DOI:
10.1039/D4NR03050H
(Review Article)
Nanoscale, 2024,
16, 17723-17760
Recent developments of artificial intelligence in MXene-based devices: from synthesis to applications
Received
23rd July 2024
, Accepted 20th August 2024
First published on 27th August 2024
Abstract
Two-dimensional transition metal carbides, nitrides, or carbonitrides (MXenes) have garnered remarkable attention in various energy and environmental applications due to their high electrical conductivity, good thermal properties, large surface area, high mechanical strength, rapid charge transport mechanism, and tunable surface properties. Recently, artificial intelligence has been considered an emerging technology, and has been widely used in materials science, engineering, and biomedical applications due to its high efficiency and precision. In this review, we focus on the role of artificial intelligence-based technology in MXene-based devices and discuss the latest research directions of artificial intelligence in MXene-based devices, especially the use of artificial intelligence-based modeling tools for energy storage devices, sensors, and memristors. In addition, emphasis is given to recent progress made in synthesis methods for various MXenes and their advantages and disadvantages. Finally, the review ends with several recommendations and suggestions regarding the role of artificial intelligence in fabricating MXene-based devices. We anticipate that this review will provide guidelines on future research directions suitable for practical applications.
1. Introduction
The intersection of materials science and artificial intelligence has yielded a compelling prospect in the form of two-dimensional (2D) materials.1–4 For the development of next generation auto-devices, 2D materials are considered as an verstile nanomaterials.5–7 Because of their high surface area, tunable physiochemical properties, desirable flexibility, optimal interlayer distance, and mechanical robustness, the scientific world is compelled to use these materials in modern appliances and sensors.8–12 Studies showed that 2D materials such as metal–organic frameworks (MOFs), graphene, metal dichalcogenides, covalent organic frameworks, etc., have successfully attracted attention for the fabrication of artificial intelligence devices.13–18 However, several barriers, including difficult functionalization, the presence of structural defects, non-smoothing electrical flow, low water dispersibility, and some selectivity problems, limit their commercial applications.19,20 On the other hand, metal nitrides and carbides (MXenes) have been explored as promising candidates for 2D materials in the development of advanced next generation devices.21–24 A significant boost has also been seen in the fabrication of artificial intelligence-based devices from MXenes, particularly, sensors, human perception devices, memristors, and energy storage and conversion devices.25,26 Artificial intelligence (AI) has recently gained popularity as a research and development tool for replicating and augmenting human intelligence.27 It demonstrated good improvement in spatial and temporal sensing features as a result of the selective interaction with analytes and quick response–recovery time observed respectively.28 Furthermore, MXene materials have the potential to tackle fundamental challenges related to the integration and performance of advanced sophisticated devices. MXene can be used for the scalable manufacture of free-standing films; their low cost, size, and complex preparation methods play a role in the smooth processing of AI devices due to high water dispersibility.29–33 MXene augmented AI technology due its exceptional electro-conductivity, mechanical robustness and versatile surface chemistry.24,34,35 This synergetic and simultaneous combination of intelligent systems and advanced 2D materials opens a new era of research and hope in the scientific world and has indicated diverse promising AI based applications on the landscape of modern technology.36–39 In fact, the AI field is particularly concerned with building sophisticated machines, including auto-responsive and computing devices that can learn, act, and reason, which usually require incorporating data with human intelligence. However, the modern advancement in technology would make it capable of completing tasks beyond normal human abilities.40–42 Furthermore, the recent development and exponential growth of AI technology increases the demand for research on 2D materials used for the preparation of modern devices.43,44 The discovery of MXene and its advancements in AI can shift the paradigm of possible required objects in artificial intelligence devices.45
In addition, the conductivity properties of MXene have a key attractive point for AI scientists because of their applications in rapid data processing integrated into AI algorithms, such as artificial electronic skin.46–48 This property not only performs required computational tasks but also increases the energy efficiency of the developed AI system, which is crucial in AI-based research.47,49,50 Furthermore, the mechanical robustness and resilience of MXene have also provided a new approach for the fabrication of flexible and wearable AI-based sensing devices and electronic devices, beating all other traditional materials from the same perspective.51,52 The greater ability of MXene to integrate with AI systems could have a significant impact on human–machine interactions and our routine lives.53 Furthermore, due to the supreme properties of MXene, it has been used for definitive sensory AI technology, AI-based memristors, and energy storage.54 The high sensitivity of MXene created an opportunity to develop intelligent sensors for the detection of any environmental stimuli, such as toxic gases, pressure and temperature variations, and biosensing abilities.55–58 Therefore, these exceptional properties of the material proved promising for real time AI applications ranging from routine health diagnosis to environmental monitoring. MXene can play a vital role in the development of sustainable energy storage devices for AI devices, as it has several attractive electrochemical properties.2,59–61 Therefore, MXene is a potential material for the fabrication of sustainable batteries and supercapacitors, which would prolong operational lifespans and encourage environmentally friendly usage.62 In this way, it has the ability to address critical environmental concerns regarding the proliferation of AI technology.63,64
In this work, we have evaluated the recent progress of AI technology in MXene-based devices, with a particular focus on sensors, energy storage devices, and memristors. First, we discuss different synthesis methods for MXenes along with their advantages and disadvantages. Then, MXene-based sensors and their usage in AI-related devices, the usage of MXene in AI-based energy storage devices, and MXene-prepared memristors and their connectivity with human perception are highlighted. In this discussion, we have tried to prove that MXene-based materials in AI applications are not only perceptive and responsive but also sustainable and adaptable. In the tapestry of AI evolution, MXenes emerge as a vibrant thread, weaving innovation, efficiency, and intelligence into the very fabric of our technological future. In this review, the multidimensional applications of MXene in AI and its potential to transform this field were explored.
2. Synthesis of pristine MXenes
The foundation for expanding the applications of MXene materials lies in the development of an efficient synthesis route. Since its discovery, extensive research has been ongoing on physical and chemical methods of synthesis. Researchers have explored diverse strategies to selectively etch the MAX phase, aiming for diverse configurations and uniqueness in the properties of MXenes.65,66 In the MAX phase, MAX bonds primarily consist of strong covalent and ionic bonds, whereas MAX bonds are predominantly metallic and characterized by weaker binding. This distinction enables the selective etching out of the “A” layer from MAX phases involving suitable etchants. Khazaei and colleagues undertook calculations involving force constants and stagnant exfoliation energies for numerous MAX phases, enabling the prediction of their etching and exfoliation potential.67 Their findings revealed a linear correlation between exfoliation energies and the total force constant of atoms present in MAX phases, offering insights into bond strength and exfoliation feasibility. Specifically, a lower total force constant of “A” atoms, influenced by neighboring atoms, indicates reduced exfoliation energy. During the production of multi-layer stacked MXenes, the A layer is replaced concurrently with surface terminations and decorations due to the effect of etchant or reactants and the surrounding medium. Normally, this phenomenon obtains MXene structures with diverse configurations and shows enriched, manipulated properties, paving the way for wider applications in several fields. Given the ongoing diversity in MXene synthesis strategies, this review focuses on the evolution of MXene preparation methods, encompassing various techniques such as HF etching, H–F forming etching, alkali-based etching, electrochemical types of etching, molten salt etching, and several others, as shown in Fig. 1. Additionally, the review explores intercalation and delamination approaches utilized for producing mono-layer MXene by using organic intercalation, inorganic intercalation, and mechanical delamination. An overview of the synthesis is covered in separate sections. The discussion delves into the factors influencing synthesis conditions, outlining the merits and drawbacks of each method. Furthermore, the review extends the conversation to encompass large-scale preparation techniques and existing anti-oxidation strategies employed for MXene storage. The review highlights the current status of large-scale synthesis methods and the reported antioxidation activities associated with MXenes. Finally, the review provides a perspective on the advancements in synthesis routes, storage methodologies, and the industrial applications of MXenes. It offers a comprehensive analysis of the progress made in MXene research and presents a forward-looking view of future developments in the field of MXene synthesis and applications.
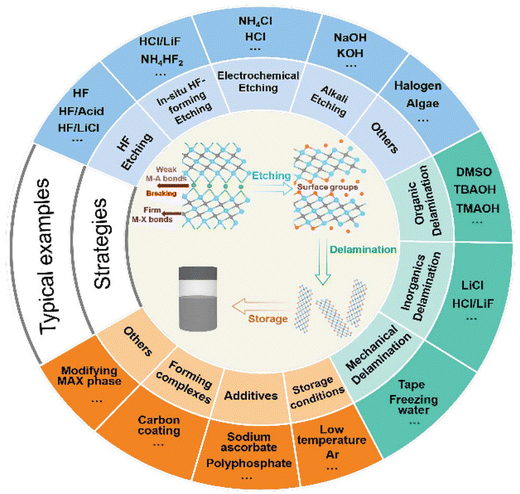 |
| Fig. 1 Overview of MXene synthesis routes, etching techniques, delamination methods, and MXene storage skills. Reprinted with permission from ref. 68. Copyright 2021, John Wiley & Sons. | |
2.1 Etching methods for the synthesis of MXenes
2.1.1 Hydrofluoric acid (HF) etching-based method.
In the earliest work, it was established that HF was the only etchant for the selective extraction of MXenes from their MAX phase precursors.69 By specifically targeting the Al atomic layers in Ti3AlC2, 50% HF facilitated the formation of accordion-like Ti3C2Tx powder. This process exploited the high reactivity between MAX phases having Al and F ions, which resulted in van der Waals forces and hydrogen bonds between layers. The resulting Ti3C2Tx powder retained the stoichiometry and crystal structure of Ti3AlC2, except for the absence of the Al atomic layer. Surface terminations such as F, OH, and O were introduced during the aqueous etching process.
2.1.1.1 Influence of HF concentration, temperature, and time.
Alhabeb et al. delved into the impact of HF concentration and etching duration on Ti3AlC2 at room temperature. Higher HF concentrations led to shortened etching times and increased efficiency. Notably, the accordion-like structure, a common feature in MXene products, did not solely indicate successful etching. This structure primarily arose from the exothermic reaction during Al removal and H2 release. Additionally, HF concentration influenced surface terminations, with lower concentrations favoring F and oxygen-containing terminations, while higher concentrations increased F terminations and reduced oxygen-containing terminations.70
2.1.1.2 Effect of etching temperature and time.
The etching temperature and duration significantly influenced the process. Extended etching times and elevated temperatures led to enlarged interlayer spacing. Post-etching and meticulous washing were essential to remove excess acid and by-products. The appropriate selection of HF concentration and etching time was vital; excess concentrations and longer durations resulted in increased defect concentrations and reduced lateral MXene size due to HF's corrosive nature. Therefore, it is necessary to use the optimal time and concentration of HF during the preparation of MXene.71
2.1.1.3 Influence of MXene properties on etching conditions.
Tailoring MXenes for specific applications requires distinct etching conditions. Optics and electronics applications demand mild conditions (low concentration of HF and a short time for etching) to yield large lateral-size MXenes with few defects.72 Conversely, MXenes for catalysis and applications like gas sensing benefit from small lateral sizes and have rich and active edges.73 The choice of etching conditions was intricately linked to the composition and structure of the MAX phases. For instance, Ti3AlC2 required low HF concentrations (e.g., 5%), while V- and Nb-based MXenes necessitated 50 wt% HF. Mixing HF with other acids, e.g., HCl, H2SO4, or oxidants, adjusted the HF content to enhance etching while minimizing HF use.74
2.1.1.4 Utilizing non-MAX phase precursors.
Non-MAX phase precursors also found utility in MXene synthesis. By selectively extracting Al3C3 layers from non-MAX Zr3Al3C5 using 50 wt% HF, novel Zr3C2Tx MXenes were obtained. This approach broadened the variety of MXenes, expanding their potential applications.75 In summary, despite the simplicity and low reaction temperatures offered by HF etching, concerns surrounding its corrosiveness and toxicity, along with operational risks, and impacts on particular environments persisted.76,77 In addition to this, the resulting products contained numerous −F groups on their surfaces, making them less favorable for energy storage applications.78 Thus, research efforts must focus on exploring alternative etching methods, emphasizing approaches that are less toxic and environmentally friendly, to advance MXene synthesis.
2.2
In situ HF forming etching methods
To mitigate the corrosive challenges associated with HF etchants, researchers have explored alternative etching approaches that facilitate the in situ formation of HF etchants. In these in situ HF forming systems, the highly reactive F ions interact with Al atoms in MAX precursors, leading to the creation of fluoride, H2, and the desired MXenes. To avoid the use of HF directly, these in situ HF forming methods offer advantages such as simplified operation, low energy consumption, and minimized chemical effects and risks associated with the etching process.
2.2.1 X-acid/fluoride salt based etching toward safer synthesis.
A significant advancement in this domain was the use of HCl/LiF solution for etching Ti3AlC2 at about 35 °C, successfully achieved by Ghidiu and coworkers.79 This method yielded Ti3C2Tx conductive clay, which displayed exceptional flexibility, high toughness, and good hydrophilicity, making it suitable for various applications, including supercapacitors. Similar to HF etching, this process resulted in multilayered accordion-like MXenes. Diverse fluoride salts, such as LiF, NaF, KF, and NH4F, have been integrated with HCl, offering flexibility in regulating the interlayer spacing of MXenes for specific applications.80 Wang et al. explored mixed solutions of HCl and different fluoride salts, tailoring the etching conditions to produce MXenes under varying temperatures and durations.81 The choice of mixed etchants, such as HCl/NH4F, significantly influenced the etching efficiency, allowing complete etching of Ti3AlC2 into Ti3C2Tx with a short etching time of 24 h at 30 °C. Furthermore, the integration of HCl with oxidants like FeF3 expands the interlayer spacing through the intercalation of Fe3+ ions. However, the presence of Fe3+ posed challenges due to potential MXene oxidation, affecting the yield. Moreover, researchers have explored hybridizing fluoride salts with other acids. Substituting H2SO4 for HCl, for instance, increased SO4 termination on Ti3C2Tx surfaces without affecting the conductivity. This strategy expanded the interlayer spacing, enhanced electrolyte ion infiltration, and contributed to enhanced supercapacitor performance.82 When using HCl/LiF, specific ratios, such as LiF
:
MAX phase = 7.5
:
1, and HCl concentrations between 6 and 12 M, have been effective. Variations in composition significantly influence the morphological and physicochemical properties. Higher LiF and HCl concentrations yield MXenes with larger lateral sizes. The resulting MXenes can have diverse properties; smaller sheets are ideal for powder applications, while larger sheets generate sliced structures.70,83 The etching duration varies based on the MAX phase, with common Ti-based MXenes requiring approximately 24 h for complete etching. However, new MAX phases might necessitate longer durations due to higher stripping energy. For example, Mo2Ga2C required 16 days for adequate etching.84 The in situ HF-forming etching method introduces surface functionalities like F, OH, and O, often accompanied by water molecule intercalations. Drying MXenes derived from this method leads to decreased interlayer spacing due to the absence of water molecules. The type and quantity of surface terminations influence interlayer spacing, with F groups inversely related to the presence of water molecules and interlayer spacing. When compared to HF etching, the acid/fluoride salt etching method is milder and safer. Accordion-like MXene nanosheets prepared via the HCL/LiF method can be easily delaminated into monolayer MXene sheets, simplifying the preparation of 2D MXene nanosheets.81 However, this method often leaves a residue of the unetched MAX phase, necessitating systematic approaches for greater yield and purity. Future research endeavors must focus on refining these methods to maximize MXene production efficiency and purity.
2.2.2 Etching with bifluoride salts.
The utilization of NH4HF2 in the etching of sputter-deposited epitaxial Ti3AlC2 films at room temperature was reported.86 This method successfully removed the Al atom layers from Ti3AlC2, as confirmed by STEM images displaying the organized arrangement of Ti and C atom layers. During the etching process, the hydrated cations detach from fluoride salts and interact with the negatively charged MXene surface, enlarging the inter-layer spacing. This fluoride salt etching technique was applicable not only to thin epitaxial Ti3AlC2 films but also to Ti3AlC2 powders. Persson et al. immersed Ti3AlC2 in a 1 M NH4HF2 solution for five days, leading to the formation of Ti3C2Tx MXenes after washing, filtering, and drying at room temperature.87 Here, NH4+ ions and water molecules intercalated into the MXene interlayers, expanding the interlayer spacing. Other fluoride salts, such as NaHF2 and KHF2, were also employed as etchants for Ti3AlC2, resulting in Ti3C2Tx formation.88 XRD patterns indicated a reduction in MAX phase peaks with increasing etching time. Introducing K+ and NH4+ ions resulted in an interlayer spacing of 24.8 Å for Ti3C2Tx, while the use of NaHF2 reduced this spacing to 21.4 because of the smaller radius of Na+. Natu et al. explored a water-free approach by utilizing NH4HF2 in propylene carbonate, generating stable MXene dispersion.89
2.2.3 Etching with other in situ HF forming media.
Researchers have explored similar principles for etching MAX phases. Wu et al. developed a hybrid type of etchant (NH4F+ low eutectic solvent) that produced HF hydrothermally in the range of 100–80 °C for 24 h by mixing NH4F with a low eutectic solvent of choline chloride and oxalic acid. In this reaction, Ti–Al bonds in Ti3AlC2 are broken down. This method yielded multi-layered Ti3C2Tx MXene intercalated with choline ions in MXene's interlayers, enhancing the interlayer spacing. This dual-functional method exhibited potential for increasing the kinetic efficiency of lithium ions and the reversible capability of MXene based anodes in lithium ion-based batteries.90 Fluoride containing ionic liquids, including 1-ethyl-3-methyl imidazolium tetrafluoroborate (EMIMBF4) and 1-butyl-3-methylimidazolium hexafluorophosphate (BMIMPF6), were also utilized for etching Ti3AlC2 and Ti2AlC at 80 °C for 20 h. Despite eliminating the need for acidic solutions, the kinetics of this process are dependent on the activity of dissociated organic anions in an aqueous system and the interactions of fluoride ions in an ionic liquid.91 These innovative methods provide diverse avenues for tailored MXene synthesis.
2.3 Synthesis of MXene through electrochemical etching methods
The electrochemical method of etching involves the preparation of MXene by selectively removing Al layers from the MAX phase and using it as an electrode under specific voltage conditions (Fig. 2). This method enables the production of carbide-derived carbon (CDC) from the MAX phase utilizing electrolytic systems such as NaCl, HCl, or HF.92 During cyclic voltammetry (CV) between 0 and 2.5 V, breaking the M–A bond removes the A-layer in the MAX phase, progressing to amorphous carbon materials as the M-layer is further eliminated.92 Raman spectroscopy confirms the successful transformation of Ti3AlC2, Ti3SiC2, and Ti2AlC MAX phases in CDCs using different electrolytes. Precise control of synthesized MXenes is achieved by modulating the etching voltage within the reaction potential range between the A and M layers and adjusting the etching time. However, surface CDCs often hinder further etching due to initial etching on the MAX electrode surface. Sun et al. effectively controlled etching by maintaining a voltage of 0.6 V (vs. Ag/AgCl) with the use of a three-electrode system.92 Ti2AlC blocks were employed as working electrodes, with Ag/AgCl, Pt, and HCl as the standard, counter-electrode, and system, respectively. The electrochemical etching process transformed Ti2AlC into Ti2CTx and CDC layers, with surface CDCs limiting further etching, forming an MXene-covered MAX. Prolonged electrochemical etching led to the complete transformation of the MAX phase into CDC. The impacts of etching time and electrolyte concentration were studied. Ti2AlC morphology and products under different conditions revealed that an etching time of 120 h with 2 M HCl resulted in accordion-like structures. Extending the etching to 14 days produced typical CDC morphology, indicating Ti2AlC conversion. Despite advantages such as low temperature and minimal corrosive acid use, the electrochemical method's CDC layer over MXenes and low yield are concerns. Yang et al. employed a two-electrode system with Ti3AlC2 MAX pieces as both working and counter electrodes, using H2SO4, HNO3, NaOH, NH4Cl, and FeCl3 as electrolytes.93 The study highlighted the Cl-containing electrolytes’ ability to etch the Al layer, achieving an etching yield of about 40%. To enhance MAX phase accessibility, intercalators increase interlayer spacing to facilitate continuous etching. For example, 1 M NH4Cl and 0.2 M TMAOH mixed electrolytes intercalated into the MAX phase to enhance electrolyte accessibility. Combining Cl− and Al3+ ions allowed efficient Ti–Al bond breakage, enabling effective MAX phase etching. Although reducing CDC layer interference, the intercalators’ toxicity raises safety concerns. Hao et al.94 introduced a thermo-assisted electrochemical etching method without intercalating agents. Using a three-electrode system and 1 M HCl, Pt, and calomel electrodes as the electrolyte, counter electrode, and reference electrode, respectively, various MAX phases (Ti2AlC, V2AlC, and Cr2AlC) were etched. Ti2AlC morphology and products at different HCl concentrations, temperatures, and reaction times displayed accordion-like structures after 3–9 h at 50 °C, with minimized CDC layer generation in 9 h, achieving improved efficiency.94 While electrochemical etching offers a green and safe synthesis with low energy consumption,95 the persistent challenge lies in minimizing the CDC layer and increasing the MXene yield. The current method, despite MAX phase electrode recyclability, yields insufficient MXenes for large-scale production. Further refinement is essential for practical applications (Fig. 3).92–94
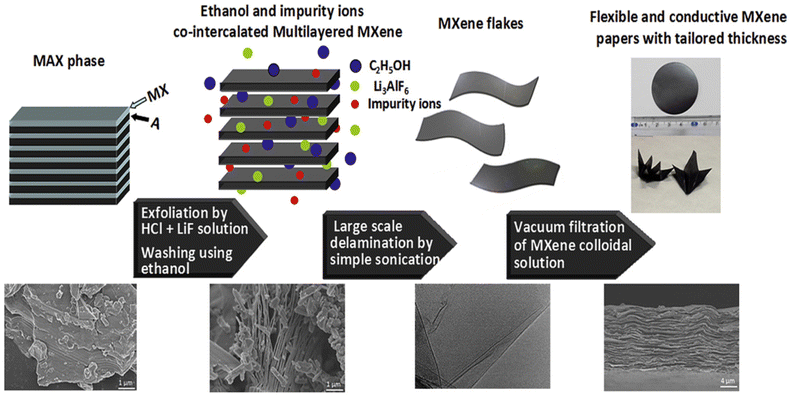 |
| Fig. 2 MAX phase etching for the preparation of MXene through the HF/LiF method. Reprinted with permission from ref. 85. Copyright 2017 Elsevier. | |
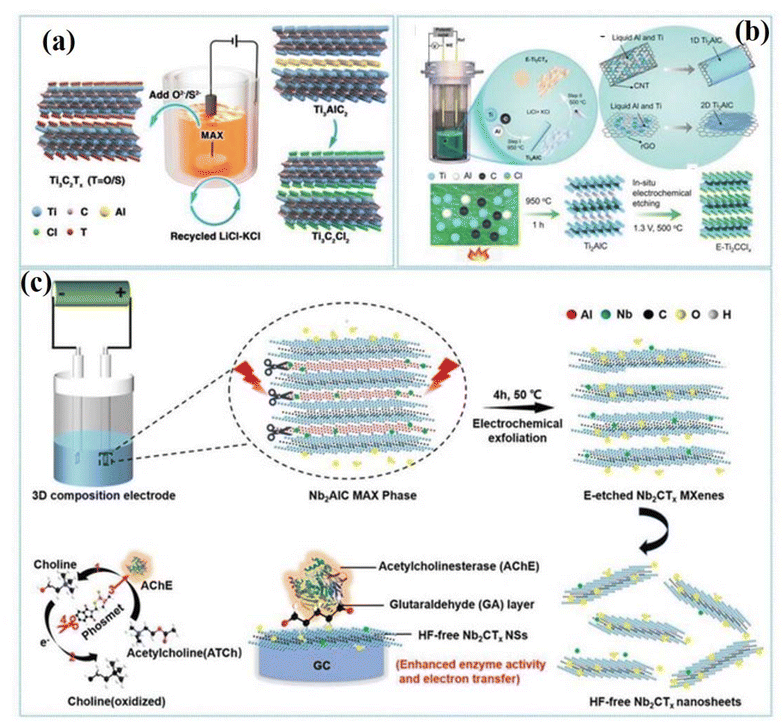 |
| Fig. 3 Electrochemical MXene etching. (a) The synthesis of MXene from the MAX phase through electrochemical etching, along with the in situ modification of surface terminations. (b) Molten salt cell schematic, utilizing a (Ti, Al, C) pellet as the working electrode, glassy carbon as the reference electrode, and a graphite crucible as the counter electrode. Reaction process outlining the production of 1D and 2D Ti2AlC using CNTs and rGO as carbon sources. (Ti, Al, C) pellet immersed in LiCl/KCl molten salt, heated at 950 °C for 1 h, to synthesize the Ti2AlC MAX phase, followed by electrochemical etching at 1.3 V for 24 h, resulting in the formation of E-Ti2CClx MXene. (c) The diagram illustrates the steps involved in the exfoliation and delamination process for the Nb2AlC MAX phase through electrochemical etching. Additionally, it highlights the enzyme inhibition effect used for the detection of phosmet with the HF-free Nb2CTx/AChE-based biosensor. Reproduced with permission from ref. 96. Copyright 2024, John Wiley & Sons. | |
2.4 Synthesis of MXene through an alkali etching method
In contrast to the acid-based methods discussed earlier, alkali-based approaches have been explored for the selective etching of the MAX phase. Xie et al. introduced a two-step etching process involving immersion of Ti3AlC2 in a 1 M NaOH solution for 100 h, followed by treatment in a 1 M H2SO4 solution for 2 h at 80 °C.97 The utilization of an alkali to eradicate Al atoms from MAX phase layers, with H2SO4 being responsible for eliminating surface exposed Al atoms, is only effective for surface etching, as it produces MXene with a lower yield compared to other traditional approaches, only etching the superficial MAX phase layer. Separating the etched MXenes from the MAX phase precursor posed challenges, and the formation of oxide hydroxide layers on the MAX phase surface hindered alkali etching. For instance, using 2 M KOH at 200 °C in a hydrothermal reaction led to the formation of a core–shell MAX@K2Ti8O17 composite, and NaOH resulted in Na2Ti7O15 formation, hindering pure MXene production. Qualitative changes occurred in the reaction when the alkali concentration and temperature increased. Successful removal of the Al layer from Ti3AlC2 was achieved using 27.5 M NaOH at 270 °C, leading to 92% yield of Ti3C2Tx (MXene).98 The reaction involved the transformation of Al into Al (oxide) hydroxides, followed by dissolution in the alkaline medium, forming fluorine-free MXenes, as shown in Fig. 4. The challenges for synthesizing MXene with this process have been addressed, along with successful synthesis. At low temperatures, this reaction showed a jamming effect, which meant the blockage of the inner layer (lattice confinement) due to the formation of dehydrated layers of insoluble Al oxides and hydroxide, as shown in Fig. 4a. In Fig. 4b, the process was conducted at high temperatures and low concentrations. Here, the material was synthesized successfully but with a very low yield and was prone to oxidation; hence, in the process conducted at high temperatures and high concentrations of alkali, the Bayers method of aluminum extraction from bauxite ore (Fig. 4c) was followed, which helped to dissolve insoluble Al-oxide/hydroxide along with enhancing the yield of MXene. The resulting MXenes exhibited a multilayer stack-like structure and superior electrochemical performance. Furthermore, Li et al. used 0.35 M KOH in 0.05 mL water at 180 °C for 24 h to etch Ti3AlC2, replacing Al atoms with OH groups and producing large Ti3C2(OH)2 nanosheets.99 Highly concentrated KOH (∼93.3 wt%) yielded MXene nanoribbons under similar hydrothermal conditions.100 The use of concentrated alkali efficiently produces hydrophilic products with F-free terminations, yet inherent dangers of high concentration and elevated temperature limit their large-scale applicability. Moreover, the resulting MXenes typically exhibited multi-lamellar, accordion-like morphology, necessitating further intercalation and delamination for single-layer MXene nanosheet production.
