DOI:
10.1039/D4RA03019B
(Paper)
RSC Adv., 2024,
14, 26873-26883
Enhancement of weak signals by applying a suppression method to high-intense methyl and methylene signals of lipids in NMR spectroscopy†
Received
23rd April 2024
, Accepted 18th August 2024
First published on 27th August 2024
Abstract
Lipids play crucial roles in human biology, serving as energy stores, cell membranes, hormone production, and signaling molecules. Accordingly, their study under lipidomics has advanced the study of living organisms. 1-Dimensional (D) and 2D NMR methods, particularly 1D 1H and 2D 1H–1H Total Correlation Spectroscopy (TOCSY), are commonly used in lipidomics for quantification and structural identification. However, these NMR methods suffer from low sensitivity, especially in cases of low concentrated molecules such as protons attached to hydroxy, esters, aliphatic, or aromatic unsaturated carbons. Such molecules are common in complex mixtures such as dairy products and plant oils. On the other hand, lipids have highly populated fractions of methyl and methylene groups that result in intense peaks that overwhelm lower peaks and cause inhomogeneities in 2D TOCSY spectra. In this study, we applied a method of suppression to suppress these intense peaks of methyl and methylene groups to detect weaker peaks. The suppression method was investigated on samples of cheese, butter, a mixture of lipids, coconut oil, and olive oil. A significant improvement in peak sensitivity and visibility of cross-peaks was observed, leading to enhanced comparative quantification and structural identification of a greater number of lipids. Additionally, the enhanced sensitivity reduced the time required for the qualitative and comparative quantification of other lipid compounds and components. This, in turn, enables faster and more reliable structural identification and comparative quantification of a greater number of lipids. Additionally, it reduces the time required for the qualitative, and comparative quantification due to the enhancement of sensitivity.
Introduction
Lipids play crucial roles in the human body, serving various functions essential for health and normal physiological processes. Lipids contribute to cell structure, membrane functions, energy storage, insulin resistance, temperature regulation, hormone growth, brain functions, cell signaling, cushioning, inflammation, immunity, vitamin absorption, electrochemical gradients, and more.1–18 At the same time, lipid dysfunction is associated with many diseases including cardiovascular diseases,19–21 Alzheimer's disease,22,23 inflammations,24 and metabolic disorders such as hypertension,25,26 diabetes,27,28 hyperlipidemia,29 and obesity.30–32
Lipidomics, a subdivision of metabolomics, involves the comprehensive investigation of pathways and interconnected networks related to cellular lipids at several biological hierarchies – proteins, cells, tissues, and organisms.33,34 It entails the precise assessment and categorization of lipids across both temporal and spatial dimensions, encompassing both quantitative and qualitative analysis, the examination of lipid transporters, enzymes involved in lipid metabolism, and the exploration of fatty acids–protein interactions.35–38
In the field of metabolite research, nuclear magnetic resonance (NMR) and mass spectrometry (MS) are frequently used in metabolite profiling.39–49 NMR analysis is advantageous because of its high reproducibility, but it has the disadvantage of low sensitivity, making it unsuitable for the analysis of low-concentration metabolites in samples, such as lipid metabolites.50,51 The precise chemical identification of lipids is important for assessing the metabolite composition and nutritional content of food products as well as in creating accurate nutritional labels tailored to specific functions or purposes.52 Analyzing lipids is notably intricate and challenging, as it involves significant time and labor, along with the need for diverse preparatory and analytical procedures.53
Lipidomics has been studied using an array of chromatography and mass spectrometry techniques, such as gas chromatography-mass spectrometry (GC-MS), gas chromatography-tandem mass spectrometry (GC-MS/MS), liquid chromatography-mass spectrometry (LC-MS), and liquid chromatography-tandem mass spectrometry (LC-MS/MS).54–58 However, the use of GC involves multiple manipulation and derivatization steps, potentially leading to lipid oxidation59 and undesired isomerization processes.60 NMR-based metabolomics is widely used in various fields, including nutrition research,61 and in the realm of food-omics, NMR-based approaches have proven successful in exploring variations in metabolite profiles.62–64 Research on the lipid fractions of milk and other dairy products has focused on the 1H-NMR characterization of cow and buffalo milk,65,66 the 13C-NMR investigation of milk across various animal species,67,68 and 1H and 13C-NMR techniques for the identification of the production chain of Asiago d'Allevo cheese.68 These studies have demonstrated that NMR spectroscopy is a reliable, rapid, and effective tool for analytical and structural investigations and requires minimal derivatization steps or specific sample preparation procedures.45,69 1D 1H NMR is used for the qualitative, and quantitative analysis of lipids,70,71 but it produces a wide range of peak intensities that complicate the quantification of weak peaks. Because aliphatic and aromatic unsaturated protons have longer longitudinal relaxation times, quantifying such peaks requires more experimental time.52 2D TOCSY NMR has been used for the structural identification of different types of molecules, such as amino acids, small organic compounds, biomolecules, and lipids52 by correlating protons within a molecule. However, it has exhibited limited performance for low concentrations of molecules, unsaturated aliphatic, and aromatic components of lipids.72,73
To expand the effectiveness of NMR to low-concentration molecules, in the present study, we suppressed methyl and methylene lipid signals (SMLS) in the 1D 1H, and 2D TOCSY.74 This approach improved the sensitivity and visibility of other lipid signals in both 1D 1H and 2D TOCSY. The method, followed by a recently published technique known as suppression of sugar's moiety signals (SSMS),75 was applied to different types of samples including stock solutions containing lipids, dairy, and oil products.
