DOI:
10.1039/D3RA08378K
(Paper)
RSC Adv., 2024,
14, 11541-11556
Amaranthus hybridus waste solid biofuel: comparative and machine learning studies
Received
8th December 2023
, Accepted 26th March 2024
First published on 10th April 2024
Abstract
The diminishing supply of fossil fuels, their detrimental environmental effects, and the challenges associated with the disposal of agro-waste necessitated the development of renewable and sustainable alternative energy sources. This study aims at developing bio-briquettes from Amaranthus hybridus waste, with cassava starch as a binder; both are agricultural wastes. Before and following delignification, alkali-treated Amaranthus hybridus (TAHB) and untreated (UAHB) briquettes were evaluated in terms of combustion and physicochemical parameters. FTIR and SEM were utilized to monitor the morphological transformation and bond restructuring of TAHB and UAHB samples. EDXRF was used to assess the Potential Toxic Elements (PTEs) composition and environmental friendliness of both TAHB and UAHB. Furthermore, Adaptive Neuro-Fuzzy Inference System (ANFIS) and fuzzy c-means (FCM) clustering machine learning models were used to optimize the production process and predict the efficiency of bio-briquettes. After delignification, a lower lignin value of 11.47 ± 0.00% in TAHB compared to 12.31 ± 0.01% (UAHB) was recorded. Calorific values of 10.43 ± 0.25 MJ kg−1 (UAHB) and 12.53 ± 0.30 MJ kg−1 (TAHB) were recorded at p < 0.05. EDXRF results showed a difference of 0.016% in Pb concentration in both samples. SEM reveals morphological restructuring, while FTIR reveals a 4 cm−1 difference in the C–O stretch. The root mean square error (RMSE), mean absolute percentage error (MAPE), and mean absolute error (MAE) gave values of 0.0249, 2.104, and, 0.0249; (MAE, training) and 0.0223 (MAE, testing) respectively. This shows that the model's predictions match the reality, thereby suggesting a strong agreement between the predicted and experimental data. The finding of this study shows that delignification-disruption improved the solid biofuel's ability to burn cleanly and sustainably.
1 Introduction
Waste management and disposal is one of the major environmental challenges faced by several countries around the globe but with varying levels of severity.1 This is hinged on major factors including, the degree of industrialization, urbanization, and population growth. Due to the nature and toxicity of the waste, the categories or sorts of garbage produced in industrialized countries greatly differ from those produced in developing ones. This fact complicates an already challenging waste disposal process due to the bulky nature of the waste generated as well as the unpleasant odors due to the decomposition of such waste.2 However, when properly investigated, this threat encapsulates certain potentials that, when compared to the challenges it presents, vastly surpass them.
Furthermore, urbanization, industrialization, and the surge in global population have increased the need for energy resources for industrial and home uses. This have overstretched the “energy cycle” that the world is exploring at the moment. Studies have shown an alarming rate at which the global non-renewable energy source (which is majorly fossils) is diminishing daily in its ability to meet the world energy demand.3–5 The recent time has witnessed the emergence of biomass material (plant and animal waste) as one of the natural energy storage systems offered by nature.1,6 Agro-wastes, which can be derived from either plant or animal sources (biomass), are resources that are easily accessible. Agro-industrial wastes, municipal solid wastes, fuels obtained from refuse, and forest residues are only a few examples of the various biomass energy supplies.4,7,8
The management of these wastes (transportation, storage, and handling problems, direct burning of agricultural leftovers) may be costly and detrimental to the environment. However, proper utilization through the development and adoption of feasible methods of turning biomass into secondary fuels with superior properties to the parent material for energy purposes is a symbiotic approach for mitigating the challenges associated with the disposal of such waste thereby providing a solution to the global energy demand and turning waste into wealth.9–11
One of the best emerging techniques for turning various waste materials into solid biofuels for energy demands is briquette production technology. Because of this, biofuel briquettes provide a long-term solution for effectively utilizing agricultural and/or other biomass waste.12 According to earlier studies, municipal solid waste feedstocks can pelletize or mould into briquettes for use in energy production.13
Briquettes made from biomass might be a practical way to address issues with solid waste and a strong reliance on fuel wood in most developing nations. Furthermore, the wide availability of enormous water bodies and excellent fertile land areas for agricultural purposes has placed Nigeria ranked among the West African countries with good agricultural practices. However, the waste generated as a result of poor storage facilities or techniques of perishable and by-products of agricultural products which include, vegetables, corn cobs and stalks, coconut husks, cassava peels, rice husks, etc., might be a challenge for handling agricultural waste.14–16 It should be noted that these wastes have enormous potential when properly utilized as feedstock and raw materials for the generation of alternative sources of renewable energy.17 The aforementioned information is in synchrony with the United Nations report which proposed that agricultural wastes might be probable solution to the world's energy crisis by potentially substituting fossil fuels and ameliorating the emissions of greenhouse gases.18
Amaranthus species commonly known as Tete abalaye in the western part of Nigeria has been regarded as one of the most important vegetables in some parts of Nigeria due to its nutritional and nutraceutical values. Amaranthus hybridus is a commercial amaranth widely cultivated all over Nigeria. It is a robust, heavy-branching plant that can reach a height of one meter or more in permissive environments. The leaves are broad, ovate, and closely packed on the main stem and branches making it a typical leafy vegetable.19 Amaranthus species are excellent sources of nutritional fiber, vitamins, minerals, beta-carotene, and proteins. Biologically active substances with anti-diabetic, anti-hyperlipidemic, and anti-hypercholesterolemic properties as well as antioxidant and antibacterial activity have been reported in this plant species.20 However, it is a perishable plant. Due to poor storage facilities large quantities of this vegetable end up as waste resulting in economic loss to farmers and constituting environmental problems during decomposition.
The major chemical components of plants and agro-waste that are responsible and/or serve as the main energy source include cellulose, hemicelluloses, and lignin.14,18,21 The development of briquetting technology is apt to fully harness this potential. This is a promising agglomeration process for improving the energy potential of biomass products by compacting their loosely held matrix to enhance the combustibility, handling, and physicochemical properties of the solid biofuel.22 However, research has shown that chemical treatment can further enhance the biomass's quality, producing an environmentally benign biofuel with a slow combustibility property and superior heating capability. Treatment of agricultural waste with NaOH, CaO, ammonia, and urea before densification through either pelletizing or briquetting fosters the aforementioned properties of solid biofuels.
Studies have proven that the quality of biofuels from biomass (plant and animal) sources can be enhanced and improved by using sodium hydroxide (NaOH) as a pretreatment for delignification.23–26 In addition, previous studies have reported the NaOH pre-treatment of cabbage waste,8 Celosia argentea27 and acid-pretreated bean chaff28 for briquette production. Therefore, it is imperative to assess the effect of alkaline pre-treatment on the physico-chemical, combustibility, and environmentally friendly nature of solid biofuel made from Amaranthus hybridus, with an emphasis on (i) the utilization of Amaranthus hybridus waste in order to produce solid biofuel. (ii) The influence of NaOH pretreatment on the produced solid biofuel characteristic. (iii) The utilization of cassava crumps (agro-waste) as the binder and (iv) to optimize the production process and predict the efficiency of bio-briquettes using machine learning models. The capability of machine learning models to handle non-linearity in data such as biofuel data makes them ideal for this study. To evaluate the effect of delignification on significant properties of the biofuel, machine learning could assist by providing useful insights into the complex relationship between several input variables and the fuel properties while unveiling vital and novel patterns in the experimental data which are critical to the energetic properties of the biofuel.
In this study, the Adaptive neuro-fuzzy inference system (ANFIS) model has been deployed to give useful insights into the impacts of delignification on the significant properties and combustion parameters of Amaranthus hybridus waste solid biofuel. The model analyses the impact of the delignification of biofuels to improve their eco-friendliness and properties, especially on two significant properties namely calorific value and fixed carbon. These findings indicate delignified biofuel as a sustainable and environmentally friendly energy source with improved performance and lower environmental effects than raw biomass. To the best of knowledge, there are currently no reports covering this area. Therefore, the article in this field is necessary to evaluate their performances to help the researcher in the field understand the current research in the implementation of Amaranthus hybridus waste as solid biofuel. This novel research provides insight into exploration these wastes for directing specific research for large/industrial-scale applications.
2 Methodology
2.1 Alkaline pre-treatment
Amaranthus hybridus wastes obtained in Ibadan, Southwest Nigeria, were given an alkaline surface modification by soaking them in a % NaOH solution. The soaking process lasted 3 hours at room temperature. A 15
:
1 ratio of biomass to alkaline solution was employed.29 After that, the samples were dried for 48 hours at ambient temperature, and overnight at 60 °C in an oven.