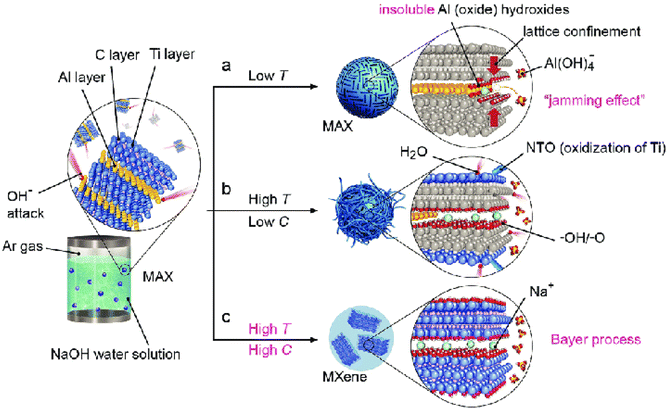 |
| Fig. 4 A visual representation of the reaction between MAX and an aqueous solution of NaOH under different conditions: (a) at low temperature, (b) at high temperature and low NaOH concentration, and (c) at high temperature and high NaOH concentration. Reproduced with permission from ref. 98. Copyright 2018 John Wiley & Sons. | |
2.5 Synthesis of MXene through molten salt etching
2.5.1 Molten salts of fluoride.
While aqueous etching methods are most suitable for Al-containing MAX phases at low operating temperatures, they fail with non-Al MAX phases like nitrides due to strong Ti–Al bonds, which present a thermodynamic limitation for etching. Urbankowski et al. successfully employed mixed molten salts (LiF, NaF, and KF) at 550 °C for 30 min to etch Ti4AlN3, resulting in Ti4N3Tx MXenes with an accordion-like morphology.87,101 Molten salt etching remains the primary method for high-energy barrier MAX phases like nitrides, as other approaches, such as thermal treatments, have proved to be less effective.102
2.5.2 MXene synthesized from fluoride-free molten salt.
In a fluoride-free approach, Huang et al. used a mixed ZnCl2/NaCl/KCl molten salt system under a nitrogen environment to etch Ti3AlC2, Ti2AlC, Ti2AlN, and V2AlC MAX phases.103 Here, ZnCl2 acted as the etchant, reacting with A atoms to form Al3+ and Zn–MAX phases, which were further etched to produce MXenes. The resulting MXenes had Cl terminations instead of F, O, or OH, as this method was fluoride-free and nonaqueous. Li et al. proposed a Lewis acid etching route using molten halides with higher electrochemical redox potentials (V, vs. Cl2/Cl−) than the MAX phase's A-site elements (V, vs. Cl2/Cl−). CuCl2, for example, etched Ti3SiC2 due to the higher redox potential of Cu2+/Cu (−0.43 V, vs. Cl2/Cl−) than Si4+/Si (−1.38 V, vs. Cl2/Cl−), resulting in Ti3C2Tx/Cu mixed powder. By replacing the halogen terminations with active ionic compounds, various terminations like O, S, or Se could be introduced, opening new pathways for surface-selective MXene design.104,105 While nonaqueous molten salt etching widens the etching range and enhances chemical safety, it is still in the early stages of development. The resulting MXenes often exhibit accordion-like structures, limiting their application in forming nanoscale complexes. Additionally, the gap between theoretically predicted properties and experimental results highlights the need for further investigation into the physical and chemical characteristics of produced MXenes, such as electric conductivity, hydrophilicity, and mechanical properties. Addressing the complex surface group combination is crucial to bridge the gap between theoretical predictions and real-world applications.
2.6 Green synthesis methods
Researchers have explored diverse methods for green, efficient, and safe MXene production. Halogens like I2 have been used for MAX phase etching. Shi et al. used I2 in anhydrous acetonitrile at 100 °C, yielding accordion-like Ti3C2Tx. Treatment with 1 M HCl converted surface I groups into OH and O, resulting in Ti3C2Tx (Ti–O, OH).106 Jawaid et al. achieved homogeneous Cl, Br, or I terminations on MXenes using halogens and interhalogen compounds in organic solvents, demonstrating the potential of that approach.107 Mechanical and electromagnetic waves have also been utilized.108 Mei et al. etched Mo2Ga2C under ultraviolet light in a phosphoric acid solution, achieving exfoliation into nanosheets.109 Ghazaly et al. used surface acoustic waves for ultrafast Ti3AlC2 conversion into Ti3C2Tx in a LiF solution.110 These methods have avoided acids and offer the potential for large-scale MXene production. The thermal reduction strategy under Ar/H2 gas produced Ti2C MXenes from Ti2SC MAX phases, although complete etching and TiO2 nucleation challenges remained.111 Algae-produced organic acids have been employed to strip MXene nanosheets from layered ternary precursors, showcasing a novel bio-inspired, environment friendly etching approach.112 Bottom-up approaches involve chemical vapor deposition (CVD) and ion sputtering. Tao et al. developed 2D nanosheets of Mo1.33CTx (MXene) with ordered metal vacancies and selectively etched away Al and Sc atoms from (Mo2/3Sc1/3)2AlC.113 The etching of i-MAX phases enables precise structural design at the atomic scale, expanding the properties of 2D materials.114–116
2.7 Large-scale preparation
It is one of the greatest goals of materials science to drive preparation from the laboratory to the industrial level so as to enhance its applicability for the production of 2D materials such as graphene and MXenes. To date, only a few methods are available for the preparative scale. In fact, the large-scale production of 2D materials is still a challenge due to the use of the bottom-up approach as a general synthesis strategy, which limits the size of the substrate during preparation. For alternative approaches, such as the hydrothermal method, the properties of the product would change upon manipulating the scale of the reaction.117 However, the MXene is prepared easily by etching the MAX phase with the utility of top-down processes. Retaining inherent properties and morphology of MXene when the synthesis process is advanced to a large scale has been a challenge until now. As in early studies, it has been investigated that HF etching has the potential to prepare MXene on a large scale for industrial purposes. Shuck et al. observed experimentally that by mixing HF/HCl the obtained product could be enhanced by up to 50 g per reaction.118,119
In addition, it has been proved that various amounts can be obtained (1 g to 50 g) after etching the MAX phase through this method, followed by the intercalation of LiCl salt for monodispersion. The resulting MXene had the same morphology and properties as the early-prepared MXene. The XRD spectra also confirm that large-scale etching has no effect on the crystal structure and peak positions measured for 1 g and 50 g of MXene. Furthermore, the consistent lateral size of 1 g and 50 g has been validated by SEM as well. However, etching out more than 50 g is still a matter of debate. Here, factors such as mechanical gradient, temperature, change in the size of the reactor, and uneven stirring may cause incomplete etching. Therefore, uniform stirring, local over-etching, corrosion of the container, and environmental issues related to HF must be considered and solved. Another challenge is to prepare MXene with a large surface area because the diameter of MXene is dependent on the size of the filter. Thus, it is necessary to explore some new method of MXene preparation that would be suitable for large-scale production, have high efficiency, and have low power consumption. Zhang et al. produced large-size MXene sheets via the etching method. They manufactured them by using a scraping blade. The produced MXene has excellent electroconductivity (15 to 100 S cm−1), a thickness of 214 nm, mechanical strength, and the capability of holding 40 g of any material without a breakdown in structure.120 In another study, Deng et al. developed a flexible membrane of MXene through electrochemical deposition as a large-scale production method in which stainless steel was used as an anode. In this process, several flexible membranes can be produced within minutes with an area of 500 cm2. Furthermore, the selectivity and size were controlled with an optimized deposition time. These developed membranes showed excellent ion rejection features for small-size metal-based ions, making them suitable for water treatment and sustainable environmental applications.121 For the large-scale production of MXenes, it is necessary to optimize the effects of precursor particle size, as it influences the quality of the final product and etching kinetics. Naguib et al. investigated that when pre-ground V2AlC precursor was added to 50% HF at room temperature, the etching time was reduced from 8 to 90 h with almost the same yield, and the interlayer spacing was enhanced from 19.72 to 24 Å. Therefore, it can be seen that the size of MXene is directly affected by particle size, as the smaller sized MAX phase (100 nm–200 nm) could form MXene with a smaller lateral size while the larger sized MAX phase could lead to incomplete etching, so the microscale MAX phase is thought to be suitable for the synthesis of MXene with optimized kinetics and size.122 Mashtalir et al. used three different fractions of Ti3AlC with sieves immersed in HF for 2 h at RT. Those sieves in sizes 38–53 showed the highest c-lattice parameter of 19.64 Å. Presently, only the Ti3C2Tx-based MXene was reported to be synthesized on a large scale, but it suffered from a low-order structure. To expand MXene etching and production on a large scale with a highly ordered structure, it is critical to use highly concentrated HF (50 wt%) with a long etching time in an aqueous system.
2.8 The delamination of multilayer MXene with intercalating reagents
2.8.1 Organic intercalation.
It is found that organic molecules are effective at the delamination and intercalation of MXene. Mashtalir et al. demonstrated Ti3C2Tx delamination into monolayer MXene nanosheets using dimethyl sulfoxide (DMSO) intercalation. The intercalation of DMSO molecules increases interlayer spacing, reduces van der Waals forces, and enables exfoliation via ultrasonication.123 Several other organic intercalating agents, such as hydrazine monohydrate (HM), N,N-dimethylformamide (DMF), urea, tetra-butyl ammonium hydroxide (TBOH), and amino-butane, are commonly used. These intercalating agents swell multilayer MXene structures, facilitating subsequent delamination through ultrasonication or hand-shaking.124 TMAOH has been used in hydrothermal processes and microwave treatments to intercalate and delaminate multilayer MXenes. TMAOH intercalates into MXene layers, allowing subsequent delamination.125,126 TMAOH, a component of commercial Al etchants, can also simultaneously etch and intercalate Ti3AlC2, leading to monolayer Ti3C2Tx production.127 While n-butyllithium was used to intercalate the MXene layers in a non-aqueous system by the centrifugation method, to prevent oxidation, it was stored in hydrazine hydrate, or N- methylformamide (NMF).104
2.8.2 Inorganic intercalation.
LiCl intercalation, typically in HF/LiCl etched multilayer MXenes, enlarges interlayer spacing and weakens van der Waals forces, enabling delamination via ultrasonication. Ion exchange can embed macromolecular compounds, fine-tuning MXene conductivity.128,129 HCl/LiF etching spontaneously inserts Li+ within multilayer MXene interlayers, weakening interlayer coupling. Delamination into monolayers is achieved through ultrasonic or hand-shaking processes. This method results in stable single-layer MXene colloidal solutions suitable for various applications.80,130
2.8.3 Mechanical delamination.
Mechanical delamination involves applying longitudinal or transverse stress to the layered structure's surface. Despite MXene's strong interlayer interactions, mechanical delamination has been attempted, achieving limited success in producing few-layer MXene nanosheets. Ti2CTx nanosheets on a Si wafer were incorporated by the adhesive tape delamination process.131,132 Though successful delamination was achieved to some extent, it can only yield few-layer MXene nanosheets. In addition, the mechanical process depicts a low-level yield of delamination, which restricts large-scale production.133 Cyclic freezing-assisted approaches have been explored, leveraging freezing water molecules to expand inter-layer spacing and weaken van der Waals forces, facilitating MXene delamination without intercalating agents; however, these experiments are still lab-based, so more work is needed to commercialize it.134
2.9 The storage of prepared MXene and its durability
The storage of MXene has been a matter of debate since its discovery because of its quick degradation due to its easy oxidation in a moisture-based environment. Therefore, these materials are unstable in terms of their active surface and composition. The surface is stabilized by surface functionalities or terminations, but the M atoms at the edges are very unstable due to weak bonding, which enables easy nucleation of outer species, particularly transition metal oxides, when they interact with the oxygen of water or air. Oxidation starts at the edge and spreads throughout the surface of MXene and causes several cracks in the structure by increasing edges. So a colloidal solution of single layer MXene is transformed into TiO2 in oxygen-based environments, and as a result, MXene loses inherent properties such as conductivity and desired reactivity.135 To tackle this key issue, several research studies have been conducted to understand the mechanism of oxidation in MXene and major operating factors, along with experiments on some new and novel processes to avoid this oxidation. Zhang et al. demonstrated the effect of air and temperature on the stability of MXene. In this investigation, it was indicated that Ti3C2Tx MXene was observed to be stable at low temperatures under an argon-based atmosphere, as shown in Fig. 5a. Through this method, the prepared material can stay stable for up to 24 days. Here, the authors also studied the effect of flake size on the extent of oxidation and found that MXene with small flakes was oxidized quickly compared to MXene with large flakes. This revealed that the process of oxidation depended on edges and oxygen interaction. So at low temperatures, there was less chance of nucleation in the flake edge of the metal, and an argon environment protected MXene from oxygen over time.136 Huang et al. conducted a comparative analysis in which they studied the contributions of water and oxygen in the oxidation process separately. It was also observed that the rate of oxidation decreased when MXene was placed in a 2-propanol medium rather than water, even in the presence of oxygen-saturated conditions, and the MXene was stable for up to a week in isopropanol and O2 gas (Fig. 5b). Therefore, it was found that the aqueous medium was responsible for the degradation and oxidation of MXene.137 The surface of MXene in an aqueous medium is negative due to the presence of oxygen terminations on it. However, the edges could have a positive charge.138 Hence, OH ions may aggregate over the edges of MXene with a positive charge under alkaline conditions; this leads to deprotonation of the surface groups and increases surface oxidation.139 It has also been observed that the oxidation process can be slowed down by decreasing the zeta potential in an acidic environment. But one must make sure that the acid is not too concentrated, as this would cause corrosive effects that could aggravate the degradation process due to more exposed edges of the surface. It is vitally important for researchers to understand the mechanism of oxidation of MXene to make more stable and durable MXene for real-time applications.
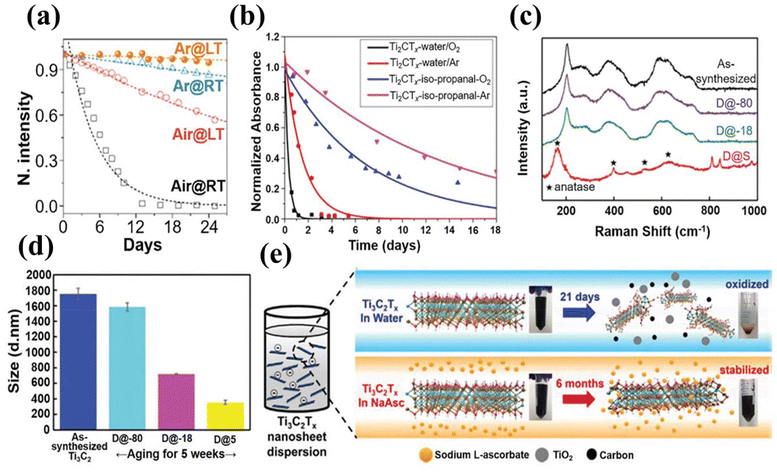 |
| Fig. 5 Antioxidant properties of MXene. (a) The concentration of Ti3C2T nanosheets varied over time under four different conditions. Reproduced with permission from ref. 136. Copyright 2017, American Chemical Society. (b) The stability of the MXene solution over time in four different environments. Reproduced with permission from ref. 137. Copyright 2019 American Chemical Society. (c) Raman spectra and (d) size distribution of MXene flakes after 40 days of aging in distilled water at 5, −18, and −80 °C. Reproduced with permission from ref. 140. Copyright 2019, Royal Society of Chemistry. (e) Two different storage strategies: first, MXene stored in water deteriorated in 21 days and changed its appearance completely, while solution-stored sodium L-ascorbate remained the same with the same appearance and composition even after 6 months. Reproduced with permission from ref. 149. Copyright 2019 Elsevier. | |
Chae et al. prepared a Ti3C2Tx solution and stored it at a very low temperature of about −80 °C; this remained stable for 39 weeks, as depicted in Fig. 5c and d. At that temperature the motion of the molecules became very slow, which resulted in a delay in the oxidation and degradation of stored MXene.140 Razal and coworkers stored delaminated Ti3C2Tx at −20 °C, and the product remained stable for up to 65 days.141 Zhang et al. used different solvent systems to enhance the antioxidant properties and durability of MXenes (Ti3C2Tx, Nb2CTx, Nb4C3Tx and Mo2Ti2C3Tx). Solvents used were N,N-dimethylacetamide (DMAc) and propylene carbonate (PC) for dispersion or storage and DMF for delamination simultaneously. The given method was observed and claimed to be well suited for the abovementioned MXenes due to the better dispersibility of solvents used during the investigations.142 The organic salt type solvent has also played a role in the stability of MXene, as sodium ascorbate was used for the storage of MXene at a concentration of 1 mg per mL. The dispersion and storage have shown the greater stability of MXene at room temperature even for six months, so this result reveals the excellent properties of sodium ascorbate as a preservative for MXene material (Fig. 5e). For future applications of MXene in aqueous systems, it is necessary to regulate surface functionalities to avoid degradation or oxidation, and changes in utilization conditions or storage conditions could be the new route to preserving inherent characteristics of MXene. On the other hand, keeping the temperature low each time is an energy-consuming process and requires a modern instrument. In this regard, Lee et al. demonstrated the electronic properties of MXene and stability could be prevented at the time of heat treatment by using reducing gases.143 It has also been observed that, unlike pure MXene sheets, MXene composites or complexes show good antioxidant behavior. For instance, MXene combined with carbon-based materials144 has increased stability. The insulated carbon coating prevented the surface from exposure to air and water; in this way, MXene remained durable for a long time. Here, carbon-based oxygen containing functionalities decrease the chance of interaction with external oxygen at elevated temperatures.145 Furthermore, Zhang et al. fabricated MXene with a uniform carbon coating that protected it from oxidation when used for lithium and Na-ion-based batteries. In addition, these developed batteries have shown good cycling stability.146 Cui et al. used carbon nanotubes (CNTs) as a covering in Pt/MXene composites to prepare Pt/MXene multistage heterostructures as catalysts for HER-based reactions. The developed materials were capable of remaining in a stable condition in aqueous media for 800 h.147 As compared to carbon materials, MXenes dispersion in ionic liquid (IL), i.e., IL@Ti3C2Tx nanosheets, have shown good performance in avoiding oxidation based on imidazolium salt ionic liquids, which can reduce the species of reactive oxygen.148
3. Role of AI in MXene-based devices
3.1 AI-based sensors
MXene-based sensors have gained significant consideration in the arena of AI because of their unique characteristics and versatility. These sensors are fabricated from 2D MXenes.150 The developed sensors are unique due to their excellent electrical conductivity, more exposed surface area, and tunable chemistry, which makes them a promising agent for several AI-based applications. AI-based sensors are crucial for data acquisition or processing, enabling the fabrication of smarter devices and sophisticated systems.151 MXene-based sensors are advantageous over others as they have the ability to detect a wide range of target analytes selectively with high sensitivity in a short time along with appreciable recovery.152 These sensors have found applications in AI-driven technologies, such as environmental monitoring equipment, healthcare diagnostics, and human–machine interfaces. MXene-based sensors can be integrated into AI systems to detect environmental pollutants, monitor ambient air and water quality, and contribute to smart city development projects. In healthcare, these sensors enable the creation of wearable devices for real-time health monitoring,153,154 providing valuable data for AI algorithms to analyze and predict overall health fluctuations and conditions.
3.1.1 MXene-based image sensors.
The process of vision involves converting light into color-based signals and then into images for brain processing. In this context, MXene, a material with favorable energy band alignment, was used in a perovskite type photodetector array for detecting images. Ren et al. innovatively developed laser-printed MXene/perovskite/MXene image sensors for photodetection.155 A fully monolithic image sensing array containing 1024 pixels was developed to confirm that the 1T1P system was functional and working properly. As for uniform electrical signals, all pixels were developed to set the ground independently with observable Vds and Vgs lines as the foundation of the integrated array (Fig. 6a). The equivalent type circuit diagram of the array is depicted in Fig. 6b. To prevent interruptions between pixels, a controller was used to control 32 channels of Vgs and Vds independently. This has initiated parallel testing of image-based signals with superior speed, as shown in Fig. 6c. The chip containing the image array was placed on a printed circuit board, and optical patterns were struck with a laser at 638 nm using a shadow mask with a transparent “E” pattern.156 This has eliminated the manual testing system by manipulating the photocurrent of each sensor pixel wisely (Fig. 6d); thereby, this auto-detection system was a key improvement for test efficiency. For all 1024 pixels, the coming output current signals showed negligible crosstalk and efficiently detected the resulting shape of the “E” pattern image with high sharpness of the edges and optimizable contrast (Fig. 6e and f). As per available information, this is the most versatile and integrated MXene-containing auto image sensing array, which has proved to be promising for applications in the field of micro-nanoelectronics.157,158
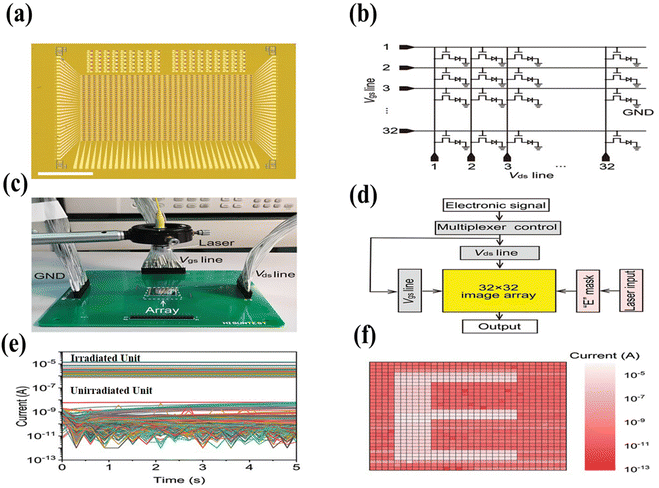 |
| Fig. 6 An array of image sensors. (a) 1024 pixel image based array of sensors for optical imaging (scale bar: 3.25 mm). (b) A circuit diagram of a sensor array with Vgs and Vds lines. (c) The laser and shadow mask on top of the array were used to test the chip mount array on PCB; the ground (GND, i.e., substrate of the chip) and Vds and Vgs lines were connected for the system measurements. (d) A multifunctional measurement system is shown, including a multiplexer control, a semiconductor analyzer in charge of electronic signal input and output (white), a laser control system for light input, and an “E” shaped mask to project “E” using laser illumination (pink). (e) Output current signals for all pixel units during measurement. (f) An array detected a clear “E” image. Reprinted with permission from ref. 155. Copyright 2020 Royal Society of Chemistry. | |
Furthermore, it has also been observed that MXene is compatible with various photosensitive materials, including sulfides and selenides of metals, which help with wavelength regulation. For example, in thermal imaging, the addition of PdSe2 to MXene could enhance the detection of near-infrared radiation.159 Another image sensor made up of a MXene–RAN heterostructure composite containing 32 × 32 pixels exhibited 70% photodetection of transmitted wavelengths, where the RAN polymer acted as a light-sensitive material while MXene layers acted as conductive electrodes. In addition, this sensor has shown robust stability and excellent performance under large bending angles, which proves it to be an ideal material for modern devices.160 The synergy of MXene with these photosensitive materials, like metal halide perovskites, metal chalcogenides, graphene, and semiconducting nanowires, will disclose several applications in the near future.161,162 This change could make them color sensitive image producers and wavelength-selection based photodetectors by controlling the bias voltage.163,164 As we know, the early image sensors were primarily focused on light intensity only, but now enhancements in the charge transport layer are crucial for improving the sensitivity and responsivity in photodetectors, emphasizing the significance of high-quality photosensitive materials in the development of next-generation optoelectronics. Future opportunities lie in advancing color recognition by predicting the wavelength of illuminated light and interconnecting sensors with the brain neural system to create a way for an artificial eye-bearing human.
