DOI:
10.1039/D3NR05130G
(Paper)
Nanoscale, 2024,
16, 4025-4038
Elucidating the reversible and irreversible self-assembly mechanisms of low-complexity aromatic-rich kinked peptides and steric zipper peptides†
Received
11th October 2023
, Accepted 29th January 2024
First published on 30th January 2024
Abstract
Many RNA-binding proteins such as fused-in sarcoma (FUS) can self-assemble into reversible liquid droplets and fibrils through the self-association of their low-complexity (LC) domains. Recent experiments have revealed that SYG-rich segments in the FUS LC domains play critical roles in the reversible self-assembly behaviors of FUS. These FUS LC segments alone can self-assemble into reversible kinked fibrils, which are markedly different from the canonical irreversible steric zipper β-sheet fibrils. However, the molecular determinants underlying the reversible and irreversible self-assembly are poorly understood. Herein we conducted extensive all-atom and coarse-grained molecular dynamics simulations of four representative hexapeptides: two low-complexity aromatic-rich kinked peptides from the amyotrophic lateral sclerosis-related FUS protein, FUS37–42 (SYSGYS) and FUS54–59 (SYSSYG); and two steric zipper peptides from Alzheimer's-associated Aβ and Tau proteins, Aβ16–21 (KLVFFA) and Tau306–311 (VQIVYK). We dissected their reversible and irreversible self-assembly dynamics, predicted their phase separation behaviors, and elucidated the underpinning molecular interactions. Our simulations showed that alternating stickers (Tyr) and spacers (Gly and Ser) in FUS37–42 and FUS54–59 facilitate the formation of highly dynamic coil-rich oligomers and lead to reversible self-assembly, while consecutive hydrophobic residues of LVFF in Aβ16–21 and IVY in Tau306–311 act as hydrophobic patches, favoring the formation of stable β-sheet-rich oligomers and driving the irreversible self-assembly. Intriguingly, we found that FUS37–42 and FUS54–59 peptides, possessing the same amino acid composition and the same number of sticker and spacer residues, display differential self-assembly propensities. This finding suggests that the self-assembly behaviors of FUS peptides are fine-tuned by the site-specific patterning of spacer residues (Ser and Gly). This study provides significant mechanistic insights into reversible and irreversible peptide self-assembly, which would be helpful for understanding the molecular mechanisms underlying the formation of biological liquid condensates and pathological solid amyloid fibrils.
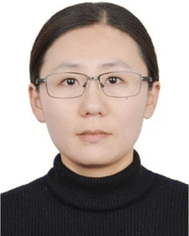 Cong Guo | Cong Guo is an associate professor in the Department of Physics, College of Sciences at Shanghai University, China. She obtained her PhD from Fudan University under the supervision of Prof. Guanghong Wei in 2014 and worked as a postdoctoral fellow under the supervision of Prof. Huan-xiang Zhou at Florida State University and then Prof. Anant Paravastu at Georgia Institute of Technology before joining Shanghai University in December 2017. Her research focuses on understanding the molecular mechanism of the assembly of peptides into ordered nanostructures or amyloid fibrils and the regulation of pathological fibrillization by endogenous proteins with molecular dynamics simulations. |
Introduction
Fibrillary deposits are the hallmark of many neurodegenerative disorders,1,2 such as Alzheimer's disease associated with the fibrillary aggregates of Aβ and Tau.3–5 Amyloid fibrils (also known as steric zipper β-sheet fibrils) share cross-β architecture, with extended β-strands stacking along the fibril axis into a β-sheet and the side chains of mating sheets interdigitating with each other.6–8 Attributed to their highly ordered inter-molecular backbone hydrogen bonds (H-bonds) and complementary dry zipper interface, steric zipper β-sheet fibrils are stable and present strong resistance to dissociation under harsh conditions, such as being in the presence of chemical denaturants9 or at an elevated temperature.10
In addition to the highly stable cross-β fibrils (irreversible fibrils), in recent years, labile kinked β-sheet fibrils with thermal-responsive reversibility (reversible fibrils) have been reported to be formed by many RNA binding proteins, such as fused-in sarcoma (FUS),11–13 TAR DNA-binding protein 43 (TDP-43)14,15 and heterogeneous nuclear ribonucleoprotein A1 (hnRNPA1)13,16 and A2 (hnRNPA2).17 These proteins are capable of undergoing liquid–liquid phase separation (LLPS) to form liquid biomolecular condensates.18,19 On the one hand, LLPS is identified as the formation mechanism of the membrane-less organelles (MLOs, composed of proteins and RNAs)20–25 and is involved in many biological cellular functions.26–28 On the other hand, LLPS plays a critical role in the formation of toxic aggregates in diseases such as amyotrophic lateral sclerosis (ALS)29–33 and frontotemporal lobar degeneration (FTLD).34–37 Under abnormal conditions, such as mutations or continuous external stimuli, the droplets formed via LLPS can turn into solid fibrillary aggregates, a process which is called liquid-to-solid phase transition (LSPT).30,37 The amyloid fibrils are a common end-stage product of LSPT.7 Recently, the structure of the reversible fibril formed by the fibril core of the FUS low complexity (FUS LC) domain has been determined by solid state nuclear magnetic resonance (ssNMR).11 This fibril core consists of residues 35–95 of FUS (FUS35–95), a 61-residue segment. The FUS35–95 fibril, unlike the steric zipper β-sheet fibril, is labile for the lack of intermolecular side chain hydrophobic interactions (or tight interdigitation).38 Apart from through maturation or aging (the time-dependent coarsening and loss of dynamicity) of a liquid-like droplet by RNA binding proteins such as FUS, pathogenic fibrils can also be formed by proteins such as Aβ directly through irreversible aggregation without LLPS, which is termed liquid–solid phase separation (LSPS).39
In addition to full-length proteins and their LC domains, short peptides are able to phase separate, forming liquid condensates40–43 and reversible or irreversible fibrils.12,13,15 Recent crystal structure studies of short peptides have provided different structural properties of the two types of fibrils.39 Unlike the irreversible fibrils, the structures of reversible fibrils are organized in kinked strands perpendicular to the fibril spine, or extended β-strands with a hydrous sheet interface, or a stacking pattern with Asp residues from individual β-strands aligned along the fibril spine.7,12,13,16 For example, fibrils of FUS37–42 (PDB ID: 5XSG) comprise kinked strands without extended β sheets (Fig. S1A†), and fibrils of FUS54–59 (PDB ID: 5XRR) consist of pairs of β-sheets with a hydrophilic interface (Fig. S1B†).12 In contrast, the irreversible fibril structures of Aβ16–21 (PDB ID: 3OW9)44 and Tau306–311 (PDB ID: 2ON9)45 (Fig. S1C ad S1D†) are composed of stacking β-strands and have large hydrophobic interfaces between β-sheets and surface complementarity. Eisenberg's group also solved the fibril structures of several segments from FUS, hnRNPA1 and nup98 proteins,13 named LARKS (low-complexity aromatic-rich kinked segment). LARKS forms reversible hydrogel, rather than irreversible fibrils.15
Reversible fibrils are easily dissociated by heating or adding detergent.12,13,16 In the thermostability experiments performed by Liu's group,12 it was found that fibrils formed from two tandem (S/G)Y(S/G) motifs of FUS, namely 37SYSGYS42 (FUS37–42) and 54SYSSYG59 (FUS54–59) at 4 °C dissolved upon heating to room temperature or higher. When cooled down back to 4 °C, both FUS37–42 and FUS54–59 fibrils were formed again.12 In contrast, the amyloid fibrils (termed irreversible fibrils) of Aβ16–21 (Ace-16KLVFFA21) and Tau306–311 (Ace-306VQIVYK311) remained intact during the heating and cooling processes, displaying high thermostability.12
In spite of the structural characteristics and thermal stabilities of reversible and irreversible fibrils being well studied experimentally, the dynamic properties and phase behaviors of those reversible/irreversible self-assembling peptides as well as their underlying molecular mechanisms remain largely elusive. In this study, we investigated the reversible/irreversible self-assembly process and predicted the LLPS/LSPS of four hexapeptides, namely FUS37–42, FUS54–59, Aβ16–21 and Tau306–311, using both all-atom molecular dynamic (AA-MD) and coarse-grained MD (CG-MD) simulations combined with explicit water solvents. We dissected the main differences in the dynamic and structural properties of reversible and irreversible peptide self-assembly, characterized the phase behaviors, and elucidated the underlying molecular interactions. Our computational work provides atomic insights into the reversible and irreversible self-assembly of short fibril-forming peptides, which may be helpful for the in-depth mechanistic understanding of LLPS and LSPS of proteins.
Models and methods
System setup
We selected four representative short peptides as our study models, two from the ALS-related FUS protein, FUS37–42 (37SYSGYS42) and FUS54–59 (54SYSSYG59),12 and another two from the Alzheimer's-associated Aβ/Tau proteins, Aβ16–21 (Ace-16KLVFFA21)44 and Tau306–311 (Ace-306VQIVYK311).45 We simulated a total of 12 systems for the four peptides: four all-atom fibril systems, four all-atom oligomer systems, and four coarse-grained oligomer systems. All the systems together with the simulation setups are provided in Table 1.
