DOI:
10.1039/D4SD00122B
(Paper)
Sens. Diagn., 2024,
3, 1854-1865
A comprehensive FTIR micro-spectroscopic analysis and classification of precancerous human oral tissue aided by machine learning†
Received
18th April 2024
, Accepted 23rd September 2024
First published on 27th September 2024
Abstract
We present an analysis of the molecular vibrational assessments of different grades of oral precancerous tissue sections, aiming to an early, alternative method other than histopathology to definitively distinguish their grades and remove the interobserver variability related to histopathological grading. Assessment of the prognosis of oral potentially malignant disorders (OPMDs) is dependent only on clinical features, and no defined criteria are still proposed to analyze the treatment outcome. Chair-side analysis of the lymph node metastasis and staging of oral squamous cell carcinoma (OSCC) is also dependent on palpatory findings followed by magnetic resonance imaging (MRI). Among these, Fourier-transform infrared (FTIR) micro-spectroscopy emerges as a highly promising and versatile approach for analyzing oral cancer and precancer specimens, enabling the identification of chemical and molecular changes in tissue samples. In this work, an adequate number of tissue sections affected by different grades of precancer (mild dysplasia, moderate dysplasia, and severe dysplasia) were investigated for biochemical changes in the epithelium and sub-epithelium layers as characterized by their corresponding molecular vibration spectrum. The current study demonstrated distinct alterations based on the spectrum shift of proteins (particularly amide I and amide III) over the progression of precancer. Additionally, using the amide I and amide III regions, a peak fitting method was employed to estimate the secondary structures of proteins. Further, chemometric techniques of principal components analysis–linear discriminant analysis (PCA–LDA) were used to create discrimination models for the precancerous and control groups. Our investigation revealed that the predictive performance of the amide III region was better than that of the amide I region, achieving a 95% accuracy rate. To the best of our knowledge, this is one of the first studies on the application of FTIR micro-spectroscopy for the classification of oral precancers in humans, aided by machine learning.
Introduction
Oral cancer ranks as the 6th most common malignancy in the world and has been a major burden to global health.1 In the Indian subcontinent, oral cancer is classified among the top three of all types of cancer, which is significantly prominent among the lower socio-economic sections of the country because of their lifestyle choices of consumption of tobacco (chewing and smoking), drinking alcohol, and betel nut chewing.1–3 In India, oral cancer poses a major health concern, with around 77
000 new cases and 52
000 fatalities reported each year.4 This represents around one-fourth of global oral cancer incidences.4 Oral squamous cell carcinoma (OSCC) can be preceded by oral potentially malignant disorders (OPMDs).5 Several discourses exist regarding diagnosing different OPMDs and their histopathological grading related to inter-observer variability.5–7 The interobserver variability is a subjective concept that can occur due to differences in knowledge, experience, and interpretation of clinical and histopathological features, leading to potential discrepancies in diagnosing these conditions and finally leading to erroneous treatment planning.6 Studies have shown that interobserver variability can lead to diagnostic errors in a range of 5–30% for OPMDs.6 Factors such as the lesion's complexity, the observers' experience, and the available diagnostic tools can all contribute to the variability in diagnoses.5
It is important for clinicians to be aware of this variability whilst declaring their prognosis compared to OSCC and similar related malignancies. Therefore, it is critical to utilize advanced diagnostic techniques to minimize the risk of diagnostic errors and estimate the disease-related complications and their progression toward OSCC. Currently, histopathological assessment of the biopsied tissue sample using optical brightfield microscopy is considered the gold standard for oral cancer diagnosis.8,9 Additional diagnostic modalities in immunohistochemistry have been explored to detect specific cancer signatures during the progression of oral cancer.10 However, the limited availability and high expenses in the identification of specific molecular markers for the detection of oral cancer leads to its late and poor diagnosis.10 Also, the time-consuming nature of the histopathologic or other molecular diagnostic techniques, such as immunohistochemistry (IHC) limits its suitability for the purpose of routine screening.11,12 On the other hand, the treatment outcome of OPMDs involves a comprehensive approach that encompasses both clinical and histopathological evaluations. Clinical symptoms alone do not necessarily reflect the actual treatment outcome, and on the other hand, repeated biopsy for assessing treatment prognosis is impractical, as it causes trauma to the tissue and adds complications.13 Thereby, it is vital to integrate new technologies for better characterization and understanding of malignant changes associated with OPMDs.7 A highly promising and versatile method for the analysis of oral cancer specimens could be Fourier-transform infrared (FTIR) micro-spectroscopy. The FTIR technique operates on the principle that particular organic molecules absorb and emit infrared (IR) waves at specific frequencies, generating characteristic fingerprint spectra for identification. When applied to biological samples, such as tissues, cells, or biofluids, FTIR micro-spectroscopy can detect and quantify alterations in the molecular composition and structure.14 These alterations often indicate pathological conditions, making FTIR a valuable tool for disease diagnosis and monitoring.14,15 Unlike an ordinary spectrometer, the FTIR micro-spectrometer is associated with an optical brightfield microscope, focusing on the region of interest (ROI) of heterogeneous samples (in our case, oral tissues). The FTIR spectroscope combined with a high-resolution microscope (hence, micro-spectroscope) can focus on specific and different areas of a sample (e.g., an oral tissue) and spectrally classify the particular chemical alterations in the focused areas. An accurate micrometer-scale chemical mapping could be obtained by repeating this process all over the sample (e.g., oral tissue). Thus, this technique could be used to gain valuable insights into the specific biochemical changes associated with different grades of OPMDS and OSCCs.16–18 In cancer research, FTIR micro-spectroscopy has demonstrated its ability to differentiate healthy tissues from malignant ones by identifying changes in protein, lipid, and nucleic acid profiles.14,19 It enables the identification of specific biomarkers associated with different cancer types and can aid in early detection, prognosis, and monitoring of therapeutic responses.16,20,21
FTIR micro-spectroscopy, thus far, has been successful in distinguishing between malignant and benign tissue specimens of breast,15,22 colon,23,24 oesophagus,25 stomach,26 lung,27 prostate,28 brain,29,30 and skin.31 This modern technique is ideal for studying biomolecules like lipids, proteins, carbohydrates, and nucleic acids in biological systems with a great spatial resolution by analyzing frozen or formalin-fixed samples in situ.32,33 In 2020, Sitnikova et al.21 used attenuated total reflection (ATR) FTIR mode on blood serum to distinguish between the control and the malignant group of breast cancer. The combination of principal component analysis (PCA) for processing the FTIR spectra has shown a potential biomarker range of 1306–1250 cm−1, which belongs to several functional groups of DNA and RNA, thereby providing a key role in discriminating between control and patients' groups of breast cancer. Besides blood samples, FTIR is also capable of establishing discrimination between benign and malignant tissue specimens. A study was performed on colorectal cancer by Dong et al.22 using FTIR spectroscopy to differentiate between normal tissue and colorectal cancer. The FTIR spectra provided a rise in nucleic acid and protein content in the malignant groups, providing a sensitivity of 96.6% using linear discriminant analysis (LDA). Therefore, the combination of FTIR with computational methods can classify spectra from different categories of samples and provide