, C. Zhang, J. He and J. Zhai, Small Struct., 2023, 4, 2300190 CrossRef CAS.
- A. Alcaraz, M. L. López, M. Queralt-Martín and V. M. Aguilella, ACS Nano, 2017, 11, 10392–10400 CrossRef CAS PubMed.
- L. Bocquet and E. Charlaix, Chem. Soc. Rev., 2010, 39, 1073–1095 RSC.
- J. de Carvalho, R. Metzler and A. G. Cherstvy, Soft Matter, 2015, 11, 4430–4443 RSC.
- L. Xie, K.-Y. Chan and V. C.-Y. Li, Mol. Simul., 2022, 48, 926–934 CrossRef CAS.
- J. Landsgesell, L. Nová, O. Rud, F. Uhlík, D. Sean, P. Hebbeker, C. Holm and P. Košovan, Soft Matter, 2019, 15, 1155–1185 RSC.
- M. Zhang, K. Guan, Y. Ji, G. Liu, W. Jin and N. Xu, Nat. Commun., 2019, 10, 1253 CrossRef PubMed.
- D. V. Andreeva,
et al., Two-dimensional adaptive membranes with programmable water and ionic channels, Nat. Nanotechnol., 2021, 16, 174–180 CrossRef CAS PubMed.
- R. A. Lucas, Ionic Amplifying Circuits Inspired by Electronics and Biology, Biophys. J., 2020, 118, 159a CrossRef.
- C. Du,
et al., Synaptic transistors and neuromorphic systems based on carbon nano-materials, Nanoscale, 2021, 13, 7498–7522 RSC.
- P. Sun,
et al., Selective ion penetration of graphene oxide membranes, ACS Nano, 2013, 7, 428–437 CrossRef CAS PubMed.
- Y. Xu, Q. Wu, Y. Sun, H. Bai and G. Shi, Three-dimensional self-assembly of graphene oxide and DNA into multifunctional hydrogels, ACS Nano, 2010, 4, 7358–7362 CrossRef CAS PubMed.
- P. Yang,
et al., Intrinsic proton conductive deoxyribonucleic acid (DNA) intercalated graphene oxide membrane for high-efficiency proton conduction, J. Membr. Sci., 2020, 606, 118136 CrossRef CAS.
- C. Jin Wan,
et al., Flexible Metal Oxide/Graphene Oxide Hybrid Neuromorphic Transistors on Flexible Conducting Graphene Substrates, Adv. Mater., 2016, 5878–5885, DOI:10.1002/adma.201600820.
- Y. van De Burgt, A. Melianas, S. T. Keene, G. Malliaras and A. Salleo, Organic electronics for neuromorphic computing, Nat. Electron., 2018, 1, 386–397 CrossRef.
- J. Sun,
et al., Optoelectronic Synapse Based on IGZO-Alkylated Graphene Oxide Hybrid Structure, Adv. Funct. Mater., 2018, 28, 1–9 Search PubMed.
- R. Peng,
et al., Understanding Carbon Nanotube-Based Ionic Diodes: Design and Mechanism, Small, 2021, 17, 2100383 CrossRef CAS PubMed.
- R. Peng,
et al., Ionotronics Based on Horizontally Aligned Carbon Nanotubes, Adv. Funct. Mater., 2020, 30, 2003177 CrossRef CAS.
- Y. Xing,
et al., Integrated opposite charge grafting induced ionic-junction fiber, Nat. Commun., 2023, 14, 2355 CrossRef CAS PubMed.
- W. Wang,
et al., Neuromorphic sensorimotor loop embodied by monolithically integrated, low-voltage, soft e-skin, Science, 2023, 380, 735–742 CrossRef CAS PubMed.
- L. Mao, Neuromorphic Sensing: A New Breed of Intelligent Sensors, ACS Sens., 2023, 8, 2896–2897, DOI:10.1021/acssensors.3c01608.
- Y. Zhao, W. Shi, B. Van der Bruggen, C. Gao and J. Shen, Adv. Mater. Interfaces, 2018, 5, 1701449 CrossRef.
- Y. Zhao, C. Zhou, J. Wang, H. Liu, Y. Xu, J. W. Seo, J. Shen, C. Gao and B. Van der Bruggen, J. Mater. Chem. A, 2018, 6, 18859–18864 RSC.
- Y. Zhang, G. Wu, W. Si, J. Ma, Z. Yuan, X. Xie, L. Liu, J. Sha, D. Li and Y. Chen, Nanoscale, 2017, 9, 930–939 RSC.
- M. Smeets, U. F. Keyser, D. Krapf, M.-Y. Wu, N. H. Dekker and C. Dekker, Nano Lett., 2005, 6, 89–95 CrossRef PubMed.
- M. Caglar and U. F. Keyser, J. Phys. D: Appl. Phys., 2021, 54, 183002 CrossRef CAS.
