DOI:
10.1039/D4RA07476A
(Review Article)
RSC Adv., 2024,
14, 39472-39497
MOF-based spectrophotometric sensors for cholesterol detection: current trends and challenges
Received
18th October 2024
, Accepted 3rd December 2024
First published on 13th December 2024
Abstract
Cholesterol detection is essential for early diagnosis and monitoring of cholesterol-related diseases, such as atherosclerosis, hypercholesterolemia, and liver diseases. A variety of nanomaterials have been designed and synthesized for cholesterol detection via electrochemical and spectrophotometric techniques. Metal organic frameworks (MOFs) have emerged as promising detector materials for cholesterol sensing. Recent research explores MOFs as spectrophotometric cholesterol sensors with remarkable performance in terms of limit of detection and selectivity. Given the growing interest in cholesterol sensing, and limited reviews on recent advances in the field, this review critically examines recent advances in MOF-based spectrophotometric cholesterol sensors, outlining the different mechanistic roles of MOFs in cholesterol detection. The review also highlights significant improvements, current challenges, and potential applications of MOF-based sensors for cholesterol detection in point-of-care devices and medical diagnostics.
1. Introduction
Cholesterol plays a vital role in human health. It is involved in several roles including hormone synthesis, cell membrane integrity and fluidity, vitamin D production, bone health, among others.1 However, excess cholesterol levels (hypercholesterolemia) can cause atherosclerosis and increase the risk of cardiovascular diseases, strokes and obesity.2–5 In fact, hypercholesterolemia is considered the third most common cardiovascular risk factor worldwide while also being the least monitored factor.5,6 In addition to atherosclerosis, high cholesterol levels can lead to toxic accumulation in the liver, pancreatic islets, kidneys, and other organs, subsequently increasing the incidence of nonalcoholic fatty liver disease, nonalcoholic steatohepatitis, islet B-cell dysfunction, renal dysfunctions, testosterone deficiencies, osteoporosis and osteoarthritis, among others.7 Hence, continuous monitoring of cholesterol levels is crucial in patients with atherosclerosis and other cholesterol-related diseases, for early detection and maintenance of diseases to maintain patient health.8
Cholesterol exists in blood serum in two forms: free cholesterol and cholesteryl esters, the latter being the most common.9,10 Due to cholesterol's insolubility in aqueous solutions, they are transported in the bloodstream via lipoproteins.10 Lipoproteins consist of a hydrophobic core carrying triglycerides and cholesteryl esters and a hydrophilic membrane made of phospholipids, free cholesterol, and apolipoproteins.10 There are many types of lipoproteins, including chylomicrons, high-density lipoproteins (HDLs), low-density lipoproteins (LDLs) and very low-density lipoprotein (VLDLs), with LDLs carrying the majority of cholesterol in the blood.9,10 LDLs carry cholesterol from the liver to cells for storage, while HDLs transport cholesterol from cells to the liver, where it is broken down or excreted, as illustrated in Fig. 1.1,8–10 Furthermore, LDLs are commonly known as “bad cholesterol,” as elevated levels are correlated with increased arterial wall build up, increasing risks of arthrosclerosis.7,10,11 Meanwhile, HDLs are commonly coined “good cholesterol,” because of its anti-inflammatory and anti-arteriosclerotic action by removing free cholesterol from cells, a process known as reverse cholesterol transport.11 Due to the complexity of cholesterol availability in the bloodstream, various cholesterol detection methods have been developed.
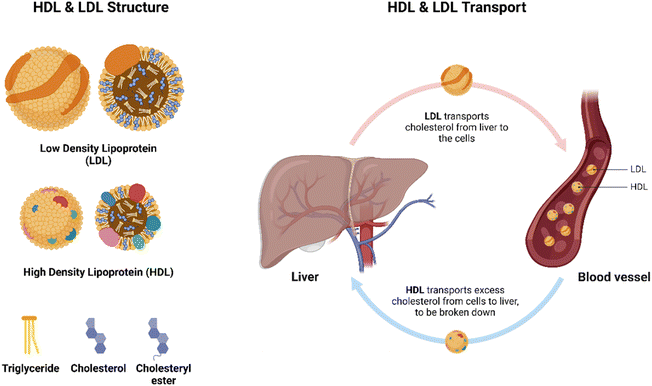 |
| Fig. 1 Structure and metabolic pathway of HDL and LDL. | |
Cholesterol detection has evolved greatly over the past years. Early methods of detection were simple, quantifying total cholesterol.12 Later techniques evolved to be more specific, quantifying HDLs, LDLs, triglycerides, free cholesterol, and total cholesterol.13–15 The current, standardized method used in hospitals and clinics is the cholesterol oxidase/peroxidase/4-aminophenazone (CHOD-POD-PAP) method, a colorimetric, enzyme-based method.14 Although widely effective, the assay requires the use of several enzymes, which is have low stability, limiting pH, temperature, and operation and storage conditions thus also introducing extra costs.13,16–18 Recent studies have shown the high potential of nanomaterials for the development of cholesterol sensors, for rapid and accurate cholesterol quantification.19 Such nanomaterials include metal nanoparticles, metal oxide nanoparticles, carbonaceous materials, and other composite materials.8,19 These have facilitated cholesterol biosensing via electrochemical, colorimetric and photoluminescent techniques.8,19–22 More recently, metal–organic-frameworks (MOFs) have emerged as superior nanomaterials for cholesterol biosensing, especially for spectrophotometric-based detection (including photoluminescent and colorimetric). This is attributed to the remarkable tunability of the MOF's structures and their large surface areas.18,23–31 Furthermore, MOFs exhibit enhanced selectivity, and stability in the immobilization of enzymes.16,32,33 Hence, MOFs have great potential for future applications in point-of-care (PoC) cholesterol biosensors.
Research on cholesterol biosensors has grown in popularity over the past two decades. Especially after 2020, a spike in research articles with cholesterol sensing or detection in the title alone is observed, as illustrated in Fig. 2; this reflects the growing interest in cholesterol sensing Comprehensive reviews have explored electrochemical biosensors for cholesterol detection19–22 along with other cholesterol biosensors.8 MOFs as photoluminescent sensors33 and nanozymes16,32,34 have also been reviewed extensively. However, to the best of our knowledge, there is a gap in existing reviews as limited focus has been dedicated to reviewing MOFs as spectrophotometric sensors specifically for cholesterol. An increasing interest in MOF-based biosensors for spectrophotometric detection of cholesterol has been also noticed over recent years, growing from 2–4 to 11 publications per year, most of which are journal articles, as illustrated in Fig. 3. Due to this increased research activity, this paper aims to review and evaluate current research on spectrophotometric MOFs for cholesterol biosensing and address current challenges, propose potential solutions, and outline future research directions. This review exclusively concentrates on spectrophotometric MOF biosensors, which include colorimetric, fluorescent, and chemiluminescent techniques, as electrochemical biosensors have been extensively covered in previous reviews.19–22,35 Moreover, this review adds value to the literature by categorizing and analyzing all spectrophotometric, MOF-based cholesterol sensing mechanisms, providing a comprehensive evaluation that has not been previously undertaken.
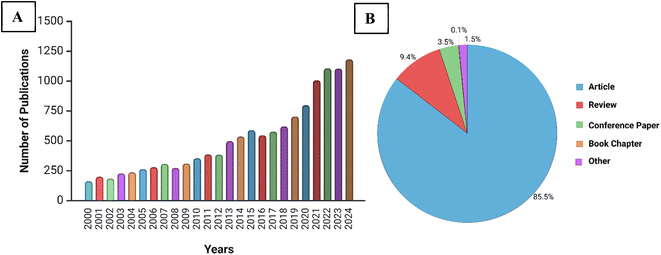 |
| Fig. 2 (A) Growth in and (B) type of Scopus publications from 2000–2024 exploring general cholesterol detection and sensing. Data obtained from Scopus.com, using “cholesterol” and “detection” or “sensor” as the search terms in the article title, keywords, or abstract. Retrieved on Nov 20, 2024. | |
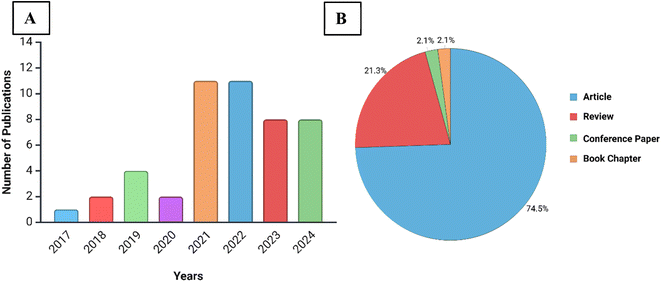 |
| Fig. 3 (A) Growth in and (B) type of Scopus publications from 2017–2024 exploring MOF-based cholesterol detection and sensing. Data obtained from https://www.scopus.com/, using “metal organic framework” and “cholesterol” and “detection” or “sensor” as the search terms in the article title, keywords, and/or abstract, retrieved on Nov 20, 2024. | |
The outline of this review is as follows: first, traditional and nanomaterial-based spectrophotometric cholesterol detection methods are reviewed (Section 2). Then, the basics of MOFs and their promising properties for applications in sensing are explored (Section 3). Next, a more detailed analysis of MOF-based spectrophotometric cholesterol sensors is conducted, by discussing the roles of MOF in sensing mechanisms and performance (Section 4). Potential integration of these MOFs into sensing platforms is then assessed (Section 5), and the paper concludes with current challenges associated with MOF stability, multi-biomarker detection, continuous, non-invasive sensing and environmental concerns and future directions (Section 5).
2. Cholesterol detection methods
Early methods of cholesterol detection utilized different reagents to enhance cholesterol's chromophore properties for quantification by measuring absorbance of the produced color, a colorimetric technique.12,36,37 Later studies utilized enzyme-based colorimetric assays for more accurate and rapid results, with methods dating back to 1972.13,38 Current standardized clinical methods for cholesterol detection also utilize enzymes for a colorimetric assay.13–15 However, with advances in research and nanotechnology over the past two decades, various new methods have emerged for cholesterol detection and quantification using different nanomaterials, including electrochemical,19,21 colorimetric,39–43 and photoluminescent techniques.1,44–47 The latter two methods fall under the general umbrella of spectrophotometric techniques. This section herein presents the traditional cholesterol detection method then explores colorimetric and photoluminescent methods for cholesterol detection.
2.1 Traditional, conventional methods
The conventional methods for cholesterol detection vary depending on the medium in which it is being detected. Cholesterol detection is conducted using two methods: clinical, colorimetric assays and reflectance photometric techniques, often adopted in hand-held devices; both of which are enzyme-based.13–15 Electrochemical methods were also introduced, but have since evolved into more recent, improved sensors.19,35 Meanwhile, cholesterol quantification in food industry and in research laboratories traditionally adopt chromatographic and spectrometric techniques, such as gas-chromatography-mass spectrometry (GCMS) and high-performance liquid chromatography (HPLC), among others.48 This paper herein focuses on medical applications of cholesterol detection, excluding chromatographic and spectrometric detection methods.
2.1.1 Traditional clinical detection. The standardized, traditional clinical cholesterol quantification technique uses an enzyme-based colorimetric bioassay, known as the cholesterol oxidase/peroxidase/4-aminophenazone (CHOD-POD-PAP) method.13–15 The analysis involves three main steps. First, blood serum is treated with cholesteryl esterase enzyme, to convert cholesteryl esters into cholesterol. Next, cholesterol oxidase (ChOx) enzyme is used to selectively react with cholesterol and produce cholest-4-ene and hydrogen peroxide (H2O2). Finally, 4-aminoantipyrine, a chemical reagent, is added to react with the produced hydrogen peroxide, in the presence of horseradish peroxidase (HRP) enzyme as a catalyst, to produce a colored quinoneimine dye. The intensity of the produced color is measured using a spectrophotometer and used to determine the concentration of cholesterol.13,14 An illustration of these reactions is shown in Fig. 4 below.
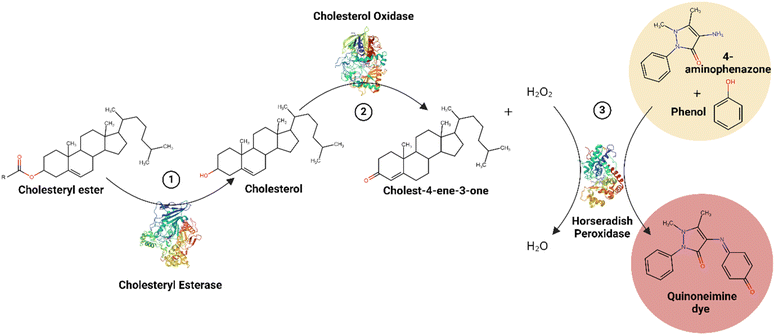 |
| Fig. 4 Traditional, clinical CHOD-POD-PAP method summary.49–51 | |
The CHOD-POD-PAP method is suitable for the detection of total cholesterols, as well as HDLs, LDLs, and triglycerides. The latter three techniques require pretreatment before analysis.14 This enzymatic, colorimetric bioassay is widely used as a reference and in clinicals, and is advantageous in its accuracy, selectivity, and the absence of corrosive chemicals.52 The method also has a wide linear range of detection, detecting up to 800 mg dL−1 and even 1000 mg dL−1 of cholesterol (equivalent to 20.7 mM and 25.9 mM, respectively), depending on the commercial kit used.14,15,53 Typical levels of cholesterol range around 200 mg dL; however, some kits can detect at lower concentrations of 10 mg dL−1, which is useful for diluted samples.15 Furthermore, detection is often quick, with incubation times ranging between 5 to10 minutes, and incubation temperatures varying between room temperature (20–25 °C) or physiological temperature (37 °C).14,15,53 Although overall advantageous, this traditional method of cholesterol detection is heavily dependent on enzymes, which require specific pH and temperature conditions for storage and operation, and are known for their low stability, short lifetime, and lack of reusability.13,16–18 Natural HRP is especially recognized for its low stability to heat and chemical changes, making the method costly and time-consuming.17,18 Hence, while advantageous, the CHOD-POD-PAP traditional cholesterol detection method can be significantly optimized.
2.1.2 Reflectance photometric methods. Reflectance photometry for cholesterol detection is among the earliest techniques introduced. Through an enzymatic assay similar to the CHOD-POD-PAP assay, hydrogen peroxide is produced and interacts with a dye to produce a color change. The color intensity is quantified by measuring the light reflectance off the colored strip.54,55 The amount of reflected light is correlated with the hydrogen peroxide concentration and can be subsequently used for cholesterol quantification.54,55 This technique dates back to 1980–1990's and was greatly effective during that time for cholesterol detection and other biomarkers, including glucose and hemoglobin.54–56 Furthermore, this method has been traditionally incorporated in handheld, commercial devices for cholesterol detection, such as Accutrend,57 STANDARD LipidoCare58 and CardioChek,59 and is advantageous in its rapid nature. However, the accuracy of such devices varies significantly, with many of which do not meet current standards.60 Accutrend Plus reported the highest sensitivity (92%) and specificity (89%).60 Nonetheless, the varying accuracy poses a challenging limitation, especially when used in commercial, PoC cholesterol detection devices.
2.2 Nanomaterial-based methods
To overcome the limitations of the conventional methods, recent studies have shifted to using different nanomaterials for cholesterol detection. These nanomaterials include metal and metal oxide nanoparticles, carbonaceous materials, including carbon nanotubes, carbon dots, and graphene, and composite materials including two or more types.8,19,61,62 Some use nanomaterials to immobilize HRP increasing its stability and prolonging its lifetime, reducing costs.44,63 For example, platinum nanoclusters,63 silicon nanoparticles,44 and poly(thionine)-modified glassy carbon electrodes64 all proved effective in immobilizing HRP for accurate and sensitive cholesterol detection. Others use nanoparticles with peroxidase-like activity, to replace HRP altogether, overcoming its stability challenges.65 Examples include nitrogen-doped carbon nanosheets with Fe–Ni entrapped inside,66 Cu–salt–Fe composites,67 copper-doped cerium dioxide nanosheets,68 all of which exhibited great sensitivity and selectivity to cholesterol. These sensors utilized spectrophotometric39–47,69–74 and electrochemical35 measurements for cholesterol quantification. Electrochemical-based cholesterol sensors determine cholesterol concentration by measuring changes in current due to a redox reaction, in potential between a reference and working electrode, or in impedance.19,35 Electrochemical sensing methods are advantageous due to their relatively lower cost, portability, and high sensitivity; comprehensive reviews on electrochemical-based cholesterol sensors can be found in ref. 19, 21 and 35. In addition, plasmonic-based, optical techniques of cholesterol detection have also emerged.75–78 For instance, bimetallic silver-shelled gold nanorods proved effective in detecting both triglycerides and cholesterol via their plasmonic properties, allowing for detection through surface enhanced Raman spectroscopy.76 Moreover, graphene oxide coupled with silver nanowires have enabled optical detection of cholesterol at low concentrations.75 Comprehensive reviews on plasmonic-based biosensors can be found in ref. 77 and 78.