Table 1 A summary of all the systems and simulation setups
Systemsa |
Number of chains |
Number of atoms/beads |
Temperature |
Simulation time |
FUS37–42: 37SYSGYS42; FUS54–59: 54SYSSYG59; Aβ16–21: Ace-16KLVFFA21; Tau306–311: Ace-306VQIVYK311. |
All-atom |
Fibrils |
FUS37–42 |
12 |
33 575 |
310 K |
500 ns × 2 |
FUS54–59 |
33 584 |
Aβ16–21 |
33 653 |
Tau306–311 |
33 620 |
Oligomers |
FUS37–42 |
6 |
60 134 |
310 K |
310 K: 500 ns × 5 |
FUS54–59 |
60 137 |
Aβ16–21 |
60 161 |
340 K |
340 K: 500 ns × 1 |
Tau306–311 |
60 203 |
|
Coarse-grained |
Condensates |
FUS37–42 |
200 |
46 854 |
310 K |
6 μs × 3 |
FUS54–59 |
46 854 |
Aβ16–21 |
47 054 |
Tau306–311 |
46 854 |
All-atom fibril systems.
The initial fibril structures of the four hexapeptides used in our study consisted of 12 peptide chains (Fig. S1†). They were taken from the fibril structures of FUS37–42 (PDB ID: 5XSG) and FUS54–59 (PDB ID: 5XRR) solved by Luo et al.,12 and Aβ16–21 (PDB ID: 3OW9) and Tau306–311 (PDB ID: 2ON9) fibril structures determined by the Eisenberg group.44,45 Aβ16–21 and Tau306–311 fibrils were acetylated at the N-terminal to keep peptides electrostatically neutral, consistent with capped peptides used for the thermostability measurements by Liu's group.12 These peptide fibrils were placed in the center of a box with a side length of ∼7.0 nm.
All-atom oligomer systems.
The initial state of each oligomer system consisted of six monomers with random coil conformations, with each monomer randomly placed in the simulation box with dimensions of ∼8.5 × 8.5 × 8.5 nm3. Peptide monomers were first constructed using PyMOL46 in extended coil states, then simulated under 410 K for 50 ns.
Coarse-grained oligomer systems.
In the initial state of each system, 200 monomers were randomly displaced in an aqueous simulation box containing about 43
000 water beads and 527 Na+ and 527 Cl− ions (∼0.15 M NaCl). The simulation box has a size of ∼18 × 18 × 18 nm3.
AA-MD simulations
AA-MD simulations were performed using the GROMACS-2016.4 software package47 in combination with the AMBER99SB-ILDN force field48 and TIP3P water model. Na+ and Cl− ions at a physiological concentration of 0.15 M were added to all the simulation boxes. We used the Verlet cutoff scheme for neighbor searching and the particle mesh Ewald (PME) method49,50 for electrostatic interaction calculations. A real-space cutoff of 1.4 nm was used for electrostatic interaction. The cutoff for van der Waals interaction was also set as 1.4 nm. Periodic boundary conditions were applied for all simulations. Before the production run of the MD simulations, all the systems were sequentially equilibrated under an NVT (310 K) ensemble for 100 ps, and then under an NPT (310 K and 1 bar) ensemble for 100 ps.51 After that, each simulation was run for 500 ns, with an integration time step of 2 fs. Velocity rescale52 and the Parrinello-Rahman53 methods were used for temperature and pressure coupling, respectively. To obtain statistically significant results, two independent simulations at 310 K were conducted for each fibril system, and five independent simulations at 310 K were performed for each of the oligomer systems. To examine the fluidity of the peptides at a higher temperature, another 500 ns simulation was conducted for each of the four oligomer systems at 340 K, starting from the final state of the MD simulation at 310 K.
CG-MD simulation
All CG-MD simulations were performed using the GROMACS-2018.3 software package. The peptides were described using the MARTINI coarse-grained force field (version 2.2)54,55 with modifications described as follows. Electrostatic interactions were calculated using the reaction field method56 with a real-space cut-off of 1.4 nm in the CG-MD simulations. The other simulation setups were the same as those used in the AA-MD simulations. Three independent 6 μs simulations were conducted for each system to ensure that each of them reached equilibrium. The previous literature has reported the overestimation of inter-residue interaction strength using the MARTINI forcefield,57–59 especially for Ser and Thr residues. Several groups have utilized a scaling factor α to adjust the pair-interaction well depth, which is aimed at reproducing experimental observations.57,58 As FUS37–42 and FUS54–59 peptides are enriched in Ser residues, rescaling the pair interaction is crucial for accurately characterizing their assembly capabilities. However, the selection of the α value is system-dependent, and experimental data for the condensates formed by these two FUS segments are not available. In a recent work, the Hummer group has shown that FUS-LCD condensation occurs above a critical α value of 0.6, and the experimental densities of both the dilute and dense phases were accurately replicated at α = 0.65.58 In spite of a much shorter sequence length, FUS37–42 and FUS54–59 have been identified as two reversible amyloid cores, which mediate the dynamic assembly of FUS LCD.12 We thus infer that α = 0.65 may also be suitable for studying the self-assembly process of FUS37–42 and FUS54–59. To test this hypothesis, we selected six α values (0.2, 0.4, 0.5, 0.6, 0.65, and 0.8) and performed short (600 ns) simulations to compare their performance in characterizing the assembly capability of the peptides. The scaling was performed following the method of Stark et al.55 by down-scaling the van der Waals parameters between Ser pseudo-atoms and the backbone beads of all residues, εα = ε0 + α(εoriginal − ε0). A value of α = 0 corresponds to a repulsion-dominated interaction in the MARTINI model (ε0 = 2 kJ mol−1), and a value of α = 1 recovers the full interaction in the MARTINI force field (ε1 = εoriginal). The results suggest that FUS37–42 and FUS54–59 presented increasing assembly capability with increasing scaling factor values (Fig. S2†). They displayed weak assembly capabilities at low α values (<0.6), especially FUS37–42, while they presented higher assembly ability than Aβ16–21 and Tau306–311 at a high α value (0.8). At a moderate α value of 0.65, FUS37–42 and FUS54–59 possessed a certain degree of assembly capability, which was lower than that of Aβ16–21 and Tau306–311 in good agreement with previous experimental observations.12 We thus selected 0.65 as the scaling factor for performing the coarse-grained simulations. To offer a quantitative comparison with all-atom simulations, we conducted coarse-grained simulations for each of the four peptides starting from six randomly dispersed peptide chains. At a scaling factor of α = 0.65, the collapse degree and SASA fluctuation values predicted by coarse-grained simulations were of the same order of magnitude as those predicted by the all-atom simulations (Fig. S3†). Moreover, both simulations predicted that FUS37–42/FUS54–59 possessed lower assembly capability and higher liquidity than Aβ16–21/Tau306–311.
Analysis methods
Data analysis was performed using the tools implemented in the GROMACS software package and our in-house developed codes. For data analysis of the AA-MD simulations, an atomic contact was defined as being when two aliphatic carbon atoms were within 0.54 nm of one another or when any other two heavy atoms came within 0.46 nm.60–62 When two monomers were located with a minimum distance between heavy atoms of less than 0.54 nm, they were regarded as belonging to one cluster. The intra- and inter-chain dihedral correlations were evaluated following previous literature.63 The correlation coefficients between two dihedrals (x and y) were calculated utilizing the method put forward by Jammalamadaka and Sengupta,64 using the following equation:
A hydrogen bond (H-bond) was considered to be formed on the basis of two conditions:65–67 (1) the distance between the H-bond donor (D) and the acceptor (A) is less than 0.35 nm, and (2) the angle of D–H⋯A is larger than 150°. The secondary structure was analyzed using the DSSP tool.68 The end-to-end distance of a monomer refers to the distance between the first and the last Cα atoms of the monomer. Two aromatic residues were in the π–π stacking state when the distance between the centroids of two aromatic rings was less than 0.7 nm.69 Three different types of stacking patterns were defined according to the angles of the two aromatic rings: 0°–30° for parallel, 30°–60° for herringbone, and 60°–90° for T-shape. The statistical results of the AA-MD simulations were all based on the data from 300 to 500 ns.
