DOI:
10.1039/D3NH00571B
(Communication)
Nanoscale Horiz., 2024,
9, 853-862
Volatile threshold switching and synaptic properties controlled by Ag diffusion using Schottky defects†
Received
18th December 2023
, Accepted 5th March 2024
First published on 5th March 2024
Abstract
We investigated diffusion memristors in the structure of Ag/Ta2O5/HfO2/Pt, in which active Ag ions control active metal ion diffusion and mimic biological brain functions. The CMOS compatible high-k metal oxide could control an Ag electrode that was ionized by applying an appropriate voltage to form a conductive filament, and the movement of Ag ions was chemically and electrically controlled due to oxygen density. This diffusion memristor exhibited diffused characteristics with a selectivity of 109, and achieved a low power consumption of 2 mW at a SET voltage of 0.2 V. The threshold transitions were reliably repeatable over 20 cycles for compliance currents of 10−6 A, 10−4 A, and no compliance current, with the largest standard deviation value of SET variation being 0.028. Upon filament formation, Ag ions readily diffused into the interface of the Ta2O5 and HfO2 layer, which was verified by investigating the Ag atomic percentage using XPS and vertical EDX and by measuring the relaxation time of 0.8 ms. Verified volatile switching device demonstrated the biological synaptic properties of quantum conductance, short-term memory, and long-term memory due to controlling the Ag. Diffusion memristors using designed control and switching layers as following film density and oxygen vacancy have improved results as low-power devices and neuromorphic devices compared to other diffusion-based devices, and these properties can be used for various applications such as selectors, synapses, and neuromorphic devices.
New concepts
We conducted an in-depth study on switching mediums that are compatible with CMOS technology to achieve atomic-level control of conductance changes, resulting in a volatile threshold switching device with multiple levels. Thin films of high-k metal oxides, namely Ta2O5 and HfO2, were characterized using XPS, XRR, and EDX, revealing partial oxidation of Ta–O, Ta, and Hf–O due to the Schottky defect, which facilitated metal ion diffusion. In our engineered memristor structure, Ag/Ta2O5/HfO2/Pt, we demonstrated atomic control over active Ag ions, as verified through electrical measurements including multi-level switching, quantum conductance, and Ag ion relaxation time. Furthermore, our atomically controllable device successfully emulated Ca2+ dynamics similar to those found in biological synapses due to ionic dynamics within the metal oxide. Our device exhibited strengths such as low power consumption, low operating voltage for multi level switching, and high selectivity when compared to other devices. These findings offer insights into the mechanisms of conductive metal-based RRAM and provide a design for controllable conductance properties using metal oxides, which can be directly applied to the semiconductor industry.
|
Introduction
The human brain processes and makes decisions based on a variety of information in a very short time with a low power consumption of ∼20 W compared to the 80 KW power consumption of a conventional computation system.1,2 Therefore, an alternative neuromorphic architecture has been proposed to overcome difficulties with scaling down, high power consumption, and increasing thermal dissipation problems of existing Si-based computing technologies. A key role in the stimulation and response in the brain involves chemical and electrical signals within synapses. The synapse consists of a two-terminal device, where the top electrode acts as a dendrite part and the bottom electrode works as an axon part to help stimulation and response to voltage and current, whereas conductance serves as a synaptic weight. As long as various synaptic weights are updated, learning and forgetting occurs inside the synapses.2–4 The two classic paradigms of synaptic plasticity are spike-rate-dependent plasticity (SRDP) and spike-timing-dependent plasticity (STDP).5 These SRDP or STDP characteristics in three-terminal synaptic devices and neuromorphic systems have been reported.6–9 However, device types that can imitate synaptic characteristics are being studied in various groups using two-terminal devices due to the complicated circuits and large power consumption of three-terminal devices.10–12 Two-terminal resistive-random-access memory (RRAM) has been studied to demonstrate the analog properties of biological synapses with synaptic plasticity, the ability to strengthen or weaken weights. A memristor based on a metal-oxide insulator was investigated to mimic the Ca2+ dynamics of synapses due to the ionic drift in the insulator layer.13–16 Memristors emulating synaptic plasticity include devised bilayer17–21 and triple-layer22,23 of insulators, which were subjected to plasma treatment24 or rapid thermal annealing, and furthermore, the second-order drift memristors were utilized.25–27 Especially, the conductive bridge memory (CBRAM) using novel metal as a top electrode has many advantages such as low power consumption and fast diffusivity of ions, e.g. Ag+ and Cu2+.1 Synaptic plasticity properties such as short-term plasticity (STP) and long-term potentiation (LTP) were demonstrated in CBRAM with the Ag top electrode.28 Diffusion memristors using Ag nanoclusters embedded in SiOxNy, HfOx, and MgOx films were also studied to mimic synaptic Ca2+ ions dynamics in the biological brain according to the STDP learning rule.29 Moreover, the HfOx-based diffusion memristor operates at very low voltages with a large dynamic window of diffusion switching of about 109 magnitudes of the RON/ROFF ratio.29 Diffusion memristors are also called threshold switching (TS), and the unique relaxation kinetics and synaptic plasticity using various materials have been reported.1,30,31 A 4.5 nm thick HfO2 exhibits volatile TS characteristic, indicating significant filament thickness dependence on this electrical behavior.32 This unique switching characteristic could be suitable for selector devices and steep-slope transistor.33 This complimentary resistive switching was studied using the Ag/graphene/h-BN based structure for applying the selector.26 A diffusion memristor of which the switching layer is Ag:SiO2 was utilized as a true random number generator (TRNG), a hardware component that generates a string of random bits for security applications for the era of the internet of things (IoT).34 In this regard, the unique properties of diffuse memristors make it possible to demonstrate short- and long-term synaptic plasticity for neuromorphic devices, and also for selector or generator devices and innovative applications.