More recently, spectrophotometric-based sensors have emerged as sensitive and accurate cholesterol sensors.39–47,69–74 Spectrophotometric detection methods include colorimetric, fluorescent, and chemiluminescent techniques, illustrated in Fig. 5. A variety of nanomaterials have been designed for spectrophotometric-based cholesterol sensing. These will be herein discussed. An overview of these nanomaterials, the cholesterol sensing operating principle, the limits of detection and linear ranges achieved are summarized in Table 1.
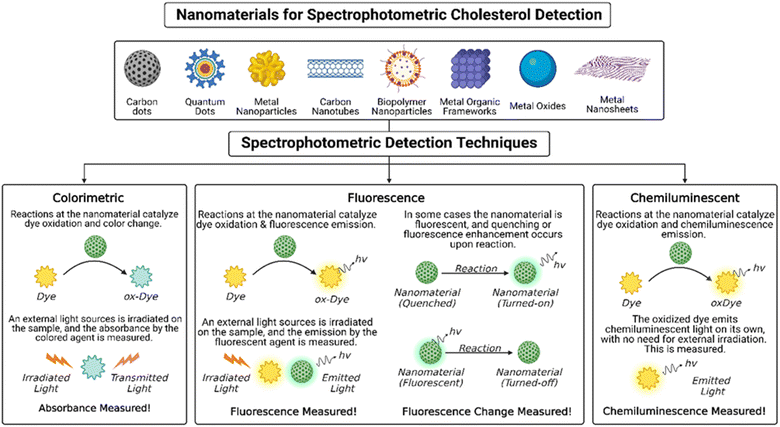 |
| Fig. 5 Overall mechanisms of nanomaterial-based spectrophotometric cholesterol sensors. | |
Table 1 Spectrophotometric-based cholesterol sensors, excluding MOFs
Nanoparticles used |
Detection method |
Cholesterol sensing operating principle |
Limit of detection (LOD) (μM) |
Linear range (μM) |
Ref. |
Gum kondagogu (a biopolymer) – reduced/stabilized palladium NPs (GK-Pd NPs) |
Colorimetric |
Sample is treated with ChOx, then GK-Pd NPs has intrinsic peroxidase properties, catalyzing hydrogen peroxide (H2O2) reduction and TMB dye oxidation, causing a color change |
3.7 |
5–100 |
39 |
ZnO NPs on carbon nanotube (CNT) surface |
Colorimetric |
After oxidation by free ChOx, peroxidase-like ZnO/CNT catalyzes H2O2 reduction and 2,2′-azino-bis(3-ethylbenzthiazoline-6-sulfonic acid) (ABTS) dye oxidation, producing a change in color |
0.0002 |
0.0005–0.5 |
40 |
Au@Ag core–shell nanoparticles (Au@Ag NPs) |
Colorimetric |
After reaction with free ChOx, produced H2O2 oxidizes Ag surrounding AuNPs to Ag+, leading to a visible color change |
0.15 |
0.3–300 |
70 |
Fe3O4 particles coated with gold nanoparticles (GoldMag) |
Colorimetric |
After treatment with ChOx, GoldMag acts as a peroxidase mimic, catalyzing H2O2 reduction and ABTS oxidation, resulting in color change |
7.8 |
259–19397 |
41 |
MXene-Ti3C2 nanosheets and CuS nanoparticles (MXene-Ti3C2/CuS) nanocomposites |
Colorimetric |
After treatment with ChOx, MXene-Ti3C2/CuS acts as a peroxidase mimic, catalyzing H2O2 reduction and TMB oxidation, resulting in color change |
1.9 |
10–100 |
42 |
Polypyrrole nanoparticles (PPy NPs) |
Colorimetric |
Cholesterol is oxidized by free ChOx and PPy NPs act as peroxidase mimic, catalyzing H2O2 reduction and TMB oxidation, resulting in color change |
3.5 |
10–100 |
43 |
Cholesterol oxidase immobilized gold nanoparticles (ChOx@GNP) |
Dual colorimetric and fluorescence |
Cholesterol is oxidized by immobilized ChOx and produced H2O2 quenches the GNP, leading to decreased fluorescence and a color change |
490 |
650–7760 |
45 |
Gold–carbon dot nanoconjugates (Au@CDs) |
Dual colorimetric and fluorescence |
Cholesterol directly interacts with Au@CDs, destabilizing surface and leading to particle agglomeration. This causes a change in color and fluorescence quenching. No ChOx or HRP needed |
2.5 |
1000–6250 |
73 |
Silicon NP (SiNP) |
Fluorescence |
Sample is pre-treated with ChOx then HRP in the presence of p-phenylenediamine (PPD), producing PPDox. Produce PPDox quenches SiNPs by the inner filter effect (IFE), and this quenching is correlated with cholesterol concentration |
0.018 |
0.025–10 |
44 |
Poly(vinylpyrrolidone)-protected gold nanoparticles (PVP-AuNPs) (peroxidase substitute) |
Fluorescence |
After ChOx treatment, produced H2O2 enlarges PVP-AuNPs, which then quenches bovine serum albumin-protected gold nanoclusters (BSA-AuNCs). Quenching is measured |
1.4 |
1–100 |
46 |
β-Cyclodextrin carbon dots (β-CDs) |
Fluorescence |
Cholesterol directly interacts with β-CD cavity, quenching its fluorescence. No ChOx or HRP needed |
0.204 |
0.5–40 |
71 |
Carbon dot/hemoglobin complex (CD/Hb) |
Fluorescence |
Cholesterol directly binds with Hb, disturbing CD quenching and restoring fluorescence. No ChOx or HRP needed |
56 |
0–800 |
72 |
Garlic-capped Ag NPs (G-AgNPs) |
Fluorescence |
Cholesterol directly interacts with G-AgNPs, via ionic or hydrogen bonds, causing fluorescence enhancement |
186 |
400–5170 |
74 |
ChOx-immobilized CdSe/ZnS quantum dots |
Fluorescent |
Cholesterol is oxidized by the immobilized ChOx, then produced H2O2 directly quenches CdSe/ZnS quantum dots |
10 |
10–9110 |
47 |
Cupric oxide NP (CuO NP) |
Chemiluminescence |
After ChOx treatment, cupric oxide NPs act as peroxidase mimic, catalyzing luminol conversion to excited state, which later relaxes. Chemiluminescence here is measured |
0.17 |
0.625–12.5 |
69 |
2.2.1 Colorimetric. Colorimetric-based sensors are optical sensors that operate by detecting a change in color upon a chemical reaction with the analyte. More specifically, this color change occurs within the visible light wavelength region (400–800 nm), which is often detectable by the naked eye, and can be quantified by detecting change in absorbance at a fixed wavelength.79 Colorimetric methods of detection are simple, less costly, rapid, and sensitive, with relatively low background interferences.40,43 The traditional, clinical cholesterol detection method, explained earlier in Section 2.1, employs colorimetric-based cholesterol detection. However, a significant limitation is the heavy dependence on enzymes, especially HRP, which is known for its instability.17,18Recent colorimetric sensors integrate nanomaterials with inherent peroxidase-like activity, to replace HRP enzyme for more efficient sensing and longer operation.39–43 For example, polypyrrole nanoparticles (PPy NPs), an organic, semiconducting NP, demonstrated good peroxidase-like activity, and allowed for complete replacement of HRP. In combination with the dye 3,3′,5,5′-tetramethylbenzidine (TMB) and ChOx, the PPy NPs facilitated cholesterol detection, with a limit of detection of 3.5 μM.43 Other works explored the use of nanocomposites, combining a minimum of two nanomaterials for improved peroxidase activity and cholesterol sensing.39–43 Examples include gold NP-coated Fe3O4 magnetic particles (GoldMag),41 MXene-Ti3C2 nanosheets combined with CuS NPs,42 and Pd-NPs stabilized with gum kondagogu biopolymer,39 all demonstrating inherent peroxidase activity and combined with a dye (TMB or 2,2′-azino-bis(3-ethylbenzthiazoline-6-sulfonic acid (ABTS))), for effective sensing of cholesterol in μM ranges. Lower detection limits were achievable in a ZnO/carbon nanotube (ZnO/CNT) nanocomposite, with a linear range of 0.0005–0.5 μM and an LOD as low as 0.0002 μM.40 Thus, nanomaterials with peroxidase-like activity worked as accurate and sensitive colorimetric cholesterol sensors when combined with a dye and ChOx.
However, the need for an external dye increases costs and materials required for the sensor design. Later studies include nanomaterials that demonstrated a color change upon exposure to hydrogen peroxide produced during cholesterol oxidation by ChOx, excluding the need for a peroxidase mimic. For instance, Au@Ag core–shell nanoparticles demonstrated a clear, color change when exposed to hydrogen peroxide after cholesterol oxidation.70 The produced hydrogen peroxide etched away the Ag shell, producing Ag+ and causing a gradual color change from orange to wine red.70 A wide linear range of cholesterol detection was achieved (0.30–300 μM) and the LOD was 0.15 μM, with good selectivity in the presence of other biomolecules.70 Hence, this unique, colorimetric sensing mechanism allowed for selective and sensitive cholesterol sensing while eliminating the need for HRP. Nonetheless, in all these nanomaterial-based colorimetric methods, ChOx enzyme is still needed as a pretreatment step for selective cholesterol sensing.
2.2.2 Fluorescent. Fluorescent analysis methods detect the emission of light by a fluorophore, or a fluorescent dye, after excitation caused by external light irradiation.80 The wavelength of the emitted light is often longer than the absorbed light, and the mechanism of sensing often involves observing fluorescence enhancement/recovery or quenching, depending on the nanomaterial used as the fluorophore.80 Nanomaterial-based fluorescent sensors have various advantages, including low costs, ultrasensitive detection to picomolar levels, enhanced selectivity, and allows for real-time, continuous monitoring.80–82 A variety of nanomaterials have been used for fluorescence-based detection of cholesterol.44–47,71–74 Some sensors use pure nanomaterials of one type, such as silicon NPs44 and β-cyclodextrin carbon dots.71 Other sensors use hybrid, nanomaterial composites combining for enhanced fluorescence detection of cholesterol, such as sensors using garlic capped AgNPs,74 CdSe/ZnS quantum dots,47 and others.Similar to colorimetric, nanomaterial-based sensors, fluorescent nanomaterial cholesterol sensors have been used in combination with ChOx and HRP44 and also as peroxidase-mimics, with the need for ChOx.45–47 For example, fluorescent silicon NPs are used in combination with ChOx and HRP enzyme treatment in the presence of p-phenylenediamine, which – upon oxidation – quenches the silicon NPs; this quenching is used to determine cholesterol concentrations.44 Meanwhile, poly(vinylpyrrolidone)-protected gold nanoparticles (PVP-AuNPs) proved to be efficient peroxidase-mimics, which replaces HRP and relies only on ChOx treatment. In the presence of oxidized cholesterol and hydrogen peroxide, PVP-AuNPs swell, causing quenching of bovine serum albumin-protected gold nanoclusters (BSA-AuNCs) and subsequent cholesterol detection.46
However, fluorescent nanomaterial-based cholesterol detectors are unique in their ability to design enzyme-free cholesterol sensors, for direct cholesterol detection without ChOx or HRP.71–74 In these sensors, cholesterol directly interacts with active sites in the nanomaterials, causing a change in fluorescence. For instance, carbon dot/hemoglobin complex demonstrated great selectivity to cholesterol, where fluorescence enhances upon cholesterol–hemoglobin binding; the sensor had a wide dynamic range (0–800 μM), with a low LOD of 56 μM.72 β-Cyclodextrin carbon dots71 and garlic-capped Ag NPs (G-AgNPs)74 also exhibited selective cholesterol fluorescent detection, with β-cyclodextrin carbon dots allowing for micro-molar range cholesterol detection and the G-AgNPs allowing for millimolar range cholesterol detection. These sensors are advantageous in their stability, due to the elimination of enzymes. Other nanomaterial sensors display dual colorimetric and fluorescent detection, such as ChOx immobilized gold nanoparticles45 and gold–carbon dot nanoconjugates,73 the latter being advantageous in its enzyme-free nature. All these fluorescent, nanomaterial-based sensors exhibited selective cholesterol detection and good detection limits.
2.2.3 Chemiluminescent. Chemiluminescence is the emission of light as a result of a chemical reaction; the light intensity is correlated with the reaction rate; thus, it can be used to determine the concentration of the limiting reactant. In sensing applications, luminol is often used as the light-emitting reagent,69 i.e. the reagent causing chemiluminescence, among other reagents. Chemiluminescence is advantageous in its rapid nature, relatively simple method, and high sensitivity.69 Different nanomaterials have been used to catalyze chemiluminescent reactions between produced hydrogen peroxide and a dye, for effective and rapid cholesterol sensing.69,83 For example, CuO NPs exhibited excellent peroxidase-like activity, catalzying the reaction between hydrogen peroxide, produced from ChOx cholesterol oxidation, and luminol, a chemiluminescent dye. The enhancement in chemiluminescence allowed for micromolar detection of cholesterol, while also replacing HRP for a more stable, long-lasting system.69 Hence, chemiluminescent nanomaterials for cholesterol detection have proven effective sensing materials. However, unlike fluorescent-based sensors, current chemiluminescent-based sensors still require the use of ChOx enzyme. Future studies can investigate the complete elimination of enzymes in chemiluminescent, nanomaterial-based cholesterol sensing.
3. MOFs as promising sensing materials
Compared to other nanomaterials, MOFs are gaining rapid attention and superiority in sensing applications. This is attributed to its highly tunable structure, surface functionalization, inherent catalytic and fluorescent properties, among other advantages.18,23–31 Understanding the basic composition, structure, and type of MOFs is crucial to better design MOF-based sensors and optimize performance. This section herein explores the basic features of MOFs, their promising advantages for sensing applications.
3.1 MOF basics
Metal organic frameworks are highly crystalline materials, with unique compositions and structures. This section herein briefly explores the basic features of MOFs, including their composition, structures, synthesis procedures, and characterization techniques.
3.1.1 Composition. MOFs are made up of two main components: metal ions or clusters and organic linkers, connected via coordination bonds.84–87 A variety of metal ions can be used, including zinc (Zn),88,89 zirconium (Zr),90–93 titanium (Ti),94,95 chromium (Cr),96,97 iron (Fe), and aluminum (Al),98 with transition metal ions of high valence and empty d-orbitals being the majority.84,99 These metal ions or clusters act as nodes, forming multiple coordination bonds to join organic linkers and form the overall MOF structure. Like metal clusters, a variety of organic linkers can be used, producing a multitude of MOFs with different structures. These organic linkers, also coined ligands, often contain O- or N-donor functional groups, to donate electrons and form the coordination bond.87 Examples of common organic linkers include carboxylates, benzenes, crown ethers, sulfonates, imidazoles, and pyridyl compounds, among others.87 Together, the different combinations of metal clusters and organic linkers produce a multitude of MOFs with different properties and structures. Within these structures many spaces exist, which can be occupied by solvent molecules, guest molecules, or others. Fig. 6 below illustrates the general composition of MOFs from its building blocks.
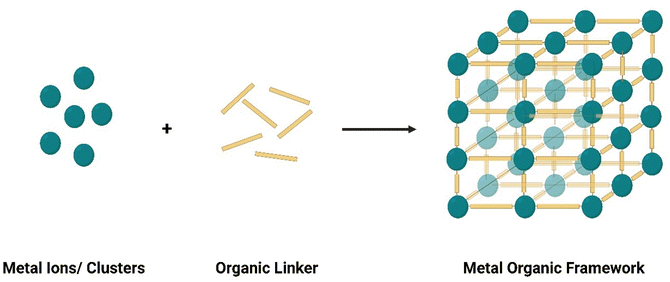 |
| Fig. 6 Generic MOF composition from metal ions and organic linkers. | |
3.1.2 Structures. Different MOF structures or geometries have been established. This variance is dependent on the metal clusters and organic linkers used. More specifically, the coordination number (or ligancy) of the metal clusters, the geometry of the metal ion clusters, the functional group of the organic linker, and the rigidity or flexibility of the organic linker all contribute to the overall MOF structure.87,99 For example, metal clusters with only two vacancies often produce linear, one-dimensional (1D) structures, to form 1D MOF nanowires, nanotubes, and other shapes depending on the synthetic protocol. Examples of 1D, linear MOFs include Co-MOF-74,100 ZIF-8 when synthesized on polycarbonate track-etched (PCTE) template,101 and Mg-MOF-74 when synthesized at a controlled pH,102 among many others reviewed in ref. 103 and 104. Meanwhile, metal clusters containing three and four vacant sites form more elaborate 3D structures, such as MIL-125(Ti), MIL-101(Cr), and MIL-53(Al), among others.99,105,106 Some of the geometries available at the metal cluster–ligand bonding site include linear, trigonal planar, T-shaped, tetrahedral, square planar, pentagonal bipyramidal, octahedral, trigonal prismatic, trigonal bipyramidal, and square pyramidal.86 These geometries contribute to the overall MOF topology, which includes cubic, spherical, spindle, and rod structures.107–109 The diversity and tunability of MOF structures and shapes is greatly advantageous, allowing for a wide range of applications.