a fast, reliable, and accurate cancer screening when tagged along with conventional histology. The analysis of human oral tissues with FTIR micro-spectroscopy-based chemical imaging can bring forward biochemical characterization during the progression of oral cancer where specific infrared (IR) spectra will contain information-rich images of the tissue and with the addition of multivariate data analysis such as PCA will provide a roadmap to find a potential biomarker, thereby delivering a fast and label-free technique alongside the conventional histology methods.16,32,34 Oral cancer is a heterogeneous type of cancer involving different grades and molecular profiles.35 Prolonged use of tobacco and alcohol leads to the development of pre-malignant disorders or oral precancers.5,11 An in-depth study of oral precancers can facilitate understanding the chemical changes occurring during the progression of precancerous dysplasia in order to assist with better oral cancer diagnosis and management.24 Distinct FTIR spectra were observed by Bruni et al.36 between normal and diseased oral tissues. It was found that significant DNA or collagen content in spectra identified tumour proliferation. Similarly, Sabbatini et al.37 performed a vibrational analysis on sections of both epithelial and connective tissues in oral squamous cell carcinoma (OSCC) and identified some spectral markers such as an increase in free glycogen during carcinogenesis, structural changes in nucleic acids, and increased RNA, suggesting higher cellular activity. A detailed study in utilizing FTIR imaging for the detection of oral squamous cell carcinoma (OSCC) was performed by Pallua et al.15 They demonstrated that with FTIR imaging and multivariate analysis approaches such as hierarchical cluster analysis (HCA) and k-means clustering (KMC) in specific spectral regions, information in infrared datasets has increased dramatically. The data processed by IR spectra contain information-rich content indicating that intra-operative and biopsy samples of oral tissue can be analyzed using FTIR imaging. Meanwhile, Ellis et al.38 had shown the efficacy of using FTIR imaging in discriminating two tissue types, lymph node metastasis of oral cancer and indigenous lymphoid tissue, which was mainly dominated by the contribution of nucleic acid.39 Here, we have utilized FTIR micro-spectroscopy techniques on human precancerous tissues to (1) explore changing the biochemical composition of the epithelium and sub-epithelium layers to understand the gradation and progression of the disease for early and fast detection; (2) perform IR chemical imaging at selected regions of different groups of tissues to visualize the biochemical compositional change; (3) investigate protein regions (specifically, amide I and amide III) to examine the conformational change in protein secondary structure during precancerous stages. Finally, chemometric analysis was performed by principal component analysis (PCA)–linear discriminant analysis (LDA) on the obtained FTIR spectra to build a discrimination model aided by machine learning (ML) algorithms for accurate classification of the various stages of OSCCs.
Experimental section
Patient selection
A number of around 300 patients underwent screening for the detection of oral leukoplakia at the outpatient department (OPD) of the Department of Guru Nanak Institute of Dental Sciences and Research for the year 2022–2023 with the following inclusion criteria: patients suffering from oral leukoplakia with a definitive history of tobacco use, and patients with a medical history of severe metabolic diseases, autoimmune diseases, visceral cancers, or who have undergone chemotherapy or radiation treatment within the past six months were excluded from the study. Following a comprehensive medical evaluation, a total of 196 cases were chosen for inclusion in the study. A total of 152 cases provided their consent for biopsy. Consent was sought from 88 clinically normal individuals without any history of oral habits, serving as the control group, among which 35 individuals volunteered for the study. Biopsies were taken from the lesioned areas that were representative of the clinical condition, and the diagnoses were confirmed through clinicopathological analysis. The histological parameters of precancerous conditions were categorized into three grades of dysplasia: mild dysplasia, moderate dysplasia, and severe dysplasia. The study excluded cases that exhibited histologically observed epithelial hyperplasia, with or without hyperkeratosis. Ultimately, a total of 125 cases of histologically confirmed precancers and 35 normal controls were analyzed. All experiments were performed in accordance with the guidelines “New Drugs and Clinical Trial Rules 2019 India, ICMR-National Ethical Guidelines for Biomedical & Health Research involving Human Participants 2017 India and ICP-GCP & WHO” and approved by the ethics committee at Guru Nanak Institute of Dental Sciences and Research, Kolkata, India. Informed consent was obtained from human participants of this study. Table S1† describes the clinicopathological characteristics of the study groups.
Sample preparation
We collected a total of 120 samples of precancerous lesions, specifically leukoplakia, from surgical removal. These samples were then divided as follows: 24 mild dysplasia (MD), 34 moderate dysplasia (MOD), and 37 severe dysplasia (SD). For comparison, 25 samples of normal buccal mucosa (NOM) from healthy patients free of any underlying periodontal diseases or inflammatory changes were excluded. Five micrometre (5 μm) thin sections of each sample were sectioned using a microtome (Leica RM 2125RT): one was dyed with haematoxylin and eosin (H&E) and placed on a glass slide for histopathological interpretation, and the other was deposited on a glass slide for infrared investigation. Oral precancer staging involved a thorough analysis of clinical grading, radiological findings, and histological findings according to WHO 2005 guidelines.39
We obtained informed consent from the ethical committee of Guru Nanak Institute of Dental Sciences and Research, Kolkata, and J.K. Cancer Institute, Kanpur, to use patient data and corresponding tissue samples for research purposes.
FTIR microscopic imaging experiments
Spectroscopic analysis was performed on 5 μm thick tissue sections, which were prepared from formalin-fixed tissue embedded (FFTE) blocks of healthy and precancerous epithelial tissue. The histological specimens were then baked at 650 ° C for 30 min, and paraffin wax was removed with two xylene treatments. After 10 min of 100% ethanol dehydration, the samples were air-dried for 30 min at ambient temperature. Spectral collection was then performed using a LUMOS FTIR micro-spectroscope (Bruker, Billerica, MA, USA) under the reflection mode. A liquid nitrogen-cooled mercury cadmium telluride detector (MCT) obtained spectral data at a 4 cm−1 resolution. Each measurement consisted of 32 scans, with an acquisition time of 6 seconds in the spectral range of 4000–600 cm−1. For imaging, specific areas were selected in agreement with the dental pathologist (co-authors KB, SKB, MP, and RRP) on which chemical maps were obtained. The spatial resolution was 10 μm × 10 μm, and for each map, spectra of 6400 were acquired. Before measurement, an adequate background spectrum was acquired from outside the sample region.2
Spectral pre-processing and chemometric analysis
PCA–LDA was employed to construct discrimination models for distinguishing between four distinct study groups using spectral features extracted from individual FTIR spectra. Principal component analysis (PCA) was initially utilized to capture the dataset's variability while minimizing information loss. The FTIR data from the four groups, namely normal, mild dysplasia, moderate dysplasia, and severe dysplasia, were input into the PCA model for classification within two specific spectral regions: (i) 1700–1600 cm−1, corresponding to amide I, and (iii) 1400–1200 cm−1, corresponding to amide III. Subsequently, the principal components (PCs) accounting for over 95% of the dataset's variance were selected for further analysis. A total of 15 PCs were used in the subsequent analysis. The supervised chemometric method, PCA–LDA, was executed using Origin Pro 10.0.0 software by Origin Lab Corporation, USA. This method used a mathematical classification algorithm to divide the classes and maximize the distance between group means while minimizing class variance. The performance of the training model was assessed through a leave-one-out cross-validation method.