In the data analysis of CG-MD simulations, two monomers were considered to have molecular contact if their minimum distance was less than 0.6 nm. In accordance with our recent study, the aggregation propensity of each peptide was characterized using the collapse degree and the clustering degree.43 The collapse degree was defined as the ratio of SASA of all hexapeptide molecules in the initial state to their SASA in the final configuration.70 The clustering degree was defined by molecules in clusters divided by the total number of molecules in the system.43 The fluidity of an aggregate was characterized using the fluctuation of SASA, fluctuation of the clustering degree and the exchange rate of the interactions.43 The statistical results of the CG-MD simulations were all based on data from 3 to 6 μs. All the structure representations were drawn using the VMD program71 or PyMOL.46
Results and discussion
Fibrils formed by the four hexapeptides display different thermoresponsive stabilities
We first examined the structural stability of the preformed fibrils of the FUS37–42, FUS54–59, Aβ16–21 and Tau306–311 peptides. Two independent 500 ns simulations were carried out at 310 K for each fibril system and the results are shown in Fig. 1. It is noted that the structures of the FUS37–42/FUS54–59 fibril and the Aβ16–21/Tau306–311 fibril were solved at 4 °C and 18 °C,12,44,45 which were lower than our simulation temperature (310 K). As seen in Fig. 1a and b, the Cα-root-mean-square-deviation (Cα-RMSD) and the radius of gyration (Rg) values of both FUS37–42 and FUS54–59 far exceeded those of Aβ16–21 and Tau306–311 and fluctuated considerably, indicating that the fibril structures of FUS37–42 and FUS54–59 were much less stable than those of the Aβ16–21 and Tau306–311 fibril structures. The fibril structures of FUS37–42 and FUS54–59 were completely disrupted in the final state. In contrast, both the Cα-RMSD and Rg curves of Aβ16–21 and Tau306–311 maintained a plateau at small values (Fig. 1a and b), although the fibril structures of Aβ16–21 and Tau306–311 were twisted and the β-sheet contents decreased a bit (Fig. 1d). Then, the side chain dynamics of the four systems were analyzed by calculating the rate of side chain dihedral angle (χ1) transition between the Cγ–exo and Cγ–endo conformations.72 It can be seen from Fig. S4† that FUS37–42 and FUS54–59 had much higher χ1 transition rates (>2.0) than Aβ16–21 and Tau306–311 (<1.2), suggesting a much higher degree of side chain dynamics of the LARKS peptides. This high degree of dynamics likely played a role in the low stability of the FUS37–42 and FUS54–59 fibrils. The previous literature has revealed that correlated backbone motions are one of the fundamental properties of the β-sheet.63 To investigate the backbone correlation of the four fibrils, we calculated the circular cross-correlation coefficients of main chain dihedrals within and between the fibril chains (Fig. 1c). The definitions of the dihedral angles for parallel and antiparallel β-strands are given in Fig. S5.† Strong local anticorrelations are observed between the φ and ψ values of each peptide chain in all of the four systems. This anticorrelation is consistent with the previous literature and indicates the rigidity of the peptide plane, which couples the motion of the dihedral angles while preserving the structure of the strands. Strong inter-chain correlations are also observed between the adjacent chains in the Aβ16–21 and Tau306–311 systems, indicating the stability of their fibrils. Interestingly, in the Aβ16–21 system φ–φ and ψ–ψ dihedral pairs are correlated while the φ–ψ pair is anticorrelated, and vice versa in the Tau306–311 system. This is due to the antiparallel and parallel nature of the Aβ16–21 and Tau306–311 β-sheets. In contrast, the inter-chain correlations of the FUS37–42 and FUS54–59 systems are much weaker, further demonstrating the instability of their fibrils. The computationally observed lower stability of the LARK segments FUS37–42/FUS37–42 than that of the Aβ16–21/Tau306–311 peptides is consistent with previous experimental observations,12 demonstrating the accuracy and suitability of the force field and the methodology that we used.
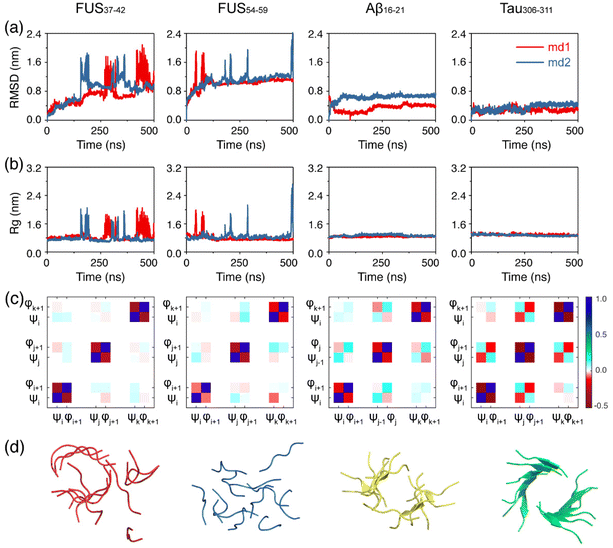 |
| Fig. 1 Thermostability of the preformed FUS37–42, FUS54–59, Aβ16–21 and Tau306–311 fibrils. Time evolution of (a) RMSD and (b) Rg values of the FUS37–42, FUS54–59, Aβ16–21 and Tau306–311 fibrils. (c) The dihedral angle correlation coefficients within and between the peptide chains in the four fibril systems. (d) Snapshots of the final states of the four fibril systems. Peptides were drawn using PyMOL. | |
The FUS37–42/FUS54–59 peptides form highly dynamic oligomers, whereas the Aβ16–21/Tau306–311 peptides assemble into stable oligomers
After the structural stability examination of the fibril structures, we investigated the self-assembly processes of the four hexapeptides using AA-MD simulations. It is shown in Fig. 2a that the numbers of clusters of FUS37–42 and FUS54–59 vary during the oligomerization process. The monomers of FUS37–42 and FUS54–59 gathered together first, then were separated, and came together again, indicating that their self-assembly processes are highly dynamic, and their aggregates are labile and easy to dissolve. In the Aβ16–21 and Tau306–311 systems, in marked contrast, most monomers self-assembled into stable aggregates, reflecting the irreversibility of their self-assembly processes. Additionally, side chain dihedral transition rates were also examined. Much higher transition rates were observed for FUS37–42 and FUS54–59 than for the Aβ16–21 and Tau306–311 oligomers, indicative of the higher side chain dynamics of the LARKS peptides (Fig. S4†). The different self-assembly dynamics were also demonstrated in the representative states of the four systems shown in Fig. 2b–e.
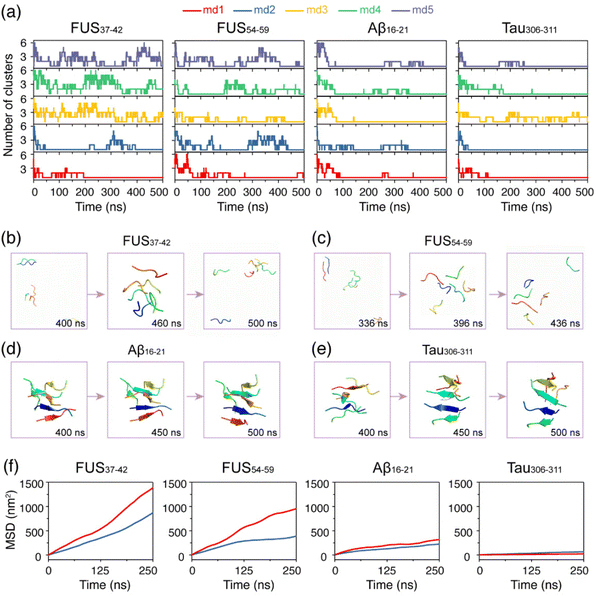 |
| Fig. 2 The self-assembly properties of the FUS37–42, FUS54–59, Aβ16–21 and Tau306–311 hexapeptides into oligomers. (a) Time evolution of the number of clusters formed by each peptide at 310 K. (b–e) Snapshots of the representative states in one out of the five simulations for each system. Peptides were drawn in cartoon form using PyMOL with different chains in different colors. (f) Mean square displacement (MSD) of the four systems at 310 and 340 K. The MD simulations at 340 K started from the final states obtained at 310 K. | |
To further explore the thermostability of the oligomers formed at 310 K, we performed a 500 ns MD simulation for each system at a higher temperature of 340 K starting from the final state obtained at 310 K. Fig. 2f shows that the slope of the mean square displacement (MSD) curves of FUS37–42 and FUS54–59 are both very large at 340 K and there is a big gap between the curves at 310 K and those at 340 K, suggesting strong temperature dependence of their fluidity. In sharp contrast, the MSD values of Aβ16–21 and Tau306–311 are quite similar at both temperatures, suggesting poor temperature dependence of their fluidity. Moreover, Aβ16–21 and Tau306–311 have much smaller MSD values than FUS37–42 and FUS54–59, indicating their weaker mobility at both temperatures. Overall, the LARK segments FUS37–42 and FUS54–59 self-assemble into dynamic aggregates with high mobility and low thermal stability, while Aβ16–21 and Tau306–311 self-assemble into more stable aggregates.