3.1.3 Synthesis & characterization. A multitude of synthetic techniques have been developed for the successful preparation of MOFs. Early methods were rather simple, whereby metal ions and organic linkers were mixed until porous, crystalline MOFs are developed. However, this method requires long synthesis times and can be costly.99 With advancements in technology and research, more complex and effective methods emerged. These include microwave-assisted, electrochemical, solvothermal, mechanochemical, sonochemical, diffusion, slow evaporation, and microemulsion methods.87,99,107 Each method has its own advantageous and limitations. For instance, slow evaporation and diffusion methods can be performed at room temperature, unlike microwave-assisted methods requiring high temperature and energy costs.87 Microemulsion synthesis of MOFs allows for precise control of MOF topology and surface area; however, it is associated with high costs and large production of environmental pollutants.87 For large-scale production, continuous flow solvothermal methods are often preferred. More recently, microfluidic methods have emerged for smaller microscale, rapid production of MOFs.110 Hence, cost-benefit analysis is required to choose the optimal synthetic protocol for the desired MOF preparation. For further analysis of MOF preparation methods, readers can refer to ref. 84, 87, 107 and 109.Characterization techniques are crucial to understand MOF properties and features and confirm accurate preparation. Mechanical and structural properties of MOFs, such as elasticity, microstructure, and surface topography, atomic force microscopy (AFM) can be used. Transmission electron microscope (TEM) and scanning electron microscope (SEM) techniques can reveal further information about the crystallography properties and defects of MOFs.89,111 However, TEM and SEM are more expensive, have risks of beam damage, with TEM being especially sensitive to contaminants.111 Meanwhile, Brunauer–Emmett–Teller (BET) has been especially useful for understanding surface area and porosity of MOF structures. Thermal stability can generally be characterized using thermal gravimetric analysis (TGA) while colloidal properties are better characterized using dynamic light scattering (DLS) analyses.111 To confirm bonding and understand chemical properties, Fourier-Transform Infrared (FTIR) and Raman spectroscopy are useful.111 Many other MOF features can be easily characterized via a variety of techniques, which have been extensively reviewed in ref. 111–115.
3.2 Promising properties of MOFs for spectrophotometric biosensing
MOFs demonstrate great promise for spectrophotometric biosensing applications, especially spectrophotometric-based sensing. This is because many MOFs demonstrate inherent fluorescent and/or colorimetric nature, which exhibit changes upon analyte detection.33,80,116 Similarly, many MOFs exhibit intrinsic catalytic activity, acting as nanozymes for sensing biological analytes requiring enzyme treatment.26,32,34 Moreover, MOFs can easily be hybridized with other nanomaterials for enhanced sensing performance.117–119 It may be argued that other nanomaterials, such as metal oxides, quantum dots, and metal NPs, all also demonstrate excellent fluorescence, intrinsic catalytic activity, and potential for hybridization – as shown in Table 1. While this is true, MOFs have additional unique properties that make it stand out as promising nanomaterials for biosensing applications. These unique advantages include high surface area and porosity, tunable structures, and functionalizable surfaces. These unique properties are discussed herein.
3.2.1 High surface area & porosity. MOFs are unique in their high surface area and porosity. MOF surface areas can reach up to 10
400 m2 g−1,120 which is significantly greater than that of graphene (∼2000 m2 g−1),121 zeolites (<1100 m2 g−1),122 and other nanomaterials.111 Pore volumes can also extend up to ∼3.60 cm3 g−1, which is superior to other nanomaterials.111,120 This massive internal surface area and pore volumes facilitate entrapment of more analyte molecules within the MOF framework, thus increasing analyte-MOF collisions and facilitating for more sensitive detection and quantification.123,124 Moreover, the large pore volume can facilitate detection of larger biomolecules and analytes, since they can fit into the structure.125 Immobilization of larger molecules can also be facilitated in MOF pores, such as enzymes for cholesterol and glucose detection.126,127 Table 2 below presents some common MOFs used for developing spectrophotometric based sensing of cholesterol, reporting their composition, surface areas, volumes, and its luminescence properties. While some MOFs do not exhibit inherent fluorescent properties, they can be modified and functionalized to be fluorescent, and can be used as nanozymes to catalyze sensing mechanisms.28,126 These MOFs may also be used for enzyme immobilization and stabilization, as discussed later in Section 4.127
Table 2 Key MOFs used in spectrophotometric cholesterol sensors and their structural properties
MOF |
Metal cluster |
Organic linker |
Morphology |
Pore volume (BET, cm3 g−1) |
BET surface area (m2 g−1) |
Luminescence property |
Ref. |
MIL-100 (Fe) |
Fe–O |
Terephthalic acid |
Microsphere shell |
— |
— |
Not fluorescent |
18 |
MIL-101 (Cr) |
Cr–O |
Terephthalic acid |
Octahedral crystal |
— |
— |
Not fluorescent |
28 |
UiO-66 |
Zr–O |
Terephthalic acid |
Octahedral |
0.5453 |
1094 |
Weakly fluorescent |
27 |
ZIF-8 |
Zn–O |
2-Methylimidazole |
Dodecahedral with rhombus faces |
0.617 |
1275–1664 |
Not fluorescent |
25, 126, 128 and 129 |
PCN-333 (Al) |
Al–O |
4′′-s-Triazine-2,4,6-triyl-tribenzoic acid (H3TATB) |
Octahedral |
2.48 |
2550 |
Not fluorescent |
127 |
MOF-5 |
Zn–O |
Terephthalic acid |
Cubic |
— |
18.52 |
Not fluorescent |
30 |
NU-1000 |
Zr–O |
1,3,6,8-Tetra(4-carboxylphenyl)pyrene (H4TBAPy) |
Rod |
1.31 |
1872 |
Fluorescent at 470 nm |
31 |
Co/2Fe bimetallic MOF |
Co–O & Fe–O |
Trimesic acid |
Irregular particles |
— |
1181 |
Not fluorescent |
24 |
MIL-101 (Fe) |
Fe–O |
1,4-Dicarboxybenzene |
Octahedral |
— |
— |
Not fluorescent |
117 |
UiO-66-NH2 |
Zr–O |
2-Aminoterephthalic acid |
Octahedral |
— |
— |
Not fluorescent |
130 |
MoCu-MI |
Cu–O & Mo–O |
2-Methylimidazole |
Nanosheet |
— |
— |
Not fluorescent |
29 |
Cu (PABA) |
Cu–O |
p-Aminobenzoic acid (PABA) |
Ellipsoid |
— |
— |
Not fluorescent |
131 |
CuMOF |
Cu–O |
Terephthalic acid |
Flake-like |
— |
293.7 |
Not fluorescent |
83 |
V-MOF |
V–O |
2-Aminoterephthalic acid |
Irregular particles |
— |
74.74 |
Not fluorescent |
132 |
3.2.2 Tunable structures. MOFs demonstrate great tunability, due to the variety of metal ions and organic linkers that can be used. Conversely, other nanomaterials demonstrate poor tunability due to limited building blocks. For instance, metal NPs are limited in tuning only the metal centers. Similarly, carbon dots and graphene have poor tunability due to limited building blocks (carbon).82 Meanwhile, the wide range of organic linkers available for MOF preparation offer unlimited MOF structures and configurations, as different organic linkers can be luminescent at different wavelengths and can interact different with varying metal nodes. Thus, for spectrophotometric sensing applications, organic linkers and metal clusters can carefully be chosen to target specific analytes, maximizing sensor selectivity.123 This flexibility in tuning nanomaterial luminescent structures to target analytes is unique to MOFs, making them promising candidates for applications in spectrophotometric sensing.
3.2.3 Functionalizable surfaces. Since MOF surfaces majorly consist of organic linkers, surface functionalization is easily facilitated. This subsequently allows for further tuning of MOF sensing properties for more specific and selective analyte detection. The presence of different chemical groups on MOFs surfaces act as potential sites for functionalization of specific molecules, which can play a role in attracting analytes (through affinity or guest–host interactions), turning MOF luminescence on or off, altering MOF dispersibility, among others.31,116,123,133 Surface functionalization can be through adsorption, coordination bonding, or covalent linkages, depending on the organic linker and molecule being attached.31,133 MOFs can also be functionalized with other nanomaterials, to form hybrid nanocomposites with enhanced properties.134 Other nanomaterials are more rigid, with less chances of successful functionalization. Hence, the easily functionalizable surfaces of MOFs can be tuned for specific analyte detection. Combined with MOF's characteristic high surface areas, surface functionalization adds an added advantage for MOFs as promising materials for spectrophotometric sensing applications.Fig. 7 summarizes the main advantageous properties of MOF that make them promising candidates for spectrophotometric cholesterol detection. These include high surface area and porosity, tunability, easy surface functionalization, and intrinsic luminescent and catalytic activity.
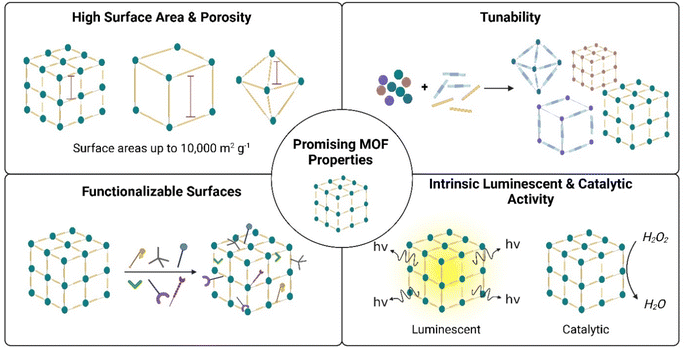 |
| Fig. 7 Summary of promising MOF properties. | |
4. MOFs for spectrophotometric cholesterol detection
Recently, MOFs have emerged as promising nanomaterials for spectrophotometric-based cholesterol detection. MOFs can be used alone24,28,30,31,127,132 or in hybrid composites with other nanomaterial,18,25,27,29,83,117,118,126,128–131 for more effective cholesterol quantification. Generally, MOF-based cholesterol sensors can be categorized into five types, according to the mechanism of sensing adopted, which in turn impacts detector performance. The mechanisms and the performance of these sensors will be discussed below.
4.1 Roles of MOFS in sensing mechanisms
Spectrophotometric sensing of cholesterol through MOFs has been reported widely in the literature. The role of MOFs in cholesterol detection can be categorized into five main types. In some cases, the MOF is used for immobilization of sensing factors,16,17,127–130 while other studies primarily adopt mechanisms where the MOF plays an active role in the sensing process.18,24,27–29,31,83,117,118,126,131,132 Furthermore, these mechanisms implement a variety of spectrophotometric methods, including colorimetry,18,27–30,83,127,128,130,131 fluorescence,24,25,27,31,117,118,126,131,132 and chemiluminescence.83,129 These mechanisms are evaluated and compared in the next few sections. A summary of these mechanisms is illustrated in Fig. 8.
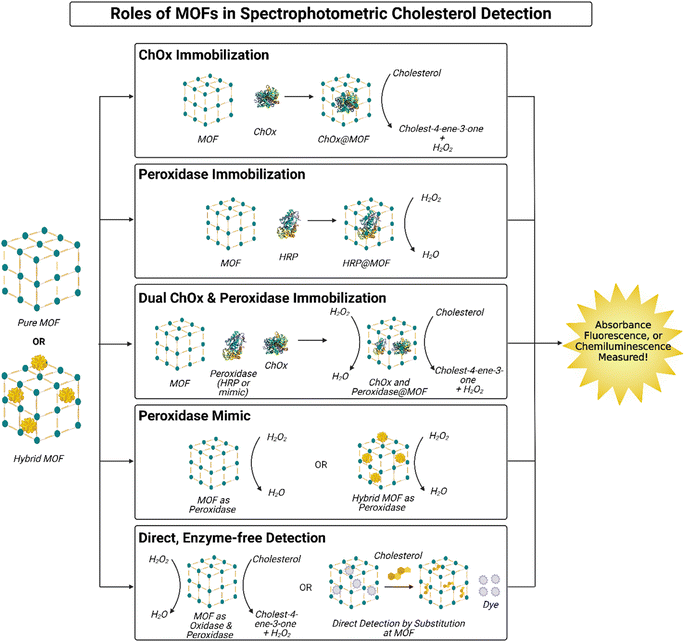 |
| Fig. 8 Summary of MOF roles in spectrophotometric cholesterol detection. | |
4.1.1 MOF for ChOx immobilization. Enzymes are highly pH- and temperature-dependent, thus requiring great care in use and storage to avoid denaturation.16,17 ChOx is a crucial enzyme in cholesterol sensing, as it is needed to catalyze the oxidation of cholesterol to produce hydrogen peroxide, which is subsequently used for cholesterol detection.16,25,30 ChOx immobilization and encapsulation in MOFs is an effective method for improving enzyme stability and enhancing its efficiency.16,17 However, for effective encapsulation, MOF pore size must be optimal to host the enzyme without extensively impacting its conformation;135 small pore dimensions will block out ChOx, preventing immobilization.32MOFs have proven to be effective hosts for ChOx immobilization, in turn facilitating cholesterol detection by prolonging ChOx stability.25,30 ChOx immobilization in MOFs can also facilitate faster oxidation of cholesterol by the enzyme, through maximizing the reaction surface area between cholesterol and ChOx and concentrating ChOx in a smaller area.25,30 For instance, Hassanzadeh et al. utilized MOF-5 for ChOx immobilization via a post-synthetic method,30 while Ding et al. used ZIF-8 (ref. 25) and adopted an in situ method, both demonstrating good performance and minimal ChOx leakage. However, it is important to highlight that the MOFs here do not actively interact with cholesterol chemically; they only act as hosts that enable the first step of the cholesterol detection process. Other agents are needed to continue the reaction. Hassanzadeh et al. couple ChOx@MOF-5 with an Ag nanocluster decorated MoS2 nanosheets, the latter acting as a peroxidase mimic to catalyze hydrogen peroxide reduction and in the presence of terephthalic acid, which is oxidized for colorimetric detection of cholesterol (illustrated in Fig. 9A).30 Meanwhile, Ding et al. opted for a fluorescent method by encapsulating Au nanoclusters (AuNCs) within the ChOx@ZIF-8 complex and decorating it with polyethyleneimine (PEI) to form a hybrid, MOF nanocomplex. Iron(II) ions (Fe2+) are introduced to the system with cholesterol, which react with hydrogen peroxide produced after cholesterol oxidation to produce hydroxyl radicals; this reaction is coined a Fenton reaction. The hydroxyl radicals subsequently oxidize the AuNCs from Au(0) to Au(I), quenching the systems fluorescence. The fluorescence quenching is correlated with cholesterol concentration; thus, cholesterol can easily be quantified.25
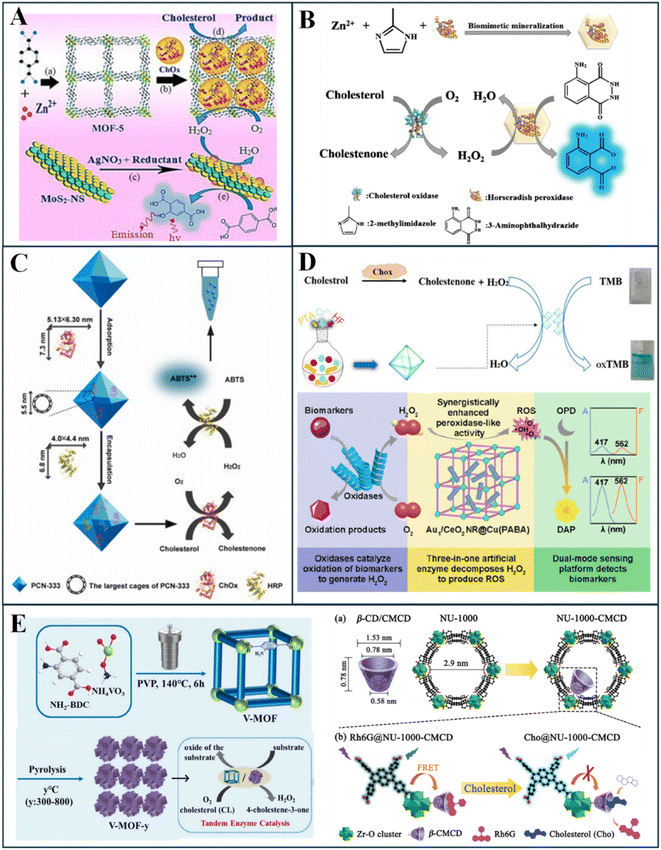 |
| Fig. 9 Roles of MOFs in spectrophotometric cholesterol sensing, including (A) ChOx immobilization, (B) peroxidase immobilization, (C) co-immobilization of ChOx and peroxidase, (D) pure (Top) and hybrid (Bottom) MOFs as peroxidase mimics, and (E) MOFs for direct, enzyme free detection of cholesterol, by dual peroxidase and oxidase activity (left) or direct substitution (right). Reprinted with permission from ref. 28, 30, 31, 67, 129, 131 and 132. | |
The above studies mostly encapsulate ChOx within the MOF pores. Recently, ChOx immobilization on MOF surfaces was explored, rather than inside its pores, via a hybrid structure.118 Xu et al. designed a nanocomposite of Tb-MOF and MnO2 nanosheets – which quench Tb-MOF fluorescence; ChOx is combined with copper phosphate for self-assembly of ChOx-copper phosphate nanoflowers on the MOF surface.118 Through this unique design, cholesterol undergoes rapid cascade reactions, where it is first oxidized by ChOx nanoflowers, then produced hydrogen peroxide reduces MnO2 sheets, thus allowing for Tb-MOF fluorescence recovery. This fluorescence is measured to determine cholesterol concentration.118 Hence, MOFs have proven useful for ChOx immobilization, in pores or on the surface, and subsequent cholesterol detection via fluorescent and colorimetric methods.