Pulse sequences of SMLS with and without 1D 1H and 2D TOCSY NMR
Fig. 1A shows the pulse sequence for acquiring the 1H-SMLS spectra. A conventional 1H pulse sequence is shown in Fig. 1B. Both experiments were recorded in magnitude mode using the window function exponential multiplication (EM). 1H-SMLS involves the strategic application of selective excitation to selected regions of chemical shifts for the effective suppression of unwanted signals. This method employs double band-selective excitation pulses at a specified frequency with dephasing gradients, featuring a 90° flip angle. Subsequently, dephasing gradients, known as homogeneous spoiling gradients, are applied to the dephasing of the signal coherence.77 This process leads to a condition where there is a minimal residual magnetization of undesirable signals, with the desired signals remaining entirely unaltered and preserving their z-magnetization. The mechanism for SMLS has been described elsewhere.75
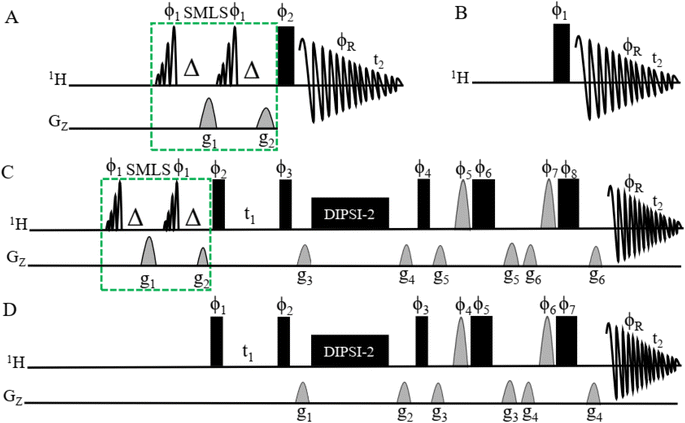 |
| Fig. 1 (A) Pulse sequences obtained by 1D 1H-SMLS NMR. The SMLS block was combined with the conventional 1D 1H NMR method. The SMLS block had two 90° pulses of EBurp2 76 for excitation of the selected bandwidth. The bandwidth of the pulse was 1000 Hz for 5 ms to cover the regions of signals of methyl, methylene, and methylene near the first methylene groups which are found in higher numbers compared to the number of other functional groups, and the phases are ϕ1 = x. Δ is the evolutionary duration of the homonuclear coupling nuclei of protons and less than 1/4 3JHH. It has two gradients for the dephasing of magnetization: g1 = 47%, and g2 = 27%. The rectangular black bar shows a hard 90° pulse with phase ϕ2 = x −x −x x y −y −y y, and receiver phase ϕR = x −x −x x y −y −y y. t2 is the time for acquiring the free induction decay (FID). (B) The pulse sequence of conventional 1D 1H NMR. The parameters have the same definitions as for 1D 1H-SMLS. This sequence excludes the SMLS block. Spectra of different types of samples were recorded using 1D 1H and 1D 1H-SMLS for comparison. (C) The pulse sequence of 2D SMLS-TOCSY NMR with the SMLS block to suppress the methyl and methylene regions. See (A) for details about the SMLS block. The narrow rectangular filled black bars are 90° hard pulses with phases of ϕ2 = x −x, ϕ3 = x x x x −x −x −x −x, and ϕ4 = x x x x x x x x −x −x −x −x −x −x −x −x, and the broad rectangular filled black bars are 180° hard pulses with phases of ϕ6 = −x −x −y −y, and ϕ8 = −x −x −x −x −y −y −y −y. The half sine shapes with gray colors are 180° angled pulses of ϕ5 = x x y y and ϕ7 = x x x x y y y y. The phase of the receiver ϕR = x −x −x x x −x −x x −x x x −x −x x x −x. Gradients are g3 = 1%, g4 = 3%, g5 = 31%, and g6 = 11%. DIPSI-2 was used to mix coherences for 60 ms. Durations t1 and t2 are homonuclear coupling evolutions and collections of FID, respectively. (D) The pulse sequence of conventional 2D TOCSY NMR. The setup is the same as in (C) except for the exclusion of the SMLS block. | |
The band-selective excitation pulses for the SMLS block are integrated into 2D TOCSY, as shown in Fig. 1C. The pulse sequence of SMLS method shown in dotted green line boxes in (Fig. 1A and C) utilized in the structured based on the principles of the WET method.77,78 This methodology, originally designed for signal suppression, shares common elements, including band-selective pulses for the targeted excitation of undesirable signals and dephasing gradients for their effective dephasing. The pulse sequence of conventional 2D TOCSY NMR is shown in Fig. 1D and was used as a comparison with the SMLS-TOCSY NMR method for different types of samples.
Results
A 1H NMR spectrum assigned with respective functional groups of lipids in dairy products dissolved in deuterated chloroform is shown in Fig. 2(A–C).
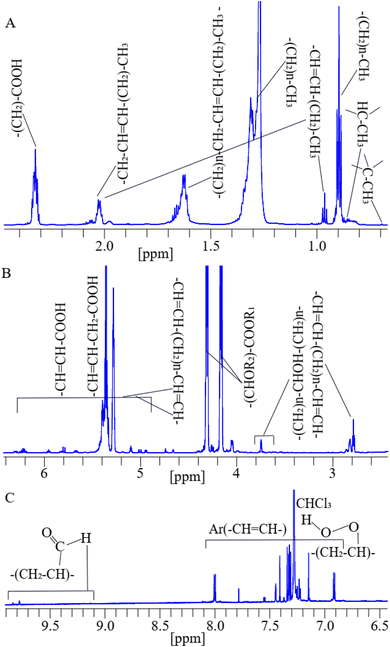 |
| Fig. 2 A representative 1D 1H-NMR spectrum assigned with functional groups of lipids at their corresponding chemical shifts of a cheese sample dissolved in deuterated chloroform.79,80 | |
The assigned peaks of lipids are as follows: –C–CH3 (0.70 ppm), –CH–CH3 (0.78 ppm), (CH2)n–CH3 (0.84 ppm), and (–CH3) (0.98 ppm) adjacent to vinyl groups in lipids, the (CH2)n– (1.34–1.75 ppm) methylene groups are bones of lipids, (–CH2–CH
CH–CH2–)n (2.00 ppm), and (OOC–CH2–CH2–)n (2.35 ppm) shown in (Fig. 2A), (–CH
CH–CH2–CH
CH–)n (2.75 ppm), (–CH2)n–CHOH–(CH2)n– (3.50–3.75 ppm), (–CH
CH–CHOR–CH–)n (4.11–4.35 ppm), (–CH2–CH
CH–CH2–)n (5.12–5.92 ppm), and (HOOC–CH
CH–)n (6.54 ppm) shown in (Fig. 2B), aromatic (Ar) (–CH
CH–)n of phenolic compounds,81 primary oxidized lipid into lipid-hydroperoxides82–84 (7.11–8.20 ppm), and secondary oxidized aldehydic-lipids85 (9.20–9.80 ppm) shown in (Fig. 2C). Double bonds show unsaturated fatty acids. C–CH3 and –CH–CH3 groups are found in cholesterol, and (–CH
CH–CHOR–CH–)n indicates ester groups.80,86,87
Application of the suppression method to the cheese sample
Fig. 3A shows two parts as 1D 1H (Fig. 3m) and 1H-SMLS (Fig. 3n) were used to analyze of cheese sample. The results show that by selectively suppressing the methyl (0.71–0.98 ppm) region by a residue factor of 0.08 and the methylene (1.34–1.75 ppm) region by a residue factor of 0.20 in 1H-SMLS compared to 1D 1H where original intensity of peaks was considered as normalized unit, the enhanced sensitivity of the low intense peaks by a factor of 2.77 in the 1H-SMLS spectrum compared to the 1H spectrum shown in sections (a)–(c) of Fig. 3A. Residue factor was measured as the relative intensity of signals of 1D 1H and 1H-SMLS spectra where intensity of signals of 1D 1H was considered as one.88 At the highest noise levels of both spectra in the regions of the aromatic, aldehydic, amine, and carboxylic groups, we could quantify the peak heights better in 1H-SLMS than the 1H method.