The popular synaptic layer of the diffusion memristor is a high-k metal oxide compatible with current CMOS devices.35 The most important thing to consider in diffusion memristor-based synaptic devices is the effective control of active metal ion diffusion to realize stable synaptic plasticity. For example, it has been characterized that metallic Ag ions preferentially diffuse through oxygen vacancies and that Ag diffusion can control nano-ion conversion.36 In addition, it has been reported that Ta2O5, another metal oxide, causes a difference in the composition ratio of the thin film depending on the process conditions when deposited in the sputtering process, affecting the filament of the RRAM device.37 The synaptic properties of these TS devices are affected by the type, thickness, and formation method of the materials used for the metal electrode and the synaptic layer. Although many studies on diffusion memristors using various high-k thin film materials and active metals have been reported, the metal ion diffusion rate in each material is different and control is difficult, so there are differences in reported synaptic properties. Therefore, rather than a single synaptic layer, thin film double layers having a different metal ion diffusion rate within the synaptic layer can be applied to more effectively control the diffusion of metal ions, and research on the electrical properties thereof is lacking, so research on this is necessary.
In this study, Ta2O5/HfO2 double layers were proposed to control active metal ions effectively in diffusion memristors with Ag electrodes. Due to the chemical combination of Ta–O, Ta, and Hf–O of Ta2O5 and HfO2 thin film, the high-k metal oxide had the difference of Ag diffusivity. The design of different oxygen vacancy layers allows us to control the conductive filament by multi-level volatile switching, showing the quantum conductance that the Ag atom controlled was quantal. The mechanism of Ag migration was verified by XPS and vertical EDX and by measuring the current during the relaxation time. This bi-layered device emulated the synaptic plasticity properties of the brain such as the forgetting curve and short- and long-term memory with low operating voltage by applying appropriate frequency electrical pulses due to controllable Ag diffusion.
Results and discussion
Verifying the single layered device with high-k metal oxide
Fig. 1 shows the schematic diagrams for HfO2 and Ta2O5 single layered devices with their accurate stacking sequences and corresponding typical I–V characteristics. Fig. 1(a) shows the bipolar switching characteristics of the HfO2-based CBRAM, where the forming voltage (VF), SET voltage (VSET), and RESET voltage (VRESET) are +1.2 V, +0.8 V, and −0.65 V, respectively. By applying the positive voltage sweep on the Ag top electrode (TE), Ag ions started to migrate into the HfO2 layer, leading to nucleation as Ag atoms at the Pt bottom electrode (BE) after getting electrons from Pt. Finally, Ag atoms formed conductive bridge filaments between the Ag and Pt electrodes. Then, the current immediately increased and the device state changes from a high resistance state (HRS) to a low resistance state (LRS). When the negative sweep was applied on the Ag TE, the RESET process occurs, where the device state changed from LRS to HRS, similar to previous reports.38–40 However, the Ta2O5-based device appears to be a diffusion memristor in I–V characteristics, unlike the HfO2-based device as shown in Fig. 1(b). Upon the positive voltage sweep, the resistance level rapidly decreased at VSET and increased around 0 V, indicating that the device works as a selector at a very small positive voltage range between +0.35 V and +0.2 V, similar to CBRAM-based selector characteristics.41 This volatile SET behavior is attributed to two reasons: filament size and metal ion surface energy. When the thin and unstable filament bridge formation happens, metal ions could be easily diffused even at a very low voltage range. Specifically, filament breakage is easily expected when the filament width is below a certain threshold width.42 In addition, small Ag ion particles with high curvature tend to be dissolved for the reduction of the effective surface energy since nucleation is thermodynamically favorable for the smallest surface energy.29,32,43 This difference in switching characteristics was believed to be due to the difference in Ag diffusivity between Ta2O5 and HfO2 thin films. P. Dorion et al. reported that irretentiveness of conductive filaments is due to the diffusion of Ag atoms, which follow the diffusion equation44 as D = D0
exp (−Ea/kBT), where D is the diffusivity of Ag at temperature T. The D0, Ea, and kB are the maximal diffusivity at infinite temperature, the activation energy for diffusion, and the Boltzmann constant, respectively.
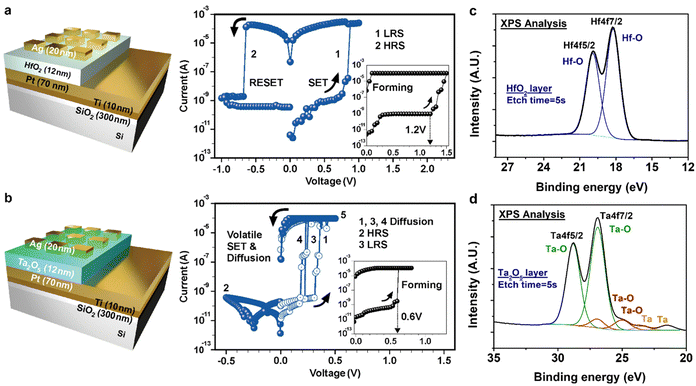 |
| Fig. 1 The schematic diagrams for single layered devices and their corresponding I–V characteristics. (a) A 12 nm-thick HfO2 device shows bipolar characteristic, while (b) a 12 nm-thick Ta2O5 device shows the threshold switching characteristic. The inset graphs in (a) and (b) are the forming properties of single layered devices, which show that VF is +1.2 and +0.6 V in the HfO2 and Ta2O5 device, respectively. The XPS analysis of the HfO2 and Ta2O5 layer devices. Binding energy at an etching time of 5 s of the (c) HfO2 and (d) Ta2O5 based thin films. | |
Therefore, different Ag diffusion behaviors within HfO2 and Ta2O5 cause bipolar and diffusion switching characteristics, respectively.