4.1.2 MOF for peroxidase immobilization. Like ChOx immobilization, MOFs have also been useful for encapsulating and immobilizing peroxidases, specifically horseradish peroxidase (HRP) – which is traditionally used for in cholesterol detection assays.129 HRP is well-known for its low stability and quick degradation.17,18 Therefore, HRP immobilization in MOFs can significantly prolong its lifetime and improve cholesterol sensing. ZIF-8 demonstrated excellent encapsulation of HRP, through a one-pot synthesis method combining HRP and ZIF-8 precursors.129 However, for a peroxidase@MOF system to effectively detect cholesterol, initial pretreatment with free ChOx is needed to produce hydrogen peroxide, which is later reduced by encapsulated HRP in the presence of a dye for quantification. Xu et al. achieved this by using luminol, a chemiluminescent dye when reacted with hydrogen peroxide, in the presence of HRP@ZIF-8, which initiates a chemiluminescence reaction triggering light emission.129 The intensity of light emitted can be used for cholesterol quantification. The entire synthetic scheme and detection mechanism is illustrated in Fig. 9B. As such, MOFs also prove effective for encapsulating peroxidases (ex: HRP) and stabilizing them for cholesterol detection.
4.1.3 MOF for co-immobilization of ChOx & peroxidase. While MOFs have proven successful for encapsulating HRP and ChOx separately,25,30,129 co-immobilization of these enzymes in one MOF offers greater potential of faster detection using less materials. Through co-immobilization of peroxidases and ChOx in one MOF, cholesterol is oxidized and the product hydrogen peroxide can immediately be oxidized by peroxidase, in the presence of a dye or fluorescent agent, for cholesterol quantification. Zhao et al. achieved this by co-encapsulating HRP and ChOx in PCN-333(Al) MOF, with a high loading capacity and good stability.127 First ChOx was encapsulated, followed by HRP, as illustrated in Fig. 9C. Peroxidase mimics may also be co-immobilized with ChOx, such as hemin128 and AuNPs.130 To effectively secure hemin molecules in ZIF-8, hemin was conjugated to poly(1-vinylimidazole) (PVI) then co-encapsulated with ChOx in ZIF- through a one-pot synthesis.128 Meanwhile, Cao et al. effectively immobilized AuNPs on UiO-66-NH2 MOF surface and then used molecular imprinting to secure ChOx.130 Combined with ABTS127,128 or TMB130 dye, colorimetric detection of cholesterol was successfully achieved by the MOF systems. For effective cascade detection of cholesterol via enzyme co-immobilization, the MOF used should have an optimal pore size and surface area to fit both enzymes while also allowing space for cholesterol to enter and interact with these enzymes. Interfering interactions may also be a concern, especially due to the close proximity of the two enzymes. Nonetheless, this approach has been proven an effective mechanism for cholesterol detection.
4.1.4 MOF as peroxidase mimic. MOFs have also demonstrated active roles in cholesterol detection, unlike the passive role as an enzyme host discussed earlier. MOFs as peroxidase mimics is the most widely studied mechanism for cholesterol detection in the literature.18,24,27–29,83,117,118,126,131 In this approach, the MOF has intrinsic peroxidase activity that catalyzes the reduction of hydrogen peroxide; often time, this is done in the presence of TMB dye, which is oxidized for colorimetric quantification of cholesterol.18,27–29 The absorbance of these colors is correlated with the cholesterol concentration. Pure MOFs can be used for this approach, such as MIL-101(Cr).28 As shown in Fig. 9D (top), MIL-101(Cr) acts as a peroxidase by reducing hydrogen peroxide to H2O, and subsequently oxidizing TMB to produce a color change for colorimetric detection.28 However, most studies adopt hybridized MOF systems as peroxidase mimics. These include Mo-doped Cu-(2-methylimidazole) (Cu-2MI) MOF,29 MIL-100(Fe) coated Fe3O4 magnetic NPs,18 and graphene quantum dots/zirconium-based MOF (GQDs/UiO-66) nanocomposite.27Meanwhile, other studies considered fluorescent dyes at oxidation, such as terephthalic acid,27 o-phenylenediamine (OPD),131 and thiamine,126 to detect emitted light rather than absorbance. These MOF systems are also often hybridized, with Pd nanoclusters,126 Au-loaded CeO2 nanorods,131 or graphene quantum dots.27 For example, Cu(PABA) MOF hybridized with Au-loaded nanorods demonstrated more effective peroxidase activity; combined with OPD fluorescent dye, Au-loaded CeO2 nanorods@Cu(PABA) MOF composite effectively oxidized the dye in the presence of cholesterol, producing a fluorescent change. This mechanism is illustrated further in Fig. 9D (bottom). Peroxidase-like MOFs have also facilitated cholesterol detection by ratiometric fluorescent measurements,24,117 whereby one area of the MOF-system is quenched while the other emits fluorescence; the ratio of these intensities can be used to determine cholesterol concentration. Yang et al. adapted this through a Eu3+-doped MIL-53(Fe) MOF, where hydrogen peroxide exposure enhances MIL-53(Fe) fluorescence and quenches Eu centers.24 MIL-53(Fe) acts as a peroxidase here, catalyzing internal linkages in the MOF thus amplifying its intensity.24 Ye et al. followed a similar approach but through a hybrid MOF-QD system, using bifunctional carbon quantum dots (CQDs)@ MIL-101(Fe). Here, MIL-101(Fe) exhibited peroxidase ability and the CQDs are fluorescent; In the presence of OPD dye, hydrogen peroxide catalyzes the oxidation of OPD into highly fluorescent 2,3-diaminophenosine (DAP), which subsequently quenches CQD fluorescence; hence, the ratio between quenching and fluorescence intensity can be used for cholesterol quantification.117 Chemiluminescence detection using peroxidase-like MOFs has also been demonstrated using Co3O4@CuMOF hybrid system in combination with the chemiluminescent rhodamine B dye.83 The MOF here catalyzes the chemiluminescent, redox reaction between hydrogen peroxide and rhodamine B dye, in turn emitting light and causing a color change.83 In all cases, ChOx pretreatment of cholesterol is required to produce hydrogen peroxide. This is often done using free ChOx, which degrades rapidly.18,24,27–29,83,117,118,126,131 Nonetheless, cholesterol sensors adopting MOFs as peroxidase mimics for the main sensing mechanism have demonstrated excellent promise.
4.1.5 MOF for direct, enzyme-free detection. The final mechanism of cholesterol detection is based on deploying MOFs for direct cholesterol sensing, eliminating the need for any enzymes.31,132 In this case, cholesterol directly interacts with active sites on the MOF, leading to a quantifiable spectrophotometric change. Enzyme-free detection of cholesterol is highly advantageous, since it eliminates the risk of enzyme degradation and related uncertainties. Direct, enzyme-free detection of cholesterol has been done through two main mechanisms: using a MOF as a dual oxidase and peroxidase agent132 and using a MOF for substitution-based detection.31 For example, Wu et al. designed a pyrolized V-MOF which oxidized cholesterol, mimicking ChOx, and catalyzed the oxidation of OPD in the presence of hydrogen peroxide, mimicking HRP. The tandem enzyme activity in this MOF facilitated rapid and accurate cholesterol detection, while also possibly saving costs.132 The synthetic protocol and subsequent tandem enzyme activity (oxidase and peroxidase) is illustrated in Fig. 9E (left). Meanwhile, the second enzyme-free cholesterol detection approach involves functionalizing a MOF using a ligand with high affinity to cholesterol, such as β-cyclodextrin.31 Gong et al. functionalized NU-1000 with carboxymethyl-β-cyclodextrin, then loaded the β-cyclodextrin ligands with rhodamine 6G dye, which quenches the MOF's initial fluorescence. Introducing cholesterol substitutes rhodamine 6G in the MOF, subsequently recovering MOF fluorescence and allowing for cholesterol quantitation, as depicted in Fig. 9E (right).31 Through this mechanism, cholesterol detection is done directly in one-step, without the need for sequential oxidation and peroxide reactions, minimizing possible interferences.31 Hence, enzyme-free cholesterol detection mechanisms are preferred due to their lower costs and enzyme elimination.Fig. 9 illustrates the roles of MOFs in spectrophotometric-based cholesterol sensors, as reported in the literature and discussed above. These include MOFs for ChOx immobilization (Fig. 9A), peroxidase immobilization (Fig. 9B), co-immobilization of ChOx and peroxidase (Fig. 9C), peroxidase mimics (Fig. 9D), and direct, enzyme-free detection of cholesterol (Fig. 9E).
4.2 MOF sensor performance
Different cholesterol sensing mechanisms impact sensor accuracy, sensitivity, selectivity, LOD, and overall performance. It is crucial to understand the relationship between the sensor performance and the associated detection mechanism to achieve superior performance in terms of response time, selectivity, and accuracy. Herein, the performance of a variety of MOF-based spectrophotometric sensors is explored, based on their mechanism of action. Table 3 summarizes all spectrophotometric MOF-based sensors, reporting the composition, mechanism of sensing, detection limits, and linear ranges.
Table 3 Summary of spectrophotometric MOFs used for cholesterol detection
MOF used |
Hybrid or pure MOF |
Sensing (light) mechanism used |
MOF mechanism |
LOD (μM) |
Linear range (μM) |
Ref. |
MOF-5 |
Pure |
Colorimetric |
MOF for ChOx immobilization |
0.03 |
0.06–15 |
30 |
AuNCs/ChOx@ZIF-8/PEI nanocomplexes |
Hybrid |
Fluorescence |
MOF for ChOx immobilization |
0.073 |
0.1–2.4 |
25 |
MnO2 nanosheets on the surface Tb-MOFs. Coupled with hybrid nanoflowers are self-assembled by ChOx and Cu3(PO4)2·3H2O |
Hybrid |
Fluorescence |
MOF for ChOx immobilization |
1.57 |
10–360 |
118 |
HRP@ZIF-8 nanocomposite |
Hybrid |
Chemiluminescence |
MOF for peroxidase immobilization |
0.04 |
0.1–100.0 |
129 |
ChOx & PVI-hemin@ZIF-8 |
Hybrid |
Colorimetric |
MOF for co-immobilization of ChOx & peroxidase |
NR |
5–50 |
128 |
PCN-333(Al)/ChOx & HRP |
Pure |
Colorimetric |
MOF for co-immobilization of ChOx & peroxidase |
0.6 |
0.0–40.0 |
127 |
UiO-66-NH2@AuNPs-ChOx@MIPs |
Hybrid |
Colorimetric |
MOF for co-immobilization of ChOx & peroxidase |
2400 |
2900–6700 |
130 |
Fe3O4 magnetic NPs that are coated by MIL-100(Fe) |
Hybrid |
Colorimetric |
MOF as peroxidase mimic |
0.8 |
2–50 |
18 |
Mo-doped Cu-2MI (2-methylimidazole) [MoCu-2MI] |
Hybrid |
Colorimetric |
MOF as peroxidase mimic |
1.2 |
2–140 |
29 |
MIL-101(Cr) |
Pure |
Colorimetric |
MOF as peroxidase mimic |
0.86 |
1.1–22.9 |
28 |
Graphene quantum dots/zirconium-based metal–organic framework (GQDs/UiO-66) |
Hybrid |
Dual colorimetric & fluorescence |
MOF as peroxidase mimic |
0.01 |
0.04–1.60 |
27 |
Bimetallic Eu/Fe-MOFs [Eu3+-doped MIL-53(Fe)] |
Pure |
Ratiometric fluorescence |
MOF as peroxidase mimic |
10.5 |
2000–8000 |
24 |
Au-loaded CeO2 nanorods (NR) in Cu-based metal–organic frameworks (Cu(PABA)) |
Hybrid |
Dual colorimetric & Fluorometric |
MOF as peroxidase mimic |
Colori: 0.162 |
0.05–40 |
131 |
Fluor: 0.178 |
Bifunctional carbon quantum dots (CQDs)@ MIL-101(Fe) |
Hybrid |
Ratiometric fluorescence |
MOF as peroxidase mimic |
4.55 |
5–100 & 100–1000 |
117 |
Co3O4@CuMOF NPs |
Hybrid |
Dual chemiluminescence & colorimetric |
MOF as peroxidase mimic |
0.026 |
0.1–300 |
83 |
Pd nanoclusters in ZIF-8 (Pd@ZIF-8) |
Hybrid |
Fluorescence |
MOF as peroxidase mimic |
0.92 |
5–1000 |
126 |
V-MOF-700 (pyrolyzed at 700 °C in N2 atm) |
Pure |
Fluorescence |
MOF for direct, enzyme-free detection |
0.38 |
2–160 |
132 |
NU-1000-CMCD |
Pure |
Fluorescent |
MOF for direct, enzyme-free detection |
0.40 |
0–180 |
31 |
4.2.1 Sensors using MOFs for ChOx immobilization. Immobilization of ChOx in MOF structures and nanocomposites can significantly improve catalytic activity and stability, thus facilitating improved cholesterol sensing performance.25,30,118 For instance, Ding et al.25 adopted an in situ technique to synthesize a AuNCs/ChOx@ZIF-8/PEI nanocomposite, which facilitated tandem reactions for cholesterol detection with an LOD of 0.073 μM. Optimal sensor performance was achieved at neutral pH and 37 °C, with a detection time of 15 min. While the results are obtained relatively quickly when compared to traditional cholesterol lab tests, the detection time is quite long for use in point-of-care devices, where cholesterol detection can be achieved in less than five minutes.136 Furthermore, the narrow linear dynamic range (0.1–2.4 μM) limits applications for real life samples, since it poses the need for 1000 time dilutions, which can be challenging and material-consuming.25 An earlier nanocomposite MOF system of ChOx/MOF-5 and AgNC@MoS2 NSs, in combination with TMB dye, achieved a lower LOD of 0.03 μM and a wider linear range of 0.06–15 μM.30 This can be attributed to the high ChOx encapsulation efficiency (25.3%), and greater, preserved catalytic activity due to post-synthetic loading.30 Fig. 10(A1) demonstrates the increase in fluorescence with cholesterol addition, while the linear cholesterol calibration curves and hydrogen peroxide production rates with time of (a) MOF-5 alone, (b) ChOx alone, (c) ChOx@MOF grafted post-synthesis, and (d) ChOx@MOF synthesized in situ are all illustrated in Fig. 10(A2 and A3), respectively. As shown, MOF-5 alone does not have any peroxidase activity, while the best sensitivity to cholesterol was achieved by post-synthetically grafted ChOx@MOF, as depicted by higher hydrogen peroxide production (Fig. 10(A3)) and greater slope of calibration curve (Fig. 10(A2)).
