DOI:
10.1039/D4SD00056K
(Paper)
Sens. Diagn., 2024,
3, 839-849
Improved point-of-care detection of P. gingivalis using optimized surface-enhanced Raman scattering in lateral flow assays†
Received
19th February 2024
, Accepted 26th March 2024
First published on 28th March 2024
Abstract
The introduction of surface-enhanced Raman scattering (SERS) in lateral flow assays (LFA) has been recently exploited to increase the sensitivity and quantification capabilities of these rapid tests. Herein, we took advantage of a SERS–LFA combination to improve LFAs designed to test for P. gingivalis, a biomarker for oral health. We have demonstrated a limit of detection (LOD) < 10 ng mL−1, which is within the range of concentration needed to monitor oral health. By comparison, conventional colorimetric LFAs achieve an LOD ≈ 100 ng mL−1, generating a lower diagnostic sensitivity. To achieve this enhanced sensitivity, we optimized the materials used in the SERS–LFA, investigating nanostars (NS) differing in size and material composition and comparing them to commercial gold nanoparticles, as a benchmark. We found that large (56 nm) NSs with a silver coating were the most sensitive nanomaterials for SERS–LFA. To prove the applicability of this SERS–LFA to point-of-care (POC) settings, we tested the optimized LFA with a portable Raman system prototype designed to work on LFAs with 3D-printed cartridges and a line-shaped laser illumination. Using this prototype, we achieved the same LOD observed with the traditional benchtop Raman system. The use of a portable Raman system has brought the SERS–LFA technology closer to the POC use.
Introduction
Lateral flow assays (LFA) have become ubiquitous for the rapid point-of-care diagnosis of multiple conditions ranging from pregnancy to infections to high stress levels, while utilizing a variety of biofluids (saliva, urine, sweat, etc.).1–5 Conventional colorimetric LFA diagnoses, however, are only possible with relatively high biomarker concentrations.6 This issue was well-documented during the SARS-CoV-2 pandemic when LFA tests were not able to detect pre-symptomatic infections, due to lower viral loads.7 Conversely, sensitive diagnostic methods, such as ELISA or PCR analysis, require sample collection and off-site analysis in specialized labs, resulting in a time lapse between sampling and results. There is a demand for developing testing methods that have sensitivity comparable to lab-based molecular tests while executed at the point-of-care sites. Without filling this technological gap, our surveillance and diagnostic infrastructure will remain vulnerable to the spread of infectious diseases.
To this end, the standard colorimetric LFA protocol has been expanded by modifying its sensing mechanism and/or readout method. Expansion of the standard LFA protocol has produced a number of techniques, including target amplification, catalytic colorimetric response, thermal response, use of smart phone image detection and processing8 and surface-enhanced Raman scattering (SERS) coupled LFAs.9–12 An LFA with non-covalent DNA catalytic amplification has been demonstrated for detection of SARS-CoV-2 with picomolar detection limits.1 These devices, dubbed PLAN-LFA, employ a padlock rolling circle amplification to increase sensitivity 1000 times, however the assays are still not rapid enough for in-office diagnosis (three hours). A catalytic colorimetric response was developed by replacing conventional gold nanoparticles (AuNP) with palladium nanoparticles (Pd NPs), which function as a catalyst for color-changing reporter dye. The presence of a catalyst amplifies the signal observed for each NP without adding significant processing time (incubation <20 min).12 Thermal contrast has been used to improve sensitivity eight-fold over standard optical detection.10 In this application, an IR camera measures the temperature at the test and control lines under laser irradiation, due to the strong plasmonic photothermal effect generated by NPs. This strategy improves the sensitivity by changing the readout method without modifying the LFA. While these methods are powerful ways of increasing the sensitivity of LFA, they require complicated protocols and long testing time under the controlled environment, resulting in a low accessibility for users.
The SERS-based LFA (SERS–LFA) diagnostic method can exploit the advantages given by the vibrational nature of spectroscopic readout, which permits for molecular-level sensitivity, highly multiplexed analysis and easy signal extraction from broad backgrounds. SERS can be a user-friendly method because it simply adds the Raman reporter on metal nanoparticles used for conventional LFA, while using the same testing procedure. After the first demonstration13 of LFA using SERS detection in 2017, SERS–LFAs have been explored extensively and summarized well in the literature.11,14–27 These researches have demonstrated increased sensitivity and multiplexed analysis enabled by the combination of LFA with a SERS readout.28,29 Studies have initially incorporated a SERS active Raman reporter onto standard AuNP19,21,22 and introduced different nanomaterials (e.g. nanostar) to further increase the sensitivity with various properties.16–18,20,23–27 Hwang et al. demonstrated the first SERS–LFA biosensor that presented three order of magnitude higher sensitivity than the corresponding ELISA-based method.13 The capability of simultaneous multiplexing detection using SERS–LFA were also demonstrated for detection of dual nucleic acids30 and two different diseases such as Zika and Dengue.31 Instead of spherical AuNP, gold nanostars with a branched structure were utilized to detect bisphenol A using SERS–LFA, presenting 20 times higher sensitivity than that of colorimetric quantification.26 However, only a few reports have compared the performance of different nanomaterials to optimize the LFA itself.26 To systematically improve the capabilities of LFAs, side-by-side comparison and associated optimization are necessary. More recently, to improve user accessibility, portable Raman systems have been demonstrated for measurement of SERS–LFAs.28,32,33 This affordable and easy-to-use portable system enables SERS–LFAs to be used for POC applications.
In this work, we focus on Porphyromonas gingivalis endotoxin, a major saliva biomarker for oral health. Endotoxin released from a Gram-negative pathogenic P. gingivalis is causing chronic inflammation in the gum tissue and leading to tooth loss if not treated in time. In addition, some important cardiovascular and neurodegenerative diseases recently revealed a very close relationship to P. gingivalis concentration in oral cavity.34,35 Therefore, it becomes more important to monitor the PG LPS level for oral health as well as whole body health using a POC monitoring capability. Previous work has reported the development of P. gingivalis colorimetric LFA detecting lipopolysaccharides (LPS) produced by the bacteria with a limit of detection (LOD) of ∼22 ng mL−1 and a dynamic range up to ∼10–20 μg mL−1.3 To utilize the LFA as a diagnostic test for early onset of oral diseases it is important to reduce the LOD below 10 ng mL−1. Clinically, the average LPS concentrations of healthy individuals and periodontitis patients are approximately 10 ng mL−1 and 31 ng mL−1, respectively.36 Here we report on the combination of this LFA for P. gingivalis with a SERS readout with the LOD < 10 ng mL−1. We explored five different metallic nanomaterials to determine the optimal nanomaterial for incorporation into SERS–LFAs: gold spheres/nanoparticles (AuNP), two sizes each of gold nanostars (AuNS) and silver-coated gold nanostars (Ag@AuNS). The Ag@AuNS has been shown to have superior single-particle signal enhancement,37 although this property has not been tested in LFA systems yet. This work represents the first analytical comparison of more than two materials for optimization of SERS–LFAs. The different nanomaterials were first characterized and functionalized with a Raman reporter and the SERS enhancement of each nanomaterial was evaluated when collected at an LFA control line formed without the target PG LPS. Then, using the optimized nanomaterial, the SERS–LFA was calibrated for the detection of LPS produced by P. gingivalis and the sensitivity was compared to that of a colorimetric LFA. Finally, the SERS–LFAs were tested with a portable Raman system, demonstrating the potential for POC applications.