Peptide chains in the FUS37–42 and FUS54–59 oligomers tend to be kinked with low β-sheet contents, while those in the Aβ16–21 and Tau306–311 oligomers are extended with high β-sheet contents
We further analyzed the structural features of the oligomers formed by each of the four peptides. Fig. 3a and b shows the probability density function (PDF) of the end-to-end distance of all the peptide chains in each system. It can be seen that the end-to-end distance distribution curves of the FUS37–42 and FUS54–59 peptides have two wide peaks, located at around 0.5 nm and 1.4 nm (Fig. 3a), respectively, corresponding to the collapsed and extended single-chain conformations (illustrated by the representative snapshots in the dashed boxes). In contrast, the curves of the Aβ16–21 and Tau306–311 peptides have only one peak at around 1.6 nm (Fig. 3b). The results suggest that the peptide chains in the FUS37–42/FUS54–59 oligomers adopted both extended and collapsed conformations, whereas those in the Aβ16–21/Tau306–311 oligomers had mostly extended conformations. Moreover, the FUS37–42/FUS54–59 chains (with an end-to-end distance of 1.4 nm) were less extended than the Aβ16–21/Tau306–311 chains (1.6 nm), indicative of the kinked conformations of FUS37–42/FUS54–59 peptides. Collapsed peptides were likely to form more intra-molecular interactions, while extended peptides tended to form more inter-molecular interactions. Therefore, the peak at 0.5/1.4 nm of the distance distribution curves of the FUS37–42 and FUS54–59 systems signified that the collapsed/kinked peptides were less likely to form stable inter-molecular interactions, which may disfavor the formation of stable cross-β structures.
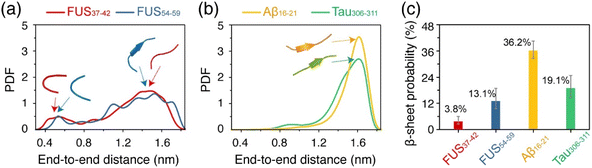 |
| Fig. 3 The structural properties of the oligomers formed by each of the four different peptides. Probability density function (PDF) of the distance between the first and the last Cα atoms of all single chains in (a) the FUS37–42 and FUS54–59 systems and (b) the Aβ16–21 and Tau306–311 systems. Arrows in (a) and (b) show the extended/kinked and extended single chain conformations. (c) β-sheet probability of the four systems. Data are from 300 to 500 ns. The error bars were calculated using a bootstrap procedure of 50 resamplings, with a confidence interval of 10%. | |
The β-sheet probability of the peptides in the four systems was calculated and is shown in Fig. 3c. The four systems with β-sheet contents from high to low are: Aβ16–21 (36.2%), Tau306–311 (19.1%), FUS54–59 (13.1%) and FUS37–42 (3.8%). Intriguingly, the FUS37–42 (SYSGYS) oligomers had much lower β-sheet content than the FUS54–59 (SYSSYG) oligomers, although the two peptides had the same amino acid composition (see below for a more detailed discussion). These results demonstrate that both Aβ16–21 and Tau306–311 peptides had a higher propensity to form β-sheet structures than the FUS37–42 and FUS54–59 peptides. Considering that β-sheets form the spines of stable amyloid fibrils, the different β-sheet preferences of the FUS37–42/FUS54–59 and Aβ16–21/Tau306–311 peptides may lead to their reversible and irreversible self-assembly.
The alternating stickers and spacers in the FUS37–42/FUS54–59 peptides result in the formation of reversible aggregates, while hydrophobic patches in the Aβ16–21/Tau306–311 peptides are responsible for their irreversible self-assembly
After examining the reversible and irreversible self-assembly properties of the two types of peptides, we further explored the underlying driving forces by calculating the number of intermolecular contacts and H-bonds (Fig. 4). There were similar numbers of contacts between the main chains (MC–MC) among the four systems, while the contacts between the side chains (SC–SC) were considerably different, i.e. much higher in the Aβ16–21 and Tau306–311 systems than in the FUS37–42 and FUS54–59 systems (Fig. 4a). These results indicate that the SC–SC contacts in the four systems were the main interactions driving the reversible and irreversible self-assembly of the FUS37–42/FUS54–59 and Aβ16–21/Tau306–311 peptides. In addition, more MC–MC H-bonds were formed in the FUS54–59, Aβ16–21 and Tau306–311 systems than in the FUS37–42 system (Fig. 4b), consistent with the results of the formation of more β-sheets in the former three systems. Fig. 4b also shows that the SC–SC H-bonds only accounted for a minor part of the forces driving the peptide self-assembly in all the systems. Moreover, the numbers of H-bonds formed were different in the FUS37–42 and FUS54–59 oligomers. Although having the same amino acid compositions and containing the tandem SYS and SYG/GYS motifs, FUS54–59 (SYSSYG) with Ser in the middle of the sequence had a greater ability to form intermolecular H-bonds than FUS37–42 (SYSGYS) with Gly in the middle of the sequence, indicating that the Ser residue at middle positions had more significant influences on peptide self-assembly than the Gly residue and that the site-specific patterning of the spacer residues (Ser and Gly) can subtly tune the self-assembly behavior.
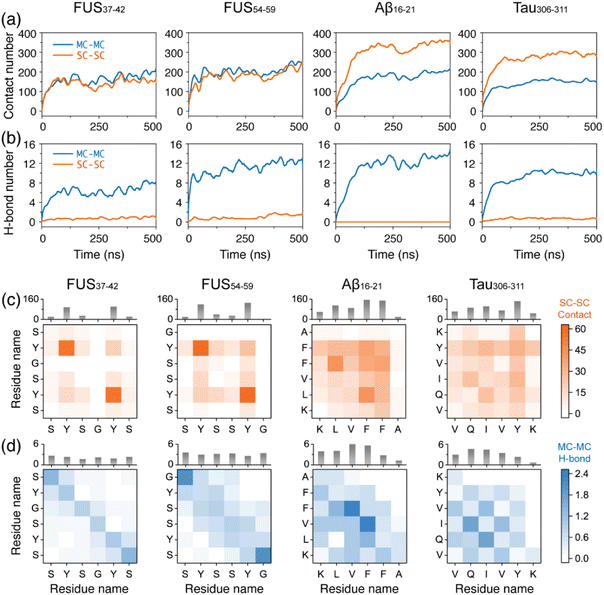 |
| Fig. 4 The driving forces underlying the reversible self-assembly of the FUS37–42/FUS54–59 peptides and irreversible self-assembly of the Aβ16–21 and Tau306–311 peptides. Time evolution of (a) main chain–main chain (MC–MC) and side chain–side chain (SC–SC) contacts, as well as (b) MC–MC and SC–SC H-bonds in the FUS37–42, FUS54–59, Aβ16–21 and Tau306–311 systems. Inter-molecular residue-wise (c) SC–SC contacts and (d) MC–MC H-bonds of the four systems. The grey bar charts show the cumulative contact numbers between each residue and other residues. | |
We note that there is an increase in the average contact number for the Aβ16–21 systems starting from ∼300 ns. To examine whether the contact number further rises with the increase of simulation time, we extended all five Aβ16–21 oligomer simulations for an additional 150 ns and calculated the time evolution of their main chain and side chain contact numbers (Fig. S6†). For some simulations, the contact numbers remained mostly constant after 500 ns (such as the main chain contact for MD3 and the side chain contact for MD5). For most simulations, the contact numbers fluctuated around their equilibrium values. Importantly, trends of continued growth were not observed after 500 ns, suggesting that the 500 ns duration was sufficient to reach a convergence for these contact numbers.
The intermolecular MC–MC and SC–SC residue-wise contacts and H-bonds were also analyzed (Fig. 4c, d and S7, S8†). As seen from Fig. 4c, only Tyr residues, separated by Ser and Gly, had strong interactions with each other in the FUS37–42 and FUS54–59 systems, suggesting that Tyr residues are crucial stickers of FUS37–42 and FUS54–59. The important role of Tyr and repeated motifs containing Tyr in contributing to protein LLPS has been reported in many studies.73–75 The amphipathic side chain of Tyr allowed it to form a hydrogen bond via its phenol group, as well as π–π interaction through the aromatic ring, the latter of which is important for the assembly of various amyloid peptides into β-rich fibrils,76,77 and has emerged as a major driver of LLPS.78–80 The intermolecular π–π stacking interactions were calculated between aromatic rings in the four systems. It is shown in Fig. S9† that more π–π stacking interactions were formed in the FUS37–42 and FUS54–59 systems than in the Aβ16–21 and Tau306–311 systems, although the number of aromatic rings was the same in FUS37–42, FUS54–59 (containing two Tyr residues) and Aβ16–21 (containing two Phe residues). The distribution patterns of the π stacking angle were alike in the four all-atom oligomer systems, mainly preferring perpendicular stacking, except for FUS54–59 with an equal preference for T-shape stacking. Although with strong SC–SC interactions via π–π stacking, H-bonding and hydrophobic interactions between Tyr residues, the occurrence of the polar spacers of Ser and Gly impeded the formation of successive strong interactions in the FUS37–42 and FUS54–59 systems, but enabled transient intermolecular interactions favorable for LLPS. The sticker–spacer architecture is ubiquitous in LLPS-prone proteins81 and the sticker–sticker, spacer–spacer and sticker–spacer interactions interplay82 during their LLPS process. FUS37–42 and FUS54–59 peptides possess alternating stickers (Tyr) and spacers (Ser and Gly), and the transient interactions among stickers and spacers may facilitate the reversible self-assembly. In contrast, the central consecutive hydrophobic residues LVFF in Aβ16–21 and IVY in Tau306–311, acting as hydrophobic patches, have higher contact numbers with all residues through side chain contacts. The hydrophobic patch provides persistent interactions, contributing to peptide irreversible self-assembly. Additionally, the correlations between side chain and main chain dynamics were examined by calculating the Pearson correlation coefficients between main chain dihedrals ϕ/ψ and side chain dihedral χ1. Our calculations suggest a low main chain–side chain correlation, with correlation coefficients <0.3 (Fig. S10 and S11†). These results further demonstrate that main chain and side chain play different roles in the assembly process.