- A. M. Dimiev, L. B. Alemany and J. M. Tour, Graphene oxide. Origin of acidity, its instability in water, and a new dynamic structural model, ACS Nano, 2013, 7, 576–588 CrossRef CAS PubMed.
- Q. Wang, L. Wang, G. Li and B. Ye, A simple and sensitive method for determination of taxifolin on palladium nanoparticles supported poly (diallyldimethylammonium chloride) functionalized graphene modified electrode, Talanta, 2017, 164, 323–329 CrossRef CAS PubMed.
- S. Kim, C. Park and J. Gang, Effect of pH and salt on adsorption of double-stranded DNA on graphene oxide, J. Nanosci. Nanotechnol., 2015, 15, 7913–7917 CrossRef CAS PubMed.
- H. G. Lee, D. Jin, W. Shim and J. S. Lee, Synergistic effects of crystal structure and surface chemistry of stacked graphene-oxide membranes on the water-permeation mechanism, Desalination, 2020, 492, 114603 CrossRef CAS.
- E. Ahn, H. Gaiji, T. Kim, M. Abderrabba, H.-W. Lee and B.-S. Kim, J. Membr. Sci., 2019, 585, 191–198 CrossRef CAS.
- L. Chu, A. V. Korobko, M. Bus, B. Boshuizen, E. J. Sudhölter and N. A. Besseling, Nanotechnology, 2018, 29, 185301 CrossRef PubMed.
-
L. S. Costanzo, Physiology, Elsevier Health Sciences, Philadelphia, 4th edn, 2009, vol. 1, ch. 1, pp. 15–28 Search PubMed.
- W. G. Regehr and L. F. Abbott, Synaptic computation, Nature, 2004, 431, 796–803 CrossRef PubMed.
-
Y. Grosberg, A. R. Khokhlov and P.-G. de Gennes, Giant molecules: Here, there, and everywhere, World Scientific, Hackensack, NJ, 2011 Search PubMed.
- M. J. Han and V. V. Tsukruk, Trainable bilingual synaptic functions in bio-enabled synaptic transistors, ACS Nano, 2023, 17, 18882–18892 Search PubMed.
- R. L. Abbett, Y. Chen and J. B. Schlenoff, Self-exchange of polyelectrolyte in multilayers: diffusion as a function of salt concentration and temperature, Macromolecules, 2021, 54, 9522–9531 CrossRef CAS.
-
W. Gerstner, W. M. Kistler, R. Naud and L. PaninskiNeuronal dynamics: From single neurons to networks and models of cognition, Cambridge University Press, 2014, pp. 11–14 Search PubMed.
- S. N. Alam, N. Sharma and L. Kumar, Synthesis of Graphene Oxide (GO) by Modified Hummers Method and Its Thermal Reduction to Obtain Reduced Graphene Oxide (rGO)*, Graphene, 2017, 06, 1–18 CrossRef CAS.
- S. Batool, M. Idrees, S.-R. Zhang, S.-T. Han and Y. Zhou, Nanoscale Horiz., 2022, 7, 480–507 RSC.
- Z. Cao, B. Sun, G. Zhou, S. Mao, S. Zhu, J. Zhang, C. Ke, Y. Zhao and J. Shao, Nanoscale Horiz., 2023, 8, 716–745 RSC.
- J. Shi, S. Kang, J. Feng, J. Fan, S. Xue, G. Cai and J. S. Zhao, Nanoscale Horiz., 2023, 8, 509–515 RSC.
- B. Chen, H. Yang, B. Song, D. Meng, X. Yan, Y. Li, Y. Wang, P. Hu, T.-H. Ou, M. Barnell, Q. Wu, H. Wang and W. Wu, Sci. Rob., 2020, 5, eabb6938 CrossRef PubMed.
- P. Kassanos, Sci. Rob., 2020, 5, eabe6818 CrossRef PubMed.
- F. Liu, S. Deswal, A. Christou, Y. Sandamirskaya, M. Kaboli and R. Dahiya, Sci. Rob., 2022, 7, eabl7344 CrossRef PubMed.
- F. Liu, S. Deswal, A. Christou, M. Shojaei Baghini, R. Chirila, D. Shakthivel, M. Chakraborty and R. Dahiya, Sci. Rob., 2022, 7, eabl7286 CrossRef PubMed.
- Y. Mosbacher, F. Khoyratee, M. Goldin, S. Kanner, Y. Malakai, M. Silva, F. Grassia, Y. B. Simon, J. Cortes, A. Barzilai, T. Levi and P. Bonifazi, Sci. Rep., 2020, 10, 7512 CrossRef CAS PubMed.
|
This journal is © The Royal Society of Chemistry 2024 |
Click here to see how this site uses Cookies. View our privacy policy here.