DOI:
10.1039/D4SD00009A
(Paper)
Sens. Diagn., 2024,
3, 863-871
A methodological study for the diagnosis of the SARS-Cov-2 infection in human serum with a macrocyclic sensor array†
Received
8th January 2024
, Accepted 1st April 2024
First published on 22nd April 2024
Abstract
This article reports the methodology and the proof of concept of a blood-based diagnostic strategy for the SARS-CoV-2 infection. The proposed method relies on the non-specific/selective array-based sensing strategy mimicking the human olfactory system using a cucurbit[7]uril macrocycle receptor conjugated with a library of environmentally sensitive fluorophores. The study cohort includes 26 samples, i.e. 12 cases and 14 controls. Statistical analysis methods such as linear discriminant and random forest were able to successfully classify and discriminate the two groups with almost 90% accuracy. This diagnostic result highlights the methodology and confirms the potential of this non-specific/selective sensing approach for non-invasive clinical diagnosis.
1. Introduction
Since the end of 2019, the COVID-19 disease has been an ongoing threat spreading worldwide, with more than 600 million infections and more than 6 million deaths.1 On March 11, 2020, it was classified as a global pandemic by the World Health Organization (WHO). Therefore, its diagnosis is considered indispensable to prevent and control the spreading of the disease. Currently, two types of diagnostic tests, based on nasopharyngeal or nasal swabs, are widely used: i) nucleic acid amplification tests based on polymerase chain reaction (PCR) technology that detect viral RNA; ii) antigen tests based on lateral flow immunoassays (LFAs) that detect viral proteins (i.e. spike, envelope or nucleotide proteins). The accuracy, specificity, and sensitivity of a test as well as the time between the test and results are the main criteria to control the spreading of the disease. Antigen detection can provide results within 15 min with low accuracy and is subject to delivering false negatives, particularly when used in people with no signs or symptoms of the infection (up to 45% of false negatives).2 This drawback results in high risk of virus dissemination. Consequently, PCR tests are often used for the confirmation of antigen tests and, therefore, might not suffer from false negatives. Even if the analytical performance of PCR tests approaches 100% by detecting 500–5000 RNA copies per mL, clinical performance approaches only 80% sensitivity due to biological and pre-analytical factors, particularly sample collection. Nasal and nasopharyngeal swabs are partly responsible for false negatives since the quality of specimen collection may be low and viral loads in the sample are neither homogeneous nor stable within the time of infection. Moreover, the delay between sampling and results can extend from 24 to 48 h due to time-consuming laboratory procedures that require certified laboratories, trained operators, and expensive equipment.3 While nasopharyngeal swabs are still the widely used specimen, many works explore diagnosis methods based on specimens from other types of samples such as the upper respiratory tract (throat and deep throat saliva), lower respiratory tract (sputum and bronchoalveolar lavage fluid), nasopharynx, feces, and blood.4–11 Herein, we propose a diagnostic strategy for the SARS-CoV-2 infection based on blood samples whose sampling method, stability, and homogeneity are highly controlled.
Blood serologic tests to detect host-derived antibodies against viruses (IgM and IgG) are not efficient for diagnosing acute COVID-19 but rather previous infection and/or vaccination. For initial diagnoses based on viral RNA detection, the US-CDC does not recommend the use of blood samples.12 However, SARS-CoV-2 infection is still responsible for pronounced changes in the blood composition. Many reviews investigate routine biochemical, immunological but also inflammatory or nucleic acid biomarkers as a promising avenue for early diagnosis and prediction of prognosis of COVID-19.13–16 A proteomic and metabolomic study found that 105 proteins and 373 metabolites were differentially expressed in the sera of COVID-19 patients compared to the control.17 However, no specific blood biomarker, even built as a combination of expressions, has been still clinically validated so far. Diagnostic strategy using proteomic or/and metabolomic signatures would have been too expensive to build for routine purpose. Routine blood parameters such as hematological (lymphocyte and neutrophil count), inflammatory (C-reactive protein), biochemical like (albumin, lactate dehydrogenase, alanine and aspartate aminotransferase and alkaline phosphatase) have been described as dysregulated in SARS-Cov-2 infected patients.18 Combining appropriate cutoffs for certain of these blood parameters could help in identifying COVID-19 positive patients but with a low accuracy.3 Also, when used in a machine learning model, they can help to differentiate the status of patients with 82–86% accuracy and 92–95% sensitivity.19 However, this strategy requires many blood parameters to be measured and therefore remains slow and expensive if transposed at the population level. Hence, the “Chemical Nose” hypothesis-free machine learning diagnostic strategy looking for global blood change composition rather than changes of some specific biomarkers or a combination of those will be suitable for a low-cost blood-based diagnostic strategy.
