DOI:
10.1039/D4RA02593H
(Paper)
RSC Adv., 2024,
14, 21203-21212
Drug repositioning identifies salvinorin A and deacetylgedunin (DCG) enriched plant extracts as novel inhibitors of Mpro, RBD–ACE2 and TMPRRS2 proteins†
Received
6th April 2024
, Accepted 21st June 2024
First published on 4th July 2024
Abstract
The coronavirus disease 2019 (COVID-19) has spread worldwide with severe health, social, and economic repercussions. Although vaccines have significantly reduced the severity of symptoms and deaths, alternative medications derived from natural products (NPs) are vital to further decrease fatalities, especially in regions with low vaccine uptake. When paired with the latest computational developments, NPs, which have been used to cure illnesses and infections for thousands of years, constitute a renewed resource for drug discovery. In the present report, a combination of computational and in vitro methods reveals the repositioning of NPs and identifies salvinorin A and deacetylgedunin (DCG) as having potential anti-SARS-CoV-2 activities. Salvinorin A was found both in silico and in vitro to inhibit both SARS-CoV-2 spike/host ACE2 protein interactions, consistent with blocking viral cell entry, and well as live virus replication. Plant extracts from Azadirachta indica and Cedrela odorata, which contain high levels of DCG, inhibited viral cell replication by targeting the main protease (Mpro) and/or inhibited viral cell entry by blocking the interaction between spike RBD–ACE2 protein at concentrations lower than salvinorin A. Our findings suggest that salvinorin A represent promising chemical starting points where further optimization may result in effective natural product-derived and potent anti-SARS-CoV-2 inhibitors to supplement vaccine efforts.
1 Introduction
The global response to the COVID-19 pandemic witnessed unprecedented efforts from scientific laboratories that rapidly advanced drug and vaccine development to confront the devastating impact of the virus.1,2 This remarkable cooperation has led to the approval of 13 vaccines by the World Health Organization (WHO) for emergency use since late 2020 and over 170 vaccines at various stages of development.3 However, despite this rapid progress, a decline in public confidence in vaccines has emerged that has contributed to lower vaccination rates and hindered full control of the virus.4,5 This hesitancy, influenced by misconceptions about vaccine safety and efficacy, as well as a general mistrust of the institutions involved, presents a substantial challenge in achieving widespread immunization.6 For example, in many high-income countries, up to 30% of the population remains unvaccinated, while in many developing nations, vaccine hesitancy surpasses 60%.2,7,8
To help to address this gap, alternative approaches, including the exploration of natural product-based drugs, are necessary.9,10 For years, natural products have been demonstrated to possess antiviral, anti-inflammatory, and antioxidant properties, offering a valuable resource in the fight against viruses.11–13 As a result, they also represent a promising resource from which to identify new therapeutic leads against SARS-CoV-2 that may be more acceptable to local communities, particularly those that rely on medicinal plants as part of their healthcare.
The entry of SARS-CoV-2 into host cells initiates viral replication, with its viral RNA genome guiding the production of key proteins. This includes papain-like cysteine protease (PLpro) and 3-chymotrypsin-like cysteine protease (3CLpro), also known as main protease (Mpro). Furthermore, the virus exploits host machinery, such as TMPRSS2, facilitating its entry and subsequent replication within the host cell.14–16 Due to their role in protein processing, both PLpro and Mpro have emerged as promising targets for drug development.17–19 Another molecular target of SARS-CoV-2 is through the inhibition of viral cell entry. For example, SARS-CoV-2 cellular binding and entry is controlled in large part by the receptor binding domain (RBD) of the viral spike protein, which interacts with the host receptor ACE2. Therapeutic antibodies that disrupt this interaction are potent inhibitors of SARS-CoV-2 replication, although viral mutation in RBD can rapidly generate resistance to these antibodies as well as circumvent host immunity.
Natural products that inhibit the RBD–ACE2 interaction through mechanisms that are distinct from therapeutic antibodies might present a higher genetic barrier to drug resistance, particularly if they can also target other essential proteins like the viral proteases. In this context, this work builds upon previous efforts, aiming to offer new therapeutic leads for combating the spread of COVID-19. The findings from this present investigation reveal the potential of salvinorin-A, as well as deacetylgedunin (DCG)-containing plant extracts such as those from Cedrela odorata (CO) and the neem tree (Azadirachta indica, AI), in inhibiting specific viral processes of SARS-CoV-2.
2 Materials and methods
2.1 In silico studies
2.1.1 Molecular docking. The computational techniques utilized in this study were executed following the methodologies outlined in our previous publication.20 Briefly, any co-crystallized water molecules and ligands were eliminated from the protein structures. Subsequently, polar hydrogen atoms and missing residues were added to the proteins. Fig. 1 shows an in-house library comprising 19 Azadirachta indica (neem) tree extracts, which was established and employed for the initial docking screening against TMPRSS2. The ligands underwent preparation, involving the addition of all hydrogen atoms at a pH of 7.4, and were then subjected to energy minimization using the Open Babel tool.21 Next, both protein and ligand structures were saved in the .pdbqt file format. Docking-based virtual screening was conducted using AutoDock Vina,22 a reliable tool known for its remarkable accuracy in predicting experimental binding affinities of small molecules in various studies. Docking simulations were conducted for a total of 20 ligands, comprising 19 ligands (Fig. 1) of interest along with the standard molecule, nafamostat. For TMPRSS2, the ligands were docked into the active site utilizing a grid approach, with the binding site coordinates set at x = 10.20, y = −8.61, and z = 42.17. The dimensions of the binding site were defined as x = 50.78, y = 49.49, and z = 42.17. Parameters for the docking process included an exhaustiveness level of 10 and the generation of 10 binding modes. For relaxed complex scheme docking calculation, the grid box for each receptor was prepared and ligands were docked to the active side. Before conducting the docking experiment, we rigorously validated our docking protocol using a dataset of experimental binding affinities and re-docking procedures as described in our previous works.20,23,24 The docking calculations for various compounds from Azadirachta indica (neem) tree extracts against TMPRSS2 indicated that ligand CID 3034112 exhibited superior binding affinity, earning it the highest score. Hence, the ligand was selected as a potential hit compound for further similarity search. The similarity search was conducted against the DrugBank and ChEMBL databases, encompassing approved drug molecules intended for other indications. This exploration was executed through Swiss ADMEt, utilizing a Tanimoto index of 0.9 to identify highly similar molecules. Compounds with notable scores were retrieved and subsequently subjected to molecular docking calculations. Additionally, docking simulations were conducted to assess the Mpro and RBD–ACE2 inhibition activities of a promising molecule identified through similarity search, as described in the flow chart (Fig. 2). Finally, molecular dynamics simulations and end-point free energy calculations based on MM-PBSA were conducted to ascertain the stability and affinity of the complexes.