3.1.2 MXene-based artificial eardrum for hearing.
Human hearing relies on the conversion of air vibrations into neural pulse signals by the auditory system, particularly the eardrum. Various factors, including genetics, aging, and environmental conditions, can lead to hearing loss. Solutions for hearing impairment include the use of hearing aid devices and the restoration of perforated eardrums. Hearing aid devices, incorporating microphones, amplification circuits, and loudspeaker components, have significantly improved the quality of life of individuals with hearing loss. However, challenges such as the bulkiness of rechargeable batteries and extra noise issues necessitate advancements in technology, emphasizing the importance of low-dimensional nanomaterials as alternatives for acoustic membranes in these devices.165,166 Low-dimensional nanomaterials containing MXene-based acoustic devices such as microphones convert regulated frequency forces from sound into electric currents; here, MXene offers a promising alternative for acoustic membranes. It is difficult to tailor or manipulate traditional materials such as mica, isinglass, wood, and large polymers. Hence, the aim of acoustic-based devices is to develop highly sensitive, tunable, low-definition-limit, and long range frequency materials, which may be facilitated by nanostructured materials such as graphene, CNTs, and MXene.167,168 Moving to MXene-based artificial eardrums, Ren et al.168 developed a piezoresistive pressure sensor mimicking eardrums. Fig. 7a shows the detection mechanism of a MXene-based ear drum. A piezoresistive-type sensor is made up of a detection layer, an electrode, and a substrate, demonstrating MXene interlayer spacing in the sensing layer that is much higher than that of graphene, which indicates its higher response under external pressure.169 The developed MXene–PDMS–PE artificial ear drum (Fig. 7b), where MXene was acting as a conductive layer that helped to detect touch and vibrations, while PDMS was used as a substrate and PE, due to its high mechanical properties,170,171 was used as a support for the substrate. Fig. 7c shows the prepared sound sensor with its large flexibility; the artificial ear model is attached to the sensor through an internal acoustic-based eardrum (Fig. 7d). Fig. 7e shows a schematic diagram of the fabrication procedure for a flexible or bendable eardrum device. Fig. 7f shows an SEM image of PDMS containing a pyramid-based array of desirable surfaces. The SEM image shows the uniform incorporation of MXene onto PDMS through the spin coating method (Fig. 5g). The MXene nanoflakes formed an array of conductive networks. The MXene artificial eardrum achieved precise audio wave recording compared to conventional recording devices, and its voice recognition algorithm accurately identified 280 voice or vocal sound signals with an accuracy of up to 96%.
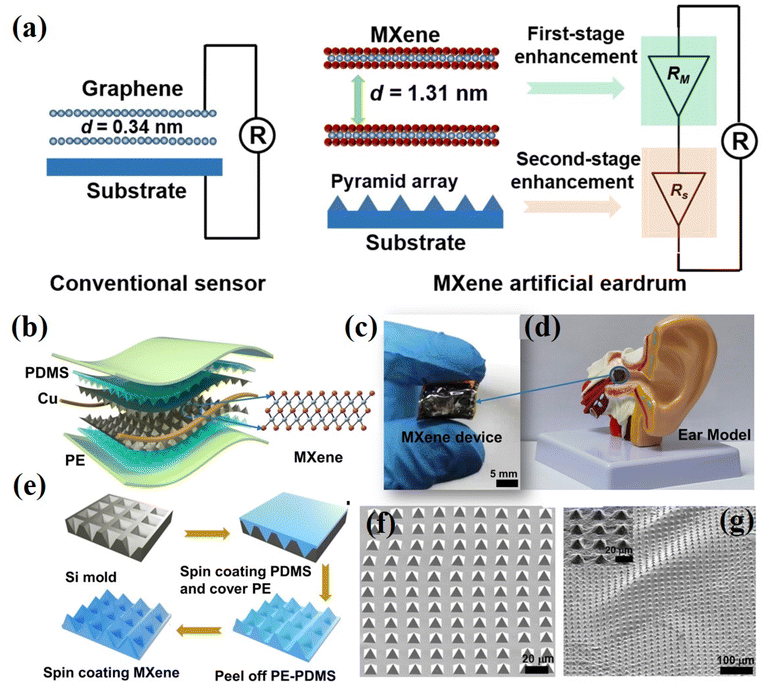 |
| Fig. 7 Two-step amplified artificial eardrum made up of MXene. (a) Operating principle for a conventional two stage enhancement eardrum. (b) MXene based eardrum illustration; two layers of MPP film were used for assembly of the device. (c) A pictorial view of the MXene eardrum. (d) Location of the MXene device on an ear model. (e) Preparation steps for the MXene/PDMS/PE film (MPP). (f) SEM image of the substrate containing PDMS with a pyramid shape. (g) SEM based image covered with MXene nanoflakes. Reprinted with permission from ref. 169. Copyright 2018, American Chemical Society. | |
Additionally, the introduction of an artificial throat based on MXene addresses the need for mimicking human voices, repairing voice loss, and reporting throat-based diseases.172 Ding et al. created an artificial throat from MXene that showed the ability to detect sound as well; it was sensitive to pressure and vibrations and gave perceived responses. Using a convolutional neural network model, it successfully recognized syllables in human pronunciation, broadening the benefits and utilization of MXene in acoustic sensors and its biocompatibility with organisms. Further exploration is required for repairing perforated eardrums using MXene membranes and its potential applications in wearable healthcare devices.173
3.1.3 MXene-based gas sensors.
The progress in gas sensing could play a vital role in the realm of AI, particularly in enhancing olfaction capabilities. The developed MXene-based gas sensing devices have demonstrated significant capabilities for the selective detection of gases such as acetone, toluene, methanol, and humidity.174 Acetone, a biomarker of diabetes, can be efficiently detected using MXene-based gas sensors.175 Wang et al.177 developed a sensor for the detection of acetone by employing a chemiresistor of an α-Fe2O3/MXene heterostructure composite. The fabricated sensor exhibits excellent selectivity for acetone, making it a valuable tool for the early detection of diabetes and for overall health monitoring.176,177 The incorporation of MXene enhances sensor performance through its large specific area and excellent conductivity. The sensing mechanism, driven by hydrogen bonds between MXene and acetone, provides a responsive and selective detection approach. Moving on to methanol detection, MXene-based sensors offer an effective method for detecting this toxic and highly volatile substance derived from biomass waste. In other work, Wang et al. developed a chemoreceptor mechanism-based composite for methanol sensing by using the In2O3/MXene heterostructure.178 The sensor's sensitivity was improved by the interaction of In2O3 with MXene, promoting electron transfer and the subsequent oxidation of methanol. Combining metal oxides with MXene, this method presents a viable option for quick and easy methanol detection.179–181 MXene-based sensors are also instrumental in detecting toluene, a highly toxic solvent used in interior decoration. In connection with this, Salma et al. designed MXene-based sensors with enhanced performance through ultrasound treatment. The MXene based sensor displayed high selectivity for toluene vapors, outperforming other gaseous molecules. The reason is the interaction of the aromatic ring with MXene,182 as well as the unique properties of toluene, which contribute to the sensor's outstanding response.183 Moreover, to monitor the sensor's performance, it is important to consider the humidity factor during experimental investigations. So here, MXene's versatility extends to humidity sensing, an essential aspect of gas detection and for AI applications. Sysoev et al. examined the effects of humidity on MXene based sensing, and found outstanding selectivity with a good limit of detection for H2O sensing.184Fig. 8a shows that the response to humidity is higher than that to other solvents, while Fig. 8b depicts that the response time of the sensor is 1000–2000 s at 10 ppm, while the recovery time observed is 1000 s. Furthermore, by increasing the concentration to an optimum level (10
000 ppm), the response time decreased to 200 s. These results showed that the sensor could be used for a wide range of humidity based applications. Fig. 8c shows that the response of the sensor was compared in a dry and moist environment over a concentration range of 4000 ppm to 10
000 ppm. Furthermore, the response to ammonia gas was also monitored over this range, a decreasing trend from 12% to 8% was seen, and the sensor looked to be insensitive to organic analytes. Fig. 8d shows a plot of three primary LDAs in space. The multi-sensor array was placed in a specific environment, as represented by points. The LDA cluster-surrounded groups were analyzed according to their related groups. A significant drop in chemical resistance as a result of heat fluctuations has also been observed. Fig. 8d represents the signal-to-noise ratio (SNR) plot, which is the best way to optimize the sensitivity and selectivity of any sensor at room temperature. The observed SNR was 70 ± 20 at a humidity of 10 ppm, which was claimed to be 300 times better than others available. The SNR recorded was 2 ± 1 at 100 °C (Fig. 8e). Fig. 8f manifests a comparative measurement of the noise level at room temperature and 100 °C. The MXene-based humidity sensor demonstrated superior performance compared to commercial alternatives. However, concerns about interference by water highlight the need for dry treatment before analyzing target analytes under humid conditions. Moreover, the integration of a multi-sensor array with Mo2CTx MXene as a sensing material opens several avenues for parallel detection of different vapors using multiplexer technology. This advancement holds promise for diverse applications in AI-driven gas sensing systems.
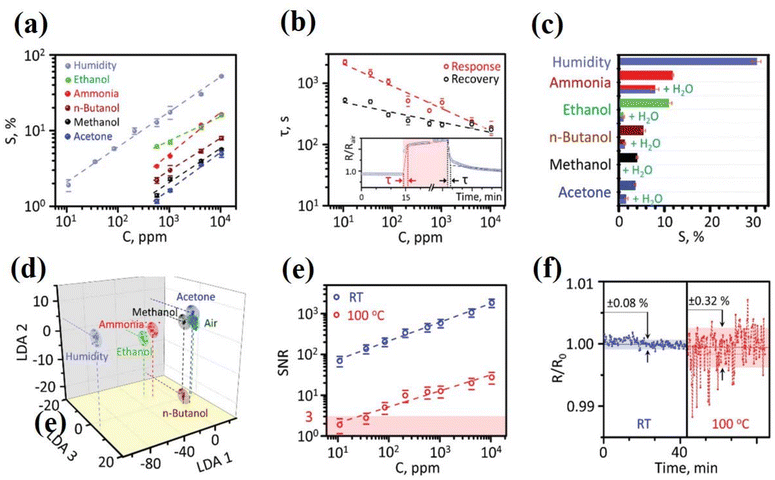 |
| Fig. 8 Performance of the MXene (MoC2Tx) based multisensor prototype with DC bias. (a) Response of the sensor to H2O and other target analytes vs. their concentration. (b) Response and recovery time τ, of the sensor versus concentration of H2O. (c) Comparison of the response to several analytes at the same concentration (4000 ppm). (d) LDA processing of a multisensor signal recorded from the sensor array, a 3D cross-section of primary 1–3 LDA components in the total 6D LDA space; ellipses confine the LDA space around gravity centers related to analytes, all at ≈104 ppm concentration, built by a Gaussian distribution with a confidence probability of 0.75. (e) Signal to noise ratio of HO measured at two different temperatures, room and at 100 °C. (f) The typical resistive noise of an exemplary sensor measured at RT and 100 °C, the values show standard deviations relative to median R0. Data in (a), (b), (c), and (e) are supplied with error bars indicating standard deviations. Reprinted with permission from ref. 184. Copyright 2012 Springer Nature. | |
3.1.4 MXene-based touch sensors.
Pressure sensors are integral to the advancement of AI, particularly in the realm of touch-sense applications. Tactile or physical sensing is facilitated by the skin of an organism sensitized through atmospheric conditions such as temperature, pressure, and humidity, which has given rise to electronic skin (e-skin), also known as pressure sensors. These sensors play a critical role in the development of prosthetic limbs, flexible electronics, and progress in biomedical monitoring, offering sensitivity, stretchability, and light weight properties ideal for wearable and flexible electronics.185 MXenes have emerged as a conductive material, surpassing traditional materials such as InSnO, metallic elements, and 2D materials such as graphene and phosphene.186 MXene incorporation into polymeric composites enhances sensitivity.187–189 The components of these pressure sensors contain sensitive material, a stretchable substrate, and conductive electrodes. There are four types of mechanisms, and on that basis, they work. The first one is piezo-capacitive, the second is piezoresistive, the next one is piezoelectric, and the last one is a triboelectric mechanism. Which mechanism the sensor would follow depends on the functionality and direction given to the touch sensor board. Tung et al. fabricated an e-skin pressure sensor from a MXene/polyacrylamide hydrogel containing heterostructure. In that sensor, the semitransparent and compressible parallel plate stretchable e-skin capacitor was prepared by placing two strips of MXene/PpyNW/VSNP/PAM back to back with patterns on their surfaces, and elastic tape (VHB 49050) was used as an insulator between them.145,190Fig. 9a and b shows the sensor attached to the forearm for sensing touch, dielectrics, and encapsulation. Several types of capacitance were observed, such as sensor capacitance, capacitance based on fringing, capacitance produced by a plate electrode, and capacitance of fringing produced in a medium above the sensor surface. In these touch sensors, the working principle is based on a change in capacitance due to a disturbance in the fringing electric field and a change in the dimensions of the dielectric. For example, when the finger of the forearm of one person is approached by the forearm of another person, a change in electric field begins, resulting in a capacitance decrease in the sensor (Fig. 9c). A rapid linear decline in capacitance was observed when the distance between the approaching finger and the sensor was less than 10 cm. Continuous capacitance changes were demonstrated as a finger repeatedly approached and withdrew from the e-skin sensor. A comparative analysis was also conducted to observe the effect of interfering insulated objects during the experiment, and it was proved that the capacitive mechanism remained ineffective. Fig. 9d depicts the change in capacitance with the movement of the finger toward the sensor and away from the sensor. As observed, when the finger is approaching the sensing area to a distance of 2 cm from it, the sensor shows a decrease in capacitance due to a disturbance in the fringing electric field, and this change is represented by a green color. In another stage where the finger touches the e-skin, an increase in capacitance was seen due to a dimension change (soft pressing). And this condition was represented by a pink color. Furthermore, as the finger moved away from the AI-based touch sensor, the capacitance started to recover to its original value and changed color from pink to white. The difference in approaching pressure, speed, and angle in each cycle can be clearly seen from the asymmetric peaks. These obtained results proved the coexistence of non-touch (proximity) mode and touch mode while e-skin sensing and also opened a new avenue for auto-robotics, realistic perception, and response in AI prosthetics-based applications. Fig. 9e depicts the deformations or distortions in pressure that could cause a change in capacitance due to pressure sensitivity (ΔC/C0ΔP). Sensitivities of 1.82 kPa−1 (<0.2 kPa range) and 6.74 kPa−1 (0.2 to 0.4 kPa range) were measured, surpassing previously reported values. The system demonstrated the ability to detect subtle pressure vibrations from gas flows. Fig. 9f represents the robustness of the e-skin sensor. The graph of capacitance vs. time was obtained by applying a pressure of 40–400 Pa with the help of an electrically insulated tip. The uniform signal without any interruptions in loading and unloading conditions provided continuous stable curves that proved the sensor's capability in real time applications. Fig. 9g and h represents the stability and durability of the sensor measured under pressure cycling. It was claimed that the sensor had long-lasting potential and was stable, with constant response. This sensor, operating with a piezo-capacitive sensing mechanism, exhibits significance in prosthetics or robots, with a natural feel. When substantial strain is applied, the piezoresistive mechanism is activated to operate. The MXene sensor boasts a vast working range, response time, elasticity, and reproducibility, making it suitable for monitoring intricate human motions.
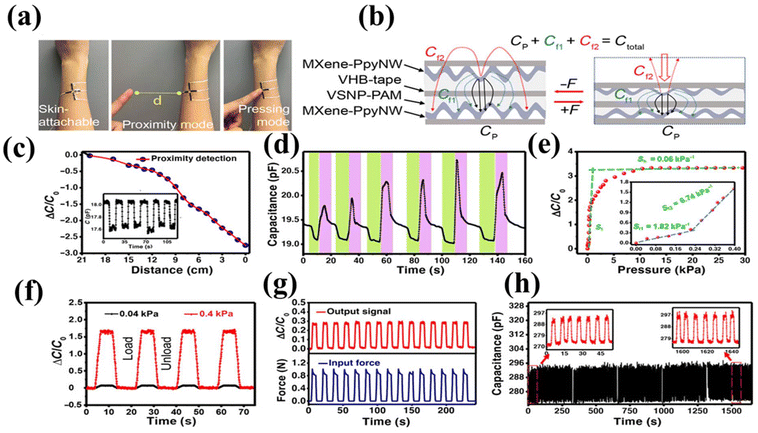 |
| Fig. 9 Capacitive tactile behavior and e-skin mechanism. (a) MXene/PpyNW/VSNP/PAM based stretchable e-skin sensor. (b) The capacitive mechanism of a parallel plate capacitor. (c) Decrease in relative capacitance when a finger repeatedly approaches an e-skin attached sensor. (d) Changes in the colour of e-skin during cycles of finger movement, i.e., near, touching and moving the finger 2 cm away. (e) Change in relative capacitance of e-skin while applying various pressures. (f) Change in capacitance versus time for a tiny pressure sensor (40 Pa) and a tensile sensor for large pressure (400 Pa). (g) Change in response to capacitance and force versus time retention curve. (h) Stability and tactile durability test of an e-skin detector conducted at 1.0 kPa of pressure at a frequency of 0.13 Hz. The changes in current curves were recorded after every 1000 cycles. Here data for 45 cycles are depicted in each record. Reproduced with permission from ref. 191. Copyright 2020 American Association for the Advancement of Science. | |
Moving to piezoresistive sensors, a group of researchers synthesized a stretchable piezoresistive based sensor using MXene–polyacrylonitrile material as a composite membrane. This sensor demonstrated exceptional sensitivity, response/recovery time, and bending cycle endurance.188 Additionally, another piezoresistance based sensor was developed to quantify human motion. This sensor was prepared from MXene and polyimide aerogels by a mixing process and was claimed to be a versatile sensor in the field of wearable devices. Beside this, some other pressure sensors based on piezo-electric potential measurements were prepared from MXene–ferroelectric polymer composites. In these sensors, charge accumulation effects were observed, which increased the piezoelectric potential output while sensing.192 Cai et al. suggested MXene-based electrodes for a triboelectric nanogenerator-based pressure sensor, demonstrating significant sensitivity and cycling test performance.193 The MXene/rGO aerogel-based pressure sensor194 and MXene-based microbridges187 show high sensitivity and potential applications in monitoring the human health status. Biodegradable electronics, incorporating MXene with biodegradable polymers, offer environmentally friendly solutions for implantable clinical applications. MXene's role in energy storage devices, such as batteries and supercapacitors, is promising but requires further technological advancement for mass production and commercial viability. In conclusion, MXene's multifaceted applications in pressure sensors can significantly contribute to the development of AI-driven technologies, providing sensitivity, flexibility, and potential for self-powered electronics. As MXene technology matures, it holds great promise for revolutionizing touch sense and response applications in various fields.
3.1.5 MXene-based actuators.
The development of actuators for artificial reflexes, aimed at stimulating the perception of humans to change and respond to that change, is a crucial aspect in the advancement of bionic robots and neural prosthetics. An actuator assists the organism's perception of heat, electric properties, and light. These AI trained agents convert various signals into a physical response to obtain the desired reflex action.195 These MXene-based actuators hold promise for diverse applications, including artificial prosthetics, service robotics, human–machine interfaces, and smart cities. In the field of artificial prosthetics, the integration of tactile sensors, temperature and humidity sensors, and interfaces with artificial muscles is envisioned. In service robotics, the incorporation of intelligent sensors and actuators is expected to enhance machine vision, speed of sensing, and actuation performances for more human-like interactions. Human–machine interfaces are anticipated to play an important role in the next generation of consumer electronic devices.196–198 Recently, Qiu et al. fabricated MXene/cellulose/polystyrene sulfonic acid (PSSA) composite membranes, which act as soft and smart actuators with multifold uses such as a humidity-based actuator response, humidity-based energy production, and self-powered sensing devices in artificial intelligence appliances along with real-time motion tracking.199 These actuators work based on asymmetric expansion under a moisture concentration difference by converting humidity into a mechanical force,200,201 and in this way the moisture difference or gradient reaction enables the diffusion of protons in a specific direction to produce electricity of high power density in an open type voltage circuit.202 Lee et al. introduced a novel MXene/cellulose bilayer heterostructure for an actuator responsive to near-infrared irradiation.195 The palisade mesophyll expands and shrinks/contracts upon absorption of water, as in a leaf, and its structure is usually stabilized by developing veins. The MXene/cellulose blend is utilized for artificial membrane formation, enabling the sensor to convert photons or light into heat energy for thermal actuation.
This flexible sensor was developed from materials including a nanofiber which has a biocompatible skeleton; the selected structure was similar to the vein skeleton in a leaf. The MXene-based composite with cellulose for activating stratum corneum, where polycarbonate acted as an epidermis or for hygroscopic actuation. These components in a sensor responded to a change in humidity, enabling the properties of shrinking and expansion like in a touch-me-not flower.195 The actuator could deform under continuous usage, so the blending of an actuator is done on the polymer area as to prevent it from deformation because deformation causes a reduction in interlayer spaces. In this process, the MXene layer was observed to be unchanged. The developed actuator was claimed to be responsive to trace amounts of water and near-infrared radiation, which was unique for its application in the fabrication of advanced machines. The shrinking property of the sensor under increasing light intensity was due to the mismatch of volume between the MXene–cellulose based flexible fiber and the polycarbonate membrane in the near infrared.195 Furthermore, programmable behavior was engineered in a rectangular type narrow area, in which MXene–cellulose patterns could be manipulated to three or two column configurations. The MXene-based actuators exhibit periodical bending and recovery under infrared irradiation, mimicking natural motions, including the folding and unfolding of a box and the blooming and closure of MXene–cellulose nanofibers to create bionic flowers. The energy density of the actuators was measured at 0.74 W kg−1 with a power density of 0.92 W kg−1, indicating their potential in applications such as information encryption for displays upon infrared irradiation and intelligent switches for nightlights.195 These MXene-based actuators can be used in brain–machine interfacing, flexible displays, and the Internet of Things. Finally, in the context of smart cities, the utilization of intelligent sensors, innovative construction materials, smart switches based on actuators, and advanced technologies like the Internet of Everything, big data, and cloud computing are intended in the future.196,203,204 The integration of human-like sensors and actuators is poised to drive technological advancements and tap into expansive markets in the future. The combination of MXene-based sensors and AI technology holds great potential for the future, enabling the development of intelligent systems that can adapt, learn, and make decisions based on real-time sensor data. This integration paves the way for innovative AI applications, ranging from advanced robotics to personalized healthcare solutions, ultimately enhancing the overall efficiency and capabilities of artificial intelligence.