To verify the mechanism of the thin film HfO2 and Ta2O5 devices, the X-ray photoelectron spectroscopy (XPS) was analyzed, as shown in Fig. 1(c) and (d). It was verified that the HfO2 thin film was fully oxidized, whereas the Ta2O5 film showed partial oxidation, which could be due to the different deposition method. The HfO2 thin film was deposited by the ALD process and Ta2O5 was the PVD process, leading to the oxygen vacancy affecting the filament formation. These Schottky defects generated by the oxygen vacancy increase metal ion mobility and enhance metal diffusion.43,45,46 Based on the verified mechanism of thin film, the Ta2O5 layer was engineered as a control layer of Ag migration and HfO2 was designed as a switching layer. Especially, the TaOx layer is often used as a selector.47,48 Furthermore, the compliance current of the Ag-based CBRAM was modulated according to the oxygen stoichiometry of TaOx, as discussed in a previous paper.49
Design and engineering volatile threshold switching
The Ta2O5/HfO2 bilayered diffusion memristor structure was implemented to enhance device stability, as shown in Fig. 2, which investigated the switching behaviors under different current compliance levels. To optimize the electrical properties of the bilayer device, we engineered and verified the thickness conditions with HfO2/Ta2O5 layers, as shown in Fig. S1 and S2 (ESI†). Fig. 2(a)–(c) correspond to the I–V characteristics for different compliance current levels of 10−6 A, 10−4 A, and the no compliance current, respectively. Compared with the single layer Ta2O5-based device, more reliable and reproducible diffusion properties were obtained with the Ta2O5/HfO2 bilayered device due to the device design of control and switching layer. The black and blue curves indicate the minimum and maximum SET during the sweeping operation and the maximum variations of SET are around 0.054 V, 0.01 V, and 0.1 V for devices with compliance current levels of 10−6, 10−4, and 0 A, respectively. The variations observed during device operation are attributed to the movement of Ag atoms to the amorphous metal oxide, as simulated using the Reverse Monte Carlo method in a previous paper.50 Apparently, the compliance current could control the width of the conductive filament bridge. The wider I–V variation as well as lower average SET are observed with increasing compliance current, which can be explained by shaping of the thicker filament.51 As a result, it has secured a stable diffusion memristor characteristics according to 3 levels of compliance current, 10−6 A, 10−4 A, and the no compliance current.
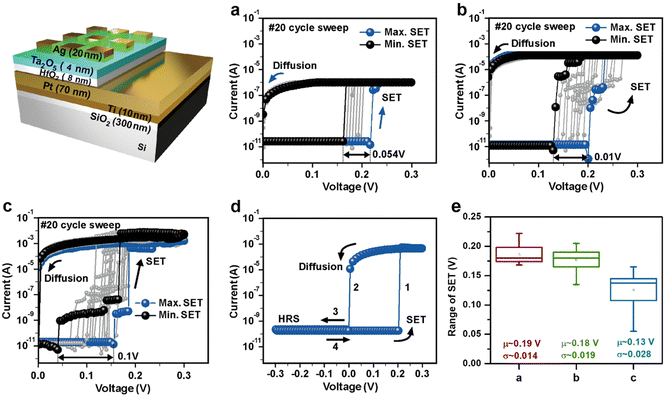 |
| Fig. 2 The schematic diagrams and the I–V curve of the Ta2O5/HfO2 hybrid device with different compliance currents; (a) 10−6 A, (b) 10−4 A, and (c) no compliance current. (d) The maintenance of a high resistance level during the application of the negative DC sweep. (e) The calculated average, μ and standard deviation, σ for different compliance current levels from (a) to (c). | |
Interestingly, the diffusion memristor resistance remains HRS during the negative sweep due to the diffused Ag ions after the SET process during the positive sweep as shown in Fig. 2(d). The control layer, Ta2O5 thin film could control the Ag diffusion and rupture the filament state according to the thermodynamic principle. Hence, we could argue that a hybrid device operated on the metal cation movement with appropriate material and suitable thicknesses can be a suitable structure for the volatile memory, which can mimic Ca2+ ion movement. Besides this tunable switching behavior, power reduction is an added benefit. The average VSET was around 0.13 V for Ta2O5/HfO2 bilayered device while these values of single layered devices were around 0.88 V in HfO2- and 0.35 V in Ta2O5-based device, indicating that the hybrid diffusion memristor is suitable for low operating device applications. Moreover, the statistical analysis of SET was calculated as the average (μ) and standard deviation (σ) in (e), where SET refers the starting voltage from HRS to LRS. The average values remain similar as the compliance current increases; however, the standard deviation values increase from 0.014 to 0.028 with higher compliance current levels. In this figure, SET represents the process of connecting the Ag filament after the formation of the volatile filament. The formation of volatile filaments is influenced by the compliance current, and as the compliance current level increases, the filament branches will also increase.51,52 As a result, the number of Ag atoms participating in filament branches increases, leading to an increase in the variation of SET.
Chemical investigation of a volatile device
Fig. 3(a) shows a high angle annular dark field (HAADF) image of the hybrid diffusion memristor using the cross-sectional scanning transmission electron microscope (TEM) analysis. The Ag TE and Ta2O5/HfO2 bilayer thin films were stacked on the 70 nm-thick Pt BE within the metal–insulator–metal (MIM) structure. Fig. 4(b) exhibits the high resolution TEM image of Ag, Ta2O5, and HfO2 layers. The thickness of Ag, Ta2O5, and HfO2 was verified with 15 nm, 4.6 nm, and 7.6 nm, respectively, and the total thickness of the bilayer device was kept with the 12 nm thickness in order to compare electrical characteristics with the single layer Ta2O5 or HfO2 devices. Fig. 3(b) displays the vertical profile of Ag, Ta, Hf, O, and Pt with thicknesses attained by an energy dispersive X-ray analysis (EDX), which is drawn with the individual components profile in Fig. 3(b). These EDX profiles correspond well to the TEM analysis results.