The “Chemical Nose” strategy mimics the human olfactory system using a set of non-specific sensors to sense the components of a simple or complex mixture, whose outputs are the inputs of one machine learning algorithm. Each sensor selectively binds to sample analytes based on electrostatic, hydrophobic, H-bonding or host–guest interactions. Recognition event between the sensor and the analyte is translated into an optical signal through the transduction element. It generates a pattern of outputs (fingerprint) for each analyte. Finally, the patterns are classified (unsupervised approach) and eventually identified (supervised approach) using one machine learning algorithm.20–22 This “Chemical Nose” strategy has been successful applied in “fingerprinting” pure proteins, protein folding states or cancerous cell lines among others, thus allowing their classification and identification.23–27 It also has the potential to be developed for clinical diagnostics. Indeed, since the onset, progression and outcomes of a disease modify the blood composition,28 sensing and fingerprinting blood samples with the set of non-specific sensors would allow samples classification/identification with regard to their physiological or pathological status. Using a set of fluorophore conjugated polymers, Rotello et al. described the classification of healthy, mild or severe liver fibrosis patients, from a cohort of blood samples, with clinically relevant specificity and accuracy ([ROC-AUC] = 0.89).29 A FRET-based polymer sensor array has also been used to discriminate cancerous or healthy mice by serum fingerprinting.30 Recently, as a proof of concept, we demonstrated the use of a set of fluorophore-conjugated cucurbit[7]uril (CB[7]-FL) to discriminate the serum of pregnant women vs. the control.25 Using a hydrophobic cavity with orthogonal H-bonding and electro-static/dipolar interaction utilizing two symmetry-equivalent uridyl carbonyl portals, CB[7] is an interesting receptor scaffold for examining biomolecules. Not only does it possess host–guest binding property toward a wide range of guest molecules but the H-bonding and electro-static/dipolar recognition elements provides an additional target probing mechanism.31–33 Moreover, these CB[7]-FL moieties offer a good compatibility with biological media due to good water solubility and strong fluorescence, easily detected in complex media.
Given the reported blood biochemistry changes in blood samples of SARS-CoV-2 infected patients, we hypothesized that this CB[7]-FL sensor array could be efficient in differentiating serum samples from patients infected or not infected by SARS-CoV-2, with clinically relevant accuracy. Herein, we propose, not only an additional proof-of concept for potential clinical diagnosis but, above all, a step-by step precise methodology to use this CB[7]-FL array-based sensor to monitor and analyse the fluorescence signals and fingerprints obtained from a small set of serum samples of pregnant women infected or not infected by SARS-CoV-2 towards each CB[7]-FL (Scheme 1). This work can be of high interest for researchers and clinicians who are interested in the assessment of hypothesis-free chemical diagnosis for clinical diagnosis with cohorts of large size.
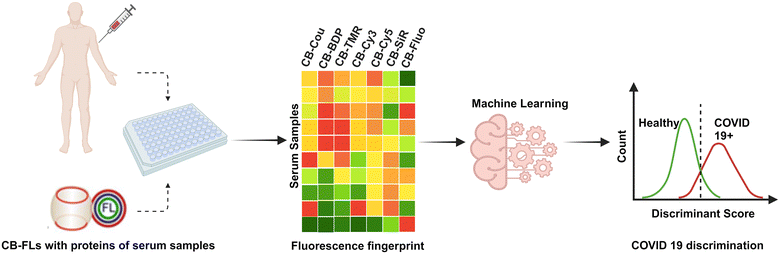 |
| Scheme 1 CB[7]-FL encoded library for fingerprinting serum samples of pregnant women infected or not infected by SARS-CoV-2. Analysis of the obtained serum-based fingerprints via machine learning algorithms to enable the differentiation of disease or healthy states. | |
2. Material and methods
2.1. Participants and samples
Cohorts.
All blood samples were collected in the frame of the COVIPREG study,34 with approval from the national ethics comity, the CPP SUD MEDITERRANEE (No. 2020-A00924-35) on April 23rd, 2020. The trial is recorded in the clinical trial registry as NCT04355234. COVIPREG study is a prospective study conducted in France in 9 maternities of Paris area during the first two waves of COVID-19 pandemic (inclusion between 04/28/2020 and 01/13/2021) and before vaccine availability. All patients have signed a consent form to the use of their serums for research purposes. Serum samples were collected in pregnant women the same day they declared a positive SARS-CoV-2 nasopharyngeal RT-PCR.
Protocol.
After centrifugation of the blood (1000 × g for 15 min at 4 °C), supernatant was transferred into a clean polypropylene tube, aliquoted, frozen within 48 hours and stored at −80 °C until analysis. The control serum samples were used after one year of conservation in accordance with French law.
Subsampling.