Experimental
Materials
Hydrochloric acid (1 M), chloroauric acid solution (HAuCl4, 5.08 mM), ammonium hydroxide (NH4OH), 1,1′,3,3,3′,3′-hexamethylindotricarbocyanine iodide (HITC), Tween 20 (10% w/v), Triton™ X-100, L-ascorbic acid, anti-P. gingivalis LPS monoclonal antibody, anti-P. gingivalis polyclonal antibody, bovine serum albumin (BSA), and cellulose fiber pad (CFSP001700) were purchased from Sigma-Aldrich (St. Louis. MO). Silver nitrate (AgNO3), sodium chloride, sodium phosphate tribasic dodecahydrate (Na3PO4·12H2O, 98%, analysis grade), sucrose, Tris-HCl (1 M, pH 8), Tris-HCl (1 M, pH 7.4), phosphate buffered saline (PBS, 10×, pH 7.4), trisodium citrate dihydrate, and 4-mercaptobenzoic acid were purchased from Fisher Scientific (Waltham, MA). Thiolated polyethylene glycol (HS-PEG, MW ≈ 5000
k) was purchased from Nanocs (New York, NY). The standard 40 nm gold nanoparticle solution (10 OD) was purchased from Cytodiagnostics (Burlington, ON). All ultrapure P. gingivalis and E. coil LPS were purchased from InvivoGen (San Diego, CA). Glass fiber pad (8950) and nitrocellulose membrane (CN140 and CN95) were obtained from Ahlstrom (Helsinki, Finland) and Sartorius (Goettingen, Germany), respectively. The backing card in a size of 60 (W) × 300 (L) mm was purchased from DCN Dx (Carlsbad, CA). All materials were used as received and solutions were prepared using Millipore Synergy UV-R Ultrapure water (18.2 MΩ cm).
Preparation of gold nanostars and silver-coated nanostars
Gold seeds were synthesized by the Turkevich method (approx. 12 nm).38 AuNS and Ag@AuNS were prepared according to previously published protocols.39 For large nanostars, 2.6 μmoles of HAuCl4 and 100 μL of Au seed solution were added to 10 mL of 1 mM HCl with vigorous stirring. To form nanostars, AgNO3 (0.125 mmoles) and L-ascorbic acid (5 mmoles) were added to the reaction flask in rapid succession, resulting in a color change from pale orange to deep blue. To make Ag@AuNS, 5 mmoles AgNO3 and 10 μL NH4OH were added in rapid succession to the blue nanostar solution and the flask was stirred until the solution was red-brown and the color was stable. Particle solutions were stored at 4 °C until use.
To produce smaller NS, the same procedure was followed with the volume of Au seed solution increased to 200 μL. All other additions were constant.
Functionalization of nanoparticles with Raman reporter and antibody
A 1 mL aliquot of the nanomaterial (i.e., AuNP, AuNS, or Ag@AuNS) was combined with 100 μL of 5 μM HITC and the mixture was incubated for 3 hours at RT. After incubation, Tween 20 was added to achieve a final surfactant concentration of 0.01% (v/v). The samples were well mixed and centrifuged at 4000 g for 10 min to separate the particles from excess Raman reporter in solution. The supernatant was removed and the particles were redispersed in 0.01× PBS buffer. After conjugating the Raman reporter, 5 μL of PG LPS monoclonal antibody (1 mg mL−1) was added to 200 μL of nanomaterial solutions. Then 195 μL of 2 mM Tris-HCl pH 8.0 with 0.05% of Tween-20 surfactant was also added. The mixed solutions were incubated overnight in the refrigerator. After incubation, the solutions were centrifuged at 1300g for 20 min, followed by the removal of the supernatant. This washing process was repeated 4 times. After the 4th washing step, the concentrations of nanomaterials were adjusted to 5 OD using the buffer made of 2 mM Tris-HCl pH 8.0, 0.05% of Tween-20, and 0.5% of BSA.
Preparation of LFA strips
Our LFA strip has 4 components – cellulose sample pad, fiberglass blocking pad, nitrocellulose (NC) membrane, and cellulose wicking pad. No “conjugation” pad was present because AuNP/AuNS are not loaded on the pad. Blocking agents were loaded to the (blocking) pad to block the nitrocellulose membrane.
First, the NC membrane (30 mm by 300 mm) and wicking pad (17 mm by 300 mm) are fixed on the adhesive backing card, and then cut into 5-mm wide strips using the Biodot CM4000 automatic guillotine cutter (Biodot, Irvine, CA). Next, P. gingivalis polyclonal antibody for the test line and protein G for the control line are printed using the Biodot AD1500 printing system (Biodot, Irvine, CA). Both concentrations were optimized at 1 mg mL−1. Printed strips were incubated in an oven at 50 °C for 10 min and stored in a nitrogen-purging box.
Both sample and blocking pad were precut into 13 mm × 5 mm and 10 mm × 5 mm, respectively, and loaded with multiple functional chemicals to provide essential functions (e.g., easy uniform flow, preventing non-specific binding, etc.) during LFA test. Sample pads were immersed into the buffer solution dissolved with 2.5 mL of Tris-HCl 1 M pH 8 buffer, 2.5 μL of NaCl 3 M solution, and 125 μL of Triton X-100 into 50 mL of ultrapure water. Blocking pads were immersed into blocking buffer solution prepared by sequentially dissolving 250 mg of BSA, 500 mg of sucrose, 38.1 mg of Na3PO4 tribasic salt, and 12.5 μL of Tween-20 surfactant into 5 mL of ultrapure water. Both pads were immersed into the solution for 40 min, dried in the oven at 60 °C for 90 min, and stored in a nitrogen purging box overnight. To complete the fabrication, pretreated sample and blocking pads were placed on the backing card pre-assembled with the NC membrane and wicking pad before LFA tests. The LFA testing process was performed as described in our previous report.3 In brief, the sample solution made of 30 μL of functionalized nanoparticles, 10 μL of 3-M NaCl, 10 μL of PG LPS and 40 μL of Tris-HCl (pH 8) buffer with 0.05% Tween-20 was dispensed on LFA devices after 40 min incubation.
Nanoparticle tracking analysis
Nanoparticle tracking analysis was performed with a Nanosight NS300 (Malvern Panalytical, Worcestershire, UK) using Nanosight NTA software. Five replicate measurements of each sample were collected for 30 seconds each.