Results and discussions
Numerous oral pre-malignancy samples of different stages underwent histological and vibrational analysis and were classified as mild dysplasia (MD), moderate dysplasia (MOD), and severe dysplasia (SD). Histopathological interpretation revealed the following findings in different stages of oral pre-malignancy, as shown in the schematics of Fig. 1: normal tissue (NOM) – the epithelium consists of highly organized stratified squamous epithelial cells with no architectural modification; mild dysplasia (MD) – within the full epithelial thickness, the architectural incontinence was restricted to the lower third of the epithelium, showing minimal cytopathological atypia corresponding to minimal criteria for dysplasia;40 moderate dysplasia (MOD)– the epithelial architectural shell was found disturbed, extending from the middle third of the epithelium, including the basal and the parabasal changes. The degree of cytological atypia was also considered;38 severe dysplasia (SD) – samples were staged as severely dysplastic when greater than two-thirds of the epithelial thickness revealed disintegration in the architectural framework and stratification, all associated with cytopathological atypia. Samples with fields showing frank invasive fronts were not included in this category.41
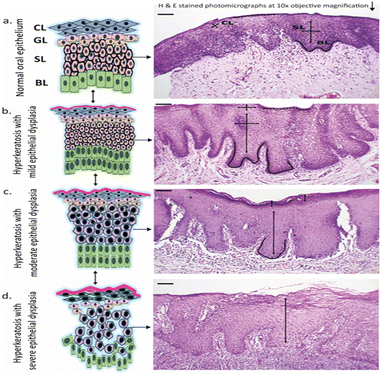 |
| Fig. 1 Represents the progression of oral precancer from normal oral epithelium to a severely dysplastic epithelium. The oral keratinized stratified squamous epithelium has 4 layers namely: corneal cell layer (CL), granular cell layer (GL), spinous cell layer (SL), basal cell layer (BL). From the photomicrographs, the marked dysplastic changes can be clearly seen from (a) normal, (b) mild epithelial dysplasia (c) moderate epithelial dysplasia to (d) severe epithelial dysplasia, all starting from the basal layer and progressing up to superficial layers. Scale bar (top left in the photomicrograph): 100 μm. | |
Spectral features of FTIR spectroscopy
In the present study, pre-processed and mean spectra of oral precancerous groups, along with their epithelial and sub-epithelial layers, were investigated by FTIR spectroscopic and imaging analysis. FTIR spectra of four study groups, normal, mild, moderate, and severe dysplasia (NOM, MD, MOD, and SD) along with their sub-epithelial layers of individual groups are illustrated in Fig. 2, and their corresponding spectral signatures are tabulated in Tables 1 and 2. Prominent absorption peaks were observed in the 1700–1600 cm−1 region, which corresponded to amide I vibrations, 1200–1400 cm−1 region of amide III vibrations, and 1000–900 cm−1 region corresponding to vibrations obtained from nucleic acids.42 A broadening of peaks around 1310 cm−1 was observed in the NOM granular layer which is shifted from 1320 cm−1 in the basal layer. Similar changes were observed where the peak at 1293 cm−1 of the spinous region of SD is broadened and shifted from 1317 cm−1 of the basal region. In this region of the amide III band, in-phase N–H bending and C–N stretching cause vibrations that respond to the folding of secondary structures.43,44
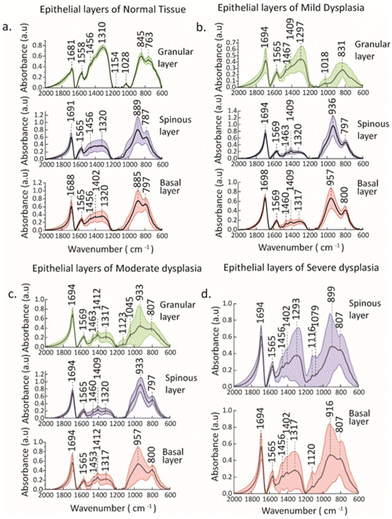 |
| Fig. 2 Pre-processed mean (black solid line) and standard deviation (SD) (colored shaded bands) spectra of (a) sub-epithelial layers of normal tissue, (b) sub-epithelial layers of mild dysplasia, (c) sub-epithelial layers of moderate dysplasia, and (d) sub-epithelial layers of severe dysplasia (light red – basal layer; blue – spinous layer; green – granular layer). | |
Table 1 Assignments of FTIR bands of normal and mild dysplasia along with their epithelial layers
Normal |
Mild dysplasia |
Assignment |
Basal layer |
Spinous layer |
Granular layer |
Basal layer |
Spinous layer |
Granular layer |
1688 |
1691 |
1681 |
1694 |
1698 |
1694 |
C O stretch + NH bend (amide I) |
1565 |
1565 |
1558, 1521 |
1569 |
1569 |
1565, 1518 |
NH bend + C–N stretch (amide II) |
1456 |
1456 |
1456 |
1463 |
1460 |
1467 |
CH
3 bending modes (proteins) |
1402 |
1399 |
— |
1409 |
1409 |
1409 |
COO
− symmetric stretch (fatty acids, amino acids) |
1320 |
1320 |
1310 |
1320 |
1317 |
1297 |
In plane NH bend, CC stretch, CN stretch, C O in plane bend (amide III) |
— |
1120 |
— |
— |
— |
1123 |
C–O stretch of ribose ring (RNA) |
— |
1079 |
1154, 1028 |
— |
— |
1018 |
COH deformation (glycogen) |
885 |
889 |
845 |
936 |
957 |
831 |
Ribose phosphate skeletal vibrations (nucleic acids) |
Table 2 Assignments of FTIR bands of moderate and severe dysplasia along with their epithelial layers
Moderate dysplasia |
Severe dysplasia |
Assignments |
Basal layer |
Spinous layer |
Granular layer |
Basal layer |
Spinous layer |
1694 |
1694 |
1694 |
1694 |
1694 |
C O stretch + NH bend (amide I) |
1565 |
1565 |
1569 |
1565 |
1565 |
NH bend + C–N stretch (amide II) |
1453 |
1460 |
1463 |
1456 |
1456 |
CH
3 bending modes (proteins) |
1412 |
1409 |
1412 |
1402 |
1402 |
COO
− symmetric stretch (fatty acids, amino acids) |
1317 |
1320 |
1317 |
1317 |
1293 |
In plane NH bend, CC stretch, CN stretch, C O in plane bend (amide III) |
1123 |
— |
1123 |
1120 |
1116 |
C–O stretch of ribose ring (RNA) |
— |
— |
1045 |
— |
1079 |
COH deformation (glycogen) |
957 |
933 |
933 |
916 |
899 |
Ribose phosphate skeletal vibrations (nucleic acids) |
Spectral regions related to CH3 bending modes of proteins (1453–1467 cm−1), COO− symmetric stretch (1401–1414 cm−1) relating to fatty acids, C
O stretch + NH bend (1600–1700 cm−1) relating to amide I were observed with distinct peaks in different sub-epithelial layers of NOM, MD and SD. Some distinct C–O stretch of ribose ring focused around 1120 cm−1 assigned to RNA were observed in the basal and spinous layer/region of SD. Prominent peaks of glycogen centered around 1045 cm−1 and 1079 cm−1 were found in the granular layer of MOD and SD, respectively, whereas these were absent in the basal and spinous layers of these groups. Similarly, peaks around 1028 cm−1 and 1018 cm−1 were observed in the granular layer of NOM and MD. The absorption of glycogen is attributed to the stretching of C–O and C–C bonds as well as the deformation motions of C–O–H.44 Ribose phosphate skeletal vibrations relating to nucleic acids (1000–830 cm−1) were observed in all the stages of precancerous groups, with shifts in their sub-epithelial layers indicating post-translational modifications of proteins.45 These modifications encompass various intricate processes, such as alternative splicing and mRNA stability, which have been strongly linked to the progression from potentially malignant disorders (PMDs) to oral cancer.46 There is growing emphasis on the role of glycogen metabolism and its interplay with glycogen synthase kinase 3, an essential enzyme pivotal in regulating glycogen dynamics.47 These studies suggest that disruptions in glycogen metabolism, such as abnormal glycogen levels or dysregulation of glycogen synthase kinase 3 activity, could lead to aberrant post-transcriptional modifications in OPMDs, thereby contributing to their progression towards oral cancer.47 Furthermore, studies have indicated that alterations in glycogen metabolism, such as abnormal levels of glycogen or dysregulated activity of glycogen synthase kinase 3, can lead to dysregulated post-transcriptional modifications in oral potentially malignant disorders.48 These modifications can ultimately influence the expression and function of key genes involved in cell proliferation, apoptosis, and other processes related to tumorigenesis.49 As a result, understanding the impact of glycogen on post-transcriptional modifications in oral potentially malignant disorders may provide crucial insights into the underlying mechanisms driving the progression of these disorders and potentially identify novel therapeutic targets and prognostic markers.48,50