The role of water in the self-assembly process of these peptides was examined by calculating the number of hydrogen bonds (H-bonds) formed between the side chain of each residue and water molecules (Fig. S12†). The results suggest that the FUS37–42/FUS54–59 peptides formed much more H-bonds with water through five out of six residues (Ser and Tyr). In contrast, only three residues (Gln, Tyr, and Lys) in the Tau306–311 peptides, and only one residue (Lys) in the Aβ16–21 peptide formed side chain H-bonds with water. These results are consistent with the lower assembly capability and the higher fluidity of FUS37–42/FUS54–59 condensates than Aβ16–21/Tau306–311 aggregates, and demonstrate the importance of water in the reversible/irreversible assembly process. A previous MD study83 has reported that the interaction of water plays an important role in the fibrillization of the Gln-containing polar heptapeptide, GNNQQNY, from the prion domain of Sup35. Interestingly, among all residues in the four peptides, the glutamine residue in the Tau306–311 peptide formed the highest number of H-bonds with water molecules, suggesting its role in mediating the assembly of Tau306–311.
Taken together, the aromatic sticker residue (Tyr) in the LARK segments, FUS37–42 (SYSGYS) and FUS54–59 (SYSSYG), are separated by spacer residues (Ser and Gly). The alternating stickers and spacers cause FUS37–42 and FUS54–59 to self-assemble into reversible aggregates. In contrast, the side chain interactions between the hydrophobic patches cause irreversible self-assembly of the Aβ16–21 and Tau306–311 peptides. These two peptides self-assemble into aggregates with high β-sheets. Side chain hydrophobic interactions and main chain H-bonding interactions collectively stabilize the aggregates (Fig. 4c and d).
FUS37–42, FUS54–59, Aβ16–21 and Tau306–311 present distinct phase separation propensities
An increasing number of studies have demonstrated that LLPS is a general property of peptides and proteins,18,19,25 and even short peptides possess sufficient interaction sites for LLPS.42,43 In order to predict the phase separation propensity of the hexapeptides, we carried out CG-MD simulations on large space and time scales based on a modified MARTINI-2.2 force field with a down-scaling of the van der Waals parameters between Ser pseudo-atoms and the backbone beads of all the amino acid residues in the FUS37–42 and FUS54–59 systems.54,55 Three individual 6 μs CG-MD simulations were carried out for each of the hexapeptide systems: FUS37–42, FUS54–59, Aβ16–21 and Tau306–311, starting from the initial states with 200 monomers randomly dispersed in the box. A detailed description of the scaling method is given in the Models and Methods section. The representative snapshots of the four hexapeptide systems in Fig. 5a show that FUS37–42, FUS54–59, Tau306–311 and Aβ16–21 were all able to self-assemble into a dense phase with ascending phase separation capabilities qualitatively consistent with the self-assembly propensity in the AA-MD simulations of the oligomer systems. Specifically, the FUS37–42 and FUS54–59 condensates exhibited a loosely packed feature and were highly dynamic. In contrast, Aβ16–21 and Tau306–311 condensates were more compact and remained relatively stable in shape as the simulation time increased. The FUS37–42 and FUS54–59 aggregates had a larger solvent accessible surface area (SASA) than the Aβ16–21 and Tau306–311 aggregates (Fig. 5b), suggesting lower phase separation propensities. The concentrations of the FUS37–42 and FUS54–59 dense phases were lower than those of the Aβ16–21 and Tau306–311 dense phases (Fig. 5c). These results indicate that the assemblies formed by the Aβ16–21 and Tau306–311 peptides were probably hydrogel-like with a high degree of solvent exclusion, and FUS37–42 and FUS54–59 assemblies were more like liquid droplets containing water molecules (Fig. 5d, larger number density of water molecules). Additionally, the cross-sections of the assemblies formed by the four peptides were provided to further demonstrate the presence of water molecules in the condensates. It can be seen from Fig. S13† that the FUS34–42 and FUS54–59 assemblies were embedded with water molecules. These water molecules may have contributed to their fluidities and also played a role in stabilizing the FUS37–42/FUS54–59 condensates. In contrast, the Aβ16–21 and Tau306–311 assemblies were densely packed and solid-like. Therefore, we infer that the LARK segments FUS37–42 and FUS54–59 underwent LLPS, and the Aβ16–21 and Tau306–311 peptides underwent LSPS. To more quantitatively characterize the different properties of the phase separations of the four peptides, we selected two order parameters, namely the collapse degree and the fluctuation of SASA (Fig. 5e), to manifest the phase separation propensities and the liquidity of the dense phase, respectively. It is shown that the aggregates formed by the Aβ16–21 and Tau306–311 peptides have a larger collapse degree and smaller SASA fluctuation, while those formed by the FUS37–42 and FUS54–59 peptides have a smaller collapse degree and larger SASA fluctuation, indicative of the higher fluidity of the FUS37–42/FUS54–59 aggregates.
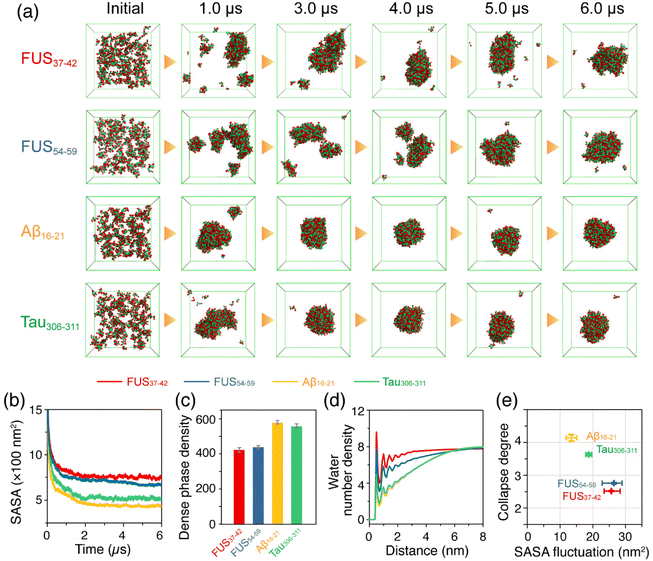 |
| Fig. 5 Phase separation behaviors of the FUS37–42, FUS54–59, Aβ16–21 and Tau306–311 hexapeptides predicted by multiple microsecond CG-MD simulations. (a) Representative snapshots of each system at six time points in 6 μs CG-MD simulations. (b) Time evolution of SASA of the FUS37–42, FUS54–59, Aβ16–21 and Tau306–311 peptides. Values were averaged over three independent 6 μs MD simulations for each system. (c) Concentrations of the dense phase of the four systems. (d) Number density of water molecules as a function of distance from the center of the condensed phase in the four systems. (e) The collapse degree values and the fluctuation of SASA of the four systems. Detailed descriptions of the parameters used are listed in the Models and Methods section. | |
Collectively, our CG-MD simulations suggest that the LARK segments FUS37–42 and FUS54–59 have low phase separation propensities and their condensates are highly dynamic and loosely packed with high mobility. In contrast, the Aβ16–21 and Tau306–311 peptides have high phase separation propensities and the aggregates are more like solids with low degrees of liquidity. Therefore, we postulate that FUS37–42 and FUS54–59 undergo liquid–liquid phase separation, while Aβ16–21 and Tau306–311 peptides undergo liquid–solid phase separation.