3.1.6 MXene-based biosensors.
Biosensors are very important agents in health monitoring throughout the world. MXene containing biosensors have emerged as promising devices for detecting various diseases in living organisms. Starting with Ti2C MXene/graphene, a hybrid FET detector was developed for the monitoring of several viruses including SARS coronavirus-2 and influenza virus. The biosensor demonstrated the ability to sense viruses within a sensing range of 25–250
000 copies per mL. The LOD for H1N1 virus was 125 copies per mL and 1–10 pg mL−1 for recombinant protein 2019-nCoV in the range of 50 ms; a detection limit of 1 fg mL−1 was observed. Furthermore, the sensor was modified for the rapid and selective detection of SARS-CoV-2 nucleocapsid genes. The sensor contained DNA/MXene based chemoresistive material with a LOD of 105 copies per mL for the selective detection of MERS and SARS-CoV-1 from saliva (Fig. 10a–g). Diverse surface terminating functionalities, such as 3-aminopropyl triethoxysilane (APTES) on MXene (Ti3C2Tx), provided binding sites, as a result, the sensor performed excellently for capturing target viruses. Furthermore, the sensing mechanism depends on an antigen–antibody binding process. In this process, during binding, charge compensation causes variations in the drain source current voltage response and these variations are detected by this sensor.205,206
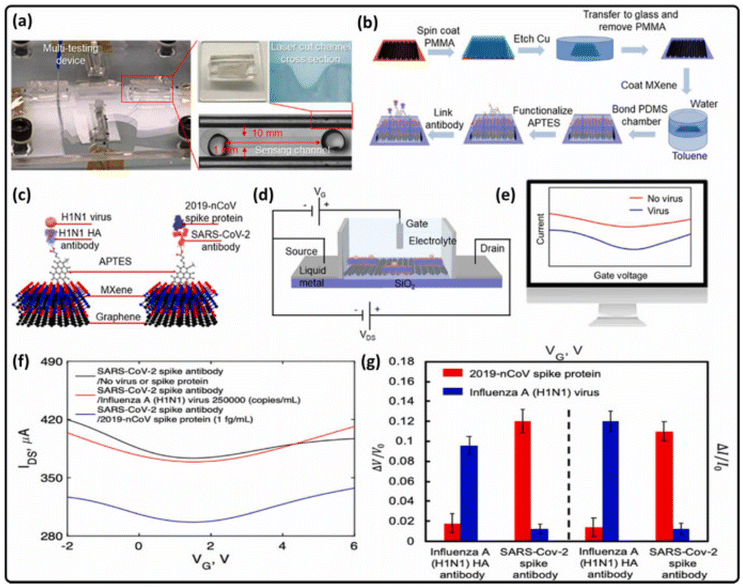 |
| Fig. 10 (a) FET based optical sensor made up of graphene–MXene hybrid material. (b) The hybridization of MXene–graphene by a VSTM deposition process. (c) Related antigen–antibody monitoring mechanism. (d) Scheme of the FET device used. (e) Variations in drain–source features due to interactions with viruses, and (f) characteristics of a FET based biosensor for immobilized SARS-CoV-2 spike antibody biosensing. (g) Normalized drain–source and gate–voltage characteristic variations with STDs in overt binding assessment. Reprinted with permission from ref. 205. Copyright 2021 MDPI. | |
An innovative approach was introduced by Shen and coworkers, who utilized layer-by-layer assembly. In this process, the substance black phosphorus was inserted periodically between layers of MXene. In this way, the deformability was increased by enhancing small interfacial gap contacts and interlayer spacing between MXene nanosheets. The black phosphorus and MXene composites were claimed to be very sensitive and could be used in the preparation of flexible pressure based biosensors. Through this design, the sensor has achieved an elasticity modulus of 0.45 MPa and a sensitivity of up to 77.61 kPa−1. Therefore, there is a strong observation that the incorporation of phosphorus into MXene layers causes modifications to its inherent properties, which results in the in the desirable deformability and outcome of the developed AI sensor. These alterations were strategically employed in the design of a flexible pressure sensor, resulting in enhanced pressure sensitivity.207 Furthermore, Alshareef et al. fabricated a flexible multifunctional biosensor featuring an innovative MXene/Prussian blue composite (Fig. 11a–c), specifically engineered for robust and responsive glucose (35.3 μA mM−1 cm−2) and lactate (11.4 μA mM−1 cm−2) detection in sweat.208 The potential of a multifunctional biosensor patch for real-time sweat monitoring during intense cycling activity was showcased (Fig. 11a–i). A wristband AI-based sweat sensor connected to a portable electrochemical analyzer transmitted real-time data wirelessly to a mobile device. The patch's sweat-take-up layer, collecting sweat for 2 min, facilitated glucose, lactate, and pH measurements. The patch, with replaceable sensors and sweat collection film, demonstrated prolonged usability. As the cycling resistance increased, the effort exerted by the subject intensified, generating sweat and elevating the lactate concentration (Fig. 11f and i). A 3 min cool-down reduced the lactate levels due to sweat dilution and decreased cycling intensity. Sweat pH variations (Fig. 11e and h) correlated with lactic acid excretion, mirroring the expected sweat-lactate/pH profile for escalating workout intensity. Sweat glucose values, corrected for pH effects, aligned with reported blood and sweat glucose levels (Fig. 11d and g). The biosensor's three electrodes exhibited acceptable repeatability. In vitro studies confirmed the biosensor's capability to simultaneously monitor sweat glucose, lactate, and pH, highlighting the MXene-based biosensor's optimized enzyme activity. The three-phase interface design has facilitated oxygen supply to the enzyme electrode, enabling a larger linear detection range and ultrahigh sensitivity to biomarkers.
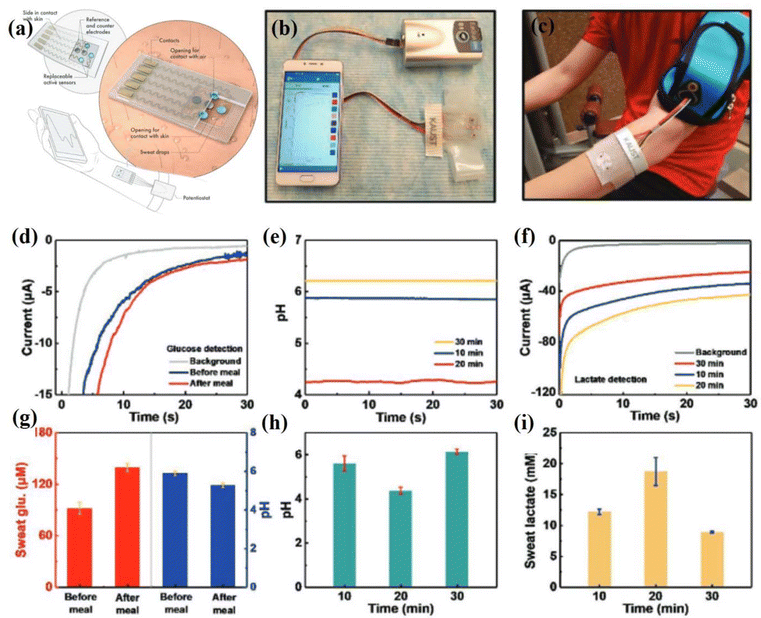 |
| Fig. 11 Real-time detection of body pH, glucose and lactate concentrations with a body-attached MXene based biosensor. (a) Systematic diagram of the sensor system. (b) A wearable sweat monitoring patch sensor is shown connected to a portable analyzer; the signal produced is communicated wirelessly to mobile phones. (c) The MXene-based wearable sensor is attached to the skin. (d) Chronoamperometric responses of three different glucose sensors and pH changes before and after meals. (e) Variation in the pH sensor at different times during exercise. (f) Variation in the lactate sensor at different times while exercising. (g) The graph shows a comparison of glucose and pH levels before and after a meal with three different sensors. (h) A pH comparison at different times with three different pH sensors. (i) Comparison of lactate detected by a lactate sensor at different times. Reprinted with permission from ref. 208. Copyright 2021 John Wiley and Sons. | |
3.2 AI-based energy storage devices
The exceptional properties of MXenes, such as high conductivity, substantial surface area, and robust electrochemical characteristics, make them attractive candidates to fabricate energy storage devices, i.e. supercapacitors and batteries. In the context of AI, where real-time processing and instantaneous energy availability are paramount, MXene's prowess is particularly valuable. Supercapacitors featuring MXene electrodes enable quick bursts of power, crucial for AI applications requiring immediate and intense computational capabilities. Moreover, high flexibility and good mechanical resilience of MXene make it ideal for integration into wearable AI devices, offering light weight and durable energy solutions for portable applications. The advent of MXene in printable electronics further revolutionizes the fabrication of energy storage components, allowing for tailored and flexible designs that cater to the specific power needs of AI systems. These combinations of knowledge serve as a crucial resource for researchers, engineers, and practitioners seeking to propel AI technology forward by harnessing the formidable capabilities of MXene-based energy storage devices. MXene could have several applications, for example, it could be incorporated into the batteries of smartphones, enhancing their energy density and overall battery performance. MXene-based wearable devices may find applications in smart watches and fitness trackers. Furthermore, MXene based materials can also have applications in the Internet of Things, such as cameras, and smart home devices to ensure long-lasting and efficient power sources. MXene has potential for high-frequency applications; it could be integrated into energy storage systems for high-performance computing devices, contributing to improved energy efficiency and rapid energy supply for AI processing. In addition, various portable electronic devices such as portable speakers, power banks, and handheld gaming consoles could benefit from MXene-based energy storage solutions, providing users with longer usage times between charges.
3.2.1 Lithium-ion batteries (LIBs).
LIBs containing a graphite anode have dominated in the commercial market of sustainable energy storage devices. However, the increasing demand for energy storage has highlighted the inadequacy of graphite anodes at meeting future needs. Consequently, the quest for novel anode materials for LIBs has become imperative. The inherent property of pristine MXene is electronic conductivity, which can be manipulated by band gap tuning and surface functionalization. It has shown semiconducting behavior with a tunable conductivity band gap.209 Naguib et al. used Ti3C2 as an anode in LIBs and evaluated its performance computationally and experimentally.209 Tang et al. studied the mechanism of diffusion, movement and adsorption of Li ions on the surface of MXene and their effects on surface termination. In addition, they found that MXene could act as a promising anode material for LIBs and was operable at low voltage with fewer diffusion barriers.210 This research is also supported by literature.211 Xie et al. proposed O-terminated MXene, having the highest capacity, supported by classic MD simulations, to investigate the dynamic charge storage mechanism of Ti3C2(OH)2 electrodes.212,213 Mashtalir et al. produced Ti3C2Tx MXene “paper” with a high capacity of 410 mA h g−1 at a rate of 1 C.214 Sun et al. investigated Ti3C2 intercalated with DMSO, achieving a capacity of 123.6 mA h g−1 at 260 mA g−1.215 Kim et al. suggested the application of MXene for high-areal-capacity electrodes, achieving 5.9 mA h cm−2 at 1.5 mA cm−1 after 50 cycles.216 Different methods were used to increase the electrochemical efficiency and performance of MXene, such as delaminating the multilayers into a single paper like structure,214 introducing small pores into the flakes,217 and protecting MXene against structural deformation and degradation.218 Mashtalir et al. synthesized Nb2CTx MXene and amine delaminated MXene; to increase the interlayer spacing, CNTs were applied, as shown in Fig. 12a.219 The synthesized composites of Nb2CTx/CNT were used as a paper electrode. The developed electrode has a high capacity rate of over 400 mA h g−1 at 0.5 °C, as shown in Fig. 12b. Halim et al. developed a “paper electrode” from Mo2CTx–CNT and evaluated its electrochemical performance at high rates. At a rate of 400 mA g−1, the reversible capacity achieved was 560 mA g−1 and after 1000 cycles; stable capacities of 250 and 75 mA g−1 at high rates of 5–10 A g−1 were obtained. So the obtained results indicated that the developed material was very encouraging for high power and voltage based batteries used in AI devices.220 The integration of AI devices is poised to revolutionize the optimization of MXene-based electrodes for LIBs. AI techniques facilitate the prediction of electrochemical behavior under various conditions, offering a more comprehensive understanding of material performance. Furthermore, AI minimizes the need for resource-intensive testing, accelerating the development and deployment of advanced energy storage solutions.221 The collaborative synergy between materials science and AI technologies propels the evolution of efficient and sustainable energy storage systems.
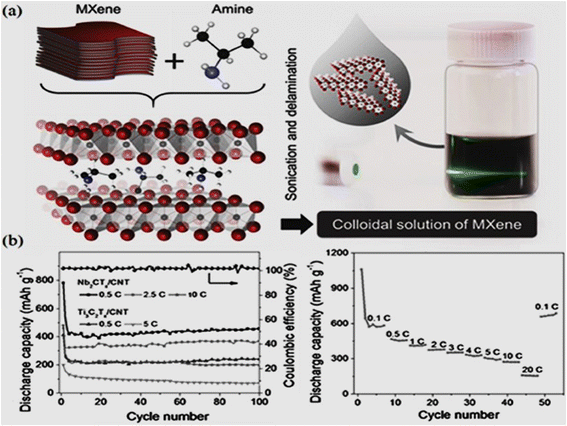 |
| Fig. 12 (a) Schematic representation of the delamination process of Nb2CTx (MXene); (b) cycling stability and rate performance of Nb2CTx/CNT paper electrodes. Reprinted with permission from ref. 222. Copyright John Wiley and Sons. | |
3.2.2 Lithium–sulfur (Li–S) batteries.
The main attraction of Li–S batteries is their exceptional energy,223 but practical applications are impeded by several challenges, including an insulation issue for both sulfur and the discharge product Li2S. In addition, the shuttle effect also decreases the efficiency and stability of batteries. The shuttle effect is produced due to the transfer of intermediate species and being dissolved in organic electrolyte, as a result, Li–S forming at the anode causes a low columbic efficiency and poor recycling capability. To tackle this issue, it is necessary to use a host material that has high conductivity and active surface area. For this purpose, MXene based materials are an excellent candidate due to their inherent properties. Let us start the discussion with work by Liang et al. who examined MXene (Ti2Cx) as a host material for the cathodes of Li–S batteries. During that process, sulfur reacted with OH ions on the surface of MXene nanosheets constituting a composite containing 70 wt% of S and MXene. After 100 cycles, the developed S/Ti2C composite showed 960 mA h g−1 capacity and a current density up to 335 mA g−1 with prominent capacity retention of 80% even after 400 cycles at 838 mA h g−1.224 Further insights into the surface reactivity of Ti2C, Ti3C2, and Ti3CN with polysulfide species were obtained through XPS and DFT computations.225 To enhance conductivity and prevent restacking, CNTs were combined with MXene, which resulted in an outstanding performance in terms of stability and long term cyclability for up to 1200 cycles. Furthermore, Bao et al. synthesized a Ti3C2Tix/rGO composite and used it as a host material for the abovementioned batteries. This developed composite efficiently trapped S and polysulfide with a high capacity of 1144 mA h g−1 along with retention of 878 mA h g−1 after 300 cycles.226 Zhao et al. examined the potential capabilities of MXenes as hosts for polysulfide materials in LiS batteries with the help of DFT calculations. In these investigations, they revealed that oxygen terminated MXene Ti2C–O2 and Ti3C2–O2 proved to be a good protective layer and observed an outstanding binding capacity with polysulfide. Hence, it was claimed that the MXene based material mitigated shuttle effects as well.227
MXene could also influence the performance of batteries beyond their usage as a host molecule only. Rao et al. suggested that coulombic interactions between the polysulfide and MXene played a dominant role in most cases during the binding process. Therefore, this would extend the applications and utilization of this magical material in energy storage devices. Beyond cathode hosting, MXene found application in functionalizing separators for Li–S batteries, leading to superior cyclability and reversibility in simple S/C black composite cathodes.228 The integration of AI devices stands to further enhance the optimization of MXene-based materials for Li–S batteries. AI techniques enable precise predictions of electrochemical behavior, offering valuable insights into material performance under varying conditions. This collaboration between materials science and AI technologies accelerates the development of efficient and sustainable energy storage solutions.229
3.2.3 Other battery systems.
The increasing demand for large storage systems to effectively harness renewable resources for grid stations has become significant in today's energy landscape. The abundance of lithium ions in the Earth's crust is 20 ppm, which cannot reach the energy requirements of today's world.230 Therefore, it is crucial to explore alternative materials to lithium for large scale energy production and for the development of non-lithium ion batteries (NLIBs).231 Xie et al. delved into the storage capabilities of MXene sheets for sodium (Na), potassium (K), magnesium (Mg), calcium (Ca), and aluminum (Al) through density functional theory (DFT) computations.232 Their findings revealed that bare MXene exhibited higher capacity and ion mobility than O-terminated MXene. Yu et al. further postulated that expanded interlayer bare and functionalized Ti3C2 MXene could significantly reduce sodium diffusion barriers, enhancing storage capacity.233 The mechanism of Na ion intercalation in MXene sheets was examined by Kajiyama and coworkers, who demonstrated that due to penetration of desolvated Na ions into MXene layers along with solvent resulted in an expansion of the interlayer distance from 9.7 to 12.4 Å, in a process known as sodiation (Fig. 13a). Furthermore, Fig. 13b illustrates that the sodium ion acts as a pillar and solvent penetrates to expand the interlayer spacing during sodiation and desodiation processes.234 Similar types of investigation were also conducted for other MXenes such as V2CTx and the mechanism of sodium ion intercalation was also examined and found to be similar.235
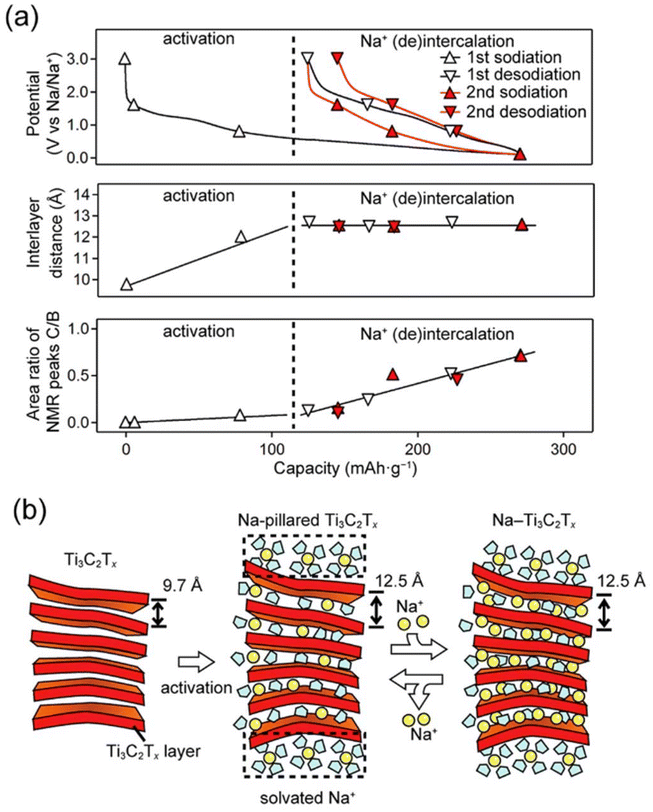 |
| Fig. 13 (a) Schematic illustration of interlayer distance and amount of Na ions intercalated during the first two cycles in an energy storage appliance; (b) mechanism of Na ion intercalation into MXene sheets. Reprinted with permission from ref. 234. Copyright 2016 American Chemical Society. | |
The prevention of restacking of MXene sheets was addressed by Xie et al., who found that through the electrostatic incorporation of CNTs, restacking could be efficiently avoided and led to an enhanced capacity of 345 mA h cm−3 at 100 mA g−1 after 500 cycles.236 Zhao et al. developed a 3D hollow macroporous MXene film that showed high capacitance.237 Wu et al. synthesized a layered structure of MoS2/Ti3C2Tx nanosheets containing a capacity of 251 mA h g−1 at 100 mA g−1 after 100 cycles along with good stability.238 Guo et al. utilized a solution-phase method to fabricate Sb2O3/Ti3C2Tx composites for SIBs, where Sb2O3 served as a sodium ion reservoir, and Ti3C2Tx provided pathways for electron and sodium ion transport.126 The integration of AI devices holds immense potential for advancing the optimization of MXene-based materials for NLIBs. AI techniques enable accurate predictions of electrochemical behavior, offering invaluable insights into material performance under diverse conditions. This collaboration between materials science and AI technologies expedites the development of efficient and sustainable energy storage solutions.238
3.2.4 Supercapacitors.
Supercapacitors and their advancement are the focal point of modern AI based research. Supercapacitors are versatile due to their rapid charge/discharge rate, higher power density and long life cycle. These are used for start–stop auto controlled AI based devices, hybrid energy systems and in fuel cell based vehicles. There are two important kinds of supercapacitors: EDLCs and pseudo-capacitors. The problem with these materials is their variable and non-sustainable energy density. So it is necessary to explore alternative materials that would be able to tackle this issue. In connection to this, MXene was chosen as a modifier because of its unique properties such as high conductivity and large active surface area. It has been selected as a promising candidate for advanced supercapacitors.239,240 Lukatskaya et al. studied the intercalation of several ions into MXene layers such as Na+, K+, NH4+, Mg2+ and Al3+ spontaneously.241 The paper electrode type MXene Ti3C2Tx was present in an alkaline solution of KOH, which was free of binder, and displayed a volumetric capacitance of 340 F cm−3 at 2 mV s−1. In addition, it was examined for 10
000 cycles at 1 A g−1 and showed no signs of degradation. Due to its pseudocapacitive behavior in sulfuric acid, the abovementioned MXene was explored as an excellent material for supercapacitors.242 Hu et al. disclosed the capacitive mechanism of sulfate based aqueous electrolytes with the help of Raman spectroscopy; their investigation revealed a pseudo-capacitance mechanism for acidic medium electrolytes and an electric double layer mechanism for other electrolytes.243 Ghidiu et al. developed clay type Ti3C2Tx and used it as a supercapacitor electrode in a H2SO4 containing aqueous system. The fabricated electrode achieved a capacitance of 900 F cm−3 at 2 MV s−1.79
An optimistic approach is needed to manipulate interlayer spacing during etching and surface redox processes can increase the performance of MXene based electrodes to the desired level. In addition, the self-assembled MXene based films can be synthesized by dropping mild baked suspension of Ti3C2Tx flakes. Such MXene showed excellent gravimetric capacitance of 499 F g−1 and high cyclic life.242 Xu et al. fabricated binder free MXene (Ti3C2Tx) by modifying electrophoretic deposition. This modified transparent and conductive electrode was employed as a solid state supercapacitor where it provided a volumetric capacitance of 676 F cm−3 along with rapid responses.244 The exceptional performance shown by MXene electrodes could play a vital role in AI based energy storing devices.136 Incorporating AI devices into the optimization of MXene-based supercapacitors holds substantial promise for advancing energy storage solutions. AI techniques enable precise predictions of electrochemical behavior, offering insights into material performance across diverse conditions.245 The collaboration between materials science and AI technologies accelerates the development of efficient and sustainable energy storage systems. Li et al. synthesized Ti3C2Tx with intercalated and surface group modifications, denoted as 400-KOH-Ti3C2.246 Compared to pristine Ti3C2Tx electrodes, the 400-KOH Ti3C2 electrode demonstrated a substantial improvement up to 211% in the original gravimetric capacity 517 F g−1 at 1 A g−1 even after 10
000 cycles in 1 M H2SO4, as shown in Fig. 14a–d. The prepared electrode showed an excellent cycling stability of more than 99%. This high performance was attributed to an enhancement in the interlayer spacing between MXene nanosheet layers and a low concentration of terminated surface groups.219
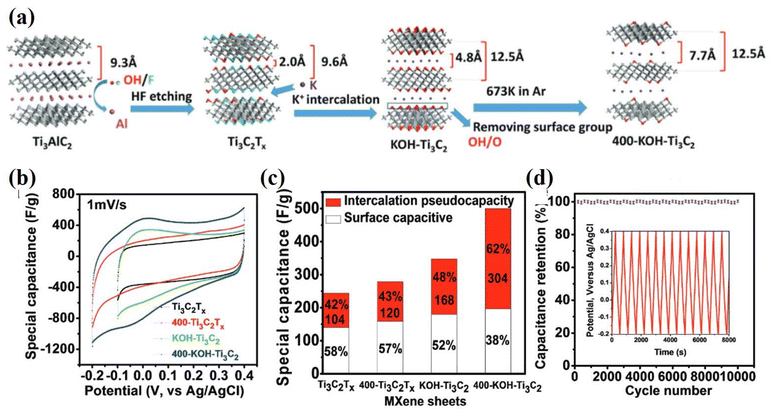 |
| Fig. 14 (a) Synthesis scheme for modified MXene; (b) cycle voltammetry profiles at 1 mV s−1 in 1 M H2SO4; (c) a comparative study of capacitance; (d) capacitance retention tests for the 400-KOH-Ti3C2 electrode in 1 M H2SO4. Inset: galvanostatic cycling data collected at 1 A g−1. Reprinted with permission from ref. 246. Copyright 2017 John Wiley and Sons. | |
Hydrazine intercalation into Ti3C2Tx was proposed by Mashtalir and coworkers and they claimed that the additional material had reduced F and OH groups and as a result the surface chemistry of the material changed. The hydrazine intercalated MXene displayed an improvement in the capacitance up to 215 F g−1 at 5 A g−1 in 1 M sulfuric acid electrolytic solution. Furthermore, there was no degradation observed after 10
000 cycles.219 Ghidiu et al. immersed Ti-based MXene in lithium chloride salt solution with the help of vacuum filtration. The lithium treated MXene exhibited a volumetric capacitance of 892 F cm−3 with long cycling durability. The obtained performance was also due to the expansion of interlayer spacing by Li-ion intercalation and an ion exchange process with trimethyl alkylammonium cations.247 Wen et al. also suggested improving the capacitance of pure MXene by the incorporation of N-atoms because N-doping could enhance the c-lattice properties of the material; this resulted in an improvement in capacitance up to 192 F g−1 surpassing those of undoped MXene materials.248 Research on MXene-based supercapacitor electrodes was extended to composite materials. Ling et al. developed Ti3C2Tx/polymer composite films and used them in KOH electrolytes. They produced a high performance in terms of capacitance and stability.249 Boota et al. synthesized a composite by combining polypyrrole (Ppy), the conductive polymer, with Ti3C2Tx based MXene, which resulted in an enhanced capacitance up to 1000 F cm−3 with 92% retention of capacitance even after 25
000 cycles. These appreciable results were obtained due to mixing of conductive polymers, which increased the storage capacity of the device along with stability.250 Xue et al. synthesized a free standing hybrid membrane by combining a similar Ppy conductivity based polymer with Ti3C2Tx, as a result, a MXene based membrane was developed, which was used in electrochemical devices as a capacitor electrode. These free standing membranes are crucial for the development of flexible electronics in the AI field.251 Furthermore, other conventional materials were also added to MXene to improve the device performance. Boota et al. added organic type intercalator polyfluorene derivatives to Ti3C2Tx to investigate the capability of the developed hybrid material for energy storage appliances.252 To understand the real applications of MXene in energy storage devices, electrolyte manipulation and optimization are essential. Lin et al. used ionic liquids, such as 1-ethyl-3-methylimidazolium bis(trifluoromethylsulfonyl) imide (EMI-TFSI), as electrolytes in a MXene supercapacitor with a voltage window of 3 V. These neat ionic liquid/MXene based supercapacitors were examined by in situ XRD and their performance was evaluated along with their electrochemical characteristics.253 These ionic liquid/MXene based supercapacitors were examined by in situ XRD and their performance was evaluated along with their electrochemical characteristics.254 Likewise, MXene also has applications in AI based solid state supercapacitors. Peng et al. developed an on-chip inter-digital solid state microcapacitor from Ti3C2Tx. This contained two layers of MXene of different optimized sizes. The fabricated supercapacitor exhibited excellent volumetric and areal capacitances of 356 F cm−3 and 27 mF cm−2 at 20 mV s−1 respectively. It retained a capacitance of 100% after 10
000 cycles at 50 mV s−1. The obtained response displayed the outstanding sustainability of micro-supercapacitors.255 Li et al. used MXene and graphene to engineer an on-chip super-microcapacitor for AI based energy consuming systems. In that process, graphene was electrochemically exfoliated and combined with MXene nanosheets, resulting in a flexible on-chip capacitor. The measurements showed areal and volumetric capacitances of 2.26 mF cm−2 and 33 F cm−3 at 5 mV s−1 respectively.256 In recent applications of Ti2CTx, wire-type supercapacitors were successfully fabricated, as evidenced by the achievement of a high specific length capacitance (3.09 mF cm−1) and a notable length energy density (210 nWh cm−1) alongside commendable cycling stability.257 Complementary to experimental findings, first-principles computations were employed to explore the impact of surface-terminated groups of MXene on electrochemical performance, and it was observed that the MXene with an O-terminated surface had potential pseudo-capacitive properties.258 Furthermore, the use of MXene in this technology should be studied from every angle to know the exact location of MXene in the arena of energy storage. Along the same lines, another advancement was achieved by adding CTAB–Sn(IV) to pillared MXene through a liquid phase method, resulting in a sophisticated electrode for hybrid capacitors. During that process, the CTAB–Sn(IV)@Ti3C2 pillared composite was used as an anode and activated carbon was used as a cathode. The developed supercapacitors displayed a high energy density of 45.3 W h kg−1 at a power density of 10.8 kW kg−1 and desirable lower ESR (effective series resistance).259 Dall'Agnese et al. tried to use V2CTx as a cathode electrode for Na ion-based capacitors and explored the electrochemical characteristics in their experiments. The assembly of a fuel cell and an anode of hard carbon showed a capacitance of 50 mA h g−1 at a maximum of 3.5 V.260 The additional use of MXene in a hybrid capacitor was investigated by Byeon and coworkers, who utilized a composite of MXene and carbon nanotubes (Nb2CTx/CNT) as a cathode for a Li ion based hybrid capacitor and evaluated the potential applications of MXene in hybrid capacitors.261 These advancements underscore the versatility of MXene-based materials in diverse electrochemical applications, ranging from supercapacitors to hybrid capacitors. The exploration of surface-terminated groups and the incorporation of innovative preparation techniques highlight the continuous efforts to enhance the electrochemical performance of MXene. As the field progresses, these findings contribute to the growing body of knowledge in the application of artificial intelligence.