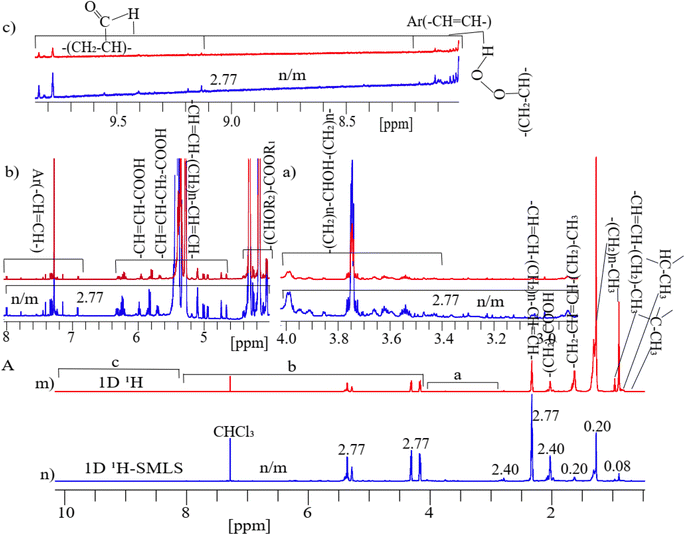 |
| Fig. 3 (A) Typical 1D 1H-NMR full spectra from conventional (m) 1D 1H and (n) 1H-SMLS of a sample of cheese dissolved in deuterated chloroform. These spectra were divided into three sections for a clear view of spectral peaks. Sections with chemical shift regions 2.90 to 4.00 ppm (a), 4.12 to 8.00 ppm (b), and 8.01 to 9.58 ppm (c) of (A). | |
At the highest saturation level of cheese (dissolved in chloroform) resulted in the highest concentration of lipids, preservatives, and other impurities, but their concentration levels for NMR are still low where methyl and methylene peaks dominate other signals (see Fig. 3A). Suppressing these peaks revealed increased sensitivity of peaks of the hydroxy, ester, aliphatic, primary oxidized lipid into lipid-hydroperoxides, and aromatic regions because level of the suppression of these two types of intense signals cause results in proportional increment.75
2D TOCSY and SMLS-TOCSY spectra of cheese samples were shown in Fig. 4A and B, respectively. The inhomogeneity of the functional groups in the case of their fractional availability in the lipids resulted in ridges of high-intensity peaks, complicating the analysis. Such as, the methyl and methylene groups were present in the highest ratio among all groups, resulting in high-intensity peaks that overwhelmed the cross peaks correlated with other groups. The overwhelming of the cross-peaks and inhomogeneity of the cross-peak intensities were reduced by applying a band-selective suppression of the methyl and methylene groups, which resulted in a more homogeneous and more visible cross-peaks over the whole spectrum (Fig. 4B). 2D spectra were compared at the same contour levels to acquire significantly better visibility of the cross-peaks with higher number in SMLS-TOCSY spectrum compared to TOCSY spectrum in the region 2.60–6.80 ppm along the F1-dimension (Fig. 4C). A reduction but not complete disappearance of cross-peaks in the region 3.20–4.00 ppm along F2-dimension, where these cross-peaks appeared due to correlation with the methyl and methylene groups, was observed. These cross-peaks play an important role in the structural identification of compounds by providing information about the correlation among protons, helping us identify compound skeletons. A reduction in cross-peak visibility of the hydroxy regions along F2-dimension is achieved by reducing the contour levels. The visibility of the peaks was increased in hydroxy, esters, and (aliphatic, and aromatic) unsaturated regions by using SMLS-TOCSY compared to TOCSY. Suppressing the methyl groups (0.75–0.98 ppm) by factors of 0.03 and 0.65 and the methylene groups (1.34–1.75 ppm) by factors of 0.07 and 0.27, where these fractions are the residues of suppressed peak intensities, rest of peak intensities are increased by a factor of 1.83 (Fig. S1A†). Similarly, the visibility of the peak intensities increased by a factor of 1.83 in the 3.00–5.15 ppm region and the appearance of new peaks, especially in the unsaturated aliphatic and aromatic groups, as well as the peak intensity in the aromatic region was achieved by factors of 8.89, 4.49, 50.00, and 7.46 due to peaks having different longitudinal relaxation times (Fig. S1†).
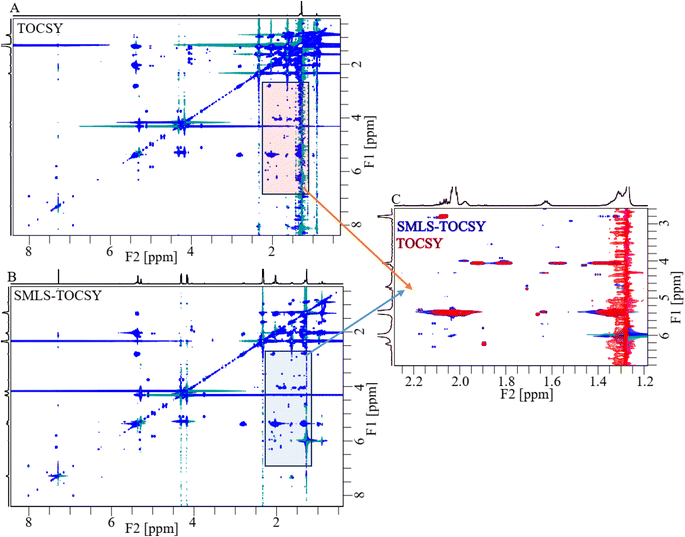 |
| Fig. 4 (A) A conventional 2D TOCSY spectrum and (B) a suppressed 2D SMLS-TOCSY spectrum. (C) Magnification of the rectangular shaded areas in (A) and (B). Suppression of the methyl and methylene groups by band-selective excitation leads to enhance visibility of revealed the cross-peaks underlying them. | |
For the comparison of 2D-SMLS-TOCSY with 2D-Suppression of Unwanted Signals (SUN)-TOCSY89 to evaluate the potential advantages of the former method over the latter. Upon comparing the 2D spectra of SMLS-TOCSY with that of SUN-TOCSY at identical contour levels, the appearance of cross-peaks is similar, encompassing both dimensions, as well as the suppression levels of methyl and methylene groups in both spectra. However, the suppression of the water signal at 1.30 ppm is less effective in the SUN-TOCSY, resulting in a more intense axial peak of zero-order along the F1-dimension compared to the SMLS-TOCSY. This is a notable drawback of the SUN-TOCSY method relative to the SMLS-TOCSY method. Despite this, the overall number of cross-peaks remains equal at different contour levels in both spectra.
Application of suppression method to butter sample
Applying the same suppression method to 1D 1H on the butter sample resulted in a significant suppression of the methyl groups (0.70–0.98 ppm) by residue factors of 0.05, and 0.13 and of the methylene groups (1.34–1.75 ppm) by residue factors of 0.11 and 0.16 (Fig. S3A†). This suppression resulted in enhancement of sensitivity of peaks of the hydroxy, ester, aliphatic, and aromatic regions by a factor of 2.65 shown in sections (a), and (b) of Fig. S3A.†
The spectra of both 2D TOCSY and SMLS-TOCSY overlapped each other at similar contour levels, which made it possible to see changes in the visibility of cross-peaks when applying the suppression method (Fig. 5).
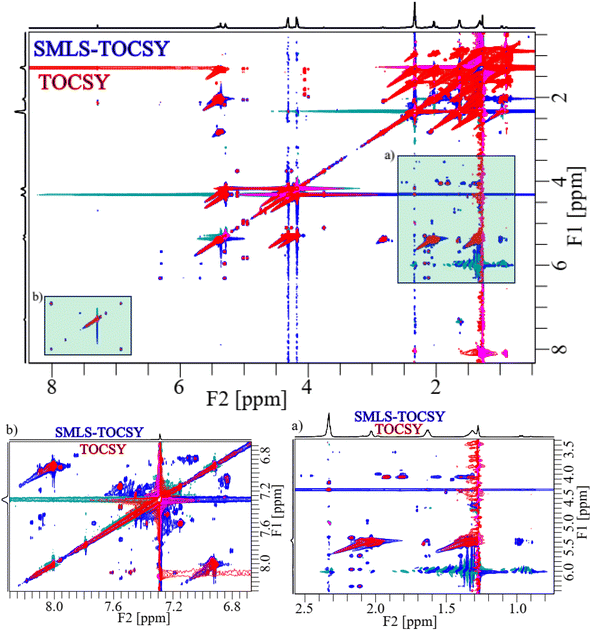 |
| Fig. 5 2D overlapped spectra of TOCSY (red) and SMLS-TOCSY (blue) of the butter sample. The green shaded rectangles (a) and (b) were expanded below. | |
The visibility of the cross-peaks in the aliphatic unsaturated, and hydroxy regions increased significantly along F1-dimension shown in light green shaded rectangular (a) of Fig. 5, which assisted with tracing the low intensity peaks. Similarly, increased visibility in the case of aromatic unsaturated region shown in light green shaded rectangular (b) of Fig. 5. The expanded spectra of these shaded rectangular regions (a), and (b) of Fig. 5 were shown for clear view to observe increased visibility. As expanded spectra with acceptable noise levels, revealing better sensitivity, and visibility in the cross-peaks in the spectrum of SMLS-TOCSY compared to that of TOCSY.