FTIR spectra of basal and spinous layers among different precancerous groups
In oral dysplastic epithelium, proliferative maturation of the cells progressively takes place from the lower basal to the uppermost layer due to faulty transcription and translation.51 Amide III band for basal layer were observed at 1320 cm−1, 1320 cm−1, 1317 cm−1, and 1317 cm−1 for NOM, MD, MOD, and SD groups, respectively (Tables 1 and 2). Peaks in amide III are attributed to the vibrations of C–N stretch and N–H bend of proteins.44 Similar observations were seen for the spinous layer where peaks centered at 1293 cm−1, 1320 cm−1, 1317 cm−1, and 1320 cm−1 for SD, MOD, MD, and NOM, indicating a spectral shift, which could be a result of an alteration in the second structure of the protein. The fluctuations in the spectral intensity along with the shifts within 1340–1240 cm−1 correspond to alterations in cellular structure conformations within the oral epithelial cells.52 However, in the amide I region around 1600–1700 cm−1, no significant shifts were observed among all the tissue groups. In addition, using the amide III band for quantitative analysis of protein secondary structure has certain advantages over the amide I region, as demonstrated by B. R. Singh et al.43,44 The water IR band around (1640 cm−1), along with interactions between random coils and α-helix bands in the amide I region, limits its usability for structural analysis of proteins, whereas no interfering OH vibrations and overlapping of random coils and α-helix bands are observed in the amide III region.44 A strong amide II peak in the range of 1500–1600 cm−1 was observed in all the tissue groups with very little shift. The peak centered at 1120 cm−1 in the basal layer corresponds to RNA (ribose ring vibrations C–O stretch) of the SD group, whereas it is absent in the NOM, MD, and MOD groups. In the spinous layer, similar sharp peaks were observed at 1116 cm−1 only for SD. These peaks indicate DNA methylation alterations in malignant tissues.37,46 Moreover, relevant shifts were observed in the ribose phosphate region around 1000–830 cm−1 among both the basal and the spinous layers of all the tissue groups, indicating the nucleic acid conformational change. Spectral peaks around 1409 cm−1 represent COO− symmetric stretch vibrations of amino acids, which vary in intensity across all tissue groups for both basal and spinous layers.53 The changes in intensity can be assigned to the alteration of protein from tissues within the cells of the epithelium, which is associated with the development of malignancy.42
Peak fitting analysis for secondary structure of protein
Protein secondary structure can be examined by analyzing the amide I and amide III areas of infrared spectra.44,54 Prior research has highlighted the use of FTIR spectra to determine the composition and structure of secondary structures using the amide I region, and it was found that there was an increase in helical structures in carcinogenesis.37 While amide I is commonly employed to analyze the secondary structure, the amide III region's lack of water interference and the greater distinction in amide III spectra could offer a potentially effective method to investigate the secondary structure.43,44 The deconvoluted protein spectra of healthy (NOM) and precancerous groups (MD, MOD, and SD) were analyzed along with their epithelial layers using both amide I and amide III bands by peak-fitting and second derivative approach.
Protein secondary structure by amide I band
The amide I band is utilized for secondary structure research due to its highly prominent protein signal.44,55 The amide I band observed in FTIR spectra primarily arises from the C
O stretching vibrations, which account for approximately 80% of the band's composition.56 Additionally, NH bending vibrations contribute to a lesser extent.56 The hydrogen bonds established between the C
O groups and the neighbouring NH groups determine the precise location of these vibrations.56 As a protein's secondary structure determines the formation of hydrogen bonds with specific NH groups, the vibrational spectra serve as distinctive fingerprints for characterizing the protein's secondary structure.56
A protein's secondary structure is thought to be the result of the accumulation of its essential secondary structural components, such as α-helix (1648–1657 cm−1), β-sheet (1623–1641 cm−1), β-turn (1662–1686 cm−1), and random coil (1643–1650 cm−1).54,55,57 The spectral intensity of each secondary structure element determines its relative percentage, as the molar absorptivity of C
O stretching vibrations is virtually constant among all secondary structural elements. The deconvoluted amide I band revealed the secondary structure of protein components such as α-helix (1656 ± 3),42,55 β-sheet (1634 ± 5) and β-turn (1680 ± 3) in all samples (Fig. 3). The analysis using the peak-fitting technique identified a prominent band at 1656 cm−1, indicating the presence of α-helical structure. However, this band was observed to decrease significantly in severe dysplasia compared to normal tissue in the basal cell layer. Conversely, the area under the β-sheet signal showed an increase in severe dysplasia, whereas it was relatively low in normal tissue. How much of the secondary structure is present in proteins can be found by looking underneath the hidden peaks in the original spectrum. However, a similar observation was not seen in the spinous layer of NOM, MD, MOD, and SD (see Fig. S1†). Further, the modifications of these secondary structures can be related to the mutations in amino acids of proteins for cancer progression.57
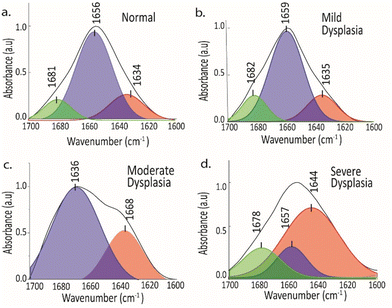 |
| Fig. 3 Deconvoluted FTIR spectra in the amide I band of the basal layer/region in (a) normal tissue, (b) mild dysplasia, (c) moderate dysplasia and (d) severe dysplasia. α-Helix (1648–1657 cm−1), β-sheet (1623–1641 cm−1), β-turn (1662–1686 cm−1), and random coil (1643–1650 cm−1). | |
Protein secondary structure by amide III band