3.3 AI-based MXene memristors
MXene-based memristors have attracted significant attention for their potential applications in AI due to their unique properties, such as desirable semiconductor characteristics, including thermal and electrical conductivity, as well as energy storage capabilities.262–265 MXene-based memristors, known for their excellent capability of data processing and biological simulation computing,266–268 have become a focal point for researchers exploring MXene materials in neuromorphic computing. MXene-based memristor devices have been investigated for their ability to emulate several biological functions/synapses and neurons, such as long-term plasticity (LTP), short-term plasticity (STP), and peak-related plasticity (STDP).269 The unique stability, durability, and low power consumption of these devices make them promising candidates for applications in neuromorphic intelligence.270–272
3.3.1 Ti3C2 MXene-based memristors.
Khot et al. developed three distinct memristors based on MXene (Ti3C2) materials i.e., silver (Ag)/MXene (Ti3C2)/platinum (Pt), Pt/MXene (Ti3C2)/Pt, and aluminum (Al)/MXene (Ti3C2)/Pt,273 as illustrated in Fig. 15a. These memristors demonstrated reproducible resistive switching (RS) behavior and properties related to non-volatile memory-based devices. The devices also exhibited synaptic properties, including potential-inhibitory, short-term plasticity-based antisymmetric Hebbian learning rules and symmetric Hebbian rules. Notably, the Al/Ti3C2/Pt memristor, when subjected to a pulse program having a 10 ns pulse width, displayed continuous modulation of resistance. This device successfully converts STP into LTP within a nanosecond pulse sequence, showcasing its potential for ultrafast synapses in a biological system and efficient neural network training (Fig. 15e and f).274 These three memristors followed the conductive mechanism, which was supported by Khot and coworkers in their investigation. For the Ag/MXene/Pt memristor, the transition between HRS and LRS resulted from the redox reaction of Ag and the movement of oxygen vacancies (Fig. 15b). The Pt/Ti3C2/Pt memristor, on the other hand, relied on the formation and rupture of oxygen vacancy filaments (Fig. 15c). Similarly, the Al/Ti3C2/Pt memristor also features the formation and fracture of oxygen vacancy filaments, with the additional presence of an extremely thin oxidized AlOx layer in the Al top electrode (Fig. 15d). Yan et al.274 investigated the conductive mechanism of the Al/Ti3C2/Pt memristor and found that the resistance of this memristor could be continuously modulated, allowing for efficient STP to LTP conversion.275
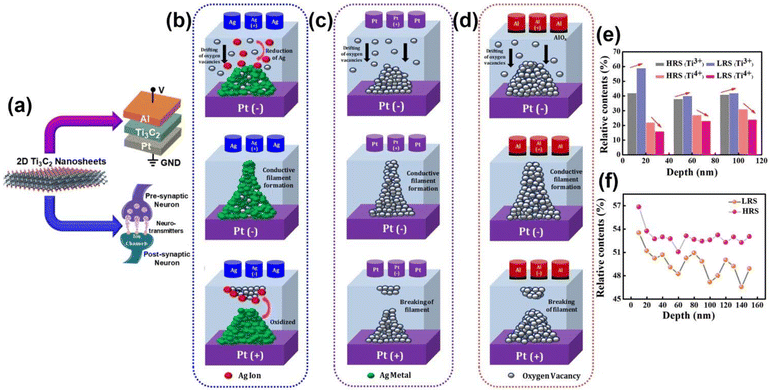 |
| Fig. 15 (a) Structural illustration of the Al/Ti3C2/Pt (MXene) memristor and its synaptic simulation structure. Reprinted with permission from ref. 71. Copyright 2016 John Wiley and Sons. (b) The conductive mechanism of the Ag/Ti3C2/Pt memristor, (c) the Pt/Ti3C2/Pt memristor, and (d) the Al/Ti3C2/Pt memristor. Reprinted with permission from ref. 273. Copyright 2021 American Chemical Society. (e) Relative contents of Ti3+ and Ti4+ in the functional layer of the Al/Ti3C2/Pt memristor at different depths, and (f) oxygen contents of the HRS and the LRS at different depths in the Al/Ti3C2/Pt memristor. Reprinted with permission from ref. 274. Copyright 2019 John Wiley and Sons. | |
Chen et al. fabricated a Cu/MXene (Ti3C2)/Cu-based memristor with a particular two-directional threshold switching (TS) behavior.276 This developed device effectively simulated the integration of leakage, robotic threshold-driven fire, and regeneration or auto-recovery function of neurons. The working mechanism involved the oxidation of Cu atoms and their injection in the functional layers of MXene to produce conductive filaments, which would break upon withdrawal of the applied voltage. Additionally, by utilizing a single layer of Ti3C2 MXene, researchers explored the effect of the deposition of multilayers for the preparation of different memristors. Later, Shen et al. developed an Ag/Ti3C2/Ti3C2/indium tin oxide (ITO) memristor with bipolar RS behavior,277 demonstrating excellent long term increment and depreciation (LTP/LTD) behavior. Doping was also investigated as a means to regulate the performance of MXene-based traditional memristors. Wang et al. fabricated an Al/MXene (Ti3C2):Ag/Pt-based memristor, which was obtained by doping Ag nanoparticles over the MXene layer, resulting in bidirectional continuous current transition behavior.278 Yu et al. developed an artificial neuron device by adding Ag nanoparticles to an insulated substrate such as PVA or ITO along with MXene (Ti3C2), which showed a low operation voltage.279 This memristor-based artificial optoelectronic hybrid-integrated neuron was observed to be very specific and delivered high recognition accuracy in a visual sensitivity and perception system that helped to simulate a human conditional response.
3.3.2 V2C MXene-based memristors.
Wang et al. prepared an Ag/MXene (V2C)/tungsten (W) memristor on a SiO2/silicon substrate wafer (Fig. 16a).280,281 The as-prepared memristor exhibited typical resistive switching and threshold switching behaviors. Moreover, the Ag/V2C/W memristor demonstrated the capability to sensitize several biological synaptic functions such as paired-pulse facilitation, excitatory postsynaptic current, pike rate dependent plasticity, and short-term plasticity. Fig. 16b and c illustrates the design of artificial neurons based on the leaky integrated-and-fire method without using an auxiliary circuit. Several neural features have been achieved including leaky integration, self-relaxation, and threshold-driven fire. The developed material followed a conductive type mechanism for the Ag/V2C/W memristor, as shown in Fig. 16d. The Ag atom was oxidized to the Ag ion and migrated to the MXene functional layer upon applying a forward voltage. A conductive filament (CF) was gradually formed with the storage of Ag ions. Conversely, while applying a reverse voltage, the CF broke down, and the developed device returned to the HRS. This research suggested that the Ag/V2C/W memristor could act as a promising candidate for the development of efficient neuromorphic-based computing circuits for SNNs. Most studies on MXene-based memristors have concentrated on V2C MXene and Ti3C2 MXene, and some researchers have also compared their performance with other sensors as well. Chen et al. fabricated Ag/V2C/W and Ag/Ti3C2/W memristor devices; they evaluated their electrical performance such as device-to-device and cycle-to-cycle variation, retentions, and ON/OFF ratios.282 The results indicated that both memristors exhibited favorable non-volatile memory-type properties. Additionally, this study delved into the diffusion coefficient and conductivities of Ag ions in the V2C or Ti3C2 layers using DFT theory. The calculations revealed that V2C MXene had more stability in its atomic structure while adding Ag ions resulted in a higher conductivity and diffusion coefficient for the prepared memristor.
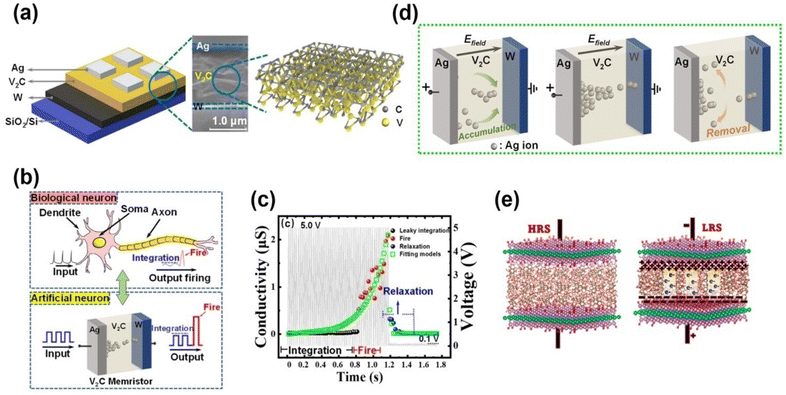 |
| Fig. 16 (a) Structural diagram of the Ag/V2C/W memristor. (b) An artificial synaptic neuron achieved with the Ag/V2C/W memristor. (c) A fitting model of the LIF neuron implemented by the Ag/V2C/W memristor. (d) The formation and fracture process of CFs in the Ag/V2C/W memristor. (e) The conductive mechanism of the Mo2TiC2/GO/Mo2TiC2 memristor in the HRS and the LRS. Reprinted with permission from ref. 283. Copyright 2022 Elsevier. | |
3.3.3 Other MXene memristors.
Besides, V2C MXene and Ti3C2-based memristors, limited research has been conducted on other MXenes for memristor applications. Wang et al. conducted first principles calculations based on DFT to explore the behavior related to the conductance of a copper/MXene (Cr2C)/TiN device.284 The results of the calculations indicated that the Cu/Cr2C/TiN device exhibited a low programmed voltage, fast switching speed, and greater stability, with more durability compared to the traditional Ag/Ti3C2/Pt devices available. This improvement has resulted in easy manipulation of the activation energy of the Cu/Cr2C/TiN device. In that study, MXene served as the middle layer and was sandwiched between the top and bottom electrodes in these memristors. Fatima et al. fabricated the Mo2TiC2/GO/Mo2TiC2 memristor and utilized a double transition metal MXene as a top/bottom electrode, which exhibited bipolar type resistive switching and showed high endurance over 5000 cycles.283 The conduction type mechanism of the Mo2TiC2/GO/Mo2TiC2 device is shown in Fig. 16e. Initially, the upper and lower electrodes were separated by an insulating GO layer, as a result, the high resistance state was created, which blocked these charges from flowing between the two metal electrodes. Through the bias method, the defects in the GO membrane produced oxygen vacancies, aligned in the direction of the applied electric field. Due to this arrangement, the oxygen ion moved to the metal electrode and was oxidized at the interface. This movement of oxygen ions produced CFs, allowing the charge carrier to move between these metal electrodes and switching the specific device from a high-resistance state to a low resistance state. In this way, fluctuations in the device during the experiment were clearly observed.
3.3.4 MXene/oxide-based composite memristors.
In the field of neuromorphic intelligence, pure MXene shows excellent properties for the development of memristors; this has generated significant interest among many researchers. Additionally, MXene mixed with other composite structural materials is another area of focus for research. The application of MXene/oxide-based memristors in the area of neuromorphic intelligence is detailed in this section. The main design of MXene/oxide-based memristors consists of five layers: the substrate layer, the bottom metal electrode, an oxide layer, the active layer of MXene, and the top metal electrode, as shown in Fig. 17.285–287
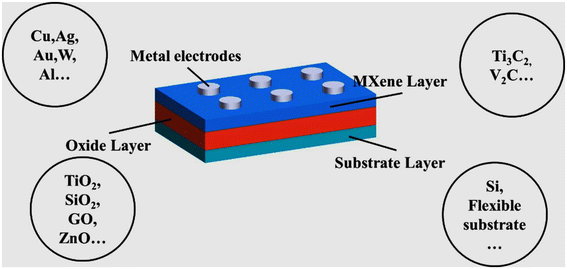 |
| Fig. 17 Basic block diagram of the MXene/oxide-based memristors. Reproduced with permission from ref. 288. Copyright 2023 Taylor & Francis. | |
3.3.4.1 MXene/SiO2-based memristor.
MXene plays a significant role in the manufacture of memristors because of the abundant functional groups on the surface that can capture charges.289,290 Recently, research revealed that another additional MXene layer on top of the metal oxide layer had the potential to control the resistance transition inside memristors, lower their consumption of energy, and make them ideal for use in neuromorphic computing applications. Lian et al. identified the memristor structure developed from MXene/SiO2, and their team were pioneers of that exploration. The Cu/MXene/SiO2/W devices exhibited superior endurance as well as lower stable operating voltages and had stable multilevel resistance states compared to traditional Cu/SiO2/W devices under the same conditions. Fig. 18a and b demonstrates the features of comparative RS. The development of low-power and highly stable neuromorphic devices could be achieved with the help of this study.291 Moreover, Wan et al. demonstrated the potential of uncontrolled networks of learning utilizing the memristor MXene/SiO2 structure. The solid function for hardware-based neuromorphic systems in memristors have been introduced through this work.292 Additionally, MINIST digits hand-written in the Ag/MXene/SiO2/Pt memristors were recognized by Lian and coworkers.293 They integrated the device into the algorithm framework with the introduction of conductance in the device by adding synaptic weights, which produced 77.39% recognition accuracy. These advancements highlight the promising prospects of memristors based on MXene/SiO2 within the domain of neuromorphic computing.292,293
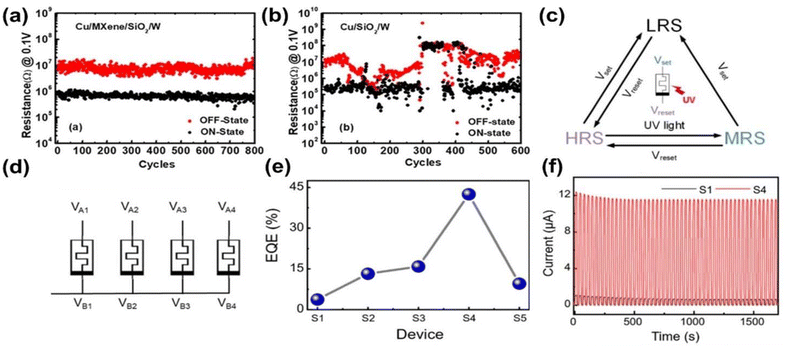 |
| Fig. 18 (a) ON/OFF resistance state in 800 cycles for the Cu–MXene–SiO2–W composite at 0.1 V, and (b) the Cu–SiO2–W device and its ON/OFF graph for 600 cycles measured at same voltage of 0.1. Reprinted with permission from ref. 291. Copyright 2019 AIP publishing. (c) Several operating input factors were applied to the Ag/MXene/TiO2 based memristor under different conditions and fluctuations are depicted, and (d) a 4-bit binary comparator constructed from the Ag/MXene/TiO2 device. Reprinted with permission from ref. 294. Copyright 2021 American Chemical Society. (e) The external quantum efficiency (EQE) of each ZnO QD/MXene nanoflake photodetector with different MXene nanoflake contents. The MXene contents in S1 to S5 were 0, 0.005, 0.01, 0.1, and 0.3 mg mL−1, and (f) performance comparison for devices S1 and S4 for 50 cycles. Reprinted with permission from ref. 295. Copyright 2021 American Chemical Society. | |
3.3.4.2 MXene/TiO2-based memristors.
The optoelectronic memristor was developed using nanosheets of MXene/TiO2 composite and silver nanoparticles (Ag NPs).294Fig. 16c illustrates that by optimizing UV rays and pulse voltage, the transition into various resistance states, excellent repeatability, and durability of this device were genuinely demonstrated. Additionally, by using the distinct RS features of the device, the author developed a binary comparator, which was 4-bit (Fig. 18d). Similarly, the comparator in that study utilized fewer devices compared to conventional digital comparators and proved to be adaptable and more suitable for AI-based devices. Based on these results, the use of 2D MXene materials to advance memristor technology in digital logic circuits and optoelectronic memory has been evaluated and has potential for the future. He et al. prepared a device based on Ag/MXene (V2C)/TiO2/W for the application of electronic synchips.296 The device demonstrated both volatile TS behavior and non-volatile RS behavior inside a single device by varying the compliance current size. Furthermore, the study successfully reproduced LTD and LTP behaviors through progressive conductance modulation under pulse stimulation. These outcomes underscore the potential of MXene/TiO2 memristors in simulating synaptic orientation.
3.3.4.3 MXene/ZnO-based memristors.
Yang et al. studied ZnO quantum dot films being consistently mixed with varying ratios of 2D MXene nanosheets to develop a simple nanosheet photodetector from ZnO quantum dots (QDs) and MXene.239Fig. 18e shows that by varying MXene nanoflakes, the nanosheets of ZnO QD/MXene demonstrated remarkably enhanced exterior quantum efficiency compared to the control ZnO QD photodetector. Moreover, the long-term stability of a nanosheet photodetector made of ZnO QD/MXene is represented in Fig. 18f. The present study offers a practical approach for the fabrication of high-performance ultraviolet photoelectrical components for both military and civilian applications, especially in low-light scenarios.223 Furthermore, the MXene/ZnO memristor, which exhibited humidity perception, preprocessing, and visual data perception functions, was designed by Wang and coworkers. The as-fabricated MXene/ZnO memristor has a potential applications as a multimodal sensor to sense data, suppress/filter noise, and specialize features (Fig. 19a and b). As shown in Fig. 19c and d, the results of image identification of an artificial retina sensing system are presented, exhibiting the MXene/ZnO memristor's ability to significantly reduce background noise and emphasize intrinsic features under varying humidity conditions.297
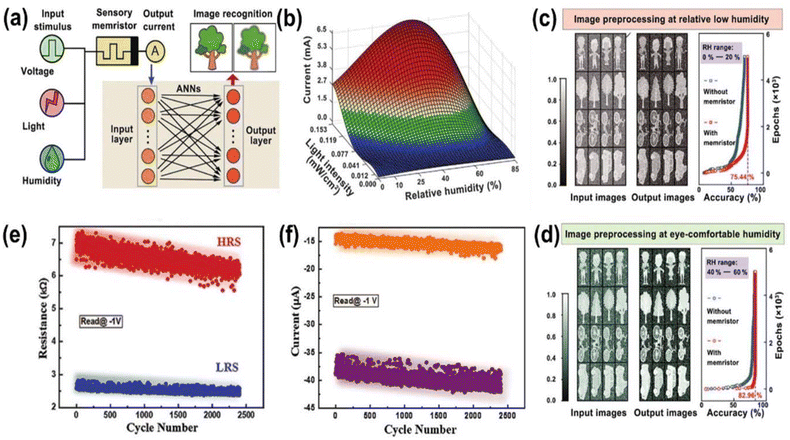 |
| Fig. 19 (a) Schematic diagram of a multimodal MXene/ZnO memristor for sensing information, suppressing/filtering noise, and specialized features. (b) The current is a function of relative humidity and light in the MXene/ZnO memristor. (c) Relative low humidity conditions and (d) eye-comfortable humidity conditions on the contrast enhancement and deionizing of the artificial retina sensing system. Reprinted with permission from ref. 297. Copyright 2021 John Wiley and Sons. (e) The M/GO/M device resistance versus several cycles at a read voltage of −1 V, and (f) the M/GO/M device current reliability check at a read voltage of −1 V. Reprinted with permission from ref. 298. Copyright 2022 John Wiley and Sons. | |
3.3.4.4 MXene/GO structured memristors.
Photoelectric devices made up of materials such as 2D carbon have revealed excellent resilience and flexibility. Fatima and coworkers prepared a memristor based on the MXene/GO/MXene paper (M/GO/M) structure.298Fig. 19e and f depicts the variation of current and schematic resistance of the M/GO/M device over 2400 cycles. Notably, in both the LRS and the HRS, the device displayed very steady resistance levels. This study establishes a strong basis for the manufacture of non-volatile memory devices and high-performance flexible devices.
