DOI:
10.1039/D3RA07598B
(Paper)
RSC Adv., 2024,
14, 3261-3266
Self-assembled copper nanoclusters used to mimic peroxidase for glucose detection
Received
7th November 2023
, Accepted 5th January 2024
First published on 19th January 2024
Abstract
A sensing system for glucose was established based on a self-assembled copper nanoclusters (Cu NCs)-based nano-enzyme and glucose oxidase (GOD). The assembled copper nanosheets (Cu NSs) were prepared in a one-step method using 2,3,4,5,6-pentafluorothiophenol (PFTP) as a reducing agent and protecting ligand. Cu NSs could be used to mimic the enzyme horseradish peroxidase. Cu NSs were endowed with excellent enzymatic catalytic activity in the oxidation of o-phenyldiamine (OPD) in the presence of H2O2. The latter could be generated in the aerobic oxidation of glucose catalyzed by GOD. Therefore, a detection method for glucose was constructed based on a Cu NSs-OPD-GOD catalytic system. This proposed sensing platform showed a standard linear range from 10 μM to 5 mM towards glucose, and the limit of detection was 5.5 μM. Finally, practical application of a sensor based on the Cu NSs nano-enzyme was verified in three sugared beverages as real samples. Our data reveal that the prepared Cu NSs could mimic peroxidase and be applied to a mixed catalytic system with GOD for glucose detection.
Introduction
Atomically precise metal nanoclusters (NCs) present considerable variety in their structures and properties. A comprehensive understanding about the natural connection between the structure and properties of nanomaterials is important for the rational design of multiple functional properties and expansion of application areas. Recently, several studies related to nanoclusters comprising metals (e.g., Au, Ag, Cu) and protected ligands (e.g., thiols, phosphines) have been published. These metal NCs with promising properties have been utilized in catalysis, sensing, medicines, bioimaging, energy conversion, and optoelectronics.1–5 Among coinage metals (e.g., Cu, Ag, Au), the [Aun(SR)n+1] unit is a common surface-structure motif of Au NCs stabilized by thiolate.6–8 However, Ag NCs and Cu NCs usually have varying and complicated geometrical motifs. Hence, they have great potential for NCs species with different configurations.9–13 The metal core and surface ligands influence the properties of metal NCs. Compared with the noble metals Au and Ag, the metal Cu is widely used in industries because it has higher conductivity, is cheaper, and is relatively abundant. Also, Cu is relatively active, so Cu NCs can be used for more diverse applications than noble-metal NCs.
Some metallic Cu-based nanomaterials (e.g., Cu2O nanoparticles, Cu2+-doped carbon nitride or carbon dots, Cu@Cu2O aerogel networks) and Cu NCs have been indicated to mimic the enzymatic performance of horseradish peroxidase.14–16 Compared with those nanoparticles, Cu NCs are disordered, aggregated or oxidized in an ambient atmosphere, which weakens their catalytic activity and restricts further application in analytical fields. Self-directed or directed assembly of nanoparticles into one-, two-, or three-dimensional nanostructures has attracted growing interest owing to their collective properties, such as mimicking of enzyme functions or high electrocatalytic activity.17–19 Therefore, developing a facile and environmental approach for assembling nanosized metal NCs into macroscopic nanostructures that can mimic the catalytical function of peroxidase enzyme is a fascinating concept.
Glucose is the elementary unit of starch, glycogen, cellulose, and other polysaccharides. In humans, the carbohydrates in foods are eventually converted into glucose after digestion and absorption, and are then present in blood. The glucose content in blood is an important reference indicator for metabolic capacity and the health of humans.20,21 Therefore, glucose detection focuses mostly on blood sugar content, but ignores the glucose content in foods. Foods with a higher proportion of glucose will lead to an increase in the glycemic index, which induces adverse effects on human health (especially for people with diabetes mellitus).22–24 Thus, accurate detection/monitoring of glucose in foods is very important for public health. Glucose oxidase (GOD)- or hexokinase-based enzymatic methods are used widely in the clinical determination of glucose. These methods have the advantages of excellent specificity and a low limit of detection.