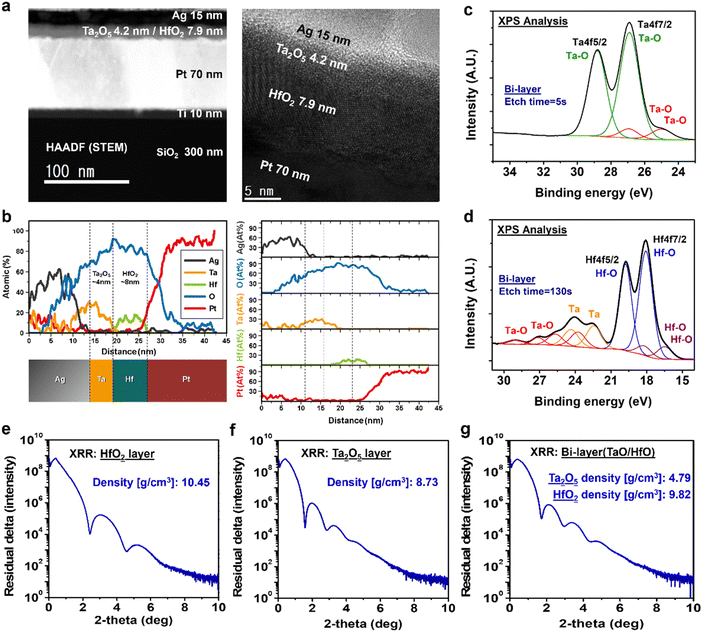 |
| Fig. 3 (a) The cross-sectional HAADF (STEM) image of the hybrid diffusion memristor and the high resolution of HRTEM. The thicknesses of Ag, Ta2O5, HfO2, and Pt layers were measured as 15, 4.6, 7.6, and 70 nm. (b) The EDX vertical line profile of all elements including thickness and the individual line profiles. XPS analysis of a bilayer with the Ag/Ta2O5/HfO2/Pt device (c) at the control layer and (d) the switching layer. XRR analysis of a single layer with (e) Ag/HfO2/Pt and (f) Ag/Ta2O5/Pt, and (g) bilayer with Ag/Ta2O5/HfO2/Pt devices. The thin film density was verified with figures. | |
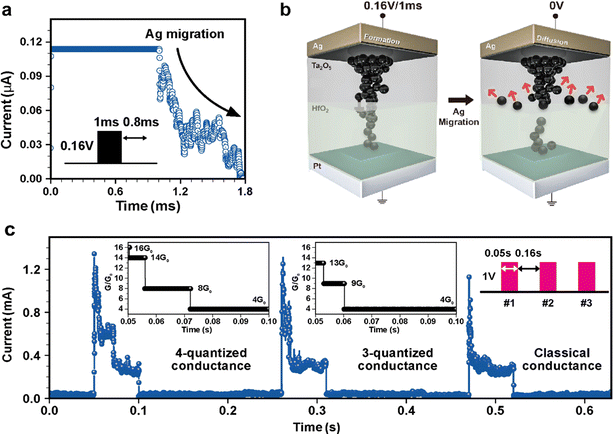 |
| Fig. 4 The Ag migration after a pulse of 0.16 V/1 ms. (a) The exponential decay was observed because the unstable Ag filament diffused at around 0.8 ms. (b) A schematic representation of before and after the Ag migration. The metal filament was formatted by the 0.16 V/1 ms pulse but metal ions diffused after the pulse. (c) The quantum conductance for the stimulation with the pulse, 1 V/0.05 s. The conductance was calculated by the equation, G0 = 2e2/h. During applying the first pulse, 4-quantized conductance was observed with 16, 14, 8, and 4G0. Secondly, the conductance was identified by 3-quantized steps with 13, 9, and 4G0 and finally decreases classically. | |
The bilayer device was analyzed by XPS as shown in Fig. 3(c) and (d). The partial oxidation was confirmed in the Ta2O5 layer in the bilayer, as shown in Fig. 1(c) and (d) of the single layer analysis results and was verified at the interface. The HfO2 thin film was analyzed as a fully oxidized layer in Fig. 1(c), but in the case of the bilayer device, the partial oxidation was confirmed by a combination of Ta–O, Ta, and Hf–O. Compared to the single layer, the bilayer with Ta2O5 and HfO2 allows for an additional supply of oxide at the interface of HfO2 and Ta2O5. As a result, the XPS results showed partial oxidation of Ta–O, Ta, and Hf–O at the interface of the bilayer at an etching time of 130 s. Atomic percentages were also determined using XPS, as shown in Fig. S4 (ESI†), which revealed a higher oxygen percentage near the interface of Ta2O5 and HfO2 at an etch time of 60 to 130 s, indicating the presence of an additional oxide supply. The partial oxidation at the interface could generate oxygen vacancies that cause self-diffusion of Ag in the thin filament. Therefore, the stable volatile characteristic independent of compliance current was observed. As in previous studies, Ag metal ion diffusion was enhanced by the oxygen vacancies.53,54 To identify the diffusion of Ag ions, EDX analysis was performed using line and dot analysis methods on the cross-sectional sample images obtained after the FIB process, as shown in Fig. S5 and S6 (ESI†). The highest Ag atomic percentage appeared in the single layer HfO2 device, which exhibited a bipolar property, and the lowest percentage was observed in the bilayer device, the diffusion memristor. However, the oxygen atomic percent exhibited the highest percentage in the bilayer. The Ag conductive filament was influenced by the oxygen vacancy, allowing the Ag atoms in the bilayer to diffuse more easily and form an unstable and thin filament, thereby demonstrating stable volatile characteristics.
Since the diffusion of metal ions is related to the density of the thin film, the density of the Ta2O5 and HfO2 single layer and bilayer was analyzed by XRR analysis data, as shown in Fig. 3(e)–(g). The film density of the single layer, Ta2O5 and HfO2 film are 8.73 g cm−3 and 10.45 g cm−3 in Fig. 3(e) and (f), respectively, but the densities of the bilayer with Ta2O5 and HfO2 film are 4.79 g cm−3 and 9.82 g cm−3 in Fig. 3(g). The film density is related to the material chemical composition. It is reported that the film density decreases as the oxygen ratio decreases for the HfO2 and SiO2 thin film density and the chemical composition.55 The film density of the bilayer decreased especially in the Ta2O5 layer compared with single layer. This oxygen stoichiometry could impact oxygen vacancy, resulting in easier metal diffusion and volatile electrical properties. According to the XPS analysis results, a change of chemical composition may occur at the interface between the Ta2O5 and HfO2 layer.