Our subsampling of the cohort is composed of 26 samples. The case group is composed of 12 samples whereas the control group is composed of 14 samples, collected from pregnant women in the pre-pandemic period (January 2019). Case vs. control were chosen to be aligned on gestational age. The baseline characteristics of the patients are provided in Table 1 and detailed characteristics for each patient are given in Table S1.†
Table 1 Baseline clinical and pathological characteristics of the infected and control groups
|
Confirmed infected group |
Control group |
n
|
12 |
14 |
Gestational age |
|
|
Mean (std) |
36.5 (4.3) |
37.4 (3.4) |
Median (range) |
38.5 (28–40) |
38 (28–41) |
Days of infection before sampling N (std) |
6.08 (6.05) |
Not applicable
|
Breathing symptoms |
8 yes, 4 no |
Not applicable
|
Hospitalization with oxygen and ICU |
2 yes, 10 no |
Not applicable
|
2.2. CB[7]-FL synthesis
Conjugated cucurbit[7]urils were synthesized as described in previous publications.25,35
2.3. Array methodology
100 nM solution of each CB[7]-FL was freshly prepared in 10 mM sodium phosphate buffer (pH 7.4). The concentration of the stock solution is controlled by Beer–Lambert law before further dilution. In the wells of a white, half-well, non-binding-surface plate (Corning®, Product Number: 3642), 25 μL of the desired CB[7]-FL solution was added. Fluorescence emission at the respective emission wavelength in each well (I(S−)) was recorded on a microplate reader (PerkinELmer®, EnSpire) by exciting the fluorophores at their respective excitation wavelength. Emission and excitation wavelengths for each CB[7]-FL are given in Table S2.† Next, 5 μL of pure serum was added to each fluorophore solutions, except in the control wells where 5 μL of PBS were added instead. Subsequently, after orbital stirring in the microplate reader and temperature control (25 °C), fluorescence emission (I(S+) for sample wells and I(PBS+) for control wells) was recorded using the same excitation/emission wavelengths. Six consecutive measurements (each 5 min apart) were taken. Experiments were performed as replicates of four for each serum sample.
2.4. Data and feature extraction
R codes were written to 1/ read and arrange the raw data in a .csv file; 2/ build the “before and after serum addition” variation of fluorescence tables for each CB[7]-FL and 3/ combine theses tables into one dataset made of 26 samples (12 cases and 14 controls) and 8 variables (the sample status and the 7 CB[7]-FL features with FL = Cy3, Cy5, TMR, SiR, Coum, Fluo, Bdp). These codes can be found in Annex 1 of ESI.† They are named respectively ‘lecture-raw-data.R’, ‘read-plate-data.R’ and ‘build-data.R’.
2.5. Data statistical analysis
The 7 variables for each sample in the two groups were compared and analyzed using different strategies of statistical analysis. Homemade R code (https://cran.r-project.org) was used and can be found in Annex 2 of ESI† (‘Covid _analysis.R’).
3. Results and discussions
The hypothesis-free approach using the CB[7]-FLs sensor array was exploratorily tested to determine whether it could “fingerprint” SARS-Cov2 infection in serum sample and later provide a potentially clinically PoC relevant assay. To this aim, we explored this sensor array-based diagnosis strategy by assessing a precise methodology, including the choice of clinical samples, careful design of experiments, observation and calculation of the fluorescence change, automatized data extraction and organization as well as statistical method comparison for serum samples' discrimination.
3.1. Clinical samples
Serum samples of pregnant women from the COVIPREG cohort34 were used, among which we selected 12 infected by SARS-Cov-2 and 14 healthy controls. For the proof-of-concept of our strategy and since we do not have access to a large number of samples, we selected patients showing good clinical homogeneity in order to reduce inter-individual variability and therefore highlight variations in the blood composition, which could be expected from SARS-Cov2-infection. Therefore, we selected samples from women that were not known to suffer from other diseases and that were in their third trimester of pregnancy (36 ± 4.3 week of gestation (WG) for the infected group and 37.4 ± 4.3 of WG for the control group). Among the SARS-Cov2 infected group, 66.5% (8 over 12) were symptomatic with breathing symptoms and 17% (2 over 12) needed hospitalization. Clinical and biological data of the 26 patients of the two groups are presented in Table 1 (see also Table S1† for individual data).
3.2. Design of experiments
To run the sensing experiment, each of the seven element of the CB[7]-FL sensor array were mixed individually towards each serum sample in the wells of a microplate.
Initially, the complete emission spectra of each CB[7]-FL was recorded on the addition of serum and serum spiked with biologically relevant proteins (1.6 mg mL−1). In some cases, significant fluorescence decrease was observed, with a more pronounced sensitivity of BDP, TMR, Cy5 and SiR. These modifications highlight the ability of CB[7]-FLs to detect subtle changes in complex matrix such as serum (Fig. S1†).
The choice of a half-well microplate has been made to minimize the needed volume of CB[7]-FL as well as serum. Indeed, with seven CB[7]-FLs to be tested, with measurements in quadruplicate, and with 26 sera to assess, the needed volume of each serum is 140 μL and the volume of 100 nM solution of CB-FL was more than 2.5 mL. The concentration of 100 nM has been fixed after preliminary studies and provide sufficient fluorescent signal, even after addition of the serum. For reproducibility concerns, this concentration has to be carefully controlled before experiments.25 Non-binding microplates were preferably used to avoid the adsorption of hydrophobic CB[7] on the well surface. To assess this phenomena, six consecutive measurements (each 5 min apart) were taken. The signal was stable overtime, as shown in Fig. S3.† Therefore, the mean of the six measurements overtime was used for the analysis. To test all the samples in quadruplicate, two microplates were necessary for each CB[7]-FL. To avoid an artificial discrimination due to samples position on the microplate, COVID+ and control samples were positioned on both plates, as shown in Fig. S4.†
3.3. Calculation of fluorescence change
Fluorescence signals of the fluorophore (FL) registered in quadruplicate before and after serum addition constitutes the output, and combining all the FL fluorescence changes later provides the fluorescence pattern for each sample. For each sample and each CB[7]-FL, the output,
, is calculated as the mean over the quadruplicate of the changes in fluorescence at emission wavelength after and before serum addition in each well as given by the formula
where I(S+) is the fluorescence intensity in each well after addition of serum, I(S−) is the fluorescence intensity before addition of serum in the corresponding well, and mean(I(S−)) is the mean of the fluorescence intensities of the quadruplicate corresponding well before addition of serum. The generated data table is given in the ESI† (Table S3).