Herein, a new sensing strategy for glucose was developed using self-assembled Cu NCs as biomimetic catalytic nanomaterials. Using 2,3,4,5,6-pentafluorothiophenol (PFTP) as the protected ligand and reductant, self-assembled copper nanosheets (Cu NSs) were prepared via a simple one-step method (reaction at room temperature without heating). Then, the morphology, components, and catalytical properties of PFTP-stabilized Cu NCs were investigated. Noteworthily, choosing o-phenyldiamine (OPD) as a substrate, Cu NSs exhibited excellent peroxidase-like catalytic activity in the presence of hydrogen peroxide (H2O2). Then, a sensing system for glucose was established based on GOD and Cu NSs nano-enzyme. In the presence of glucose, H2O2 could be generated in the aerobic oxidation of glucose catalyzed by GOD, along with generation of 2,3-diaminophenazine (DAP) (oxidized product of OPD). Therefore, the glucose level could be determined accurately by measuring the characteristic absorption at 423 nm or fluorescence emission at 538 nm of DAP. As a result, this proposed sensing platform detected the content of glucose in three types of sugary drink.
Experimental section
Materials and reagents
PFTP and GOD (lyophilized powder, 100–250 units per mg) were obtained from MilliporeSigma. Cupric nitrate (Cu(NO3)2·3H2O), glucose, OPD, H2O2, acetic acid (HAC), sodium acetate (NaAC), maltose (Mal), fructose (Fru), sucrose (Suc), lactose (Lac), NaCl, zinc acetate, potassium hexacyanoferrate, and ethanol were obtained from Sinopharm Chemical Reagents. All chemical reagents were used as received without further purification.
Characterization
UV-vis absorption spectra were recorded by a UV-vis spectrophotometer (TU-1901; Persee). Scanning electron microscopy (SEM) and energy-dispersive X-ray spectroscopy (EDS) were conducted using an electron microscope (Apreo; FEI). Transmission electron microscopy (TEM) and high-resolution TEM (HRTEM) images were obtained using an electron microscope (JEM-2100F; JEOL). X-ray diffraction (XRD) patterns were collected using Cu Kα radiation (λ = 1.54 Å) from a diffractometer (D/max-2500; Rigaku) operated at 40 kV and 200 mA. X-ray photoelectron spectroscopy (XPS) data were obtained by a spectrometer (VG ESCALAB™ MKII; Thermo Fisher Scientific). The binding-energy scale of spectra was aligned through the C (1s) peak at 284.6 eV.
Preparation of Cu NSs
Self-assembled Cu NSs were synthesized on the basis of our previous method25 with slight modification. Specifically, 12 mg Cu(NO3)2·3H2O was dissolved in 3 mL of ethanol. Then, 21 μL of PFTP was added under stirring, and the reaction mixture was stirred continuously at room temperature for 3 h. A white precipitate could be isolated via centrifugation and followed by washing repeatedly with ethanol. Finally, the pre-pared product was dried in a stream of high-purity nitrogen flow for further use.
Mimetic catalytic activity of Cu NSs
A mixture of OPD of different concentrations and H2O2 was catalyzed by Cu NSs in buffer solution. The oxidative product of OPD was confirmed by UV-vis absorbance on a spectrophotometer. The concentration of the product was calculated using its molar extinction coefficient ε at a designated wavelength. All experiments were performed thrice. The reaction rate was fitted to the Michaelis–Menten equation:
Oxidation of OPD
In the typical oxidation of OPD, 0.5 mL of OPD (final concentration = 0.25, 0.5, 1.0, 1.5, 2.0, 2.5 or 3 mM) and 0.5 mL of Cu NSs (final concentration = 20 μg mL−1) was mixed with 2 mL of HAC-NaAC buffer (pH = 5.0). Then, we added 0.5 mL of H2O2 with a final concentration of 6 mM. The catalytic oxidation of OPD was measured by recording changes in the absorption of DAP product at 423 nm (ε = 16