Conclusions
In summary, we have investigated the reversible and irreversible self-assembly of four representative hexapeptides, FUS37–42, FUS54–59, Aβ16–21 and Tau306–311, by performing AA-MD and CG-MD simulations. We characterized the self-assembly dynamics of the four hexapeptides and predicted their LLPS/LSPS propensities. Our simulations showed that the four hexapeptides self-assembled into aggregates with different physical properties. The LARKS peptides, FUS37–42 and FUS54–59, formed coil-rich aggregates with high mobility and poor thermostability, while Aβ16–21 and Tau306–311 self-assembled into β-sheets with high thermostability. Interaction analyses indicated that the persistent interactions between the hydrophobic patches, namely LVFF in Aβ16–21 and IVY in Tau306–311, acted as the main forces driving the irreversible self-assembly of Aβ16–21 and Tau306–311 and maintaining the stability of the irreversible aggregates. In contrast, the alternating sticker (Tyr) and spacers (Ser and Gly) in the FUS37–42 and FUS54–59 peptides provided transient interactions, which were strong enough to drive reversible self-assembly or LLPS and yet weak enough to inhibit irreversible aggregation or LSPS. Intriguingly, the FUS37–42 (SYSGYS) and FUS54–59 (SYSSYG) peptides had the same sequence composition and the same number of sticker and spacer residues, but exhibited different self-assembly properties, suggesting that the site-specific patterning of spacer residues (Ser and Gly) had a non-negligible effect on peptide self-assembly behaviors. Fig. 6 offers a visual illustration of the varying assembly behaviors and mechanisms of these hexapeptide aggregates. Our current work reveals the molecular mechanisms of sequence-dependent reversible and irreversible self-assembly and the phase separation behaviors of short fibril-forming peptides from amyloid proteins and LLPS-prone proteins, paving the way for further in-depth understanding of reversible LLPS and irreversible aggregation of disease-related proteins including FUS, TDP-43, and tau proteins.
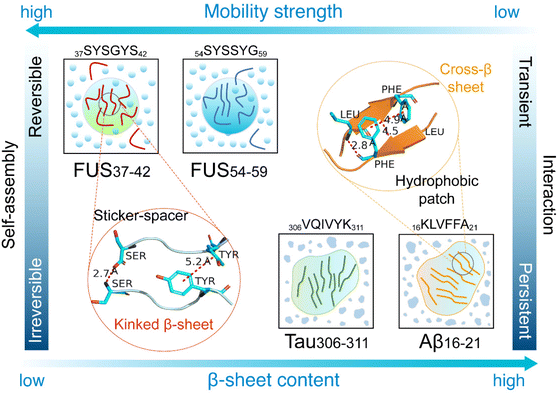 |
| Fig. 6 Schematic diagram illustrating the reversible and irreversible self-assembly behaviors and mechanisms of the four hexapeptides. | |
Author contributions
G. W., C. G., and Z. L. conceived the project and constructed the systems. Z. L. performed the all-atom simulations and analyzed the simulation data. Y. T. performed the coarse-grained simulations. G. W. and Z. L. drafted the manuscript. C. G. and G. W. edited the manuscript. All authors analyzed the data, and reviewed and approved the final version of the manuscript.
Conflicts of interest
There are no conflicts to declare.
Acknowledgements
This work is supported financially by the National Key Research and Development Program of China (Grant No. 2023YFF1204402), the National Natural Science Foundation of China (Grant No. 12074079, 11804218 and 12374208), the Natural Science Foundation of Shanghai (Grant No. 22ZR1406800), and the China Postdoctoral Science Foundation (Grant No. 2022M720815). All the MD simulations were performed using the GPU Cluster at Fudan University.
References
- Y. S. Eisele, C. Monteiro, C. Fearns, S. E. Encalada, R. L. Wiseman, E. T. Powers and J. W. Kelly, Targeting protein aggregation for the treatment of degenerative diseases, Nat. Rev. Drug Discovery, 2015, 14(11), 759–780 CrossRef CAS PubMed.
- F. Chiti and C. M. Dobson, Protein misfolding, amyloid formation, and human disease: a summary of progress over the last decade, Annu. Rev. Biochem., 2017, 86, 27–68 CrossRef CAS PubMed.
- D. J. Selkoe, Alzheimer's disease: genes, proteins, and therapy, Physiol. Rev., 2001, 81(2), 741–766 CrossRef CAS PubMed.
- F. M. LaFerla, K. N. Green and S. Oddo, Intracellular amyloid-beta in Alzheimer's disease, Nat. Rev. Neurosci., 2007, 8(7), 499–509 CrossRef CAS PubMed.
- U. Sengupta and R. Kayed, Amyloid beta, Tau, and alpha-Synuclein aggregates in the pathogenesis, prognosis, and therapeutics for neurodegenerative diseases, Prog. Neurobiol., 2022, 214, 102270 CrossRef CAS PubMed.
- D. S. Eisenberg and M. R. Sawaya, Structural studies of amyloid proteins at the molecular level, Annu. Rev. Biochem., 2017, 86, 69–95 CrossRef CAS PubMed.
- D. Li and C. Liu, Structural diversity of amyloid fibrils and advances in their structure determination, Biochemistry, 2020, 59(5), 639–646 CrossRef CAS PubMed.
- N. El Mammeri, P. Duan, A. J. Dregni and M. Hong, Amyloid fibril structures of tau: Conformational plasticity of the second microtubule-binding repeat, Sci. Adv., 2023, 9(28), eadh4731 CrossRef CAS PubMed.
- N. Vettore and A. K. Buell, Thermodynamics of amyloid fibril formation from chemical depolymerization, Phys. Chem. Chem. Phys., 2019, 21(47), 26184–26194 RSC.
- B. Morel, L. Varela and F. Conejero-Lara, The thermodynamic stability of amyloid fibrils studied by differential scanning calorimetry, J. Phys. Chem. B, 2010, 114(11), 4010–4019 CrossRef CAS PubMed.
- D. T. Murray, M. Kato, Y. Lin, K. R. Thurber, I. Hung, S. L. McKnight and R. Tycko, Structure of FUS protein fibrils and its relevance to self-assembly and phase separation of low-complexity domains, Cell, 2017, 171(3), 615–627 CrossRef CAS PubMed.
- F. Luo, X. Gui, H. Zhou, J. Gu, Y. Li, X. Liu, M. Zhao, D. Li, X. Li and C. Liu, Atomic structures of FUS LC domain segments reveal bases for reversible amyloid fibril formation, Nat. Struct. Mol. Biol., 2018, 25(4), 341–346 CrossRef CAS PubMed.
- M. P. Hughes, M. R. Sawaya, D. R. Boyer, L. Goldschmidt, J. A. Rodriguez, D. Cascio, L. Chong, T. Gonen and D. S. Eisenberg, Atomic structures of low-complexity protein segments reveal kinked beta sheets that assemble networks, Science, 2018, 359(6376), 698–701 CrossRef CAS PubMed.
- Q. Cao, D. R. Boyer, M. R. Sawaya, P. Ge and D. S. Eisenberg, Cryo-EM structures of four polymorphic TDP-43 amyloid cores, Nat. Struct. Mol. Biol., 2019, 26(7), 619–627 CrossRef CAS PubMed.
- E. L. Guenther, Q. Cao, H. Trinh, J. Lu, M. R. Sawaya, D. Cascio, D. R. Boyer, J. A. Rodriguez, M. P. Hughes and D. S. Eisenberg, Atomic structures of TDP-43 LCD segments and insights into reversible or pathogenic aggregation, Nat. Struct. Mol. Biol., 2018, 25(6), 463–471 CrossRef CAS PubMed.
- X. Gui, F. Luo, Y. Li, H. Zhou, Z. Qin, Z. Liu, J. Gu, M. Xie, K. Zhao and B. Dai,
et al., Structural basis for reversible amyloids of hnRNPA1 elucidates their role in stress granule assembly, Nat. Commun., 2019, 10(1), 2006–2017 CrossRef PubMed.
- K. A. Murray, D. Evans, M. P. Hughes, M. R. Sawaya, C. J. Hu, K. N. Houk and D. Eisenberg, Extended beta-Strands Contribute to Reversible Amyloid Formation, ACS Nano, 2022, 16(2), 2154–2163 CrossRef CAS PubMed.
- M. Hardenberg, A. Horvath, V. Ambrus, M. Fuxreiter and M. Vendruscolo, Widespread occurrence of the droplet state of proteins in the human proteome, Proc. Natl. Acad. Sci. U. S. A., 2020, 117(52), 33254–33262 CrossRef CAS PubMed.
- M. Poudyal, K. Patel, L. Gadhe, A. S. Sawner, P. Kadu, D. Datta, S. Mukherjee, S. Ray, A. Navalkar and S. Maiti,
et al., Intermolecular interactions underlie protein/peptide phase separation irrespective of sequence and structure at crowded milieu, Nat. Commun., 2023, 14(1), 6199 CrossRef CAS PubMed.
- A. Molliex, J. Temirov, J. Lee, M. Coughlin, A. P. Kanagaraj, H. J. Kim, T. Mittag and J. P. Taylor, Phase separation by low complexity domains promotes stress granule assembly and drives pathological fibrillization, Cell, 2015, 163(1), 123–133 CrossRef CAS PubMed.
- L. Guo and J. Shorter, It's raining liquids: RNA tunes viscoelasticity and dynamics of membraneless organelles, Mol. Cell, 2015, 60(2), 189–192 CrossRef CAS PubMed.
- S. Saha, C. A. Weber, M. Nousch, O. Adame-Arana, C. Hoege, M. Y. Hein, E. Osborne-Nishimura, J. Mahamid, M. Jahnel and L. Jawerth,
et al., Polar positioning of phase-separated liquid compartments in cells regulated by an mRNA competition mechanism, Cell, 2016, 166(6), 1572–1584 CrossRef CAS PubMed.