Attempts have been made to explore the amide III band to analyze the secondary structure content of proteins.43,45 Predicting the α-helix contents in proteins can be challenging due to the complex overlap of alpha-helical components with the random coil in the amide I region.43 Additionally, there is an interference caused by H2O in this area. Despite the weaker amide III signal compared to that of amide I, the in-phase vibrations of bending and stretching of N–H and C–N bonds are highly responsive to the folding of secondary structure, without any interference from water.43 Using a peak-fitting procedure, an analysis was conducted on the amide III region of the average spectra of NOM, MD, MOD and SD samples, where component bands at 1300 ± 5 (α-helix), 1288 ± 2 (β-turn), 1240 ± 2 (β-sheet) and 1270 ± 5 (random coil) were found56 (Fig. 4). Peaks centered around the β-turn were evident in MD, MOD, and SD samples and were absent in normal tissue of the basal layer. There is a decrease in the band area of the α-helical structure towards severe dysplasia, as observed in Fig. 4. Peaks of random coil structure were present in normal and mild dysplasia groups, which was previously not found using the amide I region. Moreover, there are changes in the relative content of α-helix, β-sheet, and β-turn in all the samples along basal and spinous epithelial layers (see Fig. S2†). Unraveling the complex interplay between these proteins and the surrounding oral microenvironment may provide valuable insights into the biomolecular processes occurring during the progression of oral disorders toward malignancy. The spectral changes observed in the amide III band are primarily associated with alterations in the conformation and composition of various proteins present in oral tissues. Proteins such as collagen, elastin, and keratin are known to contribute to the amide III band and play critical roles in maintaining the structural integrity and function of oral tissues.43,44 As oral disorders progress towards malignant transformation, the conformation and composition of these proteins can undergo significant changes, leading to distinct spectral features associated with the amide III band.58 Moreover, the interaction of these proteins with other biomolecules in the oral microenvironment, such as glycoproteins and proteoglycans, can also influence the spectral characteristics of the amide III band. Understanding the specific proteins and their interactions that contribute to the observed spectral changes is essential in deciphering the molecular mechanisms underlying malignant transformation in oral tissues.42,58 Further research aimed at identifying and characterizing the specific proteins involved in the spectral changes associated with amide III absorption will be instrumental in advancing our understanding of the molecular events driving oral malignancies. Additionally, unraveling the complex interplay between these proteins and the surrounding microenvironment will provide valuable insights into the biomolecular processes occurring during the progression of oral disorders toward malignancy. Understanding how these spectral changes manifest across different stages of malignancy will be crucial in developing effective diagnostic and treatment strategies. Identifying and characterizing the specific proteins involved in the spectral changes associated with amide III absorption will be instrumental in advancing our understanding of the molecular events driving oral malignancies. Moreover, unraveling the complex interplay between these proteins and the surrounding microenvironment will provide valuable insights into the biomolecular processes occurring during the progression of oral disorders toward malignancy. These changes in the secondary structure can modulate post-translational modifications such as phosphorylation of proteins.59 Thus, secondary protein structures can be better understood by combining the amide III and amide I regions.
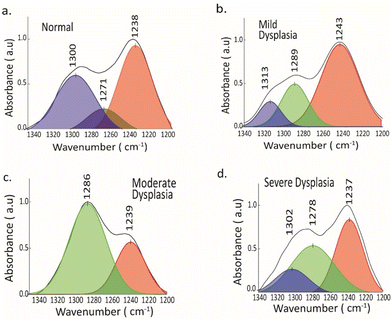 |
| Fig. 4 Deconvoluted FTIR spectra in the amide III band of the basal layer/region in (a) normal tissue, (b) mild dysplasia, (c) moderate dysplasia and (d) severe dysplasia. 1300 ± 5 (α-helix), 1288 ± 2 (β-turn), 1240 ± 2 (β-sheet) and random coil (1270 ± 5). | |
Infrared chemical mapping
The collection of an infrared spectral image requires the use of unstained tissue slices. This type of image is one in which each pixel of tissue is not merely represented as a color value but rather by a full IR spectrum. An analysis of the spectral image allows for the creation of a pseudo-color rendering of the tissue segment, focusing on its biochemical makeup rather than its shape, tissue architecture, or staining patterns. In infrared-based imaging, spectral hypercubes are used to collect spectra.15,60,61
A spectral hypercube encompasses all the relevant data regarding the tissue composition and its spatial fluctuations.15 Four sections were chosen as per the pathologist's suggestions, with the best representative cases of NOM, MD, MOD, and SD for FTIR imaging. False colour maps were obtained, with each pixel representing a single spectrum. After atmospheric corrections and noise reduction, the dataset was pre-processed (baseline correction, vector normalization) to obtain the chemical maps. After atmospheric corrections and noise reduction, the dataset was pre-processed (baseline correction, vector normalization) to obtain the chemical maps. Fig. 5 and 6 show a 50 μm × 50 μm section of an unstained oral epithelial tissue along with its corresponding chemical map. The architectural division of the tissue section involves mucosa, sub-mucosa, and connective tissue. The chemical map is obtained by plotting the total absorption intensity within the amide I (1600–1700 cm−1) and amide III (1400–1200 cm−1) region, which is indicative of peptide bonds in proteins. The spatial distribution of peptide bonds within the tissue is obtained by integrating the total absorbance as a function of pixels in the image. This provides visualization of the distribution of proteins within the epithelium tissue, allowing valuable insights into the tissue structure.
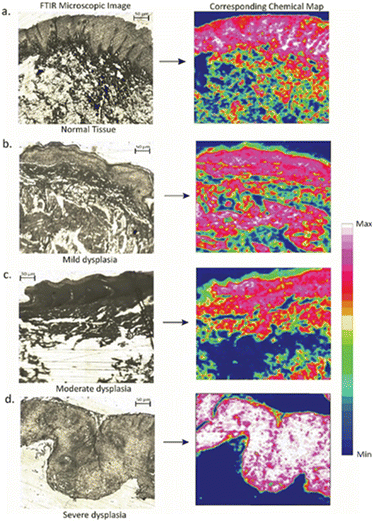 |
| Fig. 5 Photographs of FTIR images with their corresponding chemical maps in the amide I absorption band (1700–1600 cm−1) for (a) nornal tissue (b) mild dysplasia (c) moderate dysplasia and (d) severe dysplasia. | |
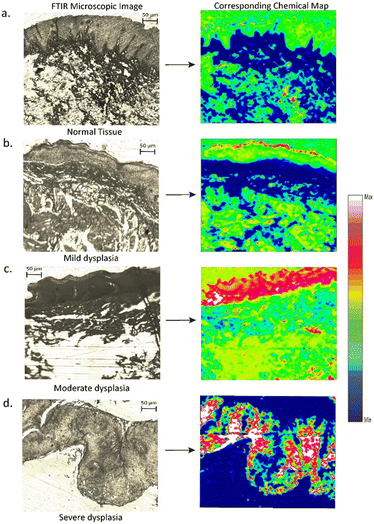 |
| Fig. 6 Photographs of FTIR images with their corresponding chemical maps in the amide III absorption band (1340–1240 cm−1) for (a) normal tissue (b) mild dysplasia (c) moderate dysplasia and (d) severe dysplasia. | |
The obtained result demonstrates a strong correlation with the tissue morphology, showing a significant increase in the amide I band within the epithelium of severe dysplasia when compared to normal epithelium. Here, white color represents the maximum intensity and blue the minimum. The chemical maps consist of false color images, where each pixel corresponds to a single spectrum. The absorption band at the amide III band (1340–1200 cm−1) region revealed higher amide III content in the epithelial layer of severe dysplasia, whereas in the normal and mild dysplasia images, a lesser distribution of amide III peptide bonds was observed along its epithelial layer. These FTIR images can be very helpful in the diagnosis systems for a pathologist with shorter acquisition times and higher spectral quality.