Transmission electron microscopy
Before TEM imaging, 10 μL of the nanomaterial solution was dispensed onto a 300-mesh grid and dried overnight. A transmission electron microscope (TEM) Talos F200i (Thermofisher) at 200 kV was used to acquire the micrographs. This instrument was used at the University of Cincinnati's Advanced Material Characterization Center (AMCC).
UV-vis spectroscopy
UV-vis absorbance spectra were collected in a 96 well plate with a Synergy HTX multi-mode plate reader (BioTek, Winooski, VT) and Gen5 software (v3.10). Measurements were collected from 300–800 nm and blank subtracted in the native software.
SERS measurements with benchtop Raman
SERS measurements were collected on a lab-built Raman setup composed of a Wasatch Photonics WP785 spectrometer, an OptoEngine 785 nm laser source (FC-D-785-300 mW) and Raman fiberoptic probe (RamanProbe by InPhotonics). Laser power was set to 74 mW for the measurements. The fiberoptic probe was connected to a 2D automated stage (Zaber). The stage was used to collect spectra on the LFA. Spectrum collection for material optimization was performed first as a single line-scan along the sample flow direction in the LFA (40 points 1 mm step-size) to identify the location of the control line. Then 3 parallel scans (of 10 points each) were performed along the control line to maximize SERS spectral information.
For the LFA operation with the targeted analyte, detecting spectra were acquired along the LFA in 6 parallel line-scans (40 points 1 mm step-size) separated by 1 mm. The line scans were averaged in the displayed results. Each measurement had 100 ms integration time for 10 accumulations. Fig. S1† shows a photo of the system and a depiction of the sampling on the LFA.
SERS measurements with portable Raman
To prove the POC capability of our test, SERS measurements were also collected on a portable Raman system. The system is a WP-785XS prototype provided by Wasatch Photonics. This system is composed of a 785 nm laser of 8 mW in power, holographic grating and an ambient CMOS detector. This portable system has a size of roughly 55 × 85 × 30 mm. The excitation/collection optics are designed to focus on a 3.5-mm line (0.5 mm thick), which is ideal for reading SERS signal from LFA test lines. The system was integrated with custom 3D-printed cartridge and sample holder. The cartridge permitted to enclose the LFA and the sample holder kept the cartridge/LFA at the focal distance. Photos of system, cartridge and sample-holder are shown later in the results section (Fig. 5). The laser spot for the benchtop Raman system (∼1 mm) has a power density of 19 W cm−2, compared to the line of the portable system with a power density of 4 W cm−2. The focal distances for the two systems are 5 and 21 mm for the benchtop and portable, respectively.
Image and data analysis
LFA colorimetric results were analyzed using ImageJ (V2.1.0/1.53c).40 Fig. S2† describes the image analysis process. Raman data were processed using scripts running in MatLab (R2021b) (The Mathworks, Inc., Natick, MA). A lab-built script with data smoothing using a Savitzky–Golay frequency filter and partial least squares baseline subtraction was used prior to data analysis. The SERS response was determined from the normalized peak intensity for the characteristic HITC band at 546
cm−1. Data visualization was executed in IGOR Pro (v8.0.4, Wavemetrics, Inc., Lake Oswego, OR).
Results and discussion
Conventional operation LFAs rely on visual detection of the test line generated by collected AuNP. To improve the performance of the LFA, SERS active reporter molecules can be incorporated on the metal surface. It has been reported that the surface plasmon of nanomaterials with higher anisotropy produces stronger SERS response than that of spherical AuNP.41 However, this effect has not been carefully studied in LFA strips. Therefore, we have synthesized AuNS and Ag@AuNS in different sizes for comparison to commercially available AuNP. Fig. 1 shows a graphical depiction of the LFA operation and the optimization of SERS reporter conjugation on nanomaterials.
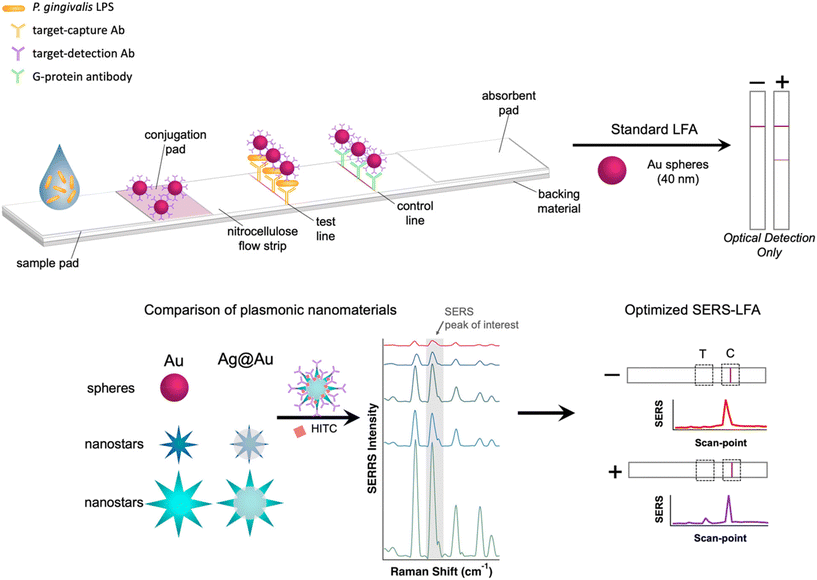 |
| Fig. 1 Depiction of the research workflow. LFA for P. gingivalis LPS were modified and optimized for SERS readout using several nanomaterials: (top) conventional LFA configuration and sensing mechanism, (bottom) comparison of different plasmonic nanomaterials for SERS and its application to LFA device. | |
Characterization of plasmonic nanomaterials
To understand the structural and plasmonic properties of the nanomaterials tested, each material was analyzed by UV-vis, NTA, and TEM. All characterization data are shown in Fig. 2. From the TEM images, the morphology and size of each material were determined. The TEM images show that the commercial AuNP are spherical and the average diameter was 37.3 ± 11.2 nm. The AuNS display the highly branched structure, with average particle diameters (tip-to-tip) of 27.0 ± 6.8 and 39.9 ± 9.3 nm for the small and large AuNS, respectively. Finally, the Ag@AuNS appears somewhat rounded, although contrast shows the branched Au structure is intact beneath the Ag shell; these particles had average diameters of 38.6 ± 10.3 and 55.5 ± 8.9 nm, for the small and large Ag@AuNS, respectively. Hereafter, these AuNS will be referred to as 27 and 40 nm and their Ag-coated analogs will be referred to as 39 or 56 nm. From the TEM size analysis, the size distributions of all lab-prepared NS were comparable to the commercial AuNP, indicating control over the synthesis and a product of particles with uniform size.