Obviously, it is impossible to discriminate two groups of samples (COVID+ vs. control) only by looking at the fingerprint on the heatmap plot (Fig. 1a). However, we observe that the mean of CB[7]-Cy5 for each of the two groups are significantly different (p < 0.05) (Fig. 1b). Individual values for each serum in each group are also plotted on Fig. 1c and S5.† These graphs offer a better visualization of the fluorescence variation and particularly differences between the two groups for CB[7]-Cy5. We can also observe the good reproducibility between the four replicates.
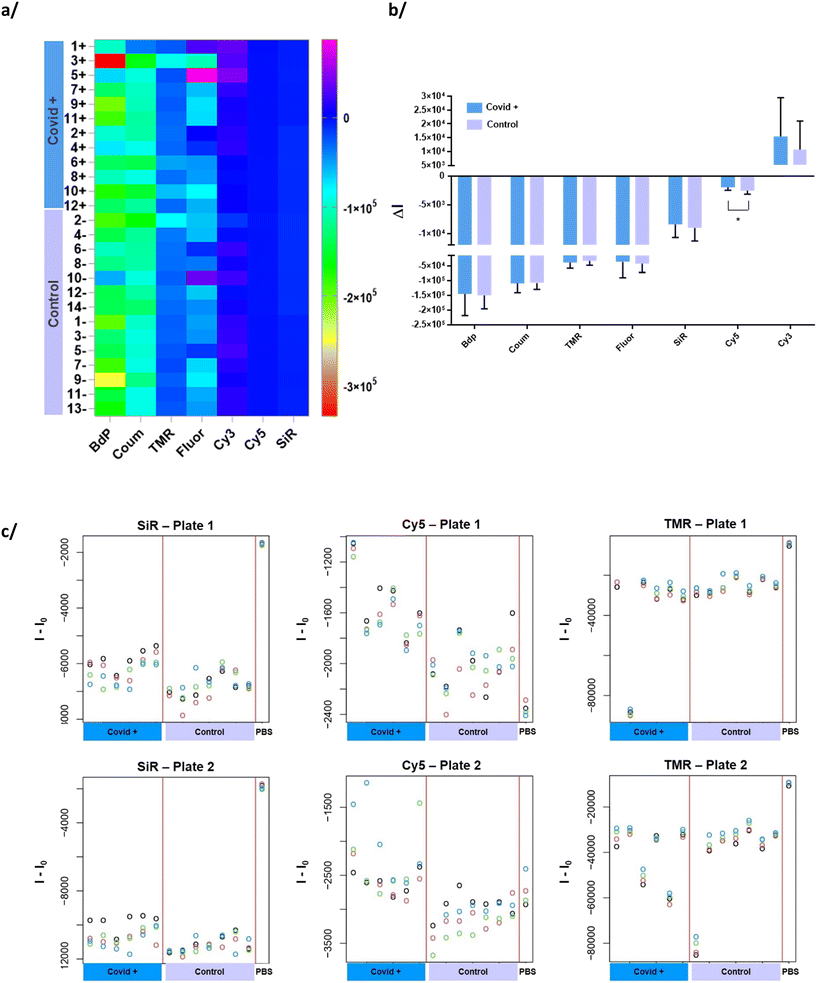 |
| Fig. 1 a/ Heatmap plot fingerprinting each serum sample. b/ Means of fluorescence response of each CB[7]-FL against the two groups COVID+ (blue) and control (light purple). The symbol * indicates p value for the t-test used for comparison of the means smaller than 0.05. c/ Individual ΔI value (y axis) for each replicate (black, red, green and blue circles) of each serum and each CB[7]-FL for the two plates and dispatched such as 1–6: COVID samples, 7–13: control samples, 14: control well. | |
3.4. Statistical method for serum samples discrimination
Based on the data of fluorescence variations for each CB[7]-FL (Table S3†), statistical analysis methods have been evaluated towards the discrimination of the two groups of samples.
3.4.1. Random Forest analysis.
Initially, Random Forest was used. Due to the low number of samples, splitting the data into train and test subset of data was not pertinent.
Using all features (7 CB[7]-FL), the classification rate for the 26 samples (12 COVID+ and 14 COVID−) evaluated using the out-of-the-bag strategy is 84.6%, with a predictive positive value of 74.5% and a negative predictive value of 92.9%. Thanks to the use of Random Forest, we get an estimation of the feature importance in the classification: CB[7]-Cy5 brings the highest contribution, followed by CB[7]-SiR and CB[7]-TMR (Fig. 2a).