Multivariate analysis of FTIR spectra
The dataset, which consists of 105 samples, was distributed into four classes (normal = 24, mild = 22, moderate = 29, and severe = 30). Then the FTIR spectra of each sample were acquired and after pre-processing the mean spectra of each group it was subjected to feature extraction using principal component analysis (PCA). The use of PCA data improves computational processing since it employs a reduced dataset. An exploratory analysis was performed by using the FTIR spectra in two specific regions: (i) 1700–1600 cm−1 corresponding to amide I and (iii) 1400–1200 cm−1 corresponding to the amide III region. From each of the two specific regions, fifteen new sets of uncorrelated variables designated as principal components (PCs) covering 95% of the data variance were obtained. These PCs were then subjected to a PCA–LDA classifier for classification. LDA is a supervised learning algorithm that aims to maximize the difference between classes within a dataset. A confusion matrix from the PCA–LDA classifier was generated, and accuracy was calculated for the two regions. Examining the PCA–LDA score plots allowed for identifying the classification in the spectra for different groups (Fig. 7). We then tested the trained PCA–LDA model using an external test set, which was not included in the model development, and obtained the confusion matrix in both the amide I and the amide III regions (see Fig. S3†). A graphical view of the workflow is shown in Fig. S4.†
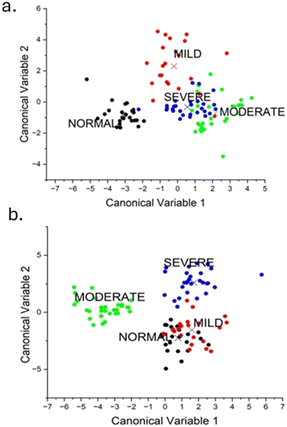 |
| Fig. 7 (a) PCA–LDA score plot in the amide I region. (b) PCA–-LDA score plot in the amide III region. | |
Amide I region (1700–1600 cm−1)
The PCA and loading plot in Fig. S5(a and c)† show that the peaks at 1667, 1632, and 1673 cm−1 were significant in distinguishing and categorizing the four different samples. The initial 15 principal components, which account for 95% of the overall variation in the dataset, were subsequently utilized as input data for PCA–LDA. 24 out of 24 normal samples, 19 out of 22 mild dysplasia, 28 out of 29 moderate dysplasia, and 26 out of 30 severe dysplasia samples were classified correctly. The algorithm underwent testing using a leave-one-out cross-validation technique. The classification rates were 100% for the normal samples, 77.2% for mild dysplasia, 96.5% for moderate dysplasia, and 87.5% for severe dysplasia (see Table S2†). The accuracy achieved was 92%.
Amide III region (1340–1240 cm−1)
The first 15 main components, which explain 95% of the total variation in the dataset, were then used as input data for PCA–LDA using the amide III region. The PCA score in the amide III region with PC1 explaining 92.7% variation is given in Fig. S3(b).† The samples were distinguished using peaks at 1239, 1335, and 1373 cm−1 using the loading plot given in Fig. S3(d).† All 23 out of 24 normal samples, 21 out of 22 mild dysplasia samples, all 29 moderate dysplasia samples, and all 30 severe dysplasia samples were accurately diagnosed. The algorithm was tested using a leave-one-out cross-validation technique. The accuracy rates were 100% for the normal samples, 86.3% for mild dysplasia, 96.5% for moderate dysplasia, and 100% for severe dysplasia (Table 3). The accuracy achieved was 95.5%. The overall confusion matrix for both amide I and amide III regions are given in Fig. S5.†
Table 3 PCA–LDA classification of the FTIR spectra in the 1400–1200 cm−1 region
|
|
Predictive model |
Normal |
Mild |
Moderate |
Severe |
LDA |
Normal |
23 samples |
1 sample |
0 |
0 |
Mild |
1 sample |
21 samples |
0 |
0 |
Moderate |
0 |
0 |
29 samples |
0 |
Severe |
0 |
0 |
0 |
30 samples |
Cross-validation |
Normal |
24 samples |
0 |
0 |
0 |
Mild |
2 samples |
19 samples |
1 sample |
0 |
Moderate |
1 sample |
0 |
28 samples |
0 |
Severe |
0 |
0 |
0 |
15 samples |
Conclusion
To the best of our understanding, this is one of the very first instances of investigation of biochemical changes in the epithelium and sub-epithelium layers during the precancerous stages of oral cancer using FTIR micro-spectroscopy. Our study reveals significant shifts in the amide I and III regions, indicating alterations in proteins' secondary structure. An extensive peak fitting procedure has been utilized to extract the quantitative data regarding the secondary structure of proteins, including α-helix, β-turn, β-sheet, and random coil. This is achieved by analyzing the infrared spectra of the amide I and III regions. Our results suggested that the amide III region provides better information on the four secondary structures than the amide I region. Further, the PCA–LDA classification model revealed an accuracy of 95.5% in the amide III region, which was better than that of amide I. Moreover, through infrared chemical imaging, the distribution of biochemical composition over the tissue architecture of interested regions was shown, which can aid modern medical diagnostics. Thus, by FTIR imaging on oral tissue samples, pathologists can obtain valuable information without damaging the samples and can be widely used to characterize tissue samples using high-quality imaging data. As the field of spectroscopic analysis continues to evolve, its potential for revolutionizing the landscape of oral health care becomes increasingly evident. The insights gained from amide I and amide III spectroscopic analysis, particularly concerning identifying specific protein alterations associated with malignant transformation, serve as a beacon of hope for developing targeted therapeutic approaches aimed at restoring normal protein conformation and composition. Ultimately, the convergence of advanced spectroscopic techniques and a deepened understanding of the molecular intricacies underlying oral disorders hold the key to enhanced patient outcomes and a new era in the management of oral health. Unravelling the intricate relationship between these proteins and their interactions within the oral microenvironment will shed light on the underlying molecular mechanisms driving malignant transformation in oral tissues. This knowledge will not only advance our understanding of oral malignancies but also pave the way for the development of targeted therapeutic interventions aimed at restoring normal protein conformation and composition, ultimately offering improved prospects for patient outcomes. In the pursuit of optimizing spectroscopic techniques for clinical applications in oral health, it is imperative to explore how these spectral changes manifest across different stages of malignancy. By identifying and characterizing the specific proteins involved in the spectral changes associated with both amide I and amide III absorption, researchers can lay the groundwork for developing more precise diagnostic and treatment strategies. This depth of knowledge regarding the molecular events driving oral malignancies holds great promise for advancing not only our understanding but also the management of these complex conditions. Recent advancements in spectroscopic techniques have significantly contributed to the development of non-invasive, effortless, faster, non-erroneous methods for analysing oral tissues, excluding interobserver variability.62 These cutting-edge approaches provide a promising avenue for early detection and ongoing monitoring of oral malignancies. As such, these non-invasive methods offer potential benefits regarding patient comfort and compliance, making them extremely valuable in clinical settings. Advancements in technology have allowed for the development of more sophisticated spectroscopic tools that can capture and analyze amide I and amide III features with higher accuracy and sensitivity. These tools will enable researchers and clinicians to gain a deeper understanding of the molecular changes occurring in oral tissues during malignant transformation. Moreover, the non-invasive nature of these spectroscopic methods makes them particularly well suited for long-term studies, where the progression of oral disorders and the efficacy of treatment interventions can be monitored over time without the need for invasive procedures, creating avenues for detecting prognostic references in OPMDs. Amide III spectroscopic features may have the potential to diagnose lymph node metastasis in oral cancer. Identifying specific spectral markers within the amide III band has proven to be closely associated with metastatic cells in lymph nodes, offering a non-invasive means for evaluating the extent of oral cancer's spread. Furthermore, as technological advancements evolve, refining non-invasive spectroscopic tools for analyzing oral tissues will play a crucial role in ensuring patient comfort and compliance during diagnostic and monitoring procedures. The ongoing research and exploration of amide III spectroscopic analysis present valuable opportunities for enhancing our understanding of oral disorders and improving clinical management. By delving deeper into the practical applications, technological advancements, and therapeutic implications of this approach, the field of oral health stands to benefit from more refined diagnostic and treatment strategies that are tailored to the unique characteristics of each patient's oral disorder. By harnessing the potential of these cutting-edge spectroscopic tools, clinicians and researchers can pave the way for improved early detection, treatment monitoring, and more personalized therapeutic strategies in oral malignancies. Henceforth, incorporating a predictive infrared spectroscopy model with clinical practice can serve as a valuable screening or diagnostic tool for oral cancer.