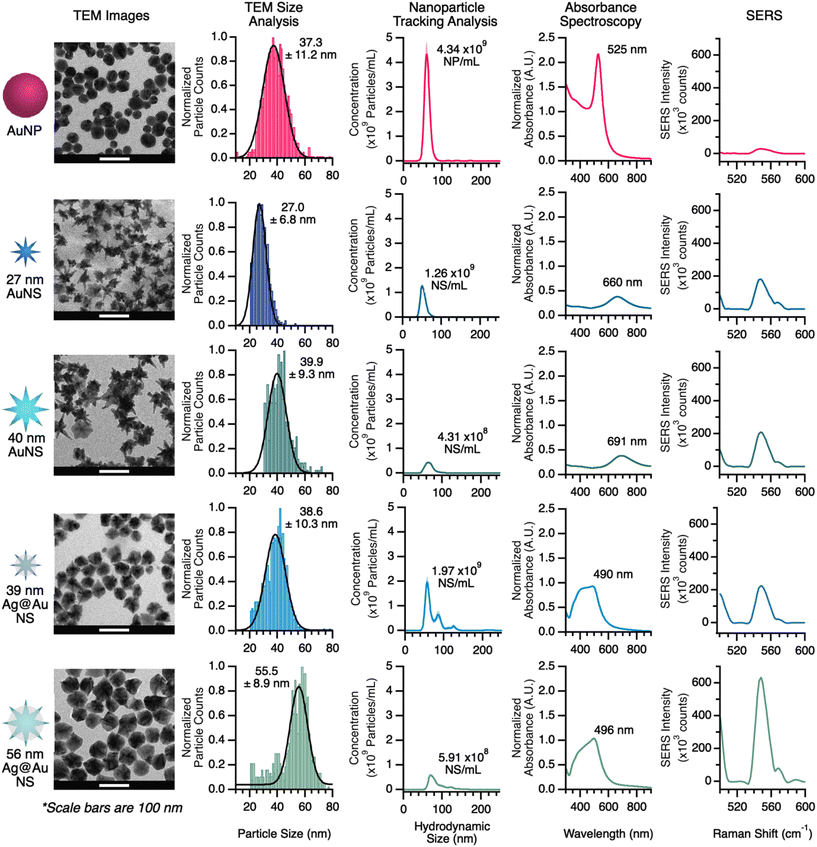 |
| Fig. 2 Nanoparticle characterization. (left-to-right) TEM images, nanoparticle tracking analysis (NTA), absorbance spectra and SERS spectra of (top-to-bottom) AuNP, AuNS, and Ag@AuNS. TEM images shown are 150 k× magnification and scale bars are 100 nm. NTA analysis of AuNP and AuNS were performed after coating with thiol–PEG to stabilize the metal surface. Background-subtracted SERS spectra showing the peak of interest (546 cm−1) for the reporter, used to determine SERS intensity. | |
Nanoparticle tracking analysis (NTA) provides a rapid assessment of size and concentration of a given nanoparticle solution. Although the size assessment is rapid, it is based on hydrodynamic radius, resulting in measurements that differ from the results of TEM image analysis. Pure Au nanomaterials were stabilized by a surface layer of thiol–PEG, contributing an average of 6 nm to the size measurements. The hydrodynamic sizes of all particles were on average 18 nm larger than the measurements taken by TEM.
The NTA results in Fig. 2 show that the as-synthesized concentration of 27 nm AuNS and 39 nm Ag@AuNS is 30–45% that of commercially available AuNP; as-synthesized 40 nm AuNS and 56 nm Ag@AuNS are 10–13% of the commercial AuNP concentration (this lower concentration was expected due to the reduced number of nucleation sites provided via Au seeds). The concentration data provided by NTA is especially useful because it provides the ability to ensure the samples being compared are similar. NTA measurements are relatively fast, but not practical for measuring every sample, thus we measured the concentration of several batches of nanostars and calibrated the corresponding UV-vis absorbance intensities against them; with this information we are able to rapidly assess the concentration of each type of nanomaterial via absorbance and adjust concentrations as needed for comparable samples. The calibration of various nanomaterials is provided in Fig. S3.† Calibration and absorbance data were used to keep the concentration of nanomaterials the same in the SERS comparison and for LFA loading.
The UV-vis absorbance spectrum of the nanomaterials also provides some indication of the plasmonic properties for the pure gold nanomaterials. Previous work has shown that the localized surface plasmon (LSP) of AuNS shifts over time, and that SERS performance correlates to the observed LSP from UV-vis absorbance measurements.42 For AuNP, the absorbance maximum is located at 525 nm, corresponding to the LSP of the materials. Thus, the SERS enhancement for AuNP is optimal with laser excitation near 525 nm. The as-synthesized AuNS, however, showed LSP bands at 660 and 691 nm for the 27 nm and 40 nm AuNS, respectively. Thus, these materials are expected to perform optimally with laser excitation around 650 nm. When Ag-coating is added to the surface of the NS, the LSP band is shifted, as the Ag surface dominates the optical properties. In these cases, however, we anticipate the LSP to correspond to those of the uncoated materials, because (as shown in the TEM images) the Au core structure is intact.
Our first comparison of SERS performance between different nanomaterials was conducted by measuring the signal of reporter-functionalized particles on the cellulose membrane used for LFA sample pads. Although the LSP bands for all of the materials studies are centered between 500 and 600 nm, the Raman reporter selected, HITC, shows resonance response with a 785 nm laser. The SERS data in Fig. 2 shows the region-of-interest for our reporter for each nanomaterial and Table 1 provides a summary of the enhancement differences between nanomaterials. The average SERS intensity of the reporter on AuNP was 2.9 × 103 counts. The signal intensities of 27 and 40 nm AuNS were ∼6.2× and 6.9× higher than AuNP, respectively. For 39 and 56 nm Ag@AuNS, the signals were further enhanced to ∼8.6× and ∼21.4× higher than that of AuNP, respectively.
Table 1 SERS enhancement factors (EF) relative to AuNP
|
On conjugation pad
|
Control line on LFA
|
Nanomaterial |
Avg. SERS intensity (St. dev) |
EF relative to AuNP |
Avg. SERS intensity (St. dev) |
EF relative to AuNP |
AuNP |
2865 (164) |
— |
1224 (1921) |
— |
27 nm AuNS |
17 656 (1550) |
6.16 |
3266 (3912) |
2.67 |
40 nm AuNS |
19 831 (1951) |
6.92 |
11 224 (3769) |
9.17 |
39 nm Ag@AuNS |
24 575 (2666) |
8.58 |
15 758 (3947) |
12.88 |
56 nm Ag@AuNS |
61 259 (8764) |
21.39 |
22 807 (8268) |
18.64 |
Comparison of SERS in LFA
To investigate the performance of each nanomaterial, we prepared LFAs with only a control line and analyzed the SERS signal across the length of the LFA strip. Photographs of post-test LFAs are shown in Fig. 3a. The images show that the LFA with AuNP produced a stronger (darker) optical control line than the LFAs with NS. This may be an indication that spherical particles flow better through the test strips than the nanostars whose physical features may become entangled in the network of the NC membrane. However, the SERS improvement of NS over NP (Fig. 3b) suggested the signal at the test line would still be significantly better for NS test strips than for NP test strips.