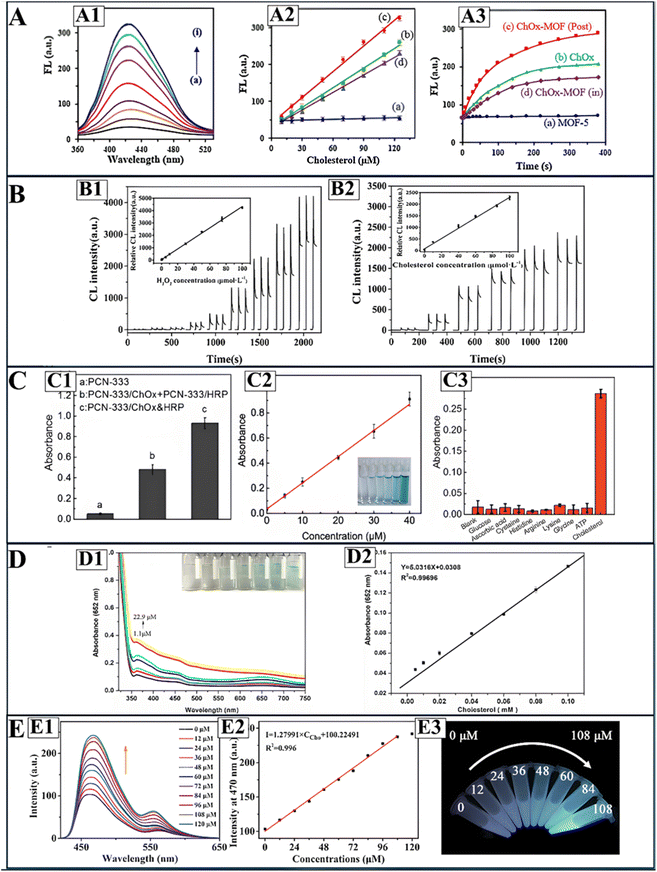 |
| Fig. 10 Sensing performance of MOF-based, spectrophotometric cholesterol sensors. (A) Depicts the performance of ChOx@MOF sensors, where (A1) illustrates turn on fluorescence in presence of cholesterol, (A2) depicts cholesterol calibration curves of (a) post synthetically immobilized ChOx@MOF, (b) free ChOx, (c) in situ ChOx@MOF immobilization, and (d) MOF-5, and (A3) illustrates H2O2 production with ChOx activity over time. (B) Illustrates the chemiluminescence signals attained using peroxidase@MOF sensors when detecting (B1) H2O2 and (B2) cholesterol; inserts represent the calibration curves. (C) Illustrates the (C1) catalytic activity, (C2) cholesterol calibration curve, and (C3) selectivity of co-immobilized ChOx and peroxidase MOF sensors. (D) shows the (D1) turn on fluorescence response to cholesterol and (D2) calibration curve of peroxidase-like MOFs. Finally, (E) depicts the (E1) fluorescence recovery and (E2) calibration curve for direct cholesterol by enzyme-free MOF, with (E3) illustrating the real samples fluorescence at different cholesterol concentrations. Reprinted (adapted) with permission from ref. 28, 30, 67 and 129. | |
Similar to the MOF earlier,25 optimal detection was achieved at neutral pH and physiological temperature (37 °C), but a lower detection time of 10 min. An additional advantage is the recyclability of the sensing material, where 8 cycles of detection maintained sensing efficiency of 90%, indicating great potential for use in reusable PoC devices.30 Both MOF nanoomposites proved effective for free cholesterol and total cholesterol detection applications, the latter requiring an additional pretreatment step with cholesteryl esterase.25,30 Meanwhile, surface functionalization nanoflower-ChOx structures on Tb-MOFs demonstrated a higher LOD of 1.57 μM, but with a wider dynamic range of 10–360 μM, thus showing potential for blood-cholesterol detection applications.118 As such, ChOx immobilization in hybrid MOF sensors have demonstrated great cholesterol sensing performance and potential for real-life applications.
4.2.2 Sensors using MOFs for peroxidase immobilization. Immobilization of HRP, the peroxidase used for traditional cholesterol detection, can improve cholesterol detection through HRP stabilization.129 Through a one-pot synthetic protocol, HRP was immobilized in ZIF-8 pores, which significantly enhanced HRP's temperature tolerance and activity at neutral pH.129 This enhancement in HRP activity allowed for cholesterol detection, by chemiluminescence reaction with luminol, at a low LOD of 0.04 μM and a wide linear dynamic range of 0.1–100.0 μM.129 The chemiluminescence response is demonstrated in the hydrogen peroxide and cholesterol chemiluminescence signals (Fig. 10(B1 and B2), respectively). Furthermore, the LOD and wide dynamic range to hydrogen peroxide and cholesterol is shown by the calibration curves (inserts of Fig. 10(B1 and B2), respectively). This LOD is comparable with results of MOFs with immobilized ChOx,25,30 as well as other nanomaterial detection systems utilizing chemiluminescence mechanisms.69 The HRP@ZIF-8 – luminol sensor performance was validated using real blood samples; however, the main drawback is the high incubation times required for ChOx pretreatment (30 min at 37 °C). Nonetheless, the system proved very precise (relative standard deviations (RSD) below 2.5%), showing promise for future real-life applications.129
4.2.3 Sensors using MOFs for co-immobilization of ChOx & peroxidase. Co-immobilization of ChOx and peroxidases in one MOF system is expected to improve sensor performance due to more rapid, cascade reactions of cholesterol. Many studies tested this through developing a variety of dual ChOx & peroxidase MOF nanocomposites.127,128,130 For instance, Zhao et al.127 co-encapsulated HRP and ChOx in a water-stable PCN-333(Al) MOF while Zhang et al.128 adopted ChOx and hemin, a peroxidase mimic, in a ZIF-8 MOF. Combined with ABTS dye, colorimetric measurements were used for cholesterol quantitation.127,128 Contrary to expectations, both these sensors demonstrated LODs (0.40 & 0.60 μM) and linear ranges (<50 μM) similar to MOF-nanocomposites encapsulating one enzyme type.30,129 For PCN-333(Al) in particular, the greatest catalytic activity was achieved when co-encapsulating ChOx and HRP in PCN-333, as compared to mixing single encapsulated ChOx@PCN-333 and HRP@PCN-33 or PCN-33 alone (Fig. 10(C1)). In contrast, the stability of co-immobilized ChOx and peroxidase MOF sensors is greatly improved, exhibiting longer shelf-life and high tolerances to pH and temperature changes.127,128 Moreover, the dual-encapsulated ChOx&HRP@PCN-333 MOF demonstrated excellent selectivity in the presence of interfering analytes (Fig. 10(C3)), and the linear range calibration curve and color change with cholesterol addition is shown in Fig. 10(C2). Meanwhile, a more recent study adapted a novel technique for grafting both ChOx and AuNPs (peroxidase mimics) on a UiO-66-NH2 MOF, through molecular imprinting.130 While encapsulation was successful, the colorimetric sensor demonstrated a relatively higher LOD in the milli-molar ranges (2.4 mM), and a narrow dynamic range of 2900–6700 μM.130 Since normal blood cholesterol concentrations generally do not exceed 5200 μM,137 the sensor does prove useful for real-life, clinical or PoC applications. However, applications in patients with hypocholesterolemia or hypercholesterolemia may be limited due to the narrow range.130,137 This is further supported by the long shelf-life stability and good reusability of the MOF sensing system, where only 4% of catalytic activity is lost after 25 days and sensor performance only drops by 2.5% after eight cycles of reuse.130 Thus, co-encapsulation of enzymes in MOFs have showing promising results for cholesterol detection, but further enhancements are needed to optimize performance.
4.2.4 Sensors using MOFs as peroxidase mimic. The need for enzyme immobilization and stabilization, as in earlier studies, can limit the overall stability and effectiveness of the MOF system, and limit temperature and pH conditions. Therefore, many studies developed MOF-based composites as peroxidase mimics, also known as nanozymes, to minimize enzyme use and maximize cholesterol sensor performance.18,24,27–29,83,117,118,126,131 Through colorimetric,18,27–29,83,131 fluorescent,24,27,117,126,131 and/or chemiluminescent83 mechanisms, these MOFs have demonstrated great performance in sensing cholesterol. These will be further discussed within.Colorimetric MOF nanocomposites coupled with TMB have facilitated cholesterol detection at micromolar levels, with LODs as low as 0.80 μM.18 Liu et al. used pure MIL-101(Cr) as a peroxidase mimic, achieving an LOD of 0.86 μM and a linear dynamic range of 1.1–22.9 μM.28 While the LOD is low, the linear range is quite narrow, requiring careful dilution of real samples to fit this range. The calibration curve is depicted in Fig. 10(D2) while the increase in color intensity and absorbance with cholesterol addition is shown in Fig. 10(D1). Furthermore, large incubation times (45 min) and higher temperature (60 °C) are required to achieve optimal results.28 Wu et al. overcame these limitations through a hybrid nanocomposite of MIL-100(Fe) coated Fe3O4 magnetic NPs.18 In addition to using iron, which is cheaper than chromium, the system achieved a slightly lower LOD (0.8 μM) and a wider linear range (2–50 μM) at a lower incubation time (15 min) and temperature (50 °C). The MOF-Fe3O4 NP hybrid system also demonstrated great recyclability, retaining over 90% of its effectiveness after 5 cycles of use.18 Meanwhile, a Mo-doped Cu-2MI MOF achieved an even wider linear range (2–140 μM) in 15 min at a lower incubation temperature of 37 °C. While the LOD is slightly higher (1.2 μM), this system is unique in its one-step sensing process, where ChOx, MOF peroxidase mimic, TMB, and cholesterol are all incubated in one step,29 unlike other systems requiring sequential treatment with ChOx then the MOF, prolonging detection.18,28 Despite the differences in sensor performance, the colorimetric mechanism adapted in these sensors is advantageous because a clear color change can be observed, allowing for applications in simple PoC devices.
MOF-based peroxidase mimics have also been used for fluorescent-based cholesterol detection.126 For example, ZIF8 MOFs were decorated with Pd nanoclusters and coupled with thiamine dye, which upon oxidation converts to thiochrome, a fluorescent agent.126 The hybrid nanocomposite demonstrated excellent peroxidase activity at alkaline conditions (pH = 10.5), and cholesterol detection was achieved after incubation for 20 min at 45 °C. Detection limits were as low as 0.92 μM, with cholesterol detection spanning over a 5 to 1000 μM, which is among the widest ranges achieved in the literature.126 However, fluorescent sensors depending on single emission sources are more susceptible to interferences from external sources, thus increasing chances of errors.117,138,139 Therefore, peroxidase-like MOF nanozyme sensors have been designed to sense cholesterol through ratiometric fluorescence,24,117 whereby the ratio of two fluorescent signals at different wavelengths is used to determine analyte concentration.138,139 Ratiometric fluorescence-based sensors demonstrate lower signal-to-noise ratios due to the elimination of background interferences.138,139 Yang et al.24 developed an Eu-doped MIL-53(Fe) MOF for cholesterol quantitation by ratiometric fluorescence, where Eu centers are quenched and MIL-53(Fe) fluorescence increases with hydrogen peroxide; MIL-53(Fe) simultaneously acts as a peroxidase mimic to catalyze hydrogen peroxide reduction. The ratio of the quenched and enhanced fluorescent signals allowed for cholesterol detection at millimolar levels, with an LOD of 0.0105 mM and a linear range of 2000–8000 μM.24 The LOD is much higher than the single-emission fluorescent sensor in ref. 126 and the use of Eu can add costs due to it being a rare metal.140 A later ratiometric fluorescent cholesterol sensor by Ye et al.117 demonstrated better cholesterol detection with an LOD of 4.55 μM and an advantageously wide linear range of 5–1000 μM. Rather than metal-doping, Ye et al. functionalized MIL-101(Fe) MOF with carbon quantum dots, which facilitated more sensitive cholesterol detection at lower concentrations.117 Therefore, MOFs as peroxidase mimics can also facilitate fluorescent-based cholesterol detection.
Recent studies designed peroxidase MOF-based systems with dual fluorescent and colorimetric detection of cholesterol.27,131 Chen et al.131 achieved this through a nanocomposite of CeO2 NRs and Cu(PABA) MOF peroxidase mimic. In combination with OPD dye, cholesterol introduction and hydrogen peroxide peroxidase reaction catalyzes the oxidation of OPD to DAP, a fluorescent, yellow dye. Colorimetric measurements of the yellow dye absorption allowed for a cholesterol detection limit of 0.162 μM, while fluorescence intensity of DAP allowed a slightly higher cholesterol detection limit of 0.178 μM. Meanwhile, both colorimetric and fluorescent measurements allowed for micromolar cholesterol quantitation in the linear range of 0.05–40 μM.131 The peroxidase activity of the MOF-nanocomposite was enhanced by the incorporation of CeO2 NRs, and the sensor demonstrated excellent stability in extreme pH and thermal conditions, as well as a long shelf-life extending to 30 days.131 A GQDs/UiO-66 nanocomposite dual mode colorimetric and fluorescent cholesterol sensor demonstrated a lower cholesterol LOD of 0.01 μM but a narrower dynamic range (0.04–1.60 μM) at room temperature, constraining the dilution needed for real samples.27 Meanwhile, Amirzehni et al.83 explored a novel, dual chemiluminescent and colorimetric detection technique, rather than fluorescence, using the chemiluminescent dye rhodamine B.83 Here, the Co3O4@CuMOF hybrid nanocomposite mimics peroxidases by catalyzing the reaction between rhodamine B and hydrogen peroxide, consequently causing a color change and producing a chemiluminescent intensity.83 Quantification of cholesterol through these signals facilitated micromolar cholesterol detection over a wide range (0.1–300 μM) with an LOD of 0.026 μM.83 Compared to fluorescence, chemiluminescence-based detectors often demonstrate greater sensitivity due to lower background noise as no external light source is needed, thus greater overall sensor performance.141–144 Nonetheless, further improvements are needed to widen the linear range. However, these dual-mode sensors demonstrate great potential for use in medical laboratories or in PoC devices for rapid cholesterol detection.
While the need for HRP is minimized using MOFs as peroxidase mimics, most cases require pretreatment with ChOx for cholesterol oxidation and hydrogen peroxide production to detect free cholesterol.18,24,27–29,83,117,118,126,131 Moreover, for total cholesterol quantitation, further pretreatment with cholesteryl esterase is needed,13,14 which all add to the overall sensor performance time and may require different conditions. However, this versatility also opens windows for using these peroxidase MOF nanozymes for sensing any biomolecules that can produce hydrogen peroxide, such as glucose, galactose, uric acid, lactic, and others, as proven in many studies.117,126,128,131 Multi-biomarker applications of MOF-based sensors are of great potential, and future directions can focus on further improving these systems for accurate detection and clinical, real-life applications.
4.2.5 Sensors using MOFs for direct, enzyme-free detection. Despite the great success of enzyme-based MOF sensors, current trends are shifting towards enzyme-free sensing systems, to minimize instabilities and prolong shelf-lives and operation times. Enzyme-free MOF-based cholesterol sensors are scarce, with only two systems reported in the literature. Wu et al.132 designed a V-MOF, pyrolyzed at 700 °C, to achieve dual oxidase and peroxidase behavior. Coupled with OPD, cholesterol can directly be oxidized by the MOF, then the produced hydrogen peroxide catalyzes conversion of OPD to DAP, resulting in fluorescence. Cholesterol detection was achieved at a LOD of 0.38 μM and over a linear range of 2–160 μM.132 In addition to applications in cholesterol detection, the MOF proved useful for in vitro quantifications of cholesterol in cell membranes, a unique application with various biological impacts.132 Gong et al.31 designed an enzyme-free MOF system with similar performance (LOD 0.4 μM), using β-CMCD-decorated NU-1000 MOFs. However, their design was based on a substitution reaction between cholesterol and rhodamine 6G dye at the β-CMCD pores, causing recovery of MOF fluorescence (Fig. 10(E1)). The dynamic range is shown in the calibration curve in Fig. 10(E2), while the fluorescence recovery can be visually seen when irradiated with UV-light in Fig. 10(E3).31 Nevertheless, both MOF-based sensors demonstrated great selectivity and stability, eliminating the need for enzymes and expanding sensor applications to in vitro assays.31,132Fig. 10 highlights the key performance of MOF-based spectrophotometric cholesterol sensors, depending on the role of the MOF: for ChOx immobilization (Fig. 10A), peroxidase immobilization (Fig. 10B), co-immobilization of ChOx and peroxidase (Fig. 10C), peroxidase mimics (Fig. 10D), and direct, enzyme-free cholesterol detection (Fig. 10E). Performance metrics illustrated include cholesterol detection fluorescent spectra, calibration curves, and selectivity studies, among others.