300 M−1 cm−1). UV-vis spectroscopy was done using a quartz cuvette of width 1.0 cm.
Sensitivity and selectivity
A standard solution of glucose of various concentrations was prepared by serial dilution of a stock solution (20 mM). For glucose detection, 0.5 mL of Cu NSs (20 μg mL−1) was mixed with 2 mL of HAC-NaAC buffer (pH = 5.0), and then 0.5 mL of glucose solution was added with GOD. The mixture was incubated in a shaker for 20 min and then transferred for measurement of UV-vis absorption. The selectivity experiment towards glucose was investigated through five types present in foods: maltose (Mal), fructose (Fru), sucrose (Suc), lactose (Lac), and NaCl.
Analysis of a real sample
We wished to evaluate practical application of the Cu NSs-GOD-OPD catalytical system for glucose detection. Three kinds of sugared beverages purchased from a local supermarket in Taiyuan were used as real samples. Sample 1 was green tea (Master Kong). Sample 2 was a vitamin drink (Mizone). Sample 3 was an electrolyte drink (Pocari Sweat). First, samples were diluted 100-times by HAC-NaAC buffer. Then, they were detected directly by the Cu NSs-GOD-OPD sensing system and HPLC. The latter was conducted according to the procedures in GB 5009.208-2023 (China).
Results and discussion
Synthesis and characterization of Cu NSs
Using PFTP as the capping ligand cum reductant, self-assembled Cu NCs were synthesized simply by a one-step method. After mixing the Cu2+ precursor and PFTP at room temperature, a white product was obtained under strong stirring. This synthetic method was simple, easy to operate, stable, environmentally friendly, and did not require a heating step for preparation. The morphology of self-assembled Cu NCs was investigated first using SEM and TEM. As shown in Fig. 1, Cu NCs were assembled to nanosheets (NSs), which appeared as well-defined polygons of approximate length 700–1300 nm.
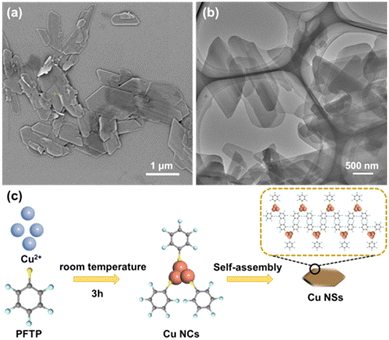 |
| Fig. 1 (a) SEM image of self-assembled Cu NSs. (b) TEM image of self-assembled Cu NSs. (c) Preparation of Cu NSs (schematic). | |
The chemical constitution of self-assembled Cu NSs was characterized further by EDS (Fig. 2a) and XPS (Fig. 2b), which indicated that the prepared Cu NCs were composed of Cu, S, F, and C, originating from the Cu ion precursor and ligand, respectively. The spectra of Cu 2p (Fig. 2c) showed typical binding peaks at 932.6 eV and 952.3 eV, and a satellite peak was not observed around 942 eV, suggesting that the valence states of Cu in Cu NCs were Cu(I) or Cu(0) rather than Cu(II).26,27 Moreover, the spectra of S 2p (Fig. 2d) showed two typical peaks attributed to S–Cu and S–C bonding, respectively.28 Therefore, the Cu2+ precursor was reduced by the sulfydryl group of PFTP through formation of the Cu–S bond, which verified that PFTP acted as a reducing agent and protecting agent in the synthesis of Cu NCs (Fig. 1c).