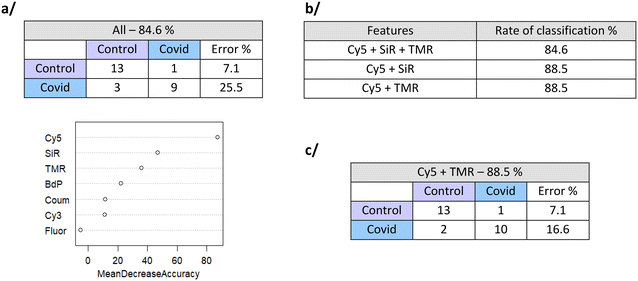 |
| Fig. 2 a/ Confusion matrix for random forest on the whole variables and importance of each feature in the random forest algorithm of discrimination. b/ Error rate of discrimination for random forest run with the most important variables. c/ Confusion matrix for the random forest runs with Cy5 and SiR features. | |
We next used Random Forest with combinations of these 3 most important features. It appears that the couple of features Cy5 and SiR or Cy5 and TMR offers estimated classification rate of 88.5% of error of discrimination and a predictive positive value of 83.4% and a negative predictive value of 92.9% (Fig. 2b and c). Stabilizations of the error rates with the number of trees are shown in Fig. S6.†
As an additional evidence toward the use of this type of non-specific sensor array for clinical sample classification, we also combined this dataset with the previous dataset discriminating pregnant and non-pregnant samples.25 Pregnant samples from these two different data sets are discriminated with 97.1% of discrimination. Then, the samples of pregnant women infected by SARS-COV-2 were tested along with the pregnant and non-pregnant groups. When keeping the most important features (Cy5, Bdp, SiR, TMR, Cy3), only 6.9% of error of discrimination was obtained with a positive predictive value of 75% (Fig. S6 and Table S4†). These results provide additional evidence that the sensor array strategy may be suitable for clinical diagnosis even if it is, of course, still mandatory to have access to big size clinical cohorts.
LDA analysis.
We also used linear discriminant analysis (LDA) model combined with leave-one-out strategy for discrimination rate estimation. The histogram showing the LDA scores distribution within the two groups is given in Fig. S8.† When using all the features, the two groups can be discriminated with 77% accuracy (to compare with the 84.6% of the Random Forest) (Fig. 3a). Again, coefficients of linear discriminant analysis confirmed that Cy5, SiR and TMR have the highest weight in the discrimination. Using these three variables, the accuracy of discrimination is improved to 85% (88.5% for Random Forest) (see Fig. 3b). As for the Random Forest, the predictive positive value is better than the predictive negative value. We carefully looked to the misassigned COVID+ samples and tried to correlate the results to clinical data from Table S1.† However, no correlation was observed whatever the considered clinical data (Fig. 3c).
 |
| Fig. 3 a/ Confusion matrix for LDA runs with the whole variables and b/ runs with the most important variables. c/ Prediction table for individual samples. Misclassified samples are highlighted in red. | |
4. Conclusion
With a small case-control cohort of SARS-CoV-2 infected serum samples, this work highlights the strategy of training a non-specific sensing array to discriminate clinical samples. It offers the proper methodology, including the design of experiments, data extraction, treatment and analysis to run these types of chemical nose experiments and alert the readers to the particular points of attention to take care of when using chemical nose sensor array. From a statistical point-of-view, even if with the small size of our cohort, we demonstrate that a such strategy is efficient to discriminate SARS-CoV-2 infected samples from control samples. By implementing a step-by-step protocol, the methodology can be easily adapted to extract and take into account relevant experimental variables on large cohorts. Linear discriminant analysis clearly shows some lack of performance; however, more machine learning oriented strategy such as Random Forest seems to be well adapted to implement a strategy of training a non-specific sensing array, thanks to its flexibility, the out-of-the-bag error rate estimation, which does not require a train/test approach, and opportunity to retrieve feature (sensor) importance. Despite the higher expected variability in larger cohort, one can anticipate that these properties of the Random Forest will help to keep high discrimination performances. The available R-codes to extract and process from raw data is made available and can be easily tuned to new clinical samples. From a chemical point-of-view, our first results shows the promising efficacy of the macrocyclic-based non-specific CB[7]-FL sensor array to discriminate the two health states between SARS-CoV-2 infected serum and control and that such an approach may constitute a strategy of diagnosis based on blood-serum samples. Interestingly, starting from a fixed size sensor array, using the knowledge regarding importance of each feature could ultimately lead to the reduction of the sensor array dimension, providing a cheaper and easier point-of-care test. From a clinical point-of-view, we can point out that two (over the 3) false negative patients were asymptomatic (see Fig. 3c) and therefore wonder about the result of the SARS-Cov2 PCR test that may be subjected to some error. To conclude, we would like to point out that within a larger and clinically well-documented cohort, the proposed strategy could be used not only to diagnose the clinical binary status but also more complex disease outcomes like severity and eventual complications.
Ethical statement
All experiments were performed in accordance with ethical standards. All blood samples were collected with approval from the national ethics comity CPP SUD MEDITERRANEE (No. 2020-A00924-35) on April 23rd, 2020. The trial is recorded in the clinical trial registry as NCT04355234. Informed consents were obtained from human participants of this study.