5. Integration into biosensing platforms
With the high costs and labor needed for laboratory testing, portable sensing platforms have grown in popularity, with research focusing on developing rapid, accurate, and portable point-of-care (PoC) sensing devices for biomarker and metabolite quantification.144,145 Few, spectrophotometric sensing platforms based on smartphone devices have been developed and tested in the literature.146–148 For instance, disposable test strips impregnated with cholesteryl esterase, cholesterol oxidase, 4-aminoantipyrine (4-AAP) and aniline sodium salt were combined with a cloud-enabled smartphone and a photochemical dongle to for colorimetric-based detection of cholesterol via red green blue (RGB) analysis.149 With only 30 μL of blood, the smartphone-based sensing platform can quantify cholesterol concentrations within 2 minutes. Oncescu et al.146 also used standard cholesterol strips for smartphone-based detection, whereby the smartphone's flash was used to illuminate the strip and measure colorimetric changes. Two ranges of cholesterol detection were achieved: low cholesterol concentrations (below 2600 μM) and high cholesterol concentrations (above 10
300 μM).146 However, since normal cholesterol ranges lie around 5.2 mM, the sensor's application is quite limited.14,15,36 Furthermore, an important factor to consider when designing enzymatic strips is the method of immobilization, because weakly immobilized enzymes can lead to leaching and inaccurate analyte quantification.149 Some immobilization techniques often used for test strips include adsorption, encapsulation, entrapment, and covalent linkages.148
Roda et al. developed a similar smartphone-based PoC while using a chemiluminescent-based system with cholesteryl esterase, ChOx, HRP and luminol.147 A 3D printed, miniature cartridge is used (shown in Fig. 11A), replacing paper strips used previously.146,148,149 Through the cartridge accessory contains a back opening where blood samples are introduced. Through a membrane, the sample travels to the reservoir where it mixes with the reagents; here the reaction starts. Since it is a chemiluminescent system, no external light is needed; therefore, the smartphone camera is used to simply capture emitted light from the sample (chemiluminescence), which is displayed on the phone screen.142,147 Only 15 μL of blood is needed for sensing, which can be easily obtained through a finger prick. The smartphone-based platform and its accessory is shown in Fig. 11A. Moreover, the sensing platform had a linear range between 20 mg dL−1 and 386 mg dL−1, equivalent to 520–10
000 μM, which lies within the typical cholesterol ranges observed in patients.147 The calibration curve and detection range, along with the expected signal shown in the smartphone at different concentrations is depicted in Fig. 11A. Although advantageous, these systems are heavily enzyme-dependent, reducing long term stability of test strips or solutions.146–149
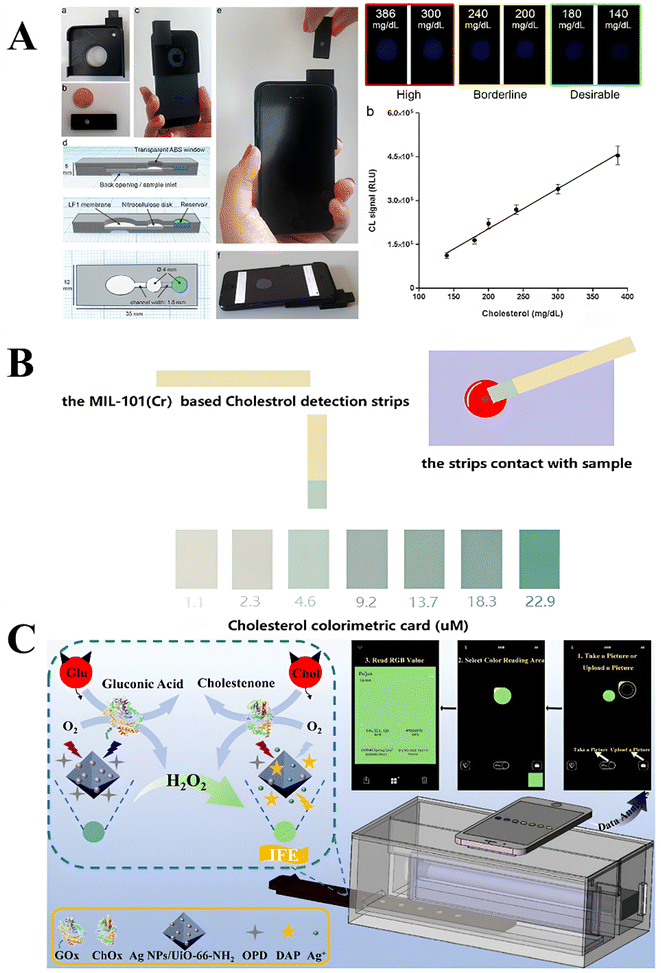 |
| Fig. 11 Illustration of different PoC devices for spectrophotometric cholesterol detection. (A) 3D printed minicartridge containing inlet for sample and reservoir for reagents is attached to smartphone, where chemiluminescence emission is detected for a range of cholesterol concentrations. Enzyme-based reagents are used. Reprinted (adapted) with permission from Roda et al.147 Copyright 2024 American Chemical Society. (B) MIL-100(Cr) based test strips for colorimetric detection of cholesterol, where different color intensities represent the cholesterol concentration. Reprinted (adapted) with permission from Liu et al.28 Copyright (2024) John and Wiley Sons. (C) A MOF-base fluorescence-based PoC device for cholesterol detection, where MOF and enzymes are immobilized in a box, and fluorescence measurements taken using smartphone camera. RGB analysis used to determine cholesterol concentration. Reprinted (adapted) with permission from Guo et al.150 Copyright 2024 American Chemical Society. | |
However, as explored earlier (Section 3.2), MOF-based spectrophotometric sensors can achieve lower LODs in rapid times with high sensitivity and selectivity. As such, future sensing platforms can replace these test strips and enzymatic solutions with MOF-based nanocomposite sensors for better sensor performance and stability, with lower costs. Guo et al.150 achieved this by creating test strips with Ag NPs/UiO-66-NH2 and OPD. Paired with a portable device, where the sample test strip is placed, and a smartphone, the system can be used to measure colorimetric intensities, determine RGB intensities, and subsequently calculate cholesterol concentrations by taking a photograph.150 The MOF sensing mechanism, the portable device, and the RGB pictures and analysis are all depicted in Fig. 11C. A much lower LOD of 10 μM was obtained, with high selectivity to cholesterol.150 However, larger response times were needed for accurate measurements, which is a drawback not observed in many PoC devices. Other MOF-based cholesterol sensors have also demonstrated potential for use in PoC devices, such as MIL-101(Cr), which was successfully developed into simple test strips.28 Once in contact with blood samples, the test strip changes color, and the resulting color is compared to a standard test strip to determine cholesterol concentration range.28 The color changes correlated with different cholesterol concentrations are shown in Fig. 11B.
Research on integration spectrophotometric-MOFs into PoC sensing device is relatively recent. Future studies can design PoC devices that use these strips for accurate cholesterol quantification by measuring RGB intensities. MOF-assays may also be developed into miniature cartridges, especially chemiluminescent systems, as inspired by earlier studies.147 The field of PoC devices for cholesterol detection using MOFs is still young, with many gaps for research and advancement of current technologies and developing new technologies. Fig. 11 illustrates current PoC devices for spectrophotometric cholesterol detection, using enzymes or MOFs.
6. Current challenges & future directions
Despite the variety of advantages offered by MOF-based spectrophotometric sensors for cholesterol detection, many challenges are faced, requiring further research and development to enhance current sensors for optimal results.
6.1 Stability of sensing elements
Cholesterol has very poor solubility in aqueous mediums, and more optimal solubility in organic solvents.9 Meanwhile, if enzymes like ChOx and HRP are used, an aqueous medium mimicking physiological condition is needed for optimal catalytic activity. Hence, MOF-based sensors must exhibit good stability in both aqueous and organic solvents, and over a range of pHs and temperatures, for best sensing applications.33 This challenge is further emphasized when introducing hybrid nanocomposites of different nanomaterials, requiring exhaustive stability tests. While most reported MOF-based sensing materials have been reported to have good performance in different mediums,130,131 one expects the process to developing these systems to be lengthy and difficult. Moreover, as MOF-nanocomposite complexity increases, challenges in identifying the most suitable buffer and pH also arises, introducing new challenges. Future research can look into tackling this issue through high throughput testing applications, to rapidly understand MOF stability in different solvents at one. Furthermore, artificial intelligence and machine learning tools can be used to predict preliminary results, which can save time and steer research towards the correct direction. Enzymes also contribute greatly to the stability of the sensing platform, due to their high sensitivity to changes in temperature, pH, solvent, etc.151–153 Hence, future studies should aim to develop enzyme-free systems with high sensing accuracy and performance for enhanced stability and expanded lifetime of sensing platform.73,151,152 Nonetheless, MOF-based sensors are still advantageous and show great promise for real-life, PoC applications.
6.2 Multi-biomarker detection
While accurate sensing of cholesterol alone is crucial, practical application of MOF-based sensors for multiple biomarker detection are of greater importance and value.131,149 However, detection of multiple biomolecules using one MOF-system is quite challenging, introducing limitations in the sensor design, input areas, materials, concentrations, and much more. This is especially highlighted in PoC applications, where buying multiple sensors for each biomolecule can be costly. Although challenging, multiplex biomarker detection has been implemented successfully in ref. 128, 131 and 149, for dual detection of glucose and cholesterol128 or for detection of glucose, cholesterol, and uric acid.131,149 However, these MOF-sensors can be time consuming. Future studies can focus on improving multiplex cholesterol, MOF-based sensors for more rapid detection at higher sensitivity, perhaps through exploring microfluidics as a promising option since it has been proven useful for electrochemical-based cholesterol detection.154
6.3 Continuous, non-invasive sensing
Patients with hypercholesterolemia, hyperlipidemia, and high risks of cardiovascular diseases require continuous monitoring of cholesterol levels for rapid treatment when spikes occur.155 While multiple, small blood samples can be taken (through finger pricks) and tested using MOF-based spectrophotometric sensors,149 the process is very invasive, and patients may resist multiple monitoring stages throughout the day. Adapting spectrophotometric MOF-based nanocomposites into real-time, non-invasive sensors is a significant challenge, and a promising future direction of research. To tackle non-invasiveness, MOF-based cholesterol sensors can be adapted for detecting cholesterol in saliva156 or tear155 samples, as done in other electrochemical sensors. Designing wearable devices with these MOFs can allow for real-time monitoring.155,157 These fields of research are still in infancy, with MOFs showing great potential in advancing the field of spectrophotometric cholesterol sensing.
6.4 Integration into wearable devices
While MOF-based spectrophotometric has demonstrated high accuracy in cholesterol detection at low concentrations, their integration into wearable devices for continuous cholesterol monitoring remains a challenge. Smart wearable devices for quantification of metabolic biomarkers, including glucose, cholesterol, etc., require miniature sizes and high accuracy and sensitivity to target analyte in the presence of various interferences.158,159 Most MOF-based sensors reported in the literature require specific temperature, pH, and environmental conditions for optimal operation, and deviations from optimized conditions can lead to poor accuracies and sensitivities.158,160 This poses a significant challenge because wearable devices are subjected to various interferences, including skin temperature and pH variations, contamination with dust, sweat, hair interference, among other factors.158,160 This can alter the performance of the wearable biosensor, leading to inaccurate readings and impeding commercialization and clinical integration. While a study succeeded in creating wearable contact lenses for cholesterol monitoring, further clinical trials are needed for full evaluation of their safety and efficacy.161 Thus, future studies should focus on developing more robust, stable sensing systems, using MOFs or other nanomaterials, for more versatile applications in wearable sensors for early detection of diseases and continuous monitoring.
6.5 Scalability & commercialization
Scaling up lab biosensing and chemo-sensing system into point-of-care (PoC) devices, with potential for commercialization and public use, is an emerging field or of high importance in healthcare.162–167 Although numerous studies have developed accurate cholesterol sensing systems, scaling up such systems into PoC devices for commercialization remains a persistent challenge faced in sensing platform development. Various electrochemical-based cholesterol sensors have been commercialized and scaled-up.21 However, more recent spectrophotometric-based cholesterol detection systems remain restricted to research labs. This can be attributed to lack of reliable methods for scaling up production of MOFs and MOF-based composites,168 and the costs associated with spectrophotometric detection components.169 Integration of these nanomaterial-based sensors into microfluidic systems may improve scalability and speed up commercialization, due to wide range of studies on scaling up production of microfluidic systems.166 Hence, future research should aim to investigate methods for improving scalability while maintaining accuracy and reliability of the sensing systems – to allow for their integration into PoC devices and future commercialization at low costs.
6.6 Environmental concerns
The high stability of MOFs, although useful in sensing applications, poses environmental concerns relating to persistence and degradability.170,171 This challenge is significantly highlighted when MOF-based sensors are implemented in PoC devices, since proper disposal becomes more challenging and control over the process is limited.170 If the MOFs used contain toxic metals or organic linkers, which is common in many MOFs, public and environmental health, including aquatic life, become threatened.171,172 Moreover, preparation of MOFs often require harsh acidic conditions and reagents, further increasing environmental concerns relating to these materials.170,171 To minimize environmental impacts concerning synthetic protocols, new synthesis pathways are being developed using less materials.173,174 Furthermore, circular economy principles show great applications in mitigating environmental impacts of MOF synthesis, whereby materials are reused, recycled, and recovered to minimize waste.170 Moreover, MOFs are now being designed using biodegradable linkers, to eliminate both environmental and biological toxicities.173,175 Still, further research is needed to design effective, non-toxic MOFs specific to cholesterol detection, through eco-friendly synthetic approaches.
7. Conclusions
Overall, research on cholesterol sensors continues to grow, with recent interests in spectrophotometric, MOF-based sensors for cholesterol detection. In comparison to other nanomaterials, MOFs have high surface areas, large pore sizes, and highly tunable structures, allowing for flexibility in designing sensitive cholesterol sensors. Spectrophotometric MOFs for cholesterol sensing have specifically grown in popularity over the last decade, as illustrated in Fig. 2. With these increased research interests and the absence of a comprehensive review of these sensors, this paper aims to analyze and review current research on spectrophotometric MOFs for cholesterol sensing. MOFs are valued over other nanomaterials due to their unique high surface area and porosity, flexible tunability, and easy surface functionalization. Furthermore, in contrast to the traditional colorimetric approaches, MOF-based cholesterol sensors follow five different mechanisms of sensing, where MOFs are used for ChOx immobilization peroxidase immobilization, dual ChOx and peroxidase immobilization, peroxidase mimics, and direct, enzyme-free detection of cholesterol. To the best of our knowledge, this paper is the first to categorize these MOF roles and analyze them. Furthermore, spectrophotometric-based MOFs have great potential in PoC device applications, especially smartphone-based devices.
However, some challenges regarding MOF stability and environmental impacts arise. Future research can help overcome these challenges by:
• Developing spectrophotometric MOFs for multi-biomarker detection, including cholesterol, glucose, uric acid, triglycerides, and insulin, etc.
• Exploring development of non-invasive and real-time monitoring cholesterol sensors. This may be done through integrating microfluidics in the sensor platform, for miniaturization and continuous detection.
• Integrating artificial intelligence (AI), where it can be used for optimizing MOF design, predicting sensor performance, and monitoring changes into cholesterol concentrations to predict patient health.
Overall, although the field is quite recent, future applications of spectrophotometric MOFs for cholesterol sensing appear highly promising.
This review paper offers a comprehensive analysis of applications of MOFs in spectrophotometric-based cholesterol sensing, which has not been previously reviewed to the best of the authors' knowledge. The review enables to categorize the various roles that MOFs play for cholesterol sensing and explains the associated attachment mechanisms. Furthermore, this review is informative given its inclusion of the recent literature and advances, as well as real-life applications of MOF-based spectrophotometric sensors in PoC devices. On the other hand, we note that the present review is greatly focused on spectrophotometric MOF detection and thus excludes many studies dealing with cholesterol sensors adapting other techniques, such as plasmonic-based detection techniques.65,77,78 Moreover, the paper focused solely on cholesterol detection, disregarding literature detecting other important biomarkers, like glucose, uric acid, etc. Thus, the review serves as a focused evaluation and analysis of the current literature on MOF-based spectrophotometric cholesterol sensors, which may not be adequate for readers interested in exploring broader sensing platforms including multiple biomarkers or nanomaterials. Nonetheless, this review remains important and timely for researchers interested in developing or improving MOF-based spectrophotometric sensors and integrating them into PoC sensing devices.