Ag filament control with the engineered pulse
Various measurements were conducted to confirm the improvement of the electrical properties by the double layer device. The electrical pulse with a pulse width of 1 ms and the voltage of 0.16 V was applied and the Ag migration was observed, as shown in Fig. 4(a). The electrical response was observed after passing the voltage pulse during the pulse interval of 1 ms and the exponential decay, Ag migration was measured during 0.8 ms after finishing the pulse. Fig. 4(b) shows schematic representations of this Ag migration phenomenon: an applied electrical pulse caused the Ag filament formation (left, SET process), but when the pulse stimulation was decreased, the Ag ions diffused and the formed Ag filament was ruptured (right, diffusion process). The diffusion time of the Ag migration was around 0.8 ms after the stimulation and could be influenced by the pulse parameters, Ag concentration, device temperature, and so on as reported before.56,57 As the results in Fig. S6 (ESI†) show, the Ag atomic percentage was not identified at the interface between Ta2O5 and HfO2 in the bilayer, but the oxygen percentage was the highest. This suggests that diffusion might occur more easily at this interface, leading to breakage of the filament. It was observed that the current levels decay in a discrete manner during the pulse measurement, as described in Fig. 4(c), showing the conductance behavior of the device during time evolution. The inserted graph indicates the normalized conductance value and the number of conductance steps following the duration time. After the pulse stimulation with 0.05 s and 1 V, the conductance of the bilayer device was decayed quantum-mechanically in the initial state and finally became the classical conductance mode. Since the bilayer device with the control Ta2O5 layer blocks the drastic Ag migration, the formed Ag bridge is not sufficient to form stable and thick filaments, and the Ag atoms of the thinnest filament part are detached in a discrete manner.43 By applying the repetitive stimulation, the quantized conductance decreased from 4 steps to 3 steps, as shown in the inserted graph of G/G0 and finally decreased following classical physics. The conductance quantum, G0 = 2e2/h, represents the quantized unit of electrical conductance. The inserted graph was calculated with the measurement value using the quantum conductance equation that was reported in the previous report.58 The change in conductance exhibited quantum behavior in the initial step and eventually transitioned to classical behavior in the repetitive environment. The measured movement of Ag atoms confirms that the designed diffusion memristor can control Ag atomic filaments on an atomic scale, which is made possible by the bilayer structure designed to have a differential layer for Ag diffusivity. Using this atomic dynamic characteristics of a bilayer diffusion memristor could even mimic LIF properties, a biological function of spiking neurons.40 When the Nfire, 4th pulse was applied under 0.2 V/0.01 s, the current abruptly increased up to 0.1 mA as in Fig. S7(a) (ESI†) because the conductive filament suddenly formed. When the input pulse exceeded a threshold value, the neuron fired an output action potential, which is schematically represented in Fig. S7(b) (ESI†).
Synaptic plasticity of the diffusion memristor with the Ta2O5/HfO2 layer
Atkinson and Shiffrin reported a psychological model of the human memory, which had three steps: sensory memory, short-term memory, and long-term memory.11 Fig. S8 (ESI†) showed the short-term synaptic properties in the voltage pulse parameter of 2 V/0.05 s and a delay time of 0.06 s. The repeated strong stimulation with 2 V amplitude changed the sensory memory to short-term memory, which was trainable but easily forgetful. The strong stimuli made the synaptic current increase suddenly, however, the increased current level became irretentive and decayed. The short-term memory characteristics, which are governed by the concentrations of ionic species (Ca2+, Na+, Mg2+, and K+) in biological systems,18 are verified in the diffusion memristor, as shown in Fig. 5(a). The synaptic weight gradually decayed for the 0.05 V pulse, which was five different sets of pulse stimuli with intervals of 0.3, 0.7, 1.1, 1.5, and 1.9 μs, respectively, as shown in the schematic diagram. The black lines, exponential graphs were the fitted forgetting curve by calculating the obtaining measurement value. I(t) = I0 + A
exp(−t/τ), I(t) is the synaptic current of the bilayer diffusion memristor at time t. The I0, A, and τ are the initial synaptic current, constant value, and relaxation time, respectively. The stimulation of 0.05 V/9 μs pulse was not sufficient to maintain the thick Ag filament, leading to an exponentially decreased synaptic current. However, the synaptic current was observed to improve by repeating the stimulation for five times as 0.006, 0.015, 0.021, 0.026, and 0.03 μA, while that of the relaxation time was calculated to increase from 52 to 56 μs. The improved synaptic current for the stimulation mimics the memory retention of the human brain, post-tetanic potentiation (PTP), a common form of short-term synaptic plasticity, which is an increase in synaptic strength.28,58 The variation spike of the synaptic current was measured in real time, indicating the spike of Ag migration as the pulse duration was increased.
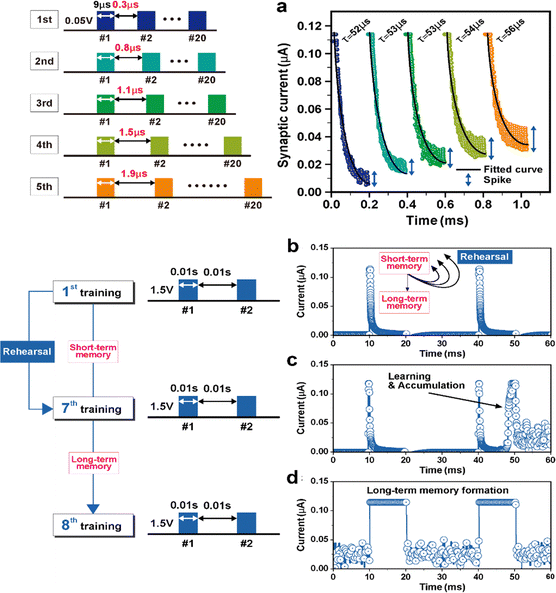 |
| Fig. 5 (a) The pulse diagram and forgetting curve of the diffusion memristor. The synaptic current grew during the fifth stimuli, which was similar to PTP characteristic as in biological brain. (b)–(d) The transition from short- to long-term memory through the rehearsal with the repetitive training pulse of a pair 1.5 V/0.01 s. (b) The initial state, (c) 7th, and (d) 8th training. | |
Finally, the bilayer synaptic device was trained and changed the long-term memory, as shown in Fig. 5(b)–(d). Takeo Ohno et al. showed that the rehearsal work with the strong input stimulation made the synaptic strength persist from short-term memory to long-term memory.28 Rehearsals were performed repeatedly using a pair of 1.5 V/0.01 s pulses with an interval time of 0.01 s. After repeated stress induced by a pair of pulses, it was observed that the bilayer synaptic device achieved the maximum synaptic current, 0.11 μA. Fig. 5(b)–(d) indicate the current behavior for initial state, 7th training state, and 8th training state, respectively. Ag migration was activated in accordance with repetitive training, especially after seven pulses, the current fluctuation of the learning and accumulation appears during the training pulse. As a result, the synaptic device transferred from short-term plasticity to long-term plasticity. The thin and unstable Ag filament due to the control layer could be changed to a thick and stable conductive filament by repetitive synaptic training. The transition of short- and long-term memory is one of the biological characteristics of the human brain, which could be influenced by the synaptic weight of stimulus frequency and stimulus strength.28
Conclusions
A volatile diffusion memristor was designed using a CMOS compatible high-k metal oxide, Ta2O5 and HfO2 layers, which could control the Ag ion diffusion effectively due to the difference of the oxidation ratio, emulating the synaptic characteristics with the Ag migration working as an ionic species similar to Ca2+ in the biological brain. The Ta2O5/HfO2 leads to a low operating voltage under 0.25 V and improved stability with any compliance current (10−6 A, 10−4 A, and without compliance current), showing multiple states. Due to the Ag migration being controllable at the interface of the bilayer device, the multi-state and synaptic properties were demonstrated such as the quantum conductance and short- and long-term plasticity, which can be affected by the applied pulse parameters of the pulse interval time, voltage value, and pulse history. Our device is compared to the previously reported TS operating based device using various metal oxides, as shown in Table 1.29,32,59–67 The double layer device shows an operating voltage of 0.2 V, Ion/off of 109, power consumption of 2 mW, 3 level states, and synaptic plasticity.