Sample CRediT author statement
Nathalie Gagey-Eilstein: conceptualization, methodology, supervision, writing – original draft preparation, funding acquisition. Yves Rozenholc: data curation, formal analysis, software, supervision. Sarit S. Agasti: resources. Christelle Vauloup-Fellous, Vassilis Tsatsaris: resources, funding acquisition. Monica Swetha Bosco, Ariadne Sossah, Soumen Pradhan: investigation. Zeki Topcu: investigation, data curation, formal analysis.
Author contributions
N. G. E. and Y. R. contributed equally to the work.
Conflicts of interest
There are no conflicts to declare.
Acknowledgements
This research has received funding from the FHU Prema foundation to N. G. E., V. T. and C. V. F. and from the Agence Nationale pour la Recherche (ANR-20-CE17-0035) to N. G. E., Y. R. and V. T. SERB support (CVD/2020/000855) to S. S. A. is also acknowledged. COVIPREG study: sponsor was Assistance Publique – Hôpitaux de Paris (Délégation à la Recherche Clinique et a l'Innovation).
References
- Coronavirus, https://www.who.int/health-topics/coronavirus, (accessed 2023-09-19).
- R. W. Peeling, D. L. Heymann, Y.-Y. Teo and P. J. Garcia, Diagnostics for COVID-19: Moving from Pandemic Response to Control, Lancet, 2022, 399(10326), 757–768, DOI:10.1016/S0140-6736(21)02346-1
.
- D. Ferrari, A. Motta, M. Strollo, G. Banfi and M. Locatelli, Routine Blood Tests as a Potential Diagnostic Tool for COVID-19, Clin. Chem. Lab. Med., 2020, 58(7), 1095–1099, DOI:10.1515/cclm-2020-0398
.
- R. F. Thudium, M. P. Stoico, E. Høgdall, J. Høgh, H. B. Krarup, M. A. H. Larsen, P. H. Madsen, S. D. Nielsen, S. R. Ostrowski, A. Palombini, D. B. Rasmussen and N. T. Foged, Early Laboratory Diagnosis of COVID-19 by Antigen Detection in Blood Samples of the SARS-CoV-2 Nucleocapsid Protein, J. Clin. Microbiol., 2021, 59(10), e01001-21, DOI:10.1128/JCM.01001-21
.
- A. Townsend, P. Rijal, J. Xiao, T. K. Tan, K.-Y. A. Huang, L. Schimanski, J. Huo, N. Gupta, R. Rahikainen, P. C. Matthews, D. Crook, S. Hoosdally, S. Dunachie, E. Barnes, T. Street, C. P. Conlon, J. Frater, C. V. Arancibia-Cárcamo, J. Rudkin, N. Stoesser, F. Karpe, M. Neville, R. Ploeg, M. Oliveira, D. J. Roberts, A. A. Lamikanra, H. P. Tsang, A. Bown, R. Vipond, A. J. Mentzer, J. C. Knight, A. J. Kwok, G. R. Screaton, J. Mongkolsapaya, W. Dejnirattisai, P. Supasa, P. Klenerman, C. Dold, J. K. Baillie, S. C. Moore, P. J. M. Openshaw, M. G. Semple, L. C. W. Turtle, M. Ainsworth, A. Allcock, S. Beer, S. Bibi, D. Skelly, L. Stafford, K. Jeffrey, D. O'Donnell, E. Clutterbuck, A. Espinosa, M. Mendoza, D. Georgiou, T. Lockett, J. Martinez, E. Perez, V. Gallardo Sanchez, G. Scozzafava, A. Sobrinodiaz, H. Thraves and E. Joly, A Haemagglutination Test for Rapid Detection of Antibodies to SARS-CoV-2, Nat. Commun., 2021, 12(1), 1951, DOI:10.1038/s41467-021-22045-y
.
- S. S. Adigal, N. V. Rayaroth, R. V. John, K. M. Pai, S. Bhandari, A. K. Mohapatra, J. Lukose, A. Patil, A. Bankapur and S. Chidangil, A Review on Human Body Fluids for the Diagnosis of Viral Infections: Scope for Rapid Detection of COVID-19, Expert Rev. Mol. Diagn., 2021, 21(1), 31–42, DOI:10.1080/14737159.2021.1874355
.
- W. Wang, Y. Xu, R. Gao, R. Lu, K. Han, G. Wu and W. Tan, Detection of SARS-CoV-2 in Different Types of Clinical Specimens, JAMA, J. Am. Med. Assoc., 2020, 323(18), 1843–1844, DOI:10.1001/jama.2020.3786
.
- R. Samson, G. R. Navale and M. S. Dharne, Biosensors: Frontiers in Rapid Detection of COVID-19, 3 Biotech, 2020, 10(9), 385, DOI:10.1007/s13205-020-02369-0
.
- P. Pokhrel, C. Hu and H. Mao, Detecting the Coronavirus (COVID-19), ACS Sens., 2020, 5(8), 2283–2296, DOI:10.1021/acssensors.0c01153
.
- G. Giovannini, H. Haick and D. Garoli, Detecting COVID-19 from Breath: A Game Changer for a Big Challenge, ACS Sens., 2021, 6(4), 1408–1417, DOI:10.1021/acssensors.1c00312
.