Data availability
Data available on request from the authors.
Conflicts of interest
The authors declare no conflict of interest.
Acknowledgements
The authors gratefully acknowledge the financial support by the American University of Sharjah through the Faculty Research Grants (FRG24-C-E60 and FRG23-C-E16). M. Ghommem is grateful to the Dana Gas Endowed Chair fund. The work in this paper was supported by the Open Access Program (OPFY25-3152-OE2523) from the American University of Sharjah. This paper represents the opinions of the author(s) and does not mean to represent the position or opinions of the American University of Sharjah. Fig. 1–8 created in BioRender. H. Abed (2024) https://BioRender.com/a99e671.
References
- D. S. Schade, L. Shey and R. P. Eaton, Endocr. Pract., 2020, 26, 1514–1523 CrossRef PubMed.
- A. Murphy, J. R. Faria-Neto, K. Al-Rasadi, D. Blom, A. Catapano, A. Cuevas, F. Lopez-Jimenez, P. Perel, R. Santos, A. Sniderman, R. Sy, G. F. Watts, D. Zhao, S. Yusuf and D. Wood, Glob. Heart, 2017, 12, 179–197 CrossRef.
- NCD Risk Factor Collaboration (NCD-RisC), Nature, 2020, 582(7810), 73–77 CrossRef.
- C. W. Tsao, A. W. Aday, Z. I. Almarzooq, C. A. M. Anderson, P. Arora, C. L. Avery, C. M. Baker-Smith, A. Z. Beaton, A. K. Boehme, A. E. Buxton, Y. Commodore-Mensah, M. S. V. Elkind, K. R. Evenson, C. Eze-Nliam, S. Fugar, G. Generoso, D. G. Heard, S. Hiremath, J. E. Ho, R. Kalani, D. S. Kazi, D. Ko, D. A. Levine, J. Liu, J. Ma, J. W. Magnani, E. D. Michos, M. E. Mussolino, S. D. Navaneethan, N. I. Parikh, R. Poudel, M. Rezk-Hanna, G. A. Roth, N. S. Shah, M. P. St-Onge, E. L. Thacker, S. S. Virani, J. H. Voeks, N. Y. Wang, N. D. Wong, S. S. Wong, K. Yaffe and S. S. Martin, Circulation, 2023, 147(8), e93–e621 Search PubMed.
- R. K. Upadhyay and R. K. Upadhyay, World J. Cardiovasc. Dis., 2023, 13, 433–469 Search PubMed.
- M. Banach, S. Surma and P. P. Toth, Arch. Med. Sci., 2023, 19, 1602–1615 Search PubMed.
- Y. Song, J. Liu, K. Zhao, L. Gao and J. Zhao, Cell Metab., 2021, 33(10), 1911–1925 CrossRef CAS PubMed.
- V. Narwal, R. Deswal, B. Batra, V. Kalra, R. Hooda, M. Sharma and J. S. Rana, Steroids, 2019, 143, 6–17 CrossRef CAS PubMed.
- D. J. McNamara, Encyclopedia of Human Nutrition, 2013, vol. 1–4, pp. 341–345 Search PubMed.
- K. R. Feingold, in Introduction to Lipids and Lipoproteins, ed. K. R. Feingold, B. Anawalt, M. R. Blackman, et al., Endotext, MDText.com, Inc., South Dartmouth (MA), 2000, available from: https://www.ncbi.nlm.nih.gov/books/NBK305896/ Search PubMed.
- N. A. Elshourbagy, H. V. Meyers and S. S. Abdel-Meguid, Med. Princ. Pract., 2014, 23, 99–111 CrossRef PubMed.
- C. Larson, Am. J. Med. Technol., 1945, 11, 266–271 CAS.
- A. N. Bukiya, H. Li, S. Mysiewicz and W. Li, Cholesterol: from Chemistry and Biophysics to the Clinic, 2022, pp. 259–288 Search PubMed.
- N. S. Biotec, https://nsbiotec.com/wp-content/uploads/2022/07/Cholesterol.pdf.
- Atlas Medical, Liquid Cholesterol (Chod/Pod Method), 2019 Search PubMed.
- S. S. Nadar and V. K. Rathod, Int. J. Biol. Macromol., 2018, 120, 2293–2302 CrossRef CAS PubMed.
- Y. Lin, J. Ren and X. Qu, Acc. Chem. Res., 2014, 47, 1097–1105 CrossRef CAS.
- Y. Wu, Y. Ma, G. Xu, F. Wei, Y. Ma, Q. Song, X. Wang, T. Tang, Y. Song, M. Shi, X. Xu and Q. Hu, Sens. Actuators, B, 2017, 249, 195–202 CrossRef CAS.
- H. M. Yadav, J. D. Park, H. C. Kang and J. J. Lee, Chemosensors, 2021, 9, 98 CrossRef CAS.
- N. Thakur, D. Gupta, D. Mandal and T. C. Nagaiah, Chem. Commun., 2021, 57, 13084–13113 RSC.
- O. Domínguez-Renedo, A. M. Navarro-Cuñado and M. A. Alonso-Lomillo, J. Pharm. Biomed. Anal., 2023, 224, 115195 CrossRef.
- K. Murugesan, S. Das and K. Dutta, Polym.-Plast. Technol. Mater., 2023, 62, 1477–1497 CAS.
- L. Luo, L. Huang, X. Liu, W. Zhang, X. Yao, L. Dou, X. Zhang, Y. Nian, J. Sun and J. Wang, Inorg. Chem., 2019, 58(17), 11382–11388 CrossRef CAS PubMed.
- H. Yang, R. Yang, P. Zhang, Y. Qin, T. Chen and F. Ye, Microchim. Acta, 2017, 184, 4629–4635 CrossRef CAS.
- J. Ding, W. Zhang, F. Xue, Y. Sun, Q. Yan, Y. Chen and G. Shan, Microchim. Acta, 2022, 189, 1–10 CrossRef PubMed.
- X. Zeng, S. Yan and B. F. Liu, Microporous Mesoporous Mater., 2022, 335, 111826 CrossRef CAS.
- H. Abdolmohammad-Zadeh and F. Ahmadian, Microchem. J., 2021, 164, 106001 CrossRef CAS.
- L. Liu, J. Wang, J. Wang, J. Wu, S. Wu and L. Xie, ChemistrySelect, 2021, 6, 7143–7149 CrossRef CAS.
- S. Li, L. Liang, L. Tian, J. Wu, Y. Zhu, Y. Qin, S. Zhao and F. Ye, J. Mater. Chem. B, 2023, 11, 7913–7919 RSC.
- J. Hassanzadeh, A. Khataee and H. Eskandari, Sens. Actuators, B, 2018, 259, 402–410 CrossRef CAS.
- M. Gong, J. Yang, Y. Li, Q. Zhuang and J. Gu, J. Mater. Chem. C, 2019, 7, 12674–12681 RSC.
- X. Huang, S. Zhang, Y. Tang, X. Zhang, Y. Bai and H. Pang, Coord. Chem. Rev., 2021, 449, 214216 CrossRef CAS.
- Y. Zhao, H. Zeng, X. W. Zhu, W. Lu and D. Li, Chem. Soc. Rev., 2021, 50, 4484–4513 RSC.
- S. Li, X. Liu, H. Chai and Y. Huang, TrAC, Trends Anal. Chem., 2018, 105, 391–403 CrossRef CAS.
- A. K. O. Aldulaimi, A. A. Majhoo, S. M. Saeed, M. Adil and A. H. Adhab, Anal. Bioanal. Electrochem., 2023, 15, 778–793 CAS.
- F. E. Kelsey, J. Biol. Chem., 1939, 127(1), 15–22 CrossRef CAS.
- W. M. Sperry and M. Webb, J. Biol. Chem., 1950, 187, 97–106 CrossRef CAS.
- W. Richmond, Clin. Sci. Mol. Med., 1974, 46(1), 6P–7P CAS.
- L. Rastogi, K. Dash and R. B. Sashidhar, Curr. Res. Biotechnol., 2021, 3, 42–48 CrossRef CAS.
- A. Hayat, W. Haider, Y. Raza and J. L. Marty, Talanta, 2015, 143, 157–161 CrossRef CAS PubMed.
- H. Guan, Y. Song, B. Han, D. Gong and N. Zhang, Spectrochim. Acta, Part A, 2020, 241, 118675 CrossRef CAS PubMed.
- Y. Li, Z. Kang, L. Kong, H. Shi, Y. Zhang, M. Cui and D. P. Yang, Mater. Sci. Eng., C, 2019, 104, 110000 CrossRef CAS PubMed.
- C. Hong, X. Zhang, C. Wu, Q. Chen, H. Yang, D. Yang, Z. Huang, R. Cai and W. Tan, ACS Appl. Mater. Interfaces, 2020, 12, 54426–54432 CrossRef CAS PubMed.
- X. Ye, Y. Jiang, X. Mu, Y. Sun, P. Ma, P. Ren and D. Song, Anal. Bioanal. Chem., 2022, 414, 3827–3836 CrossRef CAS.
- N. R. Nirala, P. S. Saxena and A. Srivastava, Spectrochim. Acta, Part A, 2018, 190, 506–512 CrossRef CAS.
- H. C. Chang and J. A. A. Ho, Anal. Chem., 2015, 87, 10362–10367 CrossRef CAS.
- K. E. Kim, T. G. Kim and Y. M. Sung, J. Nanopart. Res., 2012, 14, 1–9 Search PubMed.
- L. H. Li, E. P. Dutkiewicz, Y. C. Huang, H. B. Zhou and C. C. Hsu, J. Food Drug Anal., 2019, 27, 375–386 CrossRef CAS PubMed.
- K. Meno, S. Jennings, A. T. Smith, A. Henriksen and M. Gajhede, Acta Crystallogr. Sect. D Biol. Crystallogr., 2002, 58, 1803–1812 CrossRef PubMed.
- Q. K. Yue, I. J. Kass, N. S. Sampson and A. Vrielink, Biochemistry, 1999, 38, 4277–4286 CrossRef CAS.
- J. C. H. Chen, L. J. W. Miercke, J. Krucinski, J. R. Starr, G. Saenz, X. Wang, C. A. Spilburg, L. G. Lange, J. L. Ellsworth and R. M. Stroud, Biochemistry, 1998, 37, 5107–5117 CrossRef CAS.
- P. Srisawasdi, M. H. Kroll and P. H. Lolekha, Am. J. Clin. Pathol., 2007, 127, 906–918 CrossRef CAS PubMed.
- Anamol Laboratories, Cholesterol Method CHOD-PAP, https://www.anamollabs.com/wp-content/uploads/ALPL-IFU-LC04-CHOLESTEROL-Final-jpg.pdf, (accessed 22 June 2024).
- P. S. Bachorik, R. H. Bradford, T. Cole, I. Frantz, A. M. Gotto, K. Roberts, G. R. Warnick and O. D. Williams, Clin. Chem., 1989, 35, 1734–1739 CrossRef CAS.
- B. Sterling, T. Kiang, K. Subramanian, M. Saltman, W. Smart, M. Tsay, J. Sugarman, D. Patel, D. Monger, D. Martin, I. Gibbons and F. Voss, Clin. Chem., 1992, 38, 1658–1664 CrossRef CAS.
- A. Fazel, Z. Koutoubi, T. B. Sorg and B. Mehrotra, Diabetes Care, 1996, 19, 771–774 CrossRef CAS PubMed.
- Accutrend® Plus system, https://diagnostics.roche.com/global/en/products/instruments/accutrend-plus-ins-754.html#documents, (accessed 21 November 2024).
- SD BIOSENSOR | PRODUCTS, https://www.sdbiosensor.com/product/product_view?product_no=188#, (accessed 21 November 2024).
- CardioChek Products Overview – PTS Diagnostics, https://www.ptsdiagnostics.com/cardiochek-products-overview/, (accessed 21 November 2024).
- S. Kurstjens, E. Gemen, S. Walk, T. Njo, J. Krabbe, K. Gijzen, M. G. L. M. Elisen and R. Kusters, Ann. Clin. Biochem., 2021, 58, 289–296 CrossRef CAS PubMed.
- M. Handayani, Hendrik, A. Abbas, I. Anshori, R. Mulyawan, A. Satriawan, W. Shalannanda, C. Setianingsih, C. T. R. Pingak, Q. Zahro, A. C. S. Rurisa, I. Setiawan, K. Khotimah, G. K. Sunnardianto and Y. D. Rahmayanti, Nanotechnol. Rev., 2023, 12(1) DOI:10.1515/ntrev-2023-0168.
- M. Ameen Sha, P. C. Meenu, H. Haspel and Z. Konya, RSC Adv., 2024, 14, 24561–24573 RSC.
- K. S. Eom, Y. J. Lee, H. W. Seo, J. Y. Kang, J. S. Shim and S. H. Lee, Analyst, 2020, 145, 908–916 RSC.
- M. M. Rahman, X. B. Li, J. Kim, B. O. Lim, A. J. S. Ahammad and J. J. Lee, Sens. Actuators, B, 2014, 202, 536–542 CrossRef CAS.
- Y. Wu, J. Y. Chen and W. M. He, Sens. Actuators, B, 2022, 365, 131939 CrossRef CAS.
- J. Q. Li, Y. W. Mao, R. Zhang, A. J. Wang and J. J. Feng, Colloids Surf. B Biointerfaces, 2023, 232, 113589 CrossRef CAS PubMed.
- H. Zhao, X. Yuan, F. Bai, Y. Wang and L. Zhao, Microchim. Acta, 2023, 190, 1–11 CrossRef PubMed.
- Y. Mao, L. Wang, K. Zhang, H. Zhang and J. Yang, Langmuir, 2023, 39, 17286–17294 CrossRef CAS PubMed.
- L. Hong, A. L. Liu, G. W. Li, W. Chen and X. H. Lin, Biosens. Bioelectron., 2013, 43, 1–5 CrossRef CAS PubMed.
- X. Zhang, M. Wei, B. Lv, Y. Liu, X. Liu and W. Wei, RSC Adv., 2016, 6, 35001–35007 RSC.
- A. Kumar, A. Kumari, S. Asu, D. Laha and S. Kumar Sahu, ChemistrySelect, 2019, 4, 14222–14227 CrossRef CAS.
- T. T. Bui and S. Y. Park, Green Chem., 2016, 18, 4245–4253 RSC.
- E. Priyadarshini and K. Rawat, J. Mater. Chem. B, 2017, 5, 5425–5432 RSC.
- M. A. El-Naka, A. El-Dissouky, G. Y. Ali, S. Ebrahim and A. Shokry, Talanta, 2023, 253, 123908 CrossRef CAS PubMed.
- S. Bhaskar, S. M. S. Lis, S. Kanvah, B. N. Shivakiran Bhaktha and S. S. Ramamurthy, ACS Appl. Opt. Mater., 2023, 1, 159–172 CrossRef CAS.
- M. Basak, H. B. Nemade and D. Bandyopadhyay, Biosens. Bioelectron., 2025, 268, 116885 CrossRef CAS PubMed.
- M. Wagner, A. Seifert and L. M. Liz-Marzán, Nanoscale Horiz., 2022, 7, 1259–1278 RSC.
- S. Kumar, R. Singh, Z. Wang, M. Li, X. Liu, W. Zhang, B. Zhang and G. Li, Results Opt., 2023, 10, 100342 CrossRef.
- I. I. Ebralidze, N. O. Laschuk, J. Poisson and O. V. Zenkina, Nanomaterials Design for Sensing Applications, 2019, pp. 1–39 Search PubMed.
- A. Chandra, S. Prasad, G. Gigli and L. L. del Mercato, Front. Nanosci., 2020, 16, 117–149 CAS.
- Y. Liu, X. Feng, Y. Yu, Q. Zhao, C. Tang and J. Zhang, Anal. Chim. Acta, 2020, 1110, 141–150 CrossRef CAS PubMed.
- M. I. Gaviria-Arroyave, J. B. Cano and G. A. Peñuela, Talanta Open, 2020, 2, 100006 CrossRef.
- M. Amirzehni, H. Eskandari, B. Vahid and J. Hassanzadeh, Sens. Actuators, B, 2021, 348, 130690 CrossRef CAS.
- D. Li, A. Yadav, H. Zhou, K. Roy, P. Thanasekaran and C. Lee, Global chall., 2024, 8, 2300244 CrossRef PubMed.
- H. C. Zhou, J. R. Long and O. M. Yaghi, Chem. Rev., 2012, 112, 673–674 CrossRef CAS PubMed.