Projected 1D 1H of TOCSY and SMLS-TOCSY were compared. A significant suppression of the methyl groups (0.70–0.98 ppm) by residue factors 0.05 and 0.78 and of the methylene groups (1.34–1.75 ppm) by residue factors 0.11 and 0.33 greatly enhanced the visibility of highly intense peaks by a factor of 3.00 in the projected 1D 1H of SMLS-TOCSY and of TOCSY (Fig. 6B). The visibility of low intense peaks increased on average 3.12 times in the region 2.70–5.15 ppm, and an average of 3.54 times in the region 5.89–9.90 ppm in which resonances of lipid-aldehydes from its secondary oxidized lipids near 10 ppm chemical shifts shown in sections (a) and (b) of Fig. 6A.
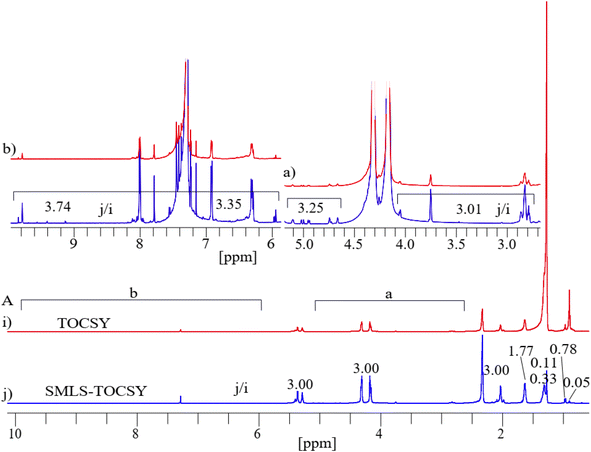 |
| Fig. 6 (A) Projected 1D 1H spectra of (i) 2D TOCSY and (j) 2D SMLS-TOCSY in stacked spectra with the estimated numbers of the peak intensities of the butter sample. A significant sensitivity enhancement was achieved using the suppression method. | |
The 2D-SMLS-TOCSY spectrum exhibits cross-peaks within the green-shadowed rectangular box, whereas the 2D-SUN-TOCSY spectrum shows an absence of these cross-peaks within its corresponding green box at identical contour levels. A similar issue in the 2D-SUN-TOCSY method is the appearance of axial peaks due to the evolution of zero-order coherence of the water signal along the F1-dimension, in contrast to the SMLS-TOCSY. Nevertheless, the overall number of cross-peaks remains consistent across different contour levels in both spectra.
Application of the suppression method to lipid stock solution
Applying the suppression method to 1D 1H in a stock solution of lipids significantly suppressed the methyl signals by a residue factor of 0.04 and the methylene groups signal by an average residue factor of 0.13 to reveal the enhanced sensitivity of peaks of hydroxy, ester, methylene adjacent to unsaturated, aliphatic, and aromatic unsaturated groups shown in (v) of Fig. S5A.† The sensitivity of the high-intensity peaks was enhanced by a factor of 2.89, while that of the low intensity peaks was enhanced by a factor of 3.01 in 1D 1H-SMLS in sections (a)–(c) of Fig. S5A.† For 2D TOCSY (Fig. S6A†), the suppression method significantly suppressed the methyl and methylene signals by residue factors of 0.10 and 0.18, respectively, leading to more visible cross-peaks that were overwhelmed by the ridges of the high-intensity peaks of the methyl and methylene groups in 2D SMLS-TOCSY (Fig. S6B†). Applying the suppression method, more intense cross-peaks were observed in the hydroxy, aliphatic, and aromatic regions, whereas a reduction in the intensity of cross-peaks correlated with the methyl and methylene regions along F2-dimension. A comparison between projected 1D 1H NMR of these two 2D TOCSY methods was done to estimate the peak intensities in their corresponding numbers of enhancements sensitivity of peaks. Peaks for the methyl and methylene groups were suppressed by residue factors of 0.10 and 0.18, respectively (Fig. S7A†). The projected spectra of TOCSY and SMLS-TOCSY showed the visibility of peaks for the hydroxy and methylene groups adjacent to unsaturated and aliphatic unsaturated regions increased by a factor of 5.46, that of the aromatic region increased by 3.91 times, and that for peaks at 9.50 ppm and 9.69 ppm were enhanced by 2.75 and 4.78 times, respectively shown in sections (a), and (b) of Fig. S7A.† These enhancements depended on the time of the longitudinal restoration of the equilibrium where aliphatic and aromatic unsaturated protons have longer longitudinal relaxation times lies in the ranges (2.8–4.9) seconds,90 and (3.33–6.19) seconds,91 respectively.
Application of suppression method to coconut oil sample
The utilization of the suppression method to 1D 1H on a coconut oil sample significantly suppressed the methyl signals by a residue factor of 0.16 and the methylene signals by an average residue factor of 0.30, leading to greater enhancement of sensitivity of signals of the other groups shown in (n) of Fig. S8A.† The enhancement of high-intensity peaks was enhanced by a factor of 6.38 and of low intensity peaks by a factor of 5.41 in the regions 2.45–4.00 ppm and 4.02–5.82 ppm and 7.50 times in the 6.90–9.56 ppm region in 1D 1H-SMLS compared to 1D 1H shown in sections (c)–(e) of Fig. S8A.† Applying the suppression method to 2D TOCSY NMR (Fig. S9A†) analysis that suppressed the high-intensity signals of the methyl and methylene groups in 2D SMLS-TOCSY (Fig. S9B†). This suppression resulted a significant reduction of the ridges of these signals, resulting in more visibility in the 2.50–5.50 ppm region along F1-dimension and in other regions. Extracting projections of 1D 1H spectra of TOCSY (s) and SMLS-TOCSY (t) in Fig. S10A† shows a great suppression of signals of the methyl groups by a residue factor of 0.03, and methylene groups by residue factors of 0.22 and 0.06, resulting in enhanced sensitivity of peaks by a factor of 1.77 in the 2.00–10.00 ppm region.