- S. Boeynaems, S. Alberti, N. L. Fawzi, T. Mittag, M. Polymenidou, F. Rousseau, J. Schymkowitz, J. Shorter, B. Wolozin and L. Van Den Bosch,
et al., Protein Phase Separation: A New Phase in Cell Biology, Trends Cell Biol., 2018, 28(6), 420–435 CrossRef CAS PubMed.
- Z. Wang and H. Zhang, Phase separation, transition, and autophagic degradation of proteins in development and pathogenesis, Trends Cell Biol., 2019, 29(5), 417–427 CrossRef CAS PubMed.
- S. Maharana, J. Wang, D. K. Papadopoulos, D. Richter, A. Pozniakovsky, I. Poser, M. Bickle, S. Rizk, J. Guillen-Boixet and T. M. Franzmann,
et al., RNA buffers the phase separation behavior of prion-like RNA binding proteins, Science, 2018, 360(6391), 918–921 CrossRef CAS PubMed.
- Y. Shin and C. P. Brangwynne, Liquid phase condensation in cell physiology and disease, Science, 2017, 357(6357), eaaf4382 CrossRef PubMed.
- S. Alberti, A. Gladfelter and T. Mittag, Considerations and Challenges in Studying Liquid-Liquid Phase Separation and Biomolecular Condensates, Cell, 2019, 176(3), 419–434 CrossRef CAS PubMed.
- H. Yoo, C. Triandafillou and D. A. Drummond, Cellular sensing by phase separation: using the process, not just the products, J. Biol. Chem., 2019, 294(18), 7151–7159 CrossRef CAS PubMed.
- J. G. Daigle, N. A. Lanson Jr., R. B. Smith, I. Casci, A. Maltare, J. Monaghan, C. D. Nichols, D. Kryndushkin, F. Shewmaker and U. B. Pandey, RNA-binding ability of FUS regulates neurodegeneration, cytoplasmic mislocalization and incorporation into stress granules associated with FUS carrying ALS-linked mutations, Hum. Mol. Genet., 2013, 22(6), 1193–1205 CrossRef CAS PubMed.
- A. Patel, H. O. Lee, L. Jawerth, S. Maharana, M. Jahnel, M. Y. Hein, S. Stoynov, J. Mahamid, S. Saha and T. M. Franzmann,
et al., A liquid-to-solid phase transition of the ALS protein FUS accelerated by disease mutation, Cell, 2015, 162(5), 1066–1077 CrossRef CAS PubMed.
- J. P. Taylor, R. H. Brown Jr. and D. W. Cleveland, Decoding ALS: from genes to mechanism, Nature, 2016, 539(7628), 197–206 CrossRef PubMed.
- G. Antonacci, V. de Turris, A. Rosa and G. Ruocco, Background-deflection Brillouin microscopy reveals altered biomechanics of intracellular stress granules by ALS protein FUS, Commun. Biol., 2018, 1, 139 CrossRef PubMed.
- A. G. Niaki, J. Sarkar, X. Cai, K. Rhine, V. Vidaurre, B. Guy, M. Hurst, J. C. Lee, H. R. Koh and L. Guo,
et al., Loss of dynamic RNA interaction and aberrant phase separation induced by two distinct types of ALS/FTD-linked FUS mutations, Mol. Cell, 2020, 77(1), 82–94 CrossRef CAS PubMed.
- E. Bogaert, S. Boeynaems, M. Kato, L. Guo, T. R. Caulfield, J. Steyaert, W. Scheveneels, N. Wilmans, W. Haeck and N. Hersmus,
et al., Molecular Dissection of FUS Points at Synergistic Effect of Low-Complexity Domains in Toxicity, Cell Rep., 2018, 24(3), 529–537 CrossRef CAS PubMed.
- S. Qamar, G. Wang, S. J. Randle, F. S. Ruggeri, J. A. Varela, J. Q. Lin, E. C. Phillips, A. Miyashita, D. Williams and F. Strohl,
et al., FUS phase separation is modulated by a molecular chaperone and methylation of arginine cation-pi interactions, Cell, 2018, 173(3), 720–734 CrossRef CAS PubMed.
- C. Chen, X. Ding, N. Akram, S. Xue and S. Z. Luo, Fused in sarcoma: properties, self-assembly and correlation with neurodegenerative diseases, Molecules, 2019, 24(8), 1622–1638 CrossRef CAS PubMed.
- S. Li, T. Yoshizawa, Y. Shiramasa, M. Kanamaru, F. Ide, K. Kitamura, N. Kashiwagi, N. Sasahara, S. Kitazawa and R. Kitahara, Mechanism underlying liquid-to-solid phase transition in fused in sarcoma liquid droplets, Phys. Chem. Chem. Phys., 2022, 24(32), 19346–19353 RSC.
- D. T. Murray and R. Tycko, Side Chain Hydrogen-Bonding Interactions within Amyloid-like Fibrils Formed by the Low-Complexity Domain of FUS: Evidence from Solid State Nuclear Magnetic Resonance Spectroscopy, Biochemistry, 2020, 59(4), 364–378 CrossRef CAS PubMed.
- W. M. Babinchak and W. K. Surewicz, Liquid-Liquid Phase Separation and Its Mechanistic Role in Pathological Protein Aggregation, J. Mol. Biol., 2020, 432(7), 1910–1925 CrossRef CAS PubMed.
- D. De Sancho, Phase, separation in amino acid mixtures is governed by composition, Biophys. J., 2022, 121(21), 4119–4127 CrossRef CAS PubMed.
- G. Valdes-Garcia, K. Gamage, C. Smith, K. Martirosova, M. Feig and L. J. Lapidus, The effect of polymer length in liquid-liquid phase separation, Cell Rep. Phys. Sci., 2023, 4(5), 101415 CrossRef CAS PubMed.
- M. Abbas, W. P. Lipiński, K. K. Nakashima, W. T. Huck and E. Spruijt, A short peptide synthon for liquid–liquid phase separation, Nat. Chem., 2021, 13(11), 1046–1054 CrossRef CAS PubMed.
- Y. Tang, S. Bera, Y. Yao, J. Zeng, Z. Lao, X. Dong, E. Gazit and G. Wei, Prediction and characterization of liquid-liquid phase separation of minimalistic peptides, Cell Rep. Phys. Sci., 2021, 2(9), 100579–100596 CrossRef CAS.
- J. P. Colletier, A. Laganowsky, M. Landau, M. Zhao, A. B. Soriaga, L. Goldschmidt, D. Flot, D. Cascio, M. R. Sawaya and D. Eisenberg, Molecular basis for amyloid-beta polymorphism, Proc. Natl. Acad. Sci. U. S. A., 2011, 108(41), 16938–16943 CrossRef CAS PubMed.
- M. R. Sawaya, S. Sambashivan, R. Nelson, M. I. Ivanova, S. A. Sievers, M. I. Apostol, M. J. Thompson, M. Balbirnie, J. J. Wiltzius and H. T. McFarlane,
et al., Atomic structures of amyloid cross-beta spines reveal varied steric zippers, Nature, 2007, 447(7143), 453–457 CrossRef CAS PubMed.
-
The PyMOL molecular graphics system, Version 1.8, 2015 Search PubMed.
- M. J. Abraham, T. Murtola, R. Schulz, S. Páll, J. C. Smith, B. Hess and E. Lindahl, GROMACS: high performance molecular simulations through multi-level parallelism from laptops to supercomputers, SoftwareX, 2015, 1–2, 19–25 CrossRef.
- K. Lindorff-Larsen, S. Piana, K. Palmo, P. Maragakis, J. L. Klepeis, R. O. Dror and D. E. Shaw, Improved side-chain torsion potentials for the Amber ff99SB protein force field, Proteins, 2010, 78(8), 1950–1958 CrossRef CAS PubMed.
- M. Deserno and C. Holm, How to mesh up Ewald sums. I. A theoretical and numerical comparison of various particle mesh routines, J. Chem. Phys., 1998, 109(18), 7678–7693 CrossRef CAS.
- M. Deserno and C. Holm, How to mesh up Ewald sums. II. An accurate error estimate for the particle-particle-particle-mesh algorithm, J. Chem. Phys., 1998, 109(18), 7694–7701 CrossRef CAS.
- X. Liu, Z. Lao, X. Li, X. Dong and G. Wei, ALS-associated A315E and A315pT variants exhibit distinct mechanisms in inducing irreversible aggregation of TDP-43 312-317 peptide, Phys. Chem. Chem. Phys., 2022, 24(26), 16263–16273 RSC.
- G. Bussi, D. Donadio and M. Parrinello, Canonical sampling through velocity rescaling, J. Chem. Phys., 2007, 126(1), 014101 CrossRef PubMed.
- M. Parrinello and A. Rahman, Polymorphic transitions in single-crystals - a new molecular-dynamics method, J. Appl. Phys., 1981, 52(12), 7182–7190 CrossRef CAS.