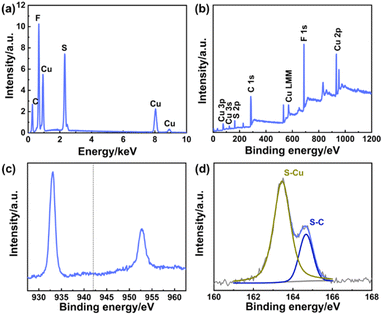 |
| Fig. 2 (a) EDS and elemental analysis of Cu NSs. XPS survey spectra of Cu NSs (b), Cu 2p (c), and S 2p (d). | |
Different from the continuous electronic-band structure of plasmonic nanoparticles, NCs possess molecular-like discrete energy levels.29,30 The UV-vis absorption spectrum of PFTP and prepared Cu NSs are shown on Fig. 3a. Compared with the thiol ligand, characteristic absorption peaks at 246, 281, and 410 nm were observed in the UV-vis absorption spectra of Cu NSs, which suggested the formation of Cu NCs. The characteristic absorption of Cu NCs could be attributed to the electron transfer between hybrid bonding orbitals.31 HRTEM (Fig. 3c) and selected-area electron-diffraction pattern (Fig. 3d) revealed diffraction rings and the polycrystalline nature of self-assembled Cu NSs. HRTEM and XRD were carried out to investigate the crystalline phase of Cu NSs. As shown in Fig. 3c, some Cu NCs were assembled into a regular crystalloid structure with an interplanar lattice of 0.33 nm, which was larger than the interplanar lattice of Cu (111). Hence, the observed crystalloid was an assembly of Cu NCs packaged with surface ligands rather than a “stack” of Cu atoms. The strongest and sharpest diffraction peak of Cu NSs at 5.98° corresponded to a d-spacing of 1.48 nm, thereby revealing the distance between adjacent layers of NCs in assembled Cu NSs.
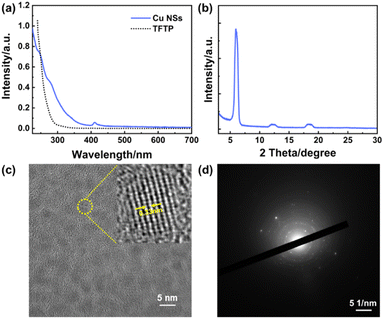 |
| Fig. 3 (a) UV-vis absorbance spectrum of PFTP and Cu NSs. (b) XRD pattern of Cu NSs. (c) HRTEM image of assembled Cu NSs. (d) Selected-area electron diffraction pattern of assembled Cu NSs. | |
Mimetic catalytic activity of Cu NSs
The creation of artificial enzymes based on nanomaterials is an important branch of biomimetic chemistry. Cu NCs could act as biocatalysts owing to their ultra-small size and their catalytic center being a metal core.32 Self-assembled Cu NSs with a potentially catalytically active center were utilized as catalytic nano-enzymes. Using OPD as a substrate, we investigated the mimic peroxidase catalytic activity of Cu NSs in the presence of H2O2. DAP (oxidized product of OPD) had characteristic UV-vis absorption and fluorescence emission upon excitation. These features could be advantageous for investigating the peroxidase-like activity of Cu NSs via colorimetric or fluorometric methods. As shown in Fig. 4a, Cu NSs, OPD, and OPD-H2O2 systems did not show an obvious absorption peak. When Cu NSs were present in the OPD-H2O2 reaction system, a distinct absorption peak at 423 nm originating from DAP appeared. The OPD-H2O2 system exhibited strong yellow fluorescence emission in the presence of Cu NSs (Fig. 4b). Hence, Cu NSs exhibited a peroxidase-like catalytic function in the oxidation reaction of the OPD-H2O2 system.
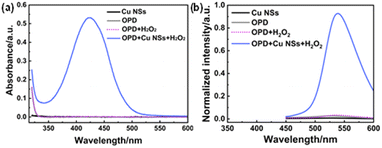 |
| Fig. 4 (a) UV-vis absorbance spectrum of OPD-H2O2 system mediated by Cu NSs. (b) Fluorescence emission spectra of the OPD-H2O2 system mediated by Cu NSs. | |
Then, several enzymatic activity-dependent reaction parameters were optimized: pH, temperature, OPD concentration, and H2O2 concentration. As shown in Fig. 5a and b, Cu NSs mimicked catalytic activity in a pH range from 3 to 8 and exhibited maximum activity at pH 5. Cu NSs exhibited higher catalytic activity under acidic conditions, which suggested that catalytic activity was dependent upon the copper core of Cu NCs in assembled Cu NSs.