Table 1 A comparison of our work of the CBRAM with the metal oxide with the published results
|
Device structure |
Methods |
Type |
V
SET, VRESET (V) |
I
ON/OFF
|
Power consumption @ON state (mW) |
Multi-states |
Synaptic plasticity |
This work |
Ag/Ta2O5/HfO2/Pt |
Sputter/ALD |
Threshold |
+0.2 |
109 |
2 |
3 @LRS |
STP/LTP |
Ref. 26 |
Au/HfOx:Ag/Au |
Sputter |
Threshold |
+0.2 |
1010 |
0.2 |
— |
STD/STP |
Ref. 29 |
Cu/HfO2/p-Si |
ALD |
Bipolar |
+4, −2 |
105 |
40 |
5 |
LTD/LTP |
Ref. 55 |
Ag/SnOx/TiN |
Sputter |
Bipolar |
+1.5, −1 |
102 |
15 |
— |
STP/LTP |
Ref. 56 |
Cu/SiO2/Pt |
PECVD |
Bipolar |
+0.35, −0.05 |
107 |
3.5 |
— |
— |
Ref. 57 |
Au/TiO2/Au |
ALD |
Bipolar |
+2, −1 |
103 |
0.02 |
3 |
— |
Ref. 58 |
Ag/SiO2:Ag/TiO2/Si++ |
Sputter |
Bipolar |
+2, −3 |
103 |
20 |
5 |
LTD/LTP |
Ref. 59 |
Cu/Cu2−xS/WO3−x/W |
Oxidation |
Bipolar |
+1.5, −3 |
103 |
1.5 |
6 @HRS |
LTD/LTP |
Ref. 60 |
Ag/HfO2/Pt Ag/TiO2/Pt |
ALD |
Threshold |
+0.3 |
107 |
0.003 |
— |
— |
Ref. 61 |
Au/Ta2O5/Au |
Sputter |
Unipolar |
+1.7 |
105 |
0.017 |
— |
— |
Ref. 62 |
Cu/Al2O3/TiN |
ALD |
Bipolar |
+1.5, −2 |
105 |
0.015 |
4 @LRS |
— |
Ref. 63 |
Cu/SiO2/Si |
PECVD |
Bipolar |
+1, −2 |
105 |
0.01 |
— |
— |
Experimental section
Device fabrication
In order to investigate the migration of Ag ions in the diffusion memristor, we fabricated two single layered devices using the HfO2 and Ta2O5 thin film with thicknesses of 12 nm by ALD and RF sputter deposition, respectively, on the Pt/Ti/SiO2 substrate. The bilayer device with the stacking sequence of Ag/Ta2O5/HfO2/Pt was prepared, of which the total thickness in metal oxide was the same as a single layer device (Ta2O5 or HfO2 only). For the ALD HfO2 deposition, the deposition temperature was 300 °C, whereas the RF power used for the Ta2O5 deposition was 50 watt. For the patterning, the 100 × 100 μm2 device dimension of the TE Ag was deposited by DC magnetron sputtering after the UV light lithography. Finally, the lift-off process was performed for all three devices in order to obtain the final device structure.
Physical characterization
The Focused Ion Beam (FIB) system, Quanta model was used to prepare sample for TEM analysis of hybrid structured device. A milling voltage of 30 kV was used for the cutting of the device by means of Ga ions. The layer thickness and stoichiometric properties of the sample prepared by FIB were extracted by using JEOL 2100-F, TEM.
Electrical characterization
A Keithley 4200 semiconductor characterization analyzer was used for measurement for the DC and AC electrical characteristics of all devices. The voltage biasing was applied on the Ag TE while Pt BE was ground using 10 μm tips.
Author contributions
Y.-R. Jeon: fabrication of the device, data analysis, and writing & editing. D. Akinwande: writing review and editing. C. Choi: supervised this study and writing & editing.
Conflicts of interest
There are no conflicts to declare.
Acknowledgements
This research was supported by the National Research Foundation of Korea (NRF) funded by the Ministry of Science, ICT & Future Planning (NRF-2022R1A2C2012333, NRF-2022M3I7A2079155, and no. RS-2023-00260527) and D. A. acknowledges the Cockrell Family Regents Chair Endowment. This work was also supported by the Technology Innovation Program (00154752, Development of ultra-vacuum PVD equipment including EHU (Extreme High Unbalanced) Magnetron for PCRAM manufacturing of PIM semiconductor) funded by the Ministry of Trade, Industry & Energy (MOTIE, Korea).
References
- Z. Wang, M. Rao, R. Midya, S. Joshi, H. Jiang, P. Lin, W. Song, S. Asapu, Y. Zhuo, C. Li, H. Wu, Q. Xia and J. J. Yang, Adv. Funct. Mater., 2018, 28, 1704862 Search PubMed.
- D. Kuzum, S. Yu and H. S. Philip Wong, Nanotechnology, 2013, 24, 382001 CrossRef PubMed.
- S. Song, K. D. Miller and L. F. Abbott, Nat. Neurosci., 2000, 3, 919–926 CAS.
- W. Nicola and C. Clopath, Nat. Neurosci., 2019, 22, 1168–1181 CAS.
- W. He, K. Huang, N. Ning, K. Ramanathan, G. Li, Y. Jiang, J. Sze, L. Shi, R. Zhao and J. Pei, Sci. Rep., 2015, 4, 4755 CrossRef PubMed.
- Y. He, Y. Yang, S. Nie, R. Liu and Q. Wan, J. Mater. Chem. C, 2018, 6, 5336–5352 RSC.