Application of suppression method to olive oil sample
The application of the suppression method to 1D 1H on an olive oil sample suppressed the methyl group signals by a residue factor of 0.03 and the methylene group signals by residue factors of 0.20 and 0.13, which revealed enhanced sensitivity of the peaks of hydroxy, ester, adjacent to unsaturated, aliphatic, and aromatic unsaturated groups in 1H-SMLS compared to 1H in (y) of Fig. S11A.† The sensitivity of high-intense peaks was increased by factors of 3.38 and 3.60 and of low-intensity peaks by a factor of 3.60 in the 1D 1H-SMLS spectrum compared to the 1D 1H spectrum shown in sections (a) and (b) of Fig. S11A.† Regarding 2D TOCSY (Fig. S12A†), suppression of signals of the methyl and methylene groups led to more visibility of cross-peaks in the 2.55–6.00 ppm region along F1-dimension in 2D SMLS-TOCSY (Fig. S12B†). Cross-peaks were observed for the hydroxy, ester, and aliphatic unsaturated groups. A comparison between the projected 1D 1H NMR spectra of 2D TOCSY (Fig. S12A†), and 2D SMLS-TOCSY (Fig. S12B†) NMR spectra were estimated the peak intensities with the respective numbers of enhancements. The projected 1D 1H spectra of TOCSY and SMLS-TOCSY were shown in Fig. S13A.† The signals of methyl groups were suppressed by a residue factor of 0.05, and the methylene groups by 0.61 and 0.40, while increased visibility estimated with the peak intensities of the hydroxy, ester, methylene adjacent to unsaturated, and aliphatic unsaturated regions increased by a factor of 5.20 shown in (b) of Fig. S13A.†
Discussion
In most lipidomics research employing 1H NMR-based methods, a process of relative and absolute quantification or spectral binning is usually carried out, followed by a multivariate statistical analysis.92,93 This approach enables the probing of alterations and impurities in lipids compositions found in different types of samples such as dairy products, plants oils, tissue and biofluids. These alterations are caused by different factors including toxin exposure, disease, preservation additives, or other sources of contamination.94–99 2D 1H–1H TOCSY NMR enhances the resolution of lipid signals by splitting the signals across two dimensions, offering improved resolution although it prolongs the experimental time by several hours.100–102
Comparing 1D 1H and 1H-SMLS NMR findings for the cheese sample revealed significant enhancements of peak intensities in the 1H-SMLS spectrum. By selectively suppressing the signals of the methyl and methylene groups with remain residues 0.08, and 0.20, the enhanced sensitivity of the other peaks was revealed by a factor of 2.77 compared to the 1H spectrum (Fig. 3). Similarly, the 2D TOCSY and SMLS-TOCSY spectra showed that the application of band-selective suppression of the signals of methyl and methylene groups with residue values of 0.03 and 0.27, respectively, resulted in more visible cross-peaks. The visibility level of the peaks was improved by a factor of 1.83 for hydroxy, ester, aliphatic unsaturated regions and by 8.89, 4.49, and 7.46 for aromatic regions (Fig. S1†). Applying the suppression method to the 1D 1H analysis of the butter sample resulted in a significant suppression of the signal intensity of the methyl and methylene groups with residue values of 0.05, and 0.11 respectively, the visibility of the other peaks increased by a factor of 2.65 (Fig. S3†). A comparison between the 2D TOCSY and SMLS-TOCSY spectra revealed an increased visibility of cross-peaks, particularly in the hydroxy and unsaturated regions (Fig. 5). The projected 1D 1H spectra showed significant suppression of signals of the methyl and methylene groups with residue values of 0.05 and 0.33 respectively, resulting in a substantial enhanced sensitivity of average 3-fold (Fig. 6). Similarly for the stock solution of lipids, suppression of methyl and methylene signals with residue factors of 0.04, and 0.13, respectively, the enhanced sensitivity of the signals of hydroxy, ester, and adjacent unsaturated groups was increased by a factor of 3.01 in 1H-SMLS compared to 1H (Fig. S5†). The 2D TOCSY spectra showed more visibility of cross-peaks after suppressing the signals for the methyl and methylene groups with residue values of 0.10, and 0.18 respectively. The projected 1D 1H spectra of 2D TOCSY also demonstrated a significant enhancement of visibility for the peaks by factors of 5.46, and 3.91 of aliphatic and aromatic unsaturated regions (Fig. S7†). Regarding the coconut oil sample, a significant suppression of the methyl and methylene group signals with residue values of 0.30, and 0.16 respectively, led to a substantial enhancement of the sensitivity for the peaks of the hydroxy, esters, aliphatic and aromatic unsaturated regions by factors of 5.41, and 7.50, respectively (Fig. S8†). The 2D TOCSY spectra showed more visibility of cross-peaks using the suppression method, and the projected 1D 1H spectra demonstrated significant suppression of methyl and methylene groups with residues values of 0.03, and 0.22 respectively, resulting in a substantial increase by a factor of 1.77 in the visibility levels of the peaks of other regions (Fig. S10†). For the olive oil sample, the suppression of the methyl and methylene group signals with residues values of 0.03, and 0.20 respectively, enhanced the sensitivity of the peaks of the hydroxy, ester, aliphatic, and aromatic unsaturated regions by a factor of 3.60. The 2D TOCSY spectra showed more visibility of the cross-peaks when using the suppression method, and the projected 1D 1H spectra demonstrated significant suppression of the methyl and methylene groups with residue values of 0.10, and 0.18 respectively, resulting in a substantial increase in the peak visibility levels by a factor of 5.20 in other region (Fig. S13†).
The above results, which are summarized in Table S2,† show that the suppression method in 1H, and TOCSY leads to significant suppression of methyl and methylene regions and enhancement of sensitivity of signals and visibility of cross-peaks of rest of the regions across various samples in 1D and 2D spectra that contributes to a more accurate structural identification of compounds. This enhancement of visibility of the cross-peaks and the homogeneity of the spectrum may play an important role in increasing the reliability of NMR for qualitative analyses in lipidomics. However, the 1D 1H NMR with suppression method was limited with regards to the absolute quantification of lipids. The enhanced intensity of the peaks makes it possible to use 1H NMR to trace minor impurities in different types of plant oils, dairy products, and food samples.103–105 In the case of 2D-SMLS-TOCSY, depends on concentration or ration of number of suppression region resulted proportionally visibility of low intense cross-peaks. The comparison between the 2D-SMLS-TOCSY and the 2D-SUN-TOCSY methods in cheese and butter samples reveals several noteworthy aspects regarding their performance and effectiveness. Upon examining the 2D spectra of both methods at identical contour levels, it is evident that the appearance of cross-peaks is generally similar, encompassing both dimensions and showing comparable suppression levels for methyl and methylene groups. However, a critical drawback of the SUN-TOCSY method becomes apparent in its handling of the water signal at 1.30 ppm. The suppression of the water signal is less effective in SUN-TOCSY, leading to a more intense axial peak of zero-order coherence along the F1 dimension compared to the SMLS-TOCSY. This inefficiency in water signal suppression is a significant limitation of the SUN-TOCSY method, as it can interfere with the clarity and accuracy of the spectral data.
While the reliability of NMR data is higher than MS data, NMR spectroscopy and its handling come with greater financial cost. Moreover, the cost increases exponentially with magnetic strength. Benchtop 1D 1H NMR uses lower strength magnets but suffers from poor sensitivity. This sensitivity problem may be solved by applying this suppression method.106
Furthermore, NMR is more complicated and time-consuming for lipidomics compared to MS, because the NMR data are processed manually to predict the structure of the lipids due to the few online databases available. For example, the COLMAR web server can be used to analyze multiple TOCSY spectra for structural identification,107 but in the case of lipids, the 2D TOCSY spectra suffer from inhomogeneities in the peak intensities, with methyl and methylene peaks showing high-intensity peaks that reduce the visibility of other peaks. To overcome this obstacle, suppression method in 2D TOCSY NMR would create more homogeneous spectra. Thus, adding the suppression method to 2D TOCSY to apply on the COLMAR web server would makes it possible to more easily trace cross-peaks and a more accurate structural identification of the molecular components of lipids.
Conclusion
The present study shows that the application of suppression methods in 1D 1H and 2D TOCSY NMR spectroscopy improves the visibility and sensitivity of peak intensity across various samples. Having tested this method on multiple types of samples (dairy, olive oil, coconut, oil, etc.), we show that many molecular groups normally not visible in NMR spectra of lipids can be seen in the hydroxy, ester, aliphatic, and aromatic unsaturated regions. This is because the suppression method specifically targets signals for methyl and methylene groups without affecting the signals of other groups. Thus, this approach will provide a more detailed structure of molecules relevant to lipidomics.