Ethical statement
All tissue samples were provided by the Guru Nanak Institute of Dental Sciences and Research (GNIDSR) with approval from their ethical committee.
Data availability
The data supporting this article have been included as part of the ESI.†
Author contributions
P. J. T., P. L., B. L.: experimentation, data analysis, manuscript preparation. K. B.: sample preparation, selection of the region of interest. S. B., S. K. D., M. P., R. R. P. & M. P. M.: tissue biopsy, tissue staining, histopathological analysis & manuscript preparation. S. M.: biochemical analysis. S. B.: machine learning analysis. P. L.: conceptualization, investigation, visualization, analysis, reviewing results, project management, funding acquisition. B. L.: overall supervision, conceptualization, data analysis, reviewing result, and manuscript preparation.
Conflicts of interest
There are no conflicts to declare.
Acknowledgements
This work has been funded by the Science and Engineering Research Board (SERB) of the Govt. of India Core Research Grant under project no. CRG/2020/002687.
References
- K. R. Coelho, J. Cancer Epidemiol., 2012, 2012, 701932 CrossRef PubMed.
- P. Balaram, H. Sridhar, T. Rajkumar, S. Vaccarella, R. Herrero, A. Nandakumar, K. Ravichandran, K. Ramdas, R. Sankaranarayanan, V. Gajalakshmi, N. Muñoz and S. Franceschi, Int. J. Cancer, 2002, 98, 440–445 CrossRef PubMed.
- B. J. Jacob, K. Straif, G. Thomas, K. Ramadas, B. Mathew, Z. F. Zhang, R. Sankaranarayanan and M. Hashibe, Oral Oncol., 2004, 40, 697–704 CrossRef PubMed.
- C. Laprise, H. P. Shahul, S. A. Madathil, A. S. Thekkepurakkal, G. Castonguay, I. Varghese, S. Shiraz, P. Allison, N. F. Schlecht, M.-C. Rousseau, E. L. Franco and B. Nicolau, Int. J. Cancer, 2016, 139, 1512–1519 CrossRef.
- S. L. Wetzel and J. Wollenberg, Dent. Clin. North Am., 2020, 64, 25–37 CrossRef PubMed.
- P. M. Speight, T. J. Abram, P. N. Floriano, R. James, J. Vick, M. H. Thornhill, C. Murdoch, C. Freeman, A. M. Hegarty, K. D'Apice, A. R. Kerr, J. Phelan, P. Corby, I. Khouly, N. Vigneswaran, J. Bouquot, N. M. Demian, Y. E. Weinstock, S. W. Redding, S. Rowan, C. K. Yeh, H. S. McGuff, F. R. Miller and J. T. McDevitt, Oral Surg. Oral Med. Oral Pathol. Oral Radiol., 2015, 120, 474–482.e472 CrossRef PubMed.
- V. Borse, A. N. Konwar and P. Buragohain, Sens. Int., 2020, 1, 100046 CrossRef PubMed.
- D. Sylvie-Louise Avon and H. Klieb, J. Can. Dent. Assoc., 2012, 78, c75 Search PubMed.
- J.-J. Lee, H.-C. Hung, S.-J. Cheng, C.-P. Chiang, B.-Y. Liu, C.-H. Yu, J.-H. Jeng, H.-H. Chang and S.-H. Kok, Oral Surg. Oral Med. Oral Pathol. Oral Radiol. Endod., 2007, 104, 217–225 CrossRef PubMed.
- S. M. Ahmed and V. Jigna, Ann. Diagn. Pathol., 2009, 13, 140–145 CrossRef PubMed.
- G. Bellisola and C. Sorio, Am. J. Cancer Res., 2012, 2, 1 CAS.
- D. C. Fernandez, R. Bhargava, S. M. Hewitt and I. W. Levin, Nat. Biotechnol., 2005, 23, 469–474 CrossRef CAS PubMed.
- A. Diajil, C. Robinson, P. Sloan and P. Thomson, Int. J. Dent., 2013, 2013, 809248 CAS.
- S. Rehman, Z. Movasaghi, J. A. Darr and I. U. Rehman, Appl. Spectrosc. Rev., 2010, 45, 355–368 CrossRef CAS.
- J. Pallua, C. Pezzei, B. Zelger, G. Schaefer, L. Bittner, V. Huck-Pezzei, S. Schoenbichler, H. Hahn, A. Kloss-Brandstaetter and F. Kloss, Analyst, 2012, 137, 3965–3974 RSC.
- S. Banerjee, M. Pal, J. Chakrabarty, C. Petibois, R. R. Paul, A. Giri and J. Chatterjee, Anal. Bioanal. Chem., 2015, 407, 7935–7943 CrossRef CAS.
- A. Zlotogorski-Hurvitz, B. Z. Dekel, D. Malonek, R. Yahalom and M. Vered, J. Cancer Res. Clin. Oncol., 2019, 145, 685–694 CrossRef CAS.
- C. L. Song, M. Z. Vardaki, R. D. Goldin and S. G. Kazarian, Anal. Bioanal. Chem., 2019, 411, 6969–6981 CrossRef CAS PubMed.
- E. Gazi, J. Dwyer, P. Gardner, A. Ghanbari-Siahkali, A. Wade, J. Miyan, N. P. Lockyer, J. C. Vickerman, N. W. Clarke and J. H. Shanks, J. Pathol., 2003, 201, 99–108 CrossRef CAS PubMed.
- C. Pezzei, A. Brunner, G. Bonn and C. Huck, Analyst, 2013, 138, 5719–5725 RSC.
- V. E. Sitnikova, M. A. Kotkova, T. N. Nosenko, T. N. Kotkova, D. M. Martynova and M. V. Uspenskaya, Talanta, 2020, 214, 120857 CrossRef CAS.
- L. Dong, X. Sun, Z. Chao, S. Zhang, J. Zheng, R. Gurung, J. Du, J. Shi, Y. Xu and Y. Zhang, Spectrochim. Acta, Part A, 2014, 122, 288–294 CrossRef CAS.
- P. Lasch, W. Haensch, D. Naumann and M. Diem, Biochim. Biophys. Acta, Mol. Basis Dis., 2004, 1688, 176–186 CrossRef CAS.
- T. D. Wang, G. Triadafilopoulos, J. M. Crawford, L. R. Dixon, T. Bhandari, P. Sahbaie, S. Friedland, R. Soetikno and C. H. Contag, Proc. Natl. Acad. Sci. U. S. A., 2007, 104, 15864–15869 CrossRef CAS PubMed.