2.2 Lignocellulosic composition analysis
The modified Chesson method was used to analyze the lignocellulosic components, including cellulose and lignin.30 The mixture with the following components: 1 g of dried sample (a) was added to 150 mL of demineralized water, the mixture was heated for 1 h in an oil bath at a temperature of 100 °C. then filtered. The filtrate was discarded, and the residue was washed with hot demineralized water (300 mL) and dried in the oven with periodic weighing until a constant weight was noted (b). Afterwards, the residue was mixed with 150 mL of 1 N H2SO4 and heated for 1 h in an oil bath at 100 °C. The mixture was washed with 300 mL demineralized water and filtered and dried (c). The dried residue was soaked with 10 mL of 72% H2SO4 at room temperature for 4 h. Thereafter, a 150 mL of 1 N H2SO4 was added into the mixture and refluxed in the oil bath for 1 h. The solid was washed with 400 mL of water demineralized, heated in the oven at 105 °C and weighed until a constant weight was noted (d). Finally, the solid was heated to ash and weighed (e). The percentage of hemicellulose, cellulose and lignin was determined as follows: |
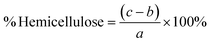 | (1) |
|
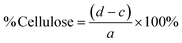 | (2) |
|
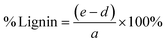 | (3) |
where; a – mass of dried sample. b – weight of residue after treated with hot demineralized water. c – weight of residue after treated with 1.0 N H2SO4. d – weight of residue after treated with 72% H2SO4 + 1.0 N H2SO4 + demineralized water. e – the residual ash weight.
2.3 Briquette production
A briquetting machine powered by a 10-ton hydraulic jack and equipped with a press chamber with a cylindrical mold for slurry containment was used to fabricate the solid biofuel (briquettes). The binder, cassava starch, was purchased in Abeokuta, Ogun state. Based on earlier research that showed starch-based binders' function best at a 4
:
1 biomass/binder ratio,31,32 the dried sample of alkali-treated and untreated pulverized Amaranthus hybridus waste was weighed into a mixing vessel. To produce a slurry, the components were thoroughly combined with a small amount of water. These were densified after being charged into the mold to produce the briquette.
2.4 Determination of physicochemical and combustion properties of briquettes
The dried Amaranthus hybridus wastes and briquette samples were analyzed following ASTM D-3172 (2002) guidelines for ash content, moisture content, fixed carbon, and volatile matter. Using a LECO AC 350 Bomb calorimeter and following standard protocol, the sample's calorific value was calculated. According to DIN 52
182 and 51
731 requirements, the bulk density of the sample was determined using.33 The D 3173-878 specifications were followed in the ELE Tritest 50 compression machine's evaluation of the briquette sample's compressive strength. To estimate the time needed to boil a specific volume of water, 500 g of the Amaranthus hybridus waste and the resulting briquettes were used to heat 500 mL of water in a thermometer-fitted jar. The time spent waiting for the water to reach boiling point was then recorded under similar conditions.
2.5 Elemental composition
The briquette samples are composed of carbon (C), hydrogen (H), and oxygen (O), which are the typical organic constituents. These were estimated at a 95% confidence level using eqn (4)–(6), respectively. This evaluation is mostly based on the findings of the proximate analysis of the biomass sample34 |
C = 0.637FC + 0.455VM
| (4) |
|
H = 0.052FC + 0.062VM
| (5) |
|
O = 0.304FC + 0.476VM
| (6) |
where FC: fixed carbon and VM: volatile matter.
2.6 Energy dispersive X-ray fluorescence
The following experimental parameters were used for the EDXRF measurement: air cooling, side window tube, high voltage tube with a maximum voltage of 30 kV, emission current with a maximum of 1 mA, and power with a maximum of 9 W. A Si (Li) detector was used to measure the energy of the X-rays the sample emits, and a pulse height analyzer processed the data (Jyothsna et al., 2020).
2.7 FTIR (fourier transform infrared spectroscopy)
The infrared spectra of the samples was obtained using an FTIR, AVATAR 330 and were analyzed at the Chemistry Department of Umaru Musa Yar'adua University in Katsina State, Nigeria.
2.8 Scanning Electron Microscopy
Scanning Electron Microscopy (SEM) was used to examine the morphology of both untreated and treated samples. They were turned into capsules, coated with palladium (Pd) at 30 mA, and examined using a JEOLJFC-5510LV scanning electron microscope.
2.9 Modelling approach
2.9.1 Adaptive neuro-fuzzy inference model. Jang35 initially introduced the ANFIS. This is the combination of neural networks with fuzzy logic to create fuzzy inference systems that are automatically generated from training data. In order to minimize output error, ANFIS employs a backpropagation technique to modify the system's membership functions and weights.36 This process is carried on repeatedly until the error decreases or a set number of iterations have been completed. It is capable of handling challenging nonlinear systems and approximate nonlinear functions, thanks to fuzzy logic and linear functions.37 The learnability and adaptability of ANFIS is advantageous for dynamic systems. Owing to fuzzy logic's ability to handle uncertainty, ANFIS can manage noisy data and yet solve classification and regression problems with accurate or missing input data.38 The creation of an ANFIS model involves several steps. Incoming data must be preprocessed for the ANFIS model. The ANFIS model is trained using a technique called backpropagation. The membership functions and input data-based rules are chosen during this time. Finally, a different set of data is used to evaluate the ANFIS model. The ANFIS model's membership function selection and rule generation are challenging. The performance of the ANFIS model may be negatively impacted by using incorrect membership functions or by developing too few or too many rules.ANFIS parameters may be non-linear in the premise section and linear in the consequent component.39 Eqn (7) and (8) illustrate a rule-based system with two inputs, a and b and one output in a Fuzzy Inference System.
|
Rule 1: If a is A1 and b is B1, F1 = X1a + Y1b + Z1
| (7) |
|
Rule 2: If a is A2 and b is B2, F2 = X2a + Y2b + Z2.
| (8) |
where
A1,
A2,
B1,
B2 represents the membership functions while
a and
b are input parameters.
F1 and
F2 are outputs acquired from the system, while
X,
Y, and
Z are nodal consequent parameters. Five functional layers make up the ANFIS architecture as presented in
Fig. 1. In the input layer, the system receives data as
eqn (9) depicts.
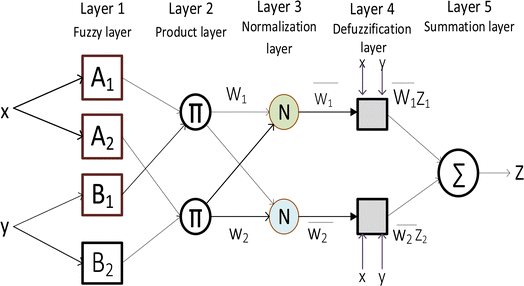 |
| Fig. 1 Architectural framework of ANFIS. | |
The membership functions transform input data into fuzzy sets in the second layer as in eqn (10).
|
Oi2 = wi = μAi(a) × μBi(b), i = 1, 2
| (10) |
Based on training data, the product rule in the third layer generates fuzzy rules as follows.
|
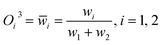 | (11) |
In the fourth layer, fuzzy output is defuzzified. The output,
Oi4, is expressed as given in
eqn (12) |
Oi4 = if = i(sia + rib + ti)
| (12) |
Finally, the system's output is produced in the fifth layer. Eqn (13) mathematically illustrates each layer respectively.
|
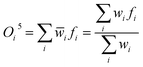 | (13) |
Clustering is an important step in the process of building ANFIS model. In this study, fuzzy c-means (FCM) clustering is used for dividing a data set into clusters, where each data point to some extent belongs to each cluster. Iterative minimization techniques are used in this method to cluster a finite set of data, X = (x1, x2…….xm), into clusters of integers, where xi, i = 1,…m is a l-dimensional vector.40 The fuzzy c-means clustering is advised for applications where speed is crucial because of its capacity to accelerate computations. The objective FCM clustering function that minimizes the distance center is given by eqn (11).41 In image segmentation, bioinformatics, and finance, FCM is frequently utilized. To segment images by pixel values, FCM has been utilized in image segmentation. In bioinformatics, FCM groups gene expression data to find patterns and connections between genes and biological processes. Financial time series data have been divided into bull and bear markets using FCM.
2.10 Statistical analysis
Graph Pad Prism® (version 6.04) was used to perform a T-test and calculate the mean of all the parameters that were investigated. All data were shown as mean ± SD (standard deviation), with the level of significance set at P < 0.05.
3 Results and discussion
3.1 Delignification-disruption of the fiber matrix
Delignification-disruption techniques are essential for enhancing the processability and quality of plant-based products. According to studies, biomass can be used to make solid biofuels from either raw plant and animal waste products through pelletization or briquetting.10,42,43 It has been established that chemical modification enhances the quality of such solid biofuel (combustion, physicochemical properties, and environmental impact) through the disruption of the biomass matrix, which is demonstrated by a reduction in the biomass's lignin concentration and a fall in the matrix's degree of crystallinity and polymerization.44
The scheme showing the disruption and lignocellulosic content of UAHB and TAHB samples are shown in Fig. 2a–g. The reported lignin contents of both UAHB and TAHB showed a discernible difference that was significant (p = 0.05). TAHB had a mean lignin value of 11.47 ± 0.00% whereas UAHB had a mean lignin value of 12.31 ± 0.01%. As indicated in Fig. 2g, the hemicellulose content of UAHB and TAHB had mean values of 4.24 ± 0.00 and 3.21 ± 0.00, respectively, at p = 0.0001. Furthermore, pre-treating the biomass matrix with NaOH increases the cellulose support of the fiber matrix (Fig. 2g), where a mean value of 12.55 ± 0.01% was reported for TAHB as opposed to 11.13 ± 0.00% for UAHB. This is consistent with our earlier investigation into Celosia argentea waste, in which a mean value of 12.87 ± 0.10% was noted in the NaOH-treated biomass sample.27
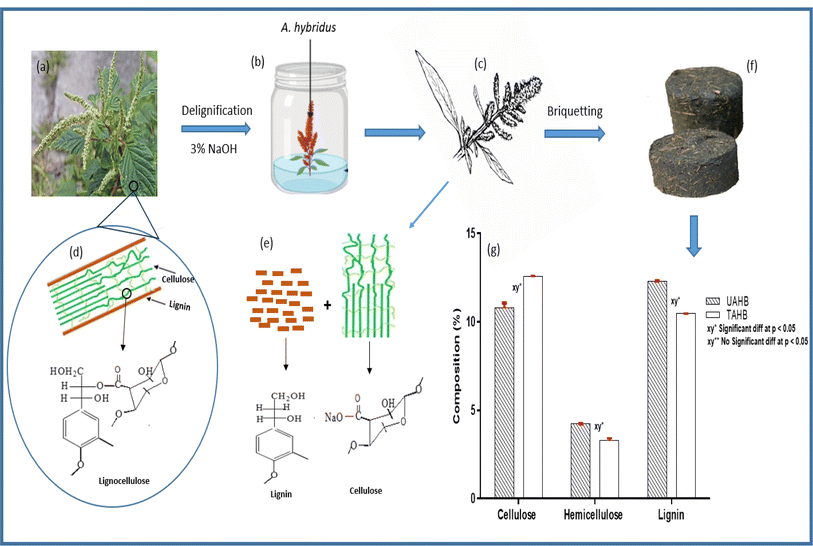 |
| Fig. 2 The scheme showing (a) Fresh Amaranthus hybridus plant (b) delignification of the fiber matrix of untreated Amaranthus hybridus (c) dried Amaranthus hybridus waste (d) delignification of lignocellulose (e) disrupted lignocellulose to lignin and cellulose (f) treated Amaranthus hybridus briquettes and (g) lignocellulose composition of treated Amaranthus hybridus briquettes. | |
3.2 Ultimate analysis
The ultimate analysis is a method for estimating the weight percentages of carbon (C), oxygen (O), and hydrogen (H) in the unprocessed, UAHB, and NaOH-treated (TAHB) samples of A. hybridus biomass, as presented in Table 2. Oxygen combines with carbon and hydrogen to create CO, H2O, phenols (OH), and other compounds. Studies have shown that oxygen has a detrimental effect on solid biofuel combustion properties.21 A marked significant difference at p = 0.0001 was noted in the oxygen value as observed in Table 2, in which a mean average value of 42.59 ± 0.00 wt% was noted in UAHB compared to the treated counterpart, which was considerably lower, 40.34 ± 0.02 wt% (TAHB). This is due to the disruption of the fiber matrix. Furthermore, recorded mean carbon content values of 42.59 0.00 wt% and 40.34 ± 0.02% wt% were noted for UAHB and TAHB respectively, at a significance value of p < 0.05, as shown in Table 2. Moreover, the average recorded hydrogen content of TAHB and UAHB were 5.76 ± 0.03 and 5.80 ± 0.00 wt% respectively. However, based on the p-value, (p = 0.2835) no significant difference was noted. However, there is a slight difference in the mean value of the treated and untreated samples, which shows the impact of alkali pretreatment on the hydrogen content of the treated samples. The finding of this research is in tandem with our previous study.27 This shows that the delignification had an appreciable impact on the biomass samples as presented in Table 2.
Table 2 Ultimate analysis of untreated Amaranthus hybridus briquettes and treated Amaranthus hybridus briquettesa
Parameters (wt%) |
UAHB |
TAHB |
p-Value |
Data are means of three replicates (n = 3) ± SD using graph pad, prism, and t-test. UAHB; untreated Amaranthus hybridus briquette; TAHB: treated Amaranthus hybridus briquette; * significant difference; ** no significant difference. |
Hydrogen |
5.80 ± 0.00** |
5.76 ± 0.03** |
0.2835 |
Oxygen |
42.59 ± 0.00* |
40.34 ± 0.02* |
0.0001 |
Carbon |
47.90 ± 0.00* |
49.86 ± 0.08* |
0.0001 |
3.3 Physicochemical and combustion properties
The following physical characteristics; density, compressive strength (CS), ignition propagation (IP) combustibility test (CT), and time taken for UAHB and TAHB samples to burn to ashes are shown in Table 3, These characteristics are crucial for deciding a biofuel's performance. The compressive strength and density factors have been reported to influence the porosity and moisture content of the bio-briquette. This parameter controls the briquette's tact and durability during handling and long-term storage. It has an impact on the calorific value of such briquettes as well as the IP and the amount of time needed to burn to ashes.43 The FC and CV values make this clear. Both UAHB and TAHB had mean densities of 0.58 ± 0.02 and 0.66 ± 0.03 g cm−3, respectively. This demonstrates that TAHB is denser than UAHB, and this relationship was shown to be direct and inversely correlated with the recorded CS value, which was observed to be higher for TAHB (0.63 ± 0.01 N mm−2). As opposed to 1.11 0.01 cm s−1 observed in UAHB, 1.41 0.02 cm s−1 is recorded for the IP of TAHB. This low combustibility test value and the noticeably different times needed for both UAHB and TAHB to burn to ashes can be attributed to the tackiness in the disrupted biomass fiber matrix caused by densification and delignification of TAHB at p < 0.05 as shown in Table 3.
Table 3 Physical characteristics and combustion properties of untreated Amaranthus hybridus briquettes and treated Amaranthus hybridus briquettesa
Sample parameters |
UAHB |
TAHB |
p-Value |
Data are means of three replicates (n = 3) ± SD using graph pad, prism, and t-test. UAHB; untreated Amaranthus hybridus briquette; TAHB: treated Amaranthus hybridus briquette; *significant difference; **no significant difference. |
Bulk density (g cm−3) |
0.58 ± 0.02** |
0.66 ± 0.03** |
0.1012 |
Compressive strength (N mm−2) |
0.59 ± 0.01* |
0.63 ± 0.01* |
0.0314 |
Ignition propagation (cm s−1) |
1.11 ± 0.01* |
1.41 ± 0.02* |
0.0003 |
Combustibility (g min−1) |
5.97 ± 0.00* |
5.11 ± 0.10* |
0.0008 |
Time is taken to burn to ashes (min) |
10.62 ± 0.20* |
12.66 ± 0.08* |
0.0007 |
Thus, according to this findings, the heating and combustibility properties of TAHB briquette samples were enhanced by delignification when compared to UAHB briquette samples. The quantity of internal energy retained by a solid biofuel in the form of heat is what determines its acceptance and use.45,46 The heating value is the main combustion parameter that illustrates the effects of modifications by the enormous heat energy a potential any solid bio-briquette or biofuel material can produce.
3.4 Calorific value
The potential of any biofuel or bio-briquette material to be utilized as a source of energy generation is hinged on the amount of heat energy it can produce when burned, which is otherwise known as its calorific value.47 The criterion for adopting any biomass material for energy purposes depends on the stored energy as heat.11,18 Furthermore, the calorific value depends on the type of biomass material used, its moisture content, the compression process, and any additives or binders included in the briquette formulation. Generally, in comparison to coal briquettes, biomass briquettes formed from sawdust, wood chips, agricultural waste, or charcoal have a lower calorific value, the calorific value is typically measured in units of energy per unit of mass.
Thermal energy generated by any biomass material as an energy source depends on the composition, density, and carbon content of the briquette. A marked significant difference at a p-value of 0.0001 was observed in the recorded value of UAHB when compared with TAHB. A recorded mean value of 10.43 ± 0.25 MJ kg−1 was noted for UCAB compared to 12.53 ± 0.30 MJ kg−1 observed in TAHB at p = 0.0002 as shown in Fig. 3. The recorded calorific value is in tandem with the previously explained recorded high FC value of TAHB, higher carbon value of 49.86 ± 0.08 wt% in the ultimate analysis, and approximately 1400 carbon counts for TAHB as against 900 counts in UAHB noted in the EDXRF of the briquette samples as shown in Fig. 4a and b. This shows that any solid biofuel's thermal efficiency is improved by particle compacting through densification and alkali pretreatment for energy-generating purposes. The high calorific value observed in TAHB could be due to the low moisture content, high fixed carbon, and high compressive strength.42
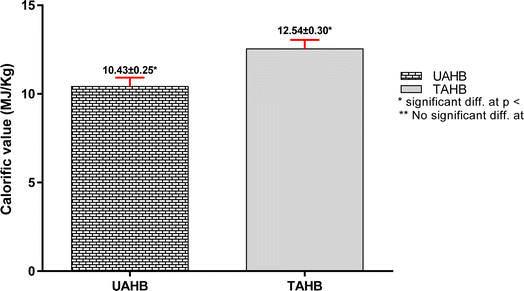 |
| Fig. 3 Calorific value of untreated Amaranthus hybridus briquettes and treated Amaranthus hybridus briquettes. | |
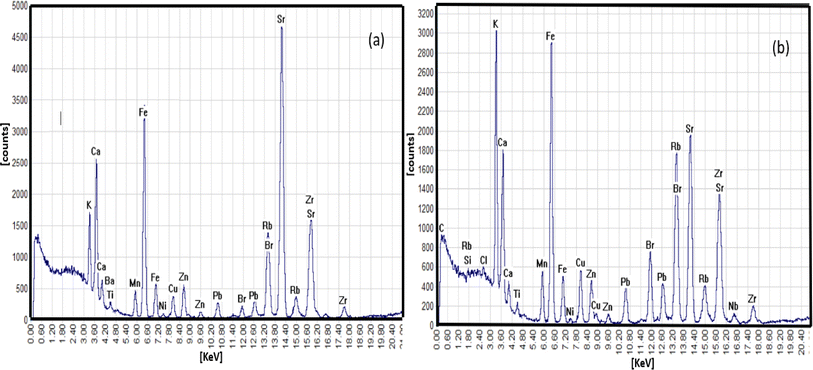 |
| Fig. 4 The EDXRF spectrum of (a) treated Amaranthus hybridus briquettes (TAHB) and (b) untreated Amaranthus hybridus briquettes (UAHB). | |
3.5 Proximate analysis
Raw Amaranthus hybridus has more volatile matter, moisture, and ash than treated Amaranthus hybridus, but treated Amaranthus hybridus contains more fixed carbon than raw Amaranthus hybridus. This can be attributed to delignification. Furthermore, amounts of volatile matter recorded in this study were observed to be less than those found in the previous studies which recorded 82.3 wt% for teak dust48 and 79.0 wt% for Switchgrass.49
Table 4 reveals a significant moisture content difference between UAHB and TAHB at p < 0.05 with mean values for UAHB and TAHB of 14.19 ± 0.01% and 13.25 ± 0.01% respectively. The residual or stored energy needed for full combustion of the fuel for water evaporation may be significantly affected by moisture content values larger than 20%. This could have a negative effect on the shelf life of such a bio-briquette or biofuel.50,51 High ash concentration is a sign that can be used to estimate a briquette's rate of dust emission.
Table 4 Proximate analysis of untreated Amaranthus hybridus briquettes and treated Amaranthus hybridus briquettesa
Parameter (%) |
UAHB |
TAHB |
p-value |
Data are means of three replicates (n = 3) ± SD using Graph Pad, Prism, and t-test. UAHB; Untreated Amaranthus hybridus Briquette; TAHB: Treated Amaranthus hybridus Briquette Ash: ash content; MC: moisture content; VM: volatile matter; FC: fixed carbon; *significant difference; **no significant difference. |
MC |
14.19 ± 0.01* |
13.25 ± 0.01* |
0.0001* |
Ash |
2.82 ± 0.01* |
2.55 ± 0.02* |
0.0001* |
VM |
71.34 ± 0.02* |
68.43 ± 0.00* |
0.0002* |
FC |
25.77 ± 0.01* |
28.92 ± 0.01* |
0.0001* |
Its typical value might range from 5 to 20%. Any numbers higher than this could result in environmental pollution.52 Mean ash values of 2.55 ± 0.02% and 2.82 ± 0.01% were noted in TAHB and UAHB respectively. A lower ash content value indicates that the briquettes are of higher quality. This implies that, as shown in Table 4, sample TAHB with a lower ash content value is considered to be of higher quality than UAHB. A significant difference of 0.0002 was observed in the recorded mean volatile matter values of 68.43 ± 0.00% and 71.34 ± 0.02% for both TAHB and UAHB at P < 0.05 respectively as presented in Table 4. Additionally, the volatile matter content affects how combustible briquette fuel is. As long as a low ash level is documented, a fuel briquette's combustibility increases with increasing volatile matter.25,53 The fixed carbon shows a significant difference with a p-value of 0.0001, in which 25.77 ± 0.01% was recorded for UAHB compared to TAHB's recorded value of 28.92 ± 0.01%. The lower value recorded for UAHB implies a prolonged cooking time at a very low rate of heat release. 0.01% compared to the recorded value of 28.92 ± 0.01% in TAHB.43
3.6 Eco-friendly nature and elemental composition of briquette
The EDXRF diffractograms of the briquettes were presented in Fig. 4a and b, and Table 5 to show the percentage of Potentially Toxic Elements (PTEs) in UAHB and TAHB which reveals a total of 16 elements in the range of macro, micro, trace and radioactive. The PTE studies have revealed that the elements such as Cu, Pb, As, Cr, etc., are of great concern owing to their high toxicity and the potential risk they pose to both urban ecosystems and human health.54,55 PTEs are chemically stable and toxic at high concentrations, which has been demonstrated over time by numerous research and may contribute to ophthalmic, respiratory, and dermatological disorders.8,27 As shown in Table 5 below, the percentage concentration of iron (Fe), copper (Cu), zinc (Zn) nickel (Ni), tungsten (W), bromine (Br), etc. are conspicuously negligible for both the UAHB and TAHB. However, the percentage concentration of phosphorous (P), chlorine (Cl), lead (Pb), sulphur (S), and silicon (Si) which are 0.389, 0.384, 2.120, 0.229 and 0.790%, respectively in UCAB were observed to have considerably reduced to 0.331 (P), 0.103 (Cl), 2.004 (Pb), 0.150 (S) and 0220% (Si), respectively in TAHB as shown in Table 5. In general, it was observed that when compared to World Health Organization (WHO) and World Surface Rock Average (WSRA) standard values, the PTE content of UHAB and TAHB were remarkably low56,57 This implies that the treatment of the biomass sample with NaOH improves both the combustibility and physicochemical properties of the briquettes, making it environmentally friendly.
Table 5 Percentage concentration of potential toxic elements in untreated Amaranthus hybridus briquettes and treated Amaranthus hybridus briquettes
S/n |
Elements |
Concentration (%) |
UAHB |
TAHB |
Difference |
1 |
Fe |
0.154 |
0.153 |
0.001 |
2 |
Cu |
0.007 |
0.005 |
0.002 |
3 |
Ni |
0.004 |
0.004 |
0.000 |
4 |
Zn |
0.010 |
0.009 |
0.001 |
5 |
Al |
0.058 |
0.042 |
0.016 |
6 |
Mg |
0.118 |
0.163 |
−0.045 |
7 |
S |
0.229 |
0.150 |
0.079 |
8 |
P |
0.389 |
0.331 |
0.058 |
9 |
Mn |
0.049 |
0.040 |
0.009 |
10 |
Rb |
0.022 |
0.011 |
0.011 |
11 |
Br |
0.019 |
0.005 |
0.014 |
12 |
Cl |
0.384 |
0.103 |
0.278 |
13 |
W |
0.036 |
0.036 |
0.000 |
14 |
Ba |
0.873 |
0.997 |
−0.124 |
15 |
Pb |
2.120 |
2.004 |
0.016 |
16 |
Si |
0.790 |
0.220 |
0.570 |
3.7 Surface chemistry and morphological characterization of solid biofuel
The FTIR and SEM are used to monitor the probable change that could result in Amaranthus hybridus fiber matrix surface due to alkali (NaOH) pretreatment of the biomass as shown in Fig. 5a and b respectively.
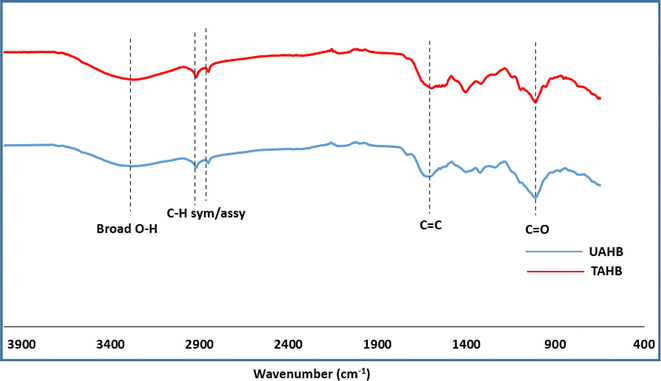 |
| Fig. 5 FTIR spectra of untreated Amaranthus hybridus briquettes and treated Amaranthus hybridus briquettes. | |
3.7.1 FTIR analysis. This was employed to monitor the appearance of the structure change seen in UAHB and TAHB presented in Fig. 5. The most informative vibrational frequencies of importance are the υO–H group attributed to hydrogen-bonded species whilst the υC–O stretch is attributed to an ester and a straight chain (aliphatic) –O–C stretch in cellulose, hemicellulose, and lignin. O–H stretch was observed in both UAHB and TAHB as shown in Table 6, but there is a bathochromic (red) shift i.e., decrease in the vibrational frequency of UAHB from 3280 cm−1 to 3272 cm−1 in TAHB, suggesting that the hydrogen bond in the fiber is broken via the alkaline treatment provided by NaOH. This observation can predict the density of the briquettes, since, a higher wavenumber is an indication of a reduction in the mass of a molecule because frequency of vibration is inversely proportional to the mass of a vibrating molecule58 Thus, the reduction in vibrational frequency in TAHB (red shift) suggests a denser briquette. Furthermore, the υC–O stretch which is a characteristic absorption band of aliphatic υP–O–C stretch and that of an ester also shows a red shift (decrease in wavenumber) from 1010 cm−1 in UAHB to 1006 cm−1 in TAHB. This reduction suggests lignin content reduction of the alkaline treated sample, TAHB as reported by.59,60
Table 6 The FTIR analysis of untreated Amaranthus hybridus briquettes and treated Amaranthus hybridus briquettes
Absorbance value range (cm−1) |
UAHB (cm−1) |
TAHB (cm−1) |
Difference (cm−1) |
Assignment |
Characteristic |
3570–3200 |
3280 |
3272 |
8 |
O–H |
Broad |
2935–2915 |
2914 |
2914 |
— |
C–H |
Methylene, assy/symstrech |
2865–2845 |
2851 |
2847 |
4 |
C–H |
Sp3 stretch |
1650–1566 |
1617 |
1580 |
37 |
C C |
Cyclo alkene |
1450–1335 |
1394 |
1397 |
3 |
C–H |
Bending vibration |
1055–1000 |
1010 |
1006 |
4 |
C–O |
C–O stretch, cyclohexane, ring vibration or ester |
3.7.2 Morphological study of briquette. The SEM micrographs aligned in agreement with the predictions of the FTIR. The SEM micrographs of UAHB and TAHB (Fig. 6) are comparable in morphology with homogeneous and uniform size of fibrous material, only that a smooth surface was observed in UAHB due to the abundance of hemicellulose and lignin. However, a rough, tacked, or compacted surface was observed in TAHB. This new surface morphology could be linked to NaOH permeation into the hemicellulose complex in the treated biomass, resulting in the rupturing of the biomass matrix as a result of breakage in the ester linkage of the biomass, resulting in the formation of a highly porous fiber matrix which when compressed resulted into a high density compacted solid biofuel sample. As a result, the fibers are held tightly for improved compaction, highly porous with small particle sizes resulting in high calorific value due to an increase in the fixed carbon content and low ash content. SEM result is in agreement with the EDXRF, FTIR, and the ultimate analysis, as earlier discussed in this study, thereby suggesting that alkaline pre-treatment of the biomass material enhanced the combustibility profile of the solid biofuel waste of Amaranthus hybridus.
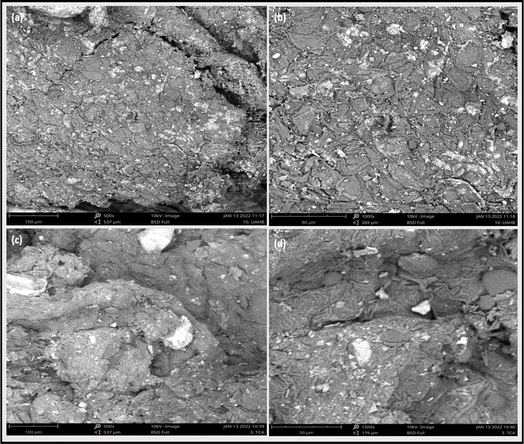 |
| Fig. 6 SEM micrographs of (a) and (b); untreated Amaranthus hybridus briquettes (c) and (d); treated Amaranthus hybridus briquettes. | |
3.8 Performance evaluation result of the FCM–ANFIS model
This section presents the performance of the ANFIS model based on selected statistical metrics. The pattern of the dataset and interactions would be revealed by the ANFIS–FCM model, perhaps resulting in new insights and a better understanding of the delignification process. The number of clusters is a significant hyper-parameter of the FCM–clustered ANFIS model which influences its performance.61–64 The performance of different cluster numbers of FCM clustering was evaluated between 2 and 6, in predicting the calorific value and fixed carbon of the Amaranthus hybridus waste solid biofuel. The FCM–ANFIS model improved marginally as the cluster numbers increased from 2–6 above which a significant decline was observed in the performance as the numbers of clusters increased above 6. This outcome could imply that prediction accuracy and data structure representation may both benefit from fewer clusters in the delignification process.
A higher number of clusters may improve data fitting, but overfitting might lead to poor generalization performance on fresh data.65 The model may have performed worse on unknown data due to overfitting or noise produced by increasing the number of clusters. The findings of this study imply that the ANFIS–FCM model with 4 clusters (ANFIS–FCM4 clusters), better reflects the underlying patterns and relationships in delignification data. The performance assessment provided more evidence for this. Based on the outcome of this study, the dataset's patterns and structure may have been captured sufficiently by 4 clusters as the FCM method was able to capture the delignification process and biofuel property variations by encoding the data with enough clusters. Further to this, overfitting, where the model grows too complex and captures noise or training dataset quirks, may occur with more than 4 clusters. By restricting the number of clusters, the model might balance data variety and complexity, which can inhibit generalization to unknown data.
Moreover, 4 clusters performed best due to the data dispersion and sample size. The appropriate cluster number depends on the dataset's patterns and complexity. The Amaranthus hybridus waste solid biofuel dataset may fit a 4-clusters representation, improving model performance. A 4-cluster model may be more interpretable and robust. While too few clusters may obscure data patterns and variances, too many clusters can complicate the model. The FCM–ANFIS model balanced interpretability and data capture by choosing 4 clusters.
Table 7 showed the developed FCM–ANFIS model statistical metric value for evaluating the effect of delignification on the calorific value. With training RMSE-value of 0.0311 and testing RMSE-value of 0.0249 of the best models (ANFIS–FCM4 clusters), it accurately and reliably captures the complicated interactions between delignification and its calorific value. The RMSE-value of 0.0311 indicates that the predicted values of calorific value deviated from the actual values by 0.0311 units during training. Despite the dataset's variety and complexity, the model can accurately approximate the target variable. The model's low RMSE value shows that delignification affects solid calorific value. The model's generalization abilities were independently assessed during testing. This phase's RMSE of 0.0249 shows that the model's predictions match reality. Even with unseen data, the model captures the underlying patterns and correlations between delignification and biofuel attributes.
Table 7 Statistical metrics of the ANFIS–FCM for calorific value at the testing and training
Cluster number |
|
RMSE |
MAD |
MAE |
MAPE |
2 |
Training |
0.0427 |
0.0283 |
0.0276 |
5.214 |
Testing |
0.0324 |
0.0236 |
0.0232 |
4.438 |
3 |
Training |
0.0411 |
0.0258 |
0.0256 |
3.163 |
Testing |
0.0286 |
0.0214 |
0.0232 |
2.056 |
4 |
Training |
0.0311 |
0.0257 |
0.0249 |
2.104 |
Testing |
0.0249 |
0.0205 |
0.0223 |
1.818 |
5 |
Training |
0.0324 |
0.0264 |
0.0254 |
2.245 |
Testing |
0.0253 |
0.0215 |
0.0237 |
1.903 |
6 |
Training |
0.0315 |
0.0287 |
0.0263 |
2.197 |
Testing |
0.0248 |
0.0221 |
0.0228 |
1.732 |
With training MAE 0.0249 and testing MAE 0.0223, the model unveils accurately the trend in the complex interactions between delignification and its energy values. The model's training MAE was 0.0249, indicating an average absolute difference between projected and actual target value of calorific value. The model's low MAE shows it can reliably forecast the effects of delignification on its heating value. The MAPE values of 2.104 during the training phase and 1.818 during the testing phase further establish the accuracy of the model in investigating the calorific value of delignified solid biofuel. During the training phase, the model achieved a relatively low MAPE value of 2.104 at the training, thus indicating that, on average, the model's predictions deviate by only 2.104% from the true values. This demonstrates the model's capability to accurately estimate the impact of delignification on the combustibility and physicochemical characteristics of the solid biofuel.
Shown in Table 8 is the statistical metric value of the FCM–ANFIS model developed for evaluating the effect of delignification on the fixed carbon. A similar trend was observed in the performance of the FCM–ANFIS model developed for the fixed carbon of the delignified fuel. The FCM–ANFIS model improved marginally as the cluster numbers increased from 2–6 above and a significant decline was observed in the performance as the numbers of clusters increased above 6. The findings of this study imply that the ANFIS–FCM model with 4 clusters (ANFIS–FCM4 clusters), better reflects the underlying patterns and relationships in delignification data based on all the selected statistical metrics. The RMSE-value of 0.0190 indicates that the predicted values of calorific value deviated from the actual values by 0.0190 units during training. The model's low RMSE-value shows that delignification affects the biomass fixed carbon content of the solid biofuel. The model's generalization abilities were independently assessed during testing with RMSE of 0.0168 showing that the model's predictions match reality. The MAPE values of 5.956 during the training phase and 8.656 during the testing phase further establish the accuracy of the model in investigating the fixed carbon of delignified solid biofuel. During the training phase, the model achieved a relatively low MAPE value of 5.956, thus indicating that, on average, the model's predictions is 94.1% accurate and deviate by 5.9% from the true values. This demonstrates the model's capability to accurately determine delignification impact on the properties of the solid biofuel.
Table 8 Statistical metrics of the ANFIS–FCM for fixed carbon at the testing and training
Cluster number |
|
RMSE |
MAD |
MAE |
MAPE |
2 |
Training |
0.0231 |
0.0183 |
0.0257 |
7.012 |
Testing |
0.0178 |
0.0143 |
0.0159 |
9.244 |
3 |
Training |
0.0224 |
0.0173 |
0.0213 |
6.593 |
Testing |
0.0201 |
0.0134 |
0.0162 |
8.955 |
4 |
Training |
0.0190 |
0.0172 |
0.0204 |
5.956 |
Testing |
0.0168 |
0.0113 |
0.0158 |
8.656 |
5 |
Training |
0.0196 |
0.0185 |
0.0224 |
6.204 |
Testing |
0.0172 |
0.0128 |
0.0165 |
8.834 |
6 |
Training |
0.0211 |
0.0176 |
0.0213 |
6.294 |
Testing |
0.0185 |
0.0131 |
0.0164 |
9.152 |
Interestingly, the result of this study showed a better performance at the testing than the training. The ANFIS model uses data to learn the effects of delignification on the briquette properties during training. Iterative parameter adjustments reduce prediction errors. During training, the model may overfit and not generalize well to unseen data. However, the testing phase evaluates the model's performance on unseen data independently. The model performs well throughout testing, suggesting it can generalize effectively and reliably forecast the effect of alkali pretreatment on the solid biofuel qualities. Testing the model on unseen data allows for a more objective evaluation while testing data shows the model's real-world predicting skills. Moreover, regularization may help the model during testing as it penalizes complex models, preventing overfitting by choosing simpler and more representative delignification–biofuel interactions. Thus, the model's testing performance may exceed its training performance.
Beyond statistical metrics values, the performance of the best model (ANFIS–FCM4 clusters) was graphically established by a comparison plot of the experimental and predicted calorific value and fixed carbon content at the training and testing. Fig. 7 and 8 represent comparison test plots of the experimental and predicted calorific values of delignified solid biofuel using the ANFIS–FCM4 clusters at the training and testing phase respectively. The comparison test plots of the experimental and predicted fixed carbon content of the delignified solid biofuel using the ANFIS–FCM4 clusters at the training and testing phase is represented in Fig. 9 and 10, respectively. The plots showed that the model accurately captured the data's underlying trends. The graphic illustrates that the model is operating well and can properly forecast the influence of chemical modification of the biomass fiber matric through delignification. Residual histograms have a normal distribution around zero. The model only had a few overestimated or underestimated protein yields. This could be attributed to the overestimation and underestimation of the model's control parameters.61 The plot of the best model's anticipated and actual biomethane yield values provides useful insight into the model's performance and can influence future model development.
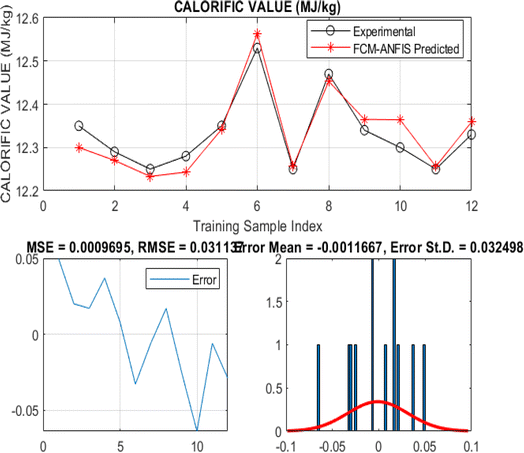 |
| Fig. 7 Comparison plot of the experimental and predicted calorific values delignified solid biofuel using the ANFIS–FCM4 clusters at the training phase. | |
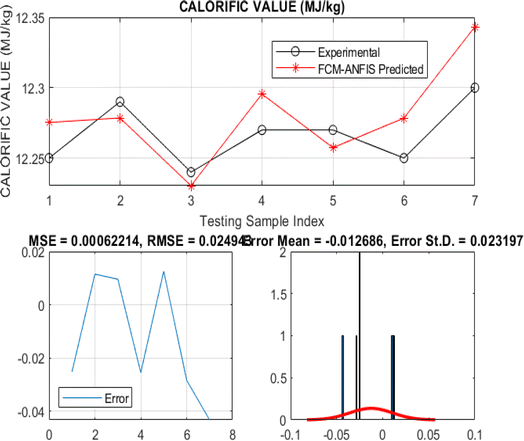 |
| Fig. 8 Comparison plot of the experimental and predicted calorific values on untreated solid biofuel using the ANFIS–FCM4 clusters at the testing phase. | |
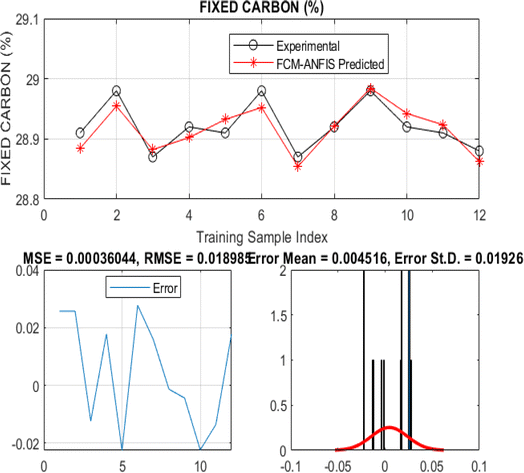 |
| Fig. 9 Comparison plot of the experimental and predicted fixed carbon content of delignified solid biofuel using the ANFIS–FCM4 clusters at the training phase. | |
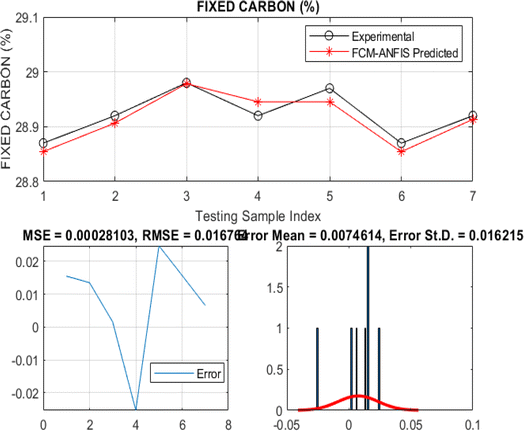 |
| Fig. 10 Comparison plot of the experimental and predicted fixed carbon content of untreated solid biofuel using the ANFIS–FCM4 clusters at the testing phase. | |
The outcome of this model can inform an intelligent and data-driven decisions in the study of the pretreatment of biofuel. In addition, the model gives useful insights into the impact of delignification of biofuel properties which can assist other researchers to optimize the delignification process and can facilitate extensive research in further studies and efforts towards enhanced biofuel production.
4 Conclusion
This study investigated and compared the physicochemical properties, combustion, and eco-friendly characteristics of NaOH pre-treated and untreated Amaranthus hybridus waste briquettes. The findings of this study show that the alkaline pre-treated bio-briquette performed better when compared with the untreated counterpart thereby recording a high mean fixed carbon content and calorific values of 12.53 ± 0.30 MJ kg−1 and 28.92 ± 0.01, respectively, with considerably mean low ash and moisture content values of 13.25 ± 0.01 and 2.55 ± 0.02% respectively. Higher ultimate analysis carbon value of 49.86 ± 0.08 wt%, and a carbon count value of approximately 1400 for TAHB as against 900 counts in UAHB shown by EDXRF analysis. Furthermore, the EDXRF evaluated the PTEs in both TAHB and UAHB showing that the percentage concentration of phosphorous (P), chlorine (Cl), lead (Pb), sulfur (S), and silicon (Si) which are 0.389, 0.384, 2.120, 0.229 and 0.790%, respectively in UCAB were observed to have considerably reduced to 0.331 (P), 0.103 (Cl), 2.004 (Pb), 0.150 (S) and 0220% (Si), respectively in TAHB. SEM micrographs showed a well-tacked surface due to a reduction in the fiber diameter (porosity), thereby signifying an improved thermal energy release with better combustibility. The surface modification as a result of delignification with recorded low mean lignin value of 11.47 ± 0.00% in TAHB compared to 12.31 ± 0.01 wt% in UAHB shown by the reduction in lignin content is confirmed by FTIR analysis with a shift in the C–O vibrational stretch from 1010 cm−1 as recorded in the UAHB sample to 1006 cm−1 in the alkaline pre-treated sample (TAHB). The machine modeling shows that the RMSE, MAPE, and MAE recorded values of 0.0249, 2.104, and, 0.0249; (MAE, training) and 0.0223 (MAE, testing) respectively. This shows that the model's predictions match reality, thereby suggesting a strong agreement between the experimental data and predicted values. Delignified alkali pre-treated bio-briquettes were observed to have better biofuel qualities than the untreated counterparts; as a result, chemical modification of biomass before manufacturing improves the environmental compatibility and combusting performance of the resulting solid biofuel.
It should be noted that this study presented the probable application of Amaranthus hybridus waste vis-à-vis the chemically modified samples as an eco-friendly alternative solid biofuel for energy purposes. However, it is imperative to conduct further research on the emissions assessment and comprehensive cost of production analysis of the biofuel to ascertain and make a coherent comparative study between this energy material and other fossil fuels, thereby substantiating the waste-to-wealth claim of this study.
Data availability
All data and materials used in this study are available within this article.
Author contributions
Abayomi Bamisaye: conceptualization, methodology, investigation, methodology, writing –reviewing and editing, and supervision. Ayodeji Rapheal Ige: methodology, interpretation, validation, software, writing – reviewing and editing, and supervision. Kayode Adesina Adegoke: interpretation, validation, visualization, writing – reviewing and editing and supervision. Idowu Abimbola Adegoke: interpretation, validation, visualization, writing – reviewing and editing. Muyideen Olaitan Bamidele: interpretation, validation, visualization, writing – reviewing and editing. Yakubu Adekunle Alli: interpretation, validation, visualization, writing – reviewing and editing. Oluwatobi Adeleke: interpretation, validation, visualization, software, writing – reviewing and editing. Mopelola Abidemi Idowu: interpretation, validation, visualization, writing – reviewing and editing.
Conflicts of interest
The authors declare no conflict of interest.
Acknowledgements
Authors acknowledge their respective Universities for the platform to carry out this study.
References
- S. Modupe Abati, A. Bamisaye, A. Abidemi Adaramaja, A. Rapheal Ige, K. Adesina Adegoke, E. Olurotimi Ogunbiyi, M. Abidemi Idowu, A. B. Olabintan and T. A. Saleh, Fuel, 2024, 364, 130847 CrossRef CAS.
- S. Mor and K. Ravindra, Process Saf. Environ. Prot., 2023, 174, 510–530 CrossRef CAS.
- A. Bamisaye and I. A. Rapheal, Biomass Convers. Biorefin., 2021, 10, 8939–8947 Search PubMed.
- P. Duarah, D. Haldar, A. K. Patel, C. Di Dong, R. R. Singhania and M. K. Purkait, Bioresour. Technol., 2022, 348, 126791 CrossRef CAS.
- N. Scarlat, J. F. Dallemand, F. Monforti-Ferrario, M. Banja and V. Motola, Renewable Sustainable Energy Rev., 2015, 51, 969–985 CrossRef.
- P. O. Oladoye, E. O. Omotola, Y. A. Alli, M. E. Oladipo, O. Ejeromedoghene and A. Bamisaye, in Microbial Biotechnology for Bioenergy, ed. N. R. Maddela, S. A. Aransiola, C. I. Ezugwu, L. K. Winkelstroter Eller, L. Scalvenzi and F. Meng, Elsevier, 2024, pp. 299–324 Search PubMed.
- A. Kumar, S. Adamopoulos, D. Jones and S. O. Amiandamhen, Waste Biomass Valorization, 2021, 12, 65–80 CrossRef.
- A. Bamisaye, A. R. Ige, A. I. Adegoke, M. A. Idowu and C. M. Elinge, Biomass Convers. Biorefin., 2022 DOI:10.1007/s13399-022-03397-x.
- M. Safaya and Y. C. Rotliwala, Mater Today Proc, 2020, 27, 454–459 CrossRef CAS.
- S. M. Aldarabseh, Biomass Convers. Biorefin., 2023, 1–20 Search PubMed.
- A. R. Proto, A. Palma, E. Paris, S. F. Papandrea, B. Vincenti, M. Carnevale, E. Guerriero, R. Bonofiglio and F. Gallucci, Fuel, 2021, 289, 119758 CrossRef CAS.
- Y. Li and H. Liu, Biomass Bioenergy, 2000, 19, 177–186 CrossRef.
- A. M. Omari, A. M. Omari, B. N. Kichonge, G. R. John, K. N. Njau and P. L. Mtui, Int. J. Renew. Energy Res., 2014, 3, 2325–3924 Search PubMed.
- N. Gupta, B. K. Mahur, A. M. D. Izrayeel, A. Ahuja and V. K. Rastogi, Environ. Sci. Pollut. Res., 2022, 29, 73622–73647 CrossRef CAS PubMed.
- A. P. Khedulkar, B. Pandit, V. D. Dang and R. an Doong, Sci. Total Environ., 2023, 869, 161441 CrossRef CAS PubMed.
- B. Koul, M. Yakoob and M. P. Shah, Environ. Res., 2022, 206, 112285 CrossRef CAS PubMed.
- R. K. Rathour, M. Devi, P. Dahiya, N. Sharma, N. Kaushik, D. Kumari, P. Kumar, R. R. Baadhe, A. Walia, A. K. Bhatt and R. K. Bhatia, Energies, 2023, 16, 1429 CrossRef CAS.
- Sonu, G. M. Rani, D. Pathania, Abhimanyu, R. Umapathi, S. Rustagi, Y. S. Huh, V. K. Gupta, A. Kaushik and V. Chaudhary, Sci. Total Environ., 2023, 875, 162667 CrossRef CAS PubMed.
- E. A. Ogie-Odia, J. K. Mensah, O. E. Ehilen and D. A. Eseigbe, Niger. J. Biotechnol., 2022, 38, 1–12 CrossRef.
- A. Martinez-Lopez, F. Rivero-Pino, A. Villanueva, R. Toscano, E. Grao-Cruces, E. Marquez-Paradas, M. E. Martin, S. Montserrat-De la Paz and M. C. Millan-Linares, Food Funct., 2022, 13, 11604–11614 RSC.
- K. A. Motghare, A. P. Rathod, K. L. Wasewar and N. K. Labhsetwar, Waste Manage., 2016, 47, 40–45 CrossRef CAS PubMed.
- A. Idowu Abimbola, International Journal of Sustainability Management and Information Technologies, 2019, 5, 35 CrossRef.
- M. Chibuikeoyibo, E. C. Moki, A. U. Birninyauri, O. A. Ogundele and M. D. Dabai, Am. J. Eng. Res., 2020, 9(3), 268–274 Search PubMed.
- A. Bamisaye and I. A. Rapheal, Biomass Convers. Biorefin., 2023, 13, 8939–8947 CrossRef CAS.
- A. Bamisaye, A. R. Ige, A. I. Adegoke, M. A. Idowu and C. M. Elinge, Biomass Convers. Biorefin., 2022 DOI:10.1007/s13399-022-03397-x.
- M. Azzam, J. Environ. Sci. Health B, 1989, 24, 421 CrossRef.
- A. Bamisaye, A. R. Ige, I. A. Adegoke, E. O. Ogunbiyi, M. O. Bamidele, O. Adeleke and K. A. Adegoke, Fuel, 2023, 340 DOI:10.1016/j.fuel.2023.127412.
- A. I. Abimbola, I. Ayodeji Rapheal, B. Abayomi, E. C. Moki and O. O. Ayodele, DOI:10.13057/biotek/c190104.
- A. Bamisaye and I. A. Rapheal, Biomass Convers. Biorefin., 2021 DOI:10.1007/s13399-021-01771-9.
- M. Mahyati, A. R. Patong, M. N. Djide, D. P. Taba, A. Rauf Patong, M. Nasir Djide, D. Paulina Taba, R. Abdul, N. Muhammad and T. Paulina, Int J Sci Technol Res, 2013, 2, 3–7 Search PubMed.
- A. Bamisaye, A. R. Ige, K. A. Adegoke, I. A. Adegoke, M. O. Bamidele, O. Adeleke, M. A. Idowu and N. W. Maxakato, Fuel, 2024, 358, 129936 CrossRef CAS.
- R. Ige, E. Ogala, C. Moki and A. Habeeb, J. Adv. Acad. Res., 2020, 221–229 CrossRef.
- M. Matúš, P. Križan, J. Beniak and L. Šooš, Acta Polytech., 2015, 55, 335–341 CrossRef.
- J. Parikh, S. A. Channiwala and G. K. Ghosal, Fuel, 2007, 86, 1710–1719 CrossRef CAS.
- J. S. R. Jang, IEEE Trans. Syst. Man Cybern., 1993, 23, 665–685 CrossRef.
- M. Mustapha, M. W. Mustafa, S. N. Khalid, I. Abubakar and A. M. Abdilahi, Indian J. Sci. Technol., 2016, 9(46), 1–8 Search PubMed.
- V. Güldal and H. Tongal, Sustain. Water Resour. Manag., 2010, 24, 105–128 CrossRef.
- O. Sanni, O. Adeleke, K. Ukoba, J. Ren and T. C. Jen, J. Mater. Res. Technol., 2022, 20, 4487–4499 CrossRef CAS.
- A. Sajadi, A. Dashti, M. Raji, A. Zarei and A. H. Mohammadi, Renewable Energy, 2020, 158, 465–473 CrossRef.
- S. Ghosh, A. P. Hazarika, A. Chandra and R. K. Mudi, Vis. Inform., 2021, 5, 67–80 CrossRef.
- Y. Gao, Z. Wang, J. Xie and J. Pan, Knowl.-Based Syst., 2022, 237, 107769 CrossRef.
- H. A. Ajimotokan, A. O. Ehindero, K. S. Ajao, A. A. Adeleke, P. P. Ikubanni and Y. L. Shuaib-Babata, Sci Afr, 2019, 6, e00202 Search PubMed.
- A. O. Olorunnisola, Agric. Eng., Int., 2007, 9, 1–11 Search PubMed.
- A. Bamisaye and I. A. Rapheal, J. Solid Waste Technol. Manage., 2022, 48, 116–123 CrossRef CAS.
- B. Derived, P. Of and R. Husk, Acta Chemica Malaysia, 2022, 6, 52–57 Search PubMed.
- B. Abayomi, I. A. Rapheal and B. M. Olaitan, International Journal of Advanced Academic Research, 2021, 7, 50–59 Search PubMed.
- S. J. Veeresh and J. Narayana, Univers. J. Environ. Res. Technol., 2012, 2, 575–581 CAS.
- M. J. Prins, K. J. Ptasinski and F. J. J. G. Janssen, J. Anal. Appl. Pyrolysis, 2006, 77, 28–34 CrossRef CAS.
- O. A. Lasode, A. O. Balogun and A. G. McDonald, J. Anal. Appl. Pyrolysis, 2014, 109, 47–55 CrossRef CAS.
- A. R. Ige, C. M. Elinge, L. G. Hassan, D. R. Akinkuotu and O. J. Ajakaye, J. Solid Waste Technol. Manag., 2020, 46, 1–4 CrossRef.
- G. Abdulkareem-Alsultan, N. Asikin-Mijan, H. V. Lee, U. Rashid, A. Islam and Y. H. Taufiq-Yap, Catalysts, 2019, 9, 1–25 CrossRef.
- A. Katimbo, N. Kiggundu, S. Kizito, H. B. Kivumbi and P. Tumutegyereize, Agric. Eng. Int.: CIGR J., 2014, 16, 146–155 Search PubMed.
- O. M. Akande and A. O. Olorunnisola, Recycling, 2018, 3(2) DOI:10.3390/recycling3020011.
- J. Apau, M. O. Siameh, J. A. Misszento, O. Gyamfi, J. Osei-Owusu, E. E. Kwaansa-Ansah and A. Acheampong, Cogent Public Health, 2022, 9 DOI:10.1080/27707571.2022.2145699.
- P. Pavlović, T. Sawidis, J. Breuste, O. Kostić, D. Čakmak, D. Đorđević, D. Pavlović, M. Pavlović, V. Perović and M. Mitrović, Int. J. Environ. Res. Public Health, 2021, 18, 6014 CrossRef PubMed.
- A. E. Ogbeibu, M. O. Omoigberale, I. M. Ezenwa, J. O. Eziza and J. O. Igwe, Nat. Environ., 2014, 2, 1 CrossRef.
- O. Ameh Sylvanus, N. Kgabi, S. H. Taole, S. Ameh, K. Nnenesi Anna and T. Simeon Halahala, Eur. J. Appl. Eng. Sci. Res., 2016, 139, 49–63 Search PubMed.
- D. R. Patil, S. Lee, A. Thakre, A. Kumar, H. Song, D. Y. Jeong and J. Ryu, J. Mater., 2023, 9, 735–744 Search PubMed.
- A. I. Abimbola, I. Ayodeji Rapheal, B. Abayomi, E. C. Moki and O. O. Ayodele, Asian J. Trop. Biotechnol., 2022, 19(1) DOI:10.13057/biotek/c190104.
- A. Bamisaye, A. R. Ige, A. I. Adegoke, M. A. Idowu and C. M. Elinge, Biomass Convers. Biorefin., 2022 DOI:10.1007/s13399-022-03397-x.
- O. Adeleke and T. C. Jen, Energy Rep., 2022, 8, 576–584 CrossRef.
- T.-C. J. Oluwatobi Adeleke, in ASME 2022 International Mechanical Engineering Congress and Exposition, ASME, Columbus, Ohio, USA, 2022 Search PubMed.
- W. Wiharto and E. Suryani, The analysis effect of cluster numbers on fuzzy c-means algorithm for blood vessel segmentation of retinal fundus image, in Proceedings of the 2019 International Conference on Information and Communications Technology, ICOIACT 2019, Yogyakarta, Indonesia, Institute of Electrical and Electronics Engineers Inc., Yogyakarta, Indonesia, 2019, pp. 106–110 Search PubMed.
- K. Benmouiza and A. Cheknane, Theor. Appl. Climatol., 2019, 137, 31–43 CrossRef.
- O. Adeleke, S. Akinlabi, T.-C. Jen and I. Dunmade, Int. J. Ambient Energy, 2020, 1–12 Search PubMed.
|
This journal is © The Royal Society of Chemistry 2024 |
Click here to see how this site uses Cookies. View our privacy policy here.