Materials and methods
Sample preparations
Preparation of cheese sample. Soft cheese was purchased from a local supermarket (Thuwal, Saudi Arabia). 50 mg of cheese was dissolved in 700 μL of CDCl3 solvent. The suspended solution was vortexed for 5 minutes at 3000 rpm. Thereafter, the solution was centrifuged for 10 minutes at 15000 g and 4 °C. The supernatant was filtered in a microtip with cotton to get a clear transparent solution of 550 μL in a 5 mm NMR tube for NMR analysis.
Preparation of butter sample. Unsalted butter was purchased from a local supermarket (Thuwal, Saudi Arabia). 20 mg of butter was dissolved in 700 μL of CDCl3 solvent. The suspended solution was vortexed for 5 minutes at 3000 rpm. The solution was then centrifuged for 10 minutes at 15000 g and 4 °C. The supernatant was filtered in a microtip with cotton to get a clear transparent solution of 550 μL in a 5 mm NMR tube for NMR analysis.
Preparation of stock solution of lipids. A stock solution of five lipids was prepared in 500 μL of CDCl3 solvent with known concentrations of cholesterol, α-linolenate (1.2 mM), palmitic acid (43.7 mM), α-lipoic acid (42.6 mM), dodecanoic acid (53.9 mM), and decanoic acid (60.4 mM).
Preparation of coconut oil sample. Virgin coconut oil was purchased from a local supermarket (Thuwal, Saudi Arabia). 10 mg of coconut oil was dissolved in 500 μL of CDCl3 solvent in a 5 mm NMR tube and vortexed for 1 minute at 3000 rpm for the NMR analysis.
Preparation of olive oil sample. Extra virgin olive oil was purchased from a local supermarket (Thuwal, Saudi Arabia). 20 μL of olive oil was dissolved in 500 μL of CDCl3 solvent in a 5 mm NMR tube and vortexed for 1 minute at 3000 rpm for the NMR analysis.
NMR analysis
A Bruker 800 MHz AVANACE NEO NMR spectrometer equipped with a Bruker TCI (2H/13C/15N) cryogenic probe (BrukerBioSpin, Rheinstetten, Germany) was used to record all NMR spectra at 298 K, and the processing of spectra was conducted on a Topspin 4.1.4. 1D and 2D NMR spectra were reproduced using MS PowerPoint and Adobe Illustrator 2022. Acquisition and processing parameters used in the 1D and 2D NMR experiments are shown in Table S1.†
Data availability
All data are contained within the manuscript and ESI.†
Author contributions
U. S. conceptualization, preparation of images, writing – original draft; A. H. E., and M. J. assistance in conceptualization, review, and editing. M. J. supervision, funding acquisition, and resources.
Conflicts of interest
The authors declare that they have no conflicts of interest with the contents of this article.
Acknowledgements
KAUST and The Smart Health Initiative (SHI) are thanked by Mariusz Jaremko for grants from the Baseline (BAS/1/1085-01-01) program for the period of 2021 to 2023. The authors would like to thank King Abdullah University of Science and Technology (KAUST) for providing funding and technical assistance.
References
- P. L. Yèagle, FASEB J., 1989, 3, 1833–1842 CrossRef.
- J. Rossy, Y. Ma and K. Gaus, Curr. Opin. Chem. Biol., 2014, 20, 54–59 CrossRef PubMed.
- A. Claude, in Advances in Protein Chemistry, Elsevier, 1949, vol. 5, pp. 423–440 Search PubMed.
- R. C. Melo, H. D'Avila, H.-C. Wan, P. T. Bozza, A. M. Dvorak and P. F. Weller, J. Histochem. Cytochem., 2011, 59, 540–556 CrossRef.
- C. Sztalryd and A. R. Kimmel, Biochimie, 2014, 96, 96–101 CrossRef PubMed.
- V. Atanasov, P. P. Atanasova, I. K. Vockenroth, N. Knorr and I. Köper, Bioconjugate Chem., 2006, 17, 631–637 CrossRef CAS PubMed.
- W. G. Blackard, E. W. Hull and A. Lopez-S, J. Clin. Invest., 1971, 50, 1439–1443 CrossRef CAS PubMed.
- G. Hussain, H. Anwar, A. Rasul, A. Imran, M. Qasim, S. Zafar, M. Imran, S. K. S. Kamran, N. Aziz and A. Razzaq, Crit. Rev. Food Sci. Nutr., 2020, 60, 351–374 CrossRef CAS.
- C. Lonez, M. Vandenbranden and J.-M. Ruysschaert, Prog. Lipid Res., 2008, 47, 340–347 CrossRef CAS PubMed.
- J. Lin, J. Szymanski, P. C. Searson and K. Hristova, Langmuir, 2010, 26, 3544–3548 CrossRef CAS PubMed.
- M. Hamosh, Neonatology, 1998, 74, 163–176 CrossRef PubMed.
- A. d. P. Rogerio, C. A. Sorgi, R. Sadikot and T. Carlo, BioMed Res. Int., 2015, 2015, 1–2 Search PubMed.
- K. N. Theken and G. A. FitzGerald, Science, 2021, 371, 237–238 CrossRef.
- V. Chiurchiu and M. Maccarrone, Curr. Opin. Pharmacol., 2016, 29, 54–62 CrossRef PubMed.
- C. A. Drevon, Free Radical Res. Commun., 1991, 14, 229–246 CrossRef CAS.
- R. M. Adibhatla and J. F. Hatcher, Future Lipidol., 2007, 2, 403–422 CrossRef CAS.
- H. C. Stevens and J. W. Nichols, J. Biol. Chem., 2007, 282, 17563–17567 CrossRef CAS PubMed.
- A. Kumar, D. Baycin-Hizal, Y. Zhang, M. A. Bowen and M. J. Betenbaugh, Curr. Opin. Biotechnol., 2015, 36, 215–221 CrossRef CAS.
- G. Tan, Z. Lou, W. Liao, X. Dong, Z. Zhu, W. Li and Y. Chai, Mol. BioSyst., 2012, 8, 548–556 RSC.
- Z. Wang, E. Klipfell, B. J. Bennett, R. Koeth, B. S. Levison, B. DuGar, A. E. Feldstein, E. B. Britt, X. Fu and Y.-M. Chung, Nature, 2011, 472, 57–63 CrossRef PubMed.
- S. Zhong, L. Li, X. Shen, Q. Li, W. Xu, X. Wang, Y. Tao and H. Yin, Free Radicals Biol. Med., 2019, 144, 266–278 CrossRef PubMed.
- W. L. F. Lim, I. J. Martins and R. N. Martins, J. Genet. Genomics, 2014, 41, 261–274 CrossRef PubMed.
- X. Han, D. M. Holtzman and D. W. McKeel Jr, J. Neurochem., 2001, 77, 1168–1180 CrossRef CAS.
- V. C. Tam, O. Quehenberger, C. M. Oshansky, R. Suen, A. M. Armando, P. M. Treuting, P. G. Thomas, E. A. Dennis and A. Aderem, Cell, 2013, 154, 213–227 CrossRef CAS.
- H. Jiang, L. Nie, Y. Li and J. Xie, J. Sep. Sci., 2012, 35, 483–489 CrossRef CAS PubMed.
- C. Hu, H. Kong, F. Qu, Y. Li, Z. Yu, P. Gao, S. Peng and G. Xu, Mol. BioSyst., 2011, 7, 3271–3279 RSC.
- E. P. Rhee, S. Cheng, M. G. Larson, G. A. Walford, G. D. Lewis, E. McCabe, E. Yang, L. Farrell, C. S. Fox and C. J. O'Donnell, J. Clin. Invest., 2011, 121, 1402–1411 CrossRef CAS.