3.3.4.5 Resistive switching mechanism of MXene/oxide-based memristors.
Despite the positive applications of memristors made of MXene/oxide, a deeper understanding of their working mechanisms is essential.299–301 By using first-principles calculations and physical characterization of the MXene/SiO2 structure, Lian et al. clarified the function of the MXene/SiO2 structure in SiO2-based memristors. The CFs further readily grow with nanostructures of MXene, as well as improving the device performance by lowering the CF uncertainty in Cu/MXene/SiO2/W devices, as shown in Fig. 20a and b.291 In addition, Wang et al. also analyzed thin films of MXene/ZnO to contrast the chemical states in both the LRS and HRS. The Ti 2p nuclear-level spectral scans concerning the HRS and LRS, both before and after a voltage pulse, are illustrated in Fig. 20e and f. The significant change in signal intensity of TiO2 2p suggested alterations in O2− distribution. In the LRS, the reduction in the intensity of the TiO2 signals was observed, and in the HRS, a high TiO2 2p intensity indicated an accumulation of O2− near the electrode. Furthermore, a graphic representation of the development of CF in both the LRS and HRS is shown in Fig. 20e and f. Fig. 20c and d presents the XPS measurements showing the low depth of the RS layer, as indicated by the dashed lines.297
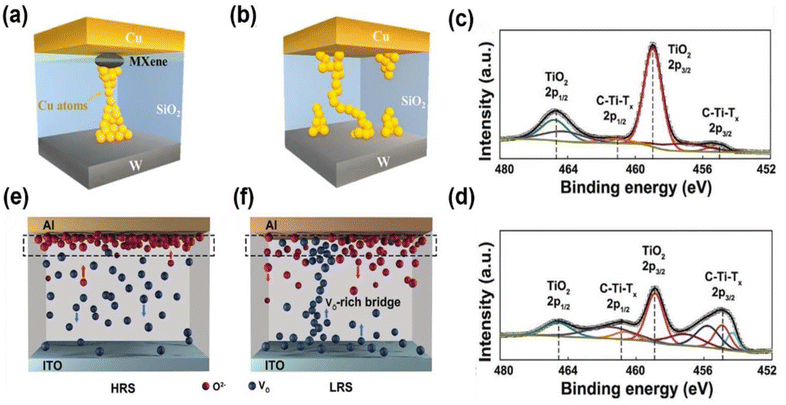 |
| Fig. 20 (a) The CFs are more likely to grow along the location of MXene, which reduces the randomness of CFs in Cu/MXene/SiO2/W memristors. Reprinted with permission from ref. 291. Copyright 2019 AIP Publisher. (b) The formation of CFs in Cu/SiO2/W devices is relatively disordered and unsystematic. Reprinted with permission from ref. 291. Ti 2p XPS spectra of the MXene/ZnO layer with fitted peaks in (c) the HRS and (d) the LRS states. Proposed schematic illustrations of the O2− spatial distribution in the MXene/ZnO memristor that contribute to the resistance state of (e) the HRS and (f) the LRS. The dashed lines correspond to the shallow depth of the resistive layer for XPS survey measurements in (c) and (d). Reprinted with permission from ref. 297. Copyright 2021 John Wiley and Sons. | |
3.3.4.6 MXene/PZT-based memristors.
In comparison with traditional memristors, ferroelectric memristors stand out as promising devices for application in biological artificial synaptic and neural components.302 Their simple structure, lower power consumption, modifiable resistance states, and high-speed switching make them highly suitable for these applications.303,304 Yet, ferroelectric type memristors encounter challenges such as variable unstable working voltages, being less durable, and a poor switching ratio. The unstable formation and fracture in a conductive wire existing in old-style ferroelectric memristor devices hinders the effective simulation of biological synaptic functions. Adding another functional layer to modify or adjust the interfacial barrier proved to be a sustainable strategy for optimizing and evaluating the electrical properties of ferroelectric memristor-based AI devices. Zhang et al. identified that MXene could specifically mitigate the sudden creation of a conducting wire in a PZT (PbZr0.52Ti0.48O3) ferroelectric AI memristor device. Compared to the Cu/PZT/Pt device, the Cu/Ti3C2/PZT/Pt MXene-based AI memory device showed a greater retention behavior (>3000 s), a greater switching ratio (106), and a lower switching voltage (1.5 V). It is crucial to express here that the above-mentioned MXene based memristor was successfully implemented with some key natural features induced artificially, such as biological synaptic neural function, which included long-term potential, paired pulse facilitation, long-term depreciation, and spike time-dependent plasticity. This has all been done without any further components or circuits. Furthermore, it was also observed during the recognition of MNIST handwritten values and digits that the developed material showed that the learning accuracy of the hardware-based neuromorphic network reached up to 96% (Fig. 21a).305 These findings have created hope for the construction of a very efficient neuromorphic and neural computing system.306,307
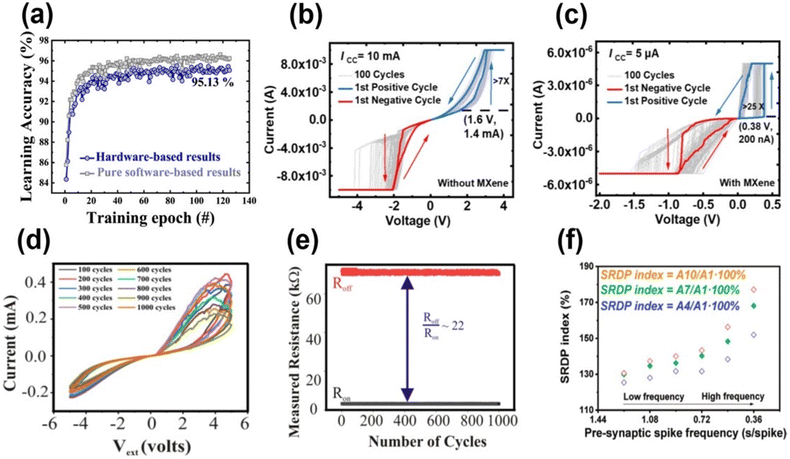 |
| Fig. 21 (a) Comparison of learning accuracy based on hardware and software for the Cu/Ti3C2/PZT/Pt memristor. Reprinted with permission from ref. 300. Copyright 2022 Elsevier. Typical I–V characteristics of (b) the Ag/GST/Pt memristor and (c) the Ag/MXene/GST/Pt memristor. Reprinted with permission from ref. 301. Copyright 2023 IOP Publisher. (d) An endurance test of 1000 cycles for the Cu/SnS/Ti3C2/Cu memristor. Reprinted with permission from ref. 314. Copyright 2022 IEEE. (e) The I–V characteristics of the Cu/SnS/Ti3C2/Cu memristor for 1000 cycles. (f) In the relatively high-frequency range, the Au/LPE/Ti3C2/Si memristor synapses have a higher filtering gain than some of the perovskite synapses. Reprinted with permission from ref. 315. Copyright 2021 John Wiley and Sons. | |
3.3.4.7 MXene/GST-based memristors.
Chalcogenide compounds, such as Ge2Sb2Te5 (GST), have gained widespread use in phase-change RAM (PCRAM) due to their rapid and reversible switching between an amorphous state and a crystalline state produced by laser pulses and current.308,309 However, traditionally used GST-PCRAMs suffer from limitations of high programming current, more power consumption, and comparatively slower transition speed.310,311 To address these issues, Lian et al. developed a very low power-consuming artificial neuron device from Ag/MXene/GST/Pt composite material that showed excellent electrical properties, such as an operational voltage of 0.38 V and a current of 200 nA, along with a very steep slope (103) in the comparative analysis, as depicted in Fig. 21b and c. The device made up of Ag/MXene/GST/Pt has advantages over a simple Ag/GST/Pt device in terms of current, as the former showed 10 mA of current, which was higher than that of 5 μA for Ag/GST/Pt. This higher performance was due to the addition of the MXene layer, leading to the set voltage being optimized at 0.38 and the current being 200 nA, which resulted in a significant reduction in power consumption. Moreover, experimental verification demonstrated that the functionalization of integrated and firing neurons was important for SNNs.301 The above findings have shown that these (Ag/MXene/GST/Pt) materials can be used practically for the development of artificial neuromorphic devices.
3.3.4.8 MXene/SnS-based memristors.
An emerging class of transition metal chalcogenides (SnS) and their combination with MXene resulted in effective resistance transition characteristics for the development of AI devices,312,313 Recently, Saha et al. designed an innovative artificial memory device from a Cu/SnS/Ti3C2/Cu MXene-based composite that showed typical I–V characteristics and OFF/ON ratio of the fabricated device over 1000 cycles (Fig. 21d and e). These results highlighted the stability, durability, and endurance of the designed Cu/SnS/Ti3C2/Cu memristor.106 In addition, low cost, easy preparation and desirable power consumption made the material a promising alternative in the IC field for AI devices. Therefore, progressive development may replace expensive multi-vibrators soon.314
3.3.4.9 MXene/LPE-based memristors.
Li-based MXene devices offer advantages in several ion transportation synaptic devices. Because of their usage, the device will use less power, contain multiple storage states, and have a stable performance. The combination of LPE/MXene enables various synaptic functions, such as dendritic integration and artificial memory improvement. Wei et al. prepared an Au/LPE/Ti3C2/Si memristor, and its simulated artificial synapse performance was investigated. By using its electrochemical behavior like insertion–extraction and adsorption–desorption, this memristor has shown replicated memory enrichment, Pavlovian learning, latent plasticity, and logic manipulation. Furthermore, the Au/LPE/Ti3C2/Si MXene-based device showed low energy consumption and greater sensitivity, and thus was well suited for its application in modern devices. In dendritic integration and memory enhancement devices, if this developed memristor is compared to a traditional perovskite synapse, it has a higher filtering gain at a higher frequency, as depicted in Fig. 21f.315 This study has introduced a novel approach for manufacturing and designing ultrasensitive artificial neural systems that will help with compatible perception monitoring and manipulations through modern memristor devices.314–316
In summary, this section provides an in-depth exploration of various 2D MXene-based memristors and their applications in modern neuromorphic artificial intelligence devices. The integration and intercalation of pure MXene with other functionalities, such as MXene/PZT, MXene/oxides, and MXene/GST memristors, has been carefully examined with a focus on their applications for neuromorphic intelligence. Furthermore, a systematic analysis of the probable mechanism operating in these memristor devices offers valuable insights for future research in the field of MXene-related memristor devices and their current applications in perception-sensitizing devices as well as in neuromorphic intelligence. Comparatively, 2D MXene-based memristors exhibit several advantages over traditional memristors, such as low energy consumption, small size, high integration capability, and greater flexibility.317,318 Additionally, compound-type structures formed by layering MXene with other functional materials demonstrated reduced power consumption and increased reliability compared to memristors with separate functional layers. Despite these advantages, some challenges persist in the widespread application of 2D materials in neuromorphic systems, particularly in achieving large-scale preparation of neuromorphic devices based on 2D MXene materials. Addressing these challenges will be crucial for advancing the field and realizing the full potential of MXene-based memristors in neuromorphic intelligence applications.
4. Challenges and future directions
MXene-based materials offer tremendous potential for advancing AI technologies, particularly in the realms of sensors, energy storage, and memristors. However, several challenges must be addressed to fully harness their capabilities. In the context of sensors, complexities in material integration and the need for enhanced selectivity present significant hurdles. For energy storage, cycling stability issues demand attention to ensure prolonged and reliable performance. Meanwhile, programming challenges associated with MXene-based memristors pose obstacles to seamless integration into AI architectures. Looking forward, future directions in research involve advancing synthesis techniques to make MXene more accessible, tailoring material properties to enhance performance characteristics, and innovating programming methods for memristors. These efforts aim to overcome existing challenges and pave the way for MXene-based materials to play a pivotal role in shaping the future of AI technology.
In conclusion, the synthesis of MXenes and their applications in AI-based technologies, specifically in MXene-based sensors, energy storage, and memristors, represent a transformative frontier with immense potential. The challenges identified in the integration of MXene materials into these applications, ranging from material compatibility to selectivity and cycling stability, underscore the need for continuing research and innovation in this area. Addressing these challenges will be pivotal in unlocking the full capabilities of MXene-based materials, making them indispensable in the evolution of AI technology. Looking forward, the future directions outlined, including advancements in synthesis techniques, tailoring material properties, and refining programming methods, offer a roadmap for researchers to navigate toward more efficient and effective use of MXene in AI applications. As we bridge the gap between synthesis and application, MXene stands poised to revolutionize AI technology, contributing to more robust, sensitive, and sustainable sensors, energy storage devices, and memristors that will shape the landscape of artificial intelligence in the years to come.
Data availability
No primary research results, software or code have been included and no new data were generated or analysed as part of this review.
Conflicts of interest
The authors proclaim that they have no competing interests or personal relationship with anyone that could influence the work reported in this review paper.
Acknowledgements
The authors acknowledge a grant from the Higher Education Commission (HEC) of Pakistan (Project No. 20-14470/NRPU/R&D/HEC/2021), Indigenous Ph.D. Fellowship 5000, phase-II-Batch-VI, and the State Key Laboratory of Mesoscience and Engineering, Institute of Process Engineering, Chinese Academy of Sciences (MESO-23-A06) for financial support of this work.
References
- V. Chaudhary, A. Kaushik, H. Furukawa and A. Khosla, ECS Sens. Plus, 2022, 1, 013601 CrossRef CAS.
- H. S. Shin, S. B. Choi and J.-W. Kim, Mater. Today Adv., 2023, 20, 100426 CrossRef.
- Q. Jiang, Y. Lei, H. Liang, K. Xi, C. Xia and H. N. Alshareef, Energy Storage Mater., 2020, 27, 78–95 CrossRef.
- A. A. Jamali, M. I. Vohra, A. Ali, A. Nadeem, S. M. Attia, A. Hyder, A. A. Memon, F. K. Mahar, R. B. Mahar and J. Yang, Phys. Chem. Chem. Phys., 2024, 26, 16369–16377 RSC.
- J. A. Buledi, A. R. Solangi, H. Ali, A. Ali, A. Mallah, S. Amin, A. A. Memon, K. H. Thebo, J. Yang and M. Kazi, New J. Chem., 2024, 48, 6201–6210 RSC.
- A. G. Chandio, A. W. Memon, N. A. Nahyoon, S. I. Memon, A. A. Memon, N. Mahar, M. Khilji, A. Hyder, M. Kazi and K. H. Thebo, Diamond Relat. Mater., 2024, 148, 111398 CrossRef CAS.
- J. A. Buledi, A. Hyder, A. Ali, A. R. Solangi, A. Mallah, S. Amin, A. A. Memon, K. H. Thebo and M. Kazi, J. Phys. Chem. Solids, 2024, 192, 112083 CrossRef CAS.
- L. Wang, M. Zhang, B. Yang, J. Tan, X. Ding and W. Li, Small Methods, 2021, 5, 2100409 CrossRef CAS PubMed.
- S.-J. Choi and I.-D. Kim, Electron. Mater. Lett., 2018, 14, 221–260 CrossRef CAS.
- C. Anichini, W. Czepa, D. Pakulski, A. Aliprandi, A. Ciesielski and P. Samorì, Chem. Soc. Rev., 2018, 47, 4860–4908 RSC.
- F. A. Janjhi, I. Chandio, D. Janwery, A. A. Memon, K. H. Thebo, G. Boczkaj, V. Vatanpour and R. Castro-Muñoz, Chem. Eng. Res. Des., 2023, 199, 327–347 CrossRef CAS.
- A. Hyder, A. Ali, A. Khalid, A. Nadeem, M. A. Khan, A. W. Memon, A. A. Memon, D. Janwery, M. Mehdi and A. Solangi, Ind. Eng. Chem. Res., 2023, 62, 21335–21346 CrossRef CAS.
- T. H. Banglani, I. Chandio, A. Ali, A. A. Memon, J. Yang, M. Kazi and K. H. Thebo, Environ. Sci.: Water Res. Technol., 2024, 10, 1061–1096 RSC.
- M.-U.-N. Khilji, A. A. Otho, R. Memon, A. Khalid, M. Kazi, A. Hyder, D. Janwery, N. A. Nahyoon, A. A. Memon and N. Memon, Anal. Lett., 2024, 57, 2067–2084 CrossRef CAS.
- A. Hyder, A. Ali, J. A. Buledi, A. A. Memon, M. Iqbal, T. H. Bangalni, A. R. Solangi, K. H. Thebo and J. Akhtar, Chem. Rec., 2024, 24, e202400006 CrossRef CAS PubMed.
- M. K. Shahzad, F. H. Memon, F. Soomro, M. Iqbal, A. Ibrar, A. A. Memon, J. H. Lim, K. H. Choi and K. H. Thebo, J. Environ. Chem. Eng., 2023, 11, 109329 CrossRef CAS.
- A. Basit, A. A. Jamali, F. A. Junejo, R. Larik, S. K. Mahar, A. Sameeu, F. K. Mahar and A. Hyder, Diamond Relat. Mater., 2024, 142, 110839 CrossRef CAS.
- A. Hyder, S. S. Memon, J. A. Buledi, S. Memon, Z.-u.-A. Memon, S. G. Shaikh and D. B. Rajpar, Chem. Pap., 2023, 77, 7737–7748 CrossRef CAS.
- W. Huang, L. Hu, Y. Tang, Z. Xie and H. Zhang, Adv. Funct. Mater., 2020, 30, 2005223 CrossRef CAS.
- S. Yang, C. Jiang and S.-h. Wei, Appl. Phys. Rev., 2017, 4, 021304 Search PubMed.
- D. H. Ho, Y. Y. Choi, S. B. Jo, J. M. Myoung and J. H. Cho, Adv. Mater., 2021, 33, 2005846 CrossRef CAS PubMed.
- S. Mehdi Aghaei, A. Aasi and B. Panchapakesan, ACS Omega, 2021, 6, 2450–2461 CrossRef CAS PubMed.
- M. Kaleem Shabbir, F. Arif, H. Asghar, S. Irum Memon, U. Khanum, J. Akhtar, A. Ali, Z. Ramzan, A. Aziz and A. A. Memon, Chem. Rec., 2024, e202400047 CrossRef CAS PubMed.
-
J. A. Buledi, A. Hyder, N. H. Khand, S. A. Memon, M. Batool and A. R. Solangi, in Handbook of Functionalized Nanostructured MXenes: Synthetic Strategies and Applications from Energy to Environment Sustainability, Springer, 2023, pp. 283–300 Search PubMed.
- Y. Wang, R. Xiao, N. Xiao, Z. Wang, L. Chen, Y. Wen and P. Li, Adv. Electron. Mater., 2022, 8, 2200370 CrossRef CAS.
- J. Yuan, Y. Li, M. Wang, X. Huang, T. Zhang, K.-H. Xue, J. Yuan, J. Ou-Yang, X. Yang, X. Miao and B. Zhu, InfoMat, 2024, 6, e12528 CrossRef CAS.
- L. Liu, M. Bi, Y. Wang, J. Liu, X. Jiang, Z. Xu and X. Zhang, Nanoscale, 2021, 13, 19352–19366 RSC.
- M. Li, Z. Wan, T. Zou, Z. Shen, M. Li, C. Wang and X. Xiao, Chem. Eng. J., 2024, 492, 152417 CrossRef CAS.
- D. Lei, N. Liu, T. Su, L. Wang, J. Su, Z. Zhang and Y. Gao, APL. Mater., 2020, 8(11), 110702 CrossRef CAS.
- Y.-Z. Zhang, K. H. Lee, D. H. Anjum, R. Sougrat, Q. Jiang, H. Kim and H. N. Alshareef, Sci. Adv., 2018, 4, eaat0098 CrossRef PubMed.
-
A. Ali, A. A. Memon, J. Yang, J. Akhtar and K. H. Thebo, Novel Materials and Water Purification: Towards a Sustainable Future, 2024, pp. 49–65 Search PubMed.
- I. Mahar, F. K. Mahar, N. Mahar, A. A. Memon, A. A. A. Pirzado, Z. Khatri, K. H. Thebo and A. Ali, Chem. Eng. Res. Des., 2023, 191, 462–471 CrossRef CAS.
- I. Mahar, F. H. Memon, J.-W. Lee, K. H. Kim, R. Ahmed, F. Soomro, F. Rehman, A. A. Memon, K.
H. Thebo and K. H. Choi, Membranes, 2021, 11, 869 CrossRef CAS PubMed.
- H. Ali, A. Ali, J. A. Buledi, A. A. Memon, A. R. Solangi, J. Yang and K. H. Thebo, Mater. Chem. Front., 2023, 7, 5519–5544 RSC.
- Y. Pei, X. Zhang, Z. Hui, J. Zhou, X. Huang, G. Sun and W. Huang, ACS Nano, 2021, 15, 3996–4017 CrossRef CAS PubMed.
-
S. Neethirajan, Human-Centric Intelligent Systems, 2023, pp. 1–16 Search PubMed.
- L. Zheng, M. Cao, Y. Du, Q. Liu, M. Y. Emran, A. K. Yousef, M. Sun, C. Ma and M. Zhou, Nanoscale, 2024, 16, 44–60 RSC.
- Q. Li, X. Zhi, Y. Xia, S. Han, W. Guo, M. Li and X. Wang, ACS Appl. Mater. Interfaces, 2023, 15, 19435–19446 CrossRef CAS PubMed.
- A. Rosenkranz, M. C. Righi, A. V. Sumant, B. Anasori and V. N. Mochalin, Adv. Mater., 2023, 35, 2207757 CrossRef CAS PubMed.
- Z. Xiao, X. Xiao, L. B. Kong, H. Dong, X. Li, B. He, S. Ruan, J. Zhai, K. Zhou and Q. Huang, Int. J. Extreme Manuf., 2024, 6, 022006 CrossRef.
- I. Hussain, U. Sajjad, O. J. Kewate, U. Amara, F. Bibi, A. Hanan, D. Potphode, M. Ahmad, M. S. Javed and P. Rosaiah, Mater. Today Phys., 2024, 101382 CrossRef CAS.
- S. Iravani, N. Rabiee and P. Makvandi, J. Mater. Chem. B, 2024, 12, 895–915 RSC.
- G. M. Sacha and P. Varona, Nanotechnology, 2013, 24, 452002 CrossRef CAS PubMed.
- X. He, Z. Xiong, C. Lei, Z. Shen, A. Ni, Y. Xie and C. Liu, Carbon, 2023, 213, 118200 CrossRef CAS.
-
L. R. Johnson, University of Pennsylvania, 2021.
-
J. G. DiStefano, Northwestern University, 2021.
- C. Rong, L. Zhou, B. Zhang and F.-Z. Xuan, Compos. Commun., 2023, 38, 101474 CrossRef.
- Y. Zhou, J.-H. Zhang, S. Li, H. Qiu, Y. Shi and L. Pan, Micromachines, 2023, 14, 1043 CrossRef PubMed.
- S. Siraj, N. Bokka, A. Gade, S. Akella, C. S. R. Kolli and P. Sahatiya, IEEE J. Flexible Electron., 2023, 2(5), 366–373 Search PubMed.
- L. Liu, S. Zhang, L. Zhang, G. Pan and J. Yu, IEEE Trans. Cybern., 2023, 53, 4015–4028 Search PubMed.
- V. Chaudhary, V. Khanna, H. T. A. Awan, K. Singh, M. Khalid, Y. K. Mishra, S. Bhansali, C.-Z. Li and A. Kaushik, Biosens. Bioelectron., 2023, 220, 114847 CrossRef CAS PubMed.
- Z. Cao, B. Sun, G. Zhou, S. S. Mao, S. H. Zhu, J. Zhang, C. Ke, Y. Zhao and J. Shao, Nanoscale Horiz., 2023, 8, 716–745 RSC.
- P. Zhou, R. Peng, M. Xu, V. Wu and D. Navarro-Alarcon, IEEE Robot. Autom. Lett., 2021, 6, 5002–5009 Search PubMed.
- A. Hermawan, T. Amrillah, A. Riapanitra, W. J. Ong and S. Yin, Adv. Healthcare Mater., 2021, 10, 2100970 CrossRef CAS PubMed.
- Y. Ma, D. Zhang, Z. Wang, H. Zhang, H. Xia, R. Mao, H. Cai and H. Luan, ACS Appl. Mater. Interfaces, 2023, 15(24), 29413–29424 CrossRef CAS PubMed.
- R. Bartholomew, C. Shahana, R. Vivek, A. Reddy and P. A. Rasheed, Nanoscale, 2023, 15, 18156–18172 RSC.
- H. Niu, N. Li, E. S. Kim, Y. K. Shin, N. Y. Kim, G. Shen and Y. Li, InfoMat, 2023, e12500 Search PubMed.
-
Y. Zhou, J. Xie, X. Zhang, W. Wu and S. Kwong, IEEE Transactions on Emerging Topics in Computational Intelligence, 2024, pp. 1–13 Search PubMed.
- T.-C. Chung, Y.-H. Hsu, T. Chen, Y. Li, H. Yang, J.-X. Yu, I.-C. Lee, P.-S. Lai, Y.-C. E. Li and P.-Y. Chen, ACS Appl. Mater. Interfaces, 2023, 15, 46460–46469 CrossRef CAS PubMed.
- Z. Ding, W. Su, F. Hakimi, Y. Luo, W. Li, Y. Zhou, L. Ye and H. Yao, Sol. Energy Mater. Sol. Cells, 2023, 262, 112563 CrossRef CAS.
- F.-L. Gao, J. Liu, X.-P. Li, Q. Ma, T. Zhang, Z.-Z. Yu, J. Shang, R.-W. Li and X. Li, ACS Nano, 2023, 17, 16036–16047 CrossRef CAS PubMed.
- K. Ou, M. Wang, C. Meng, K. Guo, N. Shariar Emon, J. Li, K. Qi, Y. Dai and B. Wang, Compos. Sci. Technol., 2024, 255, 110732 CrossRef CAS.
- D. Lan, H. Li, M. Wang, Y. Ren, J. Zhang, M. Zhang, L. Ouyang, J. Tang and Y. Wang, Mater. Res. Bull., 2023, 112630 Search PubMed.
- X. Liang, L. Zhang, Q. Tan, W. Cheng, D. Hu, S. Li, L. Jing and J. Xiong, Microsyst. Nanoeng., 2023, 9, 110 CrossRef CAS PubMed.
- L.-Y. Xiu, Z.-Y. Wang and J.-S. Qiu, Rare Met., 2020, 39, 1237–1238 CrossRef CAS.
- Y. Gogotsi and B. Anasori, ACS Nano, 2019, 13, 8491–8494 CrossRef CAS PubMed.
- M. Khazaei, A. Ranjbar, K. Esfarjani, D. Bogdanovski, R. Dronskowski and S. Yunoki, Phys. Chem. Chem. Phys., 2018, 20, 8579–8592 RSC.
- Y. Wei, P. Zhang, R. A. Soomro, Q. Zhu and B. Xu, Adv. Mater., 2021, 33, 2103148 CrossRef CAS PubMed.
- M. Naguib, M. Kurtoglu, V. Presser, J. Lu, J. Niu, M. Heon, L. Hultman, Y. Gogotsi and M. W. Barsoum, Adv. Mater., 2011, 23, 4207–4207 CrossRef.
- M. Alhabeb, K. Maleski, B. Anasori, P. Lelyukh, L. Clark, S. Sin and Y. Gogotsi, Chem. Mater., 2017, 29, 7633–7644 CrossRef CAS.
- X. Su, J. Zhang, H. Mu, J. Zhao, Z. Wang, Z. Zhao, C. Han and Z. Ye, J. Alloys Compd., 2018, 752, 32–39 CrossRef CAS.