- Q.-B. Li, X.-J. Sun, Y.-Z. Xu, L.-M. Yang, Y.-F. Zhang, S.-F. Weng, J.-S. Shi and J.-G. Wu, Clin. Chem., 2005, 51, 346–350 CrossRef CAS.
- M. Ghassemi, S. Barzegari, P. Hajian, H. Zham, H. R. Mirzaei and F. H. Shirazi, J. Mol. Struct., 2021, 1229, 129493 CrossRef.
- K. Yano, S. Ohoshima, Y. Gotou, K. Kumaido, T. Moriguchi and H. Katayama, Anal. Biochem., 2000, 287, 218–225 CrossRef CAS PubMed.
- C. Pezzei, J. D. Pallua, G. Schaefer, C. Seifarth, V. Huck-Pezzei, L. K. Bittner, H. Klocker, G. Bartsch, G. K. Bonn and C. W. Huck, Mol. BioSyst., 2010, 6, 2287–2295 RSC.
- H. J. Butler, P. M. Brennan, J. M. Cameron, D. Finlayson, M. G. Hegarty, M. D. Jenkinson, D. S. Palmer, B. R. Smith and M. J. Baker, Nat. Commun., 2019, 10, 4501 CrossRef PubMed.
- K. Gajjar, L. D. Heppenstall, W. Pang, K. M. Ashton, J. Trevisan, I. I. Patel, V. Llabjani, H. F. Stringfellow, P. L. Martin-Hirsch and T. Dawson, Anal. Methods, 2013, 5, 89–102 RSC.
- S. M. Ali, F. Bonnier, H. Lambkin, K. Flynn, V. McDonagh, C. Healy, T. Lee, F. Lyng and H. Byrne, Anal. Methods, 2013, 5, 2281–2291 RSC.
- K. Belbachir, R. Noreen, G. Gouspillou and C. Petibois, Anal. Bioanal. Chem., 2009, 395, 829–837 CrossRef CAS PubMed.
- M. J. Baker, J. Trevisan, P. Bassan, R. Bhargava, H. J. Butler, K. M. Dorling, P. R. Fielden, S. W. Fogarty, N. J. Fullwood and K. A. Heys, Nat. Protoc., 2014, 9, 1771–1791 CrossRef CAS PubMed.
- I. I. Patel, J. Trevisan, G. Evans, V. Llabjani, P. L. Martin-Hirsch, H. F. Stringfellow and F. L. Martin, Analyst, 2011, 136, 4950–4959 RSC.
- B. W. Neville and T. A. Day, Ca-Cancer J. Clin., 2002, 52, 195–215 CrossRef PubMed.
- P. Bruni, C. Conti, E. Giorgini, M. Pisani, C. Rubini and G. Tosi, Faraday Discuss., 2004, 126, 19–26 RSC.
- S. Sabbatini, C. Conti, C. Rubini, V. Librando, G. Tosi and E. Giorgini, Vib. Spectrosc., 2013, 68, 196–203 CrossRef.
- B. G. Ellis, C. A. Whitley, S. Al Jedani, C. I. Smith, P. J. Gunning, P. Harrison, P. Unsworth, P. Gardner, R. J. Shaw and S. D. Barrett, Analyst, 2021, 146, 4895–4904 RSC.
- A. Sala, D. J. Anderson, P. M. Brennan, H. J. Butler, J. M. Cameron, M. D. Jenkinson, C. Rinaldi, A. G. Theakstone and M. J. Baker, Cancer Lett., 2020, 477, 122–130 CrossRef PubMed.
- S. Muller and W. M. Tilakaratne, Head Neck Pathol., 2022, 16, 54–62 CrossRef PubMed.
-
S. P. Leong, S. D. Nathanson and J. S. Zager, Cancer metastasis through the lymphovascular system, Springer, 2022 Search PubMed.
- P. Lahiri, S. Mukherjee, B. Ghosh, D. Das, B. Lahiri, S. K. Varshney, M. Pal, R. R. Paul and J. Chatterjee, Biomolecules, 2021, 11, 889 CrossRef CAS PubMed.
- S. Cai and B. R. Singh, Biochemistry, 2004, 43, 2541–2549 CrossRef CAS PubMed.
-
B. R. Singh, Basic Aspects of the Technique and Applications of Infrared Spectroscopy of Peptides and Proteins, Infrared Analysis of Peptides and Proteins, ACS Publications, 2000, vol. 750, DOI:10.1021/bk-2000-0750.ch001.
- A. Ghosh, S. Raha, S. Dey, K. Chatterjee, A. R. Chowdhury and A. Barui, Analyst, 2019, 144, 1309–1325 RSC.
- B. G. Zimmermann and D. T. Wong, Oral Oncol., 2008, 44, 425–429 CrossRef CAS PubMed.
- R. Mishra, S. Nagini and A. Rana, Mol. Cancer, 2015, 14, 1–16 CrossRef CAS PubMed.
- J. Yang, X. Shi, M. Yang, J. Luo, Q. Gao, X. Wang, Y. Wu, Y. Tian, F. Wu and H. Zhou, Int. J. Oral Sci., 2021, 13, 12 CrossRef CAS PubMed.
- C. Marbaniang and L. Kma, Asian Pac. J. Cancer Prev., 2018, 19, 2377 CAS.
- H. Aizawa, S.-I. Yamada, T. Xiao, T. Shimane, K. Hayashi, F. Qi, H. Tanaka and H. Kurita, Arch. Oral Biol., 2017, 83, 340–347 CrossRef CAS PubMed.
- A. Anura, A. Kazi, M. Pal, R. R. Paul, S. Sengupta and J. Chatterjee, Histochem. Cell Biol., 2018, 150, 61–75 CrossRef CAS.
- J. Kong and S. Yu, Acta Biochim. Biophys. Sin., 2007, 39, 549–559 CrossRef CAS.
- Z. Movasaghi, S. Rehman and D. I. ur Rehman, Appl. Spectrosc. Rev., 2008, 43, 134–179 CrossRef CAS.
- A. Sadat and I. J. Joye, Appl. Sci., 2020, 10, 5918 CrossRef CAS.
- S. Krimm and J. Bandekar, Adv. Protein Chem., 1986, 38, 181–364 CrossRef CAS PubMed.
- P. Mandal, N. Eremina and A. Barth, J. Phys. Chem. B, 2012, 116, 12389–12397 CrossRef CAS PubMed.
- S. Khan and M. Vihinen, BMC Struct. Biol., 2007, 7, 1–18 CrossRef.
- C. Krafft, B. Dietzek and J. Popp, Analyst, 2009, 134, 1046–1057 RSC.
- J. A. Kimber, L. Foreman, B. Turner, P. Rich and S. G. Kazarian, Faraday Discuss., 2016, 187, 69–85 RSC.
- D. Perez-Guaita, P. Heraud, K. M. Marzec, M. De La Guardia, M. Kiupel and B. R. Wood, Analyst, 2015, 140, 2376–2382 RSC.
- M. Diem, A. Mazur, K. Lenau, J. Schubert, B. Bird, M. Miljković, C. Krafft and J. Popp, J. Biophotonics, 2013, 6, 855–886 CrossRef CAS PubMed.
- L. Dong, X. Duan, L. Bin, J. Wang, Q. Gao, X. Sun and Y. Xu, Spectrochim. Acta, Part A, 2023, 289, 122209 CrossRef CAS PubMed.
|
This journal is © The Royal Society of Chemistry 2024 |
Click here to see how this site uses Cookies. View our privacy policy here.