- C. Tian, L. Wei and J. Jiang, Solid-State Electron., 2022, 194, 108386 CrossRef CAS.
- C.-H. Huang, Y. Zhang and K. Nomura, ACS Appl. Mater. Interfaces, 2022, 14, 22252–22262 CrossRef CAS PubMed.
- S.-Y. Kim, J. Yu, G. S. Lee, D. Yun, M.-S. Kim, J.-K. Kim, D.-J. Kim, G. Lee, M. Kim, J. Han, M. Seo and Y. Choi, ACS Appl. Mater. Interfaces, 2022, 14, 32261–32269 CrossRef CAS PubMed.
- R. Islam, H. Li, P.-Y. Chen, W. Wan, H.-Y. Chen, B. Gao, H. Wu, S. Yu, K. Saraswat and H.-S. Philip Wong, J. Phys. D: Appl. Phys., 2019, 52, 113001 CrossRef.
-
A. S. Lele, A. Naik, L. Bandhu, B. Das and U. Ganguly, in 2020 IEEE International Symposium on Circuits and Systems (ISCAS), IEEE, 2020, pp. 1–5.
- C. Gao, H. Wang, Z. Zhu, L. Zhang, Y. Yang, G. Cao and X. Yan, Phys. Status Solidi RRL, 2020, 14, 2000389 CrossRef CAS.
- A. Wedig, M. Luebben, D. Y. Cho, M. Moors, K. Skaja, V. Rana, T. Hasegawa, K. K. Adepalli, B. Yildiz, R. Waser and I. Valov, Nat. Nanotechnol., 2016, 11, 67–74 CrossRef CAS PubMed.
- N. Ilyas, D. Li, C. Li, X. Jiang, Y. Jiang and W. Li, Nanoscale Res. Lett., 2020, 15, 30 CrossRef CAS PubMed.
- Y. Abbas, Y.-R. Jeon, A. S. Sokolov, S. Kim, B. Ku and C. Choi, Sci. Rep., 2018, 8, 1228 CrossRef PubMed.
- D. Kim, J. H. Park, D. S. Jeon, T. D. Dongale and T. G. Kim, J. Alloys Compd., 2021, 854, 157261 CrossRef CAS.
- J. Woo, K. Moon, J. Song, S. Lee, M. Kwak, J. Park and H. Hwang, IEEE Electron Device Lett., 2016, 37, 994–997 CAS.
- A. K. Jena, M. C. Sahu, S. Sahoo, S. K. Mallik, G. K. Pradhan, J. Mohanty and S. Sahoo, Appl. Phys. A: Mater. Sci. Process., 2022, 128, 1–11 CrossRef.
- D. Kumar, S. Shrivastava, A. Saleem, A. Singh, H. Lee, Y.-H. Wang and T.-Y. Tseng, ACS Appl. Electron Mater., 2022, 4, 2180–2190 CrossRef CAS.
- S. Stathopoulos, A. Khiat, M. Trapatseli, S. Cortese, A. Serb, I. Valov and T. Prodromakis, Sci. Rep., 2017, 7, 17532 Search PubMed.
- J. H. Sung, J. H. Park, D. S. Jeon, D. Kim, M. J. Yu, A. C. Khot, T. D. Dongale and T. G. Kim, Mater. Des., 2021, 207, 109845 CrossRef CAS.
- E. A. Khera, C. Mahata, M. Imran, N. A. Niaz, F. Hussain, R. M. A. Khalil, U. Rasheed and N. SungjunKim, RSC Adv., 2022, 12, 11649–11656 RSC.
- M. Ismail, C. Mahata and S. Kim, Appl. Surf. Sci., 2022, 599, 153906 CrossRef CAS.
- B. Ku, Y. Abbas, A. S. Sokolov and C. Choi, J. Alloys Compd., 2018, 735, 1181–1188 CrossRef CAS.
- R. Yang, H. M. Huang, Q. H. Hong, X. B. Yin, Z. H. Tan, T. Shi, Y. X. Zhou, X. S. Miao, X. P. Wang, S. B. Mi, C. L. Jia and X. Guo, Adv. Funct. Mater., 2018, 28, 1–10 Search PubMed.
- S. Kim, C. Du, P. Sheridan, W. Ma, S. Choi and W. D. Lu, Nano Lett., 2015, 15, 2203–2211 CrossRef CAS PubMed.
- C. Du, W. Ma, T. Chang, P. Sheridan and W. D. Lu, Adv. Funct. Mater., 2015, 25, 4290–4299 CAS.
- T. Ohno, T. Hasegawa, T. Tsuruoka, K. Terabe, J. K. Gimzewski and M. Aono, Nat. Mater., 2011, 10, 591–595 CrossRef CAS PubMed.
- Z. Wang, S. Joshi, S. E. Savel’ev, H. Jiang, R. Midya, P. Lin, M. Hu, N. Ge, J. P. Strachan, Z. Li, Q. Wu, M. Barnell, G. L. Li, H. L. Xin, R. S. Williams, Q. Xia and J. J. Yang, Nat. Mater., 2017, 16, 101–108 CrossRef CAS PubMed.
- M. K. Kim and J. S. Lee, ACS Nano, 2018, 12, 1680–1687 CrossRef CAS.
- A. S. Sokolov, M. Ali, R. Riaz, Y. Abbas, M. J. Ko and C. Choi, Adv. Funct. Mater., 2019, 29, 1807504 CrossRef.
- J. Yoo, J. Park, J. Song, S. Lim and H. Hwang, Appl. Phys. Lett., 2017, 111, 063109 CrossRef.
- R. D. Nikam and H. Hwang, Adv. Funct. Mater., 2022, 32, 2201749 CrossRef CAS.
- H. Jiang, D. Belkin, S. E. Savel’Ev, S. Lin, Z. Wang, Y. Li, S. Joshi, R. Midya, C. Li, M. Rao, M. Barnell, Q. Wu, J. J. Yang and Q. Xias, Nat. Commun., 2017, 8, 882 CrossRef PubMed.
- J. Robertson, Eur. Phys. J.: Appl. Phys., 2004, 28, 265–291 CrossRef CAS.
- W. Banerjee, S. H. Kim, S. Lee, D. Lee and H. Hwang, Adv. Electron. Mater., 2021, 7, 2100022 CrossRef CAS.