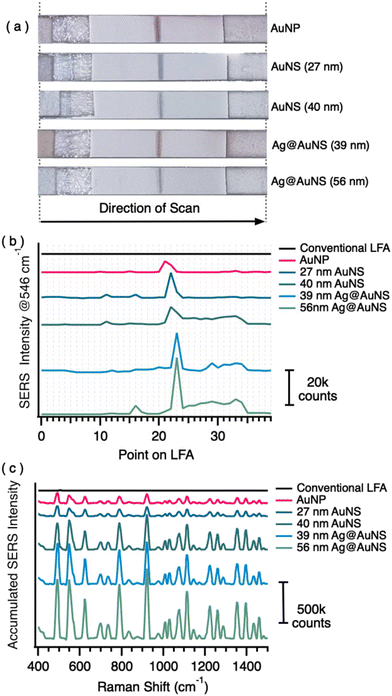 |
| Fig. 3 Analysis of nanoparticle performance in LFA strips: (a) images of LFAs with only visible control line; (b) each LFA was measured with the LFA scanner device and the reporter signal intensities for single 1 s acquisitions, the increased signal at point 21 corresponds to the control line; (c) SERS spectra accumulated from three horizontal (90° with respect to the flow direction) scans (n = 30 spectra) collected across the control line. The total scan time for a full length of LFA and region-of-interest at the test and/or control lines is ∼3 min. | |
To analyze the SERS signal from the LFAs, we employed an automated Raman scanner, which was programmed to measure the SERS signal along the length of the LFA (sample flow direction) with a chosen resolution (in this case, 1 mm increments over the 40 mm total length). This line scan is indicated by the arrow in Fig. 3a. The scanner shows in real-time signal intensity at each point (Fig. 3b). The position of the control line was used to select the point location for collecting three parallel 10-point scans across the width of the LFA to provide accumulated SERS spectra for the control line of each test strip (shown in Fig. 3c as accumulated spectra). In total, completion of the measurements require ∼5 min. Fig. S4† displays the maps obtained from the single data points in these measurements, to show the spatial variability.
The results on the LFA test strips show that the general trend for SERS improvement holds true after flow through capillary action (Table 1). The accumulated signal on the control line from AuNP was ∼1200 counts. For the 27 and 40 nm AuNS, SERS signals were ∼2.7 and 9.2× greater than that of the commercial AuNP, respectively. For the 39 and 56 nm Ag@AuNS, the SERS signals were ∼12.9 and 18.6× greater than that of the AuNP, respectively. The results on LFA strips confirmed that the 56 nm Ag@AuNS provide the highest SERS signal in the LFA and was, therefore, selected as our optimized SERS–LFA nanomaterial.
Determination of SERS–LFA sensitivity
A recent publication has reported3 an antibody-based LFA using AuNP for colorimetric detection P. gingivalis LPS with LOD of ∼20 ng mL−1 in aqueous samples and ∼45 ng mL−1 in human saliva samples. Building on those results, the goal of this work is to produce a SERS–LFA with clinically relevant limits of detection (LOD). In clinical settings, healthy individuals present ≈ 10 ng mL−1 concentrations of LPS from all bacteria types, whereas individuals with periodontitis will have LPS concentrations an order of magnitude higher.36 Thus, for clinical relevance, the SERS–LFA must have a limit of detection of ∼10 ng mL−1. To compare LODs, we performed a calibration for the conventional LFA with colorimetric detection and the SERS–LFA using 56 nm Ag@AuNS. We specifically compare the developed SERS–LFA with a AuNP-based method rather than AuNS, because AuNP are optimized for colorimetric detection and have a superior absorbance in the visible range.
Fig. 4 shows the comparison between calibrations performed on SERS–LFA and colorimetric-LFA. The Ag@AuNS LFA (Fig. 4a) were tested scanning the laser across the LFA long-axis (30 × 1 mm steps) 6 times and averaging the results (Fig. S1†). Fig. 4 reports each line-scan as a 6 × 30 colormap (Fig. 4b) and the average line-scan results (Fig. 4c) for the selected reporter peak (549 cm−1). The test line intensity (at point #10) as a function of LPS concentration is reported in Fig. 4d. Fig. 4e includes equivalent photographs of the AuNP LFAs and the analyzed optical intensity as a function of LPS concentration using ImageJ. In agreement with previous results, the colorimetric LFA analysis can detect LPS at concentrations above 10 ng mL−1, with the first concentration observed above the LOD in this test at 100 ng mL−1. However, the SERS–LFA results show a detection limit below 10 ng mL−1, with the first observable concentration at 10 ng mL−1 (Fig. 4d). These results demonstrate ∼10× improvement offered by the SERS detection mechanism, bringing the LOD within the clinically relevant range for targeted PG LPS. Interestingly, while the LOD is between 10 and 1 ng mL−1, the line scans (Fig. 4c) show the reporter peak monotonically increasing for the test line starting at 1 ng mL−1. Fig. S5a† show an expanded version of Fig. 4c to observe the target-line peak at low concentration. We believe that the LOD value was influenced by non-specific binding in the blank strip due to the low stability of NS after full functionalization (i.e., reporter + antibody), as it can be appreciated from the off-line variance observed in the maps (Fig. 4b). We believe that with further optimization of the functionalization and assay process in this specific application, LOD below 1 ng mL−1 can be achieved. Finally, our results show an improved LOD compared to the colorimetric method but also a significant improvement over simple test-line visualization (common readout method for LFA), which can be observed only above 100 ng mL−1 (Fig. 4e). Fig. S5b† shows the average as well as individual spectra for each measurement (n = 18; 6 lines × 3 sets) on the test line. As it can be observed, while the average agrees with the results shown reported in Fig. 4d (no significant difference between blank and 1 ng mL−1), it is clearly noticeable that the number of high-intensity spectra in 1 ng mL−1 is higher than for the blank (Fig. S5b†). This result suggests that there is likely an observable significant difference even for 1 ng mL−1 samples; however, conventional average of spectral signal may not be the ideal analysis to observe this difference. This observation agrees with recent studies on optimal sampling of SERS–LFA, where counting the number of SERS particles rather the average signal was used to build calibrations.43
 |
| Fig. 4 Characterization of SERS–LFA with increasing concentrations of LPS. SERS with 56 nm Ag@AuNS: (a) LFA photos; (b) SERS intensity maps (6 × 30-point parallel line-scans performed over the strips); (c) average line-scan at increasing LPS concentrations; (d) SERS intensity from the test line (point #10 in Fig. 4c) as a function of LPS concentration, showing data points from single LFA sets and LOD level (error bars represent standard deviation from 3 different LFAs). Characterization of AuNP colorimetric LFA with increasing concentrations of LPS: (e) LFA photos; (f) optical intensity from test line as a function of LPS concentration, showing data points from single LFA sets and LOD level (error bars represent standard deviation from 3 different LFAs). LOD level was calculated as the blank signal +3 × standard deviation of the blank. All SERS intensities are reported for the 546 cm−1 peak of interest. | |