- K. Elsaid, A. Elkamel, E. T. Sayed, T. Wilberforce, M. A. Abdelkareem and A. G. Olabi, Encyclopedia of Smart Materials, 2022, pp. 566–577 Search PubMed.
- C. P. Raptopoulou, Materials, 2021, 14, 1–32 CrossRef PubMed.
- M. Moharramnejad, A. Ehsani, S. salmani, M. shahi, R. E. Malekshah, Z. S. Robatjazi and H. Parsimehr, J. Inorg. Organomet. Polym. Mater., 2022, 32, 3339–3354 CrossRef CAS.
- S. A. Jasim, I. M. Amin, A. Rajabizadeh, M. A. Lima Nobre, F. Borhani, A. T. Jalil, M. M. Saleh, M. M. Kadhim and M. Khatami, Water Sci. Technol., 2022, 1 Search PubMed.
- K. Ahmad, M. A. Nazir, A. K. Qureshi, E. Hussain, T. Najam, M. S. Javed, S. S. A. Shah, M. K. Tufail, S. Hussain, N. A. Khan, H. ur R. Shah and M. Ashfaq, Mater. Sci. Eng. B, 2020, 262, 114766 CrossRef CAS.
- J. Fu and Y. nan Wu, Chem.–Eur. J., 2021, 27, 9967–9987 CrossRef CAS PubMed.
- M. Gutiérrez, F. Sánchez and A. Douhal, Phys. Chem. Chem. Phys., 2016, 18, 5112–5120 Search PubMed.
- Y. Bai, Y. Dou, L. H. Xie, W. Rutledge, J. R. Li and H. C. Zhou, Chem. Soc. Rev., 2016, 45, 2327–2367 CAS.
- J. Chen, F. Cheng, D. Luo, J. Huang, J. Ouyang, A. Nezamzadeh-Ejhieh, M. S. Khan, J. Liu and Y. Peng, Dalton Trans., 2022, 51, 14817–14832 CAS.
- L. Li, X. S. Wang, T. F. Liu and J. Ye, Small Methods, 2020, 4, 2000486 CrossRef CAS.
- P. Chowdhury, C. Bikkina and S. Gumma, J. Phys. Chem. C, 2009, 113, 6616–6621 CAS.
- L. Bromberg, Y. Diao, H. Wu, S. A. Speakman and T. A. Hatton, Chem. Mater., 2012, 24, 1664–1675 CAS.
- T. Wu, N. Prasetya and K. Li, J. Membr. Sci., 2020, 615, 118493 CAS.
- V. F. Yusuf, N. I. Malek and S. K. Kailasa, ACS Omega, 2022, 7, 44507–44531 CAS.
- L. Zou, C. C. Hou, Z. Liu, H. Pang and Q. Xu, J. Am. Chem. Soc., 2018, 140, 15393–15401 CAS.
- R. C. Arbulu, Y. B. Jiang, E. J. Peterson and Y. Qin, Angew. Chem., Int. Ed., 2018, 57, 5813–5817 CrossRef CAS PubMed.
- C. Wu, L. Y. Chou, L. Long, X. Si, W. S. Lo, C. K. Tsung and T. Li, ACS Appl. Mater. Interfaces, 2019, 11, 35820–35826 CrossRef CAS PubMed.
- Y. Dai, G. Zhang, Y. Peng, Y. Li, H. Chi and H. Pang, Adv. Colloid Interface Sci., 2023, 321, 103022 CrossRef CAS PubMed.
- X. Pan, Q. Zhu, K. Yu, M. Yan, W. Luo, S. C. E. Tsang and L. Mai, Next Mater., 2023, 1, 100010 CrossRef.
- J. Yang, W. Ni, B. Ruan, L.-C. Tsai, N. Ma, D. Shi, T. Jiang and F.-C. Tsai, ECS J. Solid State Sci. Technol., 2021, 10, 056003 CrossRef CAS.
- V. Unnikrishnan, O. Zabihi, M. Ahmadi, Q. Li, P. Blanchard, A. Kiziltas and M. Naebe, J. Mater. Chem. A, 2021, 9, 4348–4378 RSC.
- N. Stock and S. Biswas, Chem. Rev., 2012, 112, 933–969 CrossRef CAS PubMed.
- B. Valizadeh, T. N. Nguyen and K. C. Stylianou, Polyhedron, 2018, 145, 1–15 CrossRef CAS.
- Y. Qian, F. Zhang, H. Pang, Y. Qian, H. Pang and F. Zhang, Adv. Funct. Mater., 2021, 31, 2104231 CrossRef CAS.
- C. Echaide-Górriz, C. Clément, F. Cacho-Bailo, C. Téllez and J. Coronas, J. Mater. Chem. A, 2018, 6, 5485–5506 RSC.
- A. F. Payam, S. Khalil and S. Chakrabarti, Small, 2024, 2310348 CrossRef CAS PubMed.
- V. V Butova, M. A. Soldatov, A. A. Guda, K. A. Lomachenko and C. Lamberti, Russ. Chem. Rev., 2016, 85, 280–307 CrossRef.
- S. O. Odoh, C. J. Cramer, D. G. Truhlar and L. Gagliardi, Chem. Rev., 2015, 115, 6051–6111 CrossRef CAS PubMed.
- B. E. G. Lucier, S. Chen and Y. Huang, Acc. Chem. Res., 2018, 51, 319–330 CrossRef CAS.
- H. R. Abid, M. R. Azhar, S. Iglauer, Z. H. Rada, A. Al-Yaseri and A. Keshavarz, Heliyon, 2024, 10, e23840 CrossRef CAS.
- W. P. Lustig, S. Mukherjee, N. D. Rudd, A. V. Desai, J. Li and S. K. Ghosh, Chem. Soc. Rev., 2017, 46, 3242–3285 RSC.
- Q. Ye, T. Dai, J. Shen, Q. Xu, X. Hu and Y. Shu, J. Anal. Test, 2023, 7, 16–24 CrossRef.
- D. Xu, C. Li, Y. Zi, D. Jiang, F. Qu and X. E. Zhao, Nanotechnology, 2021, 32, 315502 CrossRef CAS PubMed.
- L. Giri, S. R. Rout, R. S. Varma, M. Otyepka, K. Jayaramulu and R. Dandela, Nanotechnol. Rev., 2022, 11, 1947–1976 CAS.
- H. Furukawa, N. Ko, Y. B. Go, N. Aratani, S. B. Choi, E. Choi, A. Ö. Yazaydin, R. Q. Snurr, M. O'Keeffe, J. Kim and O. M. Yaghi, Science, 2010, 329, 424–428 CrossRef CAS PubMed.
- P. Kamedulski, M. Skorupska, P. Binkowski, W. Arendarska, A. Ilnicka and J. P. Lukaszewicz, Sci. Rep., 2021, 11(1), 1–12 Search PubMed.
- M. Rahmati and H. Modarress, Mol. Simul., 2012, 38, 1038–1047 CAS.
- M. Marimuthu, S. S. Arumugam, D. Sabarinathan, H. Li and Q. Chen, Trends Food Sci. Technol., 2021, 116, 1002–1028 CAS.
- S. A. A. Razavi and A. Morsali, Coord. Chem. Rev., 2020, 415, 213299 CAS.
- A. G. Zavyalova, D. V. Kladko, I. Y. Chernyshov and V. V. Vinogradov, J. Mater. Chem. A, 2021, 9, 25258–25271 RSC.
- Y. Li, S. Li, M. Bao, L. Zhang, C. Carraro, R. Maboudian, A. Liu, W. Wei, Y. Zhang and S. Liu, ACS Appl. Nano Mater., 2021, 4, 9132–9142 CrossRef CAS.
- M. Zhao, Y. Li, X. Ma, M. Xia and Y. Zhang, Talanta, 2019, 200, 293–299 CrossRef CAS PubMed.
- X. Zhang, F. Zhang, Z. Lu, Q. Xu, C. Hou and Z. Wang, ACS Appl. Mater. Interfaces, 2020, 12, 25565–25571 CrossRef CAS PubMed.
- X. Xu, Y. Zhao, H. Tan, Y. Ma and Y. Li, Microchim. Acta, 2020, 187, 1–8 CrossRef PubMed.
- M. Cao, C. Huang, Y. Zhang, X. Yang, L. Cui, A. Li, J. Xu and J. Liu, Sens. Actuators, B, 2024, 404, 135235 CrossRef CAS.
- P. Chen, Y. Peng, L. Lin, Y. Yuan, J. Chen, J. Mo, J. Miao, H. He, Y. Jin, L. Zhang and S. Du, ACS Sustain. Chem. Eng., 2023, 11, 8106–8119 CrossRef CAS.
- Y. Wu, W. Fang, Y. Hu, J. Dang, S. Xin, M. Li, Z. Li and H. Zhao, J. Colloid Interface Sci., 2023, 649, 601–615 CrossRef CAS PubMed.
- J. Y. Oh, Y. Sim, G. Yang, M. H. Park, K. Kim and J. H. Ryu, Inorg. Chem. Front., 2024, 11, 3119–3135 RSC.
- K. Xu, S. Zhang, X. Zhuang, G. Zhang, Y. Tang and H. Pang, Adv. Colloid Interface Sci., 2024, 323, 103050 CrossRef CAS.
- D. S. Raja, W. L. Liu, H. Y. Huang and C. H. Lin, Comments Inorg. Chem., 2015, 35, 331–349 CrossRef.
- A. Plüddemann, M. Thompson, C. P. Price, J. Wolstenholme and C. Heneghan, Br. J. Gen. Pract., 2012, 62, e224 CrossRef.
- Overview: High cholesterol - InformedHealth.org - NCBI Bookshelf, https://www.ncbi.nlm.nih.gov/books/NBK279318/, (accessed 5 June 2024).
- L. Liu, L. Ga and J. Ai, Biosens. Bioelectron., 2022, 213, 114456 CrossRef CAS.
- J. Miao, W. Ji, J. Yu, J. Cheng, Y. Huang, M. Arabi, N. Zhou, B. Li, Z. Zhang, L. Chen and X. Wang, Sens. Actuators, B, 2023, 384, 133636 CAS.
- B. Van Den Bogaert, D. Havaux, K. Binnemans and T. Van Gerven, Green Chem., 2015, 17, 2180–2187 RSC.
- C. Dodeigne, L. Thunus and R. Lejeune, Talanta, 2000, 51, 415–439 CrossRef CAS.
- UV-visible Absorption, Fluorescence, and Chemiluminescence Spectroscopy, Handbook of Food Analysis, ed. L. M. L. Nollet and F. Toldra, CRC Press, 3rd edn, 2015, vol. 2, DOI:10.1201/b18668.
- M. T. Potamia and A. C. Calokerinos, Anal. Lett., 2013, 46, 2657–2672 CrossRef CAS.
- M. Zhang, X. Cui and N. Li, Mater. Today Bio., 2022, 14, 100254 CrossRef PubMed.
- W. Chen, Y. Yao, T. Chen, W. Shen, S. Tang and H. K. Lee, Biosens. Bioelectron., 2021, 172, 112788 CrossRef CAS PubMed.
- V. Oncescu, M. Mancuso and D. Erickson, Lab Chip, 2014, 14, 759–763 RSC.
- A. Roda, E. Michelini, L. Cevenini, D. Calabria, M. M. Calabretta and P. Simoni, Anal. Chem., 2014, 86, 7299–7304 CrossRef CAS.
- Y. Li, Z. Li and H. Liu, J. Electrochem. Soc., 2020, 167, 037535 CrossRef.
- W. Duan, J. Cheng and J. Guo, Analyst, 2022, 147, 3285–3290 RSC.
- L. Guo, S. Chen, Y. L. Yu and J. H. Wang, Anal. Chem., 2021, 93, 16240–16247 CrossRef CAS.
- N. Joshi, K. Rawat, P. R. Solanki and H. B. Bohidar, Biochem. Eng. J., 2015, 102, 69–73 CrossRef CAS.
- R. Chokkareddy, N. Thondavada, S. Thakur and S. Kanchi, Advanced Biosensors for Health Care Applications, 2019, pp. 315–339 Search PubMed.
- X. Chen, G. Wu, Z. Cai, M. Oyama and X. Chen, Microchim. Acta, 2014, 181, 689–705 CrossRef CAS.
- A. Wisitsoraat, P. Sritongkham, C. Karuwan, D. Phokharatkul, T. Maturos and A. Tuantranont, Biosens. Bioelectron., 2010, 26, 1514–1520 CrossRef CAS.
- H. Song, H. Shin, H. Seo, W. Park, B. J. Joo, J. Kim, J. Kim, H. K. Kim, J. Kim and J. U. Park, Adv. Sci., 2022, 9, 2203597 CrossRef PubMed.
- R. Vinoth, P. Sangavi, T. Nakagawa, M. Jayaraman and A. M. V. Mohan, Sens. Actuators, B, 2023, 379, 133214 CrossRef CAS.
- J. Gao, W. Huang, Z. Chen, C. Yi and L. Jiang, Sens. Actuators, B, 2019, 287, 102–110 CrossRef CAS.
- R. Ayyanu, A. Arul, N. Song, A. Anand Babu Christus, X. Li, G. Tamilselvan, Y. Bu, S. Kavitha, Z. Zhang and N. Liu, Analyst, 2023, 148, 4616–4636 RSC.
- Y. Yoon, P. L. Truong, D. Lee and S. H. Ko, ACS Nanosci. Au, 2022, 2, 64–92 CrossRef CAS PubMed.
- K. Bayoumy, M. Gaber, A. Elshafeey, O. Mhaimeed, E. H. Dineen, F. A. Marvel, S. S. Martin, E. D. Muse, M. P. Turakhia, K. G. Tarakji and M. B. Elshazly, Nat. Rev. Cardiol., 2021, 18(8), 581–599 CrossRef CAS.
- H. Song, H. Shin, H. Seo, W. Park, B. J. Joo, J. Kim, J. Kim, H. K. Kim, J. Kim and J. U. Park, Adv. Sci., 2022, 9, 2203597 CrossRef PubMed.
- J. Tu, R. M. Torrente-Rodríguez, M. Wang and W. Gao, Adv. Funct. Mater., 2020, 30, 1906713 CrossRef CAS.
- A. Mishra, P. K. Singh, N. Chauhan, S. Roy, A. Tiwari, S. Gupta, A. Tiwari, S. Patra, T. R. Das, P. Mishra, A. S. Nejad, Y. K. Shukla, U. Jain and A. Tiwari, Sens. Diagn., 2024, 3, 718–744 RSC.
- S. K. Vashist and J. H. T. Luong, Point-of-Care Technologies Enabling Next-Generation Healthcare Monitoring and Management, 2019, pp. 1–25 Search PubMed.
- A. R. Khan, W. L. Hussain, H. C. Shum and S. U. Hassan, Front. Lab. Chip. Technol., 2024, 3, 1394752 CrossRef.
- S. M. Yang, S. Lv, W. Zhang and Y. Cui, Sensors, 2022, 22, 1620 CrossRef CAS PubMed.
- I. M. Hwang, X. A. Lou, A. A. Toubian and D. T. Kamei, BioSensing, Theranostics, and Medical Devices: from Laboratory to Point-of-Care Testing, 2021, pp. 83–104 Search PubMed.
- T. Paul, A. Juma, R. Alqerem, G. Karanikolos, H. A. Arafat and L. F. Dumée, J. Environ. Chem. Eng., 2023, 11, 111112 CrossRef CAS.
- A. Farahani, S. Azimi and M. Azimi, Microchem. J., 2022, 182, 107930 CrossRef CAS.
- B. Farasati Far, N. Rabiee and S. Iravani, RSC Adv., 2023, 13, 34562 RSC.
- Y. Wen, P. Zhang, V. K. Sharma, X. Ma and H. C. Zhou, Cell Rep. Phys. Sci., 2021, 2, 100348 CrossRef CAS.
- P. Kumar, B. Anand, Y. F. Tsang, K. H. Kim, S. Khullar and B. Wang, Environ. Res., 2019, 176, 108488 CrossRef CAS PubMed.
- R. A. dos Reis, L. A. M. Mahmoud, E. H. Ivanovska, R. Telford, M. A. Addicoat, L. R. Terry, V. P. Ting and S. Nayak, Adv. Sustainable Syst., 2023, 7, 2300269 CrossRef.
- A. O. C. Iroegbu and S. S. Ray, Nanotechnol. Rev., 2022, 11, 1696–1721 CrossRef CAS.
- N. Singh, S. Qutub and N. M. Khashab, J. Mater. Chem. B, 2021, 9, 5925–5934 RSC.
|
This journal is © The Royal Society of Chemistry 2024 |
Click here to see how this site uses Cookies. View our privacy policy here.