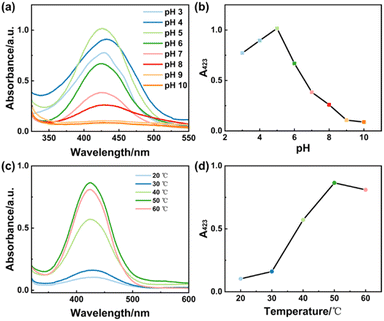 |
| Fig. 5 (a) Optimization of pH for peroxidase-like activity of Cu NSs. (b) Change in absorbance monitored at 423 nm as a function of pH. (c) Optimization of temperature for the peroxidase-like activity of Cu NSs. (d) Change in absorbance monitored at 423 nm as a function of temperature. | |
Next, we changed the temperature from 20 °C to 60 °C, and found that catalytic activity was highest at 50 °C (Fig. 5c and d). Then, all experiments on the applicability of Cu NSs as artificial enzymatic catalysts were carried out under optimized conditions: 50 °C and pH of the solution = 5.
The kinetic-reaction parameters for OPD and H2O2 were estimated under optimized reaction conditions. Based on the different oxidation rates with variable substrate concentrations, nearly ideal hyperbola relationships could be obtained, and complied with the classic Michaelis–Menten equation via nonlinear Hill function simulation (Fig. 6a and c).14 Lineweaver–Burk plots were obtained by linear fit (Fig. 6b and d). The DAP concentration was calculated using the absorbance value at 423 nm and a molar extinction coefficient (ε) of 16
300 M−1 cm−1.14 Then, the Michaelis–Menten constant (Km) and maximum initial velocity (Vmax) were calculated from these plots (Table 1). The Km for OPD was 5.94 mM, which confirmed the high binding affinity between Cu NSs and OPD. Vmax was 14.28 μM min−1, and was ascribed to the higher surface area and more exposed activity sites in Cu NSs.
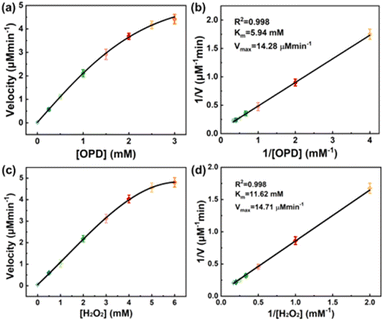 |
| Fig. 6 Michaelis–Menten curves and Lineweaver–Burk plots for OPD (a and b) and H2O2 (c and d). | |
Table 1 Comparison of the OPD activity of Cu NSs with other reported results
Catalyst |
Substrate |
Km (mM) |
Vmax (μM min−1) |
Reference |
Cu@Cu2O aeroge |
OPD |
8.88 |
1.42 |
14 |
Cu-CDs |
OPD |
1.58 |
6.48 |
15 |
Phe-Cu NCs |
OPD |
0.65 |
13.2 |
16 |
Cu NSs |
OPD |
5.94 |
14.28 |
This work |
For the H2O2 kinetic assay (Fig. 6c and d), Km and Vmax were estimated to be 11.62 mM and 14.71 μM min−1, respectively. Compared with other reported catalysts based on Cu metal (Table 1), the apparent Km of Cu NSs was lower than that reported for the Cu@Cu2O aerogel network, indicating that Cu NSs exhibited higher binding affinity for OPD. Cu NSs showed larger Vmax than most nanozymes reported for OPD, which might be ascribed to the multivalent copper of Cu NCs.15
Sensing systems for glucose
Based on the excellent peroxidase-like catalytic activity of Cu NSs, a sensing system for glucose could be constructed by indirectly monitoring H2O2 generated from GOD-catalyzed aerobic oxidation. As shown in Fig. 7a, H2O2 was generated from aerobic oxidation of glucose catalyzed by GOD, and then OPD was oxidized to DAP by the catalysis of Cu NSs. As a result, the absorbance at 423 nm (A423) of DAP increased with the glucose concentration (Fig. 7b), which verified that the proposed system based on Cu NSs was sensitive to glucose. Fig. 7c is a plot of A423 and the glucose concentration (c) in the Cu NSs-OPD-GOD catalyzed system. The equation could be indicated as A423 = 0.4382c + 0.0010 with a standard linear range between 10 μM and 5 mM. The limit of detection was calculated to be 5.5 μM at a signal-to-noise ratio of 3.