Testing with portable Raman system
The SERS–LFA designed and optimized in this work aims to provide a high-sensitivity option in POC applications. One of the hurdles in the translation of SERS–LFA to the POC domain is the complexity, size and cost of Raman systems that typically contain microscopes, benchtop laser and spectrometer systems. To enable this paradigm shift, Raman systems have been developed to be handheld and optimized for specific POC applications. For SERS–LFA, small systems with a test/control line-wide laser excitation are ideal. This type of system has been previously tested with SERS–LFA showing clinically significant results.32,33 Herein, we tested a ‘hand-held’ SERS–LFA scanner prototype from Wasatch Photonics designed for LFA-based POC applications. This system is battery operated, portable (85 × 55 × 30 mm) and the laser excitation has been designed to form a line shape, to maximize signal from typical LFA strips. The prototype was provided with a 3D-printed support and cartridge for easy reading of LFA strips (see photos in Fig. 5a). The cartridge fits in the support placing the LFA test and control lines and pre-aligned in the line-focus of the laser. To demonstrate the possible translation of the developed SERS–LFA to the POC, we compared the results obtained with the benchtop Raman system to results obtained with the portable device on a set of LFA with several target LPS concentrations. Fig. 5 reports the spectra obtained with the two systems and the average results for each concentration. The results obtained with the prototype designed for POC settings are in agreement (LOD and sensitivity) with those obtained using the benchtop system. Both systems have an LOD < 10 ng mL−1, which was our target to make the LOD of this test clinically relevant. While spectra differ in intensity and resolution due to the difference in detector size and temperature used in the two systems (10 °C for benchtop and uncooled for portable), the trends of SERS intensity as a function of concentration are remarkably similar. Fig. S6† reports the spectra for the control line, which agrees with the conclusions from the test-line results of Fig. 5. Interestingly, the SERS measurements on the benchtop system show a somewhat greater variance than for the portable system. This difference is likely due to the measurements methodology. In the benchtop system the test/control line is scanned horizontally at multiple vertical locations, while in the portable device the entire line is measured at one time. Furthermore, in the portable system the excitation position is manually adjusted to optimize the signal from the test/control line, resulting in a reduced variance in the measurements. Overall, the results indicate that there is significant variability along and across the line and, therefore, the whole-line excitation solution is ideal for SERS–LFA to reduce variability.
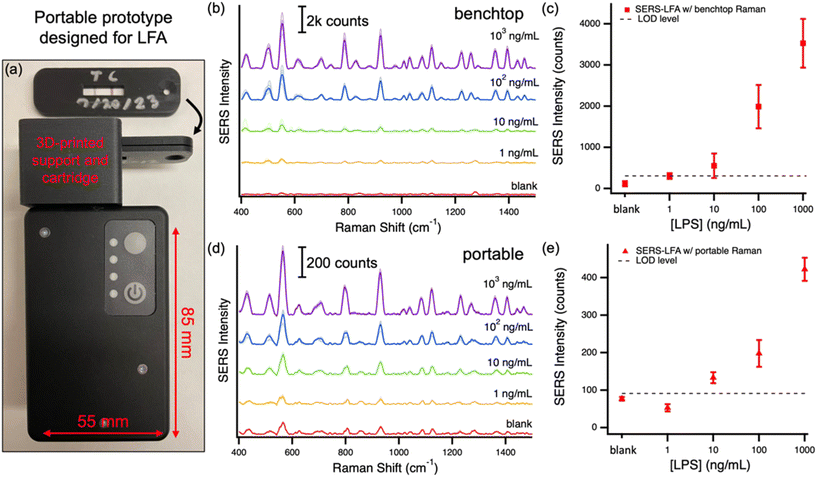 |
| Fig. 5 Comparison of SERS–LFA performance with benchtop and portable Raman systems. (a) Photo of SERS–LFA device prototype. Spectra and SERS signal (@546 cm−1) from a set of LFAs run with different concentrations of LPS for benchtop (b and c) and portable Raman system (d and e). In the SERS spectra both the average spectrum and each measurement spectrum are reported (n = 6 and 9 for b and d, respectively). The single measurement spectra are reported with a lighter shade of the corresponding color. Error bars represent the standard deviation over 6 line-scans for the benchtop instrument and 9 independent measurements (spectra) for the portable. LOD level was calculated as the blank signal +3 × standard deviation of the blank. | |
Conclusion
In summary, we have demonstrated the use of an optimized SERS–LFA to improve the analytical performance for the detection of P. gingivalis, an important bacterium deleterious to oral health. To this end, we characterized four lab-produced nanomaterials and compared their SERS performance to commercially available AuNP. Through this characterization we determined that 56 nm Ag@AuNS were the optimal material for use in SERS–LFAs. We compared the sensitivity of the optimized LFA to that of standard optical (colorimetric) LFAs and demonstrated an improvement in the LFA sensitivity that brings the detection limit of SERS–LFA below 10 ng mL−1, within the clinically relevant concentration window. Our work represents the first study to use Ag@AuNS for SERS–LFA measurements, providing exceptional SERS signal, and to compare more than two nanomaterials for application in SERS–LFAs. Additionally, the use of an automated scanner (collection time 5 min) and a portable spectrometer (collection time <1 min) shows that these tests could be administered and measured in clinical settings in under 15 min. Overall, we have demonstrated a new application for SERS–LFA, while also reporting for the first time a series of nanomaterials in these systems. By incorporating both optimized nanomaterials and portable measurements, the potential application of this technology can greatly improve the quality of clinical diagnostics at the point of care.
Conflicts of interest
The authors declare no conflicts of interest.
Acknowledgements
The authors gratefully acknowledge the many useful discussions on LPS detection with Dr. Sancai Xie from Procter and Gamble. Also, the authors thank Wasatch Photonics for providing the prototype portable Raman system. AC acknowledges an NSF-REU Fellowship (CHE-1950244).
References
- L. Khelifa, Y. Hu, N. Jiang and A. K. Yetisen, Lateral flow assays for hormone detection, Lab Chip, 2022, 22(13), 2451–2475 RSC
.
- B. Kuswandi, M. A. Hidayat and E. Noviana, Paper-based sensors for rapid important biomarkers detection, Biosens. Bioelectron.: X, 2022, 12, 100246 CAS
.
- D. Han, S. Xie and A. J. Steckl, Salivary endotoxin detection using combined mono/polyclonal antibody-based sandwich-type lateral flow immunoassay device, Sens. Diagn., 2023, 2(6), 1460–1468 RSC
.