- X. Sang, Y. Xie, M. Lin, M. Alhabeb, K. Van Aken, Y. Gogotsi, P. Kent, K. Xiao and R. Unocic, ACS Nano, 2016, 10, 9193 CrossRef CAS PubMed.
- X.-L. Shi, J. Cao, X. Li, J. Zhang, H. Gong and S. Liu, Colloids Surf., A, 2024, 694, 134160 CrossRef CAS.
- L. Zhang, W. Song, H. Liu, H. Ding, Y. Yan and R. Chen, Processes, 2022, 10, 1744 CrossRef CAS.
- J. Zhou, X. Zha, F. Y. Chen, Q. Ye, P. Eklund, S. Du and Q. Huang, Angew. Chem., Int. Ed., 2016, 55, 5008–5013 CrossRef CAS PubMed.
- Z. Liu, Z. Xu, X. Zheng, Y. Zhao and J. Wang, J. Intell. Fuzzy Syst., 2024, 46, 7021–7034 Search PubMed.
- B. Ding, J. Zhang, P. Zheng, Z. Li, Y. Wang, G. Jia and X. Yu, J. Hydrol., 2024, 632, 130761 CrossRef.
-
X.-H. Zha, J. Zhou, P. Eklund, X. Bai, S. Du and Q. Huang, 2D Metal Carbides and Nitrides (MXenes) Structure, Properties and Applications, 2019, pp. 53–68 Search PubMed.
- M. Ghidiu, M. R. Lukatskaya, M.-Q. Zhao, Y. Gogotsi and M. W. Barsoum, Nature, 2014, 516, 78–81 CrossRef CAS PubMed.
- F. Liu, A. Zhou, J. Chen, J. Jia, W. Zhou, L. Wang and Q. Hu, Appl. Surf. Sci., 2017, 416, 781–789 CrossRef CAS.
- X. Wang, C. Garnero, G. Rochard, D. Magne, S. Morisset, S. Hurand, P. Chartier, J. Rousseau, T. Cabioc'h and C. Coutanceau, J. Mater. Chem. A, 2017, 5, 22012–22023 RSC.
- M. Guo, W.-C. Geng, C. Liu, J. Gu, Z. Zhang and Y. Tang, Chem. Mater., 2020, 32, 8257–8265 CrossRef CAS.
- A. Lipatov, M. Alhabeb, M. R. Lukatskaya, A. Boson, Y. Gogotsi and A. Sinitskii, Adv. Electron. Mater., 2016, 2, 1600255 CrossRef.
- Z. W. Seh, K. D. Fredrickson, B. Anasori, J. Kibsgaard, A. L. Strickler, M. R. Lukatskaya, Y. Gogotsi, T. F. Jaramillo and A. Vojvodic, ACS Energy Lett., 2016, 1, 589–594 CrossRef CAS.
- T. Zhang, L. Pan, H. Tang, F. Du, Y. Guo, T. Qiu and J. Yang, J. Alloys Compd., 2017, 695, 818–826 CrossRef CAS.
- J. Halim, M. R. Lukatskaya, K. M. Cook, J. Lu, C. R. Smith, L.-Å. Näslund, S. J. May, L. Hultman, Y. Gogotsi and P. Eklund, Chem. Mater., 2014, 26, 2374–2381 CrossRef CAS PubMed.
- I. Persson, L.-Å. Näslund, J. Halim, M. W. Barsoum, V. Darakchieva, J. Palisaitis, J. Rosen and P. O. Å. Persson, 2D Mater., 2017, 5, 015002 CrossRef.
- A. Feng, Y. Yu, F. Jiang, Y. Wang, L. Mi, Y. Yu and L. Song, Ceram. Int., 2017, 43, 6322–6328 CrossRef CAS.
- R. Pai, V. Natu, M. Sokol, M. Carey, T. Greszler, M. W. Barsoum and V. Kalra, Mater. Today Energy, 2022, 27, 101000 CrossRef CAS.
- J. Wu, Y. Wang, Y. Zhang, H. Meng, Y. Xu, Y. Han, Z. Wang, Y. Dong and X. Zhang, J. Energy Chem., 2020, 47, 203–209 CrossRef.
- S. Husmann, Ö. Budak, H. Shim, K. Liang, M. Aslan, A. Kruth, A. Quade, M. Naguib and V. Presser, Chem. Commun., 2020, 56, 11082–11085 RSC.
- W. Sun, S. A. Shah, Y. Chen, Z. Tan, H. Gao, T. Habib, M. Radovic and M. J. Green, J. Mater. Chem. A, 2017, 5, 21663–21668 RSC.
- S. Yang, P. Zhang, F. Wang, A. G. Ricciardulli, M. R. Lohe, P. W. Blom and X. Feng, Angew. Chem., 2018, 130, 15717–15721 CrossRef.
- S.-Y. Pang, Y.-T. Wong, S. Yuan, Y. Liu, M.-K. Tsang, Z. Yang, H. Huang, W.-T. Wong and J. Hao, J. Am. Chem. Soc., 2019, 141, 9610–9616 CrossRef CAS PubMed.
- J. Zhu, B. Ma, D. Li, Y. Zhang and L. Xu, Electrochim. Acta, 2024, 478, 143818 CrossRef CAS.
- S. Kumar, Small, 2024, 20, 2308225 CrossRef CAS PubMed.
- X. Xie, Y. Xue, L. Li, S. Chen, Y. Nie, W. Ding and Z. Wei, Nanoscale, 2014, 6, 11035–11040 RSC.
- T. Li, L. Yao, Q. Liu, J. Gu, R. Luo, J. Li, X. Yan, W. Wang, P. Liu and B. Chen, Angew. Chem., Int. Ed., 2018, 57, 6115–6119 CrossRef CAS PubMed.
- G. Li, L. Tan, Y. Zhang, B. Wu and L. Li, Langmuir, 2017, 33, 9000–9006 CrossRef CAS PubMed.
- B. Zhang, J. Zhu, P. Shi, W. Wu and F. Wang, Ceram. Int., 2019, 45, 8395–8405 CrossRef CAS.
- P. Urbankowski, B. Anasori, T. Makaryan, D. Er, S. Kota, P. L. Walsh, M. Zhao, V. B. Shenoy, M. W. Barsoum and Y. Gogotsi, Nanoscale, 2016, 8, 11385–11391 RSC.
- P. Urbankowski, B. Anasori, K. Hantanasirisakul, L. Yang, L. Zhang, B. Haines, S. J. May, S. J. Billinge and Y. Gogotsi, Nanoscale, 2017, 9, 17722–17730 RSC.
- M. Li, J. Lu, K. Luo, Y. Li, K. Chang, K. Chen, J. Zhou, J. Rosen, L. Hultman, P. Eklund, P. O. Å. Persson, S. Du, Z. Chai, Z. Huang and Q. Huang, J. Am. Chem. Soc., 2019, 141, 4730–4737 CrossRef CAS PubMed.
- V. Kamysbayev, A. S. Filatov, H. Hu, X. Rui, F. Lagunas, D. Wang, R. F. Klie and D. V. Talapin, Science, 2020, 369, 979–983 CrossRef CAS PubMed.
- M. Li, X. Li, G. Qin, K. Luo, J. Lu, Y. Li, G. Liang, Z. Huang, J. Zhou and L. Hultman, ACS Nano, 2021, 15, 1077–1085 CrossRef CAS PubMed.
- H. Shi, P. Zhang, Z. Liu, S. Park, M. R. Lohe, Y. Wu, A. Shaygan Nia, S. Yang and X. Feng, Angew. Chem., Int. Ed., 2021, 60, 8689–8693 CrossRef CAS PubMed.
- A. Jawaid, A. Hassan, G. Neher, D. Nepal, R. Pachter, W. J. Kennedy, S. Ramakrishnan and R. A. Vaia, ACS Nano, 2021, 15, 2771–2777 CrossRef CAS PubMed.
- J. Hong, L. Gui and J. Cao, IEEE Trans. Energy Convers., 2023, 38, 1893–1902 Search PubMed.
- J. Mei, G. Ayoko, C. Hu, J. Bell and Z. Sun, Sustainable Mater. Technol., 2020, 25, e00156 CrossRef CAS.
- A. E. Ghazaly, H. Ahmed, A. R. Rezk, J. Halim, P. O. Persson, L. Y. Yeo and J. Rosen, ACS Nano, 2021, 15, 4287–4293 CrossRef CAS PubMed.
- J. Mei, G. A. Ayoko, C. Hu and Z. Sun, Chem. Eng. J., 2020, 395, 125111 CrossRef CAS.
- S. Zada, W. Dai, Z. Kai, H. Lu, X. Meng, Y. Zhang, Y. Cheng, F. Yan, P. Fu and X. Zhang, Angew. Chem., Int. Ed., 2020, 59, 6601–6606 CrossRef CAS PubMed.
- Q. Tao, M. Dahlqvist, J. Lu, S. Kota, R. Meshkian, J. Halim, J. Palisaitis, L. Hultman, M. W. Barsoum and P. O. Persson, Nat. Commun., 2017, 8, 14949 CrossRef PubMed.
- M. Dahlqvist, A. Petruhins, J. Lu, L. Hultman and J. Rosen, ACS Nano, 2018, 12, 7761–7770 CrossRef CAS PubMed.
- J. Lu, A. Thore, R. Meshkian, Q. Tao, L. Hultman and J. Rosén, Cryst. Growth Des., 2017, 17, 5704–5711 CrossRef CAS.
- L. Chen, M. Dahlqvist, T. Lapauw, B. Tunca, F. Wang, J. Lu, R. Meshkian, K. Lambrinou, B. Blanpain and J. Vleugels, Inorg. Chem., 2018, 57, 6237–6244 CrossRef CAS PubMed.
- D. Sun, S. Feng, M. Terrones and R. E. Schaak, Chem. Mater., 2015, 27, 3167–3175 CrossRef CAS.
- C. E. Shuck, A. Sarycheva, M. Anayee, A. Levitt, Y. Zhu, S. Uzun, V. Balitskiy, V. Zahorodna, O. Gogotsi and Y. Gogotsi, Adv. Eng. Mater., 2020, 22, 1901241 CrossRef CAS.
- C. E. Shuck and Y. Gogotsi, Chem. Eng. J., 2020, 401, 125786 CrossRef CAS.
- J. Zhang, N. Kong, S. Uzun, A. Levitt, S. Seyedin, P. A. Lynch, S. Qin, M. Han, W. Yang and J. Liu, Adv. Mater., 2020, 32, 2001093 CrossRef CAS PubMed.
- J. Deng, Z. Lu, L. Ding, Z.-K. Li, Y. Wei, J. Caro and H. Wang, Chem. Eng. J., 2021, 408, 127806 CrossRef CAS.
- M. Naguib, O. Mashtalir, M. R. Lukatskaya, B. Dyatkin, C. Zhang, V. Presser, Y. Gogotsi and M. W. Barsoum, Chem. Commun., 2014, 50, 7420–7423 RSC.
- O. Mashtalir, M. Naguib, V. N. Mochalin, Y. Dall'Agnese, M. Heon, M. W. Barsoum and Y. Gogotsi, Nat. Commun., 2013, 4, 1716 CrossRef PubMed.
- M. Naguib, R. R. Unocic, B. L. Armstrong and J. Nanda, Dalton Trans., 2015, 44, 9353–9358 RSC.
- J. Bai, Y. Ban, J. Bian, A. Chen, H. Chen, H. Chen, J. Chen, X. Chen, Y. Chen and B. Cheng, Phys. Rev. Lett., 2002, 88, 101802 CrossRef CAS PubMed.
- X. Guo, X. Xie, S. Choi, Y. Zhao, H. Liu, C. Wang, S. Chang and G. Wang, J. Mater. Chem. A, 2017, 5, 12445 RSC.
- J. Xuan, Z. Wang, Y. Chen, D. Liang, L. Cheng, X. Yang, Z. Liu, R. Ma, T. Sasaki and F. Geng, Angew. Chem., 2016, 128, 14789–14794 CrossRef.
- N. Driscoll, A. G. Richardson, K. Maleski, B. Anasori, O. Adewole, P. Lelyukh, L. Escobedo, D. K. Cullen, T. H. Lucas and Y. Gogotsi, ACS Nano, 2018, 12, 10419–10429 CrossRef CAS PubMed.
- A. S. Levitt, M. Alhabeb, C. B. Hatter, A. Sarycheva, G. Dion and Y. Gogotsi, J. Mater. Chem. A, 2019, 7, 269–277 RSC.
- F. Liu, J. Zhou, S. Wang, B. Wang, C. Shen, L. Wang, Q. Hu, Q. Huang and A. Zhou, J. Electrochem. Soc., 2017, 164, A709 CrossRef CAS.
- J. Xu, J. Shim, J. H. Park and S. Lee, Adv. Funct. Mater., 2016, 26, 5328–5334 CrossRef CAS.
- S. Lai, J. Jeon, S. K. Jang, J. Xu, Y. J. Choi, J.-H. Park, E. Hwang and S. Lee, Nanoscale, 2015, 7, 19390–19396 RSC.
- T. Hu, J. Wang, H. Zhang, Z. Li, M. Hu and X. Wang, Phys. Chem. Chem. Phys., 2015, 17, 9997–10003 RSC.
- X. Huang and P. Wu, Adv. Funct. Mater., 2020, 30, 1910048 CrossRef CAS.
- O. Mashtalir, K. M. Cook, V. N. Mochalin, M. Crowe, M. W. Barsoum and Y. Gogotsi, J. Mater. Chem. A, 2014, 2, 14334–14338 RSC.
- C. J. Zhang, S. Pinilla, N. McEvoy, C. P. Cullen, B. Anasori, E. Long, S.-H. Park, A. Seral-Ascaso, A. Shmeliov and D. Krishnan, Chem. Mater., 2017, 29, 4848–4856 CrossRef CAS.
- S. Huang and V. N. Mochalin, Inorg. Chem., 2019, 58, 1958–1966 CrossRef CAS PubMed.
- V. Natu, M. Sokol, L. Verger and M. W. Barsoum, J. Phys. Chem. C, 2018, 122, 27745–27753 CrossRef CAS.
- X. Zhao, A. Vashisth, J. W. Blivin, Z. Tan, D. E. Holta, V. Kotasthane, S. A. Shah, T. Habib, S. Liu and J. L. Lutkenhaus, Adv. Mater. Interfaces, 2020, 7, 2000845 CrossRef CAS.
- S. Chae and S.-Y. C. Kim, Nanoscale, 2019, 11, 8387 RSC.
- J. Zhang, N. Kong, D. Hegh, K. A. S. Usman, G. Guan, S. Qin, I. Jurewicz, W. Yang and J. M. Razal, ACS Appl. Mater. Interfaces, 2020, 12, 34032–34040 CrossRef CAS PubMed.
- Q. Zhang, H. Lai, R. Fan, P. Ji, X. Fu and H. Li, ACS Nano, 2021, 15, 5249–5262 CrossRef CAS PubMed.
- Y. Lee, S. J. Kim, Y.-J. Kim, Y. Lim, Y. Chae, B.-J. Lee, Y.-T. Kim, H. Han, Y. Gogotsi and C. W. Ahn, J. Mater. Chem. A, 2020, 8, 573–581 RSC.
- G. Feng, W. Xie, F. Jiang, Q. Yang, W. Jin, C. Shao, J. Yu, Q. Wu, Q. Zhang, D. Wang and J. Liu, Ceram. Int., 2024, 50, 18370–18379 CrossRef CAS.
- L. Xiu, Z. Wang, M. Yu, X. Wu and J. Qiu, ACS Nano, 2018, 12, 8017–8028 CrossRef CAS PubMed.
- P. Zhang, R. A. Soomro, Z. Guan, N. Sun and B. Xu, Energy Storage Mater., 2020, 29, 163–171 CrossRef.
- C. Cui, R. Cheng, H. Zhang, C. Zhang, Y. Ma, C. Shi, B. Fan, H. Wang and X. Wang, Adv. Funct. Mater., 2020, 30, 2000693 CrossRef CAS.
- H. Zhao, J. Ding, M. Zhou and H. Yu, ACS Appl. Nano Mater., 2021, 4, 3075–3086 CrossRef CAS.
- X. Zhao, A. Vashisth, E. Prehn, W. Sun, S. A. Shah, T. Habib, Y. Chen, Z. Tan, J. L. Lutkenhaus and M. Radovic, Matter, 2019, 1, 513–526 CrossRef.
- R. Krishnamoorthy, K. Muthumalai, T. Nagaraja, R. T. Rajendrakumar and S. R. Das, ACS Omega, 2022, 7, 42644–42654 CrossRef CAS PubMed.
- J. Yu, L. Lu, X. Meng and G. E. Karniadakis, Comput. Methods Appl. Mech. Eng., 2022, 393, 114823 CrossRef.
- S. Kalasin, P. Sangnuang and W. Surareungchai, Anal. Chem., 2022, 94, 6842–6852 CrossRef CAS PubMed.
- A. Manikkavel, V. Kumar, M. N. Alam, U. Kim and S.-S. Park, ACS Appl. Electron. Mater., 2023, 5, 5537–5554 CrossRef CAS.
- F. Zhou, X. Wu, S. Fan, X. Zhao, M. Li, F. Song, Y. Huang and X. Zhang, Sci. Total Environ., 2024, 943, 173866 CrossRef CAS PubMed.
- A. Ren, J. Zou, H. Lai, Y. Huang, L. Yuan, H. Xu, K. Shen, H. Wang, S. Wei and Y. Wang, Mater. Horiz., 2020, 7, 1901–1911 RSC.
- Y. Z. Zhang, Y. Wang, Q. Jiang, J. K. El-Demellawi, H. Kim and H. N. Alshareef, Adv. Mater., 2020, 32, 1908486 CrossRef CAS PubMed.
- J. Pang, Y. Wang, X. Yang, L. Zhang, Y. Li, Y. Zhang, J. Yang, F. Yang, X. Wang and G. Cuniberti, Mater. Adv., 2022, 3, 1497–1505 RSC.
- Q. Wang, X. Xiang and B. Chen, Curr. Opin. Food Sci., 2024, 56, 101134 CrossRef CAS.
- C. Hu, Z. Du, Z. Wei, L. Li and G. Shen, Appl. Phys. Rev., 2023, 10, 011402 Search PubMed.
- F. Zhou, J. Cui, J. Zhou, J. Yang, Y. Li, Q. Leng, Y. Wang, D. He, L. Song and M. Gao, Sci. Total Environ., 2018, 633, 776–784 CrossRef CAS PubMed.
- Z. Lou and G. Shen, Small Struct., 2021, 2, 2000152 CrossRef CAS.
- J.-F. Yang, X. Xing, L. Luo, X.-W. Zhou, J.-X. Feng, K.-B. Huang, H. Liu, S. Jin, Y.-N. Liu and S.-H. Zhang, Sci. Immunol., 2023, 8, eabq2424 CrossRef CAS PubMed.
- Y. Cao, C. Liu, J. Jiang, X. Zhu, J. Zhou, J. Ni, J. Zhang, J. Pang, M. H. Rummeli and W. Zhou, Sol. RRL, 2021, 5, 2000800 CrossRef CAS.
- S. Zhang, S. Li and Y. Lu, eScience, 2021, 1, 163–177 CrossRef.
- Y. Guo, S. Wu, Y.-B. He, F. Kang, L. Chen, H. Li and Q.-H. Yang, eScience, 2022, 2, 138–163 CrossRef.
- T.-S. Dinh Le, J. An, Y. Huang, Q. Vo, J. Boonruangkan, T. Tran, S.-W. Kim, G. Sun and Y.-J. Kim, ACS Nano, 2019, 13, 13293–13303 CrossRef CAS PubMed.
- Y. Gu, X. Wang, W. Gu, Y. Wu, T. Li and T. Zhang, Nano Res., 2017, 10, 2683–2691 CrossRef CAS.
- G.-Y. Gou, X.-S. Li, J.-M. Jian, H. Tian, F. Wu, J. Ren, X.-S. Geng, J.-D. Xu, Y.-C. Qiao and Z.-Y. Yan, Sci. Adv., 2022, 8, eabn2156 CrossRef CAS PubMed.
- Y. Cai, J. Shen, G. Ge, Y. Zhang, W. Jin, W. Huang, J. Shao, J. Yang and X. Dong, ACS Nano, 2018, 12, 56–62 CrossRef CAS PubMed.
- J. Dong, Y. Liu, S. Yuan, K. Li, F. Zhang, Z. Guan, H. K. Chai and Q. Wang, Constr. Build. Mater., 2024, 435, 136836 CrossRef CAS.
- J. F. Dong, Z. W. Guan, H. K. Chai and Q. Y. Wang, Constr. Build. Mater., 2023, 389, 131737 CrossRef CAS.
- W. Zhen, Z. Wang, Q. Wang, W. Sun, R. Wang, W. Zhang, Y. Zhang, W. Qin, B. Li, Q. Wang, B. Hong, Y. Yang, J. Xu, S. Ma, M. Da, L. Feng, X. Zang, X. Mo, X. Sun, M. Wu, J. Xu, J. Xu, Y. Huang and H. Zhang, iMeta, 2024, 3, e197 CrossRef PubMed.
- K. Wang, Z. Lou, L. Wang, L. Zhao, S. Zhao, D. Wang, W. Han, K. Jiang and G. Shen, ACS Nano, 2019, 13, 9139–9147 CrossRef CAS PubMed.
- Q. A. Drmosh, I. Olanrewaju Alade, M. Qamar and S. Akbar, Chem. – Asian J., 2021, 16, 1519–1538 CrossRef CAS PubMed.
- A. Kumar, P. Daw and D. Milstein, Chem. Rev., 2021, 122, 385–441 CrossRef PubMed.
- K. Yuan, C.-Y. Wang, L.-Y. Zhu, Q. Cao, J.-H. Yang, X.-X. Li, W. Huang, Y.-Y. Wang, H.-L. Lu and D. W. Zhang, ACS Appl. Mater. Interfaces, 2020, 12, 14095–14104 CrossRef CAS PubMed.
- M. Liu, J. Ji, P. Song, M. Liu and Q. Wang, Sens. Actuators, B, 2021, 349, 130782 CrossRef CAS.
- M. Liu, Z. Wang, P. Song, Z. Yang and Q. Wang, Ceram. Int., 2021, 47(16), 23028–23037 CrossRef CAS.
- S.-J. Young and Y.-L. Chu, J. Electrochem. Soc., 2020, 167, 147508 CrossRef CAS.
- M. Sinha, S. Neogi, R. Mahapatra, S. Krishnamurthy and R. Ghosh, Sens. Actuators, B, 2021, 336, 129729 CrossRef CAS.
- H. Zeng, Z. Yu, L. Shao, X. Li, M. Zhu, Y. Liu, X. Feng and X. Zhu, Chem. Eng. J., 2021, 403, 126281 CrossRef CAS.
- Y. Zhao, Y. Yan, Y. Jiang, Y. Cao, Z. Wang, J. Li, C. Yan, D. Wang, L. Yuan and G. Zhao, Molecules, 2024, 29, 1188 CrossRef CAS PubMed.
- A. Iqbal, P. Sambyal, J. Kwon, M. Han, J. Hong, S. J. Kim, M.-K. Kim, Y. Gogotsi and C. M. Koo, Compos. Sci. Technol., 2021, 213, 108878 CrossRef CAS.
- J. Xu, Z. Mo, D. Ye, M. Wang, F. Liu, G. Jin, C. Xu, X. Wang, Q. Shao and Z. Chen, Nat. Genet., 2012, 44, 1231–1235 CrossRef CAS PubMed.
- T. Zhang, C.-H. Li, W. Li, Z. Wang, Z. Gu, J. Li, J. Yuan, J. Ou-Yang, X. Yang and B. Zhu, Nano-Micro Lett., 2024, 16, 122 CrossRef PubMed.
- C. Qiao, H. Wu, X. Xu, Z. Guan and W. Ou-Yang, Adv. Mater. Interfaces, 2021, 8, 2100903 CrossRef CAS.
- Y. Jin, B. Wen, Z. Gu, X. Jiang, X. Shu, Z. Zeng, Y. Zhang, Z. Guo, Y. Chen and T. Zheng, Adv. Mater. Technol., 2020, 5, 2000262 CrossRef CAS.
- L. Zhao, L. Wang, Y. Zheng, S. Zhao, W. Wei, D. Zhang, X. Fu, K. Jiang, G. Shen and W. Han, Nano Energy, 2021, 84, 105921 CrossRef CAS.
- J. Sun, H. Du, Z. Chen, L. Wang and G. Shen, Nano Res., 2021, 1–7 Search PubMed.
- S. F. Leung, K. T. Ho, P. K. Kung, V. K. Hsiao, H. N. Alshareef, Z. L. Wang and J. H. He, Adv. Mater., 2018, 30, 1704611 CrossRef PubMed.
- Y. Cai, J. Shen, C.-W. Yang, Y. Wan, H.-L. Tang, A. A. Aljarb, C. Chen, J.-H. Fu, X. Wei and K.-W. Huang, Sci. Adv., 2020, 6, eabb5367 CrossRef CAS PubMed.
- Z. Zhang, Q. Yan, Z. Liu, X. Zhao, Z. Wang, J. Sun, Z. L. Wang, R. Wang and L. Li, Nano Energy, 2021, 88, 106257 CrossRef CAS.