- P. J. Meikle, G. Wong, C. K. Barlow and B. A. Kingwell, Pharmacol. Therapeut., 2014, 143, 12–23 CrossRef CAS.
- W. Chamulitrat, G. Liebisch, W. Xu, H. Gan-Schreier, A. Pathil, G. Schmitz and W. Stremmel, Mol. Pharmacol., 2013, 84, 696–709 CrossRef PubMed.
- E. L. Donovan, S. M. Pettine, M. S. Hickey, K. L. Hamilton and B. F. Miller, Diabetol. Metab. Syndrome, 2013, 5, 1–13 CrossRef.
- L. Yetukuri, M. Katajamaa, G. Medina-Gomez, T. Seppänen-Laakso, A. Vidal-Puig and M. Orešič, BMC Syst. Biol., 2007, 1, 1–15 CrossRef PubMed.
- C. M. Aguilera, M. Gil-Campos, R. Canete and A. Gil, Clin. Sci., 2008, 114, 183–193 CrossRef PubMed.
- N. I. Krinsky, Annu. Rev. Nutr., 1993, 13, 561–587 CrossRef CAS PubMed.
- F. Spener, M. Lagarde, A. Géloên and M. Record, Eur. J. Lipid Sci. Technol., 2003, 105, 481–482 CrossRef.
- C. Mulder, L.-O. Wahlund, T. Teerlink, M. Blomberg, R. Veerhuis, G. Van Kamp, P. Scheltens and P. Scheffer, J. Neural. Transm., 2003, 110, 949–955 CrossRef CAS PubMed.
- G. van Meer, B. R. Leeflang, G. Liebisch, G. Schmitz and F. M. Goni, Methods Enzymol., 2007, 432, 213–232 CAS.
- J. A. Hamilton, Prog. Lipid Res., 2004, 43, 177–199 CrossRef CAS PubMed.
- J. Simard, P. Zunszain, C.-E. Ha, J. Yang, N. Bhagavan, I. Petitpas, S. Curry and J. Hamilton, Proc. Natl. Acad. Sci. U. S. A., 2005, 102, 17958–17963 CrossRef PubMed.
- B. S. Barbosa, L. G. Martins, T. B. Costa, G. Cruz and L. Tasic, Investigations of Early Nutrition Effects on Long-Term Health: Methods and Applications, 2018, pp. 365–379 Search PubMed.
- M. Li, L. Yang, Y. Bai and H. Liu, Anal. Chem., 2014, 86, 161–175 CrossRef PubMed.
- B. Bisht, V. Kumar, P. Gururani, M. S. Tomar, M. Nanda, M. S. Vlaskin, S. Kumar and A. Kurbatova, Arch. Biochem. Biophys., 2021, 710, 108987 CrossRef.
- R. Al-Nemi, A. A. Makki, K. Sawalha, D. Hajjar and M. Jaremko, Metabolites, 2022, 12, 451 CrossRef PubMed.
- U. Singh, S. Alsuhaymi, R. Al-Nemi, A.-H. Emwas and M. Jaremko, ACS Omega, 2023, 8, 23651–23663 CrossRef CAS PubMed.
- S. Alsuhaymi, U. Singh, I. Al-Younis, N. M. Kharbatia, A. Haneef, K. Chandra, M. Dhahri, M. A. Assiri, A.-H. Emwas and M. Jaremko, Natural Products and Bioprospecting, 2023, vol. 13, p. 44 Search PubMed.
- A.-H. Emwas, R. Roy, R. T. McKay, L. Tenori, E. Saccenti, G. N. Gowda, D. Raftery, F. Alahmari, L. Jaremko and M. Jaremko, Metabolites, 2019, 9, 123 CrossRef CAS.
- K. Chandra, S. Al-Harthi, F. Almulhim, A.-H. Emwas, Ł. Jaremko and M. Jaremko, Mol. Omics, 2021, 17, 719–724 RSC.
- K. Chandra, S. Al-Harthi, S. Sukumaran, F. Almulhim, A.-H. Emwas, H. S. Atreya, Ł. Jaremko and M. Jaremko, RSC Adv., 2021, 11, 8694–8700 RSC.
- A.-H. M. Emwas, R. M. Salek, J. L. Griffin and J. Merzaban, Metabolomics, 2013, 9, 1048–1072 CrossRef.
- A.-H. Emwas, K. Szczepski, I. Al-Younis, J. I. Lachowicz and M. Jaremko, Front. Pharmacol, 2022, 13, 805782 CrossRef.
- A.-H. M. Emwas, Metabonomics: Methods and Protocols, 2015, pp. 161–193 Search PubMed.
- J. L. Markley, R. Brüschweiler, A. S. Edison, H. R. Eghbalnia, R. Powers, D. Raftery and D. S. Wishart, Curr. Opin. Biotechnol., 2017, 43, 34–40 CrossRef.
- C. G. Tsiafoulis, C. Papaemmanouil, D. Alivertis, O. Tzamaloukas, D. Miltiadou, S. Balayssac, M. Malet-Martino and I. P. Gerothanassis, Molecules, 2019, 24, 1067 CrossRef PubMed.
- K. J. Boudonck, M. W. Mitchell, J. Wulff and J. A. Ryals, Metabolomics, 2009, 5, 375–386 CrossRef CAS.
- S. E. Hancock, B. L. Poad, A. Batarseh, S. K. Abbott and T. W. Mitchell, Anal. Biochem., 2017, 524, 45–55 CrossRef CAS PubMed.
- A.-H. M. Emwas, Z. A. Al-Talla, Y. Yang and N. M. Kharbatia, Metabonomics: Methods and Protocols, 2015, pp. 91–112 Search PubMed.
- O. Fiehn, Curr. Protoc. Mol. Biol., 2016, 114, 30 Search PubMed.
- S. P. Putri, M. M. M. Ikram, A. Sato, H. A. Dahlan, D. Rahmawati, Y. Ohto and E. Fukusaki, J. Biosci. Bioeng., 2022, 133, 425–435 CrossRef CAS.
- A.-H. M. Emwas, Z. A. Al-Talla and N. M. Kharbatia, Metabonomics: Methods and Protocols, 2015, pp. 75–90 Search PubMed.
- I. Medina, S. Aubourg, J. Gallardo and R. Perez-Martin, Int. J. Food Sci. Technol., 1992, 27, 597–601 CrossRef CAS.
- M. A. de la Fuente, P. Luna and M. Juárez, Trac. Trends Anal. Chem., 2006, 25, 917–926 CrossRef.
- T. Igarashia, M. Aursandc, Y. Hirataa, I. S. Gribbestadd, S. Wadae and M. Nonakaa, J. Am. Oil Chem. Soc., 2000, 77, 737–748 CrossRef.
- A. Trimigno, F. C. Marincola, N. Dellarosa, G. Picone and L. Laghi, Curr. Opin. Food Sci., 2015, 4, 99–104 CrossRef.
- L. Laghi, G. Picone and F. Capozzi, Trac. Trends Anal. Chem., 2014, 59, 93–102 CrossRef.
- N. Kumar and V. Jaitak, Crit. Rev. Anal. Chem., 2024, 1–25 CrossRef PubMed.
- M. Antonietta Brescia, V. Mazzilli, A. Sgaramella, S. Ghelli, F. Paolo Fanizzi and A. Sacco, J. Am. Oil Chem. Soc., 2004, 81, 431–436 CrossRef.
- R. Lamanna, A. Braca, E. Di Paolo and G. Imparato, Magn. Reson. Chem., 2011, 49, S22–S26 CAS.