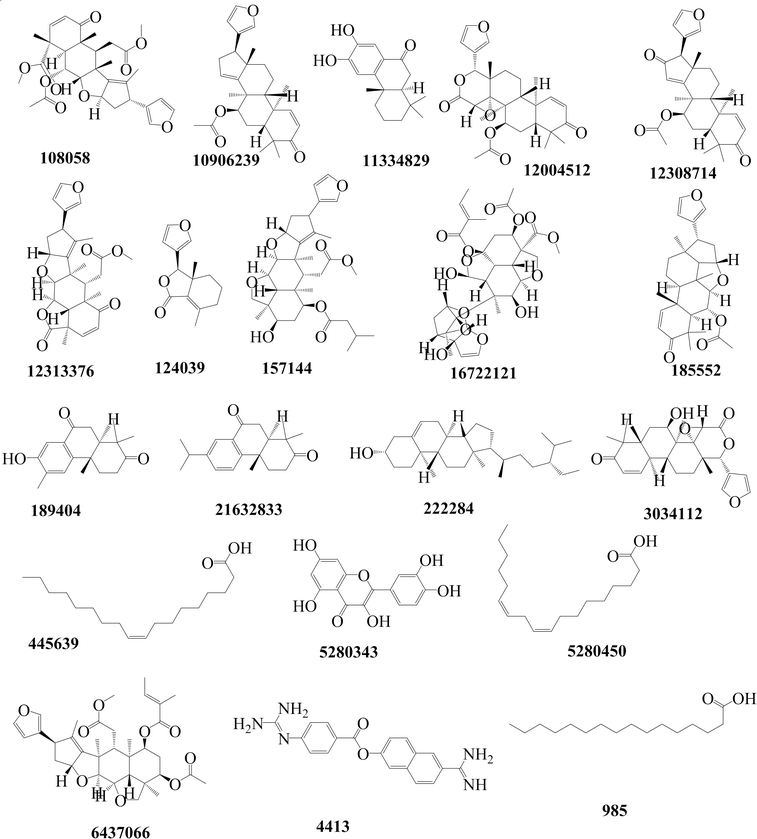 |
| Fig. 1 The nineteen molecular structures and their respective compound identifier (CID) constituting the chemical library derived from Azadirachta indica (neem) tree extracts, and the nafamostat compound (CID 4413). | |
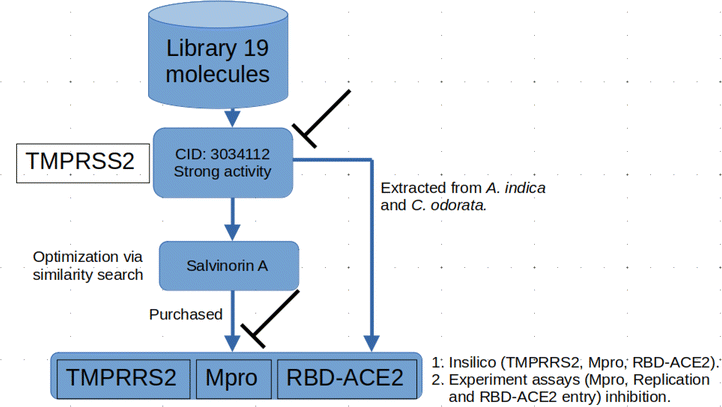 |
| Fig. 2 Flow chat showing the protocol adopted in this study. At the beginning, a library of 19 molecules from the neem tree was screened against the TMPRSS2. Compound CID 3034112 highly scored, further optimization through similarity search resulted in a highly similar molecule, salvinorin A which showed good binding affinity against TMPRSS2. The compound was further tested both in vitro and insilico against other viral proteins; Mpro, and entry inhibition via RBD–ACE2 inhibition. Since CID 3034112 was not purchased due to vendor issues, compounds were extracted and tested in vitro. | |
2.1.2 Molecular dynamics simulation. The molecular dynamics simulations were performed using GROMACS v2018. Both proteins and ligands were parameterized employing the OPLS-AA force field. The systems were solvated with TIP4P water molecules within cubic boxes, maintaining a minimum distance of 1 nm from the proteins. To neutralize the systems, Na+ ions were added at a physiological concentration of 0.15 M. The initial phase involved energy minimization using the steepest descent algorithm. Subsequently, equilibration was carried out with position restrain in an NVT (T = 310 K) ensemble for 200 ps, followed by an NTP (P = 1 bar) ensemble for 500 ps. During equilibration, temperature and pressure were controlled using the v-rescale thermostat25 and Parrinello–Rahman barostat,26 respectively. For the production run, an NTP ensemble was employed without position restrain, utilizing an integration time step of 2 fs for a duration of 100 ns. The Parrinello–Rahman barostat26 maintained the pressure at 1 bar, while the v-rescale thermostat sustained the temperature at 310 K, respectively.25,26 Throughout all simulations, periodic boundary conditions (PBC) were implemented in all directions. Covalent bonds were constrained utilizing the LINCS27 algorithm, whereas particle mesh Ewald (PME) was used to treat long-range electrostatic interactions with the cut-off distance at 12 Å. The free energy surface was calculated as F = −kBT
ln(P(x)/P(y)), where F is the free energy surface, kB is the Boltzmann constant, T is temperature and P(x)/P(y) is the probability distribution along the selected reaction coordinates.