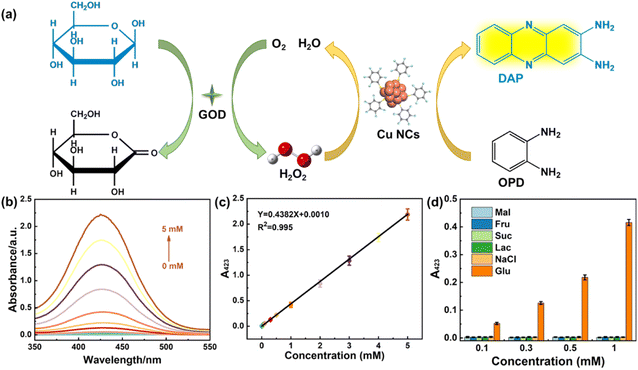 |
| Fig. 7 (a) Glucose sensing on the basis of a Cu NSs-OPD-GOD-catalyzed system (schematic). Optimization of pH for the peroxidase-like activity of Cu NSs. (b) Change in absorbance values monitored at 423 nm in the presence of different amounts of glucose. (c) Plot of A423 vs. glucose concentration. (d) Effect of various moities and glucose on the absorbance monitored at 423 nm. | |
In order to study the selectivity of the Cu NSs-GOD mixed enzyme-sensing platform, common moieties (Fru, Mal, Suc, Lac, and NaCl) coexisting with glucose in foods were investigated. Fig. 7d shows the absorbance at 423 nm in the presence of these analytes and glucose with different concentrations, which confirmed that a Cu NSs nano-enzyme-based sensor was highly selective toward glucose. Therefore, the proposed Cu NSs-OPD-GOD catalysis system was available for glucose sensing.
Practical application of the proposed sensing method was also investigated. Samples of sugary drinks spiked with glucose (0, 50, or 100 mM) were measured quantitatively. The results of these samples by the proposed Cu NSs-OPD-GOD enzyme catalytic method and HPLC (GB 5009.208-2023, China) are shown in Table 2. The detection results of a colorimetric method of this work were consistent with those for HPLC, and the recovery ratios were 94.4–103.6% with relative standard deviation (RSD) <2.3%. Therefore, the designed enzyme catalysis sensor based on assembled Cu NSs could recognize and detect glucose in real samples accurately.
Table 2 Detection of glucose in real samples
Real sample |
Glucose fortification (mM) |
Results (mean ± RSD, n = 3) |
Cu NSs-OPD-GOD (mM) |
HPLC (mM) |
Sample 1 |
0 |
105.2 ± 1.16 |
103.9 ± 0.98 |
50 |
152.4 ± 1.25 |
152.8 ± 1.04 |
100 |
206.1 ± 1.32 |
204.4 ± 1.09 |
Sample 2 |
0 |
134.5 ± 1.19 |
133.2 ± 1.01 |
50 |
186.3 ± 1.47 |
185.6 ± 1.13 |
100 |
231.8 ± 1.44 |
230.6 ± 1.21 |
Sample 3 |
0 |
200.1 ± 1.79 |
200.4 ± 1.17 |
50 |
248.2 ± 2.06 |
246.5 ± 1.36 |
100 |
297.6 ± 2.28 |
297.1 ± 1.42 |
Conclusions
Cu NCs were synthesized in a one-step method by mixing PFTP with a source of copper ions in ethanol. Cu NCs were self-assembled to NSs, which mimicked horseradish peroxidase. Using OPD as a substrate, the prepared Cu NSs showed good catalytic activity to the oxidation of OPD in the presence of H2O2. The apparent Km and Vmax for OPD were estimated to be 5.94 mM and 14.28 μM min−1, respectively. Then, a glucose sensor was constructed based on the Cu NSs-GOD mixed enzyme catalysis system. In the presence of glucose and GOD, H2O2 was generated and then the Cu NSs catalyzed the oxidation of OPD to DAP. Hence, glucose could be quantified by measuring the characteristic UV-vis absorbance of the oxidized product (DAP). This Cu NSs-OPD-GOD-catalyzed system showed a standard linear range of 10 μM to 5 mM towards glucose, and the limit of detection was 5.5 μM. The proposed sensor based on Cu NSs nano-enzymes was employed for colorimetric sensing of glucose in foods. This method could be applied to detect other analytes by changing GOD-catalyzed aerobic oxidation.