- G. Andreotti, R. Lamanna, E. Trivellone and A. Motta, J. Am. Oil Chem. Soc., 2002, 79, 123–127 CrossRef CAS.
- S. Erich, S. Schill, E. Annweiler, H.-U. Waiblinger, T. Kuballa, D. W. Lachenmeier and Y. B. Monakhova, Food Chem., 2015, 188, 1–7 CrossRef CAS PubMed.
- Y. Peng, Z. Zhang, L. He, C. Li and M. Liu, Anal. Bioanal. Chem., 2024, 1–16 Search PubMed.
- D. Castejón, P. Fricke, M. I. Cambero and A. Herrera, Nutrients, 2016, 8, 93 CrossRef.
- K. Rachineni, P. Sharma, V. S. Shirke, K. Mishra and N. P. Awasthi, Food Control, 2023, 150, 109773 CrossRef.
- A. Mika, Z. Kaczynski, P. Stepnowski, M. Kaczor, M. Proczko-Stepaniak, L. Kaska and T. Sledzinski, Sci. Rep., 2017, 7, 15530 CrossRef PubMed.
- P. Lin, L. Dai, D. R. Crooks, L. M. Neckers, R. M. Higashi, T. W. Fan and A. N. Lane, Metabolites, 2021, 11, 202 CrossRef.
- C. G. Tsiafoulis, T. Skarlas, O. Tzamaloukas, D. Miltiadou and I. P. Gerothanassis, Anal. Chim. Acta, 2014, 821, 62–71 CrossRef.
- U. Singh, R. Al-Nemi, F. Alahmari, A.-H. Emwas and M. Jaremko, Metabolomics, 2023, 20, 7 CrossRef PubMed.
- R. Freeman, Chem. Rev., 1991, 91, 1397–1412 CrossRef.
- T.-L. Hwang and A. Shaka, J. Magn. Reson., Ser. A, 1995, 112, 275–279 CrossRef.
- S. Tiziani, A.-H. Emwas, A. Lodi, C. Ludwig, C. M. Bunce, M. R. Viant and U. L. Günther, Anal. Biochem., 2008, 377, 16–23 CrossRef PubMed.
- M. Kriat, J. Vion-Dury, S. Confort-Gouny, R. Favre, P. Viout, M. Sciaky, H. Sari and P. Cozzone, J. Lipid Res., 1993, 34, 1009–1019 CrossRef.
- S. H. Smallcombe, S. L. Patt and P. A. Keifer, J. Magn. Reson., Ser. A, 1995, 117, 295–303 CrossRef CAS.
- J. O'connell and P. Fox, Int. Dairy J., 2001, 11, 103–120 CrossRef.
- E. Frankel, Lipid Oxidation, 2005 Search PubMed.
- V. G. Kontogianni and I. P. Gerothanassis, Molecules, 2022, 27, 2139 CrossRef CAS PubMed.
- R. Ahmed, M. G. Siskos, H. Siddiqui and I. P. Gerothanassis, Magn. Reson. Chem., 2022, 60, 970–984 CrossRef CAS.
- C. Siciliano, J. Phys.: Conf. Ser., 2021, 1960, 012006 CrossRef CAS.
- E. Alexandri, R. Ahmed, H. Siddiqui, M. I. Choudhary, C. G. Tsiafoulis and I. P. Gerothanassis, Molecules, 2017, 22, 1663 CrossRef PubMed.
- P. P. Lankhorst and A.-N. Chang, Modern Magnetic Resonance, 2018, pp. 1743–1764 Search PubMed.
- U. Singh, S. Bhattacharya and B. Baishya, J. Magn. Reson., 2020, 311, 106684 CrossRef CAS PubMed.
- E. Alexandersson, C. Sandström, L. C. Lundqvist and G. Nestor, RSC Adv., 2020, 10, 32511–32515 RSC.
- M. Sugiura, N. Takao and H. Fujiwara, Magn. Reson. Chem., 1988, 26, 1051–1057 CrossRef CAS.
- S. Vaish, A. Singh, A. Singh and N. Mehrotra, 2005.
- S. Khoury, C. Canlet, M. Z. Lacroix, O. Berdeaux, J. Jouhet and J. Bertrand-Michel, Biomolecules, 2018, 8, 174 CrossRef PubMed.
- A. Amiel, M. Tremblay-Franco, R. Gautier, S. Ducheix, A. Montagner, A. Polizzi, L. Debrauwer, H. Guillou, J. Bertrand-Michel and C. Canlet, Metabolites, 2019, 10, 9 CrossRef PubMed.
- R. Addepalli and R. Mullangi, ADMET and DMPK, 2021, 9, 1–22 Search PubMed.
- H. Fernando, K. K. Bhopale, S. Kondraganti, B. S. Kaphalia and G. S. Ansari, Toxicol. Appl. Pharmacol., 2011, 255, 127–137 CrossRef CAS PubMed.
- S. D. Melvin, C. M. Lanctôt, N. J. Doriean, W. W. Bennett and A. R. Carroll, Sci. Total Environ., 2019, 654, 284–291 CrossRef PubMed.
- L. Ouldamer, L. Nadal-Desbarats, S. Chevalier, G. Body, C. Goupille and P. Bougnoux, J. Proteome Res., 2016, 15, 868–878 CrossRef.
- T. Serés-Noriega, E. Ortega, V. Perea, M. Giménez, L. Boswell, K. Mariaca, C. Font, A. Mesa, C. Viñals and J. Blanco, Diabetes Ther., 2023, 14, 553–567 CrossRef.
- M. Gil, S. Samino, R. Barrilero and X. Correig, NMR-Based Metabolomics: Methods and Protocols, 2019, pp. 35–47 Search PubMed.
- D. Tanasi, E. Greco, V. Di Tullio, D. Capitani, D. Gullì and E. Ciliberto, Microchem. J., 2017, 135, 140–147 CrossRef.
- D. Tanasi, E. Greco, R. E. Noor, S. Feola, V. Kumar, A. Crispino and I. Gelis, Anal. Methods, 2018, 10, 2756–2763 RSC.
- R. M. Borges, J. V. M. Resende, A. P. Pinto and B. C. Garrido, Phytochem. Anal., 2022, 33, 533–542 CrossRef CAS PubMed.
- M. Oostendorp, U. F. Engelke, M. A. Willemsen and R. A. Wevers, Clin. Chem., 2006, 52, 1395–1405 CrossRef CAS PubMed.
- L. Mannina and A. P. Sobolev, Magn. Reson. Chem., 2011, 49, S3–S11 CrossRef CAS PubMed.
- L. Haddad, J. Francis, T. Rizk, S. Akoka, G. S. Remaud and J. Bejjani, Food Chem., 2022, 383, 132434 CrossRef CAS PubMed.
- W. Jakes, A. Gerdova, M. Defernez, A. Watson, C. McCallum, E. Limer, I. Colquhoun, D. Williamson and E. Kemsley, Food Chem., 2015, 175, 1–9 CrossRef CAS PubMed.
- F. Zhang, S. L. Robinette, L. Bruschweiler-Li and R. Brüschweiler, Magn. Reson. Chem., 2009, 47, S118–S122 Search PubMed.
Footnote |
† Electronic supplementary information (ESI) available: Experimental acquisition and processing parameters table, 1D and 2D NMR spectra for comparison of cheese, butter, a stock solution of lipids, coconut oil, and olive oil samples as well as pulse programs of these pulse sequences. See DOI: https://doi.org/10.1039/d4ra03019b |
|
This journal is © The Royal Society of Chemistry 2024 |
Click here to see how this site uses Cookies. View our privacy policy here.