- S. Dalirirad and A. J. Steckl, Lateral flow assay using aptamer-based sensing for on-site detection of dopamine in urine, Anal. Biochem., 2020, 596, 113637 CrossRef CAS PubMed
.
- S. Dalirirad and A. J. Steckl, Aptamer-based lateral flow assay for point of care cortisol detection in sweat, Sens. Actuators, B, 2019, 283, 79–86 CrossRef CAS
.
- Y. Liu, L. Zhan, Z. Qin, J. Sackrison and J. C. Bischof, Ultrasensitive and Highly Specific Lateral Flow Assays for Point-of-Care Diagnosis, ACS Nano, 2021, 15(3), 3593–3611 CrossRef CAS PubMed
.
- J. Budd, B. S. Miller, N. E. Weckman, D. Cherkaoui, D. Huang, A. T. Decruz, N. Fongwen, G.-R. Han, M. Broto, C. S. Estcourt, J. Gibbs, D. Pillay, P. Sonnenberg, R. Meurant, M. R. Thomas, N. Keegan, M. M. Stevens, E. Nastouli, E. J. Topol, A. M. Johnson, M. Shahmanesh, A. Ozcan, J. J. Collins, M. Fernandez Suarez, B. Rodriguez, R. W. Peeling and R. A. McKendry, Lateral flow test engineering and lessons learned from COVID-19, Nat. Rev. Bioeng., 2023, 1(1), 13–31 CrossRef
.
- E. Frantz, H. Li and A. J. Steckl, Quantitative hematocrit measurement of whole blood in a point-of-care lateral flow device using a smartphone flow tracking app, Biosens. Bioelectron., 2020, 163, 112300 CrossRef CAS PubMed
.
- S. Jarin, D. S. Dandy, B. J. Geiss and C. S. Henry, Padlock probe-based rolling circle amplification lateral flow assay for point-of-need nucleic acid detection, Analyst, 2021, 146(13), 4340–4347 RSC
.
- Y. Wang, Z. Qin, D. R. Boulware, B. S. Pritt, L. M. Sloan, I. J. González, D. Bell, R. R. Rees-Channer, P. Chiodini, W. C. W. Chan and J. C. Bischof, Thermal Contrast Amplification Reader Yielding 8-Fold Analytical Improvement for Disease Detection with Lateral Flow Assays, Anal. Chem., 2016, 88(23), 11774–11782 CrossRef CAS PubMed
.
- K. Kim, L. Kashefi-Kheyrabadi, Y. Joung, K. Kim, H. Dang, S. G. Chavan, M.-H. Lee and J. Choo, Recent advances in sensitive surface-enhanced Raman scattering-based lateral flow assay platforms for point-of-care diagnostics of infectious diseases, Sens. Actuators, B, 2021, 329, 129214 CrossRef CAS PubMed
.
- S. P. Mulvaney, D. A. Kidwell, J. N. Lanese, R. P. Lopez, M. E. Sumera and E. Wei, Catalytic lateral flow immunoassays (cLFIA™): Amplified signal in a self-contained assay format, Sens. Biosensing Res., 2020, 30, 100390 CrossRef
.
- J. Hwang, S. Lee and J. Choo, Application of a SERS-based lateral flow immunoassay strip for the rapid and sensitive detection of staphylococcal enterotoxin B, Nanoscale, 2016, 8(22), 11418–11425 RSC
.
- X. Cao, Q. Song, Y. Sun, Y. Mao, W. Lu and L. Li, A SERS–LFA biosensor combined with aptamer recognition for simultaneous detection of thrombin and PDGF-BB in prostate cancer plasma, Nanotechnology, 2021, 32(44), 445101 CrossRef CAS PubMed
.
- Q. Shi, J. Huang, Y. Sun, R. Deng, M. Teng, Q. Li, Y. Yang, X. Hu, Z. Zhang and G. Zhang, A SERS-based multiple immuno-nanoprobe for ultrasensitive detection of neomycin and quinolone antibiotics via a lateral flow assay, Microchim. Acta, 2018, 185(2), 84 CrossRef PubMed
.
- J. Li, J. Xu, Y. Pan, Y. Zhu, Y. Wang, S. Chen and X. Wei, Au@Ag-labeled SERS lateral flow assay for highly sensitive detection of allergens in milk, Food Sci. Hum. Wellness, 2023, 12(3), 912–919 CrossRef CAS
.
- C. Wang, C. Wang, J. Li, Z. Tu, B. Gu and S. Wang, Ultrasensitive and multiplex detection of four pathogenic bacteria on a bi-channel lateral flow immunoassay strip with three-dimensional membrane-like SERS nanostickers, Biosens. Bioelectron., 2022, 214, 114525 CrossRef CAS PubMed
.
- S. Srivastav, A. Dankov, M. Adanalic, R. Grzeschik, V. Tran, S. Pagel-Wieder, F. Gessler, I. Spreitzer, T. Scholz, B. Schnierle, O. E. Anastasiou, U. Dittmer and S. Schlücker, Rapid and Sensitive SERS-Based Lateral Flow Test for SARS-CoV2-Specific IgM/IgG Antibodies, Anal. Chem., 2021, 93(36), 12391–12399 CrossRef CAS PubMed
.
- W. A. Hassanain, J. Spoors, C. L. Johnson, K. Faulds, N. Keegan and D. Graham, Rapid ultra-sensitive diagnosis of clostridium difficile infection using a SERS-based lateral flow assay, Analyst, 2021, 146(14), 4495–4505 RSC
.
- Y. Mao, Y. Sun, J. Xue, W. Lu and X. Cao, Ultra-sensitive and high efficiency detection of multiple non-small cell lung cancer-related miRNAs on a single test line in catalytic hairpin assembly-based SERS–LFA strip, Anal. Chim. Acta, 2021, 1178, 338800 CrossRef CAS PubMed
.
- S. Yan, C. Liu, S. Fang, J. Ma, J. Qiu, D. Xu, L. Li, J. Yu, D. Li and Q. Liu, SERS-based lateral flow assay combined with machine learning for highly sensitive quantitative analysis of Escherichia coli O157:H7, Anal. Bioanal. Chem., 2020, 412(28), 7881–7890 CrossRef CAS PubMed
.
- R. Wang, K. Kim, N. Choi, X. Wang, J. Lee, J. H. Jeon, G.-E. Rhie and J. Choo, Highly sensitive detection of high-risk bacterial pathogens using SERS-based lateral flow assay strips, Sens. Actuators, B, 2018, 270, 72–79 CrossRef CAS
.
- Z. Li, Y. Gu, S. Ge, Y. Mao, Y. Gu, X. Cao and D. Lu, An aptamer-based SERS–LFA biosensor with multiple channels for the ultrasensitive simultaneous detection of serum VEGF and osteopontin in cervical cancer patients, New J. Chem., 2022, 46(43), 20629–20642 RSC
.