- G. Cai, J.-H. Ciou, Y. Liu, Y. Jiang and P. S. Lee, Sci. Adv., 2019, 5, eaaw7956 CrossRef CAS PubMed.
-
Z. Yang, S. Lv, Y. Zhang, J. Wang and L. Jiang.
- G. Cai, J. Ciou, Y. Liu, Y. Jiang and P. S. Lee, Sci. Adv., 2019, 5(7), eaaw7956 CrossRef CAS PubMed.
- D.-G. Seo, Y. Lee, G.-T. Go, M. Pei, S. Jung, Y. H. Jeong, W. Lee, H.-L. Park, S.-W. Kim and H. Yang, Nano Energy, 2019, 65, 104035 CrossRef CAS.
- Y. Zhao, J. Wang, G. Cao, Y. Yuan, X. Yao and L. Qi, IEEE Access, 2023, 11, 86645–86685 Search PubMed.
- C. Chen, D. Han and X. Shen, Knowl. Based Syst., 2023, 275, 110706 CrossRef.
- P. Li, N. Su, Z. Wang and J. Qiu, ACS Nano, 2021, 15, 16811–16818 CrossRef CAS PubMed.
- J. Xu, L. Chang, T. Chen, T. Ren, Y. Zhang and Z. Cai, Compos. Struct., 2023, 322, 117369 CrossRef.
- J. Xu, Y. Zhang, Y. Huang, L. Chang, T. Chen, T. Ren and Z. Cai, Int. J. Mech. Sci., 2024, 264, 108840 CrossRef.
- G. Zhang, W. Li, M. Yu, H. Huang, Y. Wang, Z. Han, K. Shi, L. Ma, Z. Yu, X. Zhu, Z. Peng, Y. Xu, X. Li, S. Hu, J. He, D. Li, Y. Xi, H. Lan, L. Xu, M. Tang and M. Xiao, Adv. Sci., 2023, 10, 2206264 CrossRef CAS PubMed.
- Y. Liu, F. Zhang, W. Zhu, D. Su, Z. Sang, X. Yan, S. Li, J. Liang and S. X. Dou, Carbon, 2020, 160, 88–97 CrossRef CAS.
- H. Niu, H. Zhang, W. Yue, S. Gao, H. Kan, C. Zhang, C. Zhang, J. Pang, Z. Lou and L. Wang, Small, 2021, 17, 2100804 CrossRef CAS PubMed.
- Q. Li, Y. Li and W. Zeng, Chemosensors, 2021, 9, 225 CrossRef CAS.
- M. Hui, X. Jia, X. Li, R. Lazcano-Silveira and M. Shi, J. Inflamm. Res., 2023, 16, 83–93 CrossRef CAS PubMed.
- Y. Zhang, L. Wang, L. Zhao, K. Wang, Y. Zheng, Z. Yuan, D. Wang, X. Fu, G. Shen and W. Han, Adv. Mater., 2021, 33, 2007890 CrossRef CAS PubMed.
- Y. Lei, W. Zhao, Y. Zhang, Q. Jiang, J. H. He, A. J. Baeumner, O. S. Wolfbeis, Z. L. Wang, K. N. Salama and H. N. Alshareef, Small, 2019, 15, 1901190 CrossRef PubMed.
- M. Naguib, M. Kurtoglu, V. Presser, J. Lu, J. Niu, M. Heon, L. Hultman, Y. Gogotsi and M. W. Barsoum, Adv. Mater., 2011, 23, 4248–4253 CrossRef CAS PubMed.
- Q. Tang, Z. Zhou and P. Shen, J. Am. Chem. Soc., 2012, 134, 16909–16916 CrossRef CAS PubMed.
- D. Er, J. Li, M. Naguib, Y. Gogotsi and V. B. Shenoy, ACS Appl. Mater. Interfaces, 2014, 6, 11173–11179 CrossRef CAS PubMed.
- Y. Xie, M. Naguib, V. N. Mochalin, M. W. Barsoum, Y. Gogotsi, X. Yu, K.-W. Nam, X.-Q. Yang, A. I. Kolesnikov and P. R. Kent, J. Am. Chem. Soc., 2014, 136, 6385–6394 CrossRef CAS PubMed.
- K. Xu, X. Ji, B. Zhang, C. Chen, Y. Ruan, L. Miao and J. Jiang, Electrochim. Acta, 2016, 196, 75–83 CrossRef CAS.
- O. Mashtalir, M. Naguib, V. N. Mochalin, Y. Dall'Agnese, M. Heon, M. W. Barsoum and Y. Gogotsi, Nat. Commun., 2013, 4, 1716 CrossRef PubMed.
- D. Sun, M. Wang, Z. Li, G. Fan, L.-Z. Fan and A. Zhou, Electrochem. Commun., 2014, 47, 80–83 CrossRef CAS.
- S. J. Kim, M. Naguib, M. Zhao, C. Zhang, H.-T. Jung, M. W. Barsoum and Y. Gogotsi, Electrochim. Acta, 2015, 163, 246–251 CrossRef CAS.
- C. E. Ren, M. Q. Zhao, T. Makaryan, J. Halim, M. Boota, S. Kota, B. Anasori, M. W. Barsoum and Y. Gogotsi, ChemElectroChem, 2016, 3, 689–693 CrossRef CAS.
- X. Wu, Z. Wang, M. Yu, L. Xiu and J. Qiu, Adv. Mater., 2017, 29, 1607017 CrossRef PubMed.
- O. Mashtalir, M. R. Lukatskaya, A. I. Kolesnikov, E. Raymundo-Pinero, M. Naguib, M. Barsoum and Y. Gogotsi, Nanoscale, 2016, 8, 9128–9133 RSC.
- J. Halim, S. Kota, M. R. Lukatskaya, M. Naguib, M. Q. Zhao, E. J. Moon, J. Pitock, J. Nanda, S. J. May and Y. Gogotsi, Adv. Funct. Mater., 2016, 26, 3118–3127 CrossRef CAS.
- X. Gao, X. Liu, R. He, M. Wang, W. Xie, N. P. Brandon, B. Wu, H. Ling and S. Yang, Energy Storage Mater., 2021, 36, 435–458 CrossRef.
- O. Mashtalir, M. R. Lukatskaya, M.-Q. Zhao, M. W. Barsoum and Y. Gogotsi, Adv. Mater., 2015, 27, 3501–3506 CrossRef CAS PubMed.
- K. Zhang, Z. Hu and J. Chen, J. Energy Chem., 2013, 22, 214–225 CrossRef CAS.
- X. Liang, A. Garsuch and L. F. Nazar, Angew. Chem., 2015, 127, 3979–3983 CrossRef.
- X. Liang, Y. Rangom, C. Y. Kwok, Q. Pang and L. F. Nazar, Adv. Mater., 2017, 29, 1603040 CrossRef PubMed.
- W. Bao, X. Xie, J. Xu, X. Guo, J. Song, W. Wu, D. Su and G. Wang, Chem. – Eur. J., 2017, 23, 12613–12619 CrossRef CAS PubMed.
- Y. Zhao and J. Zhao, Appl. Surf. Sci., 2017, 412, 591–598 CrossRef CAS.
- D. Rao, L. Zhang, Y. Wang, Z. Meng, X. Qian, J. Liu, X. Shen, G. Qiao and R. Lu, J. Phys. Chem. C, 2017, 121, 11047–11054 CrossRef CAS.
- S. Y. Jeong, J. S. Kim and J. H. Lee, Adv. Mater., 2020, 32, 2002075 CrossRef CAS PubMed.
- N. Yabuuchi and K. Kubota, Chem. Rev., 2014, 114, 11636–11682 CrossRef CAS PubMed.
- D. S. Su and G. Centi, J. Energy Chem., 2013, 22, 151–173 CrossRef CAS.
- Y. Xie, Y. Dall'Agnese, M. Naguib, Y. Gogotsi, M. W. Barsoum, H. L. Zhuang and P. R. Kent, ACS Nano, 2014, 8, 9606–9615 CrossRef CAS PubMed.
- Y.-X. Yu, J. Phys. Chem. C, 2016, 120, 5288–5296 CrossRef CAS.
- S. Kajiyama, L. Szabova, K. Sodeyama, H. Iinuma, R. Morita, K. Gotoh, Y. Tateyama, M. Okubo and A. Yamada, ACS Nano, 2016, 10, 3334–3341 CrossRef CAS PubMed.
- S. M. Bak, R. Qiao, W. Yang, S. Lee, X. Yu, B. Anasori, H. Lee, Y. Gogotsi and X. Q. Yang, Adv. Energy Mater., 2017, 7, 1700959 CrossRef.
- X. Xie, M.-Q. Zhao, B. Anasori, K. Maleski, C. E. Ren, J. Li, B. W. Byles, E. Pomerantseva, G. Wang and Y. Gogotsi, Nano Energy, 2016, 26, 513–523 CrossRef CAS.
- M. Q. Zhao, X. Xie, C. E. Ren, T. Makaryan, B. Anasori, G. Wang and Y. Gogotsi, Adv. Mater., 2017, 29, 1702410 CrossRef PubMed.
- Y. Wu, P. Nie, J. Jiang, B. Ding, H. Dou and X. Zhang, ChemElectroChem, 2017, 4, 1560–1565 CrossRef CAS.
- M. Y. Li, Z. Li, H. Li, S. Liu, H. Lu, X. Wen and Y. Yang, ACS Appl. Nano Mater., 2021, 4(12), 13674–13682 CrossRef CAS.
- X. Zhang, X. Cheng and Q. Zhang, J. Energy Chem., 2016, 25, 967–984 CrossRef.
- M. R. Lukatskaya, O. Mashtalir, C. E. Ren, Y. Dall'Agnese, P. Rozier, P. L. Taberna, M. Naguib, P. Simon, M. W. Barsoum and Y. Gogotsi, Science, 2013, 341, 1502–1505 CrossRef CAS PubMed.
- M. R. Lukatskaya, S.-M. Bak, X. Yu, X.-Q. Yang, M. W. Barsoum and Y. Gogotsi, Adv. Energy Mater., 2015, 5, 1500589 CrossRef.
- M. Hu, Z. Li, T. Hu, S. Zhu, C. Zhang and X. Wang, ACS Nano, 2016, 10, 11344–11350 CrossRef CAS PubMed.
- S. Xu, G. Wei, J. Li, Y. Ji, N. Klyui, V. Izotov and W. Han, Chem. Eng. J., 2017, 317, 1026–1036 CrossRef CAS.
- A. Kilic, B. Oral, D. Eroglu and R. Yildirim, J. Energy Storage, 2023, 73, 109057 CrossRef.
- J. Li, X. Yuan, C. Lin, Y. Yang, L. Xu, X. Du, J. Xie, J. Lin and J. Sun, Adv. Energy Mater., 2017, 7, 1602725 CrossRef.
- M. Ghidiu, S. Kota, J. Halim, A. W. Sherwood, N. Nedfors, J. Rosen, V. N. Mochalin and M. W. Barsoum, Chem. Mater., 2017, 29, 1099–1106 CrossRef CAS.
- Y. Wen, T. E. Rufford, X. Chen, N. Li, M. Lyu, L. Dai and L. Wang, Nano Energy, 2017, 38, 368–376 CrossRef CAS.
- Z. Ling, C. E. Ren, M.-Q. Zhao, J. Yang, J. M. Giammarco, J. Qiu, M. W. Barsoum and Y. Gogotsi, Proc. Natl. Acad. Sci. U. S. A., 2014, 111, 16676–16681 CrossRef CAS PubMed.
- M. Boota, B. Anasori, C. Voigt, M. Q. Zhao, M. W. Barsoum and Y. Gogotsi, Adv. Mater., 2016, 28, 1517–1522 CrossRef CAS PubMed.
- Q. Xue, H. Zhang, M. Zhu, Z. Pei, H. Li, Z. Wang, Y. Huang, Y. Huang, Q. Deng and J. Zhou, Adv. Mater., 2017, 29, 1604847 CrossRef PubMed.
- M. Boota, M. Pasini, F. Galeotti, W. Porzio, M.-Q. Zhao, J. Halim and Y. Gogotsi, Chem. Mater., 2017, 29, 2731–2738 CrossRef CAS.
- Z. Lin, D. Barbara, P.-L. Taberna, K. L. Van Aken, B. Anasori, Y. Gogotsi and P. Simon, J. Power Sources, 2016, 326, 575–579 CrossRef CAS.
- Z. Lin, P. Rozier, B. Duployer, P.-L. Taberna, B. Anasori, Y. Gogotsi and P. Simon, Electrochem. Commun., 2016, 72, 50–53 CrossRef CAS.
- Y.-Y. Peng, B. Akuzum, N. Kurra, M.-Q. Zhao, M. Alhabeb, B. Anasori, E. C. Kumbur, H. N. Alshareef, M.-D. Ger and Y. Gogotsi, Energy Environ. Sci., 2016, 9, 2847–2854 RSC.
- Y. Hou, M. R. Lohe, J. Zhang, S. Liu, X. Zhuang and X. Feng, Energy Environ. Sci., 2016, 9, 478–483 RSC.
- K. Krishnamoorthy, P. Pazhamalai, S. Sahoo and S.-J. Kim, J. Mater. Chem. A, 2017, 5, 5726–5736 RSC.
- X. Ji, K. Xu, C. Chen, B. Zhang, Y. Ruan, J. Liu, L. Miao and J. Jiang, Phys. Chem. Chem. Phys., 2016, 18, 4460–4467 RSC.
- Y. Gan, F. Xu, J. Luo, H. Yuan, C. Jin, L. Zhang, C. Fang, O. Sheng, H. Huang and Y. Xia, Electrochim. Acta, 2016, 209, 201–209 CrossRef CAS.
- Y. Dall'Agnese, P.-L. Taberna, Y. Gogotsi and P. Simon, J. Phys. Chem. Lett., 2015, 6, 2305–2309 CrossRef PubMed.
- A. Byeon, A. M. Glushenkov, B. Anasori, P. Urbankowski, J. Li, B. W. Byles, B. Blake, K. L. Van Aken, S. Kota and E. Pomerantseva, J. Power Sources, 2016, 326, 686–694 CrossRef CAS.
- A. Kausar, Polym.-Plast. Technol. Mater., 2021, 60, 1377–1392 CAS.
- J. Wu, H. Guan, Y. Fan, X. Xi, F. Nie and Y. Liu, Integr. Ferroelectr., 2022, 228, 254–271 CrossRef CAS.
- F. Shahzad, S. A. Zaidi and R. A. Naqvi, Crit. Rev. Anal. Chem., 2022, 52, 848–864 CrossRef CAS PubMed.
- S. Zhang, C. Wang, H. Zhang and H. Lin, Chaos, Solitons Fractals, 2024, 186, 115191 CrossRef.
- C. Yakopcic, R. Hasan and T. M. Taha, Int. J. Parallel Emergent Distrib. Syst., 2018, 33, 408–429 CrossRef.
- D. Howard, L. Bull and B. de Lacy Costello, Connect. Sci., 2015, 27, 397–416 CrossRef.
- J. Wang, X. Zhuge and F. Zhuge, Sci. Technol. Adv. Mater., 2021, 22, 326–344 CrossRef PubMed.
- L. Kang, B. Tang, J. Huang and J. Li, Comput. Methods Programs Biomed., 2024, 248, 108110 CrossRef PubMed.
- M. R. Ali, M. S. Bacchu, M. R. Al-Mamun, M. I. Hossain, A. Khaleque, A. Khatun, D. D. Ridoy, M. A. S. Aly and M. Z. H. Khan, Crit. Rev. Anal. Chem., 2022, 1–18 CrossRef PubMed.
- J. Liu and R. Xu, Int. J. Syst. Sci., 2018, 49, 1300–1315 CrossRef.
- A. Singh, IETE J. Res., 2020, 66, 182–191 CrossRef.
- A. C. Khot, T. D. Dongale, J. H. Park, A. V. Kesavan and T. G. Kim, ACS Appl. Mater. Interfaces, 2021, 13, 5216–5227 CrossRef CAS PubMed.
- X. Yan, K. Wang, J. Zhao, Z. Zhou, H. Wang, J. Wang, L. Zhang, X. Li, Z. Xiao and Q. Zhao, Small, 2019, 15, 1900107 CrossRef PubMed.
- K. Wang, J. Chen and X. Yan, Nano Energy, 2021, 79, 105453 CrossRef CAS.
- Y. Chen, Y. Wang, Y. Luo, X. Liu, Y. Wang, F. Gao, J. Xu, E. Hu, S. Samanta and X. Wan, IEEE Electron Device Lett., 2019, 40, 1686–1689 CAS.
-
Z. Shen, C. Zhao, Y. Liu, L. Yang and C. Zhao, 2021 18th International SoC Design Conference (ISOCC), 2021.
- K. Wang, J. Chen and X. Yan, Nano Energy, 2021, 79, 105453 CrossRef CAS.
- R. Yu, X. Zhang, C. Gao, E. Li, Y. Yan, Y. Hu, H. Chen, T. Guo and R. Wang, Nano Energy, 2022, 99, 107418 CrossRef CAS.
- Y. Wang, D. Shen, Y. Liang, Y. Zhao, X. Chen, L. Zhou, M. Zhang, J. Xu, X. Liu and E. Hu, Mater. Sci. Semicond. Process., 2021, 135, 106123 CrossRef CAS.
- Y. Wang, X. Chen, D. Shen, M. Zhang, X. Chen, X. Chen, W. Shao, H. Gu, J. Xu and E. Hu, Nanomaterials, 2021, 11, 2860 CrossRef CAS PubMed.
- X. Chen, Y. Wang, D. Shen, M. Zhang, Y. Zhao, L. Zhou, Q. Qin, Q. Zhang, N. He and M. Wang, IEEE Trans. Nanotechnol., 2021, 20, 512–516 CAS.
- S. Fatima, M. W. Hakim, D. Akinwande and S. Rizwan, Mater. Today Phys., 2022, 26, 100730 CrossRef CAS.
- L. Wang, J. Wen, Y. Jiang, Q. Ou, L. Yu, B.-S. Xiong, B. Yang, C. Zhang and Y. Tong, Materials, 2020, 13, 3671 CrossRef CAS PubMed.
- Y. Wang, X. Liu, Y. Chen, W. Xu, D. Liang, F. Gao, M. Zhang, S. Samanta, X. Gong and X. Lian, Appl. Phys. Express, 2019, 12, 106504 CrossRef CAS.
- A. Melianas, M. A. Kang, A. VahidMohammadi, T. J. Quill, W. Tian, Y. Gogotsi, A. Salleo and M. M. Hamedi, Adv. Funct. Mater., 2022, 32, 2109970 CrossRef CAS.
- J. Lu, Y. Zhang, Y. Tao, B. Wang, W. Cheng, G. Jie, L. Song and Y. Hu, J. Colloid Interface Sci., 2021, 588, 164–174 CrossRef CAS PubMed.
- X. Lian, Y. Shi, S. Li, B. Ding, C. Hua and L. Wang, Contemp. Phys., 2022, 63, 87–105 CrossRef.
- V. H. Nguyen, R. Tabassian, S. Oh, S. Nam, M. Mahato, P. Thangasamy, A. Rajabi-Abhari, W. J. Hwang, A. K. Taseer and I. K. Oh, Adv. Funct. Mater., 2020, 30, 1909504 CrossRef CAS.
- X. Sang, Y. Xie, M.-W. Lin, M. Alhabeb, K. L. Van Aken, Y. Gogotsi, P. R. Kent, K. Xiao and R. R. Unocic, ACS Nano, 2016, 10, 9193–9200 CrossRef CAS PubMed.
- X. Lian, X. Shen, M. Zhang, J. Xu, F. Gao, X. Wan, E. Hu, Y. Guo, J. Zhao and Y. Tong, Appl. Phys. Lett., 2019, 115, 063501 CrossRef.
- X. Wan, W. Xu, M. Zhang, N. He, X. Lian, E. Hu, J. Xu and Y. Tong, ACS Appl. Electron. Mater., 2020, 2, 3497–3501 CrossRef CAS.
- X. Lian, Y. Shi, X. Shen, X. Wan, Z. Cai and L. Wang, Chin. J. Electron., 2024, 33, 1–8 Search PubMed.
- L. Guo, B. Mu, M.-Z. Li, B. Yang, R.-S. Chen, G. Ding, K. Zhou, Y. Liu, C.-C. Kuo and S.-T. Han, ACS Appl. Mater. Interfaces, 2021, 13, 39595–39605 CrossRef CAS PubMed.
- M.-Y. Li, Z. Li, H. Li, S. Liu, H. Lu, X. Wen and Y. Yang, ACS Appl. Nano Mater., 2021, 4, 13674–13682 CrossRef CAS.
- N. He, Q. Zhang, L. Tao, X. Chen, Q. Qin, X. Liu, X. Lian, X. Wan, E. Hu and J. Xu, IEEE Electron Device Lett., 2021, 42, 319–322 CAS.
- Y. Wang, Y. Gong, L. Yang, Z. Xiong, Z. Lv, X. Xing, Y. Zhou, B. Zhang, C. Su and Q. Liao, Adv. Funct. Mater., 2021, 31, 2100144 CrossRef CAS.
- S. Fatima, X. Bin, M. A. Mohammad, D. Akinwande and S. Rizwan, Adv. Electron. Mater., 2022, 8, 2100549 CrossRef CAS.
- Y.-F. Lu, Y. Li, H. Li, T.-Q. Wan, X. Huang, Y.-H. He and X. Miao, IEEE Electron Device Lett., 2020, 41, 1245–1248 CAS.
- M. Zhang, Q. Qin, X. Chen, R. Tang, A. Han, S. Yao, R. Dan, Q. Wang, Y. Wang and H. Gu, Ceram. Int., 2022, 48, 16263–16272 CrossRef CAS.
- X.-J. Lian, J.-K. Fu, Z.-X. Gao, S.-P. Gu and L. Wang, Chin. Phys. B, 2023, 32, 017304 CrossRef.
- Z. Wen and D. Wu, Adv. Mater., 2020, 32, 1904123 CrossRef CAS PubMed.
- M. Gabel and Y. Gu, Adv. Funct. Mater., 2021, 31, 2009999 CrossRef CAS.
- A. Chen, W. Zhang, L. R. Dedon, D. Chen, F. Khatkhatay, J. L. MacManus-Driscoll, H. Wang, D. Yarotski, J. Chen and X. Gao, Adv. Funct. Mater., 2020, 30, 2000664 CrossRef CAS.
- M. Shi, M. Zhang, S. Yao, Q. Qin, M. Wang, Y. Wang, N. He, J. Zhu, X. Liu and E. Hu, J. Phys.: Conf. Ser., 2020, 038501 Search PubMed.
- R. Mahe, Z. Ye, M. Qi, W. Cai, Y. Wen, J. Xiao, Y. Liu, S. Zhu and J. Zhao, Rev. Fish. Sci. Aquac., 2024, 32, 505–525 CrossRef.
- D. Zhang, C. Du, Y. Peng, J. Liu, S. Mohammed and A. Calvi, IEEE Trans. Intell. Transp. Syst., 2024, 1–13 Search PubMed.
- M. Bertelli, A. Díaz Fattorini, S. De Simone, S. Calvi, R. Plebani, V. Mussi, F. Arciprete, R. Calarco and M. Longo, Nanomaterials, 2022, 12, 2001 CrossRef CAS PubMed.
- Q. Wang, G. Niu, R. Wang, R. Luo, Z.-G. Ye, J. Bi, X. Li, Z. Song, W. Ren and S. Song, J. Materiomics, 2022, 8, 382–391 CrossRef.
-
T. Abzhanova, I. Dolzhikova and A. P. James, 2018 International Conference on Computing and Network Communications (CoCoNet), 2018.
- Q. Wang, H. Sun, J. Zhang, X. Xu and X. Miao, J. Electron. Mater., 2012, 41, 3417–3422 CrossRef CAS.
- X. F. Lu, Y. Zhang, N. Wang, S. Luo, K. Peng, L. Wang, H. Chen, W. Gao, X. H. Chen and Y. Bao, Nano Lett., 2021, 21, 8800–8807 CrossRef CAS PubMed.
- L. Sun, Z. Wang, J. Jiang, Y. Kim, B. Joo, S. Zheng, S. Lee, W. J. Yu, B.-S. Kong and H. Yang, Sci. Adv., 2021, 7, eabg1455 CrossRef CAS PubMed.
- S. Saha, V. Adepu, K. Gohel, P. Sahatiya and S. S. Dan, IEEE Trans. Electron Devices, 2022, 69, 5921–5927 CAS.
- H. Wei, H. Yu, J. Gong, M. Ma, H. Han, Y. Ni, S. Zhang and W. Xu, Adv. Funct. Mater., 2021, 31, 2007232 CrossRef CAS.
- L. Wang, C.-H. Yang and J. Wen, Electron. Mater. Lett., 2015, 11, 505–543 CrossRef CAS.
- J. Li, T. Lu, X. Yi, M. An and R. Hao, Sustain. Energy Technol. Assess., 2024, 64, 103671 Search PubMed.
- B. Guo, J. Zhao, Y. Xu, X. Wen, X. Ren, X. Huang, S. Niu, Y. Dai, R. Gao and P. Xu, ACS Appl. Mater. Interfaces, 2024, 16, 8939–8948 CrossRef CAS PubMed.
|
This journal is © The Royal Society of Chemistry 2024 |
Click here to see how this site uses Cookies. View our privacy policy here.