- D. H. de Jong, G. Singh, W. F. Bennett, C. Arnarez, T. A. Wassenaar, L. V. Schafer, X. Periole, D. P. Tieleman and S. J. Marrink, Improved parameters for the MARTINI coarse-grained protein force field, J. Chem. Theory Comput., 2013, 9(1), 687–697 CrossRef CAS PubMed.
- A. C. Stark, C. T. Andrews and A. H. Elcock, Toward optimized potential functions for protein-protein interactions in aqueous solutions: osmotic second virial coefficient calculations using the MARTINI coarse-grained force field, J. Chem. Theory Comput., 2013, 9(9), 4176–4185 CrossRef CAS PubMed.
- I. G. Tironi, R. Sperb, P. E. Smith and W. F. Vangunsteren, A generalized reaction field method for molecular-dynamics simulations, J. Chem. Phys., 1995, 102(13), 5451–5459 CrossRef CAS.
- A. C. Stark, C. T. Andrews and A. H. Elcock, Toward optimized potential functions for protein-protein interactions in aqueous solutions: osmotic second virial coefficient calculations using the MARTINI coarse-grained force field, J. Chem. Theory Comput., 2013, 9(9), 4176–4185 CrossRef CAS PubMed.
- Z. Benayad, S. von Bulow, L. S. Stelzl and G. Hummer, Simulation of FUS Protein Condensates with an Adapted Coarse-Grained Model, J. Chem. Theory Comput., 2021, 17(1), 525–537 CrossRef CAS PubMed.
- Y. Tang, S. Bera, Y. Yao, J. Zeng, Z. Lao, X. Dong, E. Gazit and G. Wei, Prediction and characterization of liquid-liquid phase separation of minimalistic peptides, Cell Rep. Phys. Sci., 2021, 2(9), 100579 CrossRef CAS.
- A. Huet and P. Derreumaux, Impact of the mutation A21G (Flemish variant) on Alzheimer's beta-amyloid dimers by molecular dynamics simulations, Biophys. J., 2006, 91(10), 3829–3840 CrossRef CAS PubMed.
- Y. Luo, B. Ma, R. Nussinov and G. Wei, Structural Insight into Tau Protein's Paradox of Intrinsically Disordered Behavior, Self-Acetylation Activity, and Aggregation, J. Phys. Chem. Lett., 2014, 5(17), 3026–3031 CrossRef CAS PubMed.
- Z. Lao, Y. Chen, Y. Tang and G. Wei, Molecular dynamics simulations reveal the inhibitory mechanism of dopamine against human islet amyloid polypeptide (hIAPP) aggregation and its destabilization effect on
hIAPP protofibrils, ACS Chem. Neurosci., 2019, 10(9), 4151–4159 CrossRef CAS PubMed.
- R. B. Fenwick, L. Orellana, S. Esteban-Martin, M. Orozco and X. Salvatella, Correlated motions are a fundamental property of beta-sheets, Nat. Commun., 2014, 5, 4070 CrossRef CAS PubMed.
-
S. R. Jammalamadaka and A. SenGupta, Topics in circular statistics, world scientific, 2001 Search PubMed.
- H. Li, Y. Luo, P. Derreumaux and G. Wei, Carbon nanotube inhibits the formation of beta-sheet-rich oligomers of the Alzheimer's amyloid-beta(16-22) peptide, Biophys. J., 2011, 101(9), 2267–2276 CrossRef CAS PubMed.
- P. H. Nguyen, J. M. Campanera, S. T. Ngo, A. Loquet and P. Derreumaux, Tetrameric Abeta40 and Abeta42 beta-Barrel Structures by Extensive Atomistic Simulations. II. In Aqueous Solution, J. Phys. Chem. B, 2019, 123(31), 6750–6756 CrossRef CAS PubMed.
- V. H. Man, X. He, J. Gao and J. Wang, Effects of All-Atom Molecular Mechanics Force Fields on Amyloid Peptide Assembly: The Case of PHF6 Peptide of Tau Protein, J. Chem. Theory Comput., 2021, 17(10), 6458–6471 CrossRef CAS PubMed.
- W. G. Touw, C. Baakman, J. Black, T. A. te Beek, E. Krieger, R. P. Joosten and G. Vriend, A series of PDB-related databanks for everyday needs, Nucleic Acids Res., 2015, 43(D1), D364–D368 CrossRef CAS PubMed.
- S. K. Burley and G. A. Petsko, Aromatic-aromatic interaction: a mechanism of protein structure stabilization, Science, 1985, 229(4708), 23–28 CrossRef CAS PubMed.
- P. W. Frederix, G. G. Scott, Y. M. Abul-Haija, D. Kalafatovic, C. G. Pappas, N. Javid, N. T. Hunt, R. V. Ulijn and T. Tuttle, Exploring the sequence space for (tri-)peptide self-assembly to design and discover new hydrogels, Nat. Chem., 2015, 7(1), 30–37 CrossRef CAS PubMed.
- W. Humphrey, A. Dalke and K. Schulten, VMD: visual molecular dynamics, J. Mol. Graphics Modell., 1996, 14(1), 33–38 CrossRef CAS PubMed.
- A. E. Aliev, M. Kulke, H. S. Khaneja, V. Chudasama, T. D. Sheppard and R. M. Lanigan, Motional timescale predictions by molecular dynamics simulations: case study using proline and hydroxyproline sidechain dynamics, Proteins, 2014, 82(2), 195–215 CrossRef CAS PubMed.
- Y. Lin, S. L. Currie and M. K. Rosen, Intrinsically disordered sequences enable modulation of protein phase separation through distributed tyrosine motifs, J. Biol. Chem., 2017, 292(46), 19110–19120 CrossRef CAS PubMed.
- J. Wang, J. M. Choi, A. S. Holehouse, H. O. Lee, X. Zhang, M. Jahnel, S. Maharana, R. Lemaitre, A. Pozniakovsky and D. Drechsel,
et al., A molecular grammar governing the driving forces for phase separation of prion-like RNA binding proteins, Cell, 2018, 174(3), 688–699 CrossRef CAS PubMed.
- A. C. Murthy, G. L. Dignon, Y. Kan, G. H. Zerze, S. H. Parekh, J. Mittal and N. L. Fawzi, Molecular interactions underlying liquid-liquid phase separation of the FUS low-complexity domain, Nat. Struct. Mol. Biol., 2019, 26(7), 637–648 CrossRef CAS PubMed.
- D. K. Klimov and D. Thirumalai, Dissecting the assembly of Abeta16-22 amyloid peptides into antiparallel beta sheets, Structure, 2003, 11(3), 295–307 CrossRef CAS PubMed.
- D. Thirumalai, A. Kumar, D. Chakraborty, J. E. Straub and M. L. Mugnai, Conformational fluctuations and phases in fused in sarcoma (FUS) low–complexity domain, Biopolymers, 2023, e23558 CrossRef CAS PubMed.
- G. Krainer, T. J. Welsh, J. A. Joseph, J. R. Espinosa, S. Wittmann, E. de Csillery, A. Sridhar, Z. Toprakcioglu, G. Gudiskyte and M. A. Czekalska,
et al., Reentrant liquid condensate phase of proteins is stabilized by hydrophobic and non-ionic interactions, Nat. Commun., 2021, 12(1), 1085–1098 CrossRef CAS PubMed.
- J. A. Joseph, A. Reinhardt, A. Aguirre, P. Y. Chew, K. O. Russell, J. R. Espinosa, A. Garaizar and R. Collepardo-Guevara, Physics-driven coarse-grained model for biomolecular phase separation with near-quantitative accuracy, Nat. Comput. Sci., 2021, 1(11), 732–743 CrossRef PubMed.
- R. M. Vernon, P. A. Chong, B. Tsang, T. H. Kim, A. Bah, P. Farber, H. Lin and J. D. Forman-Kay, Pi-Pi contacts are an overlooked protein feature relevant to phase separation, eLife, 2018, 7, e31486 CrossRef PubMed.
- J. M. Choi, A. S. Holehouse and R. V. Pappu, Physical Principles Underlying the Complex Biology of Intracellular Phase Transitions, Annu. Rev. Biophys., 2020, 49, 107–133 CrossRef CAS PubMed.
- E. W. Martin, A. S. Holehouse, I. Peran, M. Farag, J. J. Incicco, A. Bremer, C. R. Grace, A. Soranno, R. V. Pappu and T. Mittag, Valence and patterning of aromatic residues determine the phase behavior of prion-like domains, Science, 2020, 367, 694–699 CrossRef CAS PubMed.
- G. Reddy, J. E. Straub and D. Thirumalai, Dry amyloid fibril assembly in a yeast prion peptide is mediated by long-lived structures containing water wires, Proc. Natl. Acad. Sci. U. S. A., 2010, 107(50), 21459–21464 CrossRef CAS PubMed.
|
This journal is © The Royal Society of Chemistry 2024 |
Click here to see how this site uses Cookies. View our privacy policy here.