- B. Shan, Y. Y. Broza, W. Li, Y. Wang, S. Wu, Z. Liu, J. Wang, S. Gui, L. Wang, Z. Zhang, W. Liu, S. Zhou, W. Jin, Q. Zhang, D. Hu, L. Lin, Q. Zhang, W. Li, J. Wang, H. Liu, Y. Pan and H. Haick, Multiplexed Nanomaterial-Based Sensor Array for Detection of COVID-19 in Exhaled Breath, ACS Nano, 2020, 14(9), 12125–12132, DOI:10.1021/acsnano.0c05657
.
- T. Kilic, R. Weissleder and H. Lee, Molecular and Immunological Diagnostic Tests of COVID-19: Current Status and Challenges, iScience, 2020, 23(8), 101406, DOI:10.1016/j.isci.2020.101406
.
- R. Mittal, N. Chourasia, V. K. Bharti, S. Singh, P. Sarkar, A. Agrawal, A. Ghosh, R. Pal, J. R. Kanwar and A. Kotnis, Blood-Based Biomarkers for Diagnosis, Prognosis, and Severity Prediction of COVID-19: Opportunities and Challenges, J. Family Med. Prim. Care, 2022, 11(8), 4330–4341, DOI:10.4103/jfmpc.jfmpc_2283_21
.
- COvid-19 Multi-omics Blood ATlas (COMBAT) Consortium, A Blood Atlas of COVID-19 Defines Hallmarks of Disease Severity and Specificity, Cell, 2022, 185(5), 916–938, DOI:10.1016/j.cell.2022.01.012
.
- J. Park, H. Kim, S. Y. Kim, Y. Kim, J.-S. Lee, K. Dan, M.-W. Seong and D. Han, In-Depth Blood Proteome Profiling Analysis Revealed Distinct Functional Characteristics of Plasma Proteins between Severe and Non-Severe COVID-19 Patients, Sci. Rep., 2020, 10(1), 22418, DOI:10.1038/s41598-020-80120-8
.
- A. B. Palmos, V. Millischer, D. K. Menon, T. R. Nicholson, L. S. Taams, B. Michael, G. Sunderland, M. J. Griffiths, COVID Clinical Neuroscience Study Consortium, C. Hübel and G. Breen, Proteome-Wide Mendelian Randomization Identifies Causal Links between Blood Proteins and Severe COVID-19, PLoS Genet., 2022, 18(3), e1010042, DOI:10.1371/journal.pgen.1010042
.
- B. Shen, X. Yi, Y. Sun, X. Bi, J. Du, C. Zhang, S. Quan, F. Zhang, R. Sun, L. Qian, W. Ge, W. Liu, S. Liang, H. Chen, Y. Zhang, J. Li, J. Xu, Z. He, B. Chen, J. Wang, H. Yan, Y. Zheng, D. Wang, J. Zhu, Z. Kong, Z. Kang, X. Liang, X. Ding, G. Ruan, N. Xiang, X. Cai, H. Gao, L. Li, S. Li, Q. Xiao, T. Lu, Y. Zhu, H. Liu, H. Chen and T. Guo, Proteomic and Metabolomic Characterization of COVID-19 Patient Sera, Cell, 2020, 182(1), 59–72.e15, DOI:10.1016/j.cell.2020.05.032
.
- Y.-M. Chen, Y. Zheng, Y. Yu, Y. Wang, Q. Huang, F. Qian, L. Sun, Z.-G. Song, Z. Chen, J. Feng, Y. An, J. Yang, Z. Su, S. Sun, F. Dai, Q. Chen, Q. Lu, P. Li, Y. Ling, Z. Yang, H. Tang, L. Shi, L. Jin, E. C. Holmes, C. Ding, T.-Y. Zhu and Y.-Z. Zhang, Blood Molecular Markers Associated with COVID-19 Immunopathology and Multi-Organ Damage, EMBO J., 2020, 39(24), e105896, DOI:10.15252/embj.2020105896
.
- M. Kukar, G. Gunčar, T. Vovko, S. Podnar, P. Černelč, M. Brvar, M. Zalaznik, M. Notar, S. Moškon and M. Notar, COVID-19 Diagnosis by Routine Blood Tests Using Machine Learning, Sci. Rep., 2021, 11(1), 10738, DOI:10.1038/s41598-021-90265-9
.
- J. J. Lavigne and E. V. Anslyn, Sensing A Paradigm Shift in the Field of Molecular Recognition: From Selective to Differential Receptors, Angew. Chem., Int. Ed., 2001, 40(17), 3118–3130, DOI:10.1002/1521-3773(20010903)40:17<3118::AID-ANIE3118>3.0.CO;2-Y
.
- Y. Geng, W. J. Peveler and V. Rotello, Array-based “Chemical Nose” Sensing in Diagnostics and Drug Discovery, Angew. Chem., Int. Ed., 2019, 58(16), 5190–5200, DOI:10.1002/anie.201809607
.
- K. J. Albert, N. S. Lewis, C. L. Schauer, G. A. Sotzing, S. E. Stitzel, T. P. Vaid and D. R. Walt, Cross-Reactive Chemical Sensor Arrays, Chem. Rev., 2000, 100, 2595–2626, DOI:10.1021/cr980102w
.