Author contributions
Ailing Han: writing (original draft), methodology, investigation, data analyses, and conceptualization. Yameng Zhao: investigation. Jianhu Wu: investigation and data analyses. Jianping Guo: conceptualization, data analyses, and writing (review and editing). Jianguo Xv: conceptualization and writing (review and editing).
Conflicts of interest
There are no conflicts of interest to declare.
Acknowledgements
This research was funded by the Natural Science Foundation of Shanxi Province (202203021212399, 202203021222222) and Natural Science Foundation of Shanxi Normal University (JCYJ2022021).
Notes and references
- G. Yang, Z. Wang, F. Du, F. Jiang, X. Yuan and J. Y. Ying, J. Am. Chem. Soc., 2023, 145, 11879–11898 CrossRef CAS PubMed.
- X. Liu, X. Cai and Y. Zhu, Acc. Chem. Res., 2023, 56, 1528–1538 CrossRef CAS PubMed.
- C. Zhang, M. Liang, C. Shao, Z. Li, X. Cao, Y. Wang, Y. Wu and S. Lu, ACS Appl. Bio Mater., 2023, 6, 1283–1293 CrossRef CAS PubMed.
- X. Kang, Y. Li, M. Zhu and R. Jin, Chem. Soc. Rev., 2020, 49, 6443–6514 RSC.
- M. Monti, M. F. Matus, S. Malola, A. Fortunelli, M. Aschi, M. Stener and H. Häkkinen, ACS Nano, 2023, 17, 11481–11491 CrossRef CAS PubMed.
- X. Zou, X. Kang and M. Zhu, Chem. Soc. Rev., 2023, 52, 5892–5967 RSC.
- P. D. Jadzinsky, G. Calero, C. J. Ackerson, D. A. Bushnell and R. D. Kornberg, Science, 2007, 318, 430–433 CrossRef CAS PubMed.
- X. Kang, H. Chong and M. Zhu, Nanoscale, 2018, 10, 10758–10834 RSC.
- J. Yan, B. K. Teo and N. Zheng, Acc. Chem. Res., 2018, 51, 3084–3093 CrossRef CAS PubMed.
- S. Zhuang, D. Chen, W. Fan, J. Yuan, L. Liao, Y. Zhao, J. Li, H. Deng, J. Yang, J. Yang and Z. Wu, Nano Lett., 2022, 22, 7144–7150 CrossRef CAS PubMed.
- X. Wei, H. Li, H. Li, Z. Zuo, F. Song, X. Kang and M. Zhu, J. Am. Chem. Soc., 2023, 145, 13750–13757 CrossRef CAS PubMed.
- T.-S. Zhang, W. Fei, N. Li, Y. Zhang, C. Xu, Q. Luo and M.-B. Li, Nano Lett., 2023, 23, 235–242 CrossRef CAS PubMed.
- Y. Wang, X.-K. Wan, L. Ren, H. Su, G. Li, S. Malola, S. Lin, Z. Tang, H. Häkkinen, B. K. Teo, Q.-M. Wang and N. Zheng, J. Am. Chem. Soc., 2016, 138, 3278–3281 CrossRef CAS PubMed.