- Y. Zhan, R. Fei, Y. Lu, Y. Wan, X. Wu, J. Dong, D. Meng, Q. Ge and X. Zhao, Ultrasensitive detection of multiple Alzheimer's disease biomarkers by SERS–LFA, Analyst, 2022, 147(18), 4124–4131 RSC
.
- X. Liu, X. Yang, K. Li, H. Liu, R. Xiao, W. Wang, C. Wang and S. Wang, Fe3O4@Au SERS tags-based lateral flow assay for simultaneous detection of serum amyloid A and C-reactive protein in unprocessed blood sample, Sens. Actuators, B, 2020, 320, 128350 CrossRef CAS
.
- L.-K. Lin and L. A. Stanciu, Bisphenol A detection using gold nanostars in a SERS improved lateral flow immunochromatographic assay, Sens. Actuators, B, 2018, 276, 222–229 CrossRef CAS
.
- D. Zhang, L. Huang, B. Liu, H. Ni, L. Sun, E. Su, H. Chen, Z. Gu and X. Zhao, Quantitative and ultrasensitive detection of multiplex cardiac biomarkers in lateral flow assay with core-shell SERS nanotags, Biosens. Bioelectron., 2018, 106, 204–211 CrossRef CAS PubMed
.
- W. A. Hassanain, J. Spoors, C. L. Johnson, K. Faulds, N. Keegan and D. Graham, Rapid ultra-sensitive diagnosis of clostridium difficile infection using a SERS-based lateral flow assay, Analyst, 2021, 146(14), 4495–4505 RSC
.
- Z. Wu, Simultaneous Detection of Listeria monocytogenes and Salmonella typhimuriumby a SERS-Based Lateral Flow Immunochromatographic Assay, Food Anal. Methods, 2019, 12(5), 1086–1091 CrossRef
.
- X. Wang, N. Choi, Z. Cheng, J. Ko, L. Chen and J. Choo, Simultaneous detection of dual nucleic acids using a SERS-based lateral flow assay biosensor, Anal. Chem., 2017, 89(2), 1163–1169 CrossRef CAS PubMed
.
- M. Sánchez-Purrà, M. Carré-Camps, H. De Puig, I. Bosch, L. Gehrke and K. Hamad-Schifferli, Surface-Enhanced Raman Spectroscopy-Based Sandwich Immunoassays for Multiplexed Detection of Zika and Dengue Viral Biomarkers, ACS Infect. Dis., 2017, 3(10), 767–776 CrossRef PubMed
.
- V. Tran, B. Walkenfort, M. König, M. Salehi and S. Schlücker, Rapid, Quantitative, and Ultrasensitive Point-of-Care Testing: A Portable SERS Reader for Lateral Flow Assays in Clinical Chemistry, Angew. Chem., Int. Ed., 2019, 58(2), 442–446 CrossRef CAS PubMed
.
- C. Soliman, J. Faircloth, D. Tu, S. Mabbott, K. Maitland and G. Coté, Exploring the Clinical Utility of Raman Spectroscopy for Point-of-Care Cardiovascular Disease Biomarker Detection, Appl. Spectrosc., 2023, 77(10), 1181–1193 CrossRef CAS PubMed
.
- Z. Corredor, A. Suarez-Molina, C. Fong, L. Cifuentes-C and S. Guauque-Olarte, Presence of periodontal pathogenic bacteria in blood of patients with coronary artery disease, Sci. Rep., 2022, 12, 1241 CrossRef CAS PubMed
.
- S. S. Dominy, C. Lynch, F. Ermini, M. Benedyk, A. Marczyn, A. Konradi, M. Nguyen, U. Haditsch, D. Raha, C. Griffin, L. J. Holsinger, S. Arastu-Kapur, S. Kaba, A. Lee, M. I. Ryder, B. Potempa, P. Mydel, A. Hellvard, K. Adamowicz, H. Hasturk, G. D. Walker, E. C. Reynolds, R. L. M. Faull, M. A. Curtis, M. Dragunow and J. Potempa, Porphyromonas gingivalis in Alzheimer's disease brains: Evidence for disease causation and treatment with small-molecule inhibitors, Sci. Adv., 2019, 5(1), eaau3333 CrossRef PubMed
.
- S. Zaric, A. Strachan, Y. Kurushima, A. Dong, C. McIlwaine, Z. Harrington, L. Nibali, A. Foey and M. Ide, Evaluating clinical utility of subgingival and salivary endotoxin activity levels as periodontal biomarkers, Front. Oral Health, 2022, 3, 1029806 CrossRef PubMed
.
- H. Yuan, A. M. Fales, C. G. Khoury, J. Liu and T. Vo-Dinh, Spectral Characterization and Intracellular Detection of Surface-Enhanced Raman Scattering(SERS)-Encoded Plasmonic Gold Nanostars, J. Raman Spectrosc., 2013, 44(2), 234–239 CrossRef CAS PubMed
.
- J. Turkevich, P. C. Stevenson and J. Hillier, A study of the nucleation and growth processes in the synthesis of colloidal gold, Discuss. Faraday Soc., 1951, 11, 55 RSC
.
- A. M. Fales, H. Yuan and T. Vo-Dinh, Development of Hybrid Silver-Coated Gold Nanostars for Nonaggregated Surface-Enhanced Raman Scattering, J. Phys. Chem. C, 2014, 118(7), 3708–3715 CrossRef CAS PubMed
.
- J. Schindelin, I. Arganda-Carreras, E. Frise, V. Kaynig, M. Longair, T. Pietzsch, S. Preibisch, C. Rueden, S. Saalfeld, B. Schmid, J. Y. Tinevez, D. J. White, V. Hartenstein, K. Eliceiri, P. Tomancak and A. Cardona, Fiji: an open-source platform for biological-image analysis, Nat. Methods, 2012, 9(7), 676–682 CrossRef CAS PubMed
.
- P. R. Sajanlal, T. S. Sreeprasad, A. K. Samal and T. Pradeep, Anisotropic nanomaterials: structure, growth, assembly, and functions, Nano Rev., 2011, 2, 5883 CrossRef PubMed
.
- D. Vang and P. Strobbia, Analysis of Nanostar Reshaping Kinetics for Optimal Substrate Fabrication, Appl. Spectrosc., 2023, 77(3), 270–280 CrossRef CAS PubMed
.
- A. Tuckmantel Bido and A. G. Brolo, Digital SERS Protocol Using Au Nanoparticle-Based Extrinsic Raman Labels for the Determination of SARS-CoV-2 Spike Protein in Saliva Samples, ACS Appl. Nano Mater., 2023, 6(17), 15426–15436 CrossRef CAS
.
|
This journal is © The Royal Society of Chemistry 2024 |
Click here to see how this site uses Cookies. View our privacy policy here.