- O. R. Miranda, C.-C. You, R. Phillips, I.-B. Kim, P. S. Ghosh, U. H. F. Bunz and V. M. Rotello, Array-Based Sensing of Proteins Using Conjugated Polymers, J. Am. Chem. Soc., 2007, 129(32), 9856–9857, DOI:10.1021/ja0737927
.
- M. De, S. Rana, H. Akpinar, O. R. Miranda, R. R. Arvizo, U. H. F. Bunz and V. M. Rotello, Sensing of Proteins in Human Serum Using Conjugates of Nanoparticles and Green Fluorescent Protein, Nat. Chem., 2009, 1(6), 461–465, DOI:10.1038/nchem.334
.
- N. Das Saha, S. Pradhan, R. Sasmal, A. Sarkar, C. M. Berač, J. C. Kölsch, M. Pahwa, S. Show, Y. Rozenholc, Z. Topçu, V. Alessandrini, J. Guibourdenche, V. Tsatsaris, N. Gagey-Eilstein and S. S. Agasti, Cucurbit[7]Uril Macrocyclic Sensors for Optical Fingerprinting: Predicting Protein Structural Changes to Identifying Disease-Specific Amyloid Assemblies, J. Am. Chem. Soc., 2022, 144(31), 14363–14379, DOI:10.1021/jacs.2c05969
.
- A. Bajaj, O. R. Miranda, I.-B. Kim, R. L. Phillips, D. J. Jerry, U. H. F. Bunz and V. M. Rotello, Detection and Differentiation of Normal, Cancerous, and Metastatic Cells Using Nanoparticle-Polymer Sensor Arrays, Proc. Natl. Acad. Sci. U. S. A., 2009, 106(27), 10912–10916, DOI:10.1073/pnas.0900975106
.
- N. Das Saha, R. Sasmal, S. K. Meethal, S. Vats, P. V. Gopinathan, O. Jash, R. Manjithaya, N. Gagey-Eilstein and S. S. Agasti, Multichannel DNA Sensor Array Fingerprints Cell States and Identifies Pharmacological Effectors of Catabolic Processes, ACS Sens., 2019, 4(12), 3124–3132, DOI:10.1021/acssensors.9b01009
.
- L. M. Killingsworth and J. C. Daniels, Plasma Protein Patterns in Health and Disease, Crit. Rev. Clin. Lab. Sci., 1979, 11(1), 1–30, DOI:10.3109/10408367909105852
.
- W. J. Peveler, R. F. Landis, M. Yazdani, J. W. Day, R. Modi, C. J. Carmalt, W. M. Rosenberg and V. M. Rotello, A Rapid and Robust Diagnostic for Liver Fibrosis Using a Multichannel Polymer Sensor Array, Adv. Mater., 2018, 30(28), 1800634, DOI:10.1002/adma.201800634
.
- N. D. B. Le, A. K. Singla, Y. Geng, J. Han, K. Seehafer, G. Prakash, D. F. Moyano, C. M. Downey, M. J. Monument, D. Itani, U. H. F. Bunz, F. R. Jirik and V. M. Rotello, Simple and Robust Polymer-Based Sensor for Rapid Cancer Detection Using Serum, Chem. Commun., 2019, 55(76), 11458–11461, 10.1039/C9CC04854E
.
- K. Kim, N. Selvapalam, Y. H. Ko, K. M. Park, D. Kim and J. Kim, Functionalized Cucurbiturils and Their Applications, Chem. Soc. Rev., 2007, 36(2), 267–279, 10.1039/B603088M
.
- A. R. Urbach and V. Ramalingam, Molecular Recognition of Amino Acids, Peptides, and Proteins by Cucurbit[n]Uril Receptors, Isr. J. Chem., 2011, 51(5–6), 664–678, DOI:10.1002/ijch.201100035
.
- J. W. Lee, M. H. Shin, W. Mobley, A. R. Urbach and H. I. Kim, Supramolecular Enhancement of Protein Analysis via the Recognition of Phenylalanine with Cucurbit[7]Uril, J. Am. Chem. Soc., 2015, 137(48), 15322–15329, DOI:10.1021/jacs.5b10648
.
- O. Picone, A. J. Vivanti, J. Sibiude, A.-G. Cordier, V. Alessandrini, G. Kayem, C. Borie, D. Luton, P. Manchon, C. Couffignal, C. Vauloup Fellous and COVIPREG study group, SARS-COV-2 Excretion and Maternal-Fetal Transmission: Virological Data of French Prospective Multi-Center Cohort Study COVIPREG during the First Wave, J. Gynecol. Obstet. Hum. Reprod., 2023, 52(4), 102547, DOI:10.1016/j.jogoh.2023.102547
.
- A. Som, M. Pahwa, S. Bawari, N. D. Saha, R. Sasmal, M. S. Bosco, J. Mondal and S. S. Agasti, Multiplexed Optical Barcoding of Cells via Photochemical Programming of Bioorthogonal Host–Guest Recognition, Chem. Sci., 2021, 12(15), 5484–5494, 10.1039/D0SC06860H
.
|
This journal is © The Royal Society of Chemistry 2024 |
Click here to see how this site uses Cookies. View our privacy policy here.