- P. Ling, Q. Zhang, T. Cao and F. Gao, Angew. Chem., Int. Ed., 2018, 57, 6819–6824 CrossRef CAS PubMed.
- Q. Li, D. Yang, Q. Yin, W. Li and Y. Yang, ACS Appl. Nano Mater., 2022, 5, 1925–1934 CrossRef CAS.
- S. Shekhar, R. Sarker, P. Mahato, S. Agrawal and S. Mukherjee, Nanoscale, 2023, 15, 15368–15381 RSC.
- G. Yin, M. Nishikawa, Y. Nosaka, N. Srinivasan, D. Atarashi, E. Sakai and M. Miyauchi, ACS Nano, 2015, 9, 2111–2119 CrossRef CAS PubMed.
- D. Luo, Z. Zhang, G. Li, S. Cheng, S. Li, J. Li, R. Gao, M. Li, S. Sy, Y.-P. Deng, Y. Jiang, Y. Zhu, H. Dou, Y. Hu, A. Yu and Z. Chen, ACS Nano, 2020, 14, 4849–4860 CrossRef CAS PubMed.
- M. Hesari and Z. Ding, J. Am. Chem. Soc., 2021, 143, 19474–19485 CrossRef CAS PubMed.
- P. Asen, A. Esfandiar and M. Kazemi, ACS Appl. Nano Mater., 2022, 5, 13361–13372 CrossRef CAS.
- F. Gao, Y. Yang, W. Qiu, Z. Song, Q. Wang and L. Niu, ACS Appl. Nano Mater., 2021, 4, 8520–8529 CrossRef CAS.
- H. Teymourian, A. Barfidokht and J. Wang, Chem. Soc. Rev., 2020, 49, 7671–7709 RSC.
- I. Ahmed, N. Jiang, X. Shao, M. Elsherif, F. Alam, A. Salih, H. Butt and A. K. Yetisen, Sens. Diagn., 2022, 1, 1098–1125 RSC.
- R. Li, C. Wang, L. Gou, Y. Zhou, L. Peng, F. Liu and Y. Zhang, Analyst, 2023, 148, 5873–5881 RSC.
- A. Han, Y. Yang, X. Li, S. Hao, G. Fang, J. Liu and S. Wang, ACS Appl. Nano Mater., 2021, 4, 4129–4139 CrossRef CAS.
- Z. Wu, J. Liu, Y. Gao, H. Liu, T. Li, H. Zou, Z. Wang, K. Zhang, Y. Wang, H. Zhang and B. Yang, J. Am. Chem. Soc., 2015, 137, 12906–12913 CrossRef CAS PubMed.
- A. Han, L. Xiong, S. Hao, Y. Yang, X. Li, G. Fang, J. Liu, Y. Pei and S. Wang, Anal. Chem., 2018, 90, 9060–9067 CrossRef CAS PubMed.
- D. R. Huntley, J. Phys. Chem., 1992, 96, 4550–4558 CrossRef CAS.
- T. Higaki, Q. Li, M. Zhou, S. Zhao, Y. Li, S. Li and R. Jin, Acc. Chem. Res., 2018, 51, 2764–2773 CrossRef CAS PubMed.
- H. Qian, M. Zhu, Z. Wu and R. Jin, Acc. Chem. Res., 2012, 45, 1470–1479 CrossRef CAS PubMed.
- Q. Yao, T. Chen, X. Yuan and J. Xie, Acc. Chem. Res., 2018, 51, 1338–1348 CrossRef CAS PubMed.
- D. Xu, Z. Shen, G. Wang, L. Wei, X. Gao, H. Dong, G. Wang, X. Sun, F. Li and Y. Guo, Sens. Actuators, B, 2023, 376, 133024 CrossRef CAS.
|
This journal is © The Royal Society of Chemistry 2024 |
Click here to see how this site uses Cookies. View our privacy policy here.