- J. Li, Q. Duan, T. Zhang, M. Yin, X. Sun, Y. Cai, L. Li, Y. Yang and R. Huang, RSC Adv., 2017, 7, 43132–43140 RSC.
- Y. Abbas, R. B. Ambade, S. B. Ambade, T. H. Han and C. Choi, Nanoscale, 2019, 11, 13815–13823 RSC.
- Y. R. Jeon, Y. Abbas, A. S. Sokolov, S. Kim, B. Ku and C. Choi, ACS Appl. Mater. Interfaces, 2019, 11, 23329–23336 CrossRef CAS PubMed.
- H. Abbas, Y. Abbas, G. Hassan, A. S. Sokolov, Y. R. Jeon, B. Ku, C. J. Kang and C. Choi, Nanoscale, 2020, 12, 14120–14134 RSC.
- D. Sakellaropoulos, P. Bousoulas, C. Papakonstantinopoulos, S. Kitsios and D. Tsoukalas, IEEE Electron Device Lett., 2020, 41, 1013–1016 CAS.
- S. Lee, J. Yoo, J. Park and H. Hwang, IEEE Trans. Electron Devices, 2020, 67, 2878–2883 CAS.
- J. Van Den Hurk, E. Linn, H. Zhang, R. Waser and I. Valov, Nanotechnology, 2014, 25, 425202 CrossRef PubMed.
-
P. Dorion, O. Cueto, M. Reyboz, J. C. Barbe, A. Grigoriu and Y. Maday, in 2014 International Conference on Simulation of Semiconductor Processes and Devices (SISPAD), IEEE, 2014, pp. 33–36.
- A. Walsh, C. R. A. Catlow, A. G. H. Smith, A. A. Sokol and S. M. Woodley, Phys. Rev. B: Condens. Matter Mater. Phys., 2011, 83, 220301 CrossRef.
- W. Banerjee, S. H. Kim, S. Lee, S. Lee, D. Lee and H. Hwang, Adv. Funct. Mater., 2021, 26, 2104054 CrossRef.
- Y. Wang, Z. Zhang, H. Li and L. Shi, J. Electron. Mater., 2019, 48, 517–525 CrossRef CAS.
- Y. Sun, X. Zhao, C. Song, K. Xu, Y. Xi, J. Yin, Z. Wang, X. Zhou, X. Chen, G. Shi, H. Lv, Q. Liu, F. Zeng, X. Zhong, H. Wu, M. Liu and F. Pan, Adv. Funct. Mater., 2019, 29, 1808376 CrossRef.
- Y. Sun, C. Song, S. Yin, L. Qiao, Q. Wan, R. Wang, F. Zeng and F. Pan, Adv. Electron. Mater., 2020, 6, 2000695 CrossRef CAS.
- J. Akola, K. Konstantinou and R. O. Jones, J. Comput. Electron., 2010, 9, 146–152 CrossRef.
- F. G. Aga, J. Woo, J. Song, J. Park, S. Lim, C. Sung and H. Hwang, Nanotechnology, 2017, 28, 115707 CrossRef PubMed.
- S. Yu and H. S. P. Wong, IEEE Trans. Electron Devices, 2011, 58, 1352–1360 CAS.
- B. Attarimashalkoubeh, A. Prakash, S. Lee, J. Song, J. Woo, S. H. Misha, N. Tamanna and H. Hwang, ECS Solid State Lett., 2014, 3, 120–122 CrossRef.
-
P. Gonon, C. Vallee, C. Mannequin, M. Saadi and F. Jomni, Proceedings of the IEEE International Conference on Properties and Applications of Dielectric Materials, Institute of Electrical and Electronics Engineers Inc., 2015, vol. 2015, pp.56–59 Search PubMed.
- H. P. Ma, J. H. Yang, J. G. Yang, L. Y. Zhu, W. Huang, G. J. Yuan, J. J. Feng, T. C. Jen and H. L. Lu, Nanomaterials, 2019, 9, 55 CrossRef PubMed.
- F. Ye, F. Kiani, Y. Huang and Q. Xia, Adv. Mater., 2022, 29, 2204778 Search PubMed.
- I. Valov and W. D. Lu, Nanoscale, 2016, 8, 13828–13837 RSC.
- S. R. Nandakumar, M. Minvielle, S. Nagar, C. Dubourdieu and B. Rajendran, Nano Lett., 2016, 16, 1602–1608 CrossRef CAS PubMed.
- H. Cao and H. Ren, Appl. Phys. Lett., 2022, 120, 133502 CrossRef CAS.
- O. Kwon, J. Shin, D. Chung and S. Kim, Ceram. Int., 2022, 48, 30482–30489 CrossRef CAS.
- J. Yang, H. Ryu and S. Kim, Chaos, Solitons Fractals, 2021, 145, 110783 CrossRef.
- D. H. Kwon, K. M. Kim, J. H. Jang, J. M. Jeon, M. H. Lee, G. H. Kim, X. S. Li, G. S. Park, B. Lee, S. Han, M. Kim and C. S. Hwang, Nat. Nanotechnol., 2010, 5, 148–153 CrossRef CAS PubMed.
- D. Li, N. Ilyas, C. Li, X. Jiang, Y. Jiang and W. Li, J. Phys. D: Appl. Phys., 2020, 53, 175102 CrossRef CAS.
- S. Lim, M. Kwak and H. Hwang, IEEE Trans. Electron Devices, 2018, 65, 3976–3981 CAS.
- J. Y. Chen, C. W. Huang, C. H. Chiu, Y. T. Huang and W. W. Wu, Adv. Mater., 2015, 27, 5028–5033 CrossRef CAS PubMed.
- A. Belmonte, U. Celano, Z. Chen, J. Radhaskrishnan, A. Redolfi, S. Clima, O. Richard, H. Bender, G. S. Kar, W. Vandervorst and L. Goux, Nano Res., 2018, 11, 4017–4025 CrossRef CAS.
- A. Belmonte, U. Celano, R. Degraeve, A. Fantini, A. Redolfi, W. Vandervorst, M. Houssa, M. Jurczak and L. Goux, IEEE Electron Device Lett., 2015, 36, 775–777 CAS.
|
This journal is © The Royal Society of Chemistry 2024 |
Click here to see how this site uses Cookies. View our privacy policy here.