2.1.3 MM-PBSA free energy calculations. Molecular mechanics Poisson–Boltzmann surface area (MM-PBSA) was calculated using g_mmpbsa tool28 as described in our previous work.24 Here, the binding free energy (ΔGbind) for protein–ligand interaction we express as |
ΔGbind = Gcomplex − (Gprotein + Gligand)
| (1) |
where Gcomplex represents the total free energy of the protein–ligand complex while Gprotein and Gligand are total free energies of the isolated protein and ligand in the solvent, respectively. Furthermore, the free energy for each entity was derived from |
Gx = 〈EMM〉 + 〈Gsol〉 − TΔS
| (2) |
where, x stands for the protein or ligand or protein–ligand complex, T and S denote the temperature and entropy, respectively, and TΔS refers to the entropic contribution to the free energy in a vacuum. The average molecular mechanics (MM) energy in a vacuum is denoted as 〈EMM〉, this term includes bonded and non-bonded interactions, 〈EMM〉 is calculated based on MM force-field parameters as follows |
〈EMM〉 = 〈Ebonded〉 + 〈Enon-bonded〉 = 〈Ebonded〉 + (EvdW + Eelec)
| (3) |
where Enon-bonded is bonded interactions consisting of bond, angle, dihedral, and improper interactions. The non-bonded interactions (Enon-bonded) include both electrostatic (Eelec) and van der Waals (EvdW) interactions and are modelled using Coulomb and Lennard-Jones (LJ) potential function, respectively. The free energy of solvation 〈Gsol〉 includes Gpolar and Gnon-polar and can be calculated as |
〈Gsol〉 = Gpolar + Gnon-polar = Gpolar + (γ × SASA + b)
| (4) |
where γ is a coefficient related to surface tension, SASA stands for solvent accessible surface area and b is the fitting parameter, TΔS is the entropic contributions to free energy. In g_mmpbsa an entropic contribution is not considered.28 The binding free energy was calculated using a single trajectory, where a total of 200 snapshots were evenly extracted at a predetermined time and calculated in a block of 20 ns. The solvent and solute dielectric constants were 80 and 2, respectively, and γ was 0.0227, the Poisson–Boltzmann (PB) equation was solved by using the linear PBsolver.
2.2 Plant identification and crude extraction
The collection sites for A. indica plant leaves (Pwani, Tanzania, voucher no. 980) and C. odorata bark (Kilombero, Tanzania, voucher no. 981) were selected deliberately. Botanical identification and voucher specimens were deposited in the Herbarium of the Institute of Traditional Medicine, the Muhimbili University of Health and Allied Sciences. Powdered air-dried backs of C. odorata (500 g) and leaves of A. indica (500 g) were extracted separately with methanol and ethanol. The leaves were soaked for 24 hours, and the process was repeated three times to exhaust the extracts. The filtrates obtained were concentrated under decreased pressure and kept at 4 °C until needed.
2.3 In vitro studies
2.3.1 Inhibition of live SARS-CoV-2 replication. To assess the effects of salvinorin A and the plant extracts on live SARS-CoV-2 replication, live virus infection assays were performed as previously described.29 Briefly, Vero-E6 cells were plated in D10+ medium (Dulbecco's modified Eagle medium with 4.5 g per L glucose and L-glutamine, 10% fetal bovine serum, 100 U per mL penicillin and 100 μg per mL streptomycin) at 20
000 cells per well in 96-well format, and compounds and extracts were then added to final concentrations in 3-fold replicates and incubated for an additional 2 hours before infection with 150× TCID50 of SARS-CoV-2 virus (USA-WA1/2020). Cells were incubated for an additional 96 hours, treated with Alamar Blue for 4 hours, fixed with paraformaldehyde to a final concentration of 4%, and incubated at room temperature for at least 30 minutes to inactivate the virus. Fluorescence intensity was then measured using a ClarioStar plate reader. Background fluorescence was subtracted from wells containing resazurin and D10+ medium but no cells.
2.3.2 Spike RBD–ACE2 binding assays. Assays were performed as described previously using AlphaScreen technology.29 2 nM ACE2–Fc (Sino Biological, Chesterbrook, PA, USA) was first incubated with 5 nM of His-tagged SARS-CoV-2 spike-RBD (Sino Biological) plus 5 μg per mL nickel chelate donor bead in a total of 10 μL of 20 mM Tris (pH 7.4), 150 mM KCl, and 0.05% CHAPS (3-[(3-cholamido-propyl)-dimethylammonio]1-propanesulfonate). Test compounds were then diluted to 100× final concentration in DMSO. 5 μL of ACE2–Fc/protein A acceptor beads was then added followed by the addition of 100 nL of compounds or extracts, which in turn were followed by 5 μL of CoV-spike-RBD-His/nickel chelate donor beads. All experiments were performed in duplicate in white, low-volume 384-well plates. Mixtures were incubated at room temperature for 2 hours. Fluorescent signals were measured using a ClarioStar plate reader. Data were normalized to percent inhibition, where 100% denoted signal in the absence of SARS-CoV-2-spike-RBD-His, and 0% denoted signal in the presence of both proteins and DMSO alone.
2.3.3 Mpro inhibition assays. Generation of recombinant Mpro protein and enzymatic assays were performed as described previously.29 Briefly, 5 μL of 25 nM Mpro diluted in 25 mM HEPES (pH 7.4), 150 mM NaCl, 5 mM DTT, and 0.005% Tween. 100 nL of compound or extract was then added to the mixture. Assays were performed in duplicate in black, low-volume, 384-well plates. Assays were then initiated by addition of 5 μL of 5 μM of a quenched fluorogenic substrate (Bachem, Vista, CA, USA),29 and fluorescence was monitored every 5 minutes for 50 minutes using an Envision plate reader (PerkinElmer). The rate of substrate cleavage was determined using linear regression of the raw data values obtained during the time course. The slopes of these progress curves were then normalized to percent inhibition, where 100% denoted the rate in the absence of Mpro and 0% denoted the rate of cleavage in the presence of Mpro and 0.1% DMSO.
3 Results and discussion
3.1 Salvinorin A and deacetylgedunin (DCG) block SARS-CoV-2 viral cell entry through TMPRRS2 inhibition
In this study, we began by investigating the potential of neem tree extracts to impede the enzymatic activity of TMPRSS2 protein, a critical player known for activating the ACE2 receptor binding domain, thus facilitating viral entry into human cells. It is worth noting that we have previously reported the ADMET profile of the neem tree extract, and DCG exhibited favourable drug-like properties.20 Conversely, salvinorin A is an approved molecule with a well-established ADMET profile. Furthermore, before commencing docking calculations, we thoroughly validated our docking protocols using an experimental dataset, as detailed in our previous works.20,23 Through molecular docking calculations (Fig. 3A), we identified compounds from the neem tree demonstrating noteworthy binding affinities, comparable to that of the reference molecule, nafamostat (−7.8 kcal mol−1). This suggested that these neem-derived compounds have the potential to obstruct viral cell entry by inhibiting TMPRSS2. In our pursuit of identifying clinically proven medications for combating COVID-19, we conducted a similarity search on the top-scoring neem tree extracts, with a focus on DCG (CID 3034112) which had an average binding affinity of −7.86 kcal mol−1. Our investigation also led to the identification of salvinorin A with a Tanimoto index of 0.9. We hypothesized that molecules with structural similarities often share similar modes of action. As anticipated, salvinorin A exhibited a comparable average binding affinity of −7.9 kcal mol−1. Since our objective was to find molecules in clinical applications for other indications that can be repurposed, our discussion will now focus on salvinorin A. To further elucidate the inhibitory effects of salvinorin A on the enzymatic catalytic activities of TMPRSS2, we conducted classical molecular dynamics simulations. The 2D free energy surface, considering residues–residues and residues–ligand distances, revealed three minima, with the deepest minimum observed at 0.5, 0.9 nm for residues–residues, residue–ligand distances. This indicates the potential of salvinorin A to block the enzymatic catalytic activities of TMPRSS2 by increasing the distances between residues. Notably, catalytic residues Thr242 and Asn311 are pivotal for the activation and catalytic activities of the protein. TMPRSS2 inhibitors, such as nafamostat, typically block the recognition of these residues, thereby interfering with catalytic activities. We performed MM-PBSA free energy calculation, the time-lapse binding free energy suggests that salvinorin A remained stable with a strong affinity until 50 ns, where it showed the unbinding and binding pattern from the protein, which considerably reduced its binding affinity (Fig. S1A–C†). Such observed fluctuations suggest that salvinorin A could exhibit inhibition to TMPRSS2 albeit with low efficacy. It is noteworthy that we extended our analysis by correlating the free energy with three key reaction coordinates: RMSD, pose RMSD, and distance (between the protein active site and ligand). Among these, only pose RMSD exhibited a consistent fluctuation pattern from 50 ns onwards, mirroring the observations from the time-dependent free energy calculation (Fig. S1D†). This suggests a significant role of pose RMSD in capturing the unbinding process, whereas distance and RMSD displayed negligible changes over time. This underscores the potential efficacy of pose RMSD as a reliable reaction coordinate in drug design for predicting in vitro or in vivo activities. Given that salvinorin A is an approved kappa opioid receptor (KOR) agonist and a drug for neurological diseases, this discovery motivated us to explore its efficacy in blocking the spike ACE2–RBD interaction and inhibiting Mpro, as detailed in the subsequent sections (Table 1).
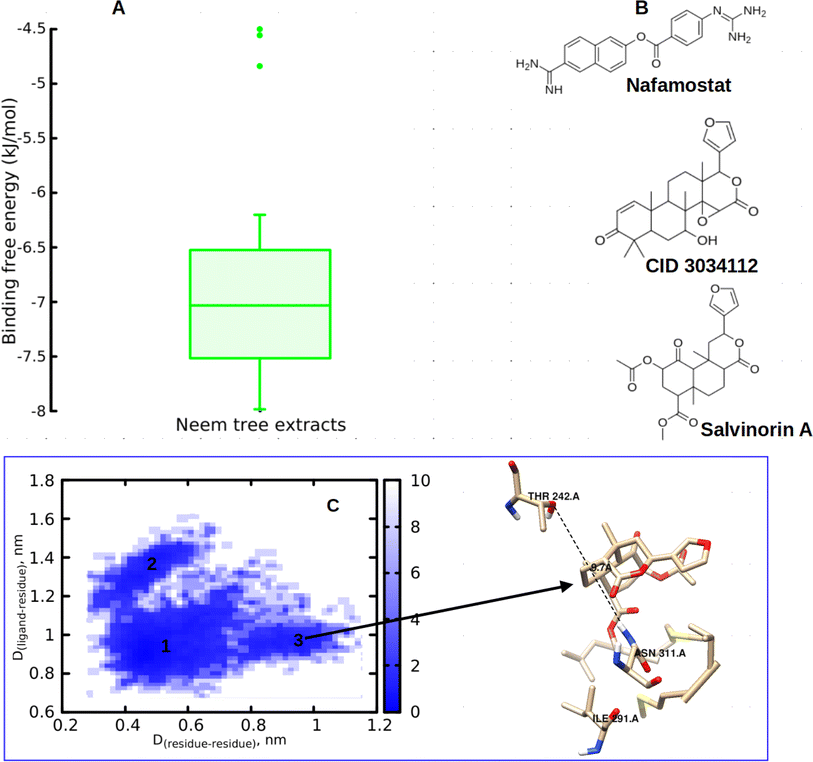 |
| Fig. 3 (A) Binding free energy of neem tree extracts against TMPRSS2, (B) chemical structure of nafamostat, DCG (CID 3034112) and salvinorin A, (C) 2D free energy surface along with visualized structure. | |
Table 1 Average binding free energy calculation (kcal mol−1) of neem tree extracts and salvinorin A
Ligand ID |
TMPRSS2 |
CID 108058 |
−6.88 |
CID 10906239 |
−7.20 |
CID 11334829 |
−6.88 |
CID 12004512 |
−7.50 |
CID 12308714 |
−7.60 |
CID 12313376 |
−7.60 |
CID 124039 |
−6.20 |
CID 157144 |
−6.50 |
CID 16722121 |
−7.50 |
CID 185552 |
−7.52 |
CID 189404 |
−7.00 |
CID 21632833 |
−7.06 |
CID 222284 |
−6.54 |
CID 3034112 |
−7.86 |
CID 445639 |
−4.56 |
CID 5280343 |
−7.50 |
CID 5280450 |
−4.84 |
CID 6437066 |
−6.54 |
CID 985 |
−4.50 |
Nafamostat |
−7.80 |
Salvinorin A |
−7.90 |
3.2 Inhibition of ACE2–RBD interaction by salvinorin A and DCG
This section explores the ability of identified salvinorin A and DCG to block viral cell entry through ACE2–RBD inhibition. To deepen our understanding, both rigid and flexible docking calculations based on a relaxed complex scheme (RCS) were performed. At first glance, the rigid docking calculation resulted in a binding affinity of −6.6 and −7.4 kcal mol−1 for salvinorin A and DCG, respectively. This encouraged us to perform an RCS which has been effective in reducing biases in docking calculations.24,30 Interestingly, some snapshots from RCS showed higher binding affinity as compared to the crystal structure with an average binding affinity of −6.75 and −7.0 kcal mol−1 for salvinorin A and DCG, respectively (Fig. 4A). Binding mode analysis shows that salvinorin A bound at the interface and interacted with Tyr505, Arg403, Tyr463, and Lys417 from the RBD and residues from the human ACE2 were His42, Asn33, Glu37, and Arg393 thereby, blocking the interaction of the human ACE2 and the viral RBD hence preventing its entry into human cells (Fig. 4B). Interestingly, although DCG bound at the same place as salvinorin A, it has a different binding mode with additional interacting residues which could be attributed to its observed strong binding affinity. The furan ring in DCG is pointing towards the spike RBD while in salvinorin A, the furan points towards the human ACE2 (Fig. 4B). Both docking calculation based on rigid and ensemble structures from MD simulation suggests that salvinorin A could show activity in vitro, despite it having slightly less affinity as compared to DCG. Indeed, this observation is consistent with our in vitro assay described in subsequent subsections. Previous in silico and in vitro studies have shown that both ligands or peptides binding at the spike ACE2–RBD and interacting with Tyr505, Arg403, Lys417, His42, Glu37, Asn33 and Arg393 can potentially block viral cell entry.24,31–33
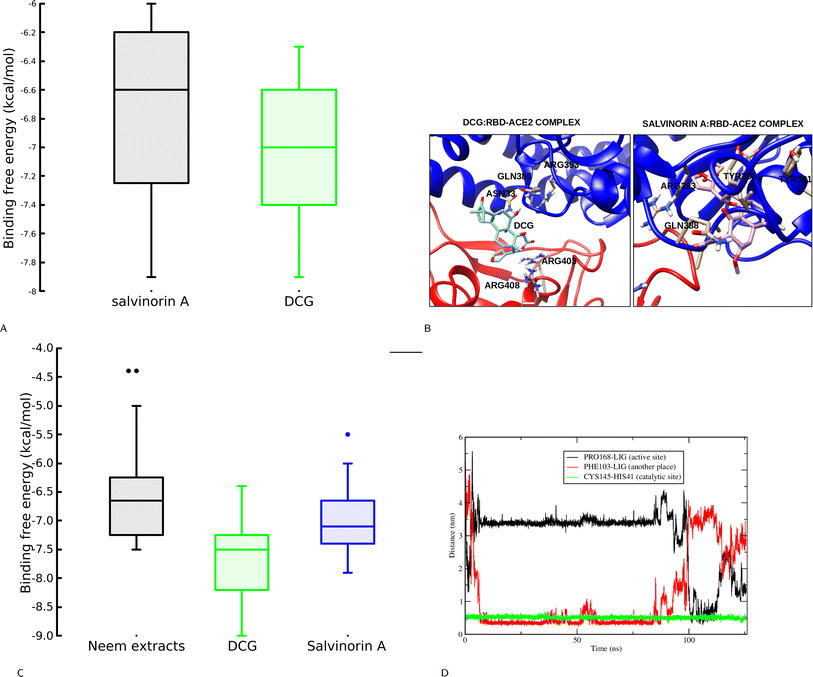 |
| Fig. 4 (A) Binding free energy distribution for salvinorin A and DCG during a relaxed complex scheme based molecular docking calculation. DCG shows a superior binding affinity compared to salvinorin A. (B) Binding orientation of salvinorin A and DCG at the RBD–ACE2 pocket, the furan ring in salvinorin A points toward the ACE2 while DCG points toward the viral RBD. (C) Binding free energy aginst the Mpro, DCG shows more inhibition activity, (D) the catalytic residues distances upon binding of salvinorin A to the Mpro. | |
3.3 Inhibition of Mpro by salvinorin A and DCG
To gain a deeper understanding of the potential inhibitory effect of salvinorin A and DCG on SARS-CoV-2 Mpro, we next conducted molecular dynamics-based docking calculations to assess the stability of its binding to the target protein. The Mpro's binding pocket and structure have been extensively characterized, consisting of three distinct subunits: S1, S2, and S3 with three domains I–III (domain I, residues 8–101, domain II, residues 102–184 and domain III, residues 201–303).34,35 It is known that a co-crystallized N3 covalent inhibitor binds to the catalytic dyad cavity which can accommodate four substrate residues spanning from position P1′ to P4.34,35 Our initial docking calculations indicated that salvinorin A binds to the Mpro pocket with a binding affinity of −6.2 kcal mol−1. It forms interactions with P′ catalytic dyad cavity residues His41 and Cys145. The limitations of the current docking algorithm, such as the lack of global protein flexibility and the omission of water molecules, caution us against placing full trust in docking poses and affinities. Consequently, we conducted classical molecular dynamics (MD) simulations to gain deeper insights into binding stability. MD simulations offer the advantage of providing protein sampling and flexibility, allowing the ligand to explore various binding poses more freely. We further extracted 20 snapshots from the MD simulation and performed docking calculations. It is interesting, to note that, when neem extracts docked to single crystal structures DCG exhibited a superior binding affinity of −7.5 kcal mol−1. DCG and salvinorin A were further docked to the 20 ensemble structures, it's worth noting, that ensemble structures improved the sensitivity of docking, as a result, both DCG and salvinorin A had good scores compared to the crystal structure (Fig. 4C).
The MD simulation of salvinorin A complex demonstrated a dynamic behavior by shifting from its initial docking position (P1′) within the first 5 nanoseconds, eventually accommodating the cavity P4 where the N3 inhibitor binds. After 100 nanoseconds, salvinorin A resumed its original docking pose at the cavity (P1′) interacting with the catalytic dyad residues with fluctuations (Fig. 4D). The observed dynamical changes suggest that salvinorin A may undergo different adaptations inside the cavity, potentially leading to the inhibition of viral enzymatic activities. Furthermore, this hypothesis is supported by the increased distance of the catalytic dyad residues His41–Cys145, which measured 5 Å throughout the simulation time, compared to the crystal structure distance of 3.8 Å (Fig. 4D). Notably, Mpro's enzymatic activity hinges on catalytic site reactions, and inhibiting these activities disrupts viral polymerization, ultimately curbing SARS-CoV-2 viral replication. These findings indicate that salvinorin A exhibits activity against Mpro, albeit with a moderate inhibitory potential. This observation is consistent with the results reported by Ullah et al., through docking calculations.36 Our in silico findings align well with our in vitro results, where salvinorin A displayed a moderate yet significant inhibition of Mpro and viral replication.
3.4 In vitro inhibition of SARS-CoV-2 by salvinorin A and plant extracts
Previous in silico subsections have highlighted the potential of salvinorin A as a potential lead to inhibit SARS-CoV-2 through multiple mechanisms. While salvinorin A was readily obtained commercially, we were unable to purchase DCG due to supplier issues. To circumvent this gap, we then searched for plants with documented high levels of DCG. Literature shows that DCG, besides being found in neem trees, can be isolated from Cedrela odorata.37 This piqued our interest to investigate the potential of DCG derived from Cedrela odorata, CO, and neem tree (Azadirachta indica, AI). As a result, we extended our research to include the assessment of crude extracts from CO and AI for their anti-SARS-CoV-2 activities.
To assess the effects of salvinorin A and the plant extracts on live SARS-CoV-2 replication, we performed cell viability restoration studies in the presence of infectious SARS-CoV-2 as previously described.29 Briefly, Vero-E6 cells were incubated with the compound for 2 hours before infection with SARS-CoV-2 (USA-WA1/2020, “wild-type” variant). After 4 days viability of infected cells was measured with Alamar Blue stain. In the absence of the drug, we observed an average 47.4 ± 21.1% (mean ± SD) reduction in viability consistent with cytopathic effects due to virus infection. In this assay, salvinorin A was observed to have moderate but reproducible antiviral activity, with 30.7 ± 1.8% and 11.7 ± 1.2% restoration of cell viability at 50 and 30 μg mL−1, respectively (Fig. 5A). Due to limits of salvinorin A solubility in this assay, we were unable to test higher concentrations; as a result, the full dose–response curve for this compound could not be achieved. In contrast, over 95% and 40.6 ± 11.0% restoration of cell viability was observed in cells treated with 10 or 3 μM, respectively, of control SARS-CoV-2 inhibitor remdesivir (Fig. 5B). These results indicate that the antiviral activity of salvinorin A, at least on its own, does not match the activity of licensed drugs like remdesivir. However, improved activity may be isolated from either compound combinations obtained from parental plants and/or future synthetic derivatives of salvinorin A. Our observation shows no major effects (i.e. >50%) on cell viability in the presence of salvinorin A in uninfected cells (Fig. 5A, purple), indicating that salvinorin A did not induce major cytotoxicity at antiviral concentrations.
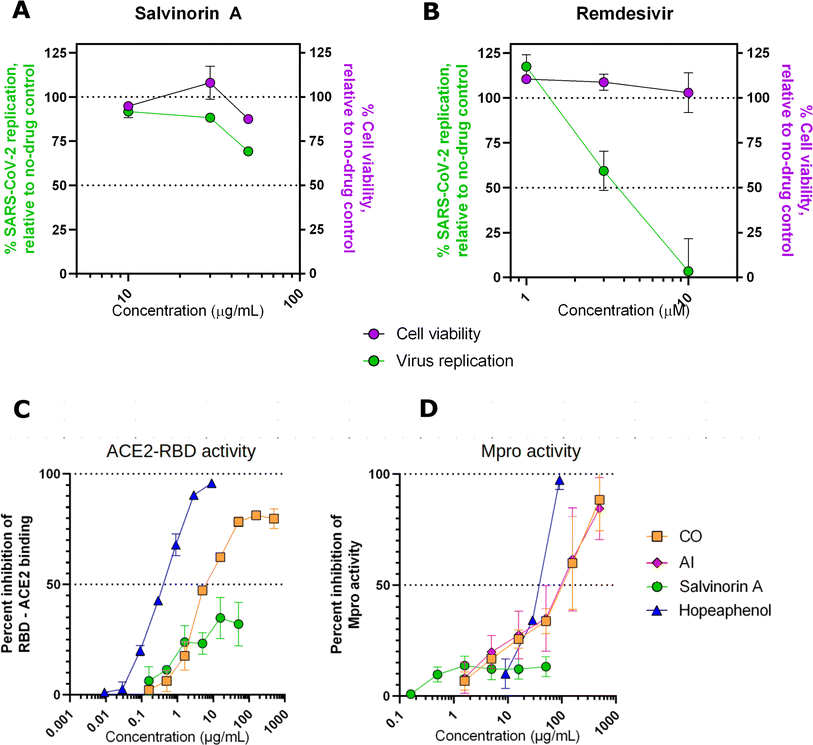 |
| Fig. 5 Effects of salvinorin A (A), and control inhibitor remdesivir (B) on viability of uninfected Vero-E6 cells (purple) and SARS-CoV-2 replication in infected Vero-E6 cells (green), as measured by virus-induced cytopathic effect. Effects of salvinorin A and plant extracts from CO and AI on the spike RBD–ACE2 binding (C) and Mpro activity (D). | |
Interestingly, as observed in our in silico calculations, salvinorin A demonstrated an appreciable viral cell entry inhibition at 10 μg mL−1 by inhibiting at least 40%, while CO crude extract showed more activity at 7 μg mL−1 by inhibiting 50% of RBD–ACE2 binding (AI could not be assessed in this assay due to autofluorescence issues). This suggests that both salvinorin A and the crude extracts possess spike RBD–ACE2 viral entry inhibitory activities. We noted that both crude extracts from Azadirachta indica (neem tree) and Cedrela odorata also exhibited strong activities compared to the pure salvinorin A. Both extracts were also able to inhibit 50% of the Mpro activities at 100 μg mL−1 concentration, while salvinorin A only inhibited ≈20% of Mpro activities at the same concentration. Based on our current findings, we hypothesize that the crude extracts have other compounds that work synergistically or additively toward inhibiting viral cell entry and the Mpro activities. The re-isolation and testing of individual compounds from the crude extracts of Cedrela odorata is highly recommended.
4 Conclusion
The discovery and development of new therapeutic leads to combat COVID-19 is an ongoing initiative in order to supplement vaccine efforts. Given the rapid advances in computational algorithms and hardware, it has become possible to screen large libraries and identify potential compounds. The work started by screening neem tree extracts and tested them for their potential to inhibit SARS-COV-2 by targeting TMPRRS2, ACE2–RBD, Mpro and viral replication. Salvinorin A obtained through similarity search demonstrated activity both in silico and in vitro by moderately inhibiting viral cell entry, Mpro and replication. Both crude extracts (CO and AI) showed more potent activity than salvinorin A. Further isolation and purification and in vitro testing of individual compounds from CO is therefore recommended. Further in vivo validation is also suggested towards developing more effective therapies for SARS-COV-2.
Data availability
The data supporting this article have been included as part of the ESI.†
Author contributions
Software, writing-review & editing: Mariana J. Shayo, Daniel M. Shadrack, Geradius Deogratias, Ester Innocent, Ian Tietjen, Baraka Samwel, Samson Hilonga. Methodology, data analysis, visualization: Ian Tietjen, Daniel M. Shadrack, Joel Cassel, Joseph M. Salvino, Luis J. Montaner, Daniel M. Shadrack, Geradius Deogratias. Funding acquisition: Ester Innocent, Ian Tietjen, Mariana J. Shayo, Daniel M. Shadrack, Geradius Deogratias. Computational resources: Lucy Kiruri, Daniel M. Shadrack.
Conflicts of interest
There are no conflicts to declare.
Acknowledgements
The authors, MJS, BS, DMS, GD, and EI, acknowledge the funding received from the Swedish International Development Cooperation Agency (Sida seed grant) for Junior Faculty Scheme through Muhimbili University of Health and Allied Sciences. LJM and JS received support from the Wistar Science Discovery Fund, while IT received assistance from the Canadian Institutes for Health Research (CIHR PJT-153057). Additionally, LJM expresses gratitude for the support provided by the Robert I. Jacobs Fund of the Philadelphia Foundation and the Herbert Kean, M. D., Family Professorship. LK and DMS recognize the support for accessing the Lengau high-performance computing facility in South Africa. DMS acknowledges the AGNES junior research grant award.
Notes and references
- J. S. Tregoning, K. E. Flight, S. L. Higham, Z. Wang and B. F. Pierce, Nat. Rev. Immunol., 2021, 21, 626–636 CrossRef CAS PubMed.
- T. Hartonen, B. Jermy, H. Sõnajalg, P. Vartiainen, K. Krebs, A. Vabalas, FinnGen, Estonian Biobank Research Team, T. Leino, H. Nohynek, J. Sivelä, R. Mägi, M. Daly, H. M. Ollila, L. Milani, M. Perola, S. Ripatti and A. Ganna, et al., Nat. Human Behav., 2023, 1–15 Search PubMed.
- Z. Huang, S. Xu, J. Liu, L. Wu, J. Qiu, N. Wang, J. Ren, Z. Li, X. Guo and F. Tao, et al., Nat. Commun., 2023, 14, 2009 CrossRef CAS PubMed.
- J. V. Lazarus, K. Wyka, T. M. White, C. A. Picchio, L. O. Gostin, H. J. Larson, K. Rabin, S. C. Ratzan, A. Kamarulzaman and A. El-Mohandes, Nat. Med., 2023, 1–10 Search PubMed.
- R. J. D. Vergara, P. J. D. Sarmiento and J. D. N. Lagman, J. Publ. Health, 2021, 43, e291–e292 CrossRef PubMed.
- J. V. Lazarus, K. Wyka, T. M. White, C. A. Picchio, K. Rabin, S. C. Ratzan, J. P. Leigh, J. Hu and A. El-Mohandes, Nat. Commun., 2022, 13, 3801 CrossRef CAS PubMed.
- A. I. Mohamud, S. A. Mohamed and K. Abdullahi, IOSR J. Dent. Med. Sci., 2021, 20, 1–4 Search PubMed.
- M. D. H. Hawlader, M. L. Rahman, A. Nazir, T. Ara, M. M. A. Haque, S. Saha, S. Y. Barsha, M. Hossian, K. F. Matin and S. R. Siddiquea, et al., Int. J. Infect. Dis., 2022, 114, 1–10 CrossRef CAS PubMed.
- J. Shawon, Z. Akter, M. M. Hossen, Y. Akter, A. Sayeed, M. Junaid, S. S. Afrose and M. A. Khan, Curr. Pharm. Des., 2020, 26, 5241–5260 CrossRef CAS PubMed.
- A. Hensel, R. Bauer, M. Heinrich, V. Spiegler, O. Kayser, G. Hempel and K. Kraft, Planta Med., 2020, 86, 659–664 CrossRef CAS PubMed.
- H. Zhang, J. M. Penninger, Y. Li, N. Zhong and A. S. Slutsky, Intensive Care Med., 2020, 46, 586–590 CrossRef CAS PubMed.
- S. Jo, S. Kim, D. H. Shin and M.-S. Kim, J. Enzym. Inhib. Med. Chem., 2020, 35, 145–151 CrossRef CAS PubMed.
- A. d. S. Antonio, L. S. M. Wiedemann and V. F. Veiga-Junior, RSC Adv., 2020, 10, 23379–23393 RSC.
- Y. Chen, Q. Liu and D. Guo, J. Med. Virol., 2020, 92, 418–423 CrossRef CAS PubMed.
- Y. W. Chen, C.-P. B. Yiu and K.-Y. Wong, F1000Research, 2020, 9, 1–19 Search PubMed.
- B. Krichel, S. Falke, R. Hilgenfeld, L. Redecke and C. Uetrecht, Biochem. J., 2020, 477, 1009–1019 CrossRef CAS PubMed.
- K. Anand, J. Ziebuhr, P. Wadhwani, J. R. Mesters and R. Hilgenfeld, Science, 2003, 300(5626), 1763–1767 CrossRef CAS PubMed.
- V. Mody, J. Ho, S. Wills, A. Mawri, L. Lawson, M. C. Ebert, G. M. Fortin, S. Rayalam and S. Taval, Commun. Biol., 2021, 4, 93 CrossRef CAS PubMed.
- J. Zhu, H. Zhang, Q. Lin, J. Lyu, L. Lu, H. Chen, X. Zhang, Y. Zhang and K. Chen, Drug Des., Dev. Ther., 2022, 1067–1082 CrossRef CAS PubMed.
- D. M. Shadrack, S. A. Vuai, M. G. Sahini and I. Onoka, RSC Adv., 2021, 11, 26524–26533 RSC.
- N. M. O'Boyle, M. Banck, C. A. James, C. Morley, T. Vandermeersch and G. R. Hutchison, J. Cheminf., 2011, 3, 1–14 Search PubMed.
- O. Trott and A. J. Olson, J. Comput. Chem., 2010, 31, 455–461 CrossRef CAS PubMed.
- D. M. Shadrack, H. S. Swai and A. Hassanali, J. Mol. Graph. Model., 2020, 96, 107510 CrossRef CAS PubMed.
- D. M. Shadrack, G. Deogratias, L. W. Kiruri, I. Onoka, J.-M. Vianney, H. Swai and S. S. Nyandoro, J. Mol. Model., 2021, 27, 1–15 CrossRef PubMed.
- G. Bussi, D. Donadio and M. Parrinello, J. Chem. Phys., 2007, 126, 014101 CrossRef PubMed.
- M. Parrinello and A. Rahman, J. Appl. Phys., 1981, 52, 7182–7190 CrossRef CAS.
- B. Hess, H. Bekker, H. J. Berendsen and J. G. Fraaije, J. Comput. Chem., 1997, 18, 1463–1472 CrossRef CAS.
- R. Kumari, R. Kumar, O. S. D. D. Consortium and A. Lynn, J. Chem. Inf. Model., 2014, 54, 1951–1962 CrossRef CAS PubMed.
- I. Tietjen, J. Cassel, E. T. Register, X. Y. Zhou, T. E. Messick, F. Keeney, L. D. Lu, K. D. Beattie, T. Rali and P. Tebas, et al., Antimicrob. Agents Chemother., 2021, 65, 10–1128 CrossRef PubMed.
- J.-H. Lin, A. L. Perryman, J. R. Schames and J. A. McCammon, J. Am. Chem. Soc., 2002, 124, 5632–5633 CrossRef CAS PubMed.
- M. Quagliata, M. A. Stincarelli, A. M. Papini, S. Giannecchini and P. Rovero, ACS Omega, 2023, 8(25), 22665–22672 CrossRef CAS PubMed.
- S. Pourmand, S. Zareei, M. Shahlaei and S. Moradi, Comput. Biol. Med., 2022, 146, 105625 CrossRef CAS PubMed.
- G. Jaiswal and V. Kumar, PLoS One, 2020, 15, e0240004 CrossRef CAS PubMed.
- Z. Jin, X. Du, Y. Xu, Y. Deng, M. Liu, Y. Zhao, B. Zhang, X. Li, L. Zhang and C. Peng, et al., Nature, 2020, 582, 289–293 CrossRef CAS PubMed.
- D. W. Kneller, G. Phillips, H. M. O'Neill, R. Jedrzejczak, L. Stols, P. Langan, A. Joachimiak, L. Coates and A. Kovalevsky, Nat. Commun., 2020, 11, 3202 CrossRef CAS PubMed.
- S. Ullah, B. Munir, A. G. Al-Sehemi, S. Muhammad, I.-u. Haq, A. Aziz, B. Ahmed and A. Ghaffar, Saudi J. Biol. Sci., 2022, 29, 103274 CrossRef CAS PubMed.
- T. S. R. Nogueira, M. d. S. Passos, L. P. S. Nascimento, M. B. d. S. Arantes, N. O. Monteiro, S. I. d. S. Boeno, A. d. C. Junior, O. d. A. Azevedo, W. d. S. Terra and M. G. C. Vieira, et al., Molecules, 2020, 25, 5401 CrossRef CAS PubMed.
|
This journal is © The Royal Society of Chemistry 2024 |
Click here to see how this site uses Cookies